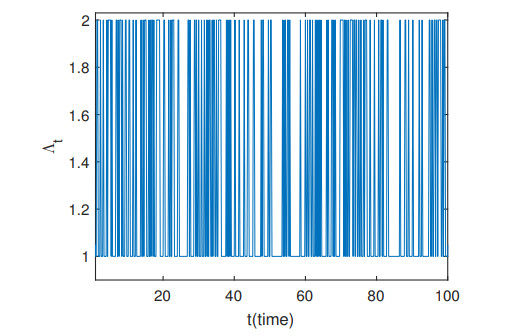
Citation: Ting Kang, Yanyan Du, Ming Ye, Qimin Zhang. Approximation of invariant measure for a stochastic population model with Markov chain and diffusion in a polluted environment[J]. Mathematical Biosciences and Engineering, 2020, 17(6): 6702-6719. doi: 10.3934/mbe.2020349
[1] | ZongWang, Qimin Zhang, Xining Li . Markovian switching for near-optimal control of a stochastic SIV epidemic model. Mathematical Biosciences and Engineering, 2019, 16(3): 1348-1375. doi: 10.3934/mbe.2019066 |
[2] | Xuehui Ji, Sanling Yuan, Tonghua Zhang, Huaiping Zhu . Stochastic modeling of algal bloom dynamics with delayed nutrient recycling. Mathematical Biosciences and Engineering, 2019, 16(1): 1-24. doi: 10.3934/mbe.2019001 |
[3] | Dongmei Li, Tana Guo, Yajing Xu . The effects of impulsive toxicant input on a single-species population in a small polluted environment. Mathematical Biosciences and Engineering, 2019, 16(6): 8179-8194. doi: 10.3934/mbe.2019413 |
[4] | Linda J. S. Allen, P. van den Driessche . Stochastic epidemic models with a backward bifurcation. Mathematical Biosciences and Engineering, 2006, 3(3): 445-458. doi: 10.3934/mbe.2006.3.445 |
[5] | Zeyan Yue, Sheng Wang . Dynamics of a stochastic hybrid delay food chain model with jumps in an impulsive polluted environment. Mathematical Biosciences and Engineering, 2024, 21(1): 186-213. doi: 10.3934/mbe.2024009 |
[6] | An Ma, Shuting Lyu, Qimin Zhang . Stationary distribution and optimal control of a stochastic population model in a polluted environment. Mathematical Biosciences and Engineering, 2022, 19(11): 11260-11280. doi: 10.3934/mbe.2022525 |
[7] | Maoxiang Wang, Fenglan Hu, Meng Xu, Zhipeng Qiu . Keep, break and breakout in food chains with two and three species. Mathematical Biosciences and Engineering, 2021, 18(1): 817-836. doi: 10.3934/mbe.2021043 |
[8] | Dawid Czapla, Sander C. Hille, Katarzyna Horbacz, Hanna Wojewódka-Ściążko . Continuous dependence of an invariant measure on the jump rate of a piecewise-deterministic Markov process. Mathematical Biosciences and Engineering, 2020, 17(2): 1059-1073. doi: 10.3934/mbe.2020056 |
[9] | H.Thomas Banks, Shuhua Hu . Nonlinear stochastic Markov processes and modeling uncertainty in populations. Mathematical Biosciences and Engineering, 2012, 9(1): 1-25. doi: 10.3934/mbe.2012.9.1 |
[10] | Linard Hoessly, Carsten Wiuf . Fast reactions with non-interacting species in stochastic reaction networks. Mathematical Biosciences and Engineering, 2022, 19(3): 2720-2749. doi: 10.3934/mbe.2022124 |
With the rapid development of industry and agriculture, the environment pollution has caused many serious ecological problems (see [1,2,3]), such as the reduction of species diversity and the extinction of some species. Therefore, it motivates many scholars' interest to study dynamic behavior of population in a polluted environment by establishing mathematical models. The population model in a polluted environment was first proposed by Hallam et al.[4,5]. From then on, more investigations and discussions on the dynamic behavior of the deterministic population model can be found (see [6,7,8,9,10]). But in practical problems, population changes are affected not only by environmental noise but also by sudden changes of temperature and climate. Thus, several scholars have introduced random perturbations into population model to study dynamic behavior. For example, Liu and Wang [11] established the stochastic population model with impulsive toxicant input and obtained sufficient conditions on extinction, persistence, stability in the mean. Subsequently, Yu et al. [12] proved the existence of global positive solution for the stochastic population model with Allee effect under regime switching and established the threshold. In [13], Wei et al. proposed a stochastic population model with partial tolerance, discussed the conditions for population the extinction and proved the stationary distribution with ergodicity by constructing the Lyapunov function. Liu et al. [14] considered the significance of white noise and color noise on population persistence and extinction and studied stochastic population model with Markov switching. More research results on the persistence, extinction, and stability of random population models and others have been presented (see [15,16,17,18,19]). However, the above mentioned references didn't consider the invariant measure of population system with diffusion.
In fact, in the real world, the population and toxins in the ecology spread around the medium such as soil and water. In addition, we also know that the existence and uniqueness of invariant measure is one of the important properties for stochastic population model with Markov switching and diffusion. Nevertheless, if we introduce diffusion into stochastic population model, the corresponding Kolmogorov-Fokker-Planck (KFP) equation will become more complicated. Furthermore, the invariant measure of stochastic population model with Markov switching and diffusion is difficult to obtain. Therefore, it is of great significance to choose an effective numerical approximation method. To the best of our knowledge, the explicit Euler-Maruyama (EM) method has the advantages of easy calculation and small calculation amount. Motivated by [21,20], in the paper, we first develop a new stochastic population model with Markov switching and diffusion. Under suitable regularity assumptions, we discuss the existence and uniqueness of numerical invariant measure generated by the EM method. Subsequently, we prove that numerical invariant measure converges to the invariant measure of exact solution in the Wasserstein distance sense. In particular, the main contributions of the paper are as follows:
● We establish a novel stochastic population model with diffusion and Markov switching in a polluted environment. By using the Chebyshev's inequality, we obtain the existence and uniqueness of invariant measure for the model.
● Under local Lipschitz conditions, we study the approximation of numerical invariant measure generated by the EM method for the newly developed model.
The structure of this article is as follows: In Section2, we introduce some necessary preliminary knowledge results for the following analysis. In Section3, based on the Perron-Frobenius theorem, we study existence and uniqueness of invariant measure for the exact solution. In Section4, we mainly study the existence and uniqueness of numerical invariant measure for the EM scheme. In addition, we also prove that the numerical invariant measure of the EM scheme converges to invariant measure of exact solution. In Section5, the numerical example is given to verify our theoretical results. In Section6, we give the conclusions of this study.
