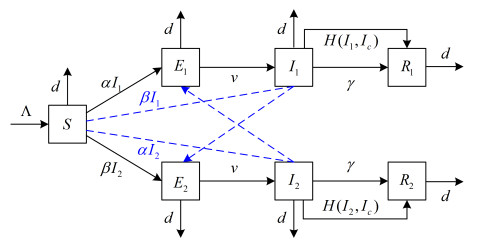
In this paper, the dynamic behaviors and control strategies of a rumor propagation model are studied in multi-lingual environment. First, an S2E2I2R rumor propagation model is proposed, which incorporates a non-smooth inhibition mechanism. Meanwhile, the existence and stability of the equilibrium are analyzed, grounded in the spreader threshold of the government intervention. Finally, the optimal control and the event-triggered impulsive control strategies are proposed to mitigate the spread of rumors, and the comparison of their effectiveness is further presented by the numerical simulation and a practical case.
Citation: Jing Liao, Jinling Wang, Jiarong Li, Xin Jiang. The dynamics and control of a multi-lingual rumor propagation model with non-smooth inhibition mechanism[J]. Mathematical Biosciences and Engineering, 2024, 21(4): 5068-5091. doi: 10.3934/mbe.2024224
[1] | Ying Yu, Jiaomin Liu, Jiadong Ren, Cuiyi Xiao . Stability analysis and optimal control of a rumor propagation model based on two communication modes: friends and marketing account pushing. Mathematical Biosciences and Engineering, 2022, 19(5): 4407-4428. doi: 10.3934/mbe.2022204 |
[2] | Yi Wang, Shicheng Zhong, Guo Wang . Dynamic selection of clarification channels in rumor propagation containment. Mathematical Biosciences and Engineering, 2023, 20(8): 14995-15017. doi: 10.3934/mbe.2023672 |
[3] | Yilin Tu, Jin-E Zhang . Event-triggered impulsive control for input-to-state stability of nonlinear time-delay system with delayed impulse. Mathematical Biosciences and Engineering, 2025, 22(4): 876-896. doi: 10.3934/mbe.2025031 |
[4] | Chenxi Huang, Qianqian Zhang, Sanyi Tang . Non-smooth dynamics of a SIR model with nonlinear state-dependent impulsive control. Mathematical Biosciences and Engineering, 2023, 20(10): 18861-18887. doi: 10.3934/mbe.2023835 |
[5] | Siyu Li, Shu Li, Lei Liu . Fuzzy adaptive event-triggered distributed control for a class of nonlinear multi-agent systems. Mathematical Biosciences and Engineering, 2024, 21(1): 474-493. doi: 10.3934/mbe.2024021 |
[6] | Liang Tian, Fengjun Shang, Chenquan Gan . Optimal control analysis of malware propagation in cloud environments. Mathematical Biosciences and Engineering, 2023, 20(8): 14502-14517. doi: 10.3934/mbe.2023649 |
[7] | Wenjing Wang, Jingjing Dong, Dong Xu, Zhilian Yan, Jianping Zhou . Synchronization control of time-delay neural networks via event-triggered non-fragile cost-guaranteed control. Mathematical Biosciences and Engineering, 2023, 20(1): 52-75. doi: 10.3934/mbe.2023004 |
[8] | Mingxia Gu, Zhiyong Yu, Haijun Jiang, Da Huang . Distributed consensus of discrete time-varying linear multi-agent systems with event-triggered intermittent control. Mathematical Biosciences and Engineering, 2024, 21(1): 415-443. doi: 10.3934/mbe.2024019 |
[9] | Na Zhang, Jianwei Xia, Tianjiao Liu, Chengyuan Yan, Xiao Wang . Dynamic event-triggered adaptive finite-time consensus control for multi-agent systems with time-varying actuator faults. Mathematical Biosciences and Engineering, 2023, 20(5): 7761-7783. doi: 10.3934/mbe.2023335 |
[10] | Nasim Ullah, Al-sharef Mohammad . Cascaded robust control of mechanical ventilator using fractional order sliding mode control. Mathematical Biosciences and Engineering, 2022, 19(2): 1332-1354. doi: 10.3934/mbe.2022061 |
In this paper, the dynamic behaviors and control strategies of a rumor propagation model are studied in multi-lingual environment. First, an S2E2I2R rumor propagation model is proposed, which incorporates a non-smooth inhibition mechanism. Meanwhile, the existence and stability of the equilibrium are analyzed, grounded in the spreader threshold of the government intervention. Finally, the optimal control and the event-triggered impulsive control strategies are proposed to mitigate the spread of rumors, and the comparison of their effectiveness is further presented by the numerical simulation and a practical case.
With the rapid development of science and technology, the arrival of the era of self-media has been promoted, and a communication pattern in which everyone has a microphone in today's society has been formed. While the public has gained an unprecedented right to speak and spread, the Internet has become a hotbed for the breeding and dissemination of rumors. In particular, the vigorous development of social media has made it possible for everyone to freely publish, disseminate, and obtain information, which has facilitated the spread of rumors and resulted extremely bad social impacts. For example, during the outbreak of COVID-19, rumors such as prevention methods and epidemic dynamics emerged in an endless stream, which has caused many hazards to public physical and mental health and social stability. Therefore, exploring the rules and characteristics of rumor propagation plays an important role in formulating rumor control strategies.
Since the rumor propagation process is similar to the spread of infectious diseases in the population, most infectious disease theories can be used to study the spread of rumors [1,2,3]. The study of rumor propagation models began in the 1860s. Daley and Kendal [4] proposed the DK model considering the difference between rumors and infectious disease transmission. Based on the DK model, Maki and Thompson [5] established the MT model by changing the propagation mechanism. Later, many rumor propagation models were derived. Among them, SI [6], SIS [7], SIR [1,2], and SEIR [8] are the classical models for studying the dynamics of rumor propagation. However, with the in-depth study of rumor propagation, many scholars believe that the establishment of a rumor propagation model on complex networks can intuitively describe the propagation process of rumors [9,10,11]. Subsequently, the psychological factors [12], behavioral performance [13], and reaction time [14,15] of the crowd were taken into account in the rumor propagation model.
However, the above research work mainly considers the spread of rumors in a single language environment. In fact, the actual spread process of rumors is more complicated, and there are cases of spreading through multiple languages. Therefore, Wang et al. [16] considered the SIR rumor propagation model with a cross-propagation mechanism in the bilingual environment, and analyzed its dynamic behaviors. Furthermore, Li et al. [17] established and analyzed the I2S2R rumor propagation model in complex networks. At present, the rumor propagation models established in multi-lingual environment are not comprehensive enough. In this paper, the exposed (E) will be introduced into the multi-lingual environment to establish the S2E2I2R model.
In addition, to effectively control the spread of rumors, more and more scholars have studied the impact of external control measures on the spread of rumors, such as, trust mechanism [18], feedback mechanism [19], and education mechanism [17]. Most of the above models considered control measures with linear or smooth functions, but there were few studies on rumor propagation with nonlinear non-smooth inhibition mechanism [20]. Before the rumor is widely spread on the Internet, the government and media will not intervene, so there is a spreader threshold to trigger the intervention mechanism, which is more in line with the actual situation. Therefore, we will introduce a non-smooth inhibition mechanism to analyze the process of multi-lingual rumor propagation.
In the process of rumor control, the implementation of control strategies requires a certain cost. In order to control the number of rumor spreaders within a certain range with less cost, many scholars use optimal control methods to study rumor spread control strategies [21,22]. In practical applications, it is very difficult to implement real-time optimal control of the rumor propagation process. As a powerful discontinuous control method, impulsive control only needs to control the system at some discrete sampling points, thus greatly reducing the control cost [23,24]. Therefore, it is of great practical value for us to study the event-triggered impulsive control strategy.
