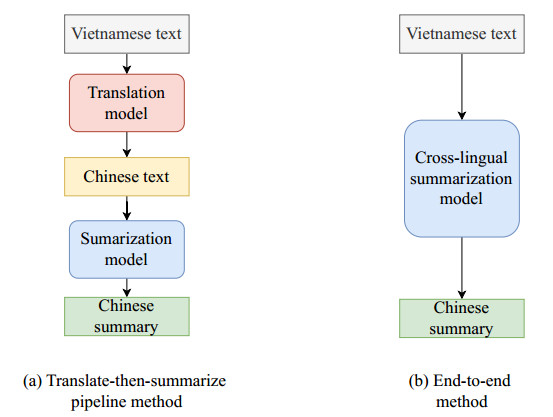
Cross-lingual summarization (CLS) is the task of condensing lengthy source language text into a concise summary in a target language. This presents a dual challenge, demanding both cross-language semantic understanding (i.e., semantic alignment) and effective information compression capabilities. Traditionally, researchers have tackled these challenges using two types of methods: pipeline methods (e.g., translate-then-summarize) and end-to-end methods. The former is intuitive but prone to error propagation, particularly for low-resource languages. The later has shown an impressive performance, due to multilingual pre-trained models (mPTMs). However, mPTMs (e.g., mBART) are primarily trained on resource-rich languages, thereby limiting their semantic alignment capabilities for low-resource languages. To address these issues, this paper integrates the intuitiveness of pipeline methods and the effectiveness of mPTMs, and then proposes a two-stage fine-tuning method for low-resource cross-lingual summarization (TFLCLS). In the first stage, by recognizing the deficiency in the semantic alignment for low-resource languages in mPTMs, a semantic alignment fine-tuning method is employed to enhance the mPTMs' understanding of such languages. In the second stage, while considering that mPTMs are not originally tailored for information compression and CLS demands the model to simultaneously align and compress, an adaptive joint fine-tuning method is introduced. This method further enhances the semantic alignment and information compression abilities of mPTMs that were trained in the first stage. To evaluate the performance of TFLCLS, a low-resource CLS dataset, named Vi2ZhLow, is constructed from scratch; moreover, two additional low-resource CLS datasets, En2ZhLow and Zh2EnLow, are synthesized from widely used large-scale CLS datasets. Experimental results show that TFCLS outperforms state-of-the-art methods by 18.88%, 12.71% and 16.91% in ROUGE-2 on the three datasets, respectively, even when limited with only 5,000 training samples.
Citation: Kaixiong Zhang, Yongbing Zhang, Zhengtao Yu, Yuxin Huang, Kaiwen Tan. A two-stage fine-tuning method for low-resource cross-lingual summarization[J]. Mathematical Biosciences and Engineering, 2024, 21(1): 1125-1143. doi: 10.3934/mbe.2024047
[1] | Di-Wen Kang, Li-Ping Mo, Fang-Ling Wang, Yun Ou . Adaptive harmony search algorithm utilizing differential evolution and opposition-based learning. Mathematical Biosciences and Engineering, 2021, 18(4): 4226-4246. doi: 10.3934/mbe.2021212 |
[2] | Xiaolin Gui, Yuanlong Cao, Ilsun You, Lejun Ji, Yong Luo, Zhenzhen Luo . A Survey of techniques for fine-grained web traffic identification and classification. Mathematical Biosciences and Engineering, 2022, 19(3): 2996-3021. doi: 10.3934/mbe.2022138 |
[3] | Suxiang Yu, Shuai Zhang, Bin Wang, Hua Dun, Long Xu, Xin Huang, Ermin Shi, Xinxing Feng . Generative adversarial network based data augmentation to improve cervical cell classification model. Mathematical Biosciences and Engineering, 2021, 18(2): 1740-1752. doi: 10.3934/mbe.2021090 |
[4] | Dehua Feng, Xi Chen, Xiaoyu Wang, Xuanqin Mou, Ling Bai, Shu Zhang, Zhiguo Zhou . Predicting effectiveness of anti-VEGF injection through self-supervised learning in OCT images. Mathematical Biosciences and Engineering, 2023, 20(2): 2439-2458. doi: 10.3934/mbe.2023114 |
[5] | Sushovan Chaudhury, Kartik Sau, Muhammad Attique Khan, Mohammad Shabaz . Deep transfer learning for IDC breast cancer detection using fast AI technique and Sqeezenet architecture. Mathematical Biosciences and Engineering, 2023, 20(6): 10404-10427. doi: 10.3934/mbe.2023457 |
[6] | Quan Zhu, Xiaoyin Wang, Xuan Liu, Wanru Du, Xingxing Ding . Multi-task learning for aspect level semantic classification combining complex aspect target semantic enhancement and adaptive local focus. Mathematical Biosciences and Engineering, 2023, 20(10): 18566-18591. doi: 10.3934/mbe.2023824 |
[7] | Shubashini Velu . An efficient, lightweight MobileNetV2-based fine-tuned model for COVID-19 detection using chest X-ray images. Mathematical Biosciences and Engineering, 2023, 20(5): 8400-8427. doi: 10.3934/mbe.2023368 |
[8] | Zahra Movahedi Nia, Ali Ahmadi, Bruce Mellado, Jianhong Wu, James Orbinski, Ali Asgary, Jude D. Kong . Twitter-based gender recognition using transformers. Mathematical Biosciences and Engineering, 2023, 20(9): 15962-15981. doi: 10.3934/mbe.2023711 |
[9] | Sonam Saluja, Munesh Chandra Trivedi, Shiv S. Sarangdevot . Advancing glioma diagnosis: Integrating custom U-Net and VGG-16 for improved grading in MR imaging. Mathematical Biosciences and Engineering, 2024, 21(3): 4328-4350. doi: 10.3934/mbe.2024191 |
[10] | Wenzhuo Chen, Yuan Wang, Xiaojiang Tang, Pengfei Yan, Xin Liu, Lianfeng Lin, Guannan Shi, Eric Robert, Feng Huang . A specific fine-grained identification model for plasma-treated rice growth using multiscale shortcut convolutional neural network. Mathematical Biosciences and Engineering, 2023, 20(6): 10223-10243. doi: 10.3934/mbe.2023448 |