In this paper, we introduce Markov switching and spatial diffusion into the model mentioned by Liu and Wang [15], and obtain the following model
{dX1(t,x)=[k1(t,x)ΔX1(t,x)+β(t,x,X2(t,x),Λt)X1(t,x)]dt−μ(t,x,X2(t,x),Λt)X1(t,x)dt+g(t,X1(t,x),Λt)dWt,in(0,T)×Γ,dX2(t,x)=[k2(t,x)ΔX2(t,x)+K(Λt)X3(t,x)−(l(Λt)+m(Λt))X2(t,x)]dt,in(0,T)×Γ,dX3(t,x)=[k3(t,x)ΔX3(t,x)−M(Λt)X3(t,x)+u(t,x)]dt,in(0,T)×Γ,X1(0,x)=s1(x),X2(0,x)=s2(x),X3(0,x)=s3(x),inx∈Γ,X1(t,x)=0,X2(t,x)=0,X3(t,x)=0,on(0,T]×∂Γ, | (2.1) |
where L:=(0,T)×Γ, Γ is a bounded domain in R3 with smooth boundary ∂Γ, t∈(0,T); X1(t,x) denotes the population density at the location x at time t. X2(t,x) is the concentration of toxicant in the organism at time t and in spatial position x. The concentration of toxicant in the environment at the location x at time t is described by X3(t,x). K(Λt) is the net organismal uptake rate of toxicant from the environment at time t. M(Λt) is the total loss rate of the toxicant from the environment. μ(t,x,X2(t,x),Λt) denotes the decreasing rate function of the population at time t and in spatial position x. ki>0,i=1,2,3 is the diffusion coefficient. β(t,x,X2(t,x),Λt) describes the intrinsic growth rate function of the population at time t and in spatial position x. u(t,x) denotes the exogenous total toxicant input into environment at time t and in spatial position x. l(Λt) is the net organismal excretion rate of toxicant and m(Λt) is depuration rate of toxicant due to metabolic process and other losses.
Throughout the paper, Let (V,‖⋅‖) and (H,|⋅|) be two separable Hilbert spaces, with norm denoted by ‖⋅‖ and |⋅|, respectively. V is viewed as a subspace of H with a continuous dense embedding. V⋐H represents the embedding is compact. V′ and H′ are the dual of V, H. We set H3:=H×H×H. Let (Ω,F,P) be a complete probability space with {Ft}0≤t≤T the natural filtration generated by the Brownian motion Wt, which means Ft=σ{Ws;0≤s≤t} augmented with all P-null sets of F0. To construct such a filtration, we denote by N the collection of P-null sets, i.e. N={B∈F:P(B)=0}. In the paper, C>0 represents different positive constants. Let Λt, t>0, be a right-continuous Markov chain on the probability space taking values in a finite state S={1,2,…,N} for some positive integer N<∞. The generator of {Λt}t>0 is specified by Q=(qij)N×N, such that for a sufficiently small Δ,
P(Λt+Δ=j|Λt=i)={qijΔ+o(Δ),i≠j,1+qiiΔ+o(Δ),i=j, | (2.2) |
where Δ>0, o(Δ) satisfies limΔ→0o(Δ)Δ=0. Here qij is the transition rate from i to j satisfying qii=−∑i≠jqij. We assume that the Markov chain {Λt} defined on the probability space above is independent of the standard Brownian motion {Wt}t≥0 and the Q matrix is irreducible and conservative. Therefore, the Markov chain {Λt}t≥0 has a unique stationary distribution π:=(π1,…,πN) which can be determined by solving the linear equation
πQ=0subjecttoN∑i=1πi=1withπi>0. |
Let P(H3×S) stand for the family of all probability measures on H3×S. For ξ=(ξ1,ξ2,ξ3)∗∈H3, ξ≫0 means each component ξi>0, i=1,2,3.
Next, let's give some necessary assumptions:
(H1) Setting Xsk,ik,t:=Xsk,ik(t,x), k=1,2,3, there exists a positive constant ρi such that for i∈S, (t,x)∈L
‖g(t,Xs1,i1,t,i)−g(t,Xˉs1,i1,t,i)‖2≤ρi|Xs1,i1,t−Xˉs1,i1,t|2, | (2.3) |
where s1 and ˉs1 are the different initial values of the first equation for system (2.1).
From (H1), for each i∈S and Xs1,i1,t∈H, we can obtain that for (t,x)∈L
‖g(t,Xs1,i1,t,i)‖2≤C+ρi|Xs1,i1,t|2, | (2.4) |
where C depends on the initial value of the function g(t,Xs1,i1,t,i).
(H2) For each i∈S, there exist positive constants ˉM, ˉβ and ˉμ such that
{ˉM:=maxi{M(Λi)},0<ˉM<∞;0≤β(t,x,Xs2,i2,t,Λi)≤ˉβ<∞;0≤μ0≤μ(t,x,Xs2,i2,t,Λi)≤ˉμ<∞. | (2.5) |
(H3) u(t,x) is non-negative measurable in L, there exists a positive constant ˉu such that
0≤u0≤u(t,x)≤ˉu<∞. | (2.6) |
We replace ((X1,t,X2,t,X3,t),Λt) with ((Xs1,i1,t,Xs2,i2,t,Xs3,i3,t),Λit), especially the initial value
((X01,X02,X03),Λ0)=((s1,s2,s3),i). |
For any p∈(0,1], we set s:=(s1,s2,s3) and define a metric on H3×S as follows
dp((s,i),(ˉs,i)):=∫H33∑k=1|sk−ˉsk|p+I{i≠j},(s,i),(ˉs,i)∈H3×S, |
where IA denotes the indicator function of the set A, and ˉs:=(ˉs1,ˉs2,ˉs3) is different initial value. For p∈(0,1], we define the Wassertein distance between ν∈P(H3×S) and ν′∈P(H3×S) by
Wp(ν,ν′)=infEdp(Xk,Xk′), |
where the infimum is taken over all pairs of random variables Xk, Xk′ on H3×S with respective laws ν, ν′. Let Pt((s1,s2,s3),i;⋅) be the transition probability kernel of the pair ((Xs1,i1,t,Xs2,i2,t,Xs3,i3,t),Λit), a time homogeneous Markov process (see [22]). Recall that π∈P(H3×S) is called an invariant measure of ((Xs1,i1,t,Xs2,i2,t,Xs3,i3,t),Λit) if
π(A×{i})=N∑j=1∫H3Pt((s1,s2,s3),j;A×{i})π(d(s1,s2,s3)×{j}),t≥0,A∈H3,i∈S | (2.7) |
holds. For any p>0, let
diag(ρ)≜diag(ρ1,…,ρN),Qp≜Q+p2diag(ρ),ηp≜−maxγReγ. | (2.8) |
where ρi is introduced in the assumptions and γ∈spec(Qp), spec(Qp) denotes the spectrum of Qp(i.e., the multi-set of its eigenvalues). Reγ is the real part of γ and diag(ρ1,…,ρN) denotes the diagonal matrix whose diagonal entries are ρ1,…,ρN, respectively.
In this section, we mainly prove the existence and uniqueness of the invariant measure for the exact solution, under the assumption conditions (H1)–(H3). Firstly, in order to prove the existence and uniqueness of the underlying invariant measure, we prepare the following lemma.
Lemma 3.1. (see [22]) Let N<∞ and assume further that
N∑i=1μiρi<0, | (3.1) |
where μi is the stationary distribution of Markov chain {Λt}t≥0, and ρi is introduced in the assumption (H1). Then
(1) ηp>0 if maxi∈Sρi≤0;
(2) ηp>0 for p<maxi∈S,ρi>0{−2qii/ρi} if maxi∈Sρi>0.
Remark 1: The system (2.1) is said to be attractive " in average " if Eq (3.1) holds. The Lemma 3.1 provides great convenience to study the existence and uniqueness of invariant measure for exact solution, i.e., the proof of Theorem 3.1.
Theorem 3.1. Let N<∞ and assume further that (H1)–(H3) hold with maxi∈Sρi>0. Then the exact solution of system (2.1) admits a unique invariant measure π∈P(H3×S).
Proof. The key point of proof is to divide the whole proof into two parts of existence and uniqueness.
