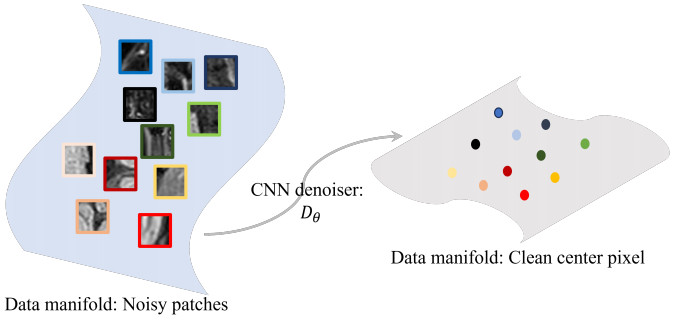
The two-dimensional (2D) cine cardiovascular magnetic resonance (CMR) technique is the reference standard for assessing cardiac function. However, one challenge with 2D cine is that the acquisition time for the whole cine stack is long and requires multiple breath holds, which may not be feasible for pediatric or ill patients. Though single breath-hold multi-slice cine may address the issue, it can only acquire low-resolution images, and hence, affect the accuracy of cardiac function assessment. To address these challenges, a Ferumoxytol-enhanced, free breathing, isotropic high-resolution 3D cine technique was developed. The method produces high-contrast cine images with short acquisition times by using compressed sensing together with a manifold-based method for image denoising. This study included fifteen patients (9.1 ± 5.6 yrs.) who were referred for clinical cardiovascular magnetic resonance imaging (MRI) with Ferumoxytol contrast and were prescribed the 3D cine sequence. The data was acquired on a 1.5T scanner. Statistical analysis shows that the manifold-based denoised 3D cine can accurately measure ventricular function with no significant differences when compared to the conventional 2D breath-hold (BH) cine. The multiplanar reconstructed images of the proposed 3D cine method are visually comparable to the golden standard 2D BH cine method in terms of clarity, contrast, and anatomical precision. The proposed method eliminated the need for breath holds, reduced scan times, enabled multiplanar reconstruction within an isotropic data set, and has the potential to be used as an effective tool to access cardiovascular conditions.
Citation: Anna Andrews, Pezad Doctor, Lasya Gaur, F. Gerald Greil, Tarique Hussain, Qing Zou. Manifold-based denoising for Ferumoxytol-enhanced 3D cardiac cine MRI[J]. Mathematical Biosciences and Engineering, 2024, 21(3): 3695-3712. doi: 10.3934/mbe.2024163
[1] | Qing Zou, Zachary Miller, Sanja Dzelebdzic, Maher Abadeer, Kevin M. Johnson, Tarique Hussain . Time-Resolved 3D cardiopulmonary MRI reconstruction using spatial transformer network. Mathematical Biosciences and Engineering, 2023, 20(9): 15982-15998. doi: 10.3934/mbe.2023712 |
[2] | Qian Wu, Yuyao Pei, Zihao Cheng, Xiaopeng Hu, Changqing Wang . SDS-Net: A lightweight 3D convolutional neural network with multi-branch attention for multimodal brain tumor accurate segmentation. Mathematical Biosciences and Engineering, 2023, 20(9): 17384-17406. doi: 10.3934/mbe.2023773 |
[3] | Zijian Wang, Yaqin Zhu, Haibo Shi, Yanting Zhang, Cairong Yan . A 3D multiscale view convolutional neural network with attention for mental disease diagnosis on MRI images. Mathematical Biosciences and Engineering, 2021, 18(5): 6978-6994. doi: 10.3934/mbe.2021347 |
[4] | Zhenyin Fu, Jin Zhang, Ruyi Luo, Yutong Sun, Dongdong Deng, Ling Xia . TF-Unet:An automatic cardiac MRI image segmentation method. Mathematical Biosciences and Engineering, 2022, 19(5): 5207-5222. doi: 10.3934/mbe.2022244 |
[5] | Sukun Tian, Ning Dai, Linlin Li, Weiwei Li, Yuchun Sun, Xiaosheng Cheng . Three-dimensional mandibular motion trajectory-tracking system based on BP neural network. Mathematical Biosciences and Engineering, 2020, 17(5): 5709-5726. doi: 10.3934/mbe.2020307 |
[6] | Hongmei Jin, Ning He, Boyu Liu, Zhanli Li . Research on gesture recognition algorithm based on MME-P3D. Mathematical Biosciences and Engineering, 2024, 21(3): 3594-3617. doi: 10.3934/mbe.2024158 |
[7] | Xue-Yao Gao, Bo-Yu Yang, Chun-Xiang Zhang . Combine EfficientNet and CNN for 3D model classification. Mathematical Biosciences and Engineering, 2023, 20(5): 9062-9079. doi: 10.3934/mbe.2023398 |
[8] | Ke Bi, Yue Tan, Ke Cheng, Qingfang Chen, Yuanquan Wang . Sequential shape similarity for active contour based left ventricle segmentation in cardiac cine MR image. Mathematical Biosciences and Engineering, 2022, 19(2): 1591-1608. doi: 10.3934/mbe.2022074 |
[9] | Tianran Yuan, Hongsheng Zhang, Hao Liu, Juan Du, Huiming Yu, Yimin Wang, Yabin Xu . Watertight 2-manifold 3D bone surface model reconstruction from CT images based on visual hyper-spherical mapping. Mathematical Biosciences and Engineering, 2021, 18(2): 1280-1313. doi: 10.3934/mbe.2021068 |
[10] | Danial Sharifrazi, Roohallah Alizadehsani, Javad Hassannataj Joloudari, Shahab S. Band, Sadiq Hussain, Zahra Alizadeh Sani, Fereshteh Hasanzadeh, Afshin Shoeibi, Abdollah Dehzangi, Mehdi Sookhak, Hamid Alinejad-Rokny . CNN-KCL: Automatic myocarditis diagnosis using convolutional neural network combined with k-means clustering. Mathematical Biosciences and Engineering, 2022, 19(3): 2381-2402. doi: 10.3934/mbe.2022110 |