Cross-lingual summarization (CLS) is the task of condensing lengthy source language text into a concise summary in a target language. This presents a dual challenge, demanding both cross-language semantic understanding (i.e., semantic alignment) and effective information compression capabilities. Traditionally, researchers have tackled these challenges using two types of methods: pipeline methods (e.g., translate-then-summarize) and end-to-end methods. The former is intuitive but prone to error propagation, particularly for low-resource languages. The later has shown an impressive performance, due to multilingual pre-trained models (mPTMs). However, mPTMs (e.g., mBART) are primarily trained on resource-rich languages, thereby limiting their semantic alignment capabilities for low-resource languages. To address these issues, this paper integrates the intuitiveness of pipeline methods and the effectiveness of mPTMs, and then proposes a two-stage fine-tuning method for low-resource cross-lingual summarization (TFLCLS). In the first stage, by recognizing the deficiency in the semantic alignment for low-resource languages in mPTMs, a semantic alignment fine-tuning method is employed to enhance the mPTMs' understanding of such languages. In the second stage, while considering that mPTMs are not originally tailored for information compression and CLS demands the model to simultaneously align and compress, an adaptive joint fine-tuning method is introduced. This method further enhances the semantic alignment and information compression abilities of mPTMs that were trained in the first stage. To evaluate the performance of TFLCLS, a low-resource CLS dataset, named Vi2ZhLow, is constructed from scratch; moreover, two additional low-resource CLS datasets, En2ZhLow and Zh2EnLow, are synthesized from widely used large-scale CLS datasets. Experimental results show that TFCLS outperforms state-of-the-art methods by 18.88%, 12.71% and 16.91% in ROUGE-2 on the three datasets, respectively, even when limited with only 5,000 training samples.
Cross-lingual summarization (CLS) aims to convert long texts in one language (i.e., the source language) into concise and meaningful summaries of another language (i.e., the target language) without losing the original intent. CLS can assist users by quickly obtaining information from different languages, promoting cross-cultural communication, and enhancing situational awareness in neighboring countries. Compared to monolingual summarization models, CLS models need to possess both translation and summarization capabilities, which means that CLS models face the challenge of translation and summarization simultaneously, that is, semantic alignment and information compression. Specifically, (1) Semantic Alignment: during the process of CLS generation, the model needs to understand the semantic information of the source language text and accurately express it in the target language. However, in many cases, there are significant differences in the vocabulary, grammar, and language structure of the source and target languages, thereby resulting in difficulties in semantic alignment. (2) Information Compression: during the process of CLS generation, the model needs to identify key information in the source text and express the key information concisely in target summary; however, due to the semantic complexity and the diversity of the text, it is difficult to compress information.
The methods for CLS can be categorized into two main types: pipeline methods (as show in Figure 1(b)) and end-to-end mothods (as show in Figure 1(b)). Pipeline methods are typically divided into two subtypes: translation-then-summarization [1,2] and summarization-then-translation [3]. These methods are intuitive. For example, a translate-then-summarize pipeline method first solves the semantic alignment problem through the translation model, and then solves the information compression problem through the monolingual summary model. However, they are prone to error propagation and heavily rely on large corpora for training translation and summarization models. In contrast, end-to-end methods effectively avoid error propagation. Neural cross-lingual summarization (NCLS) [4] pioneered the utilization of a Transformer-based encoder-decoder architecture to achieve end-to-end summarization. Furthermore, they created a CLS dataset using a back-translation strategy, which includes source language texts, source language summaries, and target language summaries. NCLS demonstrates the effectiveness of the end-to-end methods in CLS. Additionally, the emergence of multilingual pre-trained models (mPTMs) has brought new opportunities for CLS. The mPTMs are trained through self-supervised learning to reconstruct noisy multilingual text data, thereby enabling the model to better capture semantic relationships in different languages and to achieve accurate semantic alignment across multiple languages. This training paradigm has shown impressive results in resource-rich languages, thus significantly improving the performance of downstream tasks (such as Chinese-English machine translation). However, mPTMs' pre-training focuses on enhancing the model's semantic alignment capability rather than its information compression capability; moreover, their training data samples mostly come from resource-rich languages, thus limiting their information compression and semantic alignment capabilities in low-resource languages.
