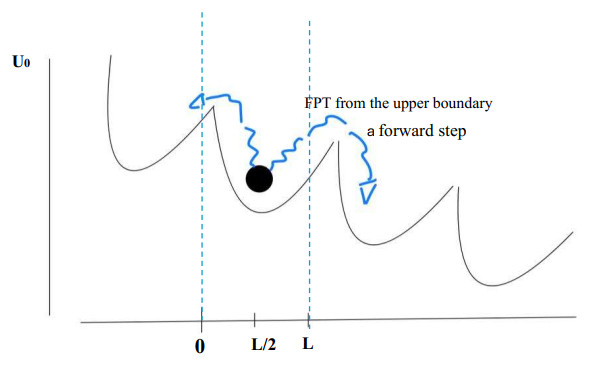
We propose two stochastic models for the interaction between the myosin head and the actin filament, the physio-chemical mechanism triggering muscle contraction and that is not yet completely understood. We make use of the fractional calculus approach with the purpose of constructing non-Markov processes for models with memory. A time-changed process and a fractionally integrated process are proposed for the two models. Each of these includes memory effects in a different way. We describe such features from a theoretical point of view and with simulations of sample paths. Mean functions and covariances are provided, considering constant and time-dependent tilting forces by which effects of external loads are included. The investigation of the dwell time of such phenomenon is carried out by means of density estimations of the first exit time (FET) of the processes from a strip; this mimics the times of the Steps of the myosin head during the sliding movement outside a potential well due to the interaction with actin. For the case of time-changed diffusion process, we specify an equation for the probability density function of the FET from a strip. The schemes of two simulation algorithms are provided and performed. Some numerical and simulation results are given and discussed.
Citation: Nikolai Leonenko, Enrica Pirozzi. The time-changed stochastic approach and fractionally integrated processes to model the actin-myosin interaction and dwell times[J]. Mathematical Biosciences and Engineering, 2025, 22(4): 1019-1054. doi: 10.3934/mbe.2025037
[1] | Jian Huang, Zhongdi Cen, Aimin Xu . An efficient numerical method for a time-fractional telegraph equation. Mathematical Biosciences and Engineering, 2022, 19(5): 4672-4689. doi: 10.3934/mbe.2022217 |
[2] | Simphiwe M. Simelane, Justin B. Munyakazi, Phumlani G. Dlamini, Oluwaseun F. Egbelowo . Projections of human papillomavirus vaccination and its impact on cervical cancer using the Caputo fractional derivative. Mathematical Biosciences and Engineering, 2023, 20(7): 11605-11626. doi: 10.3934/mbe.2023515 |
[3] | Madeleine Dawson, Carson Dudley, Sasamon Omoma, Hwai-Ray Tung, Maria-Veronica Ciocanel . Characterizing emerging features in cell dynamics using topological data analysis methods. Mathematical Biosciences and Engineering, 2023, 20(2): 3023-3046. doi: 10.3934/mbe.2023143 |
[4] | Muhammad Nadeem, Ji-Huan He, Hamid. M. Sedighi . Numerical analysis of multi-dimensional time-fractional diffusion problems under the Atangana-Baleanu Caputo derivative. Mathematical Biosciences and Engineering, 2023, 20(5): 8190-8207. doi: 10.3934/mbe.2023356 |
[5] | Ting Kang, Yanyan Du, Ming Ye, Qimin Zhang . Approximation of invariant measure for a stochastic population model with Markov chain and diffusion in a polluted environment. Mathematical Biosciences and Engineering, 2020, 17(6): 6702-6719. doi: 10.3934/mbe.2020349 |
[6] | Lin Zhang, Yongbin Ge, Xiaojia Yang . High-accuracy positivity-preserving numerical method for Keller-Segel model. Mathematical Biosciences and Engineering, 2023, 20(5): 8601-8631. doi: 10.3934/mbe.2023378 |
[7] | Hyunjin Ahn, Myeongju Kang . Emergent dynamics of various Cucker–Smale type models with a fractional derivative. Mathematical Biosciences and Engineering, 2023, 20(10): 17949-17985. doi: 10.3934/mbe.2023798 |
[8] | Maysaa Al Qurashi, Saima Rashid, Sobia Sultana, Hijaz Ahmad, Khaled A. Gepreel . New formulation for discrete dynamical type inequalities via $ h $-discrete fractional operator pertaining to nonsingular kernel. Mathematical Biosciences and Engineering, 2021, 18(2): 1794-1812. doi: 10.3934/mbe.2021093 |
[9] | Noura Laksaci, Ahmed Boudaoui, Seham Mahyoub Al-Mekhlafi, Abdon Atangana . Mathematical analysis and numerical simulation for fractal-fractional cancer model. Mathematical Biosciences and Engineering, 2023, 20(10): 18083-18103. doi: 10.3934/mbe.2023803 |
[10] | Giuseppe D'Onofrio, Enrica Pirozzi . Successive spike times predicted by a stochastic neuronal model with a variable input signal. Mathematical Biosciences and Engineering, 2016, 13(3): 495-507. doi: 10.3934/mbe.2016003 |
We propose two stochastic models for the interaction between the myosin head and the actin filament, the physio-chemical mechanism triggering muscle contraction and that is not yet completely understood. We make use of the fractional calculus approach with the purpose of constructing non-Markov processes for models with memory. A time-changed process and a fractionally integrated process are proposed for the two models. Each of these includes memory effects in a different way. We describe such features from a theoretical point of view and with simulations of sample paths. Mean functions and covariances are provided, considering constant and time-dependent tilting forces by which effects of external loads are included. The investigation of the dwell time of such phenomenon is carried out by means of density estimations of the first exit time (FET) of the processes from a strip; this mimics the times of the Steps of the myosin head during the sliding movement outside a potential well due to the interaction with actin. For the case of time-changed diffusion process, we specify an equation for the probability density function of the FET from a strip. The schemes of two simulation algorithms are provided and performed. Some numerical and simulation results are given and discussed.
The interaction between myosin and actin proteins inside the sarcomere is the basis of the muscle contraction process. The resultant mechanical work is essentially produced by the chemical energy released by ATP hydrolysis during successive Steps of the sliding of the myosin filaments. Usually, in the modeling context, we say that the myosin moves along the actin filament performing Steps mostly in a forward direction but sometimes also in a backward direction. The mechanism at the origin of this energy production is still not completely understood (see, for instance, [1,2,3] and references therein). More specifically, a skeletal muscle is composed by basic units, each called a sarcomere. Each sarcomere is essentially composed by two proteins: the actin and the myosin. The (deterministic) lever-arm theory ([4]) states that the reciprocal sliding of these proteins leads to the contraction and relaxation of muscles. The so-called subfragment-1 (S1) is the structure of the head, neck, and light chains of myosin. It is reasonable to interpret this as an independent generator of force and movement ([5,6]). Indeed, it is well-know that the molecular motor S1 acts as an enzyme that catalyzes the hydrolysis of ATP. This phenomenon occurs through a chemical and mechanical cycle: S1 attaches to the actin in the binding site, producing a cross-bridge, which contributes to hydrolyze ATP and causes a consequent conversion of chemical energy into movements and heat. Indeed, when the myosin head is bound to the actin, inorganic phosphate is released and the consequent power stroke takes place: this is the moment in which the myosin flexes pulling the actin along with it. The occurrence of the power stroke requires hydrolysis of ATP, which breaks a high-energy phosphate bond to release energy. The neck swing and the thermal bath in which the myosin is embedded determine the position of the myosin head and its potential energy, during the attachment of the myosin head to the actin filament and until to the final release of the phosphoric anion.
From a mathematical and modeling point of view, we aim to describe the dynamics of the myosin head in a binding site of the actin, during a cross-bridge, by means of the space-time evolution of a point-wise particle in a potential well; the model is a stochastic process X(t) representing the position of the particle at time t in a potential well. The potential of X(t), denoted by U(x), is a space-periodic function, and U′(x) stands for its potential energy. In the past, several potential profiles were investigated ([7]). Here, we consider the parabolic potential because, beyond the mathematical tractability of the corresponding model, the parabolic profile seems to be suggested by the shape of the actin site, i.e., it appears to be a realist representation of the binding site (for a simplified drawing, see Figure 1). Furthermore, it is also a better choice than that of the piece-wise linear profile, surely less accurate from biophysical point of view.
The stochastic modeling has been confirmed to be one of the more suitable tools for the description and comprehension of such phenomena. Indeed, consider that the Brownian motion W(t) is usually used to represent a thermal agitation (noise) in which such proteins are embedded (see, for instance, [7,8,9]). Substantially, as in [10], we start by considering a diffusion process for modeling the dynamics of a myosin particle also including time-dependent (forcing) terms in the drift of the diffusion: the F(t) term conveys the action of internal reactions and external loads, causing the tilt of the potential U(x). We call F(t) the tilting force. Such tilt contributes to driving the movement. It is worth mentioning that the inclusion of particular time-dependent tilting forces was born from the need to explain the occurrence of backward Steps of the myosin head ([10,11]).
Here, our purpose is two-fold: to provide a mathematical generalization of the time-non-homogeneous diffusion model proposed in [10] by using the fractional calculus approach for stochastic processes ([12]), and to provide a more realistic and refined biological model for such phenomenon, in particular, by including memory effects. In such a way, we trust that it will be possible to find a modeling answer to some additional aspects of muscle reactions to causes having a temporal duration, such as, for instance, tiredness, drugs, physical exercise or immobility, or exposition to high or low temperatures. It is clear that such phenomena have a corresponding condition at a microscopic level, i.e., on the actin-myosin interaction that, beyond performing forward-backward Steps, can evolve on a different time scale and embody the above conditions in a correlated behavior on time; in this sense, we say that it has memory. The introduction of time delays can help to improve the modeling of some other biological mechanisms (see, for instance, [13,14]).
Fractional calculus has proven to be an extremely useful tool for constructing new specialized stochastic processes that are able to model real phenomena including additional physiological evidences (see, for instance, [15,16,17]). Here, we show how fractional integrals and derivatives applied for time-changed processes or to stochastic differential equations are able to generate new models with specific peculiarities. The phenomenon considered here, specifically, the possibility that protein interaction takes place on different time-scales and preserves a sort of correlation, can be studied by means of two fractional stochastic modeling processes.