(I) Existence of invariant measure. Let ((Ys1,i1,t,Ys2,i2,t,Ys3,i3,t),Λit) be the exact solution of system (2.1) with ((s1,s2,s3),i) as initial value, where ((s1,s2,s3),i)∈H3×S. A simple application of the Feynman-Kac formula show that let Qp,t=etQp, where Qp is given in Eq (2.8). Then, the spectral radius Ria(Qp,t) (i.e., Ria(Qp,t)=supλ∈spec(Qp,t)|λ|) of Qp,t equals to e−ηpt. Since all coefficients of Qp,t are positive, by the Perron-Frobenius theorem (see [23]) yields that −ηp is a simple eigenvalue of Qp, all other eigenvalues have a strictly smaller real part. Note that the eigenvector of Qp,t corresponding to e−ηpt is also an eigenvector of Qp corresponding to −ηp. According to Perron-Frobenius theorem, for Qp it can be found that there is a positive eigenvector ξ(p)=(ξ(p)1,…,ξ(p)N)≫0 corresponding to the eigenvalue −ηp, and ξ(p)≫0 means that each component ξ(p)i>0. Let
p0=1∧mini∈S,ρi>0{−2qii/ρi}, | (3.2) |
where 1∧mini∈S,ρi>0{−2qii/ρi}:=min{1,mini∈S,ρi>0{−2qii/ρi}}. Combined with Lemma 3.1, we can get
Qpξ(p)i=−ηpξ(p)i≪0. | (3.3) |
In order to investigate the existence and uniqueness of invariant measure for exact solution, we need to prove the boundedness of exact solution for system (2.1). In other words, we need to prove whether the following inequality holds.
E(1+|Ys1,i1,t|p+|Ys2,i2,t|p+|Ys3,i3,t|p)≤C. |
First, using the Itˆo's formula (see [24], Theorem 1.45 of p.48), we can have
eηptE((1+|Ys1,i1,t|2+|Ys2,i2,t|2+|Ys3,i3,t|2)p/2ξ(p)Λit)=(1+|s1|2+|s2|2+|s3|2)p2ξpi+E∫t0eηpϵ(1+|Ys1,i1,ϵ|2+|Ys2,i2,ϵ|2+|Ys3,i3,ϵ|2)p2{ηpξ(p)Λiϵ+(Qξ(p))(Λiϵ)}dϵ+p2E∫t0eηpϵ(1+|Ys1,i1,ϵ|2+|Ys2,i2,ϵ|2+|Ys3,i3,ϵ|2)p2−1ξ(p)Λiϵ{2⟨Ys2,i2,ϵ,K(Λiϵ)Ys3,i3,ϵ−(l(Λiϵ)+m(Λiϵ))Ys2,i2,ϵ⟩+2⟨Ys1,i1,ϵ,k1ΔYs1,i1,ϵ+βYs1,i1,ϵ−μYs1,i1,ϵ⟩+2⟨Ys3,i3,ϵ,−M(Λiϵ)Ys3,i3,ϵ+uϵ+k3ΔYs3,i3,ϵ⟩+2⟨Ys2,i2,ϵ,k2ΔYs2,i2,ϵ⟩}dϵ+p2∫t0eηpϵE(1+|Ys1,i1,ϵ|2+|Ys2,i2,ϵ|2+|Ys3,i3,ϵ|2)p2−1×ξ(p)Λiϵ{(p−2)(1+|Ys1,i1,ϵ|2+|Ys2,i2,ϵ|2+|Ys3,i3,ϵ|2)−1‖Ys1,i1,ϵ∗g(ϵ,Ys1,i1,ϵ,Λiϵ)‖2+‖g(ϵ,Ys1,i1,ϵ,Λiϵ)‖2}dϵ. |
Using p(p−2)/2<0, due to p∈(0,p0), and combining with the following inequality,
∫t0∫Ωk(t,x)ΔYk,ϵYk,ϵdxdϵ=−∫t0∫Ωk(t,x)▽Yk,ϵ▽Yk,ϵdxdϵ≤−k0∫t0‖Yk,ϵ‖2dϵ,k=1,2,3 | (3.4) |
where 0≤k0≤k(t,x)<∞ (k0 is a constant). Further, we have
eηptE((1+|Ys1,i1,t|2+|Ys2,i2,t|2+|Ys3,i3,t|2)p/2ξ(p)Λit)≤(1+|s1|2+|s2|2+|s3|2)p2ξ(p)i+p2E∫t0eηpϵ(1+|Ys1,i1,ϵ|2+|Ys2,i2,ϵ|2+|Ys3,i3,ϵ|2)p2−1{2⟨Ys1,i1,ϵ,βYs1,i1,ϵ−μYs1,i1,ϵ⟩+ε1(K(Λiϵ))2|Ys2,i2,ϵ|2+1ε1|Ys3,i3,ϵ|2+2(l(Λiϵ)+m(Λiϵ))|Ys2,i2,ϵ|2+ε2|Ys3,i3,ϵ|2+1ε2|uϵ|2+2ˉM|Ys3,i3,ϵ|2}ξ(p)Λiϵdϵ+p2∫t0eηpϵE(1+|Ys1,i1,ϵ|2+|Ys2,i2,ϵ|2+|Ys3,i3,ϵ|2)p2−1‖g(s,Ys1,i1,ϵ,Λiϵ)‖2ξ(p)Λiϵdϵ+E∫t0eηpϵ(1+|Ys1,i1,ϵ|2+|Ys2,i2,ϵ|2+|Ys3,i3,ϵ|2)p2{ηpξ(p)Λiϵ+(Qξ(P))(Λiϵ)}dϵ. |
Therefore, based on assumption conditions (H1)–(H3) and the inequality 2ab≤εa2+1εb2, ε>0 we can obtain
eηptE((1+|Ys1,i1,t|2+|Ys2,i2,t|2+|Ys3,i3,t|2)p/2ξ(p)Λit)≤(1+|s1|2+|s2|2+|s3|2)p2ξ(p)i+p2E∫t0eηpϵ(1+|Ys1,i1,ϵ|2+|Ys2,i2,ϵ|2+|Ys3,i3,ϵ|2)p2−1{c+[2(ˉβ−μ0)+ρΛiϵ]|Ys1,i1,ϵ|2+[2(l(Λiϵ)+m(Λiϵ))+ε1ˉK2]|Ys2,i2,ϵ|2+[2ˉM+1ε2+1ε1]|Ys3,i3,ϵ|2+ε2|uϵ|2}dϵ+E∫t0eηpϵ(1+|Ys1,i1,ϵ|2+|Ys2,i2,ϵ|2+|Ys3,i3,ϵ|2)p2{ηpξ(p)Λiϵ+(Qξ(P))(Λiϵ)}dϵ, |
where ˉK:=maxi{K(Λi)}, for all i∈S, 0<ˉK<∞. Then, setting C1:=2(ˉβ−μ0)+ρ0, ρ0:=maxi∈S|ρΛiϵ| C2:=maxi∈S2(l(Λiϵ)+m(Λiϵ))+ε1ˉK2 and C3:=2ˉM+1ε2+1ε1, C4:=ε2+c are different constants and using the inequality
(|a|+|b|)r≤2r−1(|a|r+|b|r),r≥1,∀a,b∈R, | (3.5) |
we can further estimate
