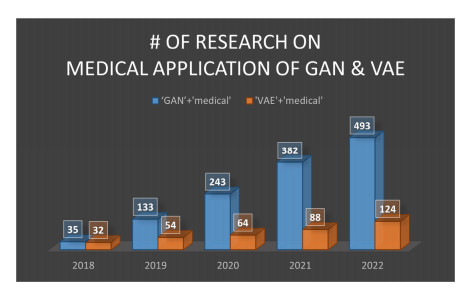
Recently, artificial intelligence generated content (AIGC) has been receiving increased attention and is growing exponentially. AIGC is generated based on the intentional information extracted from human-provided instructions by generative artificial intelligence (AI) models. AIGC quickly and automatically generates large amounts of high-quality content. Currently, there is a shortage of medical resources and complex medical procedures in medicine. Due to its characteristics, AIGC can help alleviate these problems. As a result, the application of AIGC in medicine has gained increased attention in recent years. Therefore, this paper provides a comprehensive review on the recent state of studies involving AIGC in medicine. First, we present an overview of AIGC. Furthermore, based on recent studies, the application of AIGC in medicine is reviewed from two aspects: medical image processing and medical text generation. The basic generative AI models, tasks, target organs, datasets and contribution of studies are considered and summarized. Finally, we also discuss the limitations and challenges faced by AIGC and propose possible solutions with relevant studies. We hope this review can help readers understand the potential of AIGC in medicine and obtain some innovative ideas in this field.
Citation: Liangjing Shao, Benshuang Chen, Ziqun Zhang, Zhen Zhang, Xinrong Chen. Artificial intelligence generated content (AIGC) in medicine: A narrative review[J]. Mathematical Biosciences and Engineering, 2024, 21(1): 1672-1711. doi: 10.3934/mbe.2024073
[1] | Ivan Izonin, Nataliya Shakhovska . Special issue: informatics & data-driven medicine-2021. Mathematical Biosciences and Engineering, 2022, 19(10): 9769-9772. doi: 10.3934/mbe.2022454 |
[2] | Lingli Gan, Xiaoling Yin, Jiating Huang, Bin Jia . Transcranial Doppler analysis based on computer and artificial intelligence for acute cerebrovascular disease. Mathematical Biosciences and Engineering, 2023, 20(2): 1695-1715. doi: 10.3934/mbe.2023077 |
[3] | Ivan Izonin, Nataliya Shakhovska . Special issue: Informatics & data-driven medicine. Mathematical Biosciences and Engineering, 2021, 18(5): 6430-6433. doi: 10.3934/mbe.2021319 |
[4] | Bo An . Construction and application of Chinese breast cancer knowledge graph based on multi-source heterogeneous data. Mathematical Biosciences and Engineering, 2023, 20(4): 6776-6799. doi: 10.3934/mbe.2023292 |
[5] | Juanjuan Tian, Li Li . Research on artificial intelligence of accounting information processing based on image processing. Mathematical Biosciences and Engineering, 2022, 19(8): 8411-8425. doi: 10.3934/mbe.2022391 |
[6] | Yueliang Wu, Aolong Yi, Chengcheng Ma, Ling Chen . Artificial intelligence for video game visualization, advancements, benefits and challenges. Mathematical Biosciences and Engineering, 2023, 20(8): 15345-15373. doi: 10.3934/mbe.2023686 |
[7] | Rachael C. Adams, Behnam Rashidieh . Can computers conceive the complexity of cancer to cure it? Using artificial intelligence technology in cancer modelling and drug discovery. Mathematical Biosciences and Engineering, 2020, 17(6): 6515-6530. doi: 10.3934/mbe.2020340 |
[8] | Jiajia Jiao, Xiao Xiao, Zhiyu Li . dm-GAN: Distributed multi-latent code inversion enhanced GAN for fast and accurate breast X-ray image automatic generation. Mathematical Biosciences and Engineering, 2023, 20(11): 19485-19503. doi: 10.3934/mbe.2023863 |
[9] | Qiongyang Zhou, Tianyu Zhao, Kaidi Feng, Rui Gong, Yuhui Wang, Huijun Yang . Artificial intelligence in acupuncture: A bibliometric study. Mathematical Biosciences and Engineering, 2023, 20(6): 11367-11378. doi: 10.3934/mbe.2023504 |
[10] | Yu Lei, Zhi Su, Xiaotong He, Chao Cheng . Immersive virtual reality application for intelligent manufacturing: Applications and art design. Mathematical Biosciences and Engineering, 2023, 20(3): 4353-4387. doi: 10.3934/mbe.2023202 |
Recently, artificial intelligence generated content (AIGC) has been receiving increased attention and is growing exponentially. AIGC is generated based on the intentional information extracted from human-provided instructions by generative artificial intelligence (AI) models. AIGC quickly and automatically generates large amounts of high-quality content. Currently, there is a shortage of medical resources and complex medical procedures in medicine. Due to its characteristics, AIGC can help alleviate these problems. As a result, the application of AIGC in medicine has gained increased attention in recent years. Therefore, this paper provides a comprehensive review on the recent state of studies involving AIGC in medicine. First, we present an overview of AIGC. Furthermore, based on recent studies, the application of AIGC in medicine is reviewed from two aspects: medical image processing and medical text generation. The basic generative AI models, tasks, target organs, datasets and contribution of studies are considered and summarized. Finally, we also discuss the limitations and challenges faced by AIGC and propose possible solutions with relevant studies. We hope this review can help readers understand the potential of AIGC in medicine and obtain some innovative ideas in this field.
The limited resources and complexity of medical procedures are common challenges worldwide in the field of medicine, while traditional methods of care require a high level of skill and can be time-consuming. Artificial intelligence (AI) is a new technical science that researches and develops theories, methods, technologies and application systems for simulating, extending and expanding human intelligence. AI is an important driving force of a new round of scientific and technological revolution and industrial change. As a critical and fundamental technique of AI, machine learning (ML) studies how computers can either simulate or implement human learning behaviors to acquire new knowledge or skills and reorganize existing knowledge structures to continuously improve their performance. ML can extract features from training data, learn patterns and quickly process new data in large quantities. Therefore, ML-based methods can automate the analysis of medical data and improve the efficiency of medical tasks. Thus, in the medical field, ML-based image classification, image segmentation and object detection algorithms have been widely used for tasks such as fracture detection and risk prediction [1], cancer period determination [2], and lesion detection [3]. ML is a data-based learning process, and the operational effectiveness of ML algorithms will often depend on the quality and quantity of data. However, the acquisition and labelling of much medical data is a tedious process, and the quality of data has high requirements for medical experts and medical devices. These can lead to significant limitations in the application of ML. At the same time, many medical tasks are not simply data analysis. For example, medical diagnoses involve generating medical reports based on medical data. Meanwhile, generative tasks are becoming more widespread in medicine since the concept of extend reality (XR) has been introduced to the field.
With the rise of AI generation models, such as generative pre-training transformer (GPT) [4] and DALL-E [5], artificial intelligence generated content (AIGC) has started to gain widespread attention. Researchers have also generated interest and ideas for applying AIGC to various challenges in various fields, and medicine is one of the key application areas. AIGC is a kind of content with corresponding characteristics generated by generative AI based on various forms of manual instructions and guidance. Generative AI can produce AI-generated content quickly and in large quantities to meet specific demand, which makes it a great method for solving the data generation problems faced in medical AI.
Although AI-generated content has grown rapidly in recent years and is gaining attention in various fields, research into the application of generative AI to various fields is still in its infancy. AI-generated content has a great potential for application in numerous fields, including medicine. For this reason, this paper tries to review the application of AIGC in medicine. The application of generative AI models and AI-generated content in medicine is gradually receiving wider and wider attention, as shown in Figure 1, with the study of the application of generative adversarial networks (GAN) and variational autoencoder (VAE) in medicine as an example. This review begins with an overview of the basic concepts and techniques of AI for generating content. We present generative AI in three categories: text generation models, visual generation models and multimodal generation models. Furthermore, by collecting and researching studies on AI-generated content in medicine, this paper summarizes the application of AI-generated content to various tasks in medicine. There are reviews summarizing the application of generative AI into natural language processing (NLP) tasks in medicine [6]. Instead, we specifically review the current state of research in AI generated content based on the vision generation model (VGM) and the large language model (LLM) in the field of medicine. The VGM generates specific visual data such as images, videos and so on, while the LLM is a kind of AI model with a large number of parameters that can understand and generate human language. Ultimately, based on the aforementioned information, we analyzed the directions and challenges for the development of AI-generated content in the medical field.
Generative AI refers to training a model using a given set of artificial instructions or artificial data containing feature information, so that the trained model can generate data with specific features to meet the requirements based on the input of artificial instructions or artificial data. This generated data is the AI generated content. As shown in Figure 2, generative AI can perform a variety of generative tasks, which can be categorized into prompt-based and autonomously generated tasks based on the conditions of generation, and into unimodal and multimodal tasks based on the relationship between the types of data inputs and outputs.
Text generation models are trained to generate readable text content based on the content and structure of the input data, and are now widely used in dialogue systems, translation systems and other AI systems. Text generation models can be divided into decoder models and encoder-decoder models (Figure 3). Decoder models have been widely used for text generation tasks, while encoder-decoder models make use of contextual information and autoregressive properties to enhance the performance of the model in the task.
GPT is the most common text generation model based on the decoder model. Specifically, GPT is based on the transformer model, which predicts the words in the previous text and generates a coherent text. The later GPT-2 [7], GPT-3 [8] and GPT-4 [9] built on this idea by expanding the model parameters and using a combination of multiple datasets for training. Additionally, many text generation models have been proposed based on the GPT architecture. Gopher [10] used the RSNorm layer to replace the LayerNorm layer in the GPT-3 architecture, while BLOOM [11] applied a full attention network instead of the sparse attention mechanism used in GPT-3. For pre-trained GPT models, human feedback-based reinforcement learning was introduced in InstructGPT [12] to further tune the pre-trained GPT models and ultimately improve the model performance.
Text-to-text transformer (T5) [13] is a common text generation model based on an encoder-decoder structure, which uses a transformer-based encoder and decoder structure to transform the input and output text into a prescribed text format for a variety of text generation tasks. Researchers have developed a number of text generation models based on the T5 model. The switch transformer [14] introduced the idea of "transformation" by referring to a simplified MoE routing algorithm to train T5 models in parallel, while Google's ExT5 [15], which was proposed in 2021, extended the scale of the T5 model to learn more natural language tasks across a larger number of domains. In addition to the T5 model, BART [16], which is another common encoder-decoder model, used a BERT-based bidirectional encoder and a GPT-based autoregressive decoder. Based on BART, DQ-BART [17] used distillation and quantization to reduce the model size while maintaining the original performance of the model.
Visual generation models can generate image data with specific features and content based on the input data, and such models can perform a variety of image generation tasks such as style translation, data enhancement, and so on. Commonly used visual generation models GAN [18], VAE [19], flow [20] and diffusion models [21]. Figure 4 illustrates the underlying architecture of these network models, and this section will briefly introduce these commonly used models for visual generation models and the current state of research.
GAN was an early proposal for a visual generation model and has gained widespread attention. GAN consists of a generator, which generates image data by learning the feature distribution of real data, and a discriminator, which determines whether the input data is real or not based on real data. During the training process of GAN, the discriminator's power gradually improves, while the generator aims to generate pseudo-data that the discriminator judges to be real.
Based on the original GAN model, researchers have performed numerous innovations including introducing the Laplace pyramid framework (LAPGAN [22]), the attention mechanism with spectral normalization (SAGAN [23]), adding network structure constraints (DCGAN [24]), and adding parameter constraints (CoGAN [25]). To deal with complex datasets, researchers have also attempted to increase the scale of deployment of GAN models (BigGAN [26]). For the training process of the GAN, researchers tried to address the problem of training crashes by tuning the number of generators and discriminators (D2GAN [27], GMAN [28], MGAN [29], MAD-GAN [30]). Meanwhile, others have also introduced f-scatter (f-GAN [31]), weight normalization methods (Miyato et al. [32]) and regularization methods (WGAN [33], LS-GAN [34], Che et al. [35]) into the loss function to improve the training stability of the GAN.
Based on variational Bayesian inference, the variational autoencoder learns generated data that is similar to the original data by mapping the data to a probability distribution. The variational autoencoder constructs an implicit variable space based on the statistical characteristics of the input data (including mean and variance) and reconstructs the generated data by randomly sampling the sampled data from the implicit variable space with the same probability distribution. Building on this idea, researchers have proposed jump connections for random sampling processes [36,37,38] to obtain probability distributions from different perspectives. To obtain smooth and representatively powerful implicit spaces, researchers have introduced regularization into encoders [39,40,41]. For large scale images, researchers have introduced a hierarchical network architecture [42].
Flow models map input data into intermediate data by constructing a series of reversible and microscopic mapping relationships, which, in turn, obtains generated data by inverse mapping. Zheng et al. [43] and Hoogeboom et al. [44] introduced convolutional neural networks (CNN) into streaming models. To address the problem of gradient disappearance, RevNets [45] and iRevNets [46] constructed a reversible network structure based on residual links.
The diffusion model is an advanced generative model that has excelled in recent years on generative tasks, which gradually blurs the data by adding noise in multiple stages and then learns its inverse process to generate data based on random noisy data.
Diffusion models can be divided into three main types of models: the denoising diffusion probability model (DDPM) consists of a forward process (i.e., a Markov chain) with a series of noise coefficients determined from a particular model as kernel parameters, and a backward process (i.e., a Gaussian transformation process) with learnable parameters; the score matching formulation (SMFM) aims to solve the original data distribution estimation problem by approximating the gradient of the data; and the score stochastic differential equation model (Score SDE) describes diffusion and denoising fractional matching models based on a uniform continuous form of the stochastic differential equation. Based on the three types of diffusion models, researchers have applied methods including knowledge distillation [47,48], noise scale design [49,50,51,52], dynamic programming [53,54] and a combination with other generative models [55,56,57] to further enhance the performance of the diffusion models.
Multimodal AI for generating content is currently an important research direction in generative AI. The aim of multimodal generative AI is to achieve connections and interactions between data of different modalities.
The most common type of multimodal AI-generated content is text-image-generated content, which can either be based on textual instructions to generate corresponding images or on input images to generate corresponding descriptive text. The text-image generation model mainly uses an encoder-decoder structure, which is based on the aforementioned unimodal generation model, where unimodal encoders or decoders are relatively well established. However, encoders or decoders for extracting information from multimodal data are more complex. The encoder structure of the text-image generation model uses either stitching multimodal data into the encoder (VisualBERT [58], UNITER [59]) or pairing multimodal data through the output of the corresponding unimodal encoder to a cross-alignment based encoder (LXMERT [60], ViLBERT [61]). There are two types of decoders for text-image generation models: text decoders that can use pre-trained large language models (Frozen [62]) and image decoders that can be designed based on the unimodal generation models mentioned in the previous section such as GAN (StyleCLIP [63]), diffusion models (GLIDE [64], Imagen [65]) and so on.
In addition to text-image generation models, multimodal AI generation models include text-audio generation models (AdaSpeech [66], JTAV [67]), text-code generation models (CodeBERT [68], PLBART [69]) and even mind map-text generation models (Grapher [70], GTR-LSTM [71]).
Medical images are one of the key ways of obtaining physiological information about the human body and are the most important type of medical data. The development of medical imaging methods such as computed tomography (CT), magnetic resonance imaging (MRI) and various optical imaging methods have enriched access to medical images and improved the quality of medical images. However, for medical imaging, there are limitations to the quality of medical images due to the physical characteristics of the imaging method. Furthermore, the information from different types of medical images can often be one-sided, and the costs and negative effects (such as high doses of radiation) of the image acquisition process cannot be ignored. The traditional approach to medical image tasks includes the techniques of digital image processing (DIP), which process medical images as 2D discrete signals with basic mathematical methods and cannot complete many generative tasks. To address these issues, AIGC has a great potential in the field of medical imaging due to its generation efficiency, modality and feature transferability. In this section, we discuss AIGC in tasks of medical image reconstruction, medical image translation and medical image data augmentation.
High resolution images contain more information and more detail than lower resolution images. Medical professionals can make more accurate and comprehensive diagnoses and develop more appropriate treatment plans based on high resolution images, which can lead to better outcomes of medical treatment. However, the resolution of medical images is often subject to numerous limitations, such as physical limit constraints on optical imaging, the magnetic throughput and scanning time of the scanner during image acquisition, and considerations for radiation dose. Medical image reconstruction is the generation of corresponding high-resolution images based on low resolution images by means of image generation techniques.
CT is a mainstream medical imaging technique that uses X-rays to image objects layer by layer, thereby allowing for the non-invasive acquisition of structural features within the human body. However, CT is associated with radiation problems; although low-dose CT can mitigate this problem to some extent, it's imaging resolution and quality can be greatly compromised. For this reason, it's a significant task to reconstruct normal-dose CT images based on low-dose CT images. Researchers have experimented with both network structures and training methods based on GAN. Huang et al. [74] designed a U-Net-based discriminator structure and performed adversarial training in the gradient domain of the images, which validated the reliability of the method on abdominal and chest clinical CT image datasets. Li et al. [72] applied noise-encoding transfer learning (NETL) to GAN and achieved excellent results on the low-dose CT image reconstruction AAPM dataset [75]. Based on the feature that the diffusion model can simulate the noise addition process, Gao et al. [73] introduced a recovery network using contextual information to constrain the sampling process in the diffusion model and proposed CoreDiff; the experimental results on the AAPM dataset showed a superior performance of the model.
MRI is the process of detecting the electromagnetic waves emitted by an applied gradient magnetic field to discover the location and type of atomic nuclei that make up the object, from which a picture of the object's internal structure can be drawn. The use of compression-aware methods to acquire CS-MRI scans can improve the speed of MRI image acquisition, but with the expense of image resolution. For this reason, it is also important to reconstruct high-resolution images from CS-MRI scans, and generative AI techniques have great potential for this task. Zhao et al. [76] introduced the swin transformer into a GAN structure and demonstrated the superiority of their generative AI approach by conducting experiments on MRI scans of the brain and knee region. Other generative AI architectures have also received attention. For example, Zhang et al. [77], studied MRI reconstruction tasks using conditional variational autoencoders (cVAE) and validated the effectiveness of their method on the BrainWeb dataset. Luo et al. [78] introduced joint uncertainty estimation based on a diffusion model, the experiments on image reconstruction on brain MRI scans demonstrated the effectiveness of their model.