We first propose a time-change process with the long-range dependence property ([18,19]). The need of applying the time change strategy is justified by the wide approved approach that for many physical phenomena, the time of their evolution proceeds according to the occurrence of other random events (many unknown for now), conditioning the behavior of the phenomenon under observation. Moreover, the adoption of such random time-change induces a dilation of the time and stop periods. The proteins dynamics that appear to not be regular in time and that alternate activity periods and stop periods could be described by this kind of model. Still the actin-myosin dynamics show many obscure sides, and we are confident that such kind of mathematical models can open the door to future understanding such dynamics.
Then, we propose a fractionally integrated process ([16,20]), whose time-scale can be modulated by the fractional order of a non-local differential operator, involved in its dynamics. Moreover, the motivation of the introduction of a fractionally integrated process is the mathematical nature of this process that is constructed as a solution of a time-fractional differential stochastic equation; in this case, the involved derivative is the integral over time of previous variations of the system we are describing. This means that we are recording the history of the evolution of the system, suitably weighted, and we are using this in the corresponding differential equation. Consequently, the protein's dynamics that embodies all previous variations, more or less long (i.e., with more or less memory) could be described by this kind of model.
Under additional assumptions, such as that the dynamics is over-damped, meaning that the inertial force is disregarded, we limit to describe the dynamics confined into one space-period of the periodical morphology of the actin filament. Indeed, we assume that the diffusive dynamics occurs in a symmetric one-well parabolic potential, representing the binding site, tilted by a time-dependent force.
Moreover, by taking into account that the potential has a parabolic profile, we model the myosin Steps as it escapes from the potential well: the escape happens when the particle, starting from the bottom of the potential well, crosses over a local maximum of the potential. Note that the escapes can occur in a forward or backward direction. In our models, the processes represent the time-evolution of the myosin head confined in a strip, having two boundaries: the upper with respect to the initial position of the process) and the lower one. A forward Step occurs when the process reaches the upper boundary; a backward Step occurs when the process crosses the lower boundary (see Figure 1).
The dwell time is particularly important in the description of this phenomenon. It represents the time elapsed in the binding site, which, in the modeling context, corresponds to the time elapsing in the potential well before escaping from it. Its investigation can provide useful indications about reaction times and velocity of the muscle contraction.
We model it as the first exit time (FET) of the considered processes from the strip, assuming the boundaries as constant functions in time. In particular, the FET is equal to first passage time (FPT) through the upper boundary or to FPT through the lower boundary. Rigorous definitions of such random variables will be given. Consider also that the attainment of one of two boundaries can be viewed as the variation of the potential value allowing an escape. Moreover, the width of the strip is assumed to be equal to the average distance L between two consecutive binding sites. The initial position of the particle is in the middle of the strip, corresponding to the bottom of the potential well. See Figure 1 as a graphical illustration of such modeling elements (or, for a more detailed illustration, see also Figure 1 of [10]).
In [7], by combining loose coupling ([21,22]) and lever-arm theories ([4]), we provided a stochastic washboard potential model including biased thermal effects and the power stroke action. We were able to fit the set of data measured by the Yanagida group ([9]) on the sliding of myosin II heads on immobilized actin filaments for different load conditions. In [23], we considered the jump diffusion motion of a particle in a one-well potential; the described dynamics was affected by half-period space shifts at Poisson instants. In [24], the case of a double-well potential was also studied. In [10], the inclusion of the time-dependent forcing term was considered, and a refined diffusion model was proposed, describing separately the effect of internal and external forces.
In this contribution, by adopting all above modeling assumptions, we start from the diffusion process X(t) with equilibrium level in the bottom of the potential well (in L/2), having a time-dependent drift, whose dynamics occurs in the strip (0,L), as proposed in [10] (see Section 2). Then, by preserving all involved parameters and functions to represent protein dynamics, we specialize it by applying the stochastic time change by the process Eα(t) ([25]). The obtained process X(Eα(t)) (in Section 3) is a time-changed fractional diffusion process (see, for details, [19] and references therein). It is not a Markov process but it preserves the long-range dependence feature of the stochastic time used for the temporal change ([18]). Furthermore, we specify its mean and covariance functions.
We study its FET probability density function (pdf), particularly useful for understanding of the dwell time (in Section 4). By recalling the subordination operator (see, for instance, [26] and references therein), we address the problem by adopting the characterization of the subordinated FPT for the fractional time-changed diffusion processes given in [19]. Finally, we are able to provide a new equation for the FET of such processes, specializing, for this case, the Volterra-type integral equation approach (see Proposition 4.1). The results of this study suggest that the time-changed model can be suitable to describe randomly delayed dynamics and also correlated for long times, such as those that can be involved in muscle contraction subject to some illnesses or drugs.
A second fractional model we propose is based on a fractional version of the stochastic differential equation (SDE) of [10]. This is obtained by substituting the classical derivative with the fractional Caputo derivative (see, for instance, [16,27,28], specialized for neuronal activity models). Indeed, the non-local derivative (see [29,30]) allows the construction of a process that preserves the memory of its time evolution. In Section 5, we provide in Proposition 5.12 the explicit expression of the process X(t), that is properly a fractionally integrated process ([20]). Under the specific setting for parameters and involved functions, it is suitable to model the actin-myosin interaction. We also provide its main moments. Some essentials are also recalled for specifying the theoretical setting related to solutions of fraction SDEs. Regarding this model, the study results conclude that it is suitable to describe proteins dynamics strongly sensible to the application of forces, which could correspond to more reactive (sensible) muscle contractions, even if with a greater variability.
In order to prove the applicability of the proposed models, in Section 6, we devised two simulation algorithms: Algorithm 1, for the time-changed process, and Algorithm 2, for the fractionally integrated process. Specifically, Algorithm 1 exploits the transformation formula (3.17) by using the time-changed Brownian motion, whereas Algorithm 2 implements an ad hoc iterative scheme (6.2) based on a discretization formula for the Caputo-fractional derivative. In Section 7, we show and discuss some numerical and simulation results, such as the plot of mean functions, 3-dimensional plot of the covariance, plot of sample paths, and density estimations for simulated FETs. Some concluding remarks complete the manuscript.
We start by considering the stochastic model proposed in [10] for modeling the actin-myosin interaction, based on the following SDE:
dX=−[1θ(X−L2)−F(t)β]dt+√2κBTβdW,X(0)=x0, | (2.1) |
The above model born by the corresponding Langevin equation
˙x=−1βV′(x)+√2κBTβΛ(t), | (2.2) |
is widely adopted for describing the over-damped motion of a particle subject to a tilted potential V(x)=U(x)−Fx, with U(x) the potential and F the constant tilting force. Specifically, we list all above functions and parameters and the corresponding modelling as follows :
F, the tilting force: it is the sum of an internal force Fi and an external load Fe, i.e., we have F=Fi−Fe, U(x), the potential assumed to be a space periodic function with period L (as in [10], we assume L=5.5 nm equal to the myosin Step size).
β, the drag coefficient equal to 90 pN ns/nm,
T = 293 K, the environmental temperature,
κB, the Boltzmann constant.*
*Note that (′) is used to indicate the derivative of the function with respect to its own argument; in particular, in (2.2), (′) is for the space derivative and (⋅) denotes the time derivative.
In order to include time-varying effects of the tilting force, in [10], a time-depending force F(t) was considered in the SDE (2.1) that corresponds to the Langevin equation (2.2) by choosing a parabolic potential U(x) such that
U(X)=U0L2/4(X−L2)2, | (2.3) |
where U0 is the depth of the potential well (see Figure 1 of [10]). Hence, due to the form of V(x)=U(x)−F(t)x, we also have
V′(X)=U′(X)−F(t)=8U0L2(X−L2)−F(t). |
Hence, by setting the parameter θ as follows
θ=βL28U0, | (2.4) |
we finally obtain
dX=−[U′(X)−F(t)β]dt+√2κBTβdW, | (2.5) |
with W being the standard Brownian motion, and the initial condition is X(0)=x0. For the chosen functions and parameters, the last equation is the same of (2.1): it constitutes the corresponding Itˆo version of the Langevin equation (2.2). A time-non-homogeneous Ornstein-Uhlenbeck (OU) process X(t) is the solution of SDE (2.1), which exists under suitable assumptions on the tilting force [31]. The theory and properties of Gaussian diffusion (GD) ([32,33]) processes can be exploited for the considered case of equation (2.1).
Note that (2.1) describes the stochastic dynamics of a particle, whose position is described by the value of the stochastic process {X(t),t≥0} solution of (2.1).
By setting the starting point x0=L/2 (that means in the well of the potential), it is possible to model the dwell time of the myosin as the first exit time (FET) random variable
TX=inf{t≥0:X(t)≤0orX(t)≥L}, | (2.6) |
through the constant boundaries located at the origin (the lower one) and at L (the upper one). The FET pdf is:
gX(t|x0,0)=ddtP{TX≤t},withx0=L/2, | (2.7) |
The random variable dwell time plays a key role in modeling the sliding of the myosin head along the actin filament and the consequent production of ATP energy. The study of the dwell time and of its distribution is fundamental for the description and comprehension of the mechanism triggering muscle contraction. Indeed, such study is also extremely useful to predict pathological effects of drugs or diseases on muscles.
Beyond a small number of cases for which the pdf of FET is known in closed form, at the moment it is possible to construct approximations of such pdf. For the stochastic process X(t), evaluations of the pdf of the first passage time through a constant level can be obtained by solving with numerical procedures an integral equation (see [33]) or by some transformation methods or simulation techniques. In what follows, we give specific details for the considered cases.
In order to construct a time-changed fractional model, we adopt the stochastic process {X(t),t≥0} solution of (2.1) as the parent process, and we compose it with a stochastic time. The time-change is obtained by substituting the time with the positive non-decreasing process Eα(t) that is the inverse of an α-stable subordinator process σα(t). We finally consider in place of X(t) the following process:
Yα(t)=X(Eα(t)). |
By adopting such a model many theoretical results of [19] can be exploited and contribute to enrich the description of this biological phenomenon. With this in mind, we recall some mathematical essentials about the time-changed fractional process Yα.
For α∈(0,1), an α-stable subordinator σα(t) ([25,34]) is a strictly increasing positive Lévy process that for λ>0,t>0 has the Laplace transform:
E[e−λσα(t)]=e−tλα, |
with Laplace exponent λα and E being the expectation operator. A particular property of the subordinator process is the scaling property σα(t)d=t1/ασα(1), which is extremely useful in simulation algorithms. Note that the notation d= establishes the equality between finite dimensional distributions of the involved processes.