eηptE((1+|Ys1,i1,t|2+|Ys2,i2,t|2+|Ys3,i3,t|2)p/2ξ(p)Λit)≤c(1+|s1|p+|s2|p+|s3|p)+E∫t0eηpϵ(1+|Ys1,i1,ϵ|2+|Ys2,i2,ϵ|2+|Ys3,i3,ϵ|2)p2{ηpξ(p)Λis+(Qξ(P))(Λiϵ)}dϵ+p2E∫t0eηpϵ(1+|Ys1,i1,ϵ|2+|Ys2,i2,ϵ|2+|Ys3,i3,ϵ|2)p2{C1|Ys1,i1,ϵ|2+C2|Ys2,i2,ϵ|2(1+|Ys1,i1,ϵ|2+|Ys2,i2,ϵ|2+|Ys3,i3,ϵ|2)}dϵ+p2E∫t0eηpϵ(1+|Ys1,i1,ϵ|2+|Ys2,i2,ϵ|2+|Ys3,i3,ϵ|2)p2{C3|Ys3,i3,ϵ|2+C4|uϵ|2(1+|Ys1,i1,ϵ|2+|Ys2,i2,ϵ|2+|Ys3,i3,ϵ|2)}dϵ. |
Finally, by the Gronwall's lemma, we can get the result
eηptE((1+|Ys1,i1,t|2+|Ys2,i2,t|2+|Ys3,i3,t|2)p/2ξ(p)Λit)≤CeCT, | (3.6) |
and further estimates can be obtained as follows
supt≥0E((|Ys1,i1,t|p+|Ys2,i2,t|p+|Ys3,i3,t|p)≤C. | (3.7) |
For ∀ t>0, we can define a probability measure
χt(A)=1t∫t0Pϵ(s,i;A)dϵ,A∈(H3×S). |
Then, let Ys,it:=(Ys1,i1,t,Ys2,i2,t,Ys3,i3,t), for any ε>0, by Eq (3.7) and Chebyshev's inequality, there exists an r>0 sufficiently large such that
χt(Kr×S)=1t∫t0Pϵ(s,i;Kr×S)dϵ≥1−supt≥0(E|Ys,it|p)rp≥1−ε. | (3.8) |
Hence, χt is tight since the compact embedding V⋐H, then Kr={s∈H3;|s|≤r} is a compact subset of H3 (see [25], Definition 2, p.27) for each i∈S. Combined with the Fellerian property of transition seimgroup for Pt(s,i;⋅) and according to Krylov-Bogoliubov theorem (see [26]), ((Ys1,i1,t,Ys2,i2,t,Ys3,i3,t),Λit) has an invariant measure (see [27]). Next, we prove the uniqueness of the invariant measure for ((Ys1,i1,t,Ys2,i2,t,Ys3,i3,t),Λit).
(II) Uniqueness of invariant measure. First, let ((Ys1,i1,t,Ys2,i2,t,Ys3,i3,t),Λit) and ((Yˉs1,i1,t,Yˉs2,i2,t,Yˉs3,i3,t),Λit) be the solutions of the system (2.1) satisfying the initial values ((s1,s2,s3),i) and ((ˉs1,ˉs2,ˉs3),i), respectively. Under assumption conditions (H1)–(H3), we take ∀ε∈(0,1) and use Itˆo's formula, combined with Eq (3.4), we have
eηptE((ε+|Ys1,i1,t−Yˉs1,i1,t|2+|Ys2,i2,t−Yˉs2,i2,t|2+|Ys3,i3,t−Yˉs3,i3,t|2)p/2ξ(p)Λit)≤(ε+|s1−ˉs1|2+|s2−ˉs2|2|+|s3−ˉs3|2|)p/2ξ(p)i+p2E∫t0eηpϵ(ε+|Ys1,i1,ϵ−Yˉs1,i1,ϵ|2+|Ys2,i2,ϵ−Yˉs2,i2,ϵ|2+|Ys3,i3,ϵ−Yˉs3,i3,ϵ|2)p/2−1ξ(p)Λiϵ×{[2(ˉβ−μ0)+ρΛiϵ]|Ys1,i1,ϵ−Yˉs1,i1,ϵ|2+ε1ˉK2|Ys2,i2,ϵ−Yˉs2,i2,ϵ|2+[1ε1+1ε2+2ˉM]|Ys3,i3,ϵ−Yˉs3,i3,ϵ|2+2(l(Λiϵ)+m(Λiϵ))|Ys2,i2,ϵ−Yˉs2,i2,ϵ|2+ε2|us3,iϵ−uˉs3,iϵ|2}dϵ≤(ε+|s1−ˉs1|2+|s2−ˉs2|2|+|s3−ˉs3|2)p/2ξ(p)i+p2E∫t0eηpϵ(ε+|Ys1,i1,ϵ−Yˉs1,i1,ϵ|2+|Ys2,i2,ϵ−Yˉs2,i2,ϵ|2+|Ys3,i3,ϵ−Yˉs3,i3,ϵ|2)p/2ξ(p)Λiϵ×{C1|Ys1,i1,ϵ−Yˉs1,i1,ϵ|2+C2|Ys2,i2,ϵ−Yˉs2,i2,ϵ|2+C3|Ys3,i3,ϵ−Yˉs3,i3,ϵ|2+ε2|us3,iϵ−uˉs3,iϵ|2ε+|Ys1,i1,ϵ−Yˉs1,i1,ϵ|2+|Xs2,i2,ϵ−Xˉs2,i2,ϵ|2+|Xs3,i3,ϵ−Xˉs3,i3,ϵ|2}dϵ, |
where Ci, i=1,2,3 have been explained before and ρΛiϵ is introduced in the assumption (H1). In addition, using the result of Eqs (3.5) and (3.6), we can get
eηptE((ε+|Ys1,i1,t−Yˉs1,i1,t|2+|Ys2,i2,t−Yˉs2,i2,t|2+|Ys3,i3,t−Xˉs3,i3,t|2)p/2ξ(p)Λit)≤(ε+|s1−ˉs1|2+|s2−ˉs2|2+|s3−ˉs3|2)p/2ξ(p)i+p2CE∫t0eηpϵ(ε+|Ys1,i1,ϵ−Yˉs1,i1,ϵ|2+|Ys2,i2,ϵ−Xˉs2,i2,ϵ|2+|Xs3,i3,ϵ−Xˉs3,i3,ϵ|2)p/2ξ(p)Λiϵ×{1−ε(ε+|Ys1,i1,ϵ−Yˉs1,i1,ϵ|2+|Ys2,i2,ϵ−Yˉs2,i2,ϵ|2+|Xs3,i3,ϵ−Xˉs3,i3,ϵ|2)−1}dϵ≤(ε+|s1−ˉs1|2+|s2−ˉs2|2|+|s3−ˉs3|2)p/2ξ(p)i+Cεp/2eηpt, | (3.9) |
when ε→0, we can get the following result
E(|Ys1,i1,t−Yˉs1,i1,t|p+|Ys2,i2,t−Yˉs2,i2,t|p+|Ys3,i3,t−Yˉs3,i3,t|p)≤C(|s1−ˉs1|p+|s2−ˉs2|p+|s3−ˉs3|p)e−ηpt. | (3.10) |
Define the stopping time
τ=inf{t≥0:Λit=Λjt}. |
According to the definition of S and irreducibility of Q, there exists θ>0 such that
P(τ>t)≤e−θt,t>0. | (3.11) |
Due to p∈(0,p0), and choose q>1 such that 0<pq<p0, where p0 is introduced in Eq (3.2). Using H¨older's inequality, we can have