Motivated by the above analysis, this paper proposes an S2E2I2R model with non-smooth inhibition mechanism in multi-lingual environment. The main works of this paper include the following aspects:
1) A rumor propagation model with non-smooth inhibition mechanism is established, and the jump discontinuity points divide the model into two stages, which are consistent with reality.
2) Taking the spreader threshold Ic as the boundary, the existence and stability of the rumor-free equilibrium and rumor-spreading equilibrium are analyzed here.
3) Two different control strategies, optimal control and event-triggered impulsive control, are designed, and the advantages of these control strategies are fully illustrated by comparing different control strategies in the numerical simulation.
The rest of the paper is organized as follows: In Section 2, the model formulation is presented. In terms of the spreader threshold Ic, the stability of an S2E2I2R rumor propagation model is explored in Section 3. In Section 4, both the optimal control and event-triggered impulsive control are designed to restrain the rumor's diffusion. In Section 5, numerical simulation is further addressed to display their validity. Finally, conclusions are given in Section 6.
In fact, network users can come from different regions and have diverse languages. Once a rumor is released, it can quickly spread via various languages. Besides, government and other institutions can control rumors, but when the number of rumor spreaders is below a certain threshold, it might not be essential to implement control measures. Hence, a non-smooth inhibition mechanism is proposed to establish the multi-lingual rumor propagation model (see Figure 1).
Assume that there are two languages in the networks, and the network users N(t) can be divided into seven categories: ignorants S(t) (users who do not know rumors but are easily infected), exposed Ei(t) (users who have been infected, in hesitation state and do not spread rumor), spreaders Ii(t) (users who master two languages, and use language i to spread rumors), and stiflers Ri(t) (users who identify rumors and do not spread rumors), i=1,2.
Remark 1. When rumors reach a critical level of seriousness, government and official media intervention become necessary [18,20,25]. Therefore, the phased control measures align better with the actual situation. Therefore, a non-smooth inhibition function H(Ii,Ic)(i=1,2) for multi-lingual system is proposed below:
H(Ii,Ic)={0,0≤Ii≤Ic,δ(Ii−Ic)1+ξ(I1+I2),Ii>Ic. | (2.1) |
Based on the above analysis and Figure 1, the S2E2I2R rumor propagation model can be expressed as
{dS(t)dt=Λ−(α+β)⟨k⟩S(t)(I1(t)+I2(t))−dS(t),dE1(t)dt=α⟨k⟩S(t)(I1(t)+I2(t))−(d+v)E1(t),dE2(t)dt=β⟨k⟩S(t)(I1(t)+I2(t))−(d+v)E2(t),dI1(t)dt=vE1(t)−(d+γ)I1(t)−H(I1,Ic),dI2(t)dt=vE2(t)−(d+γ)I2(t)−H(I2,Ic),dR1(t)dt=H(I1,Ic)+γI1(t)−dR1(t),dR2(t)dt=H(I2,Ic)+γI2(t)−dR2(t), | (2.2) |
where parameters are shown in Table 1, and the initial condition is
S(0)>0,Ei(0)>0,Ii(0)>0,Ri(0)>0, i=1,2. | (2.3) |
Parameter | Definition |
⟨k⟩ | Average degree of homogeneous network |
Λ | The immigration rate of S(t) per unit time |
d | The removal rate of users per unit time |
α | The probability of S(t) contact Ii(t) and become E1(t) |
β | The probability of S(t) contact Ii(t) and become E2(t) |
v | The transformation rate from Ei(t) to Ii(t) |
γ | The transformation rate from Ii(t) to Ri(t) |
Ic | The spreader threshold of government and media intervention |
δ | The control rate of spreaders under government and media intervention |
ξ | The effectiveness of inhibition mechanism |
In order to make the model have realistic significance, we first give the nonnegativity and boundedness of all solutions of system (2.2) before analyzing the stability.
Lemma 1. Under the initial conditions (2.3), the solutions of system (2.2) are positive for t>0, and a positively invariant set of system (2.2) is
Ω={(S(t),Ei(t),Ii(t),Ri(t))∈R7+|S(t)+2∑i=1[Ei(t)+Ii(t)+Ri(t)]≤Λd}, i=1,2. |
Proof. Let
q(t)=mint{S(t),Ei(t),Ii(t),Ri(t), i=1,2}. |
To prove that the solution of system (2.2) is positive, we only need to prove q(t)>0 for any t>0. By using reduction to absurdity, assume that there exists t1>0 such that q(t1)=0, q(t)>0 for t∈[0,t1). Subsequently, we need to discuss seven cases. First, if q(t1)=S(t1), then S(t1)=0, Ei(t1)>0,Ii(t1)>0,Ri(t1)>0, and q(t)>0 for t∈[0,t1). According to system (2.2), it yields ˙S(t1)=Λ>0, which contradicts S(t)>0=S(t1) for t∈[0,t1). Moreover, if q(t1)=R1(t1), then
˙R1(t1)={γI1(t1)>0,0≤I1(t1)≤Ic,H(I1(t1),Ic)+γI1(t1)>0,I1(t1)≥Ic, |
which is a contradiction to the fact that R1(t)>0=R1(t1),t∈[0,t1). Further, the other cases can be discussed similarly. Hence, we have S(t)>0,Ei(t)>0,Ii(t)>0, and Ri(t)>0 for any t>0.
Next, let
N(t)=S(t)+E1(t)+E2(t)+I1(t)+I2(t)+R1(t)+R2(t). |
According to system (2.2), we have
dN(t)dt=Λ−dN(t), |
and it follows that
limt→+∞supN(t)≤Λd. |
Therefore, the positive invariant set of system (2.2) can be obtained.
Since the first five equations of the model are independent of Ri(t), we only consider the following system in the later discussion.
{dS(t)dt=Λ−(α+β)⟨k⟩S(t)(I1(t)+I2(t))−dS(t),dE1(t)dt=α⟨k⟩S(t)(I1(t)+I2(t))−(d+v)E1(t),dE2(t)dt=β⟨k⟩S(t)(I1(t)+I2(t))−(d+v)E2(t),dI1(t)dt=vE1(t)−(d+γ)I1(t)−H(I1,Ic),dI2(t)dt=vE2(t)−(d+γ)I2(t)−H(I2,Ic). | (2.4) |
In this section, the next generation matrix method is used to calculate the basic reproduction number R0 of the system, and the stability of the rumor-free equilibrium is discussed.
Let X=(E1(t),E2(t),I1(t),I2(t),S(t))T, then system (2.4) can be written as
dXdt=F(X)−V(X), |
where
F(X)=(α⟨k⟩S(t)(I1(t)+I2(t))β⟨k⟩S(t)(I1(t)+I2(t))000), |
V(X)=((d+v)E1(t)(d+v)E2(t)−vE1(t)+(d+r)I1(t)+H(I1,Ic)−vE2(t)+(d+r)I2(t)+H(I2,Ic)−Λ+(α+β)⟨k⟩S(t)(I1(t)+I2(t))+dS(t)). |
Since the spreader threshold Ic divides the system into two stages, we discuss the existence and stability of the equilibrium in the case of 0≤Ii≤Ic and Ii>Ic, respectively.