The inverse α-stable subordinator Eα(t) is defined as follows
Eα(t):=inf{r>0: σα(r)>t}, | (3.1) |
For any t>0, the random variables σα(t) and Eα(t) are absolutely continuous ([25]). Then, the Laplace-Stieltjes transform of Eα(t) (see, for instance, [35,36]) is the following Mittag-Leffler function
E[e−zEα(t)]=Eα(−ztα), | (3.2) |
where the one-parameter Mittag-Leffler function is defined as
Eα(y)=∞∑k=0ykΓ(1+αk),y,α∈C,Re(α)>0, | (3.3) |
The inverse of α-stable subordinator Eα(t) is a self-similar processes, i.e.,
Eα(t)d=b−αEα(bt)∀t≥0,b>0, | (3.4) |
The Eα(t) process has mean
E[Eα(t)]=tαΓ(α+1), | (3.5) |
and the covariance function (cf. [18] and [19]) for 0<s≤t :
cov[Eα(s),Eα(t)]=[αs2αB(α,α+1)+F(α;s,t)](Γ(α+1))2, | (3.6) |
Equation (3.6) involves special functions: the Beta function B(a,b), the hypergeometric function ([37])
F(α;s,t)=αt2αB(α,α+1;s/t)−(st)α |
and the incomplete beta function B(a,b;x), which for x∈[0,1], is defined as
B(a,b;x)=∫x0ua−1(1−u)b−1du, |
with B(a,b)=B(a,b;1). It is immediately available from (3.6) the expression of the variance:
var[Eα(t)]=t2α[2Γ(2α+1)−1(Γ(α+1))2], | (3.7) |
It is important to recall the asymptotic power law behavior of the covariance ([18]), that is
cov[Eα(s),Eα(t)]t→∞→s2αΓ(2α+1), | (3.8) |
by which the long-range dependence of such a process turns out.
If we denote by να(x,t) the probability density function (pdf) of Eα(t) and by γα(x) the pdf of σα(1), a relationship between them can be highlighted ([25])
να(x,t)=tαx−1−1αγα(tx−1α),x≥0,t>0., | (3.9) |
Recalling that Eα(t) assumes positive values for any t>0, its density is zero for x<0, with a discontinuity in x=0 (see the Appendix of [19] for further details). In particular, we keep in mind that Eα is an increasing, continuous process with constant values corresponding to the jumps of σα. Finally, we take note of the Laplace transform of να(x,t) with respect to t:
Lt→λ[να(x,t)]=λα−1e−xλα, | (3.10) |
The time-changed process is the composition of two processes: the parent process X(t) and the inverse of an α-stable subordinator Eα(t), independent on X(t). The resulting time-changed process Yα(t)=X(Eα(t)) has continuous sample paths because the parent process is continuous. Specifically, Yα(t) is constructed by using a time-non-homogeneous GD process as the parent process (the solution process of (2.1)), and the inverse of an α−stable subordinator processes for the time-change with pdf να(s,t).
In general, if f(x,s) is the pdf of the parent process, the time-changed process has the following pdf:
fα(x,t)=∫+∞0f(x,s)να(s,t)ds∀t∈I⊂R, | (3.11) |
We note that fα(x,t) is also called the subordinated density of f(x,t) by means of να(s,t). Theory, properties, and applications of subordinated processes can be found in [29,30,34,38,39,40].
Furthermore, by means of the change of variable ts−1/α=w and (3.9), (3.11) can alternatively be written as
fα(x,t)=∫+∞0f(x,(tw)α)γα(w)dw∀t∈I, | (3.12) |
In the specific case of the process X(t) solution of (2.1), we know its pdf f(x,t) is a normal density with mean:
mX(t)=E[X(t)]=x0e−t/θ+L2(1−e−t/θ)+e−t/θ∫t0F(τ)βeτ/θdτ, | (3.13) |
and the covariance function
c(s,t)=cov[X(s),X(t)]=κBTβθ(es/θ−e−s/θ)e−t/θ(0≤s≤t), | (3.14) |
Due to the Gauss-Markov nature of process X(t), its covariance is the product of two functions
ρ(t)=√2κBTβθ2(et/θ−e−t/θ),η(t)=√2κBTβe−t/θ, | (3.15) |
whose ratio r(t)=ρ(t)/η(t) is a monotonically non-decreasing function.
Hence, from (3.11) or (3.12), we are able to specify the subordinated pdf of the time-changed process Yα(t). In particular, we can also determine its mean and covariance functions by recalling that for the GD process X(t), the Doob transform holds, i.e.,
X(t)=mX(t)+η(t)W(r(t)), | (3.16) |
where {W(t),t≥0} is the standard Brownian motion, r(t)=ρ(t)/η(t) with η(t),ρ(t) being the functions in (3.15) (see [33]). From the Doob transform, the time-changed Yα process is such that
Yα(t)=X(Eα(t))=mX(Eα(t))+η(Eα(t))W(r(Eα(t))), | (3.17) |
with η(t),mX(t)∈C1(I), r(t) positive monotone increasing C1(I)−function (with r(0)=0) and W(r(Eα(t))) is the time-changed Brownian motion.
Remark 3.1. We remark that it is possible to consider the two time-changed Brownian motions. Indeed, note that the process W(r(Eα(t))) has the following subordinated pdf:
fW,α,r(x,t)=∫+∞0fW(x,r(s))να(r(s),t)dr(s) |
with fW(x,r(s)) the pdf of a standard Brownian motion W. On the other hand, it is also possible to consider another time-changed Brownian motion defined as Wa(r(t))=W(Eα(r(t))), whose pdf is the following one:
fWα(x,r(t))=∫+∞0fW(x,s)να(s,r(t))ds. |
Now, we put into evidence that:
fW,α,r(x,r(t))=∫+∞0fW(x,r(s))να(r(s),r(t))dr(s)=∫+∞0fW(x,y)να(y,r(t))dy=fWα(x,r(t)) |
where we applied the substitution of the variable y=r(s); in such a case, the two time-changed Brownian motions are equivalent.
Remark 3.2. (The time-changed Brownian motion Wα(t)=W(Eα(t)) and the Caputo derivative)
It is well-known ([25], [41], [42]) that the subordinated pdf
fWα(x,t|y,τ)=∫+∞0fW(x,t|y,s)να(s,t)ds, |
where fW(x,t|y,s), the transition pdf of W(t), satisfies the following fractional Fokker-Planck equation
DαtfWα(x,t|y,τ)=12∂2∂x2fWα(x,t|y,τ), | (3.18) |
with the initial condition
limt→τfWα(x,t|y,τ)=δ(x−y), | (3.19) |
where δ(⋅) is the delta function. In (3.18), the differential operator Dαt is the Caputo fractional derivative with respect to t, that for a C1 function, f(t) is as in [25]:
Dαtf(t)=1Γ(1−α)∫t0f′(τ)(t−τ)−αdτ, | (3.20) |
Due to this fact, the time-changed Brownian motion is also called anomalous or fractional diffusion. Such terminology is also extended to the process Yα(t), (see [19] for details).
Note that the time-changed process Yα is no more Gaussian and no more Markov process.
By exploiting the independence of Eα from W, we can calculate the mean of Yα:
E[Yα(t)]=E[mX(Eα(t))]+E[η(Eα(t))W(r(Eα(t)))]=E[mX(Eα(t))], | (3.21) |
From (3.13), (3.5) and (3.2), we finally have:
E[Yα(t)]=E[E[mX(u)|Eα(t)=u]] | (3.22) |
E[x0e−u/θ+L2(1−e−u/θ)+e−u/θ∫u0F(τ)βeτ/θdτ] | (3.23) |
=x0Eα(−tα/θ)+L2[1−Eα(−tα/θ)]+E[e−Eα(t)/θ∫Eα(t)0F(τ)βeτ/θdτ]. | (3.24) |
Such a mean function, in case of a constant force F(t)≡F, immediately becomes:
E[Yα(t)]=x0Eα(−tα/θ)+L2[1−Eα(−tα/θ)]+Fθβ(1−Eα(−tα/θ)). | (3.25) |
In case of a decaying exponential force F(t)=Fe−t/c with t,c>0, we have
E[Yα(t)]=x0Eα(−tα/θ)+L2[1−Eα(−tα/θ)]+E[e−Eα(t)/θ∫Eα(t)0Fe−τ(1/c−1/θ)βdτ]=x0Eα(−tα/θ)+L2[1−Eα(−tα/θ)]+E[e−Eα(t)/θFδβ(1−e−δEα(t))] | (3.26) |
with δ=(1/c−1/θ), from which it finally follows:
E[Yα(t)]=x0Eα(−tα/θ)+L2[1−Eα(−tα/θ)]+Fθcβ(c−θ)[Eα(−tα/c)−Eα(−tα/θ)]. | (3.27) |
It appears evident that the mean is written in terms of several Mittag-Leffler functions.
Then, by recalling the independence of the Brownian motion W from the inverse subordinator Ea, the covariance of Yα(t) can be evaluated, for s<t, as follows:
cov(Yα(s),Yα(t))=E{(Yα(s)−E[Yα(s)])(Yα(t)−E[Yα(t)])}=E{(Yα(s)−E[mX(Eα(s))])(Yα(t)−E[mX(Eα(t))])}=E[η(Eα(s))W(r(Eα(s)))η(Eα(t))W(r(Eα(t)))] | (3.28) |
=E[η(Eα(s))η(Eα(t))]cov(W(r(Eα(s))),W(r(Eα(t)))). | (3.29) |
By taking into account that cov(W(r(Eα(s))),W(r(Eα(t))))=E(W(r(Eα(s))),W(r(Eα(t))))=E[min{r(Eα(s)),r(Eα(t))}], one has
cov(Yα(s),Yα(t))=E[η(Eα(s))η(Eα(t))]E[min{r(Eα(s)),r(Eα(t))}].=E[η(Eα(s))η(Eα(t))]E[r(Eα(s))]=E[η(Eα(s))η(Eα(t))r(Eα(s))] | (3.30) |
due to the function r(⋅) and the process Eα both increasing. The explicit expressions of the functions η(⋅) and r(⋅) of the parent process X(t) are that of the η(t) function as in (3.15) and
r(t)=θ2(e2t/θ−1), |
respectively. Hence, in our specific case, we have
cov(Yα(s),Yα(t))=κBTθβE[e−Eα(s)/θe−Eα(t)/θ(e2Eα(s)/θ−1)]=κBTθβE[e(Eα(s)−Eα(t))/θ−e−(Eα(s)+Eα(t))/θ]. | (3.31) |
For t=ks, with k>0, we apply the self-similarity property (3.4) of the process Eα, that gives Eα(t)=Eα(ks)d=kαEα(s), and finally we obtain
cov(Yα(s),Yα(t))=κBTθβE[e(Eα(s)−kαEα(s))/θ−e−(Eα(s)+kαEα(s)/θ]=κBTθβE[eEα(s)(1−kα)/θ−e−(Eα(s)(1+kα)/θ]=κBTθβ{E[e(1−kα)Eα(s)/θ]−E[e−(1+kα)Eα(s)/θ]}=κBTθβ{Eα(sα(1−kα)θ)−Eα(−sα(1+kα)θ)}=κBTθβ{Eα(−tα−sαθ)−Eα(−tα+sαθ)} | (3.32) |
where we used (3.2). The long-range dependence of the covariance of Yα(t) can be deduced from the asymptotic behaviors of the above Mittag-Leffler functions involved in its expression. Indeed, it is sufficient to recall from [43] that, for 0<α<1, and z>0,
Eα(−zα)∼z−αΓ(1−α),z→+∞. |
This also means that the long-range dependence property of Eα(t) is inherited in the time-changed process Yα(t).
