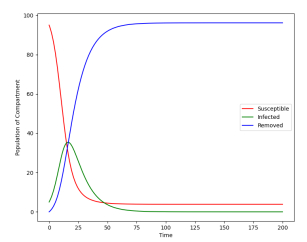
Traditional compartmental models describe the evolution of a virus over a population of nodes; one common model separates the population into compartments of susceptible, infected, and removed nodes. More complex bi-virus models describe this evolution for two competing viruses in the same population, having an additional parameter known as the virus interaction factor, which defines the effect one virus has on the rate of infection of another. Although these models are generally used in the context of infectious diseases, they could also describe the spread of ideas, or competing products in a market of consumers. In this paper, a new model was proposed that added separate interaction factors for each virus, differentiating the effects of viruses on one another. Adding this additional parameter will allow for more accurate interactions to be modeled and analyzed. A focus was placed on the limiting behavior of this model, an eventual end-state equilibrium where the number of nodes in each compartment remains constant. Relationships between the virus interaction factors and the strengths of the viruses on the limiting behavior were identified. Finally, a complete numerical solution to the model and a condition for real-valued limiting behaviors was calculated and tested against the simulation data.
Citation: Benjamin Zhang. Limiting behaviors of a bi-virus model with two interaction factors[J]. Mathematical Modelling and Control, 2024, 4(1): 64-70. doi: 10.3934/mmc.2024006
[1] | Yaxin Ren, Yakui Xue . Modeling and optimal control of COVID-19 and malaria co-infection based on vaccination. Mathematical Modelling and Control, 2024, 4(3): 316-335. doi: 10.3934/mmc.2024026 |
[2] | J. O. Akanni, S. Ajao, S. F. Abimbade, Fatmawati . Dynamical analysis of COVID-19 and tuberculosis co-infection using mathematical modelling approach. Mathematical Modelling and Control, 2024, 4(2): 208-229. doi: 10.3934/mmc.2024018 |
[3] | Abdul-Fatawu O. Ayembillah, Baba Seidu, C. S. Bornaa . Mathematical modeling of the dynamics of maize streak virus disease (MSVD). Mathematical Modelling and Control, 2022, 2(4): 153-164. doi: 10.3934/mmc.2022016 |
[4] | Salma Al-Tuwairqi, Asma Badrah . A qualitative analysis of a model on alpha-synuclein transport and aggregation in neurons. Mathematical Modelling and Control, 2023, 3(2): 104-115. doi: 10.3934/mmc.2023010 |
[5] | Oluwatayo Michael Ogunmiloro . A fractional order mathematical model of teenage pregnancy problems and rehabilitation in Nigeria. Mathematical Modelling and Control, 2022, 2(4): 139-152. doi: 10.3934/mmc.2022015 |
[6] | Rashid Jan, Normy Norfiza Abdul Razak, Sania Qureshi, Imtiaz Ahmad, Salma Bahramand . Modeling Rift Valley fever transmission: insights from fractal-fractional dynamics with the Caputo derivative. Mathematical Modelling and Control, 2024, 4(2): 163-177. doi: 10.3934/mmc.2024015 |
[7] | Elijah B. Baloba, Baba Seidu . A mathematical model of anthrax epidemic with behavioural change. Mathematical Modelling and Control, 2022, 2(4): 243-256. doi: 10.3934/mmc.2022023 |
[8] | Mohamed COULIBALY, Modeste N'Zi . A stochastic model with jumps for smoking incorporating media coverage. Mathematical Modelling and Control, 2022, 2(3): 122-130. doi: 10.3934/mmc.2022013 |
[9] | Yongming Li, Shou Ma, Kunting Yu, Xingli Guo . Vehicle kinematic and dynamic modeling for three-axles heavy duty vehicle. Mathematical Modelling and Control, 2022, 2(4): 176-184. doi: 10.3934/mmc.2022018 |
[10] | Anita T. Kurniawati, Fatmawati, Chidozie W. Chukwu, Windarto, Faishal F. Herdicho . Optimal control of dengue fever model with a logistically growing human population. Mathematical Modelling and Control, 2025, 5(1): 48-60. doi: 10.3934/mmc.2025004 |
Traditional compartmental models describe the evolution of a virus over a population of nodes; one common model separates the population into compartments of susceptible, infected, and removed nodes. More complex bi-virus models describe this evolution for two competing viruses in the same population, having an additional parameter known as the virus interaction factor, which defines the effect one virus has on the rate of infection of another. Although these models are generally used in the context of infectious diseases, they could also describe the spread of ideas, or competing products in a market of consumers. In this paper, a new model was proposed that added separate interaction factors for each virus, differentiating the effects of viruses on one another. Adding this additional parameter will allow for more accurate interactions to be modeled and analyzed. A focus was placed on the limiting behavior of this model, an eventual end-state equilibrium where the number of nodes in each compartment remains constant. Relationships between the virus interaction factors and the strengths of the viruses on the limiting behavior were identified. Finally, a complete numerical solution to the model and a condition for real-valued limiting behaviors was calculated and tested against the simulation data.