The generation of high-resolution images based on low resolution medical images is an important task to save costs and to ensure safety while obtaining high quality medical images to ensure medical efficiency. The characteristics of AIGC have led to widespread interest and use in high-resolution medical image reconstruction tasks. Tables 1 and 2 summarize recent studies based on GAN and diffusion models for medical image reconstruction tasks, respectively.
Selected works | Year | Modalities | Organs (Datasets) | Contribution |
Gu et al.[79] | 2020 | CT | Thorax (LUNA 16 Challenge [80], Private) | Introduction of residual whole map attention network |
MR | Brain (Private) | |||
Vasudeva et al. [81] | 2020 | MR | Brain (MICCAI 2013 [82]) Knee (MRNet [83], fastMRI [84]) |
1) Using complex-valued operations to take advantage of complex algebraic structure; 2) Proposing a novel activation function. |
Yuan et al. [85] | 2020 | MR | Brain (MICCAI 2013 [82]) | Combination of the self-attention mechanism and the relative discriminator |
Zehni et al. [86] | 2021 | CT | Head & Lungs | Application of Projection Formation Model |
Gajera et al. [87] | 2021 | CT | Thorax (Kaggle Super Bowl 20171, TCIA [88]) | 1) Combination of a Wasserstein adversarial loss; 2) a pre-trained VGG19 perceptual loss; 3) a Charbonnier distance structural loss. |
Aghabiglou et al. [89] | 2021 | MR | (fastMRI [84]) | Developing a densely connected residual GAN |
Lv et al. [90] | 2021 | MR | Abdomen & Knee | Combination of Parallel Imaging and GAN |
Jiang et al. [91] | 2021 | MR | Multiple | Fusion of the global feature fusion module, including the channel attention module, the self-attention module |
Kyung et al. [92] | 2022 | CT | Head & Neck | 1) Introduction of multi-task discriminator; 2) Non-difference suppression (NDS) loss; 3) Reconstruction consistency (RC) loss. |
Zhou et al. [93] | 2022 | CBCT | Hip | Combination of multiple connection methods with channel attention (CA) and pixel attention (PA) mechanisms |
Huang et al. [74] | 2022 | CT | Abdomen & Chest (AAPM [75]) | 1) Application of a U-Net-based discriminator; 2) Adversarial training in the image gradient domains. |
Yaqub et al. [94] | 2022 | MR | Brain & Knee | Application of transfer learning |
Liu et al. [95] | 2022 | MR | Brain & Knee (MICCAI 2013 [82]) | Application of two end-to-end cascaded U-Net with cross-stage skip connection in generator |
Zhang et al. [96] | 2022 | MR & CT | Multiple | 1) Introduction of a scale-attention SR architecture; 2) Application of two criteria of data preprocessing; 3) Application of perceptual loss previously defined on 2D pre-trained VGG onto 3D medical images |
Li et al. [72] | 2023 | CT | (AAPM [75]) | Application of noise encoding transfer learning |
Zhao et al. [76] | 2023 | MR | Brain (MICCAI 2013 [82], IXI2) Knee (MRNet [1]) |
Application of swin transformer |
Note: 1 https://www.kaggle.com/c/data-science-bowl-2017/data; 2 https://brain-development.org/ixi-dataset |
Selected works | Year | Modalities | Organs (Datasets) | Contribution | |
Chung et al. [97] | 2023 | MR | Knee (fastMRI [84]) | 1) Training a continuous time-dependent score function with denoising score matching; 2) Iteration between the numerical SDE solver and data consistency step to achieve reconstruction. |
|
Güngör et al. [98] | 2023 | MR | Brain (IXI1, fastMRI [84]) | 1) Leveraging a rapid diffusion process with an adversarial mapper for efficient sampling from the diffusion prior; 2) Introduction of inference adaptation |
|
Peng et al. [99] | 2023 | MR | (fastMRI [84], SKM-TEA [100]) | 1) Gradually guiding the reverse-diffusion process with observed k-space signal; 2) Proposing a coarse-to-fine sampling algorithm |
|
Xie et al. [101] | 2023 | MR | Knee (fastMRI [84]) | 1) The diffusion and sampling process are defined in measurement domain rather than image domain; 2) the diffusion process is conditioned on under-sampling mask |
|
Liu et al. [102] | 2023 | CT | Abdomen (AAPM [75]) | Incorporating the pre-trained diffusion model into the denoising framework. | |
Gao et al. [73] | 2023 | CT | (AAPM [75]) | Introduction of a novel restoration network CLEAR-Net to mitigate accumulated errors by constraining the sampling process using contextual information among adjacent slices and calibrating the time step embedding feature using the latest prediction. | |
Gao et al. [103] | 2023 | CT | (AAPM [75]) | a noise estimation network to gradually convert a residual image to a Gaussian distribution based on a Markov chain with a low-dose image as the condition. | |
Cui et al. [104] | 2022 | MR | (fastMRI [84]) | 1) A self-supervised learning score-based diffusion model for the scenario without a fully sampled MRI training set; 2) The corresponding conditioned Langevin Markov chain Monte Carlo (MCMC) sampling for MRI reconstruction. |
|
Xia et al. [105] | 2022 | CT | (AAPM [75]) | Use of a fast ordinary differential equation (ODE) solver for a much-boosted efficient sampling | |
Huang et al. [106] | 2022 | CT | (AAPM [75]) | 1) Combining the low-rank structural-Hankel matrix with the diffusion model to generate the ideal sinogram from the low-dose projection data; 2) Introduction of penalized weighted least-squares (PWLS) and TV to achieve superior image quality. |
|
Note: 1 https://brain-development.org/ixi-dataset |
Different modalities of medical images contain different structural information and physiological characteristics of the human body due to different imaging principles. Therefore, multimodal medical images are of a great significance for the accuracy and comprehensiveness of disease diagnoses and treatments. However, the cost of acquiring multimodal medical images directly from an imaging device is high, so the modal translation of unimodal medical images to obtain multimodal medical images has also become an important task in the field of medical image processing. AIGC has strong modal migration characteristics. Thus, a generative AI model becomes a critical tool for implementing modal translation in medical images.
CT images are essential for radiotherapy, as target depiction and dose calculation must be performed on the CT images. However, the low contrast of soft tissues makes it difficult to depict target areas on CT images for critical organs such as the brain, liver and pelvis in particular. MRI scans have excellent soft tissue resolution and do not produce ionizing radiation. However, MRI scans lack the electron density information that CT images can provide, and thus do not allow for the calculation of radiation dosages. As a result, researchers have proposed aligning MRI scans with CT images to achieve information fusion. However, this method requires both MRI scans and CT images, which increases the economic cost. Generating corresponding CT images based on MRI scans is an important way to address this problem. Generative AI based on a variety of visual generation models has been intensively studied and widely used in such tasks. Zhao et al. [107] designed a hybrid CNN and transformer generator structure based on GAN networks to extract multi-level information of images. Additionally, they introduced feature reconstruction loss, thus ensuring the sensitivity of the network to structural features of the image. Their experiments on the pelvic MR-CT multimodal dataset demonstrated the superiority of their method. On the other hand, Li et al. [108] used MRI and sampled CT information as a prior knowledge embedded in a diffusion model and introduced the null-space measurement inference (N-SMI) module into their inverse inference process for the CT image generation task (Figure 7). Similarly, they demonstrated the performance of their method on the pelvic MR-CT multimodal dataset. Additionally, AIGC is used in other cross-modal medical image generation efforts. Due to the overdose and cost of radioactive tracers, positron emission tomography (PET) imaging is rarely used in routine medical examinations. However, PET has an important role in the treatment of tumors and neurological diseases due to its high specificity and sensitivity. Therefore, Wei et al. [109] used multiple sequences of MRI scans to predict myelin content in PET images and measured changes in myelin content in vivo by conditional flexible self-attentive generative adversarial networks (Figure 7), which are essential for understanding the mechanisms of multiple sclerosis.
In addition to modal transitions between different types of medical images, similar medical images may also have different modalities containing different features or information. For instance, the common modalities of MRI scans include T1, T2 and FLAIR modalities. T1 modalities can better show the anatomical structure of the imaging area, while T2 modalities are more sensitive to tissue lesions. Therefore, it is also important to convert between different modalities in MRI scans. Hu et al. [110] introduced unsupervised domain adaption to perform the modal migration task based on a 2D variational autoencoder (Figure 6) and performed experiments on the BraTS 2019 dataset to generate T1-MRI using T2-MRI and FLAIR-MRI, their method performed excellently. Meng et al. [111] designed a multi-in multi-out conditional score network based on the diffusion model to reverse the diffusion process in the full modal space, thereby using conditional diffusion and a score-based reverse generation process to accomplish cross-modal image generation. Their approach achieved superior results in various modal MRI generation tasks on the BraTS 2019 dataset [114].
Multimodal medical image generation based on modal migration is an important task to assist in improving the efficiency and quality of medical care. Generative AI can learn the features of different modal images and achieve the effect of feature migration. Thus, AIGC has shown an excellent performance in the field of medical image translation. Table 3 shows the latest studies on AIGC for medical image translation based on different kinds of architecture.
Selected works | Year | Modalities (Organs) {Datasets} | Contribution |
Bharti et al. [112] (G) | 2023 | MRI-CT{[113]}, T1 MRI-T2 MRI {[114]} stain translation of pathology images {ANHIR-Challenge1} |
employment of evolutionary computation, multiobjective optimization, and an intelligent selection scheme |
Zhao et al. [107] (G) | 2023 | MRI-CT (pelvic) {Gold Atlas [115]} | 1. Extract multi-level features by CNN and Transformer; 2. The feature reconstruction loss is used to further constrain the image potential features. |
Wei et al. [109] (G) | 2023 | MRI-PET | Design an attention regularization for MS lesion in conditional flexible self-attention GAN (CF-SAGAN) |
Jiang et al. [116] (D) | 2023 | Multimodal MRI (brain) {BraTS2018 [114], IXI2} | 1. Design a bespoke architecture to facilitate diffusion operations in the latent space; 2. Introduction of structural guidance of brain regions in each step of the diffusion process; 3. Propose an approach for adapting condition weights automatically. |
Jiang et al. [116] (D) | 2023 | Multimodal MRI (brain) {BraTS2018 [114], IXI2} | 1. Design a bespoke architecture to facilitate diffusion operations in the latent space; 2. Introduction of structural guidance of brain regions in each step of the diffusion process; 3. Propose an approach for adapting condition weights automatically. |
Ozbey et al. [117] (D) | 2023 | Multi-contrast MRI (brain) {BraTS2018 [114], IXI2} MRI-CT (pelvic) {Gold Atlas [115]} |
Proposing a novel source-conditional adversarial projector |
Peng et al. [118] (D) | 2023 | CBCT-CT (Head & Neck) | Proposing a conditional denoising diffusion probabilistic model (DDPM) that utilizes a time-embedded U-net architecture with residual and attention blocks |
Li et al. [108] (D) | 2023 | MRI-CT (pelvic){Gold Atlas [115]} T2 MRI-FLAIR (brain){BraTS2018 [114]} |
1. integrates both an implicit data distribution prior mapping from MRI to CT images and effective information derived from sparse sampled CT measurements; 2. Enabling the use of the model in challenging conditions(w noise) |
Meng et al. [111] (D) | 2022 | Flair & T1 & T1c & T2 (brain){BraTS2019 [114]} | Employment of a novel multi-in multi-out Conditional Score Network (mm-CSN) |
Lyu et al. [119] (D) | 2022 | MRI-CT (pelvic) {Gold Atlas [115]} | Adaptation of denoising diffusion probabilistic and score-matching models |
Pan et al. [120] (D) | 2022 | MRI-CT (Brain, Prostate) {Private} | 1. Implement diffusion processes with a shifted-window transformer network; 2. Capture the complex structures of high-dimensional data; 3. More stable than GANs |
Bazangani et al. [121] (G) | 2022 | FDG PET-T1 MRI (brain) {ADNI3} | 1. Split learning 3D features with separable convolution in a generative model; 2. A Sobel filter for transmission of the geometrical information; 3. improved the stability of learning with a weighted version of a hybrid loss function. |
Emami et al. [122] (G) | 2022 | FDG PET-T1 MRI (brain) {ADNI3} |
1. Integrate contrastive learning in both the generator and discriminator of a GAN model; 2. Learn two separate embeddings for the source and target domains |
Emami et al. [123] (G) | 2022 | FDG PET-T1 MRI (brain) {ADNI3} | 1. Integrate contrastive learning in both the generator and discriminator of a GAN model; 2. Learn two separate embeddings for the source and target domains |
Hu et al. [110] (V) | 2022 | T1 MRI + FLAIR-T2 MRI (brain) {BraTS2019 [114]} | Proposing an efficient 2D variational-autoencoder approach to perform unsupervised domain adaptation (UDA) in a 3D manner. |
Note: 1 https://anhir.grand-challenge.org/Data/ 2 https://brain-development.org/ixi-dataset 3 https://www.adni-info.org/ The letter after the related works represents which model the method is based on: (D): Diffusion Model, (G): Generative Adversarial Network (GAN), (V): Variational Autoencoder (VAE) |
The acquisition of medical images is generally costly, and the application of AI to many medical tasks such as medical diagnoses require large amounts of medical image data for training, this making the task of generating data from medical images critical to the application of AI in the medical field. Data augmentation of medical image is based on a small amount of existing medical image data to generate artificial data with similar distributions and features. While traditional geometric and intensity transforms can certainly accomplish this process, the distribution of data generated by these traditional methods is very limited. The distributed feature learning capability and diversity of AI generated content makes generative AI techniques of great value for medical data augmentation.
Diagnoses based on medical images include the detection of medical image lesion areas. However, the number of lesion images is often limited, which can have a significant impact on the training and performance of the AI. Thus, the synthesis of lesion images based on normal images is one of the important directions for the application of AIGC in the field of medical data augmentation. Generative adversarial networks applying self-attentive mechanisms have been widely used in lesion image generation tasks. Abdelhalim et al. [123] used this approach to generate fine-grained skin lesion images while Ambita et al. [124] applied this type of network to synthesize CT scan images of COVID-19. Hajij et al. [125] used realNVP, which is a commonly used normalizing flow model, to demonstrate its effectiveness in medical image data augmentation tasks on a CXR dataset [126].
Microscopic studies of diseased tissue by pathologists have been the cornerstone of cancer diagnoses and predictions. While deep learning methods have made significant progress in recent years in the analysis of pathological tissue images, the generation of high-quality pathological images by AI can further expand the volume of data, and thus facilitate the application of deep learning methods. Moghadam et al. [127] proposed the use of a diffusion probability model for histopathology image generation and improved the performance of the model using a method called color normalization. They demonstrated the effectiveness of their model by testing it on low-grade glioma images from the TCGA dataset [3].
Medical image data augmentation is an important task to facilitate the development of medical AI, and AIGC shows a great potential in this task with its diversity, high speed of generation and feature learning. Researchers have applied AIGC to medical image data augmentation tasks in a variety of modalities, as shown in Table 4.
Selected works | Year | Modalities (Organs) {Datasets} | Contribution |
Pan et al. [130] (D) | 2023 | X-ray(Chest) {NIH [131]} MRI(Heart) {ACDC [132]} CT(Pelvic) {Private} CT(Abdomen) {BTCV [133]} | Propose a U-Swin-transformer network to perform the 2D denoising diffusion process |
Moghadam et al. [127] (D) | 2023 | Histopathology Images (Low grade gliomas) {TCGA[129]} | 1. Firstly propose exploiting diffusion probabilistic models to generate synthetic histopathology images; 2. Introduction of color normalization. |
Zhang et al. [134] (G) | 2023 | Ultrasound (Thyroid) {[135]} | 1. Propose a Reference-guided Module to select a finite number of structural references within the dataset by K-Means; 2. Propose a Texture Fuzzy integral Module (TFM) for texture uncertainty; 3. Propose a Structure Fuzzy integral Module (SFM) for structure uncertainty. |
Guo et al. [136] (G) | 2023 | {Private} | Mitigate the issue of mode collapse and convergence failure in learning visually realistic medical images |
Kim et al. [137] (D) | 2022 | MRI (Heart) {ACDC [132]} | 1. Propose a diffusion deformable model for 4D medical image generation; 2. Provide non-rigid continuous deformation of the source image toward the target. |
Pinaya et al. [138] (D) | 2022 | T1w MRI (Brain) {Self-made1} | Combination of diffusion models with compression models following the architecture of Latent Diffusion Models (LDM) |
Zhang et al. [135] (G) | 2022 | Ultrasound (Thyroid) {Described in the paper} | 1. Propose Progressive Texture Generative Adversarial Network (PTGAN) that integrates the repair processof structure and texture; 2. Propose An Image Data Augmentation Strategy based on Mask-Reconstruction (MR-IDAS) |
Abdelhalim et al. [123] (G) | 2021 | Dermoscopic image (Skin Lesion) {HAM10000 [139]} | 1. Application of a stabilized training procedure forincreased stability of training behavior; 2. Apply the Two-Timescale Update Rule (TTUR) to Self-attention Progressive Growing GAN (SPGGAN), with classical Domain Adaptation (DA). |
Note: 1 They made a synthetic dataset and their dataset is available at three following links: Academics Torrents (https://academictorrents.com/details/63aeb864bbe2115ded0aa0d7d36334c026f0660b) FigShare (https://figshare.com/), HDRUK Gateway (https://www.healthdatagateway.org/) The letter after the related works represents which model the method is based on. (D): Diffusion Model, (G): Generative Adversarial Network (GAN) |
Medical text is an important carrier of medical data. Medical texts include medical diagnostic reports, medical research reports, medical terminology and so on. These kinds of medical text are also important elements in various medical tasks including medical diagnoses, medical education, doctor-patient communication, etc. The task of generating medical texts is important as the acquisition of various medical texts requires a lot of time and effort, which is a great challenge for medical resources. Medical texts are often generated based on inputs such as medical images, contents of doctor-patient dialogues, etc., for goals such as diagnoses, summaries, explanations, etc. Based on all kinds of demands, the LLM can quickly and automatically generate various types of textual data. Thus, the LLM has received widespread attention and applications in the medical field. [128] Based on studies of various LLMs for medical text generation tasks, as shown in Table 5, AIGC can be applied into many medical domains, while Figure 8 displays the application of generative AI in the tasks of medical text. This section discusses AIGC in data augmentation, medical Q & A and medical summarization in detail.