From (3.32), we can also specify the variance:
Var(Yα(t))=κBTθβ[1−Eα(−2tαθ)]. | (3.33) |
In order to clarify the results about the investigation of first passage times of time-changed processes and how these can be used for the specific model of the dwell-time, we resume some essentials. From [19], we recall that estimations of the FPT pdf for the time-changed process can be obtained by numerical resolution of an integral equation for the subordinated first passage time through a constant level. Then, we formulate the problem for the specific FET for the time-changed process Yα(t) and specialize the integral equation approach.
Indeed, we recall that for a GM process ([33], [10]) with the Doob representation (3.16) and f[x,t|y,τ] its normal transition pdf, the pdf of the FPT through the level L, for x0<L, is defined as
TL=inf{t≥0:X(t)≥L}, | (4.1) |
with density gTL(t|x0,0). For m(t),η(t),ρ(t),r(t), C1(I)-functions defined in subsection 3.2, gTL(t|x0,0) is a solution of a non-singular second-kind Volterra integral equation:
gTL(t|x0,0)=−2Ψ[L,t|x0,0]+2∫t0gTL(τ|x0,0)Ψ[L,t|L,τ]dτ(x0<L) | (4.2) |
where
Ψ[L,t|y,τ]={−m′(t)2+m(t)2ρ′(t)η(τ)−η′(t)ρ(τ)ρ(t)η(τ)−η(t)ρ(τ)−y−m(τ)2η′(t)ρ(t)−η(t)ρ′(t)ρ(t)η(τ)−η(t)ρ(τ)}f[L,t|y,τ]. | (4.3) |
For Yα(t) process, consider the α-stable subordinated pdf
gTL,α(t|x0,0)=∫∞0gTL(ϑ|x0,0)να(ϑ,t)dϑ, | (4.4) |
Then, denote by TL,α the subordinated FPT with pdf gTL,α(t|x0,0). In Theorem 4.1 of [19], was proved that gTL,α(L,t|x0,0) satisfies the equation
gTL,α[t|x0,0]=−2Ψα[L,t|x0,0]+2I0,tΨαgTL[t|x0,0] | (4.5) |
with the following integral operator
I0,tΨαgTL[t|x0,0]=∫t0gTL[τ|x0,0]Ψα[L,t|L,τ]dτ | (4.6) |
and
Ψα[L,t|y,τ]=∫∞0Ψ[L,ϑ|y,τ]να(ϑ,t)dϑ. | (4.7) |
Numerical quadrature procedures applied to equation (4.5) provide approximations of gTL,α for which no closed form results are available.
From [10] and [44], we give some known results about the FET TX of X(t) defined in (2.6) evolving in the strip [0,L]∈R with L>0, starting from X(0)=x0∈[0,L] (in particular, we chose X0=L/2). It is such that TX=min{T0,TL}, where T0 and TL are the FPTs through the lower constant boundary 0 and the upper one L, respectively, i.e.,
T0=inf{t≥0:X(t)≤0andX(s)<L,∀0≤s<t}, | (4.8) |
TL=inf{t≥0:X(t)≥LandX(s)>0,∀0≤s<t}, | (4.9) |
Moreover, gT0(t|x0,0) and gTL(t|x0,0) are the density functions of FPTs T0 and TL, respectively. Note that the random variable TL is different from TL defined in (4.1), because TL is a FPT of the same process through the level L in the presence of an additional absorbing boundary in zero. And, vice versa, T0 is the FPT of the X(t) process through the level zero in the presence of the additional absorbing boundary in L. Hence, the FET pdf of T of X(t) defined in (2.6) is such that
gX(t|x0,0)=gT0(t|x0,0)+gTL(t|x0,0), | (4.10) |
From [44], gT0(t|x0,0) and gTL(t|x0,0) are solutions of the two following coupled integral equations:
gT0(t|x0,0)=ψ1(t|x0,0)−∫t0[ψ1(t|0,τ)gT0(τ|x0,0)+ψ1(t|L,τ)gTL(τ|x0,0)]dτgTL(t|x0,0)=−ψ2(t|x0,0)+∫t0[ψ2(t|0,τ)gT0(τ|x0,0)+ψ2(t|L,τ)gTL(τ|x0,0)]dτ | (4.11) |
where
ψj(t|z,τ):=−{m′(t)+[Sj−m(t)]ρ′(t)η(τ)−η′(t)ρ(τ)ρ(t)η(τ)−η(t)ρ(τ)+[z−m(τ)]η′(t)ρ(t)−ρ′(t)η(t)ρ(t)η(τ)−η(t)ρ(τ)}fX[Sj,t|z,τ](j=1,2) | (4.12) |
with fX[x,t|z,τ] being the transition pdf of X(t) and with S1=0,S2=L.
Note that the FET pdf (4.10) of X(t) can be evaluated by applying the standard numerical algorithms for the resolution of this kind of integral equation as (4.11) or a specific algorithm given in [44]. Due to the Gaussian distribution of the X(t) process, only the mean and covariance functions of the process are required to apply the numerical procedure; consequently, the functions ψi(t|z,τ) (i=1,2) involved in the integral equations are also completely specified.
For the FET of the time-changed process Yα(t), we can proceed similarly to the case of subordinated FPT. Specifically, we define the subordinated FPT pdfs:
gT0,α(t|x0,0)=∫∞0gT0(ϑ|x0,0)να(ϑ,t)dϑ, | (4.13) |
gTL,α(t|x0,0)=∫∞0gTL(ϑ|x0,0)να(ϑ,t)dϑ, | (4.14) |
in such a way that the FET is:
gX,α(t|x0,0)=gT0,α(t|x0,0)+gTL,α(t|x0,0), | (4.15) |
Proposition 4.1. The subordinated FPT pdf gT0,α(t|x0,0) and gTL,α(t|x0,0) are solutions of the two following coupled equations:
gT0,α(t|x0,0)=ψ1,α(t|x0,0)−∫t0[ψ1,α(t|0,τ)gT0(τ|x0,0)+ψ1,α(t|L,τ)gTL(τ|x0,0)]dτgTL,α(t|x0,0)=−ψ2,α(t|x0,0)+∫t0[ψ2,α(t|0,τ)gT0(τ|x0,0)+ψ2,α(t|L,τ)gTL(τ|x0,0)]dτ | (4.16) |
and
ψj,α[t|y,τ]=∫∞0ψj[ϑ|y,τ]να(ϑ,t)dϑ. | (4.17) |
Proof. The proof follows the proof of Theorem 4.1 of [19].
Indeed, we substitute gT0(t|x0,0) and gTL(t|x0,0) as in (4.11) in the right-hand side of (4.13) and (4.14), respectively, and we have
gT0,α(t|x0,0)=ψ1,α(t|x0,0)−∫∞0∫θ0[ψ1(ϑ|0,τ)gT0(τ|x0,0)+ψ1(ϑ|L,τ)gTL(τ|x0,0)]dτνα(ϑ,t)dϑgTL,α(t|x0,0)=−ψ2,α(t|x0,0)+∫∞0∫θ0[ψ2(ϑ|0,τ)gT0(τ|x0,0)+ψ2(ϑ|L,τ)gTL(τ|x0,0)]dτνα(ϑ,t)dϑ. | (4.18) |
All involved functions are L1 and the Fubini theorem can be applied to the integral terms at the right-hand side of equations in (4.18), and we can write
∫∞0∫θ0[ψ1(ϑ|0,τ)gT0(τ|x0,0)+ψ1(ϑ|L,τ)gTL(τ|x0,0)]dτνα(ϑ,t)dϑ | (4.19) |
=∫t0[gT0(τ|x0,0)∫∞0ψ1(ϑ|0,τ)να(ϑ,t)dϑ+gTL(τ|x0,0)∫∞0ψ1(ϑ|L,τ)να(ϑ,t)dϑ]dτ | (4.20) |
and
∫∞0∫θ0[ψ2(ϑ|0,τ)gT0(τ|x0,0)+ψ2(ϑ|L,τ)gTL(τ|x0,0)]dτνα(ϑ,t)dϑ | (4.21) |
=∫t0[gT0(τ|x0,0)∫∞0ψ2(ϑ|0,τ)να(ϑ,t)dϑ+gTL(τ|x0,0)∫∞0ψ2(ϑ|L,τ)να(ϑ,t)dϑ]dτ | (4.22) |
where we used
∫∞0ψi[ϑ|Sj,τ]να(ϑ,t)dϑ=0,τ>t,i,j=1,2,S1=0,S2=L. |
Finally, by using (4.17) in the right-hand sides of (4.19) and (4.21), we obtain the coupled equations (4.16) that complete the proof.
Numerical procedures can be devised in order to solve the coupled equations (4.16), but note that for each value of t, a quadrature procedure is also required for evaluations of (4.17) and finally to obtain approximations of the FET density (4.15). Additionally, evaluations of FPT pdfs for the parent process X(t) are also required. Even if it is a practicable method, a particular effort can be done to reduce the computational cost of such procedures. This will be the object of our future work. An alternative method is provided by simulation algorithms (see Section 6).