Case 1. 0≤Ii≤Ic
When 0≤Ii≤Ic, we have H(Ii,Ic)=0. Obviously, for system (2.4) there exists a rumor-free equilibrium E0=(0,0,0,0,Λd). The Jacobian matrices of F(X) and V(X) at E0 are
DF(E0)=(F000),DV(E0)=(V0J1J2), |
where
F=(00α⟨k⟩Λdα⟨k⟩Λd00β⟨k⟩Λdβ⟨k⟩Λd00000000),V=(d+v0000d+v00−v0d+r00−v0d+r), |
and J1=(00(α+β)⟨k⟩Λd(α+β)⟨k⟩Λd),J2=d. According to the next generation matrix method, it follows that
R0=ρ(FV−1)=(α+β)⟨k⟩Λvd(d+γ)(d+v). |
Theorem 1. If R0<1, the rumor-free equilibrium E0 of system (2.4) is locally asymptotically stable for 0≤Ii≤Ic.
Proof. The Jacobian matrix of system (2.4) at the rumor-free equilibrium E0 is
J(E0)=(−(d+v)0α⟨k⟩Λdα⟨k⟩Λd00−(d+v)β⟨k⟩Λdβ⟨k⟩Λd0v0−(d+γ)000v0−(d+γ)000−(α+β)⟨k⟩Λd−(α+β)⟨k⟩Λd−d). |
By calculation, the characteristic equation is
(λ+d)(λ+d+γ)(λ+d+v)[λ2+(2d+γ+v)λ+(d+γ)(d+v)(1−R0)]=0. |
Hence, the characteristic roots are given as λ1=−d<0,λ2=−(d+γ)<0, and λ3=−(d+v)<0, and the rest of the characteristic roots are determined by
λ2+(2d+γ+v)λ+(d+γ)(d+v)(1−R0)=0. | (3.1) |
According to the Routh-Hurwitz criterion, if R0<1, all roots of Eq (3.1) have negative real parts. Therefore, the rumor-free equilibrium E0 of system (2.4) is locally asymptotically stable.
Theorem 2. If R0<1, the rumor-free equilibrium E0 of system (2.4) is globally asymptotically stable for 0≤Ii≤Ic.
Proof. Consider the following Lyapunov function
V(t)=E1(t)+E2(t)+(α+β)⟨k⟩Λd(d+γ)(I1(t)+I2(t)). |
By calculating the derivative of V(t) along system (2.4), we can obtain
dV(t)dt=(α+β)⟨k⟩S(t)(I1(t)+I2(t))−(d+v)(E1(t)+E2(t))+(α+β)⟨k⟩Λd(d+γ)[v(E1(t)+E2(t))−(d+γ)(I1(t)+I2(t))]≤(α+β)⟨k⟩Λd(I1(t)+I2(t))+(R0−1)(d+v)(E1(t)+E2(t))−(α+β)⟨k⟩Λd(I1(t)+I2(t))=(R0−1)(d+v)(E1(t)+E2(t)). |
If R0<1, we have dV(t)dt≤0. In addition, dV(t)dt=0 if and only if E1(t)=E2(t)=0.
According to LaSalle's invariance principle, the rumor-free equilibrium E0 of system (2.4) is globally asymptotically stable.
Remark 2. Note that if 0≤Ii≤Ic, the rumor-free equilibrium E0 of system (2.4) is locally and globally asymptotically stable with R0<1 from the above theorem. Meanwhile, when Ii>Ic, the system has no rumor-free equilibrium E0 since the density of spreaders is always greater than the spread threshold Ic. Hence, government and media intervention can adjust Ic for dissemination via the information credibility, thereby reducing the impact and speed of rumor propagation.
The existence and stability of the rumor-spreading equilibrium E∗=(E∗1,E∗2,I∗1,I∗2,S∗) in system (2.4) are further discussed under the spreader threshold Ic below.
Case 1. 0≤Ii≤Ic
Theorem 3. If R0>1, system (2.4) has a unique rumor-spreading equilibrium E∗ for 0≤Ii≤Ic.
Proof. Let η=I∗1+I∗2≥0 and substitute it into system (2.4). We have
S∗=Λ(α+β)⟨k⟩η+d, E∗1=α⟨k⟩ηS∗d+v, E∗2=β⟨k⟩ηS∗d+v. |
From further calculation, one can obtain
I∗1+I∗2=v(E∗1+E∗2)d+γ=(α+β)⟨k⟩Λvη(d+γ)(d+v)[(α+β)⟨k⟩η+d]=η. |
Construct the following function
f(η)=1−(α+β)⟨k⟩Λv(d+γ)(d+v)[(α+β)⟨k⟩η+d]. |
Since ˙f(η)>0 and limη→+∞f(η)=1, it yields that f(η)=0 has a unique solution if and only if f(0)=1−R0<0. Hence, when 0≤Ii≤Ic, system (2.4) has a unique rumor-spreading equilibrium E∗ with R0<1.
Theorem 4. Suppose P=(α+β)⟨k⟩(I∗1+I∗2)+dd, if 1<R0<P, then the rumor-spreading equilibrium E∗ of system (2.4) is locally asymptotically stable for 0≤Ii≤Ic.
Proof. The Jacobian matrix of the linearized system of system (2.4) at the rumor-spreading equilibrium E∗ is
J(E∗)=(−(d+v)0α⟨k⟩S∗α⟨k⟩S∗α⟨k⟩η0−(d+v)β⟨k⟩S∗β⟨k⟩S∗β⟨k⟩ηv0−(d+γ)000v0−(d+γ)000−(α+β)⟨k⟩S∗ −(α+β)⟨k⟩S∗ −(α+β)⟨k⟩η−d). |
By calculation, the characteristic equation of J(E∗) is
(λ+d+v)(λ+d+γ)[λ3+A2(η)λ2+A1(η)λ+A0(η)]=0, |
where
A2(η)=2d+v+γ+(α+β)⟨k⟩η+d>0,A1(η)=(d+v)(d+γ)[1−R0d(α+β)⟨k⟩η+d]+(2d+v+γ)[(α+β)⟨k⟩η+d],A0(η)=(d+v)(d+γ)[(α+β)⟨k⟩η+d)][1−R0d2((α+β)⟨k⟩η+d)2]. |
Obviously, it yields λ1=−(d+v)<0,λ2=−(d+γ)<0, and the rest of the characteristic roots are determined by
λ3+A2(η)λ2+A1(η)λ+A0(η)=0. | (3.2) |
In fact, if 1<R0<(α+β)⟨k⟩(I∗1+I∗2)+dd, it follows that A1(η)>0,A0(η)>0. Ulteriorly, one has
A2(η)A1(η)−A0(η)=(2d+v+γ)[(α+β)⟨k⟩η+d]2+(α+β)⟨k⟩Λvd(α+β)⟨k⟩η+d+(2d+v+γ)2[(α+β)⟨k⟩η+d]−(α+β)⟨k⟩Λv+(2d+v+γ)(d+v)(d+γ)[1−R0d(α+β)⟨k⟩η+d]>(2d+v+γ)[(α+β)⟨k⟩η+d]2+(α+β)⟨k⟩Λvd(α+β)⟨k⟩η+d+(d+v)(d+γ)[(α+β)⟨k⟩η+d][1−R0d(α+β)⟨k⟩η+d]+(2d+v+γ)(d+v)(d+γ)[1−R0d(α+β)⟨k⟩η+d]>0. |
According to the Routh-Hurwitz criterion, all roots of Eq (3.2) have negative real parts. Therefore, the rumor-spreading equilibrium E∗ of system (2.4) is locally asymptotically stable.
Case 2. Ii>Ic
Similar to the case of 0≤Ii≤Ic, the cubic equation for η by calculation is present as
g(η)=B3η3+B2η2+B1η+B0, |
where
B3= (d+v)(d+γ)(α+β)⟨k⟩ξ>0,B2= (d+v)(α+β)⟨k⟩δ+(d+v)(d+γ)(α+β)⟨k⟩+d(d+v)(d+γ)ξ−(α+β)⟨k⟩Λvξ,B1= d(d+v)δ+d(d+v)(d+γ)−2(d+v)(α+β)⟨k⟩δIc−(α+β)⟨k⟩Λv,B0=−2d(d+v)δIc<0. |
For convenience, let Δ=4B22−12B3B1, η11,η12(η11<η12) be two roots of g′(η), and η1–η3 be three roots of g(η), then we can acquire following conclusion:
(H1) Suppose Δ>0, if B2>0,B1>0 (or B2>0,B1<0 or B2<0,B1<0) holds, then the system (2.4) has a unique positive equilibrium.