To address these issues, this paper integrates the intuitiveness of pipeline methods and the effectiveness of mPTMs, and then proposes a two-stage fine-tuning method for low-resource cross-lingual summarization (TFLCLS), which focus on enhancing the semantic alignment and information compression capabilities of mPTMs to improve their CLS ability in low-resource scenarios. Specifically, while considering the current lack of real low-resource language CLS datasets and according to the back-translation strategy, we first construct a Vietnamese-Chinese CLS dataset, called Vi2ZhLow, from scratch. This dataset contains three parts: source language texts, source language summaries, and target language summaries. In addition, in order to further verify the generalization ability of TFLCLS, we also synthesize two pseudo low-resource datasets, called En2ZhLow and Zh2Enlow, from widely used large-scale CLS datasets. The composition of these two datasets is the same as Vi2ZhLow. In the first stage of TFLCLS, by recognizing the deficiency in semantic alignment for low-resource languages in mPTMs, a semantic alignment fine-tuning method is employed to enhance the mPTMs' understanding of such languages. Taking Vietnamese-Chinese CLS as an example, we treat the Vietnamese summaries and their corresponding Chinese summaries as translation datasets and fine-tuning the mPTM (i.e., mBART) to enhance the semantic alignment of the mPTMs. In the second stage of TFLCLS, while considering that mPTMs are not originally tailored for information compression and CLS demands the model to simultaneously align and compress, an adaptive joint fine-tuning method is proposed. Taking Vietnamese-Chinese CLS as an example, we first treat Vietnamese texts and their Vietnamese summaries as monolingual summarization dataset, and Vietnamese texts with Chinese summaries as CLS dataset. Then, we design a novel loss weighting scheme to the adaptive joint fine-tuning of the mPTM on the above two datasets. Through training on monolingual summarization data, we improve the encoder's information compression ability. Additionally, through training on CLS data, the model's alignment and compression capabilities is simultaneously enhanced.
In summary, this method is simple but effective, which explicitly models the alignment and compression process of low-resource CLS, effectively guides mPTMs to learn the semantic alignment between the source language and the target language, and compresses information between the source text and the summary. Experimental results show that TFCLS outperforms state-of-the-art methods by 18.88%, 12.71%, and 16.91% in ROUGE-2 on the three datasets, respectively, even when limited with only 5,000 training samples. In addition, we conduct an in-depth analysis of TFLCLS through an ablation experiment, a comparison experiment of loss weighting schemes, a hyperparameter experiment, a human evaluation and a case study. In summary, our contributions are three-fold:
1) We have constructed and released a high-quality Vietnamese-Chinese CLS dataset. Moreover, we made our code and dataset publicly available to the research community. The dataset and code of this paper are available at https://github.com/Zhangkaixiongyyds/TFLCLS
2) This paper proposed a two-stage fine-tuning method to address the challenges of semantic alignment and information compression in CLS under low-resource scenarios.
3) This paper systematically evaluated the performance of TFLCLS through a comparison experiment, an ablation experiment, a hyperparameter experiment, a human evaluation and a case study. The experimental results demonstrate the superiority of TFLCLS.
Monolingual summarization is the process of generating a concise summary of texts written in the same language. Existing monolingual summarization methods are usually classified into two categories: extractive summarization and abstractive summarization.
In extractive summarization, statistical [5,6] and deep learning methods [7] are used to identify the important sentences or phrases from the source text and combine them to form a summary. The advantages of extractive summarization methods include low computational complexity and high factual consistency. However, they struggle to fully capture the content of source text.
On the other hand, abstractive summarization methods usually use deep learning models, such as a recurrent neural network (RNN) and a Transformer, to generate summaries by interpreting and rephrasing the source text. For example, Nallapati et al.[8] utilized RNN to capture the hierarchical structure between sentences and words in the source text. Khandelwal et al.[9] employed a pre-trained transformer decoder to generate summaries. Moreover, Huang et al.[10] used a Transformer to construct a heterogeneous graph of article elements, and then used the heterogeneous graph to influence the text decoder to generate a concise and smooth summary. Although abstractive summarization methods can generate more human-like summaries, they may suffer from information omission and inconsistency in the generated summaries.
The initial studies on CLS mainly concentrated on pipeline-based techniques, which can be categorized into two main types: translate-then-summarize, as demonstrated in Wan et al. [1] and Zhang et al. [2], and summarize-then-translate, as illustrated by Wan et al. [3]. Although these methods seem straightforward, they are susceptible to error amplification, demands substantial corpora for training translation and summarization models, incurs costs for translation services, and introduces delays in the inference phase.
With the development of deep learning, many researches turned their attention to end-to-end methods. These methods typically include an encoder and a decoder, where the source language text is the input to the encoder, and the decoder utilizes the hidden features generated by the encoder to generate the cross-lingual summary. For example, Zhu et al.[4] constructed the first large-scale CLS dataset by back-translation, and used a transformer framework to model the CLS task. Futhermore, they found that there exists a translation pattern in CLS; therefore, they first translated some important words into the target language, and then generated a final summary based on these keywords [11]. Qin et al.[12] constructed a case study to explore the advantages of fune-tuning, adapter-tuning and prefix-tuning for CLS. Wang et al.[13] systematically studied the phenomenon of translationese, which appears in translation-based CLS datasets. They concluded that though translation-based CLS datasets involve translationese, they are very useful for training CLS models of low-resource languages. Recently, Wang et al.[14] tried to unify multilingual summarization (MLS) and CLS into a more general setting. Taunk et al.[15] proposed XWikiGen, which produced a cross-lingual multi-document summarization of text from multiple reference articles, written in various languages, to generate a Wikipedia-style text.