E(|Ys1,i1,t−Yˉs1,j1,t|p+|Ys2,i2,t−Yˉs2,j2,t|p+|Ys3,i3,t−Yˉs3,j3,t|p)=E(|Ys1,i1,t−Yˉs1,j1,t|p1{τ>t/2})+E(|Ys1,i1,t−Yˉs1,j1,t|p1{τ≤t/2})+E(|Ys2,i2,t−Yˉs2,j2,t|p1{τ>t/2})+E(|Ys2,i2,t−Yˉs2,j2,t|p1{τ≤t/2})+E(|Ys3,i3,t−Yˉs3,j3,t|p1{τ>t/2})+E(|Ys3,i3,t−Yˉs3,j3,t|p1{τ≤t/2})≤(E|Ys1,i1,t−Yˉs1,j1,t|pq1{τ>t/2})1/q(P(τ>t/2))1/p+E(1{τ≤t/2}E|YYs1,i1,τ,Λiτ1,t−τ−YYˉs1,j1,τ,Λjτ1,t−τ|p)+(E|Ys2,i2,t−Yˉs2,j2,t|pq1{τ>t/2})1/q(P(τ>t/2))1/p+E(1{τ≤t/2}E|YYs2,i2,τ,Λiτ2,t−τ−YYˉs2,j2,τ,Λjτ2,t−τ|p)+(E|Ys3,i3,t−Yˉs1,j3,t|pq1{τ>t/2})1/q(P(τ>t/2))1/p+E(1{τ≤t/2}E|YYs3,i3,τ,Λiτ3,t−τ−YYˉs3,j1,τ,Λjτ3,t−τ|p). | (3.12) |
Applying the result of Eq (3.11), we further obtain
E(|Ys1,i1,t−Yˉs1,j1,t|p+|Ys2,i2,t−Yˉs2,j2,t|p+|Ys3,i3,t−Yˉs3,j3,t|p)≤e−q−12qθt(E|Ys1,i1,t−Yˉs1,j1,t|pq)1q+CE(1{τ≤t/2}e−ηp(t−τ)E|Ys1,i1,τ−Yˉs1,j1,τ|p)+e−q−12qθt(E|Ys2,i2,t−Yˉs2,j2,t|pq)1q+CE(1{τ≤t/2}e−ηp(t−τ)E|Ys2,i2,τ−Yˉs2,j2,τ|p)+e−q−12qθt(E|Ys3,i3,t−Yˉs3,j3,t|pq)1q+CE(1{τ≤t/2}e−ηp(t−τ)E|Ys3,i3,τ−Xˉs3,j3,τ|p)≤e−q−12qθt(E|Ys1,i1,t−Yˉs1,j1,t|pq)1q+Ce−ηp2tE|Ys1,i1,τ−Y¯s1,j1,τ|p+e−q−12qθt(E|Ys2,i2,t−Yˉs2,j2,t|pq)1q+Ce−ηp2tE|Ys2,i2,τ−Y¯s2,j2,τ|p+e−q−12qθt(E|Ys3,i3,t−Yˉs3,j3,t|pq)1/q+Ce−ηp2tE|Ys3,i3,τ−Y¯s3,j3,τ|p≤C(1+|s1|p+|ˉs1|p+|s2|p+|ˉs2|p+|s3|p+|ˉs3|p)e−σt, | (3.13) |
where σ:=(q−1)θ2q∧ηp2, and in the last step, it follows from Eqs (3.7) and (3.10) such that
supt≥0E(|Ys1,i1,t|pq+|Ys2,i2,t|pq+|Ys3,i3,t|pq)≤C, |
and
supt≥0E(|Yˉs1,j1,t|pq+|Yˉs2,j2,t|pq+|Yˉs3,j3,t|pq)≤C. |
Thus, we also have assertion
limt→∞E(|Ys1,i1,t−Yˉs1,j1,t|p+|Ys2,i2,t−Yˉs2,j2,t|p+|Ys3,i3,t−Yˉs3,j3,t|p)=0. |
Then, according to Eq (3.11), we can get
P(Λit≠Λjt)=P(τ>t)≤e−θtt>0. | (3.14) |
Next, according to Eqs (3.14) and (3.13) that
Wp(δ((s1,s2,s2),i)Pt,δ((ˉs1,ˉs2,ˉs3),j)Pt)≤E(|Ys1,i1,t−Yˉs1,j1,t|p+|Ys2,i2,t−Yˉs2,j2,t|p+|Ys3,i3,t−Yˉs3,j3,t|p)+P(Λit≠Λjt)≤C(1+|s1|p+|ˉs1|p+|s2|p+|ˉs2|p+|s3|p+|ˉs3|p)e−σt+e−θt≤Ce−σ∗t, | (3.15) |
where σ∗:=σ∧θ. Assume π, ν∈P(H3×S) are invariant measures of ((Ys1,i1,t,Ys2,i2,t,Ys3,i3,t),Λit), it follows from Eq (3.15) that
Wp(π,ν)=Wp(πPt,νPt)≤N∑i,j=1∫H3×S∫H3×Sπ(d(s1,s2,s3)×{i})ν(d(ˉs1,ˉs2,ˉs3)×{j})Wp(δ((s1,s2,s3),i)Pt,δ((ˉs1,ˉs2,ˉs3),j)Pt). |
When t→∞, we find Wp(π,ν)→0. Hence, uniqueness of invariant measure follows immediately. The proof of Theorem 3.1 has been completed.
In the following section, we will investigate existence and uniqueness of numerical invariant measure and prove the convergence of numerical invariant measure.
In this section, we mainly discuss existence and uniqueness of numerical invariant measure for system (2.1) under the assumption conditions (H1)–(H3). In order to facilitate the discussion, we consider the numerical solution in the discrete-time for system (2.1). For a given step size δ∈(0,1), we define the discrete-time Euler-Maruyama (EM) scheme associated with model (2.1) as follows
{ˉXs1,i1,(n+1)δ=ˉXs1,i1,nδ+[k1(nδ,x)ΔˉXs1,i1,nδ+β(nδ,x,ˉXs2,i2,nδ,Λinδ)ˉXs1,i1,nδ]δ−μ(nδ,x,ˉXs2,i2,nδ,Λinδ)ˉXs1,i1,nδδ+g(nδ,ˉXs1,i1,nδ,Λinδ)ΔWn,ˉXs2,i2,(n+1)δ=ˉXs2,i2,nδ+[k2(nδ,x)ΔˉXs2,i2,nδ+K(Λinδ)ˉXs3,i3,nδ−(l(Λinδ)+m(Λinδ))ˉXs2,i2,nδ]δ,ˉXs3,i3,(n+1)δ=ˉXs3,i3,nδ+[k3(nδ,x)ΔˉXs3,i3,nδ−M(Λinδ)ˉXs3,i3,nδ+u(nδ,x)]δ, | (4.1) |
where n≥0 and ΔWn≜W(n+1)δ−Wnδ denotes Brownian motion increment, ΔˉXsk,ik,nδ is the Laplace of ˉXsk,ik,nδ, with the initial data ((ˉX01,ˉX02,ˉX03),Λ0)=((s1,s2,s3),i)∈H3×S which is introduced before. Equations (4.1) and (4.2) are the discrete-time EM scheme and continuous-time EM scheme of the corresponding system (2.1), respectively. For convenience, we define the corresponding approximate solution to the system (2.1) on continuous time.