(H2) Suppose Δ≤0, then the system (2.4) has a unique positive equilibrium.
Theorem 5. Under condition (H1) or (H2), let
Θ=(α+β)⟨k⟩Λvd(d+v)(d+γ+ˆH∗12+ˆH∗22)<P, |
then the rumor-spreading equilibrium E∗ of system (2.4) is locally asymptotically stable for Ii>Ic.
Proof. The Jacobian matrix of the linearized system of system (2.4) at the rumor-spreading equilibrium E∗ is
J(E∗)=(−(d+v)0α⟨k⟩S∗α⟨k⟩S∗α⟨k⟩η0−(d+v)β⟨k⟩S∗β⟨k⟩S∗β⟨k⟩ηv0−(d+γ)−ˆH∗11−ˆH∗1200v−ˆH∗21−(d+γ)−ˆH∗22000−(α+β)⟨k⟩S∗ −(α+β)⟨k⟩S∗ −(α+β)⟨k⟩η−d), |
where ˆH∗ij(i,j=1,2) is the value of ∂H(Ii,Ic)∂Ij at E∗.
By calculation, the characteristic equation of J(E∗) is
(λ+d+v)(λ+d+γ+ˆH∗11−ˆH∗12)[λ3+C2(η)λ2+C1(η)λ+C0(η)]=0, | (3.3) |
where
C2(η)= 2d+v+γ+ˆH∗12+ˆH∗22+(α+β)⟨k⟩η+d>0, |
C1(η)= d(d+v)(d+γ+ˆH∗12+ˆH∗22)(α+β)⟨k⟩η+d[(α+β)⟨k⟩η+dd−Θ]+(2d+v+γ+ˆH∗12+ˆH∗22)[(α+β)⟨k⟩η+d], |
C0(η)= d2(d+v)(d+γ+ˆH∗12+ˆH∗22)(α+β)⟨k⟩η+d[[(α+β)⟨k⟩η+d]2d2−Θ]. |
Similarly, note that if Θ<P, then the eigenvalues of J(E∗) have negative real parts. Therefore, the rumor-spreading equilibrium E∗ of system (2.4) is locally asymptotically stable.
Remark 3. In fact, Theorem 5 only discusses the stability of system (2.4) when it has a unique positive equilibrium. However, when Δ>0, and B2<0,B1>0,g(η11)>0,g(η12)<0, the system (2.4) has three positive equilibrium points. Besides, when Δ>0, the system (2.4) has two positive equilibrium points with B2<0,B1>0,g(η11)=0,g(η12)<0 or B2<0,B1>0,g(η11)>0,g(η12)=0. Regarding the issue of multiple equilibria, we will conduct further research in future.
In order to further curb the spread of rumors while simultaneously minimizing the associated control costs, an optimal control rooted in a non-smooth inhibition mechanism is proposed in this section. Now, let the control set U={(u1(t),u2(t))∣0≤u1(t)≤umax1,0≤u2(t)≤umax2,t∈[0,T]}, where umax1∈(0,1] and umax2∈(0,1] are the upper bounds of u1(t) and u1(t), and the optimal control system is
{dS(t)dt=Λ−(α+β)⟨k⟩S(t)(I1(t)+I2(t))−dS(t),dE1(t)dt=α⟨k⟩S(t)(I1(t)+I2(t))−(d+v)E1(t),dE2(t)dt=β⟨k⟩S(t)(I1(t)+I2(t))−(d+v)E2(t),dI1(t)dt=vE1(t)−(d+γ+u1(t))I1(t)−H(I1,Ic),dI2(t)dt=vE2(t)−(d+γ+u2(t))I2(t)−H(I2,Ic),dR1(t)dt=H(I1,Ic)+(γ+u1(t))I1(t)−dR1(t),dR2(t)dt=H(I2,Ic)+(γ+u2(t))I2(t)−dR2(t), | (4.1) |
where u1(t) and u2(t) represent the degree of control over the spreader, and the control system (4.1) satisfies the initial conditions. In order to describe the relationship between control cost and spreads, we use θi and ϕi(i=1,2) to denote the weight coefficients between I1(t), I2(t) and the degree of control, respectively. Assume that the termination time is T and the objective function is
J(u1(t),u2(t))=∫T0θ1I1(t)+θ2I2(t)+ϕ1u21(t)+ϕ2u22(t)dt. | (4.2) |
Moreover, the optimal controls u∗1(t) and u∗2(t) satisfy
J(u∗1(t),u∗2(t))=min{J(u1(t),u2(t)):(u1(t),u2(t))∈U}. |
To further describe the optimization problem, the Lagrange function is defined as
L(I1(t),I2(t),u1(t),u2(t))=θ1I1(t)+θ2I2(t)+ϕ1u21(t)+ϕ2u22(t), |
and the Hamiltonian function is
H(S(t),Ei(t),Ii(t),Ri(t),ui(t),λj(t))=L(Ii(t),ui(t))+λT(t)f(S(t),Ei(t),Ii(t),Ri(t)), |
where i=1,2,j=1,2,⋯, 7, and λ(t) denotes the adjoint function. According to the Pontryagin's maximum principle, the following conclusions can be obtained.
Theorem 6. Let (ˉS,ˉE1,ˉE2,ˉI1,ˉI2,ˉR1,ˉR2) be the optimal state solution of system (4.1) under the optimal control (u∗1(t),u∗2(t)). Then, the adjoint variables satisfy the equation
{dλ1(t)dt=α⟨k⟩(ˉI1(t)+ˉI2(t))(λ1(t)−λ2(t))+β⟨k⟩(ˉI1(t)+ˉI2(t))(λ1(t)−λ3(t))+dλ1(t),dλ2(t)dt=(d+v)λ2(t)−vλ4(t),dλ3(t)dt=(d+v)λ3(t)−vλ5(t),dλ4(t)dt=−θ1+α⟨k⟩ˉS(t)(λ1(t)−λ2(t))+β⟨k⟩ˉS(t)(λ1(t)−λ3(t))+dλ4(t)+(γ+u1(t)+ˆH11)(λ4(t)−λ6(t))+ˆH21(λ5(t)−λ7(t)),dλ5(t)dt=−θ2+α⟨k⟩ˉS(t)(λ1(t)−λ2(t))+β⟨k⟩ˉS(t)(λ1(t)−λ3(t))+dλ5(t)+(γ+u2(t)+ˆH22)(λ5(t)−λ7(t))+ˆH12(λ4(t)−λ6(t)),dλ6(t)dt=dλ6(t),dλ7(t)dt=dλ7(t), |
with transversal conditions λj(T)=0, j=1,2,⋯,7, and the optimal controls u∗1(t) and u∗2(t) are
u∗1(t)=min{max{(λ4(t)−λ6(t))ˉI1(t)2ϕ1,0},umax1}, |
u∗2(t)=min{max{(λ5(t)−λ7(t))ˉI2(t)2ϕ2,0},umax2}. | (4.3) |
Proof. According to the Pontryagin's maximum principle, let
S(t)=ˉS, Ei(t)=ˉEi, Ii(t)=ˉIi, Ri(t)=ˉRi. |
Under the transversal condition, it follows that
{dλ1(t)dt=−∂H∂S(t), dλ2(t)dt=−∂H∂E1(t), dλ3(t)dt=−∂H∂E2(t),dλ4(t)dt=−∂H∂I1(t), dλ5(t)dt=−∂H∂I2(t), dλ6(t)dt=−∂H∂R1(t), dλ7(t)dt=−∂H∂R2(t), |
and from the optimal control condition
∂H(t)∂u1(t)|u1(t)=u∗1(t)=2ϕ1u∗1(t)−ˉI1(t)λ4(t)+ˉI1(t)λ6(t)=0,∂H(t)∂u2(t)|u2(t)=u∗2(t)=2ϕ2u∗2(t)−ˉI2(t)λ5(t)+ˉI2(t)λ7(t)=0. | (4.4) |
Then, we can obtain
u∗1(t)=(λ4(t)−λ6(t))ˉI1(t)2ϕ1, u∗2(t)=(λ5(t)−λ7(t))ˉI2(t)2ϕ2. |
Hence, combined with the range of control set U, u∗1(t) and u∗2(t) of (4.1) can be acquired here.