Selected works | Year | Model name | LLMs | Datasets | Tasks |
Luo et al. [165] | 2022 | BioGPT | GPT [4] | BC5CDR [166], KD-DTI [167], DDI [168] | End-to-End Relation Extraction |
PubMedQA [146] | Question Answering | ||||
HOC [169] | Document Classification | ||||
(Self-created) | Text Generation | ||||
Venigalla et al. [170] | 2022 | BioMedLM | GPT [4] | MedQA [143], PubMedQA [146], BioASQ [171] | Question Answering |
Singhal et al. [149] | 2022 | MedPaLM | PaLM [150] | MedQA [143], MedMCQA [144], PubMedQA [146], LiveQA [147], MedicationQA [148], MMLU [145] | Question Answering |
Yuan et al. [164] | 2022 | BioBART | BART [15] | CovidDialog1 | Dialogue |
iCliniq, HealthCareMagic, MeQSum [162], MEDIQA-ANS, MEDIQA-QS, MEDIQA-MAS [172] | Summarization | ||||
MedMentions [173], BC5CDR [166], NCBI [174], COMETA [175], AskAPatient[176] | Entity linking | ||||
ShARe13 [177], ShARe14 [178], CADEC [179], GENIA [180] | Named entity recognition | ||||
Dai et al. [141] | 2023 | AugGPT | ChatGPT6 | Symptoms2, PubMed20K | Data augmentation |
Li et al. [181] | 2023 | ChatDoctor | LLaMA [152] | HealthCareMagic-100k3 | Patient-doctor Interactions |
Toma et al. [182] | 2023 | Clinical Camel | LLaMA [152] | ShareGPT4, MedQA [143], (Clinical Articles) | Clinical note, multi-step clinical management, standardized alignment questions |
Wang et al. [183] | 2023 | ClinicalGPT | BLOOM [10] | cMedQA2 [184], cMedQA-KG5, MD-EHR, MedQA [143], MedDialog [161] | Question answering, medical diagnosis, medical record analysis |
Lai et al. [185] | 2023 | KEBLM | BioBERT [186] SciBERT [187] | PubMedQA [146] | Question answering |
NCBI [174], BC5CDR [166], COMETA [175] | Entity linking | ||||
MedNLI [188] | Natural language inference | ||||
Wu et al. [151] | 2023 | PMC-LLaMA | LLaMA [152] | S2ORC [153], PubMedQA [146], MedMCQA [144], MedQA [143] | Question answering |
Li et al. [142] | 2023 | PULSAR | FlanT5 [189] | BioNLP Task 1A [190] | Automatic summarization problems from the provider's progress notes during hospitalization. |
Ma et al. [191] | 2023 | ImpressionGPT | ChatGPT6 | MIMIC-CXR [156] OpenI [157] |
Derive diagnostic impression from radiology findings |
Zhou et al. [158] | 2023 | SkinGPT-4 | MiniGPT-4 [160] | SKINCON [159], Dermnet (Private) | Diagnosis of skin diseases |
Thawkar et al. [154] | 2023 | XrayGPT | Vicuna [155] | MIMIC-CXR [156], OpenI [157] | Analyze and Answer open-ended questions about chest radiographs |
Note: 1 https://github.com/UCSD-AI4H/COVID-Dialogue. 2 https://www.kaggle.com/datasets/paultimothymooney/medicalspeech-transcription-and-intent 3 https://www.healthcaremagic.com/ 4 https://sharegpt.com/ 5 http://cmekg.pcl.ac.cn, https://github.com/baiyang2464/chatbot-base-on-Knowledge-Graph, https://github.com/zhihao-chen/QASystemOnMedicalGraph 6 https://openai.com/blog/chatgpt |
Access to text data, especially medical text data, is not so easy due to privacy, ethical, and security reasons. In turn, the AI model performance is affected by the amount of training data. Thus, few-shot learning is an important method for training AI models. Data augmentation is the key technique for small sample learning, which can generate a large amount of data for training based on a small amount of existing data by transforming it. The high sensitivity of medical texts makes data augmentation significant in medical text processing tasks.
AIGC enables medical text data augmentation, which in turn assists AI models in accomplishing a series of downstream tasks related to medical texts, such as text categorization, text extraction, and even other text generation tasks. Inspired by InstructGPT, Dai et al. [141] applied the reinforcement learning from human feedback (RLHF) method into ChatGPT to augment medical text and further assist in improving the performance of the text categorization task. Li et al. [142] used BioMedLM, which is a medical large language model based on GPT, as a data augmentation model to expand the corresponding medical text according to the corresponding generalized content. Additionally, BioMedLM assisted PULSAR, which is a large language model they designed based on FlanT5, in the task of generalizing physical problems based on medical records. Data augmentation of medical text is an important task for developing AI models in the medical field, as well as an important basis for applying the LLM to assist healthcare.
Medical question answering are tasks that automatically answer corresponding questions given a specific context. Medical question answering is one of the most important tasks in the field of medicine. Medical question answering can be categorized into two types: direct question and answer (Q & A) and reading comprehension. Direct Q & A refers to the generation of corresponding responses based on the content of the questioner's question and the internal knowledge of the model, such as the explanation of medical terms. On the other hand, reading comprehension analyzes and interprets based on the provided material (e.g., analyses of medical conditions based on medical images, explanations of problems related to clinical notes, etc). Medical question answering is based on a large amount of medical knowledge and information. However, the human learning ability is limited. So completing a variety of medical quiz tasks requires a large number of highly qualified medical personnel. Combining with various carriers of medical knowledge, LLMs can efficiently learn medical knowledge and information, then complete various medical question answering tasks.
Various forms of datasets provide ample learning resources for the LLMs. The sources of datasets include medical exams, medical papers, and medical market surveys. MedQA [143] uses medical questions from the US Medical License Exam (USMLE) as a vehicle for medical knowledge, while MedMCQA [144] contains 194k multiple-choice questions from Indian medical entrance examinations (i.e., AIIMS/NEET) to store medical knowledge. Similarly, with the contents of exams, MMLU [145] contains 57 domains of medical knowledge. PubMedQA [146] designed 1k expert-labeled Q & A using the content of abstracts on PubMed as context. On the other hand, LiveQA [147], MedicationQA [148], and HealthSearchQA [149] are datasets based on medical knowledge and questions frequently sought by users.
Various medical knowledge datasets in conjunction with the LLMs generate AIGC, which plays a great role in medical question answering. Singhal et al. [149] showed encouraging results on medical question answering based on PaLM [150] using a variety of datasets as training and test data. The LLMs can actually extract medical knowledge from these datasets and generate compliant AIGCs in medical question answering. Wu et al. [151] designed PMC-LLaMA using the large language model LLaMA [152], and they used the dataset S2ORC [153], which contains medical English papers, to train the large language model, and tested the model's performance in medical Q & A on PubMedQA, MedMCQA, and MedQA datasets. The model's excellent experimental performance demonstrated the reliability of AIGC in medical Q & A, as well as the ability of the LLM to extract knowledge information from data in different modalities.
Some research on medical applications based on the LLMs will focus on more specific medical domains. Thawkar et al. [154] proposed XrayGPT, which is a medical generative AI for radiology. Their model was based on Vicuna [155], which used the MIMIC-CXR dataset [156] that contained chest radiology images and corresponding medical reports, and OpenI [157] for training. XrayGPT ultimately accomplished the diagnosis and interpretation of radiology images. Following this, Zhou et al. [158] used a combination of SKINCON [159], which is a dataset that pairs skin lesion images with annotations, Dermnet, which is a dataset that pairs skin lesion images with corresponding disease categories, and a private dataset that combines skin lesion images with physicians' descriptions to train MiniGPT-4 [160]. Additionally, they designed SkinGPT-4, which is a large language model that can diagnose, analyze and answer questions about skin diseases based on pictures.
AIGC plays an important role and excels in automated medical Q & A systems. The automation and high quality of medical Q & A can assist patients in obtaining the appropriate diagnosis faster and more accurately, as well as more timely and adequate communication during the medical process. Additionally, medical Q & A can provide students with an efficient way to acquire knowledge and resolve doubts, which is meaningful for enhancing the efficiency of medical education and alleviating the pressure on educational resources.
Text summarization is a common natural language processing task. A text summarization task is essentially the generation of short, easy-to-understand text based on the input of long text. In medicine, the task of summarizing medical texts is difficult due to the diversity and complexity of medical language expressions and terminology. For this reason, AIGC in medical text summarization tasks has received increased attention.
Doctor-patient dialogues are an important foundation for physicians to make medical judgements. For patients, they are critical way to obtain medical information. However, dialogues are often long and can contain redundancies in their content. Thus, summarization of the doctor-patient dialogue is significant for improving the efficiency of healthcare. For this task, a large number of datasets can provide a basis for the application of the LLM in this task. The iCliniq and HealthCareMagic datasets extract a large number of doctor-patient dialogues from the MedDialog dataset [161] and combine them with the corresponding summarizations. Questions from patients are often verbose due to their lack of medical knowledge. Therefore, Ben Abacha et al. constructed the MeQSum dataset [162] using medical experts' summarizations regarding 1000 patient health questions selected from a dataset distributed by U.S. National Library of Medicine. To assist patients in understanding answers to medical questions, Savery et al. proposed the MEDIQA-ANS dataset [163], which contains the corresponding answers of 156 medical questions and their corresponding summarizations. Yuan et al. [164] designed a medical large language model, BioBART, based on the LLM, BART [15], and trained the model using a series of datasets including those mentioned above. Their model provided a good summarization for the content of doctor-patient dialogues.
A medical report is the analysis of medical data and has an important role in the healthcare process. Medical reports will often contain a findings section describing the detailed content of observations, as well as an impression section containing representational content in the observations (e.g., abnormal areas of the image). Summarizing the impression section of medical reports is a crucial step. However, this task is very time-consuming and requires a high level of physician experience. As a result, automatic impression generation (AIG) has attracted a lot of attention from researchers. Hu et al. [191] designed a kind of graph encoder based on the idea of graph neural networks (GNN) to exploit both findings and extra knowledge. Their proposed model achieved excellent performance on the MIMIC-CXR and OpenI datasets. Ma et al. [192] created dynamic indications by a similarity search. Based on this, they performed iterative optimization for the LLM and further used domain-specific data to fine-tune it. They proposed ImpressionGPT based on ChatGPT using the aforementioned approach. For the summarization of radiology reports, their model achieved state-of-the-art (SOTA) performance on the MIMIC-CXR and OpenI datasets.
Literature is an important carrier of medical knowledge, but it's not easy to extract valid information from it and understanding the information due to its long length and complex structure. AIGC can perform well in the task of summarizing literature. Pang et al. [193] proposed a model for text summarization by combining an encoder designed based on the idea of top-down and bottom-up inferences with a decoder based on the initialization by BART. Their model performed excellently on multi-domain text summarization datasets including literature datasets such as PubMed and arXiv. Due to the excellent performance of AIGC in multi-domain text summarization, some studies have also applied the LLM into medical literature summarization. Frisoni et al. [194] introduced the idea of GNNs into the large language model BART [15] and used reinforcement learning to optimize the network. Additionally, they proposed Cogito Ergo Summ, which is the first single-document abstractive summarization model applied to the biomedical domain. They achieved a near SOTA performance on CSDR [195] with fewer parameters.
Medical text summarization is of a great significance in the field of medicine. For example, the summarization of doctor-patient dialogues not only assists the doctor in making a diagnosis, but also makes it easier for the patient to understand his or her condition. The summarization of medical reports can improve the efficiency of doctors in diagnosing, treating and following up with patients. Besides, the summarization of medical literature facilitates information retrieval while making it possible to increase the utilization of medical education resources. With the development and application of the LLM, AIGC can automatically and quickly achieve medical text summarization, which is useful for many aspects of the medical field.
Based on the current state of research, AIGC can play a great role in various medical tasks. However, there are still many limitations and challenges in widely applying AIGC in medicine, and this chapter will specifically discuss the current problems and potential solutions for the application of AIGC in medicine.
Generative AI can generate a large quantity of medical contents. However, they are more like deductions or guesses. Thus, it's possible that AIGC seems plausible but is not correct. Especially for medical image generation, the acquisition of medical images is generally based on certain biological and physical principles, whereas AIGC simply generates images by numerical computation to reason. The difference in generation methods makes AIGC not always reflect the detailed information of the imaged area as correctly as real images. In addition, AIGC has a poor interpretability compared to real medical images. Furthermore, the LLMs sometimes face similar problems when generating medical text. Hallucination of AIGC may provide fake or wrong information for doctors and patients, which can lead to severe and even life-threatening medical problems. A lack of interpretability makes doctors not believe AIGC in some important medical cases. For this reason, hallucinations and interpretability strongly limit the broad application of AIGC in medicine. For this problem, RLHF can be a possible solution. RLHF is proposed to finetune the AI agent from ordinary people by allowing them to provide social feedback, such as evaluative feedback, advice or instruction. However, there are still some challenges for RLHF on obtaining human feedback, such as designing reward models and policies [196].
With more studies and findings, medical knowledge changes rapidly over time (i.e., it expands and is updated with deeper studies). In particular, the development of medical knowledge, including new terminologies, updating medical concepts, innovative schemes for medical treatment, new standards for medical diagnosis and so on, can be much faster. In order to generate correct and more credible medical content, AIGC needs to not only retain existing knowledge, but also go on to incorporate new knowledge. To address this problem, generative AI can build on existing models to continue learning about new knowledge [197]. In some cases, continual learning does not perform as well as learning from scratch [198]; however, the costs of learning from scratch are very high. As a result, for the application of AIGC, it is important to clarify the applicable circumstances of continuous learning and learning from scratch, or to identify the corresponding appropriate learning scenarios for the different modules in the model.
The AI models for generating AIGC generally have a large scale, including a large number of training parameters, a large dataset and a high demand for computational resources. The high complexity of medical generative tasks and the large amount of knowledge increases the scale of generative AI models applied to medicine. However, large-scale AI models come with significant time and resource costs and higher deployment requirements. Thus, one of the key challenges in applying AIGC to medicine is a model scale setting that ensures the model performance while avoiding resource wastage. Hoffmann et al. [199] proposed a formal scaling law to predict the model performance based on the size of parameters and datasets. Based on the validation of this law, Aghajanyan et al. [200] investigated the relationship between different training tasks under multimodal training. These studies provided valuable insights into controlling the complexity of large models.
AIGC often suffers from data bias problems [201]. For instance, models trained for English text can generate content that better matches English features. This problem is even more pronounced in medicine. People of different races and countries may have different physiological characteristics and medical standards due to geographical factors and differences in living habits. Additionally, gender has a greater impact on the medical process. However, existing datasets are difficult to guarantee complete equalization and will not contain detailed information due to privacy concerns. This may lead to a significant bias in the AIGC in favor of data that accounts for a larger proportion of the dataset. This kind of bias will seriously affect the accuracy of medical diagnoses, the efficiency of medical treatment, etc., which is a great challenge for the application and promotion of medical AIGC. To tackle such a problem for GNNs, Dong et al. [202] designed a novel bias metric and proposed a model-agnostic debiasing framework named EDITS. A similar idea can be applied into AIGC for data bias.
AIGC has been rapidly developing in various fields, and at the same time, faces various ethical and legal issues. Medical information, both text and images, is important information that involves personal privacy and effects an individual's life and health. One of the key ethical and legal concerns faced by AIGC in medicine is illegal dissemination and utilization of fake information. The collaboration of Deepfake recognition technology [203] and generative AI provides an important way to deal with this problem. Additionally, AIGC in medicine faces privacy protection challenges. In response to this problem, Federated Learning is an effective solution that helps multiple organizations use and model data while meeting the requirements of user privacy protection, data security, and government regulations [204,205]. It's also significant to consider the privacy risks and existing solutions in the whole life cycle of the AI, including project planning, data collection, data preparation and model deployment [206]. Most importantly, the generation and application of AIGC needs to be controlled by established laws.
This review focuses on the current state of research on the application of AIGC in medicine. First, we briefly described generative AI models for generating AIGC from the perspective of different modalities. On this basis, this paper summarized the innovative research work in recent years on applying AIGC to various medical tasks from two aspects: medical image tasks and medical text tasks- while focusing on their datasets, methodologies, and innovations. Finally, we discussed the limitations and challenges faced by AIGC in the medical field, and proposed potential solutions and research directions in view of relevant studies. We hope that this review can provide readers with a better understanding of AIGC in medicine and inspire ideas for the further application of AIGC in the medical field. We discussed most common application of AIGC in the medical field in this review, and would further explore and analyze AIGC in medicine.
The authors declare they have not used Artificial Intelligence (AI) tools in the creation of this article.
This work was supported by the Key Research and Development Plan of Ningxia Hui Autonomous Region (Grant No.2023BEG03043), the Key Research and Development Plan of Ningxia Hui Autonomous Region (Grant No.2023BEG02035) and the Shanghai Jiao Tong University School of Medicine Affiliated Renji Hospital Baoshan Branch Fund (Grant No. 2023-rbcxjj-005).
The authors declare there is no conflict of interest.