For low-resource languages, Bai et al.[16] utilized a shared decoder to generate monolingual and cross-lingual summaries at the same time, which can transfer knowledge from high-resource languages into low-resource languages. Nguyen et al. [17] utilized knowledge distillation to explicitly construct cross-lingual correlation by transfering knowledge from a monolingual summarization teacher into a cross-lingual summarization student. Both of these methods require a large-scale monolingual summarization dataset (e.g., English summarization) to pre-train their models, which alleviates the information compression problem. However, the monolingual summarization datasets (e.g., Vietnamese summarization) are as scarce as CLS datasets (e.g., Vietnamese-Chinese summarization).
The proposed TFLCLS is based on mBART; therefore, we will briefly introduce mBART first, and then introduce TFLCLS in detail.
Among many mPTMs, we chose mBART [18] as the foundation of TFLCLS. mBART is pre-trained on a variety of large-scale multilingual corpora using the BART training paradigm, endowing it with the capabilities of text comprehension and generation inherent to BART, as well as the ability to comprehend multiple languages. mBART has achieved significant results in both natural language understanding and generation tasks. The pre-trained process of mBART is illustrated in Figure 2; it first injects noise into the input text by masking words and swapping sentence orders, and then forces a Transformer to recover the text. This process is repeated multiple times on corpora in different languages. When feeding different language texts into the model, the specific language ID is appended at the end of the input text and added at the beginning of the reference text. The optimization goal of mBART can be formulated as follows:
L(θ)=∑Di∈D∑X∈DilogP(X|g(X);θ) | (3.1) |
where g represents the Transformer encoder and decoder, D represents the entire language document collection, Di represents the monolingual documents in the i-th language, and X denotes a text belonging to the i-th language. The model aims to maximize L(θ), which is the probability of predicting the source text given the noisy text. The documents in D are written in different languages, which enables the model to capture the grammar and semantic features of different languages; therefore, mBART has achieved an excellent performance on various natural language processing tasks. It should be noted that mBART in this paper refers to mbart-large-cc25 (https://huggingface.co/facebook/mbart-large-cc25), which was pre-trained on 25 languages' monolingual corpus.
TFLCLS consists of two fine-tuning stages, which are described in detail below.
In the first stage, we perform fine-tuning of the pre-trained mBART on a translation dataset. The CLS dataset generated by the back-translation strategy naturally contains translation pairs, that is, the source language summary and the target language summary. As illustrated in Figure 3(a), the purpose of this stage is to stimulate the semantic alignment ability of pre-trained mBART between the source language and the target language. Therefore, the model can have a deeper comprehension of the semantic content in the source language text and accurately express such semantic information in the target language. Given a source language document S and a target language document T, our objective is to minimize the loss function Ltra, which is formulated as follows:
Ltra=−ΣNi=1logP(yti|ysi;ω) | (3.2) |
where N denotes the number of training samples, ysi represents the i-th source language summary, yti represents the i-th target language summary, and ω represents the parameters of the pre-trained mBART model. The parameters are initialized with the weights of original mBART (i.e., mbart-large-cc25).
In the second stage, we incorporate an additional monolingual summarization decoder into the mBART obtained from the first stage and train the encoder of mBART and the monolingual summarization decoder on a monolingual summarization dataset. This monolingual summarization decoder is responsible for generating the source language summary to enhance the information compression capability of the encoder of mBART. At the same time, a CLS dataset is used to train the encoder and the cross-lingual summarization decoder of mBART, which can simultaneously improve the semantic alignmet and the information compression capabilities of the mBART. As illustrated in Figure 3(b), ml_Decoder represents the monolingual summarization decoder, cl_Decoder represents the cross-lingual summarization decoder. The parameters of ml_Decoder are initialized with the weights of the original mBART decoder (i.e., the decoder of mbart-large-cc25); the encoder and cl_Decoder are initialized using the encoder-decoder parameters fine-tuned in the first stage. In the training process, we first utilize the encoder to encode the source language text into hidden representations. Then, we employ cl_Decoder to decode the hidden representations into the target language summary, while simultaneously using the ml_Decoder to decode the hidden representations into the source language summary. We calculate the loss for the generated source language summary and the target language summary by measuring their cross-entropy with their corresponding reference summaries. The loss formulas are as follows:
Lmls=−ΣNi=1logP(ysi|xi;ωen,ωdem) | (3.3) |
Lcls=−ΣNi=1logP(yti|xi;ωen,ωdec) | (3.4) |
where Lmls and Lcls are the loss of monolingual and cross-lingual summarization, respectively. N represents the number of training samples, xi represents the i-th source language document, ysi represents the i-th source language summary, yti represents the i-th target language summary, ωen represents the parameters of the encoder, ωdec represents the parameters of the cross-lingual summarization decoder, and ωdem represents the parameters of the monolingual summarization decoder.
Furthermore, while considering that the importance of monolingual summarization and cross-lingual summarization should not be the same, we design a loss weighting scheme to adaptively trade-off these two tasks, which is inspired by revised homoscedastic uncertainty (RHU) [19]. The formula for calculating the loss weight is as follows:
L(x,y,y′;ωen,ωde)=12(rc)2⋅Lmls+ln(1+(r⋅c)2)12+12c2⋅Lcls+ln(1+c2) | (3.5) |
where L represents the overall model loss, c is a trainable parameter used to adaptively trade-off two tasks, and r is a manually specified fixed parameter to set the initial task importance to the loss, so that the model can be optimized to a more correct direction from the beginning.