In practical applications, continuous control of rumor propagation, while feasible, is resource-intensive. However, impulsive control, as a potent discontinuous control method, offers a solution by necessitating control interventions only at discrete sampling points. Ulteriorly, in order to optimize resource utilization and cost-effectively intervene in rumor dissemination when necessary, this paper adopts event-triggered impulsive control to suppress the spread of rumors.
The event-triggered impulsive control system is shown as follows.
For t∈[tk−1,tk),
{dS(t)dt=Λ−(α+β)⟨k⟩S(t)(I1(t)+I2(t))−dS(t),dE1(t)dt=α⟨k⟩S(t)(I1(t)+I2(t))−(d+v)E1(t),dE2(t)dt=β⟨k⟩S(t)(I1(t)+I2(t))−(d+v)E2(t),dI1(t)dt=vE1(t)−(d+γ)I1(t),dI2(t)dt=vE2(t)−(d+γ)I2(t),dR1(t)dt=γI1(t)−dR1(t),dR2(t)dt=γI2(t)−dR2(t), | (4.5) |
and for t=tk,
{S(t+k)=S(t−k),E1(t+k)=(1−εμ)E1(t−k),E2(t+k)=(1−εμ)E2(t−k),I1(t+k)=(1−εμ)I1(t−k),I2(t+k)=(1−εμ)I2(t−k),R1(t+k)=εμ(I1(t+k)+E1(t−k))+R1(t−k),R2(t+k)=εμ(I2(t+k)+E2(t−k))+R2(t−k). | (4.6) |
Let A(t)=(E1(t),E2(t),I1(t),I2(t))T, and construct the following auxiliary variables
{B(t)=−μA(t),t∈[tk−1,tk),ΔA(t)=εB(t),t=tk, |
where B(t) indicates the reduction of the exposed and spreader in [tk−1,tk) compared to the previous time period due to the control, and ΔA(t)=A(t+k)−A(t−k),A(tk)=A(t+k). tk is the impulsive sequence which satisfies 0=t0<t1<⋯<tk<⋯, limk→∞tk=∞. Moreover, ε∈(0,1) and μ∈(0,1) represent impulsive strength and control strength, respectively.
Next, define the following event-triggered function
h(x)=‖ | (4.7) |
where a > 0 , b > 0 , e(t) = B({t_k}) - B(t) , and the k+1 -th impulsive time is {t_{k + 1}} = \inf \left\{ {t|h(t) \ge 0, t > {t_k}} \right\} .
Theorem 7. For the event-triggered impulsive control system (4.5), if there is a positive definite matrix U such that
\begin{equation} \mathop {\lim }\limits_{t \to \infty } \left[ {k\ln (1 - \varepsilon \mu ) + \frac{{k + 1}}{2}\ln (\frac{{{\lambda _{\max }}(U)}}{{{\lambda _{\min }}(U)}}) + \frac{{{\lambda _{\max }}(\varphi )}}{{2{\lambda _{\min }}(U)}}(t - {t_0})} \right] \to - \infty , \end{equation} | (4.8) |
where \varphi = KU + {U^T}K , then \mathop {\lim }\limits_{t \to \infty } \left\| {A(t)} \right\| = 0 , that is, the rumor will disappear.
Proof. For t \in \left[ {{t_k}, {t_{k + 1}}} \right) , we study the equation
\frac{{\mathrm{d}A(t)}}{{\mathrm{d}t}} = KA(t), |
where
K = \left( {\begin{array}{*{20}{c}} { - (d + v)}&0&{\alpha \langle k \rangle S(t)}&{\alpha \langle k \rangle S(t)}\\ 0&{ - (d + v)}&{\beta \langle k \rangle S(t)}&{\beta \langle k \rangle S(t)}\\ v&0&{ - (d + \gamma )}&0\\ 0&v&0&{ - (d + \gamma )} \end{array}} \right). |
Since S(t) \le \frac{\Lambda }{d} , S(t) can be replaced by \frac{\Lambda }{d} . Now, construct the Lyapunov function
V(t) = {A^T}(t)UA(t), |
where U is a positive definite matrix. The derivative of V(t) is
\dot V(t) = {A^T}(t)(KU + {U^T}K)A(t) \le {\lambda _{\max }}(\varphi ){A^T}(t)A(t), |
where {\lambda _{\max }}(\varphi) denotes the maximum eigenvalue of \varphi = KU + {U^T}K .
For t \in \left[ {{t_0}, {t_1}} \right) , based on Gronwall's inequality [26], one has
\begin{align*} {\left\| {A(t)} \right\|^2} \le &\ \frac{1}{{{\lambda _{\min }}(U)}}[V({t_0}) + \int_{{t_0}}^t {{\lambda _{\max }}(\varphi )} {\left\| {A(s)} \right\|^2}\mathrm{d}s]\\ \le &\ \frac{{{\lambda _{\max }}(U)}}{{{\lambda _{\min }}(U)}}{\left\| {A({t_0})} \right\|^2}\exp \left\{ {\frac{{{\lambda _{\max }}(\varphi )}}{{{\lambda _{\min }}(U)}}(t - {t_0})} \right\}. \end{align*} |
When t = {t_1} , it can yield that
{\left\| {A({t_1})} \right\|^2} \le {(1 - \varepsilon \mu )^2}\frac{{{\lambda _{\max }}(U)}}{{{\lambda _{\min }}(U)}}{\left\| {A({t_0})} \right\|^2}\exp \left\{ {\frac{{{\lambda _{\max }}(\varphi )}}{{{\lambda _{\min }}(U)}}(t - {t_0})} \right\}. |
Based on mathematical induction, for any t \in \left[ {{t_k}, {t_{k + 1}}} \right) , the following inequality holds.
{\left\| {A(t)} \right\|^2} \le {(1 - \varepsilon \mu )^{2k}}{(\frac{{{\lambda _{\max }}(U)}}{{{\lambda _{\min }}(U)}})^{k + 1}}{\left\| {A({t_0})} \right\|^2}\exp \left\{ {\frac{{{\lambda _{\max }}(\varphi )}}{{{\lambda _{\min }}(U)}}(t - {t_0})} \right\}. |
Further, we obtain \mathop {\lim }\limits_{t \to \infty } \left\| {A(t)} \right\| = 0 by condition (4.8).
Remark 4. Differing from existing discontinuous control methods, the impulsive instants are determined by the event-triggered function (4.7), and the Zeno phenomenon can be excluded via the term \frac{b}{{{{(t - {t_0})}^2}}} . In addition, note that the extinction of rumors depends on the selection of parameters \varepsilon and \mu , which means that government and relevant agencies can provide more cost-effective interventions.
In this section, different parameters are selected to verify the correctness of the theoretical analysis and the effectiveness of the optimal control. In addition, the actual rumor data is simulated. Several sets of parameters of system (2.2) are shown in Table 2.