[1] |
M. E. Sahin, Image processing and machine learning‐based bone fracture detection and classification using X‐ray images, Int. J. Imaging Syst. Technol., 33 (2023), 853–865. https://doi.org/10.1002/ima.22849 doi: 10.1002/ima.22849
![]() |
[2] |
Z. Zhao, Y. Tian, Z. Yuan, P. Zhao, F. Xia, S. Yu, A machine learning method for improving liver cancer staging, J. Biomed. Inf., 137 (2023), 104266. https://doi.org/10.1002/ima.22849 doi: 10.1002/ima.22849
![]() |
[3] |
S. Maurya, S. Tiwari, M. C. Mothukuri, C. M. Tangeda, R. N. S. Nandigam, D. C. Addagiri, A review on recent developments in cancer detection using Machine Learning and Deep Learning models, Biomed. Signal Process. Control, 80 (2023), 104398. https://doi.org/10.1016/j.bspc.2022.104398 doi: 10.1016/j.bspc.2022.104398
![]() |
[4] | A. Radford, K. Narasimhan, T. Salimans, I. Sutskever, Improving language understanding by generative pre-training, OpenAI, 2018. |
[5] | A. Ramesh, P. Dhariwal, A. Nichol, C. Chu, M. Chen, Hierarchical text-conditional image generation with CLIP latents, preprint, arXiv.2204.06125. https://doi.org/10.48550/arXiv.2204.06125 |
[6] |
A. J. Thirunavukarasu, D. S. J. Ting, K. Elangovan, L. Gutierrez, T. F. Tan, D. S. W. Ting, Large language models in medicine, Nat. Med., 29 (2023), 1930–1940. https://doi.org/10.1038/s41591-023-02448-8 doi: 10.1038/s41591-023-02448-8
![]() |
[7] | A. Radford, J. Wu, R. Child, D. Luan, D. Amodei, I. Sutskever, Language models are unsupervised multitask learners, OpenAI blog, 1 (2019), 9. |
[8] | T. Brown, B. Mann, N. Ryder, M. Subbiah, J. D. Kaplan, P. Dhariwal, et al., Language models are few-shot learners, Adv. Neural Inf. Process. Syst., 33 (2020), 1877–1901. |
[9] | S. Bubeck, V. Chandrasekaran, R. Eldan, J. Gehrke, E. Horvitz, E. Kamar, et al., Sparks of artificial general intelligence: Early experiments with gpt-4, preprint, arXiv: 2303.12712. https://doi.org/10.48550/arXiv.2303.12712 |
[10] | J. W. Rae, S. Borgeaud, T. Cai, K. Millican, J. Hoffmann, F. Song, et al., Scaling language models: Methods, analysis & insights from training gopher, preprint, arXiv: 2112.11446. https://doi.org/10.48550/arXiv.2112.11446 |
[11] | T. L. Scao, A. Fan, C. Akiki, E. Pavlick, S. Ilić, D. Hesslow, et al., Bloom: A 176b-parameter open-access multilingual language model, preprint, arXiv: 2211.05100. https://doi.org/10.48550/arXiv.2211.05100 |
[12] | L. Ouyang, J. Wu, X. Jiang, D. Almeida, C. Wainwright, P. Mishkin, et al., Training language models to follow instructions with human feedback, Adv. Neural Inf. Process. Syst., 35 (2022), 27730–27744. |
[13] | C. Raffel, N. Shazeer, A. Roberts, K. Lee, S. Narang, M. Matena, et al., Exploring the limits of transfer learning with a unified text-to-text transformer, J. Machine Learn. Res., 21 (2020), 5485–5551. |
[14] | W. Fedus, B. Zoph, N. Shazeer, Switch transformers: Scaling to trillion parameter models with simple and efficient sparsity, J. Machine Learn. Res., 23 (2022), 5232–5270. |
[15] | V. Aribandi, Y. Tay, T. Schuster, J. Rao, H. S. Zheng, S. V. Mehta, et al., Ext5: Towards extreme multi-task scaling for transfer learning, preprint, arXiv: 2111.10952. https://doi.org/10.48550/arXiv.2111.10952 |
[16] | M. Lewis, Y. Liu, N. Goyal, M. Ghazvininejad, A. Mohamed, O. Levy, et al., Bart: Denoising sequence-to-sequence pre-training for natural language generation, translation, and comprehension, preprint, arXiv: 1910.13461. https://doi.org/10.48550/arXiv.1910.13461 |
[17] | Z. Li, Z. Wang, M. Tan, R. Nallapati, P. Bhatia, A. Arnold, et al., Dq-bart: Efficient sequence-to-sequence model via joint distillation and quantization, preprint, arXiv: 2203.11239. https://doi.org/10.48550/arXiv.2203.11239 |
[18] |
I. Goodfellow, J. Pouget-Abadie, M. Mirza, B. Xu, D. Warde-Farley, S. Ozair, et al., Generative adversarial networks, Commun. ACM, 63 (2020), 139–144. https://doi.org/10.1145/3422622 doi: 10.1145/3422622
![]() |
[19] | D. P. Kingma, M. Welling, Auto-encoding variational bayes, preprint, arXiv: 1312.6114. https://doi.org/10.48550/arXiv.1312.6114 |
[20] | L. Dinh, D. Krueger, Y. Bengio, Nice: Non-linear independent components estimation, preprint, arXiv: 1410.8516. https://doi.org/10.48550/arXiv.1410.8516 |
[21] | Y. Song, S. Ermon, Generative modeling by estimating gradients of the data distribution, Adv. Neural Inf. Process. Syst., 32 (2019). |
[22] | E. L. Denton, S. Chintala, R. Fergus, Deep generative image models using a laplacian pyramid of adversarial networks, Adv. Neural Inf. Process. Syst., 28 (2015). |
[23] | H. Zhang, I. Goodfellow, D. Metaxas, A. Odena, Self-attention generative adversarial networks, in International Conference on Machine Learning, (2019), 7354–7363. |
[24] | A. Radford, L. Metz, S. Chintala, Unsupervised representation learning with deep convolutional generative adversarial networks, preprint, arXiv: 1511.06434. https://doi.org/10.48550/arXiv.1511.06434 |
[25] | M. Liu, O. Tuzel, Coupled generative adversarial networks, Adv. Neural Inf. Process. Syst., 29 (2016). |
[26] | A. Brock, J. Donahue, K. Simonyan, Large scale GAN training for high fidelity natural image synthesis, preprint, arXiv: 1809.11096. https://doi.org/10.48550/arXiv.1809.11096 |
[27] | T. Nguyen, T. Le, H. Vu, D. Phung, Dual discriminator generative adversarial nets, Adv. Neural Inf. Process. Syst., 30 (2017). |
[28] | I. Durugkar, I. Gemp, S. Mahadevan, Generative multi-adversarial networks, preprint, arXiv: 1611.01673. https://doi.org/10.48550/arXiv.1611.01673 |
[29] | Q. Hoang, T. D. Nguyen, T. Le, D. Phung, Multi-generator generative adversarial nets, preprint, arXiv: 1708.02556. https://doi.org/10.48550/arXiv.1708.02556 |
[30] | A. Ghosh, V. Kulharia, V. P. Namboodiri, P. H. Torr, P. K. Dokania, Multi-agent diverse generative adversarial networks, in Proceedings of the IEEE Conference on Computer Vision and Pattern Recognition, (2018), 8513–8521. https://doi.org/10.1109/CVPR.2018.00888 |
[31] | S. Nowozin, B. Cseke, R. Tomioka, f-gan: Training generative neural samplers using variational divergence minimization, Adv. Neural Inf. Process. Syst., 29 (2016). |
[32] | T. Miyato, T. Kataoka, M. Koyama, Y. Yoshida, Spectral normalization for generative adversarial networks, preprint, arXiv: 1802.05957. https://doi.org/10.48550/arXiv.1802.05957 |
[33] | I. Gulrajani, F. Ahmed, M. Arjovsky, V. Dumoulin, A. C. Courville, Improved training of wasserstein gans, Adv. Neural Inf. Process. Syst., 30 (2017). |
[34] |
G. Qi, Loss-sensitive generative adversarial networks on lipschitz densities, Int. J. Comput. Vis., 128 (2020), 1118–1140. https://doi.org/10.1007/s11263-019-01265-2 doi: 10.1007/s11263-019-01265-2
![]() |
[35] | T. Che, Y. Li, A. P. Jacob, Y. Bengio, W. Li, Mode regularized generative adversarial networks, preprint, arXiv: 1612.02136. https://doi.org/10.48550/arXiv.1612.02136 |
[36] | L. Maaløe, M. Fraccaro, V. Liévin, O. Winther, Biva: A very deep hierarchy of latent variables for generative modeling, Adv. Neural Inf. Process. Syst., 32 (2019). |
[37] | A. Vahdat, J. Kautz, NVAE: A deep hierarchical variational autoencoder, Adv. Neural Inf. Process. Syst., 33 (2020), 19667–19679. |
[38] | B. Wu, S. Nair, R. Martin-Martin, L. Fei-Fei, C. Finn, Greedy hierarchical variational autoencoders for large-scale video prediction, in Proceedings of the IEEE/CVF Conference on Computer Vision and Pattern Recognition, (2021), 2318–2328. |
[39] | A. Ramesh, M. Pavlov, G. Goh, S. Gray, C. Voss, A. Radford, et al., Zero-shot text-to-image generation, in International Conference on Machine Learning, (2021), 8821–8831. |
[40] | P. Ghosh, M. S. Sajjadi, A. Vergari, M. Black, B. Schölkopf, From variational to deterministic autoencoders, preprint, arXiv: 1903.12436. https://doi.org/10.48550/arXiv.1903.12436 |
[41] | A. V. D. Oord, O. Vinyals, Neural discrete representation learning, Adv. Neural Inf. Process. Syst., 30 (2017). |
[42] | A. Razavi, A. V. Oord, O. Vinyals, Generating diverse high-fidelity images with vq-vae-2, Adv. Neural Inf. Process. Syst., 32 (2019). |
[43] | G. Zheng, Y. Yang, J. Carbonell, Convolutional normalizing flows, preprint, arXiv: 1711.02255. https://doi.org/10.48550/arXiv.1711.02255 |
[44] | E. Hoogeboom, R. Van Den Berg, M. Welling, Emerging convolutions for generative normalizing flows, in International Conference on Machine Learning, (2019), 2771–2780. |
[45] | A. N. Gomez, M. Ren, R. Urtasun, R. B. Grosse, The reversible residual network: Backpropagation without storing activations, Adv. Neural Inf. Process. Syst., 30 (2017). |
[46] | J. Jacobsen, A. Smeulders, E. Oyallon, i-revnet: Deep invertible networks, preprint, arXiv: 1802.07088. https://doi.org/10.48550/arXiv.1802.07088 |
[47] | T. Salimans, J. Ho, Progressive distillation for fast sampling of diffusion models, preprint, arXiv: 2202.00512. https://doi.org/10.48550/arXiv.2202.00512 |
[48] | E. Luhman, T. Luhman, Knowledge distillation in iterative generative models for improved sampling speed, preprint, arXiv: 2101.02388. https://doi.org/10.48550/arXiv.2101.02388 |
[49] | Z. Kong, W. Ping, On fast sampling of diffusion probabilistic models, preprint, arXiv: 2106.00132. https://doi.org/10.48550/arXiv.2106.00132 |
[50] | A. Q. Nichol, P. Dhariwal, Improved denoising diffusion probabilistic models, in International Conference on Machine Learning, (2021), 8162–8171. |
[51] | D. Kingma, T. Salimans, B. Poole, J. Ho, Variational diffusion models, Adv. Neural Inf. Process. Syst., 34 (2021), 21696–21707. |
[52] | R. San-Roman, E. Nachmani, L. Wolf, Noise estimation for generative diffusion models, preprint, arXiv: 2104.02600. https://doi.org/10.48550/arXiv.2104.02600 |
[53] | D. Watson, W. Chan, J. Ho, M. Norouzi, Learning fast samplers for diffusion models by differentiating through sample quality, in International Conference on Learning Representations, 2021. |
[54] | D. Watson, J. Ho, M. Norouzi, W. Chan, Learning to efficiently sample from diffusion probabilistic models, preprint, arXiv: 2106.03802. https://doi.org/10.48550/arXiv.2106.03802 |
[55] | H. Zheng, P. He, W. Chen, M. Zhou, Truncated diffusion probabilistic models, preprint, arXiv: 2202.09671. https://doi.org/10.48550/arXiv.2202.09671 |
[56] | K. Pandey, A. Mukherjee, P. Rai, A. Kumar, Diffusevae: Efficient, controllable and high-fidelity generation from low-dimensional latents, preprint, arXiv: 2201.00308. https://doi.org/10.48550/arXiv.2201.00308 |
[57] | Q. Zhang, Y. Chen, Diffusion normalizing flow, Adv. Neural Inf. Process. Syst., 34 (2021), 16280–16291. |
[58] | L. H. Li, M. Yatskar, D. Yin, C. Hsieh, K. Chang, Visualbert: A simple and performant baseline for vision and language, preprint, arXiv: 1908.03557. https://doi.org/10.48550/arXiv.1908.03557 |
[59] | L. Zhou, H. Palangi, L. Zhang, H. Hu, J. Corso, J. Gao, Unified vision-language pre-training for image captioning and vqa, in Proceedings of the AAAI Conference on Artificial Intelligence, (2020), 13041–13049. |
[60] | H. Tan, M. Bansal, Lxmert: Learning cross-modality encoder representations from transformers, preprint, arXiv: 1908.07490. https://doi.org/10.48550/arXiv.1908.07490 |
[61] | J. Lu, D. Batra, D. Parikh, S. Lee, Vilbert: Pretraining task-agnostic visiolinguistic representations for vision-and-language tasks, Adv. Neural Inf. Process. Syst., 32 (2019). |
[62] | M. Tsimpoukelli, J. L. Menick, S. Cabi, S. M. Eslami, O. Vinyals, F. Hill, Multimodal few-shot learning with frozen language models, Adv. Neural Inf. Process. Syst., 34 (2021), 200–212. |
[63] | O. Patashnik, Z. Wu, E. Shechtman, D. Cohen-Or, D. Lischinski, Styleclip: Text-driven manipulation of stylegan imagery, in Proceedings of the IEEE/CVF International Conference on Computer Vision, (2021), 2085–2094. https://doi.org/10.1109/ICCV48922.2021.00209 |
[64] | A. Nichol, P. Dhariwal, A. Ramesh, P. Shyam, P. Mishkin, B. McGrew, et al., Glide: Towards photorealistic image generation and editing with text-guided diffusion models, preprint, arXiv: 2112.10741. https://doi.org/10.48550/arXiv.2112.10741 |
[65] |
C. Saharia, W. Chan, S. Saxena, L. Li, J. Whang, E. L. Denton, et al., Photorealistic text-to-image diffusion models with deep language understanding, Adv. Neural Inf. Process. Syst., 35 (2022), 36479–36494. https://doi.org/10.1145/3528233.3530757 doi: 10.1145/3528233.3530757
![]() |
[66] | M. Chen, X. Tan, B. Li, Y. Liu, T. Qin, S. Zhao, et al., Adaspeech: Adaptive text to speech for custom voice, preprint, arXiv: 2103.00993. https://doi.org/10.48550/arXiv.2103.00993 |
[67] | H. Liang, H. Wang, J. Wang, S. You, Z. Sun, J. Wei, et al., JTAV: Jointly learning social media content representation by fusing textual, acoustic, and visual features, preprint, arXiv: 1806.01483. https://doi.org/10.48550/arXiv.1806.01483 |
[68] | Z. Feng, D. Guo, D. Tang, N. Duan, X. Feng, M. Gong, et al., Codebert: A pre-trained model for programming and natural languages, preprint, arXiv: 2002.08155. https://doi.org/10.48550/arXiv.2002.08155 |
[69] | W. U. Ahmad, S. Chakraborty, B. Ray, K. Chang, Unified pre-training for program understanding and generation, preprint, arXiv: 2103.06333. https://doi.org/10.48550/arXiv.2103.06333 |
[70] | I. Melnyk, P. Dognin, P. Das, Knowledge graph generation from text, preprint, arXiv: 2211.10511. https://doi.org/10.48550/arXiv.2211.10511 |
[71] | B. Distiawan, J. Qi, R. Zhang, W. Wang, GTR-LSTM: A triple encoder for sentence generation from RDF data, in Proceedings of the 56th Annual Meeting of the Association for Computational Linguistics, 1 (2018), 1627–1637. |
[72] | M. Li, J. Wang, Y. Chen, Y. Tang, Z. Wu, Y. Qi, et al., Low-dose CT image synthesis for domain adaptation imaging using a generative adversarial network with noise encoding transfer learning, IEEE Trans. Med. Imaging, 2023. |
[73] | Q. Gao, Z. Li, J. Zhang, Y. Zhang, H. Shan, CoreDiff: Contextual Error-Modulated Generalized Diffusion Model for Low-Dose CT Denoising and Generalization, preprint, arXiv: 2304.01814. https://doi.org/10.48550/arXiv.2304.01814 |
[74] |
Z. Huang, J. Zhang, Y. Zhang, H. Shan, DU-GAN: Generative adversarial networks with dual-domain U-Net-based discriminators for low-dose CT denoising, IEEE Trans. Instrum. Meas., 71 (2021), 1–12. https://doi.org/10.1109/TIM.2021.3128703 doi: 10.1109/TIM.2021.3128703
![]() |
[75] | B. Chen, S. Leng, L. Yu, D. Holmes III, J. Fletcher, C. McCollough, An open library of CT patient projection data, in Medical Imaging 2016: Physics of Medical Imaging, 9783 (2016), 330–335. https://doi.org/10.1117/12.2216823 |
[76] |
X. Zhao, T. Yang, B. Li, X. Zhang, SwinGAN: A dual-domain Swin Transformer-based generative adversarial network for MRI reconstruction, Comput. Biol. Med., 153 (2023), 106513. https://doi.org/10.1016/j.compbiomed.2022.106513 doi: 10.1016/j.compbiomed.2022.106513
![]() |
[77] |
C. Zhang, R. Barbano, B. Jin, Conditional variational autoencoder for learned image reconstruction, Computation, 9 (2021), 114. https://doi.org/10.3390/computation9110114 doi: 10.3390/computation9110114
![]() |
[78] | G. Luo, M. Heide, M. Uecker, MRI reconstruction via data driven markov chain with joint uncertainty estimation, preprint, arXiv: 2202.01479. https://doi.org/10.48550/arXiv.2202.01479 |
[79] |
Y. Gu, Z. Zeng, H. Chen, J. Wei, Y. Zhang, B. Chen, et al., MedSRGAN: medical images super-resolution using generative adversarial networks, Multimed. Tools Appl., 79 (2020), 21815–21840. https://doi.org/10.1007/s11042-020-08980-w doi: 10.1007/s11042-020-08980-w