Another way to model memory effects in the dynamics of the actin-myosin is to reconsider equation (2.1) and substitute the classical derivative with the fractional Caputo derivative as defined in (3.20), in such a way that the following fractional SDE can be assumed for the model:
DαX=−[1θ(X−L2)−F(t)β]dt+√2κBTβdW,X(0)=x0∈(0,L), | (5.1) |
Similar fractional models can be found in [16,27,28,45] and, specialized for neuronal activity models. Here, by following the approach adopted in [16], we first recall the theoretical results of [46] and specialize them for this kind of equations. Note that the stochastic fractional differential equation (5.1) will be understood in a sense of equation (5) of [46] (to avoid collision with the theory developed in [47] and [48]). Indeed, by comparing with Eq. (2) of [46], this fractional SDE can also be written as follows
1Γ(1−α)∫t0DX(τ)(t−τ)−αdτ=−[1θ(X(t)−L2)−F(t)β]+√2κBTβdWdt,X(0)=x0∈(0,L), | (5.2) |
with D=ddt the usual derivative. Regarding such a model, we give the same biological interpretation as explained in Introduction and in Section 2, due to the fact that the RHS of Eq.(5.1) is the same of RHS of Eq.(2.1), but with a difference related to the LHS. Indeed, by focusing on the LHS of Eq.(5.2), it is possible to give the following biological justification: differently from the integer case of Eq.(2.1), the solution process X of Eq.(5.2) is a process whose time evolution at time t takes into account its whole past evolution until time t. This is essentially due to the presence of the special integro-differential operator on the LHS of Eq.(5.2). In the application context, this process can be adopted for modeling biological phenomenon including some memory (i.e., more or less memory depending of the α value). This could be the case of muscle contraction, and all underlying protein dynamics, in tiredness condition or aging.
Equation (5.1) is a Caputo-fractional SDE. Indeed, consider T>0 a real number and the bounded interval [0,T], a typical Caputo-fractional stochastic differential equation is
DαX(t)=[AX(t)+B(t,X(t))]dt+σ(t,X(t))dW(t)X(0)=X0, | (5.3) |
with {Wt}t∈[0,∞) a real standard Brownian motion in (Ω,F,{Ft}t∈[0,∞),P) a complete filtered probability space, α∈(1/2,1), A,B,σ real-valued measurable functions on [0,T] satisfying the following assumptions:
(ⅰ) there exist L>0 such that ∀x,z∈R,∀t∈[0,T]
‖B(t,x)−B(t,z)‖+‖σ(t,x)−σ(t,z)‖≤L‖x−z‖ |
(ⅱ) ∫T0‖B(s,0)‖2ds<∞, esssups∈[0,T]‖σ(s,0)‖<∞.
We are in the suitable space L2(Ω,Ft,P), for any t∈[0,∞), that is the space of mean square integrable functions f:Ω→R with the mean square norm ‖f‖ms=√E(‖f‖2). Consider a process X:[0,∞)→L2(Ω,F,P) that is F-adapted if X(t)∈L2(Ω,Ft,P),∀t≥0. Similarly to [49], we specify that a classical solution of (5.3) is a F-adapted process X with initial condition X(0)=X0∈L2(Ω,F0,P) such that for t∈[0,T]:
X(t)=X0+1Γ(α)∫t0(t−s)α−1(AX(s)+B(s,X(s)))ds+1Γ(α)∫t0(t−s)α−1σ(s,X(s))dWs, | (5.4) |
or, equivalently,
X(t)=X0+Iα(AX(t)+B(t,X(t)))+Iα(σ(t,X(t))dWt), | (5.5) |
where Iα is the Riemann-Liouville fractional integral (see, for instance, [26], [50]) of order α defined as follows
Iα(f)(t)=1Γ(α)∫t0(t−s)α−1f(s)ds,∀t∈R+, | (5.6) |
with Γ the Gamma Euler function, i.e., Γ(z)=∫+∞0tz−1e−tdt z>0.
Moreover, in (5.5), the operator Iα(σ(s,X(s))dWs) is a stochastic fractional integral defined as
Iα(σ(t,X(t))dWt)=1Γ(α)∫t0(t−s)α−1σ(s,X(s))dWs, | (5.7) |
We note that this integral can be viewed as a generalization of a fractional integral that defines the Lévy fractional Brownian motion, i.e.,
Iα(dW(t))=1Γ(α)∫t0(t−τ)α−1dW(τ), | (5.8) |
where W is a standard Brownian motion and the integral has to be interpreted in the Itô sense. We recall that if α∈(12,1), for fixed t>0, the function (t−τ)α−1 is in L2(0,t). Thus, the above process is well-defined and it is a Gaussian process starting from 0 at time 0 with probability 1.
Specifically, we recall that in [49], it was proved that for any given X0, there exists a unique solution, whose explicit form was found with a variation constant formula. We can write the specific result in the next proposition.
Proposition 5.1. The solution process of the fractional SDE (5.3) with initial condition X(0)=X0∈L2(Ω,F0,P), ∀t∈[0,T], is:
X(t)=Eα(tαA)X0+∫t0(t−s)α−1Eα,α((t−s)αA)B(s,Xs)ds+∫t0(t−s)α−1Eα,α((t−s)αA)σ(s,Xs)dWs | (5.9) |
with Eα,α(z)=∑∞k=0zkΓ(αk+α) and Eα(z)=Eα,1(z) Mittag-Leffler functions ([51]).
The proof is given in [46].
We will apply the following corollary to the fractional SDE (5.1)
Corollary 5.1. The fraction SDE:
DαX(t)=[AX(t)+B(t)]dt+σ(t)dW(t)X(0)=X0, | (5.10) |
with B∈L2([0,T],R), σ∈L∞([0,T],R) admits the following solution process
X(t)=Eα(tαA)X0+∫t0(t−s)α−1Eα,α((t−s)αA)B(s)ds+∫t0(t−s)α−1Eα,α((t−s)αA)σ(s)dWs. | (5.11) |
Note that for α=1, the fractional derivative and integral reduce to the corresponding classical ones ([52]). Consequently, the solution process is X(t) of the integer stochastic model.
Proposition 5.2. The solution process of (5.1) is
X(t)=Eα(−tα/θ)x0+L2θ∫t0(t−s)α−1Eα,α(−(t−s)α/θ)ds+1β∫t0(t−s)α−1Eα,α(−(t−s)α/θ)F(s)ds+√2κBTβ∫t0(t−s)α−1Eα,α(−(t−s)α/θ)dWs. | (5.12) |
Proof. By applying Corollary 5.1 to equation (5.1) with the following setting
A=−1/θ,B(t)=βL+2θF(t)2β,σ(t)≡√2κBTβ∀t>0 |
with all above parameters and functions under assumptions (ⅰ) and (ⅱ) of this subsection, we obtain the solution (5.12).
The solution process (5.12) is a Gaussian process (see, for instance, [53]:Theorem 4) with the following mean:
E[X(t)]=Eα(−tα/θ)x0+L2θ∫t0(t−s)α−1Eα,α(−(t−s)α/θ)ds+1β∫t0(t−s)α−1Eα,α(−(t−s)α/θ)F(s)ds. | (5.13) |
The calculus of its covariance leads to:
Cov(X(u),X(t))=E[(X(u)−E[X(u)])⋅(X(t)−E[X(t)])]=2κBTβE[∫u0(u−s)α−1Eα,α(−(u−s)α/θ)dWs∫t0(t−s)α−1Eα,α(−(t−s)α/θ)dWs]=2κBTβE[Iα(Eα,α(−(u−s)α/θ)dWu)Iα(Eα,α(−(t−s)α/θ)dWt)] | (5.14) |
where the stochastic fractional integral Iβ is that defined in (5.7). Finally, by solving Eq.(5.14) as in [45], we obtain, for u<t,
Cov(X(u),X(t))=2κBTβ∫u0(u−s)α−1Eα,α(−(u−s)α/θ)(t−s)α−1Eα,α(−(t−s)α/θ)ds. | (5.15) |
From the covariance we can also derive the variance as follows:
Var(X(t))=2κBTβ∫t0(t−s)2α−2(Eα,α(−(t−s)α/θ))2ds. | (5.16) |
We remark that the provided expressions of the mean (5.13) and of the covariance (5.14) of the process X(t) are extremely useful to obtain simulations of these processes. Details of a possible simulation strategy are given in the next section.
We propose some possible simulation algorithms for fractional stochastic models suitably specified for the actin-myosin dynamics. First, we provide the algorithm for the simulation of trajectories of the time-changed process Yα(t) and the sampling of its first exit times. Then, we propose a further simulation algorithm for the fractionally integrated process X(t) of Section 5. Finally, we suggest for comparison another discretization scheme that can be adopted for time-changed fractional stochastic differential equation due to [54].
We refer to the time-changed process Yα(t) studied in Section 3. Trajectories of the time-changed process can be simulated following the Steps listed below, and then samples of simulated dwell-times can be provided. Consequentially, from simulations, it is possible to give estimations of the pdf of the dwell-time of the actin-myosin interaction for the proposed model.
Algorithm 1
The main Steps of the simulation algorithm are:
● Step1: in INPUT, provide the values of parameters: α,β,θ,L, the force F, the Step size value Δt for the time discretization, N as the maximum number of time Steps, and M the sample size of dwell times to be simulated;
● Step2: generate in correspondence to time instants 0=t0<tΔt<t2Δt<⋯<tNΔt the random variables Eα(tΔt)<Eα(t2Δt)<⋯<Eα(tNΔt) by using ad hoc functions of R programming packages. In short: use the R-package stabledist to generate the random time σα(s) of the α-stable subordinator; then, according to the definition (3.1), determine the value Eα(t) as the FPT of σα(s) by t.
● Step3: generate a random path of the time-changed Brownian motion in the random times r(Eα(tΔt))<r(Eα(t2Δt))<⋯<r(Eα(tNΔt)), i.e., W(r(Eα(tΔt))),…,W(r(Eα(tNΔt)) as
W(r(Eα(tiΔt))=W(r(Eα(t(i−1)Δt))+√r(Eα(tiΔt))−r(Eα(t(i−1)Δt))Zi |
for i=1,…,N, with W(r(Eα(t0))=0, with Zi∼N(0,1) as standard normal random variables;
● Step4: evaluate a random path of Yα(iΔt)=X(Eα(tiΔt)) by means of (3.17) for i=1,…,N, i.e.,
X(Eα(tiΔt))=mX(Eα(tiΔt))+η(Eα(tiΔt))W(r(Eα(tiΔt)) |
for i=1,…,N, with function mX(⋅) being given in (3.13) and η(⋅) in (3.15);
● Step5: check if X(Eα(tiΔt)) is over the level L or under level zero; if so, and if it is the first time this occurs, record the correspondent time instant Eα(tiΔt) that will be an instance of the dwell time (i.e., the FPT of the time-changed process);
● Step6: go to Step2 and repeat the procedure M times with different seeds for the generation of the sequences of N random instants Eα(tΔt)<Eα(t2Δt)<⋯<Eα(tNΔt) providing the M simulated trajectories of the time-changed process.