The spread of viruses over a population network is an extremely important topic of study in today's society, especially after the events of the COVID-19 pandemic. In the field of epidemiology, compartmental models are commonly used to simulate the progression of a virus over a population space. There are many commonly used single-virus models, such as the SIR model: Consisting of susceptible, infected, and removed compartments. While modeling, nodes in populations travel between these compartments from one to another: Susceptible nodes are infected at a certain attack rate β, which are then removed at a certain healing rate δ. These three compartments can be modeled together using a system of ordinary differential equations (ODEs), which represent the change in the number of nodes in each compartment with respect to time. When analyzing the behavior of these compartmental models, after initial fluctuations, there is a limiting behavior of constant end-state values (a steady state) for each compartment [1]. This limiting behavior is demonstrated in the SIR model in Figure 1.
However, SIR and other similar models fail to consider competition within the population. A type of model that adds another layer of complexity are bi-virus models, which model the progression of two viruses over the same population. One previously proposed bi-virus model is based off of the susceptible-infected-susceptible (SIS) single-virus model [1, 2], where infected nodes have no immunity and are susceptible to the virus afterwards (there is no recovered compartment). A new compartment I12 is created to represent nodes infected with both viruses[3]. In order to model competition between the viruses, the virus interaction factor ε is incorporated, which alters the attack rate of one virus on a node that has already been infected with the other virus. This model is named the susceptible-infected 1 or 2-susceptible (SI1|2S) model. An important discovery with this model is a critical interaction factor value εcritical, that represents an equilibrium point of coexistence between the two viruses [3, Theorem 1]:
εcritical={σ1−σ2σ2(σ1−1),if σ1+σ2≥2,2(1+√1−σ1σ2)σ1σ2,if σ1+σ2<2, |
where σ1≥σ2, and σ represents the strength of a virus: the size of the population multiplied by its rate of infection (attack rate), divided by its healing rate (see Table 1).
Variable | Definition |
N | Size of population =S+I1+I2+I12 |
β1,β2 | Attack rates of viruses 1 and 2 |
δ1,δ2 | Healing rates of viruses 1 and 2 |
σ1,σ2 | Strength of viruses 1 and 2 =Nβδ |
ε1,ε2 | Interaction factors of viruses 1 and 2 |
I1,I2,I12 | \# of nodes infected with either or both viruses |
S | Number of susceptible nodes |
I am motivated by the reality where competing viruses have interactions that are individualized in their impact on the opposing virus's attack rate; as opposed to the SI1|2S model previously cited where the viruses exhibit the same effect on each other. Therefore, a new model is proposed that incorporates two virus interaction factor values, one for each virus. For example, this will differentiate the effect of having COVID-19 on catching the flu and the effect of having flu on catching COVID-19. This would allow for the ability to model more realistic interactions, expanding outside the scope of epidemiology in applications such as products in a market or political opinions over a population [4]. This model is then analyzed to determine different limiting behaviors based on initial parameters.