Parameter | \langle k\rangle | \Lambda | d | \alpha | \beta | v | \gamma | \delta | \xi | I_{c} |
data 1 | 12 | 0.01 | 0.06 | 0.02 | 0.01 | 0.2 | 0.1 | - | - | 1 |
data 2 | 12 | 0.02 | 0.06 | 0.06 | 0.04 | 0.2 | 0.1 | - | - | 1 |
data 3 | 12 | 0.02 | 0.06 | 0.06 | 0.04 | 0.2 | 0.1 | 0.3 | 0.1 | 0.03 |
data 4 | 12 | 0.01 | 0.06 | 0.02 | 0.01 | 0.02 | 0.01 | - | 0.1 | 0.01 |
data 5 | 12 | 0.01 | 0.06 | 0.05 | 0.03 | 0.4 | 0.06 | 0.3 | 0.1 | 0.01 |
data 6 | 12 | 0.02 | 0.06 | 0.06 | 0.04 | 0.2 | 0.1 | 0.8 | 0.1 | 0.02 |
In this part, we will numerically simulate the stability of the equilibrium for the cases of 0 \le {I_i} \le {I_c} and I_i > I_c , respectively.
Case 1. 0 \le {I_i} \le {I_c}
Choose the system parameter data 1 and the initial densities S(0) = 0.2 , {E_1}(0) = 0.3 , {E_2}(0) = 0.2 , {I_1}(0) = 0.12 , {I_2}(0) = 0.08 , {R_1}(0) = 0.05 , {R_2}(0) = 0.05 . Then, {R_0} = 0.2885 < 1 . From Theorem 1, the rumor-free equilibrium E_0 is locally asymptotically stable, as shown in Figure 2. To verify the global stability of E_0 , we select different initial values to simulate in Figure 3. Obviously, the density of the ignorant S(t) stabilizes to 0.167, and the density of {E_i}(t) , {I_i}(t) , and {R_i}(t) tend to 0 eventually. Meanwhile, Figure 4 shows the influence of model parameters on the basic reproduction number R_0 . That is, the higher propagation rate \alpha or \beta and the conversion rate v , the greater R_0 , and the R_0 decreases with the increase of the recovery rate \gamma .
In addition, consider the parameter data 2 and the initial densities S(0) = 0.3 , {E_1}(0) = 0.15 , {E_2}(0)\; = \; 0.1 , {I_1}(0) = 0.12 , {I_2}(0) = 0.08 , {R_1}(0) = 0.05 , {R_2}(0) = 0.05 . Then, it yields {R_0} = 1.9231 > 1 . From Theorems 3.3 and 3.4, the system exists a unique rumor-spreading equilibrium E^{*} for {R_0} < P = 1.924 , which E^{*} is locally asymptotically stable in Figure 5.
Case 2. {I_i} > {I_c}
To compare the influence of rumor propagation with and without the non-smooth inhibition mechanism, consider the system parameter data 3. From Figure 6, note that under the non-smooth inhibition mechanism ( NSIM ), the peak value of spreader density {I_i}(t) is lower than that without the non-smooth inhibition mechanism, and stabilizes faster. The valley value of ignorant density S(t) is higher than that without non-smooth inhibition mechanism, and stabilizes faster. This shows that the non-smooth inhibition mechanism can effectively suppress the spread of rumors.
Next, to further show the influence on different government and media control intensity, we choose the parameter data 4 here. For better visual effects, the figures of E_2(t), I_2(t) , and R_2(t) are omitted here. Evidently, it indicates that the larger the value of \delta , the faster the densities of {E_1}(t) and {I_1}(t) tend to balance (see Figure 7(b), (c)), the higher the peak density of {R_1}(t) (see Figure 7(d)), and the greater the density of S(t) (see Figure 7(a)). That is, if the density of spreader exceeds the threshold, the greater the intervention is.
Remark 5. In fact, Figure 6 shows that the non-smooth inhibition mechanism can effectively suppress rumor propagation. Besides, in Figure 7, the greater \delta (representing the intensity of intervention by the government and related media), the more effective the control of rumor propagation, which implies that we can adjust the value of parameter \delta in conjunction with the severity of rumor propagation to achieve effective intervention in rumor dissemination.
Consider the optimal control system (4.1), where the parameters are selected as data 5, and take \theta_1 = 1, \theta_2 = 1, \phi_1 = 2, \phi_2 = 3 . Figure 8 shows the density changes of each population with control and without control. Note that under the optimal control, the density of the ignorant S(t) and the stifler {R_i}(t) increase, while the density of the spreader {I_i}(t) and the exposed {E_1}(t) decreases rapidly and stabilizes faster.
The strength change of optimal control and control cost with time is shown in Figure 9. From Figure 9(a), the control strength is the largest in the initial stage, but gradually decreases to 0 with the increase of time. At the same time, it can be found from Figure 9(b) that as the control time increases, the control cost gradually increases, and the increase rate is faster in the early stage than in the later stage.
For system (4.5), choose \mu = 0.5 , \varepsilon = 0.8 , a = 1 , b = 0.008 , and data 6. Then, from Theorem 7, Figure 10(a) shows the trajectories of E_i(t) and I_i(t) under the event-triggered impulsive control ( ETIC ), and the rumor will eventually disappear. In Figure 10(b), 0 means not triggered and 1 means triggered. In addition, the comparison of optimal control ( OC ) and the ETIC is further presented in Figure 11.
Remark 6. From the comparison of ETIC and OC on the rumor propagation in Figure 11, it shows that the OC combined with non-smooth inhibition mechanism more effectively suppresses the spread of rumors in a short time. Besides, ETIC only requires to control at some discrete moments which can reduce resource consumption, and can let the rumors die out via adjusting parameters \varepsilon and \mu . Therefore, we can judiciously choose which control measures to implement based on the severity of rumor propagation and available resources.
In this part, we use an actual example to verify the validity of the theoretical analysis with the data provided in Zhiwei Data [27]. The background of the actual rumor is as follows. At 16:00 on January 29, 2020, some netizens on social media broke the news through video on social media that SF Express Courier privately opened a user's parcel by intercepting the goods and publicly hawked it in the video. At 23:00 on January 29, SF Group responded that the video was taken by the sender himself, and there was no behavior of intercepting others' express. At 15:00 on January 30, the Kunming Panlong Public Security Bureau of Yunnan Province issued a notice to refute this information. Table 3 shows the hourly propagation data of the event within 48 hours, where "T" is the propagation time and "N" is the number of rumor propagation.
T | N | T | N | T | N | T | N | T | N | T | N |
1 | 25 | 9 | 134 | 17 | 292 | 25 | 291 | 33 | 39 | 41 | 39 |
2 | 26 | 10 | 72 | 18 | 221 | 26 | 191 | 34 | 20 | 42 | 51 |
3 | 27 | 11 | 46 | 19 | 196 | 27 | 136 | 35 | 16 | 43 | 59 |
4 | 50 | 12 | 35 | 20 | 173 | 28 | 114 | 36 | 12 | 44 | 47 |
5 | 110 | 13 | 29 | 21 | 108 | 29 | 94 | 37 | 7 | 45 | 56 |
6 | 225 | 14 | 38 | 22 | 79 | 30 | 120 | 38 | 18 | 46 | 64 |
7 | 517 | 15 | 140 | 23 | 183 | 31 | 82 | 39 | 23 | 47 | 45 |
8 | 321 | 16 | 242 | 24 | 272 | 32 | 73 | 40 | 42 | 48 | 32 |
In order to make the model closer to the actual data, we choose these parameters \langle k \rangle = 12, \Lambda = 0.002, d = 0.001, \alpha = 0.4, \beta = 0.1, v = 0.5, \gamma = 0.06, \delta = 0.3, \xi = 0.1, {I_c} = 0.01 . Figure 12 describes the actual data trajectory and the spreader trajectory with \delta = 0.3 and \delta = 0.9 . From Figure 12, the density trajectory of rumor spreader is basically consistent with the overall trend of actual data, which means that our system can roughly reflect the spread process of the rumor. Moreover, the greater the intensity of government and media intervention, the lower the peak of the density of spreader, indicating that the non-smooth inhibition mechanism can effectively suppress the spread of rumors.