The two-dimensional (2D) cine cardiovascular magnetic resonance (CMR) technique is the reference standard for assessing cardiac function. However, one challenge with 2D cine is that the acquisition time for the whole cine stack is long and requires multiple breath holds, which may not be feasible for pediatric or ill patients. Though single breath-hold multi-slice cine may address the issue, it can only acquire low-resolution images, and hence, affect the accuracy of cardiac function assessment. To address these challenges, a Ferumoxytol-enhanced, free breathing, isotropic high-resolution 3D cine technique was developed. The method produces high-contrast cine images with short acquisition times by using compressed sensing together with a manifold-based method for image denoising. This study included fifteen patients (9.1 ± 5.6 yrs.) who were referred for clinical cardiovascular magnetic resonance imaging (MRI) with Ferumoxytol contrast and were prescribed the 3D cine sequence. The data was acquired on a 1.5T scanner. Statistical analysis shows that the manifold-based denoised 3D cine can accurately measure ventricular function with no significant differences when compared to the conventional 2D breath-hold (BH) cine. The multiplanar reconstructed images of the proposed 3D cine method are visually comparable to the golden standard 2D BH cine method in terms of clarity, contrast, and anatomical precision. The proposed method eliminated the need for breath holds, reduced scan times, enabled multiplanar reconstruction within an isotropic data set, and has the potential to be used as an effective tool to access cardiovascular conditions.
The two-dimensional (2D) cine cardiovascular magnetic resonance (CMR) technique obtains a series of 2D images in quick succession and presents them as a movie to show the heart's motion [1]. This method allows for the functional assessment of the heart, myocardial motion, and cardiac valves [2]. It serves as a valuable tool for quantifying both global and regional functions of the left and right ventricles by measuring key parameters, including the stroke volume, ejection fraction, end-diastolic and end-systolic volumes, and masses [3]. It is considered the reference standard for diagnosing cardiac function [1].
Conventionally, 2D cine is used in conjunction with the balanced steady state free precession (bSSFP) sequence [4], which is a field echo (FE) based sequence that provides high contrast between blood and the myocardium [2]. While the bSSFP sequence has a high signal-to-noise ratio (SNR) and creates high contrast images, it does pose some challenges. For example, the sequence is overly sensitive to magnetic field inhomogeneities, thus creating image banding artifacts [2]. Another challenge of 2D cine is that the acquisition time for the whole cine stack is long and requires multiple breath holds, which may not be feasible for pediatric or ill patients. Additionally, the extended scan time increases the likelihood of motion-based artifacts due to an inconsistent breath-holding amplitude and bulk body movement, thus potentially leading to slice-to-slice misregistration and errors in ventricular volume measurements [5].
Various new techniques have aimed to overcome the challenges of 2D cine. To overcome the challenges that breath-holding presents, a free-breathing 2D cine technique has been developed [6,7,8]. This method increases the spatiotemporal resolution due to an increased acquisition window and the minimization of slice misregistration. However, the challenge of 2D cine is that ventricles must be imaged in multiple orientations because the method has anisotropic spatial resolution and can only acquire data in a specific geometry [9]. Moreover, adjusting the plane orientations for cardiac slice angulation requires rigorous scan planning from an expert operator [10].
A recent advancement in cardiac imaging is the introduction of 3D cine [11,12]. This method simplifies planning and reduces the scan time due to its isotropic spatial resolution, thus allowing for reformatting into any plane. Moreover, 3D cine is important and valuable, as it captures the complex anatomy of patients with congenital heart disease. This is unlike 2D cine, which has high a slice thickness (e.g. 8mm) and cannot capture all of the details. However, despite its promising capabilities, there are some drawbacks. Some studies propose acquisition within a single breath hold [13]. Due to the short acquisition window, this method produces low spatiotemporal resolution images. Mehdi and colleagues [10] proposed a gadolinium-enhanced 3D cine solution that used the steady state free precession (SSFP) sequence [14] with parallel imaging and sensitivity encoding (SENSE) [15]. They used Heart-Nav [16] to prospectively gate and track the heart's position during free breathing. This method provided promising results in obtaining heart function by acquiring 3D whole-heart cine images. However, the acquisition time reported in the work (average of 6 minutes) was long. This led to some difficulties in applying the method to some patients, such as young children without general anesthesia, who have difficulties staying still for such a long time. Another challenge is with the contrast agent. Gadolinium can be washed out in a short time and the 3D cine sequence is usually run at the end of the exam. This limits the image contrast and can lower the success rate for running this sequence.
In this study, we advance the field of 3D cine acquisition by employing compressed sensing [17], thus dramatically reducing acquisition time to an average of just 3.5 minutes. This approach involves using a high compressed sensing (CS) factor, which is a pioneering technique that allows for the rapid capture of 3D cine data. The purpose of this work is the application of a convolutional neural network (CNN) to denoise images from an accelerated acquisition. In other words, though they are initially noisy due to the high CS factor, the initial images are effectively refined using a novel manifold-based image denoising method, thus showcasing our commitment to image quality.