![]() |
[80] |
A. A. A. Setio, A. Traverso, T. D. Bel, M. S. Berens, C. V. D. Bogaard, P. Cerello, et al., Validation, comparison, and combination of algorithms for automatic detection of pulmonary nodules in computed tomography images: the LUNA16 challenge, Med. Image Anal., 42 (2017), 1–13. https://doi.org/10.1016/j.media.2017.06.015 doi: 10.1016/j.media.2017.06.015
![]() |
[81] | B. Vasudeva, P. Deora, S. Bhattacharya, P. M. Pradhan, Co-VeGAN: Complex-valued generative adversarial network for compressive sensing MR image reconstruction, preprint, arXiv: 2002.10523. https://doi.org/10.48550/arXiv.2002.10523 |
[82] | B. Landman, S. Warfield, Diencephalon standard challenge, 2013. https://doi.org/10.7303/syn3270351 |
[83] |
N. Bien, P. Rajpurkar, R. L. Ball, J. Irvin, A. Park, E. Jones, et al., Deep-learning-assisted diagnosis for knee magnetic resonance imaging: development and retrospective validation of MRNet, PLoS Med., 15 (2018), e1002699. https://doi.org/10.1371/journal.pmed.1002699 doi: 10.1371/journal.pmed.1002699
![]() |
[84] | J. Zbontar, F. Knoll, A. Sriram, T. Murrell, Z. Huang, M. J. Muckley, et al., fastMRI: An open dataset and benchmarks for accelerated MRI, preprint, arXiv: 1811.08839. https://doi.org/10.48550/arXiv.1811.08839 |
[85] |
Z. Yuan, M. Jiang, Y. Wang, B. Wei, Y. Li, P. Wang, et al., SARA-GAN: Self-attention and relative average discriminator based generative adversarial networks for fast compressed sensing MRI reconstruction, Front. Neuroinf., 14 (2020), 611666. https://doi.org/10.3389/fninf.2020.611666 doi: 10.3389/fninf.2020.611666
![]() |
[86] | M. Zehni, Z. Zhao, UVTOMO-GAN: An adversarial learning based approach for unknown view X-ray tomographic reconstruction, in 2021 IEEE 18th International Symposium on Biomedical Imaging (ISBI), (2021), 1812–1816. https://doi.org/10.1109/ISBI48211.2021.9433970 |
[87] |
B. Gajera, S. R. Kapil, D. Ziaei, J. Mangalagiri, E. Siegel, D. Chapman, CT-scan denoising using a charbonnier loss generative adversarial network, IEEE Access, 9 (2021), 84093–84109. https://doi.org/10.1109/ACCESS.2021.3087424 doi: 10.1109/ACCESS.2021.3087424
![]() |
[88] | M. A. Gavrielides, L. M. Kinnard, K. J. Myers, J. Peregoy, W. F. Pritchard, R. Zeng, et al., Data from phantom FDA. The cancer imaging archive, Nat. Cancer Inst., Bethesda, MD, USA, Tech. Rep, 2015. |
[89] | A. Aghabiglou, E. M. Eksioglu, MR image reconstruction based on densely connected residual generative adversarial network–DCR-GAN, in Advances in Computational Collective Intelligence: 13th International Conference, ICCCI 2021, Kallithea, Rhodes, Greece, September 29–October 1, 2021, Proceedings 13, (2021), 679–689. https://doi.org/10.1007/978-3-030-88113-9_55 |
[90] |
J. Lv, C. Wang, G. Yang, PIC-GAN: a parallel imaging coupled generative adversarial network for accelerated multi-channel MRI reconstruction, Diagnostics, 11 (2021), 61. https://doi.org/10.3390/diagnostics11010061 doi: 10.3390/diagnostics11010061
![]() |
[91] |
M. Jiang, M. Zhi, L. Wei, X. Yang, J. Zhang, Y. Li, et al., FA-GAN: Fused attentive generative adversarial networks for MRI image super-resolution, Comput. Med. Imaging. Graph., 92 (2021), 101969. https://doi.org/10.1016/j.compmedimag.2021.101969 doi: 10.1016/j.compmedimag.2021.101969
![]() |
[92] | S. Kyung, J. Won, S. Pak, G. Hong, N. Kim, MTD-GAN: Multi-task discriminator based generative adversarial networks for low-dose CT denoising, in International Workshop on Machine Learning for Medical Image Reconstruction, (2022), 133–144. https://doi.org/10.1007/978-3-031-17247-2_14 |
[93] |
H. Zhou, X. Liu, H. Wang, Q. Chen, R. Wang, Z. Pang, et al., The synthesis of high-energy CT images from low-energy CT images using an improved cycle generative adversarial network, Quant. Imaging Med. Surg., 12 (2022), 28. https://doi.org/10.21037/qims-21-182 doi: 10.21037/qims-21-182
![]() |
[94] |
M. Yaqub, F. Jinchao, S. Ahmed, K. Arshid, M. A. Bilal, M. P. Akhter, et al., Gan-tl: Generative adversarial networks with transfer learning for mri reconstruction, Appl. Sci., 12 (2022), 8841. https://doi.org/10.3390/app12178841 doi: 10.3390/app12178841
![]() |
[95] |
X. Liu, H. Du, J. Xu, B. Qiu, DBGAN: A dual-branch generative adversarial network for undersampled MRI reconstruction, Magn. Reson. Imaging, 89 (2022), 77–91. https://doi.org/10.1016/j.mri.2022.03.003 doi: 10.1016/j.mri.2022.03.003
![]() |
[96] |
K. Zhang, H. Hu, K. Philbrick, G. M. Conte, J. D. Sobek, P. Rouzrokh, et al., SOUP-GAN: Super-resolution MRI using generative adversarial networks, Tomography, 8 (2022), 905–919. https://doi.org/10.3390/tomography8020073 doi: 10.3390/tomography8020073
![]() |
[97] |
H. Chung, J. C. Ye, Score-based diffusion models for accelerated MRI, Med. Image Anal., 80 (2022), 102479. https://doi.org/10.1016/j.media.2022.102479 doi: 10.1016/j.media.2022.102479
![]() |
[98] |
A. Güngör, S. U. Dar, Ş. Öztürk, Y. Korkmaz, H. A. Bedel, G. Elmas, et al., Adaptive diffusion priors for accelerated MRI reconstruction, Med. Image Anal., (2023), 102872. https://doi.org/10.1016/j.media.2023.102872 doi: 10.1016/j.media.2023.102872
![]() |
[99] | C. Peng, P. Guo, S. K. Zhou, V. M. Patel, R. Chellappa, Towards performant and reliable undersampled MR reconstruction via diffusion model sampling, in International Conference on Medical Image Computing and Computer-Assisted Intervention, (2022), 623–633. https://doi.org/10.1007/978-3-031-16446-0_59 |
[100] | A. D. Desai, A. M. Schmidt, E. B. Rubin, C. M. Sandino, M. S. Black, V. Mazzoli, et al., Skm-tea: A dataset for accelerated mri reconstruction with dense image labels for quantitative clinical evaluation, preprint, arXiv: 2203.06823. https://doi.org/10.48550/arXiv.2203.06823 |
[101] | Y. Xie, Q. Li, Measurement-conditioned denoising diffusion probabilistic model for under-sampled medical image reconstruction, in International Conference on Medical Image Computing and Computer-Assisted Intervention, (2022), 655–664. https://doi.org/10.1007/978-3-031-16446-0_62 |
[102] | X. Liu, Y. Xie, S. Diao, S. Tan, X. Liang, A diffusion probabilistic prior for low-dose CT image denoising, preprint, arXiv: 2305.15887. https://doi.org/10.48550/arXiv.2305.15887 |
[103] | Q. Gao, H. Shan, CoCoDiff: a contextual conditional diffusion model for low-dose CT image denoising, in Developments in X-Ray Tomography XIV, 2022. |
[104] | Z. Cui, C. Cao, S. Liu, Q. Zhu, J. Cheng, H. Wang, et al., Self-score: Self-supervised learning on score-based models for mri reconstruction, preprint, arXiv: 2209.00835. https://doi.org/10.48550/arXiv.2209.00835 |
[105] | W. Xia, Q. Lyu, G. Wang, Low-Dose CT Using Denoising Diffusion Probabilistic Model for 20× Speedup, preprint, arXiv: 2209.15136. https://doi.org/10.48550/arXiv.2209.15136 |
[106] | B. Huang, L. Zhang, S. Lu, B. Lin, W. Wu, Q. Liu, One sample diffusion model in projection domain for low-dose CT imaging, preprint, arXiv: 2212.03630. https://doi.org/10.48550/arXiv.2212.03630 |
[107] |
B. Zhao, T. Cheng, X. Zhang, J. Wang, H. Zhu, R. Zhao, et al., CT synthesis from MR in the pelvic area using residual transformer conditional GAN, Comput. Med. Imaging. Graph., 103 (2023), 102150. https://doi.org/10.1016/j.compmedimag.2022.102150 doi: 10.1016/j.compmedimag.2022.102150
![]() |
[108] | X. Li, K. Shang, G. Wang, M. D. Butala, DDMM-Synth: A denoising diffusion model for cross-modal medical image synthesis with sparse-view measurement embedding, preprint, arXiv: 2303.15770. https://doi.org/10.48550/arXiv.2303.15770 |
[109] |
W. Wei, E. Poirion, B. Bodini, M. Tonietto, S. Durrleman, O. Colliot, et al., Predicting PET-derived myelin content from multisequence MRI for individual longitudinal analysis in multiple sclerosis, Neuroimage, 223 (2020), 117308. https://doi.org/10.1016/j.neuroimage.2020.117308 doi: 10.1016/j.neuroimage.2020.117308
![]() |
[110] | Q. Hu, H. Li, J. Zhang, Domain-adaptive 3D medical image synthesis: An efficient unsupervised approach, in International Conference on Medical Image Computing and Computer-Assisted Intervention, (2022), 495–504. https://doi.org/10.1007/978-3-031-16446-0_47 |
[111] | X. Meng, Y. Gu, Y. Pan, N. Wang, P. Xue, M. Lu, et al., A novel unified conditional score-based generative framework for multi-modal medical image completion, preprint, arXiv: 2207.03430. https://doi.org/10.48550/arXiv.2207.03430 |
[112] |
V. Bharti, B. Biswas, K. K. Shukla, Qemcgan: quantized evolutionary gradient aware multiobjective cyclic gan for medical image translation, IEEE J. Biomed. Health Inf., 2023. https://doi.org/10.1109/JBHI.2023.3263434 doi: 10.1109/JBHI.2023.3263434
![]() |
[113] |
O. S. Al-Kadi, I. Almallahi, A. Abu-Srhan, A. M. Abushariah, W. Mahafza, Unpaired MR-CT brain dataset for unsupervised image translation, Data Brief, 42 (2022), 108109. https://doi.org/10.1016/j.dib.2022.108109 doi: 10.1016/j.dib.2022.108109
![]() |
[114] |
B. H. Menze, A. Jakab, S. Bauer, J. Kalpathy-Cramer, K. Farahani, J. Kirby, et al., The multimodal brain tumor image segmentation benchmark (BRATS), IEEE Trans. Med. Imaging, 34 (2014), 1993–2024. https://doi.org/10.1109/TMI.2014.2377694 doi: 10.1109/TMI.2014.2377694
![]() |
[115] |
T. Nyholm, S. Svensson, S. Andersson, J. Jonsson, M. Sohlin, C. Gustafsson, et al., MR and CT data with multiobserver delineations of organs in the pelvic area—Part of the Gold Atlas project, Med. Phys., 45 (2018), 1295–1300. https://doi.org/10.1002/mp.12748 doi: 10.1002/mp.12748
![]() |
[116] | L. Jiang, Y. Mao, X. Chen, X. Wang, C. Li, CoLa-Diff: Conditional latent diffusion model for multi-modal MRI synthesis, preprint, arXiv: 2303.14081. https://doi.org/10.48550/arXiv.2303.14081 |
[117] |
M. Özbey, O. Dalmaz, S. U. Dar, H. A. Bedel, Ş. Özturk, A. Güngör, et al., Unsupervised medical image translation with adversarial diffusion models, IEEE Trans. Med. Imaging, 2023. https://doi.org/10.1109/TMI.2023.3290149 doi: 10.1109/TMI.2023.3290149
![]() |
[118] | J. Peng, R. L. Qiu, J. F. Wynne, C. Chang, S. Pan, T. Wang, et al., CBCT-based synthetic CT image generation using conditional denoising diffusion probabilistic model, preprint, arXiv: 2303.02649. https://doi.org/10.48550/arXiv.2303.02649 |
[119] | Q. Lyu, G. Wang, Conversion between CT and MRI images using diffusion and score-matching models, preprint, arXiv: 2209.12104. https://doi.org/10.48550/arXiv.2209.12104 |
[120] | S. Pan, E. Abouei, J. Wynne, T. Wang, R. L. Qiu, Y. Li, et al., Synthetic CT generation from MRI using 3D transformer-based denoising diffusion model, preprint, arXiv: 2305.19467. https://doi.org/10.48550/arXiv.2305.19467 |
[121] |
F. Bazangani, F. J. Richard, B. Ghattas, E. Guedj, FDG-PET to T1 weighted MRI translation with 3D elicit generative adversarial network (E-GAN), Sensors, 22 (2022), 4640. https://doi.org/10.3390/s22124640 doi: 10.3390/s22124640
![]() |
[122] | H. Emami, M. Dong, C. Glide-Hurst, CL-GAN: Contrastive learning-based generative adversarial network for modality transfer with limited paired data, in European Conference on Computer Vision, (2022), 527–542. https://doi.org/10.1007/978-3-031-25066-8_30 |
[123] |
I. S. A. Abdelhalim, M. F. Mohamed, Y. B. Mahdy, Data augmentation for skin lesion using self-attention based progressive generative adversarial network, Expert Syst. Appl., 165 (2021), 113922. https://doi.org/10.1016/j.eswa.2020.113922 doi: 10.1016/j.eswa.2020.113922
![]() |
[124] | A. A. E. Ambita, E. N. V. Boquio, P. C. Naval Jr, Covit-gan: vision transformer forcovid-19 detection in CT scan imageswith self-attention GAN for data augmentation, in International Conference on Artificial Neural Networks, (2021), 587–598. https://doi.org/10.1007/978-3-030-86340-1_47 |
[125] | M. Hajij, G. Zamzmi, R. Paul, L. Thukar, Normalizing flow for synthetic medical images generation, in 2022 IEEE Healthcare Innovations and Point of Care Technologies (HI-POCT), (2022), 46–49. https://doi.org/10.1109/HI-POCT54491.2022.9744072 |
[126] | R. Summers, Nih chest x-ray dataset of 14 common thorax disease categories, NIH Clinical Center: Bethesda, MD, USA, 2019. |
[127] | P. A. Moghadam, S. V. Dalen, K. C. Martin, J. Lennerz, S. Yip, H. Farahani, et al., A morphology focused diffusion probabilistic model for synthesis of histopathology images, in Proceedings of the IEEE/CVF Winter Conference on Applications of Computer Vision, (2023), 2000–2009. https://doi.org/10.1109/WACV56688.2023.00204 |
[128] |
S. Shahriar, S. Allana, M. H. Fard, R. Dara. A survey of privacy risks and mitigation strategies in the artificial intelligence life cycle, IEEE Access, 2023. https://doi.org/10.1109/ACCESS.2023.3287195 doi: 10.1109/ACCESS.2023.3287195
![]() |
[129] |
R. L. Grossman, A. P. Heath, V. Ferretti, H. E. Varmus, D. R. Lowy, W. A. Kibbe, et al., Toward a shared vision for cancer genomic data, N. Engl. J. Med., 375 (2016), 1109–1112. https://doi.org/10.1056/NEJMp1607591 doi: 10.1056/NEJMp1607591
![]() |
[130] |
S. Pan, T. Wang, R. L. Qiu, M. Axente, C. Chang, J. Peng, et al., 2D medical image synthesis using transformer-based denoising diffusion probabilistic model, Phys. Med. Biol., 68 (2023), 105004. https://doi.org/10.1088/1361-6560/acca5c doi: 10.1088/1361-6560/acca5c
![]() |
[131] | X. Wang, Y. Peng, L. Lu, Z. Lu, M. Bagheri, R. M. Summers, Chestx-ray8: Hospital-scale chest x-ray database and benchmarks on weakly-supervised classification and localization of common thorax diseases, in Proceedings of the IEEE Conference on Computer Vision and Pattern Recognition, (2017), 2097–2106. https://doi.org/10.1109/CVPR.2017.369 |
[132] |
O. Bernard, A. Lalande, C. Zotti, F. Cervenansky, X. Yang, P. Heng, et al., Deep learning techniques for automatic MRI cardiac multi-structures segmentation and diagnosis: is the problem solved?, IEEE Trans. Med. Imaging, 37 (2018), 2514–2525. https://doi.org/10.1109/TMI.2018.2837502 doi: 10.1109/TMI.2018.2837502
![]() |
[133] | B. Landman, Z. Xu, J. E. Igelsias, M. Styner, T. R. Langerak, A. Klein, 2015 miccai multi-atlas labeling beyond the cranial vault workshop and challenge, in Proc. MICCAI Multi-Atlas Labeling Beyond Cranial Vault—Workshop Challenge, 2015. |
[134] |
R. Zhang, W. Lu, J. Gao, Y. Tian, X. Wei, C. Wang, et al., RFI-GAN: A reference-guided fuzzy integral network for ultrasound image augmentation, Inf. Sci., 623 (2023), 709–728. https://doi.org/10.1016/j.ins.2022.12.026 doi: 10.1016/j.ins.2022.12.026
![]() |
[135] |
R. Zhang, W. Lu, X. Wei, J. Zhu, H. Jiang, Z. Liu, et al., A progressive generative adversarial method for structurally inadequate medical image data augmentation, IEEE J. Biomed. Health Inf., 26 (2021), 7–16. https://doi.org/10.1109/JBHI.2021.3101551 doi: 10.1109/JBHI.2021.3101551
![]() |
[136] |
K. Guo, J. Chen, T. Qiu, S. Guo, T. Luo, T. Chen, et al., MedGAN: An adaptive GAN approach for medical image generation, Comput. Biol. Med., (2023), 107119. https://doi.org/10.1016/j.compbiomed.2023.107119 doi: 10.1016/j.compbiomed.2023.107119
![]() |
[137] | B. Kim, J. C. Ye, Diffusion deformable model for 4D temporal medical image generation, in International Conference on Medical Image Computing and Computer-Assisted Intervention, (2022), 539–548. https://doi.org/10.1007/978-3-031-16431-6_51 |
[138] | W. H. Pinaya, P. Tudosiu, J. Dafflon, P. F. D. Costa, V. Fernandez, P. Nachev, et al., Brain imaging generation with latent diffusion models, in MICCAI Workshop on Deep Generative Models, (2022), 117–126. https://doi.org/10.1007/978-3-031-18576-2_12 |