In this paper, we have discussed the stability problem and control strategies of the rumor propagation S2E2I2R model with non-smooth inhibition mechanism in a multi-lingual environment. First, considering the spreader threshold I_c of government and media intervention as the boundary, we have given the basic reproduction number R_0 and analyzed its stability. In addition, both optimal control and event-triggered impulsive control have been proposed to suppress the spread of rumors, and the correctness of the theory was analyzed via numerical simulation. Due to the complexity of rumor propagation in social networks, there are many factors that affect rumor propagation. In future work, we will introduce more factors into the rumor propagation model.
The authors declare they have not used Artificial Intelligence (AI) tools in the creation of this article.
This work was supported in part by the NSFC (Nos. 62006196 and 62366049), in part by Natural Science Foundation of Xinjiang Uygur Autonomous Region (No. 2021D01C113).
The authors declare there is no conflict of interest.
[1] | J. Liu, K. Niu, Z. He, J. Lin, Analysis of rumor spreading in communities based on modified SIR model in microblog, in Artificial Intelligence: Methodology, Systems, and Applications, Springer, 8722 (2014), 69–79. https://doi.org/10.1007/978-3-319-10554-3_7 |
[2] |
J. Huo, H. Zhao, Dynamical analysis of a fractional SIR model with birth and death on heterogeneous complex networks, Physica A, 448 (2016), 41–56. https://doi.org/10.1016/j.physa.2015.12.078 doi: 10.1016/j.physa.2015.12.078
![]() |
[3] |
L. Zhu, G. Guan, Y. Li, Nonlinear dynamical analysis and control strategies of a network-based SIS epidemic model with time delay, Appl. Math. Modell., 70 (2019), 512–531. https://doi.org/10.1016/j.apm.2019.01.037 doi: 10.1016/j.apm.2019.01.037
![]() |
[4] | D. Daley, D. Kendall, Stochastic rumours, IMA J. Appl. Math., 1 (1965), 42–55. https://doi.org/10.1093/imamat/1.1.42 |
[5] | D. Maki, M. Thompson, Mathematical Models and Applications, with Emphasis on Social, Life, and Management Sciences, Prentice Hall, 1973. |
[6] |
R. Jie, J. Qiao, G. Xu, Y. Meng, A study on the interaction between two rumors in homogeneous complex networks under symmetric conditions, Physica A, 454 (2016), 129–142. https://doi.org/10.1016/j.physa.2016.02.048 doi: 10.1016/j.physa.2016.02.048
![]() |
[7] |
K. Kandhway, J. Kuri, How to run a campaign: Optimal control of SIS and SIR information epidemics, Appl. Math. Comput., 231 (2014), 79–92. https://doi.org/10.1016/j.amc.2013.12.164 doi: 10.1016/j.amc.2013.12.164
![]() |
[8] |
Q. Liu, T. Li, M. Sun, The analysis of an SEIR rumor propagation model on heterogeneous network, Physica A, 496 (2017), 372–380. https://doi.org/10.1016/j.physa.2016.11.067 doi: 10.1016/j.physa.2016.11.067
![]() |
[9] |
S. Dong, F. Fan, Y. Huang, Studies on the population dynamics of a rumor-spreading model in online social networks, Physica A, 482 (2018), 10–20. https://doi.org/10.1016/j.physa.2017.09.077 doi: 10.1016/j.physa.2017.09.077
![]() |
[10] |
Y. Moreno, M. Nekovee, A. Pacheco, Dynamics of rumor spreading in complex networks, Phys. Rev. E, 69 (2004), 066130. https://doi.org/10.1103/PhysRevE.69.066130 doi: 10.1103/PhysRevE.69.066130
![]() |
[11] |
W. Zhang, H. Deng, X. Li, H. Liu, Dynamics of the rumor-spreading model with control mechanism in complex network, J. Math., 2022 (2022), 5700374. https://doi.org/10.1155/2022/5700374 doi: 10.1155/2022/5700374
![]() |
[12] |
X. Liu, T. Li, M. Tian, Rumor spreading of a SEIR model in complex social networks with hesitating mechanism, Adv. Differ. Equations, 2018 (2018), 391. https://doi.org/10.1186/s13662-018-1852-z doi: 10.1186/s13662-018-1852-z
![]() |
[13] |
L. Huo, L. Wang, N. Song, C. Ma, B. He, Rumor spreading model considering the activity of spreaders in the homogeneous network, Physica A, 468 (2017), 855–865. https://doi.org/10.1016/j.physa.2016.11.039 doi: 10.1016/j.physa.2016.11.039
![]() |
[14] |
M. Ye, J. Li, X. Han, H. Jiang, Global analysis of a fractional-order reaction-diffusion rumor-spreading model in multi-lingual environment, Eur. Phys. J. Plus, 138 (2023), 102. https://doi.org/10.1140/epjp/s13360-023-03698-8 doi: 10.1140/epjp/s13360-023-03698-8
![]() |
[15] |
H. Guo, X. Yan, Y. Niu, J. Zhang, Dynamic analysis of rumor propagation model with media report and time delay on social networks, J. Appl. Math. Comput., 69 (2023), 2473–2502. https://doi.org/10.1007/s12190-022-01829-5 doi: 10.1007/s12190-022-01829-5
![]() |
[16] |
J. Wang, H. Jiang, T. Ma, C. Hu, Global dynamics of the multi-lingual SIR rumor spreading model with cross-transmitted mechanism, Chaos Solitons Fractals, 126 (2019), 148–157. https://doi.org/10.1016/j.chaos.2019.05.027 doi: 10.1016/j.chaos.2019.05.027
![]() |
[17] |
J. Li, H. Jiang, X. Mei, C. Hu, G. Zhang, Dynamical analysis of rumor spreading model in multi-lingual environment and heterogeneous complex networks, Inf. Sci., 536 (2020), 391–408. https://doi.org/10.1016/j.ins.2020.05.037 doi: 10.1016/j.ins.2020.05.037
![]() |
[18] |
Y. Wang, X. Yang, Y. Han, X. Wang, Spreading model with trust mechanism in complex social networks, Commun. Theor. Phys., 59 (2013), 510–516. https://doi.org/10.1088/0253-6102/59/4/21 doi: 10.1088/0253-6102/59/4/21
![]() |
[19] |
J. Zhang, J. Sun, Stability analysis of an SIS epidemic model with feedback mechanism on networks, Physica A, 394 (2014), 24–32. https://doi.org/10.1016/j.physa.2013.09.058 doi: 10.1016/j.physa.2013.09.058
![]() |
[20] |