A major contribution of our work is the use of Ferumoxytol [18] for superior image contrast enhancement. This agent offers prolonged retention in the body and exhibits a higher T1 relaxivity, thus marking a significant improvement over traditional contrast agents. Furthermore, our research provides a detailed comparison between the 3D cine images acquired using our advanced method and the conventional 2D cine bSSFP images. By focusing on key cardiac metrics like stroke volume, ejection fraction, end-diastolic, and end-systolic volumes, this comparison highlights the practical and clinical benefits of our proposed 3D cine protocol, thus underlining its potential impact in medical imaging.
All the data in this work were collected on a 1.5T Philips scanner (Best, The Netherlands). The Institutional Review Board (IRB) at the University of Texas Southwestern Medical Center approved all study procedures and protocols. We used a 3D T1-weighted turbo field echo sequence for data acquisition. The sequence parameters include the following: TE / TR = 1.06 ms / 2.3 ms, FOV (Field of view) = 512 mm × 512 mm × 175 mm (FH [Foot-Head] × AP [Anterior-Posterior] × RL [Right-Left]), reconstruction matrix size: 432×432×146, and flip angle = 25∘. Due to the size of the patients, we adjusted the FOV in the RL direction. The matrix size on the third dimension was also adjusted accordingly to keep the reconstructed voxel size in the RL direction at 1.2 mm. The acquisition used the sagittal slice orientation, and the foldover direction was set as AP. The scanner-calculated default turbo field echo (TFE) start-up echo was used for the acquisition. Electrocardiography (ECG) cardiac gating was used to acquire 30 cardiac phases, and the prospective respiratory motion compensation was based on the Heart-Nav approach proposed in [16]. The first start-up pulse was used to create the HeartNav signal, which was then sent to the respiratory navigator process module. To speed up the whole acquisition, the data were 4-fold undersampled, and we reconstructed the undersampled data with compressed-sensing (vendor-supplied algorithm) to obtain the initial reconstruction.
In order to validate the Ferumoxytol-enhanced 3D cine with a manifold-based denoising framework, we carried out a comprehensive retrospective study. The primary objective of this study was to assess the feasibility and clinical applicability of this enhanced imaging technique. We selected the data from a subset of 15 patients who were referred for clinical cardiovascular magnetic resonance imaging (MRI) with Ferumoxytol contrast (Feraheme ®, AMAG Pharmaceuticals, Inc.). This specific contrast agent was chosen due to its unique properties of producing strong signal enhancement, thereby improving the visibility of vascular structures in the MRI. The cohort was selected to ensure a diverse and representative set of patients with various congenital heart diseases. This patient group was deemed suitable for the study, as their clinical requirements necessitated the use of advanced imaging methods. The necessity was driven by the complexity of their cardiovascular conditions, which demanded more comprehensive and detailed images. The attending cardiologists, which are highly trained professionals with experience in managing cardiovascular disease, prescribed the 3D cine sequence for these patients as part of their routine clinical care. The decision to use the 3D cine sequence was made based on the individual patient requirements, aiming for optimal treatment outcomes. The raw k-space data were saved during data acquisition for post-processing. Saving the raw data neither adds extra scan time nor poses any additional requirements for the patients. The demographic information of the 15 patients can be found in the table in the appendix.
In this study, we introduce a novel method of using a manifold-based CNN denoiser to minimize the noise in the initially reconstructed images. The core concept behind this approach is to leverage the learning capabilities of CNNs to perform intricate patch-based denoising [19]. When employed as a patch-based approach for image denoising, manifold learning offers several compelling motivations that leverage the intrinsic structure of the image data. First, it exploits the geometric structure of the data. Images naturally form manifolds in a high-dimensional space. Similar to patch-based methods, manifold learning techniques capitalize on this structure by considering each small patch as a point in a high-dimensional space. This approach allows for the exploration of the underlying geometric and topological properties of the image data, which are essential for effective denoising. Moreover, manifold learning inherently involves reducing the dimensionality of the data. By mapping high-dimensional patches onto a lower-dimensional manifold, these methods can effectively filter out noise. The essential structures and features of the image are preserved in the lower-dimensional space, thus separating them from the noise.
We introduce a CNN denoiser function, denoted by Dθ:Rp2→R, where R represents the set of real numbers p is a real number determining the patch size, θ represents the network parameters. In this study, the patch size is p×p, and we have selected p=9, which translates into a 9×9 patch. The function of the denoiser, Dθ, is to intake a noisy image patch and output the value of the clean central pixel value. Mathematically speaking,
Dθ:Rp2→R,Dθ(X)=x, |
where X is the p×p noisy patch, and x represents the clean center pixel value. During the training process, we want to find the optimal network parameter θ∗ following the optimization problem below:
θ∗=argminθ||Dθ(X)−x||2. |
Here, the underlying assumption is that both the noisy patches and clean center pixel values are present on some unknown high-dimensional data manifolds [20]. Consequently, the CNN denoiser is tasked with learning a non-linear map between these two data manifolds. The architecture of the CNN employed is a 5-layer network using the rectified linear unit (ReLU) activation function [21]. Specifically, the first four layers are convolutional layers, using 100 filters of kernel size 3×3 each layer. The last layer is a fully connected layer. This type of network is particularly effective for image denoising tasks due to its capacity to learn complex features from images. The illustration of the manifold-based denoising framework is given in Figure 1.