[139] |
P. Tschandl, C. Rosendahl, H. Kittler, The HAM10000 dataset, a large collection of multi-source dermatoscopic images of common pigmented skin lesions, Sci. Data, 5 (2018), 1–9. https://doi.org/10.1038/sdata.2018.161 doi: 10.1038/sdata.2018.161
![]() |
[140] | J. Nada, S. Bougleux, J. Lapuyade-Lahorgue, S. Ruan, F. Ghazouani, MR image synthesis using Riemannian geometry constrained in VAE, in 2022 16th IEEE International Conference on Signal Processing (ICSP), (2022), 485–488. https://doi.org/10.1109/ICSP56322.2022.9965357 |
[141] | H. Dai, Z. Liu, W. Liao, X. Huang, Y. Cao, Z. Wu, et al., AugGPT: Leveraging ChatGPT for text data augmentation, preprint, arXiv: 2302.13007. https://doi.org/10.48550/arXiv.2302.13007 |
[142] | H. Li, Y. Wu, V. Schlegel, R. Batista-Navarro, T. Nguyen, A. R. Kashyap, et al., PULSAR: Pre-training with extracted healthcare terms for summarising patients' problems and data augmentation with black-box large language models, preprint, arXiv: 2306.02754. https://doi.org/10.48550/arXiv.2306.02754 |
[143] |
D. Jin, E. Pan, N. Oufattole, W. Weng, H. Fang, P. Szolovits, What disease does this patient have? a large-scale open domain question answering dataset from medical exams, Appl. Sci., 11 (2021), 6421. https://doi.org/10.3390/app11146421 doi: 10.3390/app11146421
![]() |
[144] | A. Pal, L. K. Umapathi, M. Sankarasubbu, Medmcqa: A large-scale multi-subject multi-choice dataset for medical domain question answering, in Conference on Health, Inference, and Learning, (2022), 248–260. |
[145] | D. Hendrycks, C. Burns, S. Basart, A. Zou, M. Mazeika, D. Song, et al. Measuring massive multitask language understanding, preprint, arXiv: 2009.03300. https://doi.org/10.48550/arXiv.2009.03300 |
[146] | Q. Jin, B. Dhingra, Z. Liu, W. W. Cohen, X. Lu, Pubmedqa: A dataset for biomedical research question answering, preprint, arXiv: 1909.06146. https://doi.org/10.48550/arXiv.1909.06146 |
[147] | A. B. Abacha, E. Agichtein, Y. Pinter, D. Demner-Fushman, Overview of the medical question answering task at TREC 2017 LiveQA, in TREC, (2017), 1–12. |
[148] | A. B. Abacha, Y. Mrabet, M. Sharp, T. R. Goodwin, S. E. Shooshan, D. Demner-Fushman, Bridging the gap between consumers' medication questions and trusted answers., in MedInfo, (2019), 25–29. |
[149] | K. Singhal, S. Azizi, T. Tu, S. S. Mahdavi, J. Wei, H. W. Chung, et al., Large language models encode clinical knowledge, preprint, arXiv: 2212.13138. https://doi.org/10.48550/arXiv.2212.13138 |
[150] | A. Chowdhery, S. Narang, J. Devlin, M. Bosma, G. Mishra, A. Roberts, et al., Palm: Scaling language modeling with pathways, preprint, arXiv: 2204.02311. https://doi.org/10.48550/arXiv.2204.02311 |
[151] | C. Wu, X. Zhang, Y. Zhang, Y. Wang, W. Xie, Pmc-llama: Further finetuning llama on medical papers, preprint, arXiv: 2304.14454. https://doi.org/10.48550/arXiv.2304.14454 |
[152] | H. Touvron, T. Lavril, G. Izacard, X. Martinet, M. Lachaux, T. Lacroix, et al., Llama: Open and efficient foundation language models, preprint, arXiv: 2302.13971. https://doi.org/10.48550/arXiv.2302.13971 |
[153] | K. Lo, L. L. Wang, M. Neumann, R. Kinney, D. S. Weld, S2ORC: The semantic scholar open research corpus, preprint, arXiv: 1911.02782. https://doi.org/10.48550/arXiv.1911.02782 |
[154] | O. Thawkar, A. Shaker, S. S. Mullappilly, H. Cholakkal, R. M. Anwer, S. Khan, et al., Xraygpt: Chest radiographs summarization using medical vision-language models, preprint, arXiv: 2306.07971. https://doi.org/10.48550/arXiv.2306.07971 |
[155] | W. Chiang, Z. Li, Z. Lin, Y. Sheng, Z. Wu, H. Zhang, et al., Vicuna: An open-source chatbot impressing gpt-4 with 90%* chatgpt quality, Available from: https://vicuna.lmsys.org. |
[156] |
A. E. Johnson, T. J. Pollard, S. J. Berkowitz, N. R. Greenbaum, M. P. Lungren, C. Deng, et al., MIMIC-CXR, a de-identified publicly available database of chest radiographs with free-text reports, Sci. Data, 6 (2019), 317. https://doi.org/10.1038/s41597-019-0322-0 doi: 10.1038/s41597-019-0322-0
![]() |
[157] |
D. Demner-Fushman, M. D. Kohli, M. B. Rosenman, S. E. Shooshan, L. Rodriguez, S. Antani, et al., Preparing a collection of radiology examinations for distribution and retrieval, J. Am. Med. Inf. Assoc., 23 (2016), 304–310. https://doi.org/10.1093/jamia/ocv080 doi: 10.1093/jamia/ocv080
![]() |
[158] | J. Zhou, X. He, L. Sun, J. Xu, X. Chen, Y. Chu, et al., SkinGPT-4: An interactive dermatology diagnostic system with visual large language model, medRxiv, (2023), 2023–2026. |
[159] | R. Daneshjou, M. Yuksekgonul, Z. R. Cai, R. Novoa, J. Y. Zou, Skincon: A skin disease dataset densely annotated by domain experts for fine-grained debugging and analysis, Adv. Neural Inf. Process. Syst., 35 (2022), 18157–18167. |
[160] | D. Zhu, J. Chen, X. Shen, X. Li, M. Elhoseiny, Minigpt-4: Enhancing vision-language understanding with advanced large language models, preprint, arXiv: 2304.10592. https://doi.org/10.48550/arXiv.2304.10592 |
[161] | G. Zeng, W. Yang, Z. Ju, Y. Yang, S. Wang, R. Zhang, et al., MedDialog: Large-scale medical dialogue datasets, in Proceedings of the 2020 Conference on Empirical Methods in Natural Language Processing (EMNLP), (2020), 9241–9250. https://doi.org/10.18653/v1/2020.emnlp-main.743 |
[162] | A. B. Abacha, Y. Mrabet, M. Sharp, T. R. Goodwin, S. E. Shooshan, D. Demner-Fushman, Bridging the Gap Between Consumers' Medication Questions and Trusted Answers, in MedInfo, (2019), 25–29. |
[163] |
M. Savery, A. B. Abacha, S. Gayen, D. Demner-Fushman, Question-driven summarization of answers to consumer health questions, Sci. Data, 7 (2020), 322. https://doi.org/10.1038/s41597-020-00667-z doi: 10.1038/s41597-020-00667-z
![]() |
[164] | H. Yuan, Z. Yuan, R. Gan, J. Zhang, Y. Xie, S. Yu, BioBART: Pretraining and evaluation of a biomedical generative language model, preprint, arXiv: 2204.03905. https://doi.org/10.48550/arXiv.2204.03905 |
[165] |
R. Luo, L. Sun, Y. Xia, T. Qin, S. Zhang, H. Poon, et al., BioGPT: generative pre-trained transformer for biomedical text generation and mining, Brief. BioInf., 23 (2022), bbac409. https://doi.org/10.1093/bib/bbac409 doi: 10.1093/bib/bbac409
![]() |
[166] |
J. Li, Y. Sun, R. J. Johnson, D. Sciaky, C. Wei, R. Leaman, et al., BioCreative V CDR task corpus: a resource for chemical disease relation extraction, Database, 2016 (2016). https://doi.org/10.1093/database/baw068 doi: 10.1093/database/baw068
![]() |
[167] |
Y. Hou, Y. Xia, L. Wu, S. Xie, Y. Fan, J. Zhu, et al., Discovering drug-target interaction knowledge from biomedical literature, Bioinformatics, 38 (2022), 5100–5107. https://doi.org/10.1093/bioinformatics/btac648 doi: 10.1093/bioinformatics/btac648
![]() |
[168] |
M. Herrero-Zazo, I. Segura-Bedmar, P. Martínez, T. Declerck, The DDI corpus: An annotated corpus with pharmacological substances and drug–drug interactions, J. Biomed. Inf., 46 (2013), 914–920. https://doi.org/10.1016/j.jbi.2013.07.011 doi: 10.1016/j.jbi.2013.07.011
![]() |
[169] |
S. Baker, I. Silins, Y. Guo, I. Ali, J. Högberg, U. Stenius, et al., Automatic semantic classification of scientific literature according to the hallmarks of cancer, Bioinformatics, 32 (2016), 432–440. https://doi.org/10.1093/bioinformatics/btv585 doi: 10.1093/bioinformatics/btv585
![]() |
[170] | A. Venigalla, J. Frankle, M. Carbin, Biomedlm: a domain-specific large language model for biomedical text, MosaicML. Accessed: Dec, 23 (2022), 2. |
[171] | G. Balikas, A. Krithara, I. Partalas, G. Paliouras, Bioasq: A challenge on large-scale biomedical semantic indexing and question answering, in Multimodal Retrieval in the Medical Domain: First International Workshop, MRMD 2015, Vienna, Austria, March 29, 2015, Revised Selected Papers, (2015), 26–39. https://doi.org/10.1007/978-3-319-24471-6_3 |
[172] | A. B. Abacha, Y. M Rabet, Y. Zhang, C. Shivade, C. Langlotz, D. Demner-Fushman, Overview of the MEDIQA 2021 shared task on summarization in the medical domain, in Proceedings of the 20th Workshop on Biomedical Language Processing, (2021), 74–85. https://doi.org/10.18653/v1/2021.bionlp-1.8 |
[173] | S. Mohan, D. Li, Medmentions: A large biomedical corpus annotated with umls concepts, preprint, arXiv: 1902.09476. https://doi.org/10.48550/arXiv.1902.09476 |
[174] |
R. I. Doğan, R. Leaman, Z. Lu, NCBI disease corpus: a resource for disease name recognition and concept normalization, J. Biomed. Inf., 47 (2014), 1–10. https://doi.org/10.1016/j.jbi.2013.12.006 doi: 10.1016/j.jbi.2013.12.006
![]() |
[175] | M. Basaldella, F. Liu, E. Shareghi, N. Collier, COMETA: A corpus for medical entity linking in the social media, preprint, arXiv: 2010.03295. https://doi.org/10.48550/arXiv.2010.03295 |
[176] | N. Limsopatham, N. Collier, Normalising medical concepts in social media texts by learning semantic representation, in Proceedings of the 54th Annual Meeting of the Association for Computational Linguistics (Volume 1: Long papers), (2016), 1014–1023. https://doi.org/10.18653/v1/P16-1096 |
[177] | S. Pradhan, N. Elhadad, B. R. South, D. Martinez, L. M. Christensen, A. Vogel, et al., Task 1: ShARe/CLEF eHealth Evaluation Lab 2013., CLEF (working notes), 1179 (2013). |
[178] | D. L. Mowery, S. Velupillai, B. R. South, L. Christensen, D. Martinez, L. Kelly, et al., Task 2: ShARe/CLEF eHealth evaluation lab 2014, in Proceedings of CLEF 2014, (2014). |
[179] |
S. Karimi, A. Metke-Jimenez, M. Kemp, C. Wang, Cadec: A corpus of adverse drug event annotations, J. Biomed. Inf., 55 (2015), 73–81. https://doi.org/10.1016/j.jbi.2015.03.010 doi: 10.1016/j.jbi.2015.03.010
![]() |
[180] |
J. Kim, T. Ohta, Y. Tateisi, J. I. Tsujii, GENIA corpus—a semantically annotated corpus for bio-textmining, Bioinformatics, 19 (2003), i180–i182. https://doi.org/10.1093/bioinformatics/btg1023 doi: 10.1093/bioinformatics/btg1023
![]() |
[181] | Y. Li, Z. Li, K. Zhang, R. Dan, Y. Zhang, Chatdoctor: A medical chat model fine-tuned on llama model using medical domain knowledge, preprint, arXiv: 2303.14070. https://doi.org/10.48550/arXiv.2303.14070 |
[182] | A. Toma, P. R. Lawler, J. Ba, R. G. Krishnan, B. B. Rubin, B. Wang, Clinical camel: An open-source expert-level medical language model with dialogue-based knowledge encoding, preprint, arXiv: 2305.12031. https://doi.org/10.48550/arXiv.2305.12031 |
[183] | G. Wang, G. Yang, Z. Du, L. Fan, X. Li, ClinicalGPT: Large language models finetuned with diverse medical data and comprehensive evaluation, preprint, arXiv: 2306.09968. https://doi.org/10.48550/arXiv.2306.09968 |
[184] |
S. Zhang, X. Zhang, H. Wang, L. Guo, S. Liu, Multi-scale attentive interaction networks for chinese medical question answer selection, IEEE Access, 6 (2018), 74061–74071. https://doi.org/10.1109/ACCESS.2018.2883637 doi: 10.1109/ACCESS.2018.2883637
![]() |
[185] |
T. M. Lai, C. Zhai, H. Ji, KEBLM: Knowledge-enhanced biomedical language models, J. Biomed. Inf., 143 (2023), 104392. https://doi.org/10.1016/j.jbi.2023.104392 doi: 10.1016/j.jbi.2023.104392
![]() |
[186] |
J. Lee, W. Yoon, S. Kim, D. Kim, S. Kim, C. H. So, et al., BioBERT: a pre-trained biomedical language representation model for biomedical text mining, Bioinformatics, 36 (2020), 1234–1240. https://doi.org/10.1093/bioinformatics/btz682 doi: 10.1093/bioinformatics/btz682
![]() |
[187] | I. Beltagy, K. Lo, A. Cohan, SciBERT: A pretrained language model for scientific text, preprint, arXiv: 1903.10676. https://doi.org/10.48550/arXiv.1903.10676 |
[188] | A. Romanov, C. Shivade, Lessons from natural language inference in the clinical domain, preprint, arXiv: 1808.06752. https://doi.org/10.48550/arXiv.1808.06752 |
[189] | H. W. Chung, L. Hou, S. Longpre, B. Zoph, Y. Tay, W. Fedus, et al., Scaling instruction-finetuned language models, preprint, arXiv: 2210.11416. https://doi.org/10.48550/arXiv.2210.11416 |
[190] | Y. Gao, T. Miller, M. Afshar, D. Dligach, BioNLP Workshop 2023 Shared Task 1A: Problem List Summarization, in Proceedings of the 22nd Workshop on Biomedical Language Processing, 2023. |
[191] | J. Hu, Z. Li, Z. Chen, Z. Li, X. Wan, T. Chang, Graph enhanced contrastive learning for radiology findings summarization, preprint, arXiv: 2204.00203. https://doi.org/10.48550/arXiv.2204.00203 |
[192] | C. Ma, Z. Wu, J. Wang, S. Xu, Y. Wei, Z. Liu, et al., ImpressionGPT: an iterative optimizing framework for radiology report summarization with chatGPT, preprint, arXiv: 2304.08448. https://doi.org/10.48550/arXiv.2304.08448 |
[193] | B. Pang, E. Nijkamp, W. Kryściński, S. Savarese, Y. Zhou, C. Xiong, Long document summarization with top-down and bottom-up inference, preprint, arXiv: 2203.07586. https://doi.org/10.48550/arXiv.2203.07586 |
[194] | G. Frisoni, P. Italiani, S. Salvatori, G. Moro, Cogito ergo summ: abstractive summarization of biomedical papers via semantic parsing graphs and consistency rewards, in Proceedings of the AAAI Conference on Artificial Intelligence, (2023), 12781–12789. https://doi.org/10.1609/aaai.v37i11.26503 |
[195] | Y. Guo, W. Qiu, Y. Wang, T. Cohen, Automated lay language summarization of biomedical scientific reviews, in Proceedings of the AAAI Conference on Artificial Intelligence, (2021), 160–168. https://doi.org/10.1609/aaai.v35i1.16089 |
[196] | S. Casper, X. Davies, C. Shi, T. K. Gilbert, J. Scheurer, J. Rando, et al., Open problems and fundamental limitations of reinforcement learning from human feedback, preprint, arXiv: 2307.15217. https://doi.org/10.48550/arXiv.2307.15217 |
[197] | O. Ostapenko, T. Lesort, P. Rodriguez, M. R. Arefin, A. Douillard, I. Rish, et al., Continual learning with foundation models: An empirical study of latent replay, in Conference on Lifelong Learning Agents, (2022), 60–91. |
[198] | I. Chalkidis, M. Fergadiotis, P. Malakasiotis, N. Aletras, I. Androutsopoulos, LEGAL-BERT: The muppets straight out of law school, preprint, arXiv: 2010.02559. https://doi.org/10.48550/arXiv.2010.02559 |
[199] | J. Hoffmann, S. Borgeaud, A. Mensch, E. Buchatskaya, T. Cai, E. Rutherford, et al., Training compute-optimal large language models, preprint, arXiv: 2203.15556. https://doi.org/10.48550/arXiv.2203.15556 |
[200] | A. Aghajanyan, L. Yu, A. Conneau, W. Hsu, K. Hambardzumyan, S. Zhang, et al., Scaling laws for generative mixed-modal language models, preprint, arXiv: 2301.03728. https://doi.org/10.48550/arXiv.2301.03728 |
[201] | D. Shah, H. A. Schwartz, D. Hovy, Predictive biases in natural language processing models: A conceptual framework and overview, preprint, arXiv: 2301.03728. https://doi.org/10.48550/arXiv.2301.03728 |
[202] | Y. Dong, N. Liu, B. Jalaian, J. Li, Edits: Modeling and mitigating data bias for graph neural networks, in Proceedings of the ACM Web Conference 2022, (2022), 1259–1269. https://doi.org/10.1145/3485447.3512173 |
[203] | H. Zhao, W. Zhou, D. Chen, T. Wei, W. Zhang, N. Yu, Multi-attentional deepfake detection, in Proceedings of the IEEE/CVF Conference on Computer Vision and Pattern Recognition, (2021), 2185–2194. https://doi.org/10.1109/CVPR46437.2021.00222 |
[204] |
A. Brauneck, L. Schmalhorst, M. M. K. Majdabadi, M. Bakhtiari, U. Völker, J. Baumbach, et al., Federated machine learning, privacy-enhancing technologies, and data protection laws in medical research: Scoping review, J. Med. Internet Res., 25 (2023), e41588. https://doi.org/10.2196/41588 doi: 10.2196/41588
![]() |
[205] |
Q. Yang, Y. Liu, T. Chen, Y. Tong, Federated machine learning: Concept and applications, ACM Trans. Intell. Syst. Technol., 10 (2019), 1–19. https://doi.org/10.1145/3298981 doi: 10.1145/3298981