The proposed model with two interaction factors is represented by the following system of ODEs:
dI1dt=β1S(I1+I12)+δ2I12−δ1I1−ε2β2I1(I2+I12), | (2.1) |
dI2dt=β2S(I2+I12)+δ1I12−δ2I2−ε1β1I2(I1+I12), | (2.2) |
dI12dt=ε1β1I2(I1+I12)+ε2β2I1(I2+I12)−(δ1+δ2)I12. | (2.3) |
In this model, the size of the population N stays constant, which implies
dSdt=−dI1dt−dI2dt−dI12dt. | (2.4) |
As illustrated in Figure 2, susceptible nodes move into infected compartments at the attack rate β, and move back to the susceptible compartment at the healing rate δ.
Nodes infected with one virus also move into the I12 compartment at a rate defined by the interaction factor ε multiplied by the original attack rate of the other virus. Nodes infected with both viruses also move back into single-virus compartments with their respective healing rate.
It is important to consider the positivity and boundedness [5] of the proposed model in order for it to have a physically relevant meaning, i.e., ensuring all values will stay positive and bounded within the population. The work is presented under the following assumptions, where i1=I1N, i2=I2N, i12=I12N:
(1) i1,i2,i12,(1−i1−i2−i12)∈[0,1]
The initial fraction of infected and susceptible nodes are between 0 and 1.
(2) β1,β2,δ1,δ2≥0
All attack rates and healing rates are nonnegative.
Consider a time t where i1=0. Under Assumption 2, Eq (2.1) now implies that dI1dt≥0. The same applies to i2 and i12. If i1=1 at time t, then under Assumption 2 and Eq (2.1), dI1dt≤0. The same applies to i2 and i12.
Under Assumption 1 and the above arguments, we can conclude i1,i2,i12,i1+i2+i12∈[0,1] for all t≥0 and that the set
D={(i1,i2,i12)∣i1≥0,i2≥0,i12≥0,i1+i2+i12≤1} | (2.5) |
is positively invariant in the context of the system of ODEs. The analysis of the model will be carried out with these assumptions and therefore exclusively within domain D.
The stability of the model during a steady state must be confirmed before analyzing its limiting behaviors. In a steady state
dI1dt,dI2dt,dI12dt=0, | (2.6) |
which implies
dSdt=0. | (2.7) |
Consider the derivative of Eq (2.1) with respect to time:
d2I1dt2=β1S(dI1dt+dI12dt)+β1dSdt(I1+I12)+δ2dI12dt−δ1dI1dt−ε2β2dI1dt(I1+I12)−ε2β2I1(dI2dt+dI12dt). | (2.8) |
Given Eqs (2.6) and (2.7), d2I1dt2=0. As time elapses, the derivatives of the compartments are not effected, demonstrating stability across the dimension of time. The same applies to the other compartments in the model.
Let
I′1(t)≡dI1dt. | (2.9) |
Consider the partial derivative of (2.9) with respect to I1
∂I′1(t)∂I1=β1S+β1∂S∂I1(I1+I12)−δ1−ε2β2(I2+I12). | (2.10) |
Substituting from Eq (2.6) and ∂S∂I1=−1
∂I′1(t)∂I1=β1S−β1(I1+I12)−δ1−β1S(I1+I12)+δ2I12−δ1I1I1=−β1(I1+I12)−β1SI12+δ2I12I1. | (2.11) |
Under Assumptions 1 and 2, ∂I′1(t)∂I1≤0. Any fluctuations in I1 will result in
I′1(t)=dI1dt |
being a force that pulls I1 back to the stable state, demonstrating stability. The same applies to the other compartments in the model.
This model is simulated in silico by integrating the system of ODEs along a unitless timescale (code can be found at: https://github.com/benz5460/bi-virus-model). The procedure is split into two parts with different goals:
(1) Observations of the effect of varying virus interaction factors ε1 and ε2 on the number of nodes infected with each virus at its end behavior.