In this section, we present the Vi2ZhLow, En2ZhLow and Zh2EnLow dataset construction methods, the experimental setup, and the experimental results and analyses.
To perform low-resource CLS experiments, we conducted a Vi2ZhLow dataset. Specifically, we first crawled 142,000 news articles and summaries from Vietnamese news websites (e.g., https://moc.gov.vn). Then, we translated the Vietnamese summaries into Chinese as target language summaries by Google Translation. However, due to the low-resource nature of Vietnamese, the translation results were unsatisfactory. Therefore, we back-translated the target language summaries into the source language and performed Rouge evaluation [4] between the Vietnamese summaries and the back-translated summaries to obtain high-quality CLS samples. Finally, we retained the best 11,000 results according to the Rouge scores. Moreover, we synthesised two other CLS datasets, namely En2ZhLow and Zh2EnLow, from two widely used large-scale CLS datasets (i.e., En2Zh and Zh2En) [4,20] by random sampling. This way has been used in MCLAS [16] Nguyen et al. [17] to simulate low resource scenarios. The purpose is to provide a dataset for further verification of the generalization ability of TFLCLS.
All training samples consist of a source language text, a monolingual language summary, and a cross-lingual language summary. To simulate different low-resource scenarios, we randomly selected 1,000, 3,000 and 5,000 training samples from a dataset to evaluate the performance of TFLCLS. Additionally, the validation and test dataset were both fixed to 3,000. The statistics of the three datasets are shown in Table 1.
Vi2ZhLow | train | valid | test | En2ZhLow | train | valid | test | Zh2EnLow | train | valid | test |
#Docs | 5,000 | 3,000 | 3,000 | #Docs | 5,000 | 3,000 | 3,000 | #Docs | 5,000 | 3,000 | 3,000 |
#AvgW (S) | 715 | 715 | 717 | #AvgW (S) | 755 | 760 | 745 | #AvgC (S) | 104 | 104 | 104 |
#AvgW (R) | 48 | 48 | 48 | #AvgW (R) | 55 | 55 | 55 | #AvgC (R) | 18 | 18 | 18 |
#AvgC (R) | 53 | 53 | 54 | #AvgC (R) | 96 | 96 | 95 | #AvgW (R) | 14 | 14 | 14 |
S represents source texts, R represents reference summaries. AvgW (S) represents the average number of Vietnamese/English words in the source texts. AvgW (R) represents the average number of words in the Vietnamese/English summaries. AvgC (S) represents the average number of Chinese characters in the source texts. AvgC (R) represents the average number of characters in Chinese summaries. |
In 2004, Lin [21] proposed an automatic summarization evaluation method called the ROUGE score, which has been widely used by a large number of researchers. The ROUGE score is calculated based on the overlap of n-grams, word pairs, and word sequences between the generated summary and reference summary. In this paper, we adopted the ROUGE-1, ROUGE-2 and ROUGE-L score to automatically evaluate the quality of generated summaries, which can be formulated as follows:
ROUGE-1=ΣS(w)ΣR(w) | (4.1) |
where S(w) represents the count of word w in the generated summary, and R(w) represents the count of word w in the reference summary;
ROUGE-2=ΣS(wi,wi+1)ΣR(wi,wi+1) | (4.2) |
where S(wi,wi+1) represents the count of the consecutive word pair (wi,wi+1) in the generated summary, and R(wi,wi+1) represents the count of the consecutive word pair (wi,wi+1) in the reference summary;
ROUGE-L=2.0∗RlcsPlcsRlcs+Plcs | (4.3) |
Rlcs=LCS(S,R)len(R) | (4.4) |
Plcs=LCS(S,R)len(S) | (4.5) |
where the Rlcs denotes the recall rate, and Plcs represents the precision. LCS(S,R) is the length of the longest common subsequence between the generated summary S and the reference summary R. len(S) denotes the length of the generated summary, while len(R) is the length of the reference summary. In subsequent experiments, in order to make the results more concise, we use R-1, R-2 and R-L to represent ROUGE-1, ROUGE-2 and ROUGE-L, respectively.
To evaluate the performance of TFLCLS, we compared TFLCLS with the following CLS models on the Vi2ZhLow, En2ZhLow and Zh2EnLow datasets:
(1) NCLS: an end-to-end CLS framework proposed by Zhu et al. [4], which is based on a transformer encoder-decoder architecture. To our best knowledge, this is the first end-to-end CLS model.
(2) NCLS+MS: a multitask framework proposed by Zhu et al. [4], which includes an additional monolingual summarization decoder as compared to NCLS.
(3) MCLAS: a multitask low-resource CLS framework proposed by Bai et al. [16], which utilizes mBERT as the encoder and a transformer's decoder as the decoder. Different from the original MCLAS, which was pre-trained on large-scale monolingual summarization datasets, we pre-trained MCLAS on small-scale monolingual summarization datasets (i.e., 1,000, 3,000 and 5,000). The purpose is to simulate a more realistic low-resource scenario and make a fairer comparison.
(4) Nguyen et al.: a knowledge-distillation-based low-resource CLS framework proposed by Nguyen [17], which distills knowledge from the monolingual summarization teacher model into the CLS student model. Different from the original Nguyen et al., which trained the teacher model on large-scale monolingual summarization datasets, we trained the teacher model on small-scale monolingual summarization datasets (i.e., 1,000, 3,000, and 5,000). The purpose is the same as MCLAS.