![]() |
[206] |
P. Zhang, M. N. K. Boulos, Generative AI in medicine and healthcare: promises, opportunities and challenges, Future Internet, 15 (2023), 286. https://doi.org/10.3390/fi15090286 doi: 10.3390/fi15090286
![]() |
1. | Xiaoying Tang, Silu Zheng, Zitao Liu, 2024, Chapter 20, 978-3-031-60613-7, 278, 10.1007/978-3-031-60611-3_20 | |
2. | Shao-Feng Wang, Chun-Ching Chen, Explore the driving factors of designers’ AIGC usage behavior based on SOR framework, 2024, 6, 2624-9898, 10.3389/fcomp.2024.1417016 | |
3. | Yaju Liu, Xi Lin, Siyuan Li, Gaolei Li, Qinghua Mao, Jianhua Li, 2024, Towards Multi-Task Generative-AI Edge Services with an Attention-based Diffusion DRL Approach, 979-8-3503-8950-0, 60, 10.1109/SmartCloud62736.2024.00018 | |
4. | Yujie Sun, Dongfang Sheng, Zihan Zhou, Yifei Wu, AI hallucination: towards a comprehensive classification of distorted information in artificial intelligence-generated content, 2024, 11, 2662-9992, 10.1057/s41599-024-03811-x | |
5. | Jian Rao, Mengzhen Xiong, 2023, A New Art Design Method Based on AIGC: Analysis from the Perspective of Creation Efficiency, 979-8-3503-4257-4, 129, 10.1109/ICID60307.2023.10396804 | |
6. | Ruiyang Yin, Xuebin Liu, 2024, Enabling media production with AIGC and its ethical considerations, 979-8-3503-7713-2, 100, 10.1109/EdgeCom62867.2024.00023 | |
7. | Jiesi Guo, Ying Ma, Tingting Li, Michael Noetel, Kewen Liao, Samuel Greiff, Harnessing Artificial Intelligence in Generative Content for enhancing motivation in learning, 2024, 116, 10416080, 102547, 10.1016/j.lindif.2024.102547 | |
8. | Xinrui Wang, Zhenda Liu, Xiao Lin, Yanlong Hong, Lan Shen, Lijie Zhao, A novel paradigm on data and knowledge-driven drug formulation development: Opportunities and challenges of machine learning, 2025, 44, 2452414X, 100796, 10.1016/j.jii.2025.100796 | |
9. | Maite Puerta-Beldarrain, Oihane Gómez-Carmona, Rubén Sánchez-Corcuera, Diego Casado-Mansilla, Diego López-de-Ipiña, Liming Chen, A Multifaceted Vision of the Human-AI Collaboration: A Comprehensive Review, 2025, 13, 2169-3536, 29375, 10.1109/ACCESS.2025.3536095 | |
10. | Tao Zhou, Mengru Wang, Examining Generative AI User Discontinuance from a Dual Perspective of Enablers and Inhibitors, 2025, 1044-7318, 1, 10.1080/10447318.2025.2470280 | |
11. | Jie Wu, Beilin Han, Yihang Zhang, Chuyue Huang, Shengqiang Qiu, Wang Feng, Zhiwei Liu, Chao Zou, Enhancing Bolt Object Detection via AIGC-Driven Data Augmentation for Automated Construction Inspection, 2025, 15, 2075-5309, 819, 10.3390/buildings15050819 | |
12. | Tien-Li Chen, Yu Chuang, Rui Zhu, 2024, The Impact of Different Prompt Structures and Parts of Speech on the Image Generation Results of Generative AI, 979-8-3315-1815-8, 275, 10.1109/ICCR64365.2024.10927462 |
Selected works | Year | Modalities | Organs (Datasets) | Contribution |
Gu et al.[79] | 2020 | CT | Thorax (LUNA 16 Challenge [80], Private) | Introduction of residual whole map attention network |
MR | Brain (Private) | |||
Vasudeva et al. [81] | 2020 | MR | Brain (MICCAI 2013 [82]) Knee (MRNet [83], fastMRI [84]) |
1) Using complex-valued operations to take advantage of complex algebraic structure; 2) Proposing a novel activation function. |
Yuan et al. [85] | 2020 | MR | Brain (MICCAI 2013 [82]) | Combination of the self-attention mechanism and the relative discriminator |
Zehni et al. [86] | 2021 | CT | Head & Lungs | Application of Projection Formation Model |
Gajera et al. [87] | 2021 | CT | Thorax (Kaggle Super Bowl 20171, TCIA [88]) | 1) Combination of a Wasserstein adversarial loss; 2) a pre-trained VGG19 perceptual loss; 3) a Charbonnier distance structural loss. |
Aghabiglou et al. [89] | 2021 | MR | (fastMRI [84]) | Developing a densely connected residual GAN |
Lv et al. [90] | 2021 | MR | Abdomen & Knee | Combination of Parallel Imaging and GAN |
Jiang et al. [91] | 2021 | MR | Multiple | Fusion of the global feature fusion module, including the channel attention module, the self-attention module |
Kyung et al. [92] | 2022 | CT | Head & Neck | 1) Introduction of multi-task discriminator; 2) Non-difference suppression (NDS) loss; 3) Reconstruction consistency (RC) loss. |
Zhou et al. [93] | 2022 | CBCT | Hip | Combination of multiple connection methods with channel attention (CA) and pixel attention (PA) mechanisms |
Huang et al. [74] | 2022 | CT | Abdomen & Chest (AAPM [75]) | 1) Application of a U-Net-based discriminator; 2) Adversarial training in the image gradient domains. |
Yaqub et al. [94] | 2022 | MR | Brain & Knee | Application of transfer learning |
Liu et al. [95] | 2022 | MR | Brain & Knee (MICCAI 2013 [82]) | Application of two end-to-end cascaded U-Net with cross-stage skip connection in generator |
Zhang et al. [96] | 2022 | MR & CT | Multiple | 1) Introduction of a scale-attention SR architecture; 2) Application of two criteria of data preprocessing; 3) Application of perceptual loss previously defined on 2D pre-trained VGG onto 3D medical images |
Li et al. [72] | 2023 | CT | (AAPM [75]) | Application of noise encoding transfer learning |
Zhao et al. [76] | 2023 | MR | Brain (MICCAI 2013 [82], IXI2) Knee (MRNet [1]) |
Application of swin transformer |
Note: 1 https://www.kaggle.com/c/data-science-bowl-2017/data; 2 https://brain-development.org/ixi-dataset |
Selected works | Year | Modalities | Organs (Datasets) | Contribution | |
Chung et al. [97] | 2023 | MR | Knee (fastMRI [84]) | 1) Training a continuous time-dependent score function with denoising score matching; 2) Iteration between the numerical SDE solver and data consistency step to achieve reconstruction. |
|
Güngör et al. [98] | 2023 | MR | Brain (IXI1, fastMRI [84]) | 1) Leveraging a rapid diffusion process with an adversarial mapper for efficient sampling from the diffusion prior; 2) Introduction of inference adaptation |
|
Peng et al. [99] | 2023 | MR | (fastMRI [84], SKM-TEA [100]) | 1) Gradually guiding the reverse-diffusion process with observed k-space signal; 2) Proposing a coarse-to-fine sampling algorithm |
|
Xie et al. [101] | 2023 | MR | Knee (fastMRI [84]) | 1) The diffusion and sampling process are defined in measurement domain rather than image domain; 2) the diffusion process is conditioned on under-sampling mask |
|
Liu et al. [102] | 2023 | CT | Abdomen (AAPM [75]) | Incorporating the pre-trained diffusion model into the denoising framework. | |
Gao et al. [73] | 2023 | CT | (AAPM [75]) | Introduction of a novel restoration network CLEAR-Net to mitigate accumulated errors by constraining the sampling process using contextual information among adjacent slices and calibrating the time step embedding feature using the latest prediction. | |
Gao et al. [103] | 2023 | CT | (AAPM [75]) | a noise estimation network to gradually convert a residual image to a Gaussian distribution based on a Markov chain with a low-dose image as the condition. | |
Cui et al. [104] | 2022 | MR | (fastMRI [84]) | 1) A self-supervised learning score-based diffusion model for the scenario without a fully sampled MRI training set; 2) The corresponding conditioned Langevin Markov chain Monte Carlo (MCMC) sampling for MRI reconstruction. |
|
Xia et al. [105] | 2022 | CT | (AAPM [75]) | Use of a fast ordinary differential equation (ODE) solver for a much-boosted efficient sampling | |
Huang et al. [106] | 2022 | CT | (AAPM [75]) | 1) Combining the low-rank structural-Hankel matrix with the diffusion model to generate the ideal sinogram from the low-dose projection data; 2) Introduction of penalized weighted least-squares (PWLS) and TV to achieve superior image quality. |
|
Note: 1 https://brain-development.org/ixi-dataset |
Selected works | Year | Modalities (Organs) {Datasets} | Contribution |
Bharti et al. [112] (G) | 2023 | MRI-CT{[113]}, T1 MRI-T2 MRI {[114]} stain translation of pathology images {ANHIR-Challenge1} |
employment of evolutionary computation, multiobjective optimization, and an intelligent selection scheme |
Zhao et al. [107] (G) | 2023 | MRI-CT (pelvic) {Gold Atlas [115]} | 1. Extract multi-level features by CNN and Transformer; 2. The feature reconstruction loss is used to further constrain the image potential features. |
Wei et al. [109] (G) | 2023 | MRI-PET | Design an attention regularization for MS lesion in conditional flexible self-attention GAN (CF-SAGAN) |
Jiang et al. [116] (D) | 2023 | Multimodal MRI (brain) {BraTS2018 [114], IXI2} | 1. Design a bespoke architecture to facilitate diffusion operations in the latent space; 2. Introduction of structural guidance of brain regions in each step of the diffusion process; 3. Propose an approach for adapting condition weights automatically. |
Jiang et al. [116] (D) | 2023 | Multimodal MRI (brain) {BraTS2018 [114], IXI2} | 1. Design a bespoke architecture to facilitate diffusion operations in the latent space; 2. Introduction of structural guidance of brain regions in each step of the diffusion process; 3. Propose an approach for adapting condition weights automatically. |
Ozbey et al. [117] (D) | 2023 | Multi-contrast MRI (brain) {BraTS2018 [114], IXI2} MRI-CT (pelvic) {Gold Atlas [115]} |
Proposing a novel source-conditional adversarial projector |
Peng et al. [118] (D) | 2023 | CBCT-CT (Head & Neck) | Proposing a conditional denoising diffusion probabilistic model (DDPM) that utilizes a time-embedded U-net architecture with residual and attention blocks |
Li et al. [108] (D) | 2023 | MRI-CT (pelvic){Gold Atlas [115]} T2 MRI-FLAIR (brain){BraTS2018 [114]} |
1. integrates both an implicit data distribution prior mapping from MRI to CT images and effective information derived from sparse sampled CT measurements; 2. Enabling the use of the model in challenging conditions(w noise) |
Meng et al. [111] (D) | 2022 | Flair & T1 & T1c & T2 (brain){BraTS2019 [114]} | Employment of a novel multi-in multi-out Conditional Score Network (mm-CSN) |
Lyu et al. [119] (D) | 2022 | MRI-CT (pelvic) {Gold Atlas [115]} | Adaptation of denoising diffusion probabilistic and score-matching models |
Pan et al. [120] (D) | 2022 | MRI-CT (Brain, Prostate) {Private} | 1. Implement diffusion processes with a shifted-window transformer network; 2. Capture the complex structures of high-dimensional data; 3. More stable than GANs |
Bazangani et al. [121] (G) | 2022 | FDG PET-T1 MRI (brain) {ADNI3} | 1. Split learning 3D features with separable convolution in a generative model; 2. A Sobel filter for transmission of the geometrical information; 3. improved the stability of learning with a weighted version of a hybrid loss function. |
Emami et al. [122] (G) | 2022 | FDG PET-T1 MRI (brain) {ADNI3} |
1. Integrate contrastive learning in both the generator and discriminator of a GAN model; 2. Learn two separate embeddings for the source and target domains |
Emami et al. [123] (G) | 2022 | FDG PET-T1 MRI (brain) {ADNI3} | 1. Integrate contrastive learning in both the generator and discriminator of a GAN model; 2. Learn two separate embeddings for the source and target domains |
Hu et al. [110] (V) | 2022 | T1 MRI + FLAIR-T2 MRI (brain) {BraTS2019 [114]} | Proposing an efficient 2D variational-autoencoder approach to perform unsupervised domain adaptation (UDA) in a 3D manner. |
Note: 1 https://anhir.grand-challenge.org/Data/ 2 https://brain-development.org/ixi-dataset 3 https://www.adni-info.org/ The letter after the related works represents which model the method is based on: (D): Diffusion Model, (G): Generative Adversarial Network (GAN), (V): Variational Autoencoder (VAE) |
Selected works | Year | Modalities (Organs) {Datasets} | Contribution |
Pan et al. [130] (D) | 2023 | X-ray(Chest) {NIH [131]} MRI(Heart) {ACDC [132]} CT(Pelvic) {Private} CT(Abdomen) {BTCV [133]} | Propose a U-Swin-transformer network to perform the 2D denoising diffusion process |
Moghadam et al. [127] (D) | 2023 | Histopathology Images (Low grade gliomas) {TCGA[129]} | 1. Firstly propose exploiting diffusion probabilistic models to generate synthetic histopathology images; 2. Introduction of color normalization. |
Zhang et al. [134] (G) | 2023 | Ultrasound (Thyroid) {[135]} | 1. Propose a Reference-guided Module to select a finite number of structural references within the dataset by K-Means; 2. Propose a Texture Fuzzy integral Module (TFM) for texture uncertainty; 3. Propose a Structure Fuzzy integral Module (SFM) for structure uncertainty. |
Guo et al. [136] (G) | 2023 | {Private} | Mitigate the issue of mode collapse and convergence failure in learning visually realistic medical images |
Kim et al. [137] (D) | 2022 | MRI (Heart) {ACDC [132]} | 1. Propose a diffusion deformable model for 4D medical image generation; 2. Provide non-rigid continuous deformation of the source image toward the target. |
Pinaya et al. [138] (D) | 2022 | T1w MRI (Brain) {Self-made1} | Combination of diffusion models with compression models following the architecture of Latent Diffusion Models (LDM) |
Zhang et al. [135] (G) | 2022 | Ultrasound (Thyroid) {Described in the paper} | 1. Propose Progressive Texture Generative Adversarial Network (PTGAN) that integrates the repair processof structure and texture; 2. Propose An Image Data Augmentation Strategy based on Mask-Reconstruction (MR-IDAS) |
Abdelhalim et al. [123] (G) | 2021 | Dermoscopic image (Skin Lesion) {HAM10000 [139]} | 1. Application of a stabilized training procedure forincreased stability of training behavior; 2. Apply the Two-Timescale Update Rule (TTUR) to Self-attention Progressive Growing GAN (SPGGAN), with classical Domain Adaptation (DA). |
Note: 1 They made a synthetic dataset and their dataset is available at three following links: Academics Torrents (https://academictorrents.com/details/63aeb864bbe2115ded0aa0d7d36334c026f0660b) FigShare (https://figshare.com/), HDRUK Gateway (https://www.healthdatagateway.org/) The letter after the related works represents which model the method is based on. (D): Diffusion Model, (G): Generative Adversarial Network (GAN) |
Selected works | Year | Model name | LLMs | Datasets | Tasks |
Luo et al. [165] | 2022 | BioGPT | GPT [4] | BC5CDR [166], KD-DTI [167], DDI [168] | End-to-End Relation Extraction |
PubMedQA [146] | Question Answering | ||||
HOC [169] | Document Classification | ||||
(Self-created) | Text Generation | ||||
Venigalla et al. [170] | 2022 | BioMedLM | GPT [4] | MedQA [143], PubMedQA [146], BioASQ [171] | Question Answering |
Singhal et al. [149] | 2022 | MedPaLM | PaLM [150] | MedQA [143], MedMCQA [144], PubMedQA [146], LiveQA [147], MedicationQA [148], MMLU [145] | Question Answering |
Yuan et al. [164] | 2022 | BioBART | BART [15] | CovidDialog1 | Dialogue |
iCliniq, HealthCareMagic, MeQSum [162], MEDIQA-ANS, MEDIQA-QS, MEDIQA-MAS [172] | Summarization | ||||
MedMentions [173], BC5CDR [166], NCBI [174], COMETA [175], AskAPatient[176] | Entity linking | ||||
ShARe13 [177], ShARe14 [178], CADEC [179], GENIA [180] | Named entity recognition | ||||
Dai et al. [141] | 2023 | AugGPT | ChatGPT6 | Symptoms2, PubMed20K | Data augmentation |
Li et al. [181] | 2023 | ChatDoctor | LLaMA [152] | HealthCareMagic-100k3 | Patient-doctor Interactions |
Toma et al. [182] | 2023 | Clinical Camel | LLaMA [152] | ShareGPT4, MedQA [143], (Clinical Articles) | Clinical note, multi-step clinical management, standardized alignment questions |