(2) A numerical method to calculate the end behavior of the system using the initial parameters.
Throughout the simulations, the end behavior is measured at time t=5000. This was empirically determined to be a reasonable stopping point that was far into the end behavior of all tests. Initial compartment values of I1=50, I2=50, and I12=0 were used, although it is observed that initial values do not influence end state behavior.
When running simulations on this model, there are three possible end states: Both viruses survive, one virus survives while the other dies out, or both viruses die out. It is found that the strengths σ1 and σ2 have a large effect on the end behaviors of this model when varying the virus interaction factors ε1 and ε2. Two conditions based on strength values are investigated by graphing the effect of changes in virus interaction factor, on end state values of nodes infected with virus 1 (I1+I12) and virus 2 (I2+I12).
When the strengths of the two viruses have a sum greater than or equal to two, then
εcritical=σ1−σ2σ2(σ1−1) |
in the single interaction factor model. The stronger virus will be able to dominate the weaker until ε2 is high enough to ensure survival through collaboration. In Figures 3 and 4, example simulations are shown where the weaker virus will die out unless its virus interaction factor ε2 is greater than or equal to the aforementioned εcritical value. This indicates that within this condition, the model behaves in a similar way to if there were only one virus interaction factor.
Within this condition, either both viruses have strengths less than 1, or one virus has a strength greater than 1. In the first case, both viruses do not have enough strength to survive on their own, and collaboration is required for survival. This results in a graph that shows a relationship between interaction factors that act as a threshold between the flat region of extinction and raised region of survival (see Figure 5).
In the case where one virus has a strength greater than 1, a drastically different behavior is observed. The stronger virus will dominate until ε2 reaches a threshold where both viruses will jump to increased numbers of infected nodes. This threshold is observed to be near the εcritical values but not exact, through the observation of several simulations (see Figure 6).
An end state equilibrium is achieved when all three ODEs are equal to 0. With this, a concrete condition for real-valued, positive end state behavior is described, as well as a numerical method for calculating end state behavior given initial parameters
β1S(I1+I12)+δ2I12−δ1I1−ε2β2I1(I2+I12)=0, | (3.1) |
β2S(I2+I12)+δ1I12−δ2I1−ε1β1I2(I1+I12)=0, | (3.2) |
ε1β1I2(I1+I12)+ε2β2I1(I2+I12)−(δ1+δ2)I12=0. | (3.3) |
These equations are then manipulated into the following:
Nκ1β1[1−κ1−(1−ε1)i2]=δ1κ1, | (3.4) |
Nκ2β2[1−κ2−(1−ε2)i1]=δ2κ2, | (3.5) |