However, given the unavailability of clean 3D cardiac MRI data, we used 2D cardiac cine images to train the network. The 2D cine was acquired using the T1-TFE sequence with Ferumoxytol contrast. This results in a 2D denoiser, thus implying that the denoising for the 3D cine data was performed slice by slice. In single-coil MRI systems, the noise in the magnitude of MRI data adheres to a Rician distribution. Additionally, in areas where the SNR is high, the noise that follows a Rician distribution can be closely estimated by a Gaussian distribution. More commonly, the noise that impacts the pixels within an image is of a Gaussian type, which disrupts the pixels containing information in a consistent manner across the image. Therefore, we used the Gaussian noise model in this work. To train the network, we simulated various noise conditions by manually adding Gaussian white noise with standard deviations ranging from 5 to 30, in increments of 5, into four types of cardiac cine: short-axis cine, two-chamber cine, four-chamber cine, and axial stack cine. The training data were gathered from 10 patients (different than the patients for 3D cine denoising). Each patient had about 500 2D images for the training, and the image size depended on the patient's size. We used overlapped patches while generating the patches. A vendor-provided denoising method was used during the 2D cine acquisition; hence, we regard the 2D cine images as clean images, with the clean center pixel values originating from these images. During the training phase, the noisy patches were input into the network, and the output was compared to the true clean center pixel value using the mean-square-error (MSE).
Following the training phase, we utilized the denoiser to eliminate noise from the initially reconstructed 3D cine images, thus yielding the final 3D cine. This approach highlights a significant leap forward in image reconstruction and denoising, thereby promising a substantial potential for improving the quality and precision of 3D cardiac MRI examinations.
In this study, our aim was to evaluate and compare the effectiveness of the proposed 3D cine method against the conventional 2D bSSFP cine method. Our evaluation focused on various critical ventricular function parameters, including stroke volume (SV), ejection fraction (EF), end-diastolic volume (EDV), and end-systolic volume (ESV).
To execute this comparison, we analyzed images from both imaging methods. The 3D cine images were processed using Arterys, which is a cloud-based medical imaging platform. The platform provides an advanced visualization tool capable of multiplanar reconstruction, thereby enabling the transformation of the 3D cine data into multiple views: short-axis, 2-chamber, 3-chamber, and 4-chamber views. This transformation is facilitated by identifying the cardiac valves, which are critical anatomical landmarks.
These multiplanar reconstructed images serve as a robust foundation for the quantification of cardiac function. To achieve this quantification, the left and right ventricles are first identified and manually segmented in the short-axis view. This crucial step requires a high level of precision, as the accuracy of the segmentation can directly impact the final calculation of the cardiac function parameters.
After the successful segmentation of the ventricles, the Arterys platform can then compute the SV, EF, EDV, and ESV for each ventricle. These parameters provide a comprehensive assessment of cardiac function.
The calculated values provide critical insights into the functional status of the heart, thus aiding clinicians in making an accurate diagnosis and treatment plan. Therefore, the comparison of these parameters between the 3D cine method and the conventional 2D cine bSSFP method offers valuable information on the effectiveness and potential advantages of the proposed 3D cine imaging technique.
Additionally, we analyzed the SNR for the images before and after the manifold-based denoising to illustrate the effectiveness of the proposed denoising algorithm. The SNR is calculated as follows:
SNR=20logμσ, |
where μ is the mean intensity value of the selected region in the region of interest, and σ is the standard deviation of the intensity value of a selected noisy region.
In this study, we utilized a variety of statistical analyses to quantify the accuracy of the proposed 3D cine method when calculating ventricular measurements, compared with the standard 2D cine bSSFP method.
Paired T-tests [22] were performed for each of the measurements. We conducted T-tests for each cardiac function parameter (stroke volume, ejection fraction, end-diastolic, and end-systolic volumes) and evaluated the p-values to test whether any differences in measurements between the two methods were statistically significant.
Additionally, we calculated R2 values [23] for each of the measurements. In our context, the R2 values indicate the proportion of the variability in ventricular measurements that can be explained by the 3D cine method.
Additionally, a Bland-Altman analysis [24] was conducted to assess the level of agreement between the 2D cine bSSFP method and the proposed 3D cine method.
These statistical analyses provide a robust framework for evaluating the accuracy and reliability of the proposed 3D cine method, thus offering insights into how this new method compares with the standard 2D cine bSSFP method in terms of both statistical significance and clinical relevance.
In this section, we demonstrate the denoising capability of the proposed method through the visualization of results. We selected representative images from two patients to exhibit the effectiveness of the method, as shown in Figure 2. Additionally, the signal intensity profiles were used to illustrate the denoising. We chose one dataset to showcase this, selecting both end-diastolic and end-systolic phases before and after denoising, as displayed in Figure 3. From the plots, it is evident that after the denoising, the signal intensity profiles become smoother, thus indicating the effectiveness of the denoising. Movies to illustrate the denoising can also be found in the Supplemental material.
Observation of the figure indicates a significant improvement in the image quality following the denoising process. Most notably, the noise within the blood pool region is substantially suppressed. This suppression is of a particular significance, since the noise in this area often obstructs the visualization of critical cardiac structures, thus hindering the accurate assessment of cardiovascular health.
For example, in the case of patient 1, the image prior to denoising shows the aortic valve as somewhat blurred due to the noise. This blurring can make it challenging for clinicians to accurately assess the valve's structure and function. However, after applying the denoising technique, the aortic valve becomes clear, thus enabling a much more precise evaluation. Similarly, other areas such as the liver region and the spine also exhibit substantial improvements in clarity post-denoising. Previously, noise in these regions may have obscured anatomical details or pathological changes. By applying our denoising method, we can see these regions with greater clarity, thus enhancing the potential diagnostic value of the images.
The improved image quality is further demonstrated by the quantitative results. In Table 1, we compare the SNR of the heart, liver, spine, and lung region before and after denoising.