(5) mBART-CLS: a mBART model, which was directly fine-tuned on the low-resource CLS dataset.
For TFLCLS, we employed the Pytorch Lightning (http://www.pytorchlightning.ai) deep learning framework and conducted experiments on a single NVIDIA 4090 GPU. In the first stage, during the fine-tuning of the mBART pre-trained model on a translation dataset, a batch size of four was set with gradient accumulation after every four steps. The Adam optimizer was employed with a learning rate of 0.00005, and the remaining parameters were set to default values. In the second stage, the batch size was adjusted to two with gradient accumulation after every eight steps, and the learning rate was adjusted to 0.000025. At the beginning of each training epoch, the learning rate was set to 0.95 times the value of the previous epoch. The r is set to 2 by a grid search on the validation set of Vi2ZhLow.
Table 2 presents the comparison results between TFLCLS and the baseline models on the Vi2ZhLow, En2ZhLow and Zh2EnLow datasets with the number of training samples of 1,000, 2,000 and 3,000.
Model | # of training samples | Vi2ZhLow | En2ZhLow | Zh2EnLow | ||||||||
R-1 | R-2 | R-L | R-1 | R-2 | R-L | R-1 | R-2 | R-L | ||||
NCLS | 1000 | 23.362 | 3.161 | 20.889 | 20.093 | 2.221 | 17.954 | 13.723 | 1.122 | 12.920 | ||
3000 | 24.753 | 4.429 | 21.296 | 21.999 | 3.037 | 19.769 | 14.279 | 1.329 | 13.729 | |||
5000 | 25.234 | 5.015 | 21.828 | 22.345 | 3.285 | 19.715 | 15.564 | 1.555 | 14.265 | |||
NCLS+MS | 1000 | 22.777 | 3.740 | 20.057 | 13.148 | 1.974 | 11.772 | 15.419 | 1.169 | 13.785 | ||
3000 | 23.916 | 4.079 | 20.695 | 18.536 | 3.043 | 15.660 | 13.788 | 1.245 | 12.272 | |||
5000 | 24.892 | 4.477 | 22.145 | 20.284 | 3.034 | 17.770 | 14.437 | 1.463 | 12.876 | |||
MCLAS | 1000 | 24.395 | 7.467 | 19.760 | 22.625 | 4.609 | 14.383 | 10.364 | 1.456 | 9.270 | ||
3000 | 27.598 | 9.853 | 22.430 | 23.872 | 6.257 | 16.522 | 13.376 | 2.748 | 11.960 | |||
5000 | 28.086 | 10.288 | 22.875 | 29.031 | 9.459 | 19.690 | 16.642 | 3.785 | 14.701 | |||
Nguyen et al. | 1000 | 25.262 | 8.064 | 20.287 | 23.930 | 5.045 | 15.354 | 10.894 | 2.037 | 10.106 | ||
3000 | 27.617 | 9.846 | 22.405 | 25.880 | 7.212 | 17.587 | 15.003 | 3.356 | 13.615 | |||
5000 | 29.030 | 10.665 | 23.503 | 31.147 | 10.577 | 20.998 | 16.552 | 4.147 | 14.939 | |||
mBART-CLS | 1000 | 26.557 | 7.553 | 21.875 | 26.912 | 7.839 | 19.004 | 16.575 | 3.271 | 14.275 | ||
3000 | 28.816 | 9.310 | 24.035 | 30.233 | 10.632 | 21.383 | 19.782 | 4.755 | 16.891 | |||
5000 | 29.386 | 9.664 | 24.407 | 32.122 | 12.251 | 22.968 | 21.197 | 5.631 | 18.139 | |||
TFLCLS | 1000 | 28.192 | 8.439 | 23.292 | 28.555 | 9.219 | 20.502 | 18.182 | 4.065 | 15.599 | ||
3000 | 30.600 | 10.540 | 25.436 | 32.177 | 12.483 | 23.107 | 21.384 | 5.542 | 18.217 | |||
5000 | 31.627 | 11.489 | 26.568 | 33.234 | 13.808 | 24.272 | 22.645 | 6.583 | 19.609 |
The resluts from Vi2ZhLow show that there was a slight decrease in the performance of NCLS+MS compared to NCLS, suggesting that in low-resource scenarios, models that are not pre-trained struggle to fully exploit the advantages of multiple tasks. The performance of MCLAS and Nguyen et al. were similar and both were better than NCLS, which demonstrates the effectiveness of knowledge transfer/knowledge distillation in low-resource scenarios. mBART-CLS achieved the best performance among all baseline models, thus demonstrating the advantages of mPTMs. In comparison, TFLCLS achieved further performance improvements. When the sample sizes were 1,000, 3,000 and 5,000, TFLCLS demonstrated an improvement of approximately 6.16%, 6.19%, and 7.62% in the R-1 score, 11.73%, 13.21% and 18.88% in the R-2 score, and 6.48%, 5.83% and 8.85% in the R-L score, respectively, as compared to mBART-CLS. Furthermore, TFLCLS's performance when trained on 1,000 samples was better than NCLS and NCLS+MS trained on 5,000 samples and, in some cases, was similar to MCLAS and Nguyen et al. trained on 3,000 or even 5,000 samples. This highlights TFLCLS's effectiveness in handling low-resource CLS tasks.