● Step7: plot an histogram and/or an approximating density for the sample of the simulated M dwell times.
Note that, for the numerical evaluation of Mittag-Leffler functions in Step4, we use the MittagLeffleR R-package.
Algorithm for the simulation of fractionally integrated processes X(t) of Section 5, we can indicate that it is possible to adopt the simulation algorithm as that in subsection 4.1.1 of [20] based on the Cholesky decomposition method of the covariance matrix of the process by which pseudo-Gaussian sample paths can be obtained. Note that in order to apply such algorithm, the numerical evaluation of the mean (5.13) and of the covariance (5.14) of the process is required. High computational costs and possible round-off errors can affect this method.
A second strategy for simulations of sample paths of X(t) is that adopted in Section 3 of [27], substantially based on the following discretization formula of the Caputo derivative defined in (3.20), for t=NΔt:
DαX(t)≈(Δt)−αΓ(2−α)N−1∑k=0[X((k+1)Δt)−X(kΔt)][(N−k)1−α−(N−k−1)1−α], | (6.1) |
From (6.1), an iterative discretization scheme of the fractional SDE (5.1) can be derived and, specifically, the simulation of sample paths is obtained as follows:
Algorithm 2
● Step1: in INPUT, provide the values of parameters: α,β,θ,L, the force F, the Step size value Δt for the time discretization, N the maximum number of time Steps, and M the sample size of dwell times to be simulated;
● Step2: in correspondence to time instants 0=t0<tΔt<t2Δt<⋯<tNΔt construct the path of X(t) process adopting the following iterative scheme:
X(nΔt)=X((n−1)Δt)−n−2∑k=0[X((k+1)Δt)−X(kΔt)][(n−k)1−α−(n−k−1)1−α] | (6.2) |
+(Δt)αΓ(2−α)(−1θX((n−1)Δt)+L2θ+F((n−1)Δt)β+√2κBTβZnΔt),n=2,…,N, | (6.3) |
with Zn∼N(0,Δt) normal random variables, X(0)=x0 and
X(Δt)=X(0)+(Δt)αΓ(2−α)2(−1θX(0)+L2θ+F(0)β+√2κBTβZ1Δt) |
,
● Step3: check if X(nΔt) is over the L value or under zero; if so, and if it is the first time this occurs, record the correspondent time instant nΔt that will be an instance of the dwell time;
● Step4: go to Step2 and repeat the procedure M times with different seeds for the generation of the sequences of N independent random pseudo-Gaussian numbers Zn∼N(0,Δt),n=1,…,N, providing the M simulated trajectories of the process X(t).
● Step5: plot an histogram or/and an approximating density for the sample of the simulated M dwell times.
Now, by referring to some relevant results obtained in [47], [54], [55], [56], we consider the time-changed Brownian motion Wα(t)=W(Eα(t)) and the process Zα(t) solution of the following equation:
dZα(t)=−[1θ(Zα(t)−L2)−F(t)β]dEα(t)+√2κBTβdWα(t),Zα(0)=L/2, | (6.4) |
that we propose as an additional model for the protein dynamics. It is considered for comparisons, validations, and further investigations of the proposed models. Note that the process Zα(t) is such that, for α=1, Zα(t)≡X(t) because the (6.4) reduces to (2.1).
Regarding the well-posedness of the considered stochastic equation, we are under all assumptions to guarantee the existence and path-wise uniqueness of the solution ([54]). Then, we define the solution of the equation (6.4) as the following one
Zα(t)=Zα(0)−∫t0[1θ(Zα(s)−L2)−F(s)β]dEα(s)+√2κBTβWα(t) |
that is the sum of a stochastic integral with respect to Eα(t) and the time-changed Brownian motion Wα(t). The stochastic integral with respect to Eα(t) is well-defined as a stochastic integral with respect to semi-martingales (see, for instance, [54]).
Such fractional differential model allows an alternative simulation algorithm based on an Euler-Maruyama discretization scheme (cf. [56]). Indeed, from (6.6), we have
Zα(t+Δt)=Zα(t)−[1θ(Zα(t)−L2)−F(t)β](Eα(t+Δt)−Eα(t))+√2κBTβ(Wα(t+Δt)−Wα(t)), | (6.5) |
where it is possible to follow Step2 of Algorithm 1 to generate random times Eα(t) and to follow Step3 of Algorithm 1 to simulate the values of the time-changed Brownian motion Wα(t).
Remark 6.1. A comparison between Zα(t) and the time-changed process X(Eα(t)): the last one can be assumed as the solution of the following equation:
dX(Eα(t))=−[1θ(X(Eα(t))−L2)−F(t)β]dEα(t)+√2κBTβdW(Eα(t)), | (6.6) |
In order to see the similarities and differences between the two processes, it is possible to note that a possible Euler-Maruyama scheme for a simulation algorithm would be the following
X(Eα(t+Δt))=X(Eα(t))−[1θ(X(Eα(t))−L2)−F(t)β](Eα(t+Δt)−Eα(t))+√2κBTβ(Wα(t+Δt)−Wα(t)), | (6.7) |
This could be an alternative algorithm for simulation of the process X(Eα(t)), even if some consistency conditions should be investigated about the equivalence between the solution process of (6.6) and the process X(Eα(t)) constructed in Section 3. All these and other mathematical refinements will be the subject of a next work.
We perform the suggested simulation algorithms in order to construct sample paths of the proposed processes and we derive the statistical density estimations of the simulated dwell times, as described in our models. No quantitative comparisons and/or fit with experimental data is done, but comparisons with the classical integer case are provided by specifying the innovations of such models in terms of the biological modeling of actin-myosin interactions. The following numerical results are given in graphical form in order to show visible validations of the used algorithms and explain the adequacy of the models.
The set of fundamental parameters is done as indicated in Section 2. Other choices are specified in the caption of each figure.
Figure 2 shows the plots of the mean function of Yα(t) (solid line) and a corresponding simulated path. Specifically, (a)-(b)-(c) refer to mean function (3.25) of Yα(t) for different α values in the presence of a constant tilting force F, whereas (d)-(e)-(f) refer to the mean function (3.27) of Yα(t) in the presence of a time dependent force F(t)=Fe−t/c. The simulated trajectories are performed by means of Algorithm 1. By comparing the classical integer case (a) with fractional cases (b)-(c) (and, respectively, (d) with (e)-(f)), it is possible to see how the choice of the fractional order affects the mean function of the processes and the corresponding behavior of random paths. Periods of constancy in the evolution time of its trajectories appear more evident and frequent as α decreases, in both cases of a constant tilting force F (Figure 2 (a)-(b)-(c)) and also for a time-dependent tilting force (Figure 2 (d)-(e)-(f)). This feature derives from the stretching effect of the time due to the adoption of the time-change regulated by the fractional order α. In terms of biological modeling, such adaptability feature of the proposed model Yα reveals to be especially useful to describe (and predict) anomalous periods of muscle rest alternating with periods of movement, circumstances that can be verified in the presence of illness conditions. Just in this last case, a delayed muscle dynamics can be observed. Furthermore, periods of immobility, possibly caused by uncontrolled (i.e., random) events, are encoded by the stochastic time Eα(t).
Figure 3 shows the plots of the mean function of Yα(t) subject to a constant tilting force (3.25) on the left side, and to time dependent tilting forces (3.27) on the middle and right side. For different values of the fractional order α used in the time change, the average of a delayed evolution of the corresponding means appears evident in these plots; the more or less evidence of the delay is regulated just by the values of α. In particular, the behavior of the mean function of Yα(t) is gradually more delayed for decreasing values of α. The biological interpretation analogous to that of Figure 2 can be done, taking into account that in this figure, we provide mean functions corresponding to further values of α.
Figure 4 show the plots of approximated FET probability densities of Yα as estimates of dwell times. It is possible to see how the effect of the fractional order in the time-changed process is relevant also on the profiles of the FET-estimated density for different α values. It is noteworthy the evident heavy tails of these densities, essentially due to the long-range dependence property of the process Yα(t). In terms of biological modeling, this means that this model is able to describe slow movements represented by means of the non-zero probability of long dwell times (FETs). These circumstances can occur in the presence of a delayed muscle response to stimulus caused by physical damages or mental diseases.
We proceed by presenting our investigations about the process X similarly to what was previously carried out for the process Yα, while highlighting differences and similarities.
Figure 5 shows (on the left) the mean functions of the fractional integrated process X(t), their tails (on the middle), and some corresponding simulated paths (on the right) by means of Algorithm 2 for specified α values when a time-depending tilting force is applied. The main feature we captured by this numerical investigations is that such a process reacts to the tilting force; alternatively, we can say that it is very sensitive to the tilting force due to the high value of the mean in correspondence to short times. In particular, the peak of the mean plot is higher for higher values of α, until α=0.9, which is closer to the classical integer case. Instead, successively, i.e., for long times, we can observe a rapid decay differently from the mean of Yα in Figure 3. Look then at the behavior of the means in the tails in the middle of Figure 5 and observe that an opposite order of tails occurs: indeed, the decay is slower for lower values of α. In a biological context, this can be interpreted as an instantaneous reaction of the mean behavior of the particle to the effect of the tilting driving force similarly to the mean of Yα (compare with the right side of Figure 3), even if for Yα such a reaction persists for longer. Such a reaction appears to be less evident for lower values of α. We can still specify that, due to the mathematical construction of such a process, the order α of the fractional derivative affects the process by including memory effects in its time evolution as α decreases. This is also shown in the middle side of Figure 5, regarding the behavior of the mean tails. Hence, for lower values of α, the model seems less sensitive to the effect of the driving force, but the model still encodes such effect in longer times, which can be explained as the preservation of memory effects.