Heart | Liver | Spine | Lung | |
Initial Reconstruction | 46.69 | 36.16 | 38.97 | 18.73 |
After Denoising | 51.55 | 40.66 | 43.19 | 22.29 |
Overall, the results illustrated in the figure and table highlight the potential of our proposed method to improve the quality of 3D cine images. By significantly reducing noise, particularly in critical regions such as the blood pool, we enhance the visibility of essential structures and thereby contribute to more accurate diagnoses and improved patient outcomes.
In this section, we demonstrate the effectiveness of the 3D cine technique with manifold denoising in the diagnosis and treatment of congenital heart defects (CHD). To demonstrate its utility, we present images from two patients with complex CHDs, thus exhibiting vastly differing coronary anatomies.
As depicted in Figure 4, the images of the first patient display an {S, L, L} transposition of the great arteries, a large inlet ventricular septal defect, and subpulmonary stenosis. These abnormalities result in an unconventional coronary structure, as visible in the multiplanar reconstructed (MPR) images. In contrast, the second patient has Fontan anatomy and hypoplastic right heart syndrome. Despite the complex underlying anatomy, the coronary image for this patient shows normal coronary structures.
To further illustrate the potential of the proposed technique, we also present representative images with different views from two additional patients in Figure 5. The first patient was diagnosed with Tetralogy of Fallot, which is a congenital cardiac malformation, and has undergone a transannular patch repair. The presented images label important anatomical structures, thus demonstrating that our method can clearly delineate these structures and providing enough diagnostic information for clinicians to make informed decisions. The second patient has a particularly unique cardiac anatomy, resulting from heterotaxy, dextrocardia, a complex single ventricle with severely unbalanced right dominant atrioventricular canal, aortic atresia, and a common atrioventricular valve. Moreover, the patient underwent a 20 mm extracardiac fenestrated Fontan procedure, which was situated on the left side. Despite the unusual cardiac anatomy, our method has successfully visualized some of the critical structures and the Fontan pathway.
This section emphasizes the powerful capabilities of the proposed 3D cine method with manifold denoising to facilitate the understanding and management of complex congenital heart diseases. By providing clear, accurate visualizations of unique anatomical structures and postoperative conditions, this method can significantly assist clinicians in diagnosing, monitoring, and treating these conditions.
In this section, we present a visual comparison between the images obtained from our proposed 3D cine with manifold denoising technique and those obtained using the traditional 2D breath-hold (BH) cine method. To facilitate this comparison, we employed MPR to derive three distinct views from our 3D cine images: the short-axis view, the four-chamber view, and the two-chamber view. These three views provide comprehensive visual information about different aspects of the heart's structure and function.
We compared these 3D cine-derived views with the corresponding views obtained from 2D BH cine, at both the end-diastolic phase and the end-systolic phase, as shown in Figure 6. Upon comparison, we observe that our proposed 3D cine technique is capable of producing visually comparable results to the traditional 2D BH cine method. The images obtained using 3D cine exhibit similar clarity, contrast, and anatomical precision to those produced using 2D BH cine, thus suggesting that our proposed method can effectively match the gold standard in terms of the visual quality. This indicates that 3D cine with manifold denoising could potentially serve as an effective alternative to traditional 2D BH cine for capturing and analyzing cardiac images.
In this section, we present a quantitative comparison between the 3D cine images obtained using our proposed method and the conventional 2D cine bSSFP images, focusing on measurements of the left and right ventricles. This comparison was conducted to illustrate the potential applications of the proposed 3D cine protocol in clinical cardiology. The results of this comparative analysis are presented in Table 2 and Figure 7.
Left Ventricle | Right Ventricle | |||||||
LVEF (%) | EDV (ml) | ESV (ml) | SV (ml) | RVEF (%) | EDV (ml) | ESV (ml) | SV (ml) | |
2D Cine (bSSFP) | 54.0±7.8 | 92.5±55.7 | 43.7±34.0 | 48.7±28.1 | 50.0±14.1 | 137.4±1023.6 | 76.9±77.0 | 60.5±42.5 |
3D Cine (SSFP) | 54.6±9.2 | 93.4±55.4 | 43.7±32.0 | 48.5±26.9 | 49.9±15.7 | 137.1±102.1 | 78.7±81.7 | 58.4±41.2 |
Mean Difference (3D-2D) | 0.6±2.2 | 0.9±2.8 | -0.03±3.5 | -0.2±2.7 | 0.2±5.0 | -0.3±6.3 | 1.9±10.8 | -2.1±7.0 |
Mean % difference (3D-2D) | 3.6±4.2 | 3.9±3.7 | 4.9±3.6 | 5.8±4.6 | 9.1±9.6 | 4.4±6.4 | 10.2±10.0 | 11.1±11.2 |
p value | 0.362 | 0.275 | 0.980 | 0.756 | 0.915 | 0. 897 | 0.598 | 0.376 |
R2 value | 0.960 | 0.997 | 0.992 | 0.992 | 0.902 | 0.996 | 0.985 | 0.973 |
Table 2 compares the key volumetric parameters – stroke volume, ejection fraction, end-diastolic volume, and end-systolic volume – obtained from both the 3D cine and 2D cine bSSFP methods. The statistical analysis shows that there are no significant differences between the measurements derived from the two methods, as indicated by the p-values reported in the table, all of which were greater than 0.05. Furthermore, the R2 values indicate that a large proportion of the variability in the ventricular measurements can be explained by the 3D cine method, thus corroborating its potential as a reliable alternative to the traditional 2D cine bSSFP technique.