For En2ZhLow, when the sample sizes were 1,000, 3,000 and 5,000, TFLCLS exhibited improvements of approximately 6.11%, 6.43% and 3.46% in the R-1 score, 17.6%, 17.41% and 12.71% in the R-2 score, and 7.88%, 8.06% and 5.68% in the R-L score, respectively, as compared to mBART-CLS. In Zh2EnLow, with sample sizes of 1,000, 3,000, and 5,000, TFLCLS achieved improvements of approximately 9.70%, 8.10% and 6.83% in the R-1 score, 24.27%, 16.55% and 16.91% in the R-2 score, and 9.27%, 7.85% and 8.10% in the R-L score, respectively, as compared to mBART-CLS. The results in En2ZhLow and Zh2EnLow indicated that TFLCLS has the potential to generalize to other low-resource languages.
In order to further analyze the role of different components in TFLCLS, we constructed the following experiments:
(1) TFLCLS: the same as Sction 4.3, in the first stage, we utilized source language summaries and target language summaries to perform semantic alignment fine-tuning on the mBART pre-trained model. In the second stage, the encoder and cross-lingual summarization decoder were initialized using the checkpoint from the first stage with the highest ROUGE-2 score of the validation set, while the monolingual summarization decoder was initialized using the parameters of the bart-large-cc25's decoder.
(2) TFLCLSw/o Tra: different from TFLCLS, the semantic alignment fine-tuning stage was removed. Therefore, the parameters of the encoder were initialized by the encoder of bart-large-cc25, and the parameters of the monolingual summarization decoder and the cross-lingual summarization decoder were both initialized using the decoder of bart-large-cc25.
(3) TFLCLSw/o Mul: different from TFLCLS, the monolingual decoder in the second stage was removed. Therefore, only the cross-lingual decoder was used to generate target language summaries in the second stage, that is, first perform semantic alignment fine-tuning, then perform cross-lingual fine-tuning.
(4) TFLCLSw/o TraMul: The semantic alignment fine-tuning stage and the monolingual decoder in the second stage were removed. Only CLS fine-tuning was performed on the mBART pre-trained model. Essentially, the model is the mBART-CLS in the previous section.
The experimental results are shown in Figure 4. Taking the Vi2ZhLow as an example (shown as Figure 4(a)–(c)), the performances of TFLCLSw/o Mul and TFLCLSw/o Tra were better than TFLCLSw/o TraMul, where TFLCLSw/o Mul is better than TFLCLSw/o Tra in most cases. This may indicate that both semantic alignment fine-tuning and adaptive fine-tuning are effective, while semantic alignment fine-tuning contributes more. Additionally, the combination of the two methods (i.e., TFLCLS) further improves the performance. Similar results and conclusions can also be observed from En2ZhLow (shown as Figure 4(d)–(f)) and Zh2EnLow (shown as Figure 4(g)–(i)). In addition, we have also tried to rearrange the order and combination of the three tasks (i.e., translation, monolingual summarization and CLS), for example, first fine-tuning on monolingual summarization, and then joint fine-tuning on translation and CLS; however, the generated summaries were of a low-quality.
To evaluate the adaptive loss weighting scheme in Stage 2, we compare TFLCLS with three other loss weighting schemes:
(1) TFLCLSbase: no additional schemes were employed during model training to calculate the loss values. Instead, the loss values of the two tasks were simply added together without any modifications.
(2) TFLCLSHU: the loss was calculated by a homoscedastic uncertainty (HU) scheme [22], which weighed multiple loss by considering the homoscedastic uncertainty of each task.
(3) TFLCLSRHU: the loss was calculated by an RHU scheme [19], which added the concept of auxiliary tasks and introduced self-learning multi-task weights.
(4) TFLCLS: the loss was calculated by our adaptive loss weighting scheme in Section 3.2.2.
The experimental results are presented in Table 3. It can be found that TFLCLSHU and TFLCLSRHU were worse than TFLCLSbase in some cases, while TFLCLS has achieved better results than all comparison methods in most cases. This demonstrates the effectiveness of our weighting scheme.