Wang et al. [183] | 2023 | ClinicalGPT | BLOOM [10] | cMedQA2 [184], cMedQA-KG5, MD-EHR, MedQA [143], MedDialog [161] | Question answering, medical diagnosis, medical record analysis |
Lai et al. [185] | 2023 | KEBLM | BioBERT [186] SciBERT [187] | PubMedQA [146] | Question answering |
NCBI [174], BC5CDR [166], COMETA [175] | Entity linking | ||||
MedNLI [188] | Natural language inference | ||||
Wu et al. [151] | 2023 | PMC-LLaMA | LLaMA [152] | S2ORC [153], PubMedQA [146], MedMCQA [144], MedQA [143] | Question answering |
Li et al. [142] | 2023 | PULSAR | FlanT5 [189] | BioNLP Task 1A [190] | Automatic summarization problems from the provider's progress notes during hospitalization. |
Ma et al. [191] | 2023 | ImpressionGPT | ChatGPT6 | MIMIC-CXR [156] OpenI [157] |
Derive diagnostic impression from radiology findings |
Zhou et al. [158] | 2023 | SkinGPT-4 | MiniGPT-4 [160] | SKINCON [159], Dermnet (Private) | Diagnosis of skin diseases |
Thawkar et al. [154] | 2023 | XrayGPT | Vicuna [155] | MIMIC-CXR [156], OpenI [157] | Analyze and Answer open-ended questions about chest radiographs |
Note: 1 https://github.com/UCSD-AI4H/COVID-Dialogue. 2 https://www.kaggle.com/datasets/paultimothymooney/medicalspeech-transcription-and-intent 3 https://www.healthcaremagic.com/ 4 https://sharegpt.com/ 5 http://cmekg.pcl.ac.cn, https://github.com/baiyang2464/chatbot-base-on-Knowledge-Graph, https://github.com/zhihao-chen/QASystemOnMedicalGraph 6 https://openai.com/blog/chatgpt |
Selected works | Year | Modalities | Organs (Datasets) | Contribution |
Gu et al.[79] | 2020 | CT | Thorax (LUNA 16 Challenge [80], Private) | Introduction of residual whole map attention network |
MR | Brain (Private) | |||
Vasudeva et al. [81] | 2020 | MR | Brain (MICCAI 2013 [82]) Knee (MRNet [83], fastMRI [84]) |
1) Using complex-valued operations to take advantage of complex algebraic structure; 2) Proposing a novel activation function. |
Yuan et al. [85] | 2020 | MR | Brain (MICCAI 2013 [82]) | Combination of the self-attention mechanism and the relative discriminator |
Zehni et al. [86] | 2021 | CT | Head & Lungs | Application of Projection Formation Model |
Gajera et al. [87] | 2021 | CT | Thorax (Kaggle Super Bowl 20171, TCIA [88]) | 1) Combination of a Wasserstein adversarial loss; 2) a pre-trained VGG19 perceptual loss; 3) a Charbonnier distance structural loss. |
Aghabiglou et al. [89] | 2021 | MR | (fastMRI [84]) | Developing a densely connected residual GAN |
Lv et al. [90] | 2021 | MR | Abdomen & Knee | Combination of Parallel Imaging and GAN |
Jiang et al. [91] | 2021 | MR | Multiple | Fusion of the global feature fusion module, including the channel attention module, the self-attention module |
Kyung et al. [92] | 2022 | CT | Head & Neck | 1) Introduction of multi-task discriminator; 2) Non-difference suppression (NDS) loss; 3) Reconstruction consistency (RC) loss. |
Zhou et al. [93] | 2022 | CBCT | Hip | Combination of multiple connection methods with channel attention (CA) and pixel attention (PA) mechanisms |
Huang et al. [74] | 2022 | CT | Abdomen & Chest (AAPM [75]) | 1) Application of a U-Net-based discriminator; 2) Adversarial training in the image gradient domains. |
Yaqub et al. [94] | 2022 | MR | Brain & Knee | Application of transfer learning |
Liu et al. [95] | 2022 | MR | Brain & Knee (MICCAI 2013 [82]) | Application of two end-to-end cascaded U-Net with cross-stage skip connection in generator |
Zhang et al. [96] | 2022 | MR & CT | Multiple | 1) Introduction of a scale-attention SR architecture; 2) Application of two criteria of data preprocessing; 3) Application of perceptual loss previously defined on 2D pre-trained VGG onto 3D medical images |
Li et al. [72] | 2023 | CT | (AAPM [75]) | Application of noise encoding transfer learning |
Zhao et al. [76] | 2023 | MR | Brain (MICCAI 2013 [82], IXI2) Knee (MRNet [1]) |
Application of swin transformer |
Note: 1 https://www.kaggle.com/c/data-science-bowl-2017/data; 2 https://brain-development.org/ixi-dataset |
Selected works | Year | Modalities | Organs (Datasets) | Contribution | |
Chung et al. [97] | 2023 | MR | Knee (fastMRI [84]) | 1) Training a continuous time-dependent score function with denoising score matching; 2) Iteration between the numerical SDE solver and data consistency step to achieve reconstruction. |
|
Güngör et al. [98] | 2023 | MR | Brain (IXI1, fastMRI [84]) | 1) Leveraging a rapid diffusion process with an adversarial mapper for efficient sampling from the diffusion prior; 2) Introduction of inference adaptation |
|
Peng et al. [99] | 2023 | MR | (fastMRI [84], SKM-TEA [100]) | 1) Gradually guiding the reverse-diffusion process with observed k-space signal; 2) Proposing a coarse-to-fine sampling algorithm |
|
Xie et al. [101] | 2023 | MR | Knee (fastMRI [84]) | 1) The diffusion and sampling process are defined in measurement domain rather than image domain; 2) the diffusion process is conditioned on under-sampling mask |
|
Liu et al. [102] | 2023 | CT | Abdomen (AAPM [75]) | Incorporating the pre-trained diffusion model into the denoising framework. | |
Gao et al. [73] | 2023 | CT | (AAPM [75]) | Introduction of a novel restoration network CLEAR-Net to mitigate accumulated errors by constraining the sampling process using contextual information among adjacent slices and calibrating the time step embedding feature using the latest prediction. | |
Gao et al. [103] | 2023 | CT | (AAPM [75]) | a noise estimation network to gradually convert a residual image to a Gaussian distribution based on a Markov chain with a low-dose image as the condition. | |
Cui et al. [104] | 2022 | MR | (fastMRI [84]) | 1) A self-supervised learning score-based diffusion model for the scenario without a fully sampled MRI training set; 2) The corresponding conditioned Langevin Markov chain Monte Carlo (MCMC) sampling for MRI reconstruction. |
|
Xia et al. [105] | 2022 | CT | (AAPM [75]) | Use of a fast ordinary differential equation (ODE) solver for a much-boosted efficient sampling | |
Huang et al. [106] | 2022 | CT | (AAPM [75]) | 1) Combining the low-rank structural-Hankel matrix with the diffusion model to generate the ideal sinogram from the low-dose projection data; 2) Introduction of penalized weighted least-squares (PWLS) and TV to achieve superior image quality. |
|
Note: 1 https://brain-development.org/ixi-dataset |
Selected works | Year | Modalities (Organs) {Datasets} | Contribution |
Bharti et al. [112] (G) | 2023 | MRI-CT{[113]}, T1 MRI-T2 MRI {[114]} stain translation of pathology images {ANHIR-Challenge1} |
employment of evolutionary computation, multiobjective optimization, and an intelligent selection scheme |
Zhao et al. [107] (G) | 2023 | MRI-CT (pelvic) {Gold Atlas [115]} | 1. Extract multi-level features by CNN and Transformer; 2. The feature reconstruction loss is used to further constrain the image potential features. |
Wei et al. [109] (G) | 2023 | MRI-PET | Design an attention regularization for MS lesion in conditional flexible self-attention GAN (CF-SAGAN) |
Jiang et al. [116] (D) | 2023 | Multimodal MRI (brain) {BraTS2018 [114], IXI2} | 1. Design a bespoke architecture to facilitate diffusion operations in the latent space; 2. Introduction of structural guidance of brain regions in each step of the diffusion process; 3. Propose an approach for adapting condition weights automatically. |
Jiang et al. [116] (D) | 2023 | Multimodal MRI (brain) {BraTS2018 [114], IXI2} | 1. Design a bespoke architecture to facilitate diffusion operations in the latent space; 2. Introduction of structural guidance of brain regions in each step of the diffusion process; 3. Propose an approach for adapting condition weights automatically. |
Ozbey et al. [117] (D) | 2023 | Multi-contrast MRI (brain) {BraTS2018 [114], IXI2} MRI-CT (pelvic) {Gold Atlas [115]} |
Proposing a novel source-conditional adversarial projector |
Peng et al. [118] (D) | 2023 | CBCT-CT (Head & Neck) | Proposing a conditional denoising diffusion probabilistic model (DDPM) that utilizes a time-embedded U-net architecture with residual and attention blocks |
Li et al. [108] (D) | 2023 | MRI-CT (pelvic){Gold Atlas [115]} T2 MRI-FLAIR (brain){BraTS2018 [114]} |
1. integrates both an implicit data distribution prior mapping from MRI to CT images and effective information derived from sparse sampled CT measurements; 2. Enabling the use of the model in challenging conditions(w noise) |
Meng et al. [111] (D) | 2022 | Flair & T1 & T1c & T2 (brain){BraTS2019 [114]} | Employment of a novel multi-in multi-out Conditional Score Network (mm-CSN) |
Lyu et al. [119] (D) | 2022 | MRI-CT (pelvic) {Gold Atlas [115]} | Adaptation of denoising diffusion probabilistic and score-matching models |
Pan et al. [120] (D) | 2022 | MRI-CT (Brain, Prostate) {Private} | 1. Implement diffusion processes with a shifted-window transformer network; 2. Capture the complex structures of high-dimensional data; 3. More stable than GANs |
Bazangani et al. [121] (G) | 2022 | FDG PET-T1 MRI (brain) {ADNI3} | 1. Split learning 3D features with separable convolution in a generative model; 2. A Sobel filter for transmission of the geometrical information; 3. improved the stability of learning with a weighted version of a hybrid loss function. |
Emami et al. [122] (G) | 2022 | FDG PET-T1 MRI (brain) {ADNI3} |
1. Integrate contrastive learning in both the generator and discriminator of a GAN model; 2. Learn two separate embeddings for the source and target domains |
Emami et al. [123] (G) | 2022 | FDG PET-T1 MRI (brain) {ADNI3} | 1. Integrate contrastive learning in both the generator and discriminator of a GAN model; 2. Learn two separate embeddings for the source and target domains |
Hu et al. [110] (V) | 2022 | T1 MRI + FLAIR-T2 MRI (brain) {BraTS2019 [114]} | Proposing an efficient 2D variational-autoencoder approach to perform unsupervised domain adaptation (UDA) in a 3D manner. |
Note: 1 https://anhir.grand-challenge.org/Data/ 2 https://brain-development.org/ixi-dataset 3 https://www.adni-info.org/ The letter after the related works represents which model the method is based on: (D): Diffusion Model, (G): Generative Adversarial Network (GAN), (V): Variational Autoencoder (VAE) |
Selected works | Year | Modalities (Organs) {Datasets} | Contribution |
Pan et al. [130] (D) | 2023 | X-ray(Chest) {NIH [131]} MRI(Heart) {ACDC [132]} CT(Pelvic) {Private} CT(Abdomen) {BTCV [133]} | Propose a U-Swin-transformer network to perform the 2D denoising diffusion process |
Moghadam et al. [127] (D) | 2023 | Histopathology Images (Low grade gliomas) {TCGA[129]} | 1. Firstly propose exploiting diffusion probabilistic models to generate synthetic histopathology images; 2. Introduction of color normalization. |
Zhang et al. [134] (G) | 2023 | Ultrasound (Thyroid) {[135]} | 1. Propose a Reference-guided Module to select a finite number of structural references within the dataset by K-Means; 2. Propose a Texture Fuzzy integral Module (TFM) for texture uncertainty; 3. Propose a Structure Fuzzy integral Module (SFM) for structure uncertainty. |
Guo et al. [136] (G) | 2023 | {Private} | Mitigate the issue of mode collapse and convergence failure in learning visually realistic medical images |
Kim et al. [137] (D) | 2022 | MRI (Heart) {ACDC [132]} | 1. Propose a diffusion deformable model for 4D medical image generation; 2. Provide non-rigid continuous deformation of the source image toward the target. |
Pinaya et al. [138] (D) | 2022 | T1w MRI (Brain) {Self-made1} | Combination of diffusion models with compression models following the architecture of Latent Diffusion Models (LDM) |
Zhang et al. [135] (G) | 2022 | Ultrasound (Thyroid) {Described in the paper} | 1. Propose Progressive Texture Generative Adversarial Network (PTGAN) that integrates the repair processof structure and texture; 2. Propose An Image Data Augmentation Strategy based on Mask-Reconstruction (MR-IDAS) |
Abdelhalim et al. [123] (G) | 2021 | Dermoscopic image (Skin Lesion) {HAM10000 [139]} | 1. Application of a stabilized training procedure forincreased stability of training behavior; 2. Apply the Two-Timescale Update Rule (TTUR) to Self-attention Progressive Growing GAN (SPGGAN), with classical Domain Adaptation (DA). |
Note: 1 They made a synthetic dataset and their dataset is available at three following links: Academics Torrents (https://academictorrents.com/details/63aeb864bbe2115ded0aa0d7d36334c026f0660b) FigShare (https://figshare.com/), HDRUK Gateway (https://www.healthdatagateway.org/) The letter after the related works represents which model the method is based on. (D): Diffusion Model, (G): Generative Adversarial Network (GAN) |
Selected works | Year | Model name | LLMs | Datasets | Tasks |
Luo et al. [165] | 2022 | BioGPT | GPT [4] | BC5CDR [166], KD-DTI [167], DDI [168] | End-to-End Relation Extraction |
PubMedQA [146] | Question Answering | ||||
HOC [169] | Document Classification | ||||
(Self-created) | Text Generation | ||||
Venigalla et al. [170] | 2022 | BioMedLM | GPT [4] | MedQA [143], PubMedQA [146], BioASQ [171] | Question Answering |
Singhal et al. [149] | 2022 | MedPaLM | PaLM [150] | MedQA [143], MedMCQA [144], PubMedQA [146], LiveQA [147], MedicationQA [148], MMLU [145] | Question Answering |
Yuan et al. [164] | 2022 | BioBART | BART [15] | CovidDialog1 | Dialogue |
iCliniq, HealthCareMagic, MeQSum [162], MEDIQA-ANS, MEDIQA-QS, MEDIQA-MAS [172] | Summarization | ||||
MedMentions [173], BC5CDR [166], NCBI [174], COMETA [175], AskAPatient[176] | Entity linking | ||||
ShARe13 [177], ShARe14 [178], CADEC [179], GENIA [180] | Named entity recognition | ||||
Dai et al. [141] | 2023 | AugGPT | ChatGPT6 | Symptoms2, PubMed20K | Data augmentation |
Li et al. [181] | 2023 | ChatDoctor | LLaMA [152] | HealthCareMagic-100k3 | Patient-doctor Interactions |
Toma et al. [182] | 2023 | Clinical Camel | LLaMA [152] | ShareGPT4, MedQA [143], (Clinical Articles) | Clinical note, multi-step clinical management, standardized alignment questions |
Wang et al. [183] | 2023 | ClinicalGPT | BLOOM [10] | cMedQA2 [184], cMedQA-KG5, MD-EHR, MedQA [143], MedDialog [161] | Question answering, medical diagnosis, medical record analysis |
Lai et al. [185] | 2023 | KEBLM | BioBERT [186] SciBERT [187] | PubMedQA [146] | Question answering |
NCBI [174], BC5CDR [166], COMETA [175] | Entity linking | ||||
MedNLI [188] | Natural language inference | ||||
Wu et al. [151] | 2023 | PMC-LLaMA | LLaMA [152] | S2ORC [153], PubMedQA [146], MedMCQA [144], MedQA [143] | Question answering |
Li et al. [142] | 2023 | PULSAR | FlanT5 [189] | BioNLP Task 1A [190] | Automatic summarization problems from the provider's progress notes during hospitalization. |
Ma et al. [191] | 2023 | ImpressionGPT | ChatGPT6 | MIMIC-CXR [156] OpenI [157] |
Derive diagnostic impression from radiology findings |
Zhou et al. [158] | 2023 | SkinGPT-4 | MiniGPT-4 [160] | SKINCON [159], Dermnet (Private) | Diagnosis of skin diseases |
Thawkar et al. [154] | 2023 | XrayGPT | Vicuna [155] | MIMIC-CXR [156], OpenI [157] | Analyze and Answer open-ended questions about chest radiographs |
Note: 1 https://github.com/UCSD-AI4H/COVID-Dialogue. 2 https://www.kaggle.com/datasets/paultimothymooney/medicalspeech-transcription-and-intent 3 https://www.healthcaremagic.com/ 4 https://sharegpt.com/ 5 http://cmekg.pcl.ac.cn, https://github.com/baiyang2464/chatbot-base-on-Knowledge-Graph, https://github.com/zhihao-chen/QASystemOnMedicalGraph 6 https://openai.com/blog/chatgpt |