N(ε1β1κ1i2+ε2β2κ2i1)=(δ1+δ2)i12, | (3.6) |
where
κ1=I1+I12N, κ2=I2+I12N, i1=I1N, i2=I2N, i12=I12N |
Assuming positive, non-zero values of κ1 and κ2, and substituting
1σ=δNβ, i1=κ1−i12, i2=κ2−i12 |
into the three equations:
1−κ1−(1−ε1)i2=1/σ1, | (3.7) |
1−κ2−(1−ε2)i1=1/σ2, | (3.8) |
κ1κ2(ε1σ1δ1+ε2σ2δ2)=i12(δ1+δ2+ε1σ1δ1κ1+ε2σ2δ2κ2). | (3.9) |
Equation (3.10) is a rearrangement of Eq (3.9), while Eq (3.11) is the rearrangement of
Eq(3.7)×(1−ε2)−Eq(3.8)×(1−ε1). |
Equation (3.12) is an expanded form of Eq (3.7), substituting i2=κ2−i12
i12=κ1κ2(ε1σ1δ1+ε2σ2δ2)δ1+δ2+ε1σ1δ1κ1+ε2σ2δ2κ2, | (3.10) |
κ1=(1−ε1)ε2(1−ε2)ε1κ2+ε1−ε2−1−ε2σ1+1−ε1σ2ε1(1−ε2), | (3.11) |
1−κ1−(1−ε1)(κ2−i12)=1/σ1. | (3.12) |
Substituting Eqs (3.10) and (3.11) into Eq (3.12) results in a quadratic equation in the form of:
a(κ2)2+b(κ2)+c=0, | (3.13) |
where:
a=−δ1(ε2)2(σ1)2(σ2)2+δ1ε1(ε2)2(σ1)2(σ2)2 +δ2ε1(ε2−1)ε2σ1(σ2)3,b=−2δ1ε2(σ1)2σ2+2δ1ε1ε2(σ1)2σ2+δ2ε1(ε2−1)σ1(σ2)2−δ1ε1(ε2−1)2σ1(σ2)2−δ1(ε2−1)ε2σ1(σ2)2+δ2(ε2−1)ε2σ1(σ2)2+δ1ε1(ε2−1)ε2σ1(σ2)2−δ1ε1ε2(σ1)2(σ2)2+2δ1(ε2)2(σ1)2(σ2)2−δ1ε1(ε2)2(σ1)2(σ2)2−δ2ε1(ε2−1)ε2σ1(σ2)3,c=−δ1(σ1)2+δ1ε1(σ1)2−δ1(ε2−1)σ1σ2+δ2(ε2−1)σ1σ2+δ1ε1(ε2−1)σ1σ2−δ1ε1(σ1)2σ2+2δ1ε1(σ1)2σ2−δ1ε1ε2(σ1)2σ2+δ2(ε2−1)2(σ2)2−δ1ε1(ε2−1)σ1(σ2)2+δ1(ε2−1)ε2σ1(σ2)2−δ2(ε2−1)ε2σ1(σ2)2+δ1ε1ε2(σ1)2(σ2)2 −δ1(ε2)2(σ1)2(σ2)2. |
Equation (3.13) can be used in conjunction with Eq (3.11) to calculate the end state behavior of both viruses given initial parameters δ1,δ2,ε1,ε2,σ1,σ2. This agrees with the earlier observation that initial compartment values do not influence the end behavior.
For κ2 to be real-valued, the discriminant of the quadratic must be positive: b2−4ac≥0. In addition, only accepting roots where κ2∈[0,1] will further constrain the condition. This condition describes a numerical constraint for real-valued end state conditions based on initial parameters.
The original SI1|2S model exists as a unique case in the new model where ε=ε1=ε2. When this is true, the constraint can be reduced to the form:
σ1σ2ε2−4ε+4≥0. | (3.14) |
This agrees with the result found for the original model [3, Lemma 4], demonstrating the new model's validity.
Results from the numerical method are compared to those from the simulations. To achieve this, parameter values from selected conditions in Section 3.1 are plugged into Eq (3.13). Parameters from Figure 3 are first tested: β1=0.005,δ1=0.4,β2=0.0002,δ2=0.05,σ1=3.75,σ2=1.2. Plugging this into Eq (3.13),
[0.324ε1(ε2−1)−8.1ε22+8.1ε1ε22]κ22+[0.27ε1(ε2−1)−2.16ε1(ε2−1)2−13.5ε2+5.4ε1ε2−1.89(ε2−1)ε2+1.836ε1(ε2−1)ε2+16.2ε22−8.1ε1ε22]κ2−5.625−1.125ε1−1.575(ε2−1)−0.36ε1(ε2−1)+0.072(ε2−1)2+13.5ε2+1.35ε1ε2+1.89(−1+ε2)ε2−8.1ε22=0. | (3.15) |
Given ε1 and the condition κ2∈[0,1], the corresponding lower bound for ε2 is found from Eq (3.15) (e.g., let ε1=1, then ε2≥0.772727). This is repeated for a range of ε1 values and results are compared to the original simulation.