Figure 6 shows the estimations of dwell time (FET) density from 103 simulated paths by means of Algorithm 2 of the fractionally integrated process X(t). In this case, (quasi) heavy tails also appear in the FET density estimations, but they appear very early, i.e., they are visible on a reduced time period with respect to that of Yα case. Indeed, in this case, we can talk about a preservation of memory effects as α decreases, while for Yα we can talk about an enlarged time-scale. We understand that this phenomenon is also due to the increased variability (variance) that is in this model as α decreases. In this regard, compare the simulated paths of Yα (Figure 2) and those of X (in the right side of Figure 5 right): we will show that the difference is also due to the different variance values of the two processes (see subsection 7.3).
We investigate how the behavior of the covariance and variance of the two proposed models changes for different values of α.
In Figure 7, we plot the covariance of Yα(t) as in Eq. (3.32) on the left and of the variance as in Eq. (3.33). The resulting curves are ordered by values of α. The covariance curves decay slower for lower values of α; we expect this due to the power decay already discussed about this covariance. The variance curves increase slower for lower values of α.
A wide investigation into covariance is performed by using 3-dimensional plots; indeed, Figure 8 is composed by three different 3-dimensional plots of covariance for 3 different values of α. Figure 9 completes the illustrations of the cov(Yα(s),Yα(t)) as in Eq. (3.32) in order to improve the understanding of the behavior of such a function. In particular, the color maps put in evidence that the correlation persists even for long periods especially for low values of α. This feature allows to adopt such a model when the proteins interaction seems randomly delayed (as well as the muscle contraction).
Analogous investigations were carried out for process X. Figures 10, 11, 12 correspond to Figures 7, 8, 9. The behaviors of the covariance and variance of X are different than those of Yα. The covariance decay occurs more rapidly, whereas the variance increases as α increases. Then, we can focus on the color maps of Figure 12 in which it is visible that an extreme concentration of high values of the covariance is in the variance and just for α=0.6. Compare Figure 12 with Figure 9: the high covariance values are distributed in different zones in the plane (s,t).
For biological applications, we understand that this feature of the two models allows to distinguish when a model is preferable to the other one. Indeed, if we need to describe the phenomenon enlarging time, we can adopt the Yα process that preserves the correlated behavior. On the other hand, if we want to preserve the history of the phenomenon, we can use the X process that includes memory effects. In this last case, the associated higher variance can sometimes also be used to explain some anomalous events.
Finally, in Figure 13, we compare the FET density estimations of the two processes Yα and X subject to the same tilting force. Differences: the height of the peaks is greater for X (on the right side) than for Yα (on the left side); hence, the process X seems to be more sensitive to the effect of the tilting force in such a way that there is also much more probability that the exits occur quite quickly, before those of Yα. Similarities: in both cases, we note heavier tails as α decreases but longer for Yα. Consequently, one can conclude that the heavy-tailed FET densities come out for both processes, but, after the detailed analysis of the covariances and variances of the two processes, we can conclude that, for the time-changed process Yα, this is a consequence of the long-range dependence property, while in case of X process it is related to the increasing variance as α decreases.
The heavy-tailed distributions founded in both models, even if triggered by different causes, turn out especially useful for modeling the actin-myosin dynamics because the corresponding models can explain and predict exits (jumps) occurring after long times and also include (under suitable tilting forces) specified percentages of backward jumps. In order to confirm this last deduction, a quantitative analysis of both models by means of validation procedures on real data will be the object of a future work.
Furthermore, to validate the capability of the proposed model for modeling not only dwell times related to forward Steps of the myosin head but also to backward ones, we show in Figure 14 that for a suitable choice of the values of parameters and the tilting forces (as indicated in the caption of Figure 14) the trajectories with backward Steps (note that these sample paths also attain negative values) can also be generated for time-changed processes (left side of Figure 14) and for fractionally integrated processes (right side of Figure 14).
We propose two fractional stochastic models for the description, understanding, and prediction of the mechanism triggering muscle contraction: the actin-myosin dynamics and the corresponding dwell time. We started with a stochastic model [10] that was able to fit many experimental features and data, and we generalized such a model by means of the fractional calculus approach. The main motivation of such proposed models was born from the need to provide more refined models including some memory effects.
The first proposed model is based on a time-changed diffusion process, for which we provide some theoretical details. In particular, we are able to adapt the integral equation approach for the corresponding FET from a strip. This model is characterized by a long-range dependent covariance, and such property is due to the applied time-change that stretches, in some sense, the time.
The second fractional model is based on a fractionally integrated process, which is the solution of a Caputo-fractional stochastic differential equation for which we provide expressions for the mean and covariance function. This model has memory of its time-evolution, a consequence of the use of the fractional non-local derivative.
In order to obtain statistical estimations of the dwell time, we provide two algorithmic schemes useful to simulate these kinds of processes. Only to validate the simulation algorithms and the agreement with the theoretical settings, we perform simulations of paths of the considered processes and show how to derive statistical estimations of the corresponding dwell time pdf.
A detailed analysis of the covariances of the two proposed models is provided by means of several numerical evaluations, figures, comparisons, and comments related to the potential applications to the biological context.
For application purposes, we can conclude that the time-changed Yα model can describe biological randomly delayed dynamics, but correlated for long times, whereas the X model proves to be suitable for more impulsive reactions of muscle contractions, associated with more variability.
An ad hoc implementation of such algorithmic schemes for a detailed investigations about forward and backward Steps of the myosin head will be successively carried out. In addition, the adaptability of the proposed models and the simulation approach to real experimental data is the main purpose of our next investigation.
The authors declare they have not used Artificial Intelligence (AI) tools in the creation of this article.
This study was partially funded by the projects: PRIN-PNRR P2022XSF5H of European Union – NextGenerationEU–(MUR) Project Title “Stochastic Models in Biomathematics and Applications”; PRIN2022-MUR 2022XZSAFN; Project DAISY: “Dynamic Analysis of Interacting Biological Systems through Statistics” of the Univ. Vanvitelli (D.R. 111, 09/02/2024). Pirozzi is a member of GNCSINdaM group.
The authors thank the anonymous reviewers for their precious suggestions and greatly appreciates comments, which led to the improved revised version of this paper.
The authors declare there is no conflict of interest.
[1] |
M. O. Magnasco, G. Stolovitzky, Feynman's Ratchet and Pawl, J. Statist. Phys., 93 (1998), 615–632. https://doi.org/10.1023/B:JOSS.0000033245.43421.14 doi: 10.1023/B:JOSS.0000033245.43421.14
![]() |
[2] |
P. Reimann, Brownian motors: Noisy transport far from equilibrium, Phys. Rep., 361 (2002), 57–265. https://doi.org/10.1016/S0370-1573(01)00081-3 doi: 10.1016/S0370-1573(01)00081-3
![]() |
[3] |
H. Wang, G. Oster, Ratchets, power strokes, and molecular motors, Appl. Phys. A, 75 (2002), 315–323. https://doi.org/10.1007/s003390201340 doi: 10.1007/s003390201340
![]() |
[4] |
J. A. Spudich, How molecular motors work. Nature, 372 (1994), 515–518. https://doi.org/10.1038/372515a0 doi: 10.1038/372515a0
![]() |
[5] |
T. Masuda, Molecular dynamics simulation of a myosin subfragment-1 dockingwith an actin filament, BioSystems, 113 (2013), 144–148. https://doi.org/10.1016/j.biosystems.2013.06.001 doi: 10.1016/j.biosystems.2013.06.001
![]() |
[6] |
J. E. Molloy, J. E.Burns, J. Kendrick-Jones, R. T. Tregear, D. C. White, Movement and force produced by a single myosin head, Nature, 378 (1995), 209–212. https://doi.org/10.1038/378209a0 doi: 10.1038/378209a0
![]() |
[7] |
A. Buonocore, L. Caputo, Y. Ishii, E. Pirozzi, T. Yanagida, L. M. Ricciardi, On Myosin II dynamics in the presence of external loads, BioSystems, 81 (2005), 165–177. https://doi.org/10.1016/j.biosystems.2005.04.002 doi: 10.1016/j.biosystems.2005.04.002
![]() |
[8] |
S. Abdal, S. Hussain, I. Siddique, A. Ahmadian, M. Ferrara, On solution existence of MHD Casson nanofluid transportation across an extending cylinder through porous media and evaluation of priori bounds, Sci. Rep., 11 (2021), 7799. https://doi.org/10.1038/s41598-021-86953-1 doi: 10.1038/s41598-021-86953-1
![]() |
[9] |
K. Kitamura, M. Tokunaga, A. H. Iwane, T. Yanagida, A single myosin head moves along an actin filament with regular Steps of 5.3 nanometres, Nature, 397 (1999), 129–134. https://doi.org/10.1038/16403 doi: 10.1038/16403