{Xs1,i1,t=s1+∫t0[k1(⌊ϵ/δ⌋δ,x)ΔˉXs1,i1,⌊ϵ/δ⌋δ+β(⌊ϵ/δ⌋δ,x,ˉXs2,i2,⌊ϵ/δ⌋δ,Λi⌊ϵ/δ⌋δ)ˉXs1,i1,⌊ϵ/δ⌋δ]dϵ−∫t0μ(⌊ϵ/δ⌋δ,x,ˉXs2,i2,⌊ϵ/δ⌋δ,Λi⌊ϵ/δ⌋δ)ˉXs1,i1,⌊ϵ/δ⌋δdϵ+∫t0g(⌊ϵ/δ⌋δ,ˉXs1,i1,⌊ϵ/δ⌋δ,Λi⌊ϵ/δ⌋δ)dWϵ,Xs2,i2,t=s2+∫t0[k2(⌊ϵ/δ⌋δ,x)ΔˉXs2,i2,⌊ϵ/δ⌋δ+K(Λi⌊ϵ/δ⌋δ)ˉXs3,i3,⌊ϵ/δ⌋δ]dϵ−∫t0(l(Λi⌊ϵ/δ⌋δ)+m(Λi⌊ϵ/δ⌋δ))ˉXs2,i2,⌊ϵ/δ⌋δdϵ,Xs3,i3,t=s3+∫t0[k3(⌊ϵ/δ⌋δ,x)ΔˉXs3,i3,⌊ϵ/δ⌋δ−M(Λi⌊ϵ/δ⌋δ)ˉXs3,i3,⌊ϵ/δ⌋δ+u(⌊ϵ/δ⌋δ,x)]dϵ, | (4.2) |
where t>0,Λi0=i∈S, ∀b≥0, ⌊b⌋ is the interger part of b. Obviously, by a straightforward calculation, we can have (Xs1,i1,⌊ϵ/δ⌋δ,Xs2,i2,⌊ϵ/δ⌋δ,Xs3,i3,⌊ϵ/δ⌋δ)=(ˉXs1,i1,⌊ϵ/δ⌋δ,ˉXs2,i2,⌊ϵ/δ⌋δ,ˉXs3,i3,⌊ϵ/δ⌋δ).
Let Pδnδ((s1,s2,s3),j;⋅) be the transition probability kernel of ((ˉXs1,i1,nδ,ˉXs2,i2,nδ,ˉXs3,i3,nδ),Λinδ). If πδ∈P(H3×S) satisfies the following equation
πδ(A×{i})=N∑j=1∫H3Pδnδ((s1,s2,s3),j;A×{i})πδ(d(s1,s2,s3)×{j}),t≥0,A∈H3,i∈S, | (4.3) |
then we call πδ∈P(H3×S) an invariant measure of ((ˉXs1,i1,nδ,ˉXs2,i2,nδ,ˉXs3,i3,nδ),Λinδ) or a numerical invariant measure of ((Xs1,i1,t,Xs2,i2,t,Xs3,i3,t),Λit). Let
q0:=maxi∈S(−qii),ρ0=maxi∈S|ρi|,^ξ0≜maxi∈Sξ(p)i,˘ξ0≜(maxi∈Sξ(p)i)−1. |
Our main result in this section is as follows
Lemma 2. Under the conditions of Lemma 3.1 and combining Eq (3.2) with (3.3), it holds that
E(|ˉXs1,i1,nδ−ˉXˉs1,j1,nδ|p+|ˉXs2,i2,nδ−ˉXˉs2,j2,nδ|p+|ˉXs3,i3,nδ−ˉXˉs3,j3,nδ|p)≤C(1+|s1|p+|s2|p+|s3|p+|ˉs1|p+|ˉs2|p+|ˉs3|p)e−ηpnδ, | (4.4) |
for any p∈(0,p0), (s,i)=((s1,s2,s3),i), (ˉs,j)=((ˉs1,ˉs2,ˉs3),j)∈H3×S. p0 is given in Eq (3.2).
Lemma 4.1 shows that numerical solution (ˉXs1,i1,nδ,ˉXs2,i2,nδ,ˉXs3,i3,nδ) tends to (ˉXˉs1,j1,nδ,ˉXˉs2,j2,nδ,ˉXˉs3,j3,nδ) when n→∞ and δ→0 under different initial values and states. This lemma provides a great convenience for the proof of Theorem 4.1. Applying a method similar to Theorem 3.1 can prove the conclusion of Lemma 4.1, so it is omitted.
Theorem 4.1. Under the conditions of Theorem 3.1, there exists a sufficiently small δ∗ such that for any δ∈(0,δ∗), the solutions of the EM method (4.2) converge to a unique invariant measure πδ∈P(H3×S) with some exponential rate ˉγ>0 in the Wassertein distance.
Proof. In fact, for any the initial data (s1,s2,s3), by Eq (4.2) and the Chebyshev's inequality, we derive that {δ(s1,s2,s3)Pδnδ} is tight. Therefore, there exists an exact subsequence which converges weakly to an invariant measure denoted by πδ∈P(H3×S). According to the Eq (3.14), we have the following result
P(Λinδ≠Λjnδ)=P(τδ>n)≤e−θnδ. | (4.5) |
For any n>0, combining with Eq (4.4), it is not difficult to get
Wp(δ((s1,s2,s2),i)Pδnδ,δ((ˉs1,ˉs2,ˉs3),j)Pδnδ)≤E(|ˉXs1,i1,nδ−ˉXˉs1,j1,nδ|p+|ˉXs2,i2,nδ−ˉXˉs2,j2,nδ|p+|ˉXs3,i3,nδ−ˉXˉs3,j3,nδ|p)+P(Λinδ≠Λjnδ)≤C(1+|s1|p+|s2|p+|s3|p+|ˉs1|p+|ˉs2|p+|ˉs3|p)e−ˉγnδ, | (4.6) |
where ˉγ:=ϱ∧θ, and using the Kolmogorov-Chapman equation and Eq (4.6), for any n,m>0, we have
Wp(δ((s1,s2,s2),i)Pδnδ,δ((s1,s2,s2),i)Pδ(n+m)δ)=Wp(δ((s1,s2,s2),i)Pδnδ,δ((s1,s2,s2),i)PδnδPδmδ)≤∫H3×SWp(δ((s1,s2,s2),i)Pδnδ,δ((ˉs1,ˉs2,ˉs3),j)Pδnδ)Pδmδ((s1,s2,s2),i;d(ˉs1,ˉs2,ˉs3),j)≤∑j∈S∫H3C(1+|s1|p+|s2|p+|s3|p+|ˉs1|p+|ˉs2|p+|ˉs3|p)e−ˉγnδH1≤Ce−ˉγnδ, | (4.7) |
where H1=Pδmδ((s1,s2,s2),i;d(ˉs1,ˉs2,ˉs3),j), then taking m→∞ such that
Wp(δ((s1,s2,s2),i)Pδnδ,πδ)→0,n→∞, | (4.8) |
in other words, πδ is the unique invariant measure of {δ(s1,s2,s3)Pδnδ}. ∀πδ,νδ∈P(H3×S) are invariant measures of ((ˉXs1,i1,nδ,ˉXs2,i2,nδ,ˉXs3,i3,nδ),Λinδ) and ((ˉXˉs1,j1,nδ,ˉXˉs2,j2,nδ,ˉXˉs3,j3,nδ),Λjnδ), respectively. Further, we have
Wp(πδ,νδ)=Wp(πδPδnδ,νδPδnδ)≤N∑i,j=1∫H3×S∫H3×Sπδ(d(s1,s2,s3)×{i})νδ(d(ˉs1,ˉs2,ˉs3)×{j})Wp(δ((s1,s2,s3),i)Pδnδ,δ((ˉs1,ˉs2,ˉs3),j)Pδnδ). | (4.9) |
The uniqueness for the numerical invariant measure have been completed. Therefore, the proof of Theorem 4.1 is complete. To show that the numerical invariant measure πδ converges to the invariant measure of the corresponding exact solution under the Wasserstein distance, the following theorem is given.