To further quantify the degree of agreement between the ventricular measurements derived from the 3D and 2D cine sequences, we conducted a Bland-Altman analysis. The results of this analysis are presented as Bland-Altman plots in Figure 7. In these plots, the solid line represents the average difference, or bias, between the two methods. The specific numerical values for these mean differences are also detailed in Table 2.
The mean differences are found to be very close to zero, thus indicating a minimal bias between the 2D and 3D cine methods. The dotted lines on the Bland-Altman plots denote the 95% confidence interval, representing ± 1.96 standard deviations from the mean bias. The narrowness of these confidence intervals in Figure 7 suggests a high degree of agreement between the 2D cine bSSFP and the proposed 3D cine methods.
Collectively, these results highlight the potential of our proposed 3D cine protocol as a reliable and accurate tool for ventricular measurements, thus matching the established 2D cine bSSFP method in both precision and reliability.
In this work, we studied Ferumoxytol-enhanced 3D cine with manifold-based denoising, which is a promising technique that has the potential to significantly advance the field of cardiovascular MRI. This technique leverages the superior contrast offered by Ferumoxytol, which is a type of iron oxide nanoparticle used as a contrast agent in MRI, in combination with 3D cine MRI to obtain high-resolution, dynamic images of the cardiovascular system.
One of the key advantages of this technique is that it does not require patients to hold their breath during the procedure, thus making it more comfortable for those who have difficulty holding their breath. This can be particularly beneficial for individuals with respiratory or cardiovascular issues, who may find traditional methods challenging.
Our results show that this technique is not only feasible, but also comparable to the conventional 2D breath-hold cine bSSFP method, which is considered the reference standard for ventricular measurements. Statistical analyses, including T-tests, R2 calculations, and Bland-Altman analyses, all support this finding, with no significant differences between the two methods. These findings are in line with the existing results shown in [10]. Both our work and theirs demonstrate that 3D cine is reliable in assessing ventricular function. Distinguishing ourselves from [10], we proposed to use Ferumoxytol contrast and a high compressed sensing factor alongside a manifold-based denoising algorithm to reduce the scan time and enhance the visibility of the images. With the cleaner images, not only ventricular function can be obtained, but also the dynamics of the whole heart can be clearly visualized. In other words, the cleaner images provide comprehensive 3D information about the heart, including intricate structures and abnormal anatomy, which are critical for planning surgical or interventional procedures.
However, it should be noted that although the manifold-based denoising framework is powerful, its performance depends on the quality of the training data used. The potential for variations in noise profiles across different scanning environments, scanner types, and patient groups calls for a diverse and representative training set to ensure the robustness of the denoising network. Additionally, the current denoising framework performed slice-by-slice denoising due to the lack of 3D training data for 3D denoiser training. In the future, an unsupervised 3D denoiser might provide an alternative solution for the denoising of the 3D cine without requiring clean 3D training images.
The Ferumoxytol-enhanced 3D cine with manifold-based denoising presents the potential to offer improved image quality, patient comfort, and diagnostic accuracy. Future studies are needed to optimize and validate this technique in broader clinical settings and populations.
A Ferumoxytol-enhanced free breathing 3D cine sequence was developed. The method produced high-contrast cine images with short acquisition times by using compressed sensing alongside a manifold-based method for image denoising. The technique can accurately measure ventricular function with no significant differences compared to the conventional 2D breath-hold cine bSSFP method. Additionally, the multiplanar reconstructed images of the proposed 3D cine method were visually comparable to the golden standard 2D BH cine method in terms of clarity, contrast, and anatomical precision. The proposed method eliminates the need for breath holds, reduces scan times, enables multiplanar reconstruction within an isotropic data set, and has the potential to be used as an effective tool in the assessment of cardiovascular conditions.