The same procedure is repeated with the parameters from Figure 5, with the results shown in Figure 7. As shown in Figures 7 and 8, the calculated threshold values for mutual survival of both viruses of ε2 match closely with simulation data for both observation conditions of virus strength values outlined in Section 3.1.
A new bi-virus model is proposed and analyzed using a system of ODEs, which introduce unique virus interaction factors for each virus. This allows the impact of one virus on another to be fine-tuned and model more realistic interactions. With the analysis, the following is concluded:
● End behaviors in this model are somewhat reliant on the original εcritical value. This is seen most clearly in the case where σ1+σ2≥2, while a less concrete dependence is seen when σ1+σ2<2.
● When σ1+σ2<2 and both are less than 1, the threshold of survival for the interaction factors are related in the form of a curve.
● A numerical solution of the model's end behavior is found in the form of a quadratic equation using initial parameters. The solution is tested against simulation data and found to be accurate.
● Similarly, a numerical constraint for real-valued solutions is found, of which the original model's constraint is uncovered as a unique case of the new model.
● Future work can be done to identify the exact relation between the interaction factors when σ1+σ2<2 and both are less than 1. Additionally, fitting the model to data of epidemics or sales of products can demonstrate the model's flexibility and applicability in the real world.
● Other interesting possibilities include models with greater than 2 viruses, with interactions determined through a virus interaction matrix, or one that incorporates the effect of interaction on healing rates.
The author declares he has not used Artificial Intelligence (AI) tools in the creation of this article.
The author would like to thank the CSIRE program at Stony Brook University, as well as Dr. Ji Liu for his support and advice throughout the program.
The author declares that he has no conflict of interest in this paper.
[1] | F. Brauer, Compartmental models in epidemiology, In: F. Brauer, P. Driessche, J. Wu, Mathematical epidemiology, 3 Eds., Berlin: Springer, 1945, 19–79. https://doi.org/10.1007/978-3-540-78911-6_2 |
[2] | E. Kuhl, The classical SIS model, In: Computational epidemiology, 3 Eds., Switzerland: Springer, Cham, 2021. https://doi.org/10.1007/978-3-030-82890-5_2 |
[3] | A. Beutel, A. Prakash, R. Rosenfeld, C. Faloutsos, Interacting viruses in networks: can both survive? KDD '12: Proceedings of the 18th ACM SIGKDD International Conference on Knowledge Discovery and Data Mining, 2012,426–434. https://doi.org/10.1145/2339530.2339601 |
[4] | K. Servick, How will COVID-19 affect the coming flu season? Scientists struggle for clues, Science, 2020. Available from: https://www.science.org/content/article/how-will-covid-19-affect-coming-flu-season-scientists-struggle-clues |
[5] |
J. Liu, P. E. Paré, A. Nedić, C. Y. Tang, C. L. Beck, Analysis and control of a continuous-time bi-virus model, IEEE Trans. Autom. Control, 64 (2019), 4891–4906. https://doi.org/10.1109/TAC.2019.2898515 doi: 10.1109/TAC.2019.2898515
![]() |
Variable | Definition |
N | Size of population =S+I1+I2+I12 |
β1,β2 | Attack rates of viruses 1 and 2 |
δ1,δ2 | Healing rates of viruses 1 and 2 |
σ1,σ2 | Strength of viruses 1 and 2 =Nβδ |
ε1,ε2 | Interaction factors of viruses 1 and 2 |
I1,I2,I12 | \# of nodes infected with either or both viruses |
S | Number of susceptible nodes |
Variable | Definition |
N | Size of population =S+I1+I2+I12 |
β1,β2 | Attack rates of viruses 1 and 2 |
δ1,δ2 | Healing rates of viruses 1 and 2 |
σ1,σ2 | Strength of viruses 1 and 2 =Nβδ |
ε1,ε2 | Interaction factors of viruses 1 and 2 |
I1,I2,I12 | \# of nodes infected with either or both viruses |
S | Number of susceptible nodes |