Theorem 4.2. Under the assumptions of Theorem 4.1 and Eq (4.8), for δ∈(0,1) there exists C>0 such that
Wp(π,πδ)≤Cδp2,p∈(0,p0), |
where p0>0 is defined in Eq (3.2).
Proof. For p∈(0,p0), due to
Wp(δ((s1,s2,s2),i)Pnδ,π)≤∫H3×Sπ(d(ˉs1,ˉs2,ˉs3)×{j})Wp(δ((s1,s2,s2),i)Pδnδ,δ((ˉs1,ˉs2,ˉs3),j)Pδnδ), |
and
Wp(δ((s1,s2,s2),i)Pδnδ,πδ)≤∫H3×Sπ(d(ˉs1,ˉs2,ˉs3)×{j})Wp(δ((s1,s2,s2),i)Pδnδ,δ((ˉs1,ˉs2,ˉs3),j)Pδnδ). |
Then based on the assumption conditions of (H1)–(H3) and Eq (4.8), there exists a sufficiently small δ∗ such that for any δ∈(0,δ∗), there is n>0 sufficiently large such that
Wp(δ((s1,s2,s2),i)Pnδ,π)+Wp(δ((s1,s2,s2),i)Pδnδ,πδ)≤Cδp2, | (4.10) |
For fixed n>0 and using the triangle inequality, and by the similar way of [22], we can obtain limδ→0Wp(δ((s1,s2,s2),i)Pnδ,δ((s1,s2,s2),i)Pδnδ)=0. In other words, there exists a positive constant ˉν such that Wp(δ((s1,s2,s2),i)Pnδ,δ((s1,s2,s2),i)Pδnδ)≤Ceˉνδnδp2. According to Theorem 3.1 and Eq (4.8), we can get the following result
Wp(δ((s1,s2,s2),i)Pnδ,π)+Wp(δ((s1,s2,s2),i)Pδnδ,πδ)≤Ce−γ∗nδ, | (4.11) |
where γ∗:=σ∗∧ˉγ. Let ˉC be the integer part of constant −plnδ/[2(ˉν+γ∗)δ], obviously, ˉC→0 as δ→0. On the other hand, we have eˉνˉCδδp2≤δpσ∗2(ˉν+γ∗)≤δp2, e−σ∗ˉCδ≤eγ∗δ∗δp2. Therefore, Wp(π,πδ)≤Cδp2 holds.
Further, to illustrate the validity of our theory which are discussed in the previous section, we will give a numerical example.
Let Λt be a Markov chain with the state space S={1,2}, and the generator
Γ=(3−3−44). |
It is easy to show that its unique stationary distribution π=(π1,π2) is given by π1=1/2, π1=1/2. On the other hand, we give the following setting: V(Λt):=l(Λt)+m(Λt), when Λt=1, we choose M(1)=12exp(21+2t), K(1)=0.01sin(1(3+0.2t)2) and V(1)=l(1)+m(1)=1.99; when Λt=2, we choose M(2)=910(11+t), K(2)=0.05sin(1(3+0.2t)2) and V(2)=l(2)+m(2)=1.6. In the state 1 and 2, setting T=1, t∈(0,1), β:=β(t,x,X2(t,x),Λt)=12(1−0.5X2(t,x)0.5(1+X2(t,x)))(1−x5+x), μ:=μ(t,x,X2(t,x),Λt)=310(0.5−0.8X2(t,x)1+0.5X2(t,x))(1−x0.5+x), g:=g(t,x,X1(t,x),Λt)=0.05+0.3X1(t,x), and taking k1=0.005, k2=k3=0.05, s1(x)=s2(x)=0.2(1+x)2, s3(x)=0.2(1+1.5x)2, the system (2.1) is described as follows
{dX1(t,x)=[0.005ΔX1(t,x)+βX1(t,x)−μX1(t,x)]dt+gdWt,in(0,T)×Γ,dX2(t,x)=[0.05ΔX2(t,x)+K(Λt)X3(t,x)−V(Λt)X2(t,x)]dt,in(0,T)×Γ,dX3(t,x)=[0.05ΔX3(t,x)−M(Λt)X3(t,x)+u(t,x)]dt,in(0,T)×Γ,X1(0,x)=X2(0,x)=0.2(1+x)2,X3(0,x)=0.2(1+1.5x)2,inx∈Γ,X1(t,x)=0,X2(t,x)=0,X3(t,x)=0,on(0,T]×∂Γ, | (5.1) |
First, for the system (5.1), we use the discrete-time EM method for numerical simulation. Figure 1 is a simulation of Markov chain which describes switching between different states.
Then, taking T=1, N=100, |Wi+1−Wi|=√δ and t∈(0,1), step sizes δ=0.005. Among them, the values of X3(t,x) and X2(t,x) do not exceed 0.4. This satisfies the practical significance, i.e., 0≤X2(t,x)≤1, 0≤X3(t,x)≤1.
As far as we know, the exact solution for system (5.1) is difficult to find. Inspired by [30] and based on the method of [28], we can take the "explicit solution" Y1(t,x)=exp(12−11−x−t22)(1+ΔW), Y2(t,x)=K∫t0Y3(t,x)exp{(l+m)(s−t)}ds+CY2exp{−(l+m)t} and Y3(t,x)=∫t0u(t,x)exp{h(s−t)}ds+CY3exp{−ht} replace exact solution, where CY2,CY3 are initial values of Y2 and Y3, respectively. Setting CY3=0.2(1+1.5x)2, CY2=0.2(1+x)2, K=0.05, l+m=1.9 and h=0.5, u(t,x)=15(4(1+2x)2−12)(1−t)3. Then, The simulation results are presented separately in Figure 2(a), Figure 4(a) and Figure 6(a). In Figure 6(b) and Figure 4(b) reflect the numerical simulation of X3(t,x) and X2(t,x) with Markov switching when the step size is 0.005 under the state "1" and "2" switching.
In addition, Figure 7, Figure 5 and Figure 3 show mean-square error between "explicit solutions Y3, Y2 and Y1" and the corresponding numerical solutions X3, X2 and X1(Figure 6(b), Figure 4(b) and Figure 2(b)) of stochastic population with diffusion and Markov switching in a polluted environment system (5.1), when we take step sizes δ=0.005,0.0001. Obviously, when the step size δ changes from 0.005 to 0.0001, the error values decreases from 0.14, 0.4 and 0.04 to 0.012, 0.025 and 0.02, respectively. Combining Figure 7, Figure 5 and Figure 3, we have the assertion that the smaller the step size, the smaller the error. Hence, it is not difficult to conclude that when δ→0, the numerical solution X3(t,x), X2(t,x), X1(t,x) under discrete-time EM method converges to the explicit solution Y3(t,x), Y2(t,x), Y1(t,x), respectively.
In this paper, we establish a new stochastic population model with Markov chain and diffusion in a polluted environment. Based on the Perron-Frobenius theorem, when the diffusion coefficient satisfies the local Lipschitz, the criterion on the existence and uniqueness of invariant measure for the exact solution is given. Moreover, we also discuss the existence and uniqueness of numerical invariance measure for model (2.1) under the discrete-time Euler-Maruyama scheme, and prove that numerical invariance measure converges to invariance measure of the corresponding exact solution in the Wasserstein distance sense. At the end of this paper, the accuracy of the theoretical results is verified by numerical simulation.