The authors declare they have not used Artificial Intelligence (AI) tools in the creation of this article.
The authors declare there is no conflict of interest.
Patient | Age | Sex | Diagnosis |
Deidentified patient information. Abbreviations: HLHS: Hypoplastic Left Heart Syndrome; DORV: Double Outlet Right Ventricle; ASD: Atrial Septal Defect; VSD: Ventricular septal defect; DILV: Double inlet left ventricle; DORV: Double outlet of right ventricle; d-TGA: Dextro-Transposition of the great arteries; ToF: Tetralogy of Fallot | |||
Subject 1 | 2 yo | F | Pulmonary stenosis status post pulmonary valvuloplasty |
Subject 2 | 9 yo | M | Ebstein anomoly status post tricuspid valve replacement |
Subject 3 | 14 yo | F | pulmonary atresia with intact septum, hypoplastic RV, and coronary sinusoids |
Subject 4 | 12 yo | F | ToF status post transannular patch repair |
Subject 5 | 6 yo | F | {S, D, D} DORV, mitral atresia, pulmonary atresia |
Subject 6 | 7 yo | M | HLHS status post Glenn anastomosis |
Subject 7 | 8 yo | M | DILV status post Fontan |
Subject 8 | 13 yo | M | DORV with doubly committed VSD, d-TGA |
Subject 9 | 16 yo | F | Pulmonary atresia with intact ventricular septum |
Subject 10 | 13 yo | M | {S, L, L} TGA large inlet VSD closed with AVV tissue |
Subject 11 | 10 mo | F | {S, D, D} DORV, remote VSD |
Subject 12 | 19 mo | M | {S, L, D} DORV, subpulmonary VSD |
Subject 13 | 12 yo | M | HLHS |
Subject 14 | 20 yo | M | Ebstein anomaly |
Subject 15 | 13 yo | M | ToF status post transannular patch repair |
[1] |
S. V. Raman, M. Markl, A. R. Patel, J. Bryant, B. D. Allen, S. Plein, et al., 30-minute cmr for common clinical indications: a society for cardiovascular magnetic resonance white paper, J. Cardiovasc. Magn. Reson., 24 (2022), 13. https://doi.org/10.1186/s12968-022-00844-6 doi: 10.1186/s12968-022-00844-6
![]() |
[2] | M. A. Syed, S. V. Raman, O. P. Simonetti, Basic principles of cardiovascular MRI: physics and imaging techniques, Springer, 2015. |
[3] |
R. Menchón-Lara, F. Simmross-Wattenberg, P. Higuera, M. Martín-Fernández, C. Alberola-López, Reconstruction techniques for cardiac cine mri, Insights Imag., 10 (2019), 1–16. https://doi.org/10.1186/s13244-019-0754-2 doi: 10.1186/s13244-019-0754-2
![]() |
[4] |
C. M. Kramer, J. Barkhausen, C. Bucciarelli-Ducci, S. D. Flamm, R. J. Kim, E. Nagel, Standardized cardiovascular magnetic resonance imaging (cmr) protocols: 2020 update, J. Cardiovasc. Magn. Reson., 22 (2020), 1–18. https://doi.org/10.1186/s12968-020-00607-1 doi: 10.1186/s12968-020-00607-1
![]() |
[5] |
J. Liu, P. Spincemaille, N. C. F. Codella, T. D. Nguyen, M. R. Prince, Y. Wang, Respiratory and cardiac self-gated free-breathing cardiac cine imaging with multiecho 3d hybrid radial ssfp acquisition, Magn. Reson. Med., 63 (2010), 1230–1237. https://doi.org/10.1002/mrm.22306 doi: 10.1002/mrm.22306
![]() |
[6] |
Q. Lyu, H. Shan, Y. Xie, A. C. Kwan, Y. Otaki, K. Kuronuma, et al., Cine cardiac mri motion artifact reduction using a recurrent neural network, IEEE Trans. Med. Imaging, 40 (2021), 2170–2181. https://doi.org/10.1109/TMI.2021.3073381 doi: 10.1109/TMI.2021.3073381
![]() |
[7] |
Q. Zou, A. H. Ahmed, P. Nagpal, S. Kruger, M. Jacob, Dynamic imaging using a deep generative storm (gen-storm) model, IEEE Trans. Med. Imaging, 40 (2021), 3102–3112. https://doi.org/10.1109/TMI.2021.3065948 doi: 10.1109/TMI.2021.3065948
![]() |
[8] |
Q. Zou, A. H. Ahmed, S. Dzelebdzic, T. Hussain, Free-breathing and ungated cardiac mri reconstruction using a deep kernel representation, Appl. Sci., 13 (2023), 2281. https://doi.org/10.3390/app13042281 doi: 10.3390/app13042281
![]() |
[9] |
D. C. Peters, R. Nezafat, H. Eggers, C. Stehning, W. J. Manning, 2d free-breathing dual navigator-gated cardiac function validated against the 2d breath-hold acquisition, J. Magn. Reson. Imaging, 28 (2008), 773–777. https://doi.org/10.1002/jmri.21417 doi: 10.1002/jmri.21417
![]() |
[10] |
M. H. Moghari, A. Barthur, M. E. Amaral, T. Geva, A. J. Powell, Free-breathing whole-heart 3d cine magnetic resonance imaging with prospective respiratory motion compensation, Magn. Reson. Med., 80 (2018), 181–189. https://doi.org/10.1002/mrm.27021 doi: 10.1002/mrm.27021
![]() |
[11] |
M. Usman, B. Ruijsink, M. S. Nazir, G. Cruz, C. Prieto, Free breathing whole-heart 3d cine mri with self-gated cartesian trajectory, Magn. Reson. Med., 38 (2017), 129–137. https://doi.org/10.1016/j.mri.2016.12.021 doi: 10.1016/j.mri.2016.12.021
![]() |
[12] |
J. Liu, L. Feng, H. -W. Shen, C. Zhu, Y. Wang, K. Mukai, et al., Highly-accelerated self-gated free-breathing 3d cardiac cine mri: Validation in assessment of left ventricular function, Magn. Reson. Mater. Phys. Biol. Med., 30 (2017), 337–346. https://doi.org/10.1007/s10334-017-0607-2 doi: 10.1007/s10334-017-0607-2