Model | # of training samples | Vi2ZhLow | En2ZhLow | Zh2EnLow | ||||||||
R-1 | R-2 | R-L | R-1 | R-2 | R-L | R-1 | R-2 | R-L | ||||
TFLCLSbase | 1000 | 28.376 | 8.579 | 23.494 | 28.573 | 8.842 | 20.041 | 17.935 | 4.036 | 15.522 | ||
3000 | 30.420 | 10.259 | 25.414 | 32.399 | 12.776 | 23.351 | 20.943 | 5.478 | 17.888 | |||
5000 | 31.411 | 11.380 | 26.334 | 33.009 | 13.652 | 24.243 | 22.587 | 6.542 | 19.568 | |||
TFLCLSHU | 1000 | 28.097 | 8.178 | 23.088 | 28.426 | 8.896 | 20.032 | 17.954 | 4.131 | 15.422 | ||
3000 | 30.472 | 10.189 | 25.333 | 32.204 | 12.804 | 23.381 | 21.088 | 5.468 | 17.951 | |||
5000 | 31.126 | 11.064 | 26.022 | 33.151 | 13.628 | 24.201 | 22.628 | 6.525 | 19.468 | |||
TFLCLSRHU | 1000 | 28.156 | 8.303 | 23.303 | 28.670 | 8.920 | 20.257 | 18.077 | 4.028 | 15.569 | ||
3000 | 30.439 | 10.278 | 25.363 | 32.194 | 12.571 | 23.116 | 21.106 | 5.519 | 17.975 | |||
5000 | 31.366 | 11.379 | 26.325 | 33.772 | 13.753 | 24.201 | 22.296 | 6.484 | 19.360 | |||
TFLCLS | 1000 | 28.192 | 8.439 | 23.292 | 28.555 | 9.219 | 20.502 | 18.182 | 4.065 | 15.599 | ||
3000 | 30.600 | 10.540 | 25.436 | 32.177 | 12.483 | 23.107 | 21.384 | 5.542 | 18.217 | |||
5000 | 31.627 | 11.489 | 26.568 | 33.234 | 13.808 | 24.272 | 22.645 | 6.583 | 19.609 |
To investigate the impact of the hyperparameter r in our loss function, we conducted a hyperparameter experiment on the Vi2ZhLow dataset with 5,000 training samples. The results are presented in Figure 5. Specifically, r is set to 1.0, 1.5, 2.0, 2.5, 3.0 and 3.5, and the best results was achieved when r=2.5. Taking r=2.5 as the starting point, both the decrement and increment of the value led to the decrement of the performance, though the magnitude of the change was small. Therefore, TFLCLS is robust to the hyperparameter r. In TFLCLS, based on the results of the validation set of Vi2ZhLow, r is set to 2.
For a more comprehensive evaluation of TFLCLS, we conducted a human evaluation on the Vi2ZhLow test set. We randomly selected 25 instances from the Vi2ZhLow test set and invited eight native Chinese-speaking graduate students to independently assess the model-generated summaries and the reference summaries, following the Best-Worst Scaling method [23]. Our evaluation was based on three perspectives [16]: informativeness (IF), conciseness (CC), and fluency (FL). The score represents the percentage of times each model-generated summary was selected as the best minus the percentage of the worst: –1 is worst, 1 is best. The results are presented in Table 4.
Model | 1000 training samples | 3000 training samples | 5000 training samples | ||||||||
IF | CC | FL | IF | CC | FL | IF | CC | FL | |||
NCLS | –0.365 | –0.503 | –0.382 | –0.354 | –0.423 | –0.400 | –0.383 | –0.503 | –0.423 | ||
NCLS+MS | –0.331 | –0.342 | –0.314 | –0.331 | –0.440 | –0.463 | –0.394 | –0.434 | –0.377 | ||
MCLAS | –0.154 | –0.057 | –0.240 | –0.171 | –0.051 | –0.068 | –0.143 | –0.046 | –0.074 | ||
Nguyen et al. | –0.137 | –0.029 | –0.137 | –0.114 | –0.051 | –0.040 | –0.040 | –0.023 | –0.103 | ||
TFLCLS | 0.148 | 0.171 | 0.200 | 0.297 | 0.326 | 0.251 | 0.331 | 0.337 | 0.394 | ||
Reference | 0.851 | 0.800 | 0.771 | 0.737 | 0.703 | 0.657 | 0.708 | 0.629 | 0.566 |
Compared to the four baseline models, TFLCLS has achieved the best results across all metrics. The summaries generated by MCLAS and Nguyen et. al. are noticeably shorter in length, but they significantly deviate from the source text. Furthermore, we have observed that TFLCLS sometimes generates summaries that exhibit an improved fluency compared to the reference summaries, but overall, there is still a gap. The reason for this phenomenon is that the Chinese reference summary is translated from Vietnamese, which cannot guarantee the fluency. However, with the powerful generation capability of the mBART pre-trained model, the generated Chinese summaries are sometimes more fluent.
In order to intuitively show the performance of TFLCLS, we constructed a case study on Vi2ZhLow. The results are shown in Figure 6, where keywords are highlighted and the same keywords are represented by the same color. Specifically, the generated Chinese summary from the four baseline models exhibit issues such as inconsistent facts, repetition, and poor fluency. For example, the summary generated by NCLS mentioned a slight increase in the price of gold on the world market on February 3, 2017; however, there is no relevant content in the source text. This indicates that the NCLS model failed to correctly understand the content of the source text and generated a summary that is irrelevant to the source text. The summary generated by the NCLS+MS model mentions the Vietnamese market, but the logic of the summary is confusing, thus suggesting that the NCLS+MS model may not have effectively transformed the information from the source text into a meaningful summary. The summary generated by the MCLAS model mentions strong growth in the Vietnamese Real Estate Association and a growth rate of 2%. However, there is no relevant information in the source text, and the abbreviation "bx" mentioned in the summary is not the correct abbreviation for the Vietnamese Real Estate Association. The summary generated by Nguyen et. al matches more words than the other three baseline methods, though the meaning of the generated summary still has a big gap with the source text. Compared with the aforementioned models, the summary generated by the TFLCLS model is more similar to the reference summary, and has better informativeness, conciseness, and fluency. However, there is still a minor flaw in which the abbreviation "VNREA" is not correctly spelled in the summary, and the timeframe is not comprehensive enough.
In this study, in order to solve semantic alignment and information compression problems of low-resource CLS, we proposed TFLCLS, which integrated the intuitiveness of pipeline methods and the effectiveness of mPTMs. To evaluate the performance of TFLCLS on real low-resource CLS dataset, we