The authors are very grateful to the anonymous reviewers for their insightful comments and helpful suggestions. The research was supported by the Natural Science Foundation of China (Grant numbers 11661064). This research was funded by the "Major Innovation Projects for Building First-class Universities in China's Western Region" (ZKZD2017009).
The authors declare there is no conflict of interest.
[1] | L. Duan, Q. Lu, Z. Yang, L. Chen, Effects of diffusion on a stage-structured population in a polluted environment, Appl. Math. Comput., 02 (2004), 347-359. |
[2] | A. J. Shaw, Ecological genetics of plant populations in polluted environment. Ecological Genetics and Air Pollution, Springer New York, 1991. |
[3] |
G. P. Samanta, A. Maiti, Dynamical model of a single-species system in a polluted environment, J. Appl. Mathe. Comput., 16 (2004), 231-242. doi: 10.1007/BF02936164
![]() |
[4] |
T. G. Hallam, C. E. Clark, R. R. Lassiter, Effects of toxicants on populations: a qualitative approach I. Equilibrium environmental exposure, Ecol. Model., 18 (1983), 291-304. doi: 10.1016/0304-3800(83)90019-4
![]() |
[5] | T. G. Hallam, C. E. Clar, G. S. Jordan, Effects of toxicant on population: a qualitative approach II. First Order Kinetics, J. Math. Biol., 109 (1983), 411-429. |
[6] | D. Mukherjee, Persistence and global stability of a population in a polluted environment with delay, J. Biol. Syst., 10 (2008), 225-232. |
[7] | Z. Ma, G. Cui, W. Wang, Persistence and extinction of a population in a polluted environment, Math. Biosci., 101 (2004), 75-97. |
[8] |
T. G. Hallam, Z. Ma, Persistence in Population models with demographic fluctuations, J. Math. Biol., 24 (1986), 327-339. doi: 10.1007/BF00275641
![]() |
[9] |
J. Pan, Z. Jin, Z. Ma, Thresholds of survival for an n-dimensional Volterra mutualistic system in a polluted environment, J. Math. Anal. Appl., 252 (2000), 519-531. doi: 10.1006/jmaa.2000.6853
![]() |
[10] |
Z. Ma, B. J. Song, T. G. Hallam, The threshold of survival for systems in a fluctuating environment, Bull. Math. Biol., 51 (1989), 311-323. doi: 10.1016/S0092-8240(89)80078-3
![]() |
[11] | M. Liu, K. Wang, Persistence and extinction of a stochastic single-species population model in a polluted environment with impulsive toxicant input, Electron. J. Differ. Equ., 230 (2013), 823-840. |
[12] |
X. Yu, S. Yuan, T. Zhang, Persistence and ergodicity of a stochastic single species model with Allee effect under regime switching, Commun. Nonlinear Sci. Numer. Simul., 59 (2018), 359-374. doi: 10.1016/j.cnsns.2017.11.028
![]() |
[13] |
F. Wei, S. A. H. Geritz, J. Cai, A stochastic single-species population model with partial pollution tolerance in a polluted environment, Appl. Math. Lett., 63 (2017), 130-136. doi: 10.1016/j.aml.2016.07.026
![]() |
[14] | M. Liu, K. Wang, Persistence and extinction of a stochastic single-specie model under regime switching in a polluted environment. J. Theor. Biol., 267 (2010), 283-291. |
[15] | M. Liu, K. Wang, Survival analysis of a stochastic single-species population model with jumps in a polluted environment, Int. J. Biomath., 09 (2016), 1-15. |
[16] |
Y. Zhao, S. Yuan, Q. Zhang, The effect of Lévy noise on the survival of a stochastic competitive model in an impulsive polluted environment, Appl. Math. Model., 40 (2016), 7583-7600. doi: 10.1016/j.apm.2016.01.056
![]() |
[17] |
T. C. Gard, Stability for multi-species population models in random environments, Nonlinear Anal., 10 (1986), 1411-1419. doi: 10.1016/0362-546X(86)90111-2
![]() |
[18] | J. Tong, Z. Zhang, J. Bao, The stationary distribution of the facultative population model with a degenerate noise, Discrete Continuous Dyn. Syst., 83 (2013), 655-664. |
[19] |
M. Liu, K. Wang, Y. Wang, Long term behaviors of stochastic single-species growth models in a polluted environment, Appl. Math. Model., 35 (2011), 4438-4448. doi: 10.1016/j.apm.2011.03.014
![]() |
[20] |
C. Yuan, X. Mao, Stationary distributions of Euler-Maruyama-type stochastic difference equations with Markovian switching and their convergence, J. Differ. Equ. Appl., 11 (2005), 29-48. doi: 10.1080/10236190412331314150
![]() |
[21] |
G. Yin, X. Mao, K. Yin, Numerical approximation of invariant measures for hybrid diffusion systems, IEEE Trans. Automat. Contr., 50 (2005), 934-946. doi: 10.1109/TAC.2005.851437
![]() |
[22] |
J. Bao, J. Shao, C. Yuan, Approximation of invariant measures for regime-switching diffusions, Potential Anal., 44 (2016), 707-727. doi: 10.1007/s11118-015-9526-x
![]() |
[23] |
H. Yang, X. Li, Explicit approximations for nonlinear switching diffusion systems in finite and infinite horizons, J. Differ. Equ., 265 (2018), 2921-2967. doi: 10.1016/j.jde.2018.04.052
![]() |
[24] | X. Mao, C. Yuan, Stochastic differential equations with Markovian switching, Imperial College Press, 2006. |
[25] |
G. Dhariwal, A. Jungel, N. Zamponi, Global martingale solutions for a stochastic population crossdiffusion system, Stoch. Process. their Appl., 129 (2019), 3792-3820. doi: 10.1016/j.spa.2018.11.001
![]() |
[26] | G. Da Prato, J. Zabczyk, Ergodicity for infinite dimensional systems, Cambridge University Press, Cambridge, 1996. |
[27] | G. Yin, C. Zhu, Hybrid swithching diffusion: Properties and Applications, Springer, 2010. |
[28] | Y. Zhao, S. Yuan, Q. Zhang, Numerical solution of a fuzzy stochastic single-species age-structure model in a polluted environment, Appl. Math. Comput., 260 (2015), 385-396. |
[29] | W J. Anderson, Continuous-time Markov chains, Springer, Berlin, 1991. |
[30] | J. Tan, A. Rathinasamy, Y. Pei, Convergence of the split-step θ-method for stochastic agedependent population equations with Poisson jumps, Elsevier Science Inc, 254 (2015), 305-317. |
1. | An Ma, Qimin Zhang, Global attractor and threshold dynamics of a reaction–diffusion population model in a polluted environment, 2023, 69, 1598-5865, 989, 10.1007/s12190-022-01781-4 | |
2. | An Ma, Shuting Lyu, Qimin Zhang, Stationary distribution and optimal control of a stochastic population model in a polluted environment, 2022, 19, 1551-0018, 11260, 10.3934/mbe.2022525 | |
3. | An Ma, Jing Hu, Qimin Zhang, DYNAMIC ANALYSIS AND OPTIMAL CONTROL OF A TOXICANT-POPULATION MODEL WITH REACTION-DIFFUSION, 2024, 14, 2156-907X, 579, 10.11948/20210438 | |
4. | An Ma, Jing Hu, Ming Ye, Qimin Zhang, Investigation of sliding mode dynamics and near-optimal controls for a reaction–diffusion population model in a polluted environment, 2024, 79, 09473580, 101097, 10.1016/j.ejcon.2024.101097 |