![]() |
[13] |
T. Küstner, A. Bustin, O. Jaubert, R. Hajhosseiny, P. G. Masci, R. Neji, et al., Isotropic 3d cartesian single breath-hold cine mri with multi-bin patch-based low-rank reconstruction, Magn. Reson. Med., 84 (2020), 2018–2033. https://doi.org/10.1002/mrm.28267 doi: 10.1002/mrm.28267
![]() |
[14] |
H. Y. Carr, Steady-state free precession in nuclear magnetic resonance, Phys. Rev., 112 (1958), 1693. https://doi.org/10.1103/PhysRev.112.1693 doi: 10.1103/PhysRev.112.1693
![]() |
[15] |
J. Hamilton, D. Franson, N. Seiberlich, Recent advances in parallel imaging for mri, Prog. Nucl. Magn. Reson. Spectrosc., 101 (2017), 71–95. https://doi.org/10.1016/j.pnmrs.2017.04.002 doi: 10.1016/j.pnmrs.2017.04.002
![]() |
[16] |
M. H. Moghari, T. Geva, A. J. Powell, Prospective heart tracking for whole-heart magnetic resonance angiography, Magn. Reson. Med, 77 (2017), 759–765. https://doi.org/10.1002/mrm.26117 doi: 10.1002/mrm.26117
![]() |
[17] |
M. Lustig, D. L. Donoho, J. M. Santos, J. M. Pauly, Compressed sensing mri, IEEE Signal Process. Mag., 25 (2008), 72–82. https://doi.org/10.1109/MSP.2007.914728 doi: 10.1109/MSP.2007.914728
![]() |
[18] |
M. R. Bashir, L. Bhatti, D. Marin, R. C. Nelson, Emerging applications for ferumoxytol as a contrast agent in mri, J. Magn. Reson. Imaging, 41 (2015), 884–898. https://doi.org/10.1002/jmri.24691 doi: 10.1002/jmri.24691
![]() |
[19] |
Y. Zhang, H. Lin, Y. Li, H. Ma, A patch based denoising method using deep convolutional neural network for seismic image, IEEE Access, 7 (2019), 156883–156894. https://doi.org/10.1109/ACCESS.2019.2949774 doi: 10.1109/ACCESS.2019.2949774
![]() |
[20] |
J. He, L. Ding, L. Jiang, Z. Li, Q. Hu, Intrinsic dimensionality estimation based on manifold assumption, J. Vis. Commun. Image Represent., 25 (2014), 740–747. https://doi.org/10.1016/j.jvcir.2014.01.006 doi: 10.1016/j.jvcir.2014.01.006
![]() |
[21] | T. Kessler, G. Dorian, J. H. Mack, Application of a rectified linear unit (relu) based artificial neural network to cetane number predictions, in Internal Combustion Engine Division Fall Technical Conference, American Society of Mechanical Engineers, 58318 (2017), V001T02A006. https://doi.org/10.1115/ICEF2017-3614 |
[22] |
T. K. Kim, T test as a parametric statistic, Korean J. Anesthesiol., 68 (2015), 540–546. https://doi.org/10.4097/kjae.2015.68.6.540 doi: 10.4097/kjae.2015.68.6.540
![]() |
[23] | G. B. Barrett, The coefficient of determination: Understanding r squared and r squared, Math. Teacher, 93 (2000), 230–234. |
[24] |
D. Giavarina, Understanding bland altman analysis, Biochem. Med., 25 (2015), 141–151. https://doi.org/10.11613/BM.2015.015 doi: 10.11613/BM.2015.015
![]() |
1. | James C. L. Chow, Computational physics and imaging in medicine, 2025, 22, 1551-0018, 106, 10.3934/mbe.2025005 |
Heart | Liver | Spine | Lung | |
Initial Reconstruction | 46.69 | 36.16 | 38.97 | 18.73 |
After Denoising | 51.55 | 40.66 | 43.19 | 22.29 |
Left Ventricle | Right Ventricle | |||||||
LVEF (%) | EDV (ml) | ESV (ml) | SV (ml) | RVEF (%) | EDV (ml) | ESV (ml) | SV (ml) | |
2D Cine (bSSFP) | 54.0±7.8 | 92.5±55.7 | 43.7±34.0 | 48.7±28.1 | 50.0±14.1 | 137.4±1023.6 | 76.9±77.0 | 60.5±42.5 |
3D Cine (SSFP) | 54.6±9.2 | 93.4±55.4 | 43.7±32.0 | 48.5±26.9 | 49.9±15.7 | 137.1±102.1 | 78.7±81.7 | 58.4±41.2 |
Mean Difference (3D-2D) | 0.6±2.2 | 0.9±2.8 | -0.03±3.5 | -0.2±2.7 | 0.2±5.0 | -0.3±6.3 | 1.9±10.8 | -2.1±7.0 |
Mean % difference (3D-2D) | 3.6±4.2 | 3.9±3.7 | 4.9±3.6 | 5.8±4.6 | 9.1±9.6 | 4.4±6.4 | 10.2±10.0 | 11.1±11.2 |
p value | 0.362 | 0.275 | 0.980 | 0.756 | 0.915 | 0. 897 | 0.598 | 0.376 |
R2 value | 0.960 | 0.997 | 0.992 | 0.992 | 0.902 | 0.996 | 0.985 | 0.973 |
Heart | Liver | Spine | Lung | |
Initial Reconstruction | 46.69 | 36.16 | 38.97 | 18.73 |
After Denoising | 51.55 | 40.66 | 43.19 | 22.29 |
Left Ventricle | Right Ventricle | |||||||
LVEF (%) | EDV (ml) | ESV (ml) | SV (ml) | RVEF (%) | EDV (ml) | ESV (ml) | SV (ml) | |
2D Cine (bSSFP) | 54.0±7.8 | 92.5±55.7 | 43.7±34.0 | 48.7±28.1 | 50.0±14.1 | 137.4±1023.6 | 76.9±77.0 | 60.5±42.5 |
3D Cine (SSFP) | 54.6±9.2 | 93.4±55.4 | 43.7±32.0 | 48.5±26.9 | 49.9±15.7 | 137.1±102.1 | 78.7±81.7 | 58.4±41.2 |
Mean Difference (3D-2D) | 0.6±2.2 | 0.9±2.8 | -0.03±3.5 | -0.2±2.7 | 0.2±5.0 | -0.3±6.3 | 1.9±10.8 | -2.1±7.0 |
Mean % difference (3D-2D) | 3.6±4.2 | 3.9±3.7 | 4.9±3.6 | 5.8±4.6 | 9.1±9.6 | 4.4±6.4 | 10.2±10.0 | 11.1±11.2 |
p value | 0.362 | 0.275 | 0.980 | 0.756 | 0.915 | 0. 897 | 0.598 | 0.376 |
R2 value | 0.960 | 0.997 | 0.992 | 0.992 | 0.902 | 0.996 | 0.985 | 0.973 |