![]() |
[10] |
G. D'Onofrio, E. Pirozzi, Two-boundary first exit time of Gauss-Markov processes for stochastic modeling of acto-myosin dynamics, J. Math. Biol., 74 (2017), 1511–1531. https://doi.org/10.1007/s00285-016-1061-x doi: 10.1007/s00285-016-1061-x
![]() |
[11] |
T. Masuda, A possible mechanism for determining the directionality of myosin molecular motors, Biosystems, 93 (2008), 172–180. https://doi.org/10.1016/j.biosystems.2008.03.009 doi: 10.1016/j.biosystems.2008.03.009
![]() |
[12] | M. M. Meerschaert, A. Sikorskii, Stochastic Models for Fractional Calculus, in: series De Gruyter Studies in Mathematics, 43 (2012). https://doi.org/10.1515/9783110258165 |
[13] |
D. Chalishajar, D. Geary, G. Cox, Review study of detection of diabetes models through delay differential equations, Appl. Math., 7 (2016), 1087–1102. http://dx.doi.org/10.4236/am.2016.710097 doi: 10.4236/am.2016.710097
![]() |
[14] | D. Chalishajar, A. Stanford, Mathematical Analysis of Insulin-glucose feedback system of Detection of Diabetes, Int. J. Eng. Appl. Sci., 5 (2014), 36–58. |
[15] |
S. Jain, D. N. Chalishajar, Chikungunya Transmission of Mathematical Model Using the Fractional Derivative, Symmetry, 15 (2023), 952. https://doi.org/10.3390/sym15040952 doi: 10.3390/sym15040952
![]() |
[16] |
E. Pirozzi, Some fractional stochastic models for neuronal activity with different time-scales and correlated inputs, Fract. Fract., 8 (2024), 57. https://doi.org/10.3390/fractalfract8010057 doi: 10.3390/fractalfract8010057
![]() |
[17] | S. Salahshour, A. Ahmadian, F. Ismail, D. Baleanu, N. Senu, A new fractional derivative for differential equation of fractional order under interval uncertainty, Adv. Mechan. Eng., 7 (2015). https://doi.org/10.1177/1687814015619138 |
[18] | N. Leonenko, M. M. Meerschaert, R. L. Schilling, A. Sikorskii, Correlation Structure of Time-Changed Lévy Processes, Commun. Appl. Indust. Math., (2014), e-483 https://doi.org/10.1685/journal.caim.483 |
[19] |
N. Leonenko, E. Pirozzi, First passage times for some classes of fractional time-changed diffusions, Stochast. Anal. Appl., 40 (2021), 735-763. https://doi.org/10.1080/07362994.2021.1953386 doi: 10.1080/07362994.2021.1953386
![]() |
[20] |
M. Abundo, E. Pirozzi, Fractionally integrated Gauss-Markov processes and applications, Commun. Nonlinear Sci. Numer. Simul., 101 (2021), 105862. https://doi.org/10.1016/j.cnsns.2021.105862 doi: 10.1016/j.cnsns.2021.105862
![]() |
[21] |
D. Cyranoski, Swimming against the tide, Nature, 408 (2000), 764–766. https://doi.org/10.1038/35048748 doi: 10.1038/35048748
![]() |
[22] |
F. Oosawa, The loose coupling mechanism in molecular machines of living cells, Genes Cells, 5 (2000), 9–16. https://doi.org/10.1046/j.1365-2443.2000.00304.x doi: 10.1046/j.1365-2443.2000.00304.x
![]() |
[23] |
A. Buonocore, L. Caputo, E. Pirozzi, On a pulsating Brownian motor and its characterization, Math. Biosci., 207 (2007), 387–401. https://doi.org/10.1016/j.mbs.2006.11.013 doi: 10.1016/j.mbs.2006.11.013
![]() |
[24] | A. Buonocore, L. Caputo, E. Pirozzi, L. M.Ricciardi, Simulation of Myosin II dynamics modeled by a pulsating ratchet with double-well potentials, in Lecture Notes in Computer Science, Computer Aided System Theory - EUROCAST (eds. R. Moreno-diaz, F. Pichler, A. Quesada-arencibia), Springer-Verlag, BERLIN, (2007), 154–162. https://doi.org/10.1007/978-3-540-75867-9_20 |
[25] |
M. M. Meerschaert, P. Straka, Inverse stable subordinators, Math. Model. Natural Phenom., 8 (2013), 1–16. https://doi.org/10.1051/mmnp/20138201 doi: 10.1051/mmnp/20138201
![]() |
[26] | G. Ascione, Y. Mishura, E. Pirozzi, Fractional deterministic and stochastic calculus, De Gruyter, 2024. https://doi.org/10.1515/9783110780017 |
[27] |
E. Pirozzi, Colored noise and a stochastic fractional model for correlated inputs and adaptation in neuronal firing, Biol. Cybernet., 112 (2018), 25–39. https://doi.org/10.1007/s00422-017-0731-0 doi: 10.1007/s00422-017-0731-0
![]() |
[28] | W. Teka, T. M. Marinov, F. Santamaria, Neuronal spike timing adaptation described with a fractional leaky Integrate-and-Fire model, PLoS Comput. Biol., 10 (2014). https://doi.org/10.1371/journal.pcbi.1003526 |
[29] | A. N. Kochubei, General fractional calculus, evolution equations, and renewal processes, Integr. Equ. Oper. Theory, 71 (2011), 583–600. https://doi.org/10.1007/s00020-011-1918-8 |
[30] |
B. Toaldo, Convolution-Type Derivatives, Hitting-Times of Subordinators and Time-Changed C 0-semigroups, Potential Anal, 42 (2015), 115-140. https://doi.org/10.1007/s11118-014-9426-5 doi: 10.1007/s11118-014-9426-5
![]() |
[31] | L. Arnold, Stochastic Differential Equations: Theory and Applications, Wiley-Interscience, (1974). |
[32] |
A. Buonocore, L. Caputo, E. Pirozzi, L. M. Ricciardi, The first passage time problem for Gauss-diffusion processes: Algorithmic approaches and applications to LIF neuronal model, Methodol. Comput. Appl. Probab., 13 (2011), 29–57. https://doi.org/10.1007/s11009-009-9132-8 doi: 10.1007/s11009-009-9132-8
![]() |
[33] |
E. Di Nardo, A. G. Nobile, E. Pirozzi, L. M. Ricciardi, A computational approach to first-passage-time problems for Gauss-Markov processes, Adv. Appl. Probab., 33 (2001), 453–482. https://doi.org/10.1017/S0001867800010892 doi: 10.1017/S0001867800010892
![]() |
[34] | J. Bertoin, Lévy processes, Cambridge University Press, 121 (1996). |
[35] |
N. H. Bingham, Limit theorems for occupation times of Markov processes, Z. Wahrscheinlichkeitstheorie und Verw. Gebiete., 17 (1971), 1–22. https://doi.org/10.1007/BF00538470 doi: 10.1007/BF00538470
![]() |
[36] |
L. Bondesson, G. K. Kristiansen, F. W. Steutel, Infinite divisibility of random variables and their integer parts, Stat. Probab. Letters, 28 (1996), 271–278. https://doi.org/10.1016/0167-7152(95)00135-2 doi: 10.1016/0167-7152(95)00135-2
![]() |
[37] | I. Gradshteyn, I. Ryzhik, Table of integrals, series, and products, Elsevier/Academic Press, Amsterdam, Seventh edition, (2007). |
[38] | S. Bochner, Harmonic Analysis and the Theory of Probability, University of California Press, Berkeley, (1955). https://doi.org/10.1525/9780520345294 |
[39] | F. Mainardi, G. Pagnini, R. Gorenflo, Mellin transform and subordination laws in fractional diffusion processes, Fract. Calculus Appl. Anal., 6 (2003), 441–459. |
[40] | H. Sato, Levy Processes and Infinitely Divisible Distributions, Cambridge University Press, (1999). |
[41] |
M. Hahn, J. Ryvkina, K. Kobayashi, S. Umarov, On time-changed Gaussian processes and their associated Fokker-Planck-Kolmogorov equations, Electron. Commun. Probab., 16 (2011), 150–164. https://doi.org/10.1214/ECP.v16-1620 doi: 10.1214/ECP.v16-1620
![]() |
[42] | S. Umarov, Introduction to fractional and Pseudo-differential equations with singular symbols, Developments Math., 41 (2015). https://doi.org/10.1007/978-3-319-20771-1 |
[43] |
F. Mainardi, On some properties of the Mittag-Leffler function Eα(−tα), completely monotone for t>0 with 0<α<1, Discrete Cont. Dynam. Syst. B, 19 (2014), 2267–2278. https://doi.org/10.3934/dcdsb.2014.19.2267 doi: 10.3934/dcdsb.2014.19.2267
![]() |
[44] | A. G. Nobile, E. Pirozzi, L. M. Ricciardi, On the two-boundary first-passage time for a class of Markov processes, Sci. Math. Japon., 64 (2006), 421–442. |
[45] |
E. Pirozzi, Mittag–Leffler fractional stochastic integrals and processes with applications, Mathematics, 12 (2024), 3094. https://doi.org/10.3390/math12193094 doi: 10.3390/math12193094
![]() |
[46] |
P. T. Anh, T. S. Doan, P. T. Huong, A variation of constant formula for Caputo fractional stochastic differential equations, Stat. Probab. Letters, 145 (2019), 351–358. https://doi.org/10.1016/j.spl.2018.10.010 doi: 10.1016/j.spl.2018.10.010
![]() |
[47] |
M. G. Hahn, K. Kobayashi, S. Umarov, Fokker-Planck-Kolmogorov equations associated with time-changed fractional Brownian motion, Proceed. Am. Math. Soc., 139 (2011), 691-–705. https://doi.org/10.1090/S0002-9939-2010-10527-0 doi: 10.1090/S0002-9939-2010-10527-0
![]() |
[48] |
K. Kobayashi, Stochastic calculus for a time-changed Semimartingale and the associated stochastic differential equations, J. Theor. Probab., 24 (2011), 789-820. https://doi.org/10.1007/s10959-010-0320-9 doi: 10.1007/s10959-010-0320-9
![]() |
[49] |
T. S. Doan, P. Kloeden, P. Huong, H. T. Tuan, Asymptotic separation between solutions of Caputo fractional stochastic differential equations, Stoch. Anal. Appl., 36 (2018), 654-664. https://doi.org/10.1080/07362994.2018.1440243 doi: 10.1080/07362994.2018.1440243
![]() |
[50] | K. Diethelm, The analysis of fractional differential equations, Lecture Notes in Mathematics, Springer-Verlag Berlin Heidelberg, (2010). |
[51] |
R. Garrappa, E. Kaslik, M. Popolizio, Evaluation of fractional integrals and derivatives of elementary functions: Overview and tutorial, Mathematics, 7 (2019), 407. https://doi.org/10.3390/math7050407 doi: 10.3390/math7050407
![]() |
[52] | I. Podlubny, Fractional differential equations, An introduction to fractional derivatives, fractional differential equations, to methods of their solution and some of their applications, Academic Press, Inc., San Diego, CA, (2019). |
[53] |
G. Ascione, E. Pirozzi, Generalized fractional calculus for Gompertz-Type models, Mathematics, 9 (2021), 2140. https://doi.org/10.3390/math9172140 doi: 10.3390/math9172140
![]() |
[54] | E. Jum, K. Kobayashi, A strong and weak approximation scheme for stochastic differential equations driven by a time-changed Brownian motion, Probab. Math. Statist., 36 (2016), 201-220. |
[55] |
S. X. Jin, K. Kobayashi, Strong approximation of stochastic differential equations driven by a time-changed Brownian motion with time-space-dependent coefficients, J. Math. Anal. Appl., 476 (2019), 619–636. https://doi.org/10.1016/j.jmaa.2019.04.001 doi: 10.1016/j.jmaa.2019.04.001
![]() |
[56] |
M. Magdziarz, A. Weron, K. Weron, Fractional Fokker-Planck dynamics: Stochastic representation and computer simulation, Phys. Rev. E, 75 (2007), 016708. https://doi.org/10.1103/PhysRevE.75.016708 doi: 10.1103/PhysRevE.75.016708
![]() |