X. Ma, S. Shen, L. Zhu, Complex dynamic analysis of a reaction-diffusion network information propagation model with non-smooth control, Inf. Sci., 622 (2023), 1141–1161. https://doi.org/10.1016/j.ins.2022.12.013 doi: 10.1016/j.ins.2022.12.013
![]() |
[21] |
L. Huo, L. Wang, X. Zhao, Stability analysis and optimal control of a rumor spreading model with media report, Physica A, 517 (2019), 551–562. https://doi.org/10.1016/j.physa.2018.11.047 doi: 10.1016/j.physa.2018.11.047
![]() |
[22] |
T. Li, Y. Guo, Nonlinear dynamical analysis and optimal control strategies for a new rumor spreading model with comprehensive interventions, Qual. Theory Dyn. Syst., 20 (2021), 84. https://doi.org/10.1007/s12346-021-00520-7 doi: 10.1007/s12346-021-00520-7
![]() |
[23] |
Z. He, Z. Cai, J. Yu, X. Wang, Y. Sun, Y. Li, Cost-efficient strategies for restraining rumor spreading in mobile social networks, IEEE Trans. Veh. Technol., 66 (2017), 2789–2800. https://doi.org/10.1109/TVT.2016.2585591 doi: 10.1109/TVT.2016.2585591
![]() |
[24] |
S. Yu, Z. Yu, H. Jiang, X. Mei, J. Li, The spread and control of rumors in a multilingual environment, Nonlinear Dyn., 100 (2020), 2933–2951. https://doi.org/10.1007/s11071-020-05621-7 doi: 10.1007/s11071-020-05621-7
![]() |
[25] |
J. Wang, H. Jiang, C. Hu, Z. Yu, J. Li, Stability and Hopf bifurcation analysis of multi-lingual rumor spreading model with nonlinear inhibition mechanism, Chaos Solitons Fractals, 153 (2021), 111464. https://doi.org/10.1016/j.chaos.2021.111464 doi: 10.1016/j.chaos.2021.111464
![]() |
[26] |
T. Gronwall, Note on the derivatives with respect to a parameter of the solutions of a system of differential equations, Ann. Math., 20 (1919), 292–296. https://doi.org/10.2307/1967124 doi: 10.2307/1967124
![]() |
[27] | Zhiwei Data, "Shunfeng express couriers intercept packages to sell masks" is a rumor (in Chinese), 2020. Available from: https://ef.zhiweidata.com/event/6be161c61bd1592d10027795/trend. |
1. | Liuqin Huang, Jinling Wang, Jiarong Li, Tianlong Ma, Analysis of rumor spreading with different usage ranges in a multilingual environment, 2024, 9, 2473-6988, 24018, 10.3934/math.20241168 | |
2. | Jinling Wang, Jing Liao, Jun-Guo Lu, Jiarong Li, Mei Liu, Dynamic Analysis of the Multi-Lingual S2IR Rumor Propagation Model Under Stochastic Disturbances, 2025, 27, 1099-4300, 217, 10.3390/e27030217 |
Parameter | Definition |
\langle k \rangle | Average degree of homogeneous network |
\Lambda | The immigration rate of S(t) per unit time |
d | The removal rate of users per unit time |
\alpha | The probability of S(t) contact I_i(t) and become E_1(t) |
\beta | The probability of S(t) contact I_i(t) and become E_2(t) |
v | The transformation rate from E_i(t) to I_i(t) |
\gamma | The transformation rate from I_i(t) to R_i(t) |
I_c | The spreader threshold of government and media intervention |
\delta | The control rate of spreaders under government and media intervention |
\xi | The effectiveness of inhibition mechanism |
Parameter | \langle k\rangle | \Lambda | d | \alpha | \beta | v | \gamma | \delta | \xi | I_{c} |
data 1 | 12 | 0.01 | 0.06 | 0.02 | 0.01 | 0.2 | 0.1 | - | - | 1 |
data 2 | 12 | 0.02 | 0.06 | 0.06 | 0.04 | 0.2 | 0.1 | - | - | 1 |
data 3 | 12 | 0.02 | 0.06 | 0.06 | 0.04 | 0.2 | 0.1 | 0.3 | 0.1 | 0.03 |
data 4 | 12 | 0.01 | 0.06 | 0.02 | 0.01 | 0.02 | 0.01 | - | 0.1 | 0.01 |
data 5 | 12 | 0.01 | 0.06 | 0.05 | 0.03 | 0.4 | 0.06 | 0.3 | 0.1 | 0.01 |
data 6 | 12 | 0.02 | 0.06 | 0.06 | 0.04 | 0.2 | 0.1 | 0.8 | 0.1 | 0.02 |
T | N | T | N | T | N | T | N | T | N | T | N |
1 | 25 | 9 | 134 | 17 | 292 | 25 | 291 | 33 | 39 | 41 | 39 |
2 | 26 | 10 | 72 | 18 | 221 | 26 | 191 | 34 | 20 | 42 | 51 |
3 | 27 | 11 | 46 | 19 | 196 | 27 | 136 | 35 | 16 | 43 | 59 |
4 | 50 | 12 | 35 | 20 | 173 | 28 | 114 | 36 | 12 | 44 | 47 |
5 | 110 | 13 | 29 | 21 | 108 | 29 | 94 | 37 | 7 | 45 | 56 |
6 | 225 | 14 | 38 | 22 | 79 | 30 | 120 | 38 | 18 | 46 | 64 |
7 | 517 | 15 | 140 | 23 | 183 | 31 | 82 | 39 | 23 | 47 | 45 |
8 | 321 | 16 | 242 | 24 | 272 | 32 | 73 | 40 | 42 | 48 | 32 |
Parameter | Definition |
\langle k \rangle | Average degree of homogeneous network |
\Lambda | The immigration rate of S(t) per unit time |
d | The removal rate of users per unit time |
\alpha | The probability of S(t) contact I_i(t) and become E_1(t) |
\beta | The probability of S(t) contact I_i(t) and become E_2(t) |
v | The transformation rate from E_i(t) to I_i(t) |
\gamma | The transformation rate from I_i(t) to R_i(t) |
I_c | The spreader threshold of government and media intervention |
\delta | The control rate of spreaders under government and media intervention |
\xi | The effectiveness of inhibition mechanism |
Parameter | \langle k\rangle | \Lambda | d | \alpha | \beta | v | \gamma | \delta | \xi | I_{c} |
data 1 | 12 | 0.01 | 0.06 | 0.02 | 0.01 | 0.2 | 0.1 | - | - | 1 |
data 2 | 12 | 0.02 | 0.06 | 0.06 | 0.04 | 0.2 | 0.1 | - | - | 1 |
data 3 | 12 | 0.02 | 0.06 | 0.06 | 0.04 | 0.2 | 0.1 | 0.3 | 0.1 | 0.03 |
data 4 | 12 | 0.01 | 0.06 | 0.02 | 0.01 | 0.02 | 0.01 | - | 0.1 | 0.01 |
data 5 | 12 | 0.01 | 0.06 | 0.05 | 0.03 | 0.4 | 0.06 | 0.3 | 0.1 | 0.01 |
data 6 | 12 | 0.02 | 0.06 | 0.06 | 0.04 | 0.2 | 0.1 | 0.8 | 0.1 | 0.02 |
T | N | T | N | T | N | T | N | T | N | T | N |
1 | 25 | 9 | 134 | 17 | 292 | 25 | 291 | 33 | 39 | 41 | 39 |
2 | 26 | 10 | 72 | 18 | 221 | 26 | 191 | 34 | 20 | 42 | 51 |
3 | 27 | 11 | 46 | 19 | 196 | 27 | 136 | 35 | 16 | 43 | 59 |
4 | 50 | 12 | 35 | 20 | 173 | 28 | 114 | 36 | 12 | 44 | 47 |
5 | 110 | 13 | 29 | 21 | 108 | 29 | 94 | 37 | 7 | 45 | 56 |
6 | 225 | 14 | 38 | 22 | 79 | 30 | 120 | 38 | 18 | 46 | 64 |
7 | 517 | 15 | 140 | 23 | 183 | 31 | 82 | 39 | 23 | 47 | 45 |
8 | 321 | 16 | 242 | 24 | 272 | 32 | 73 | 40 | 42 | 48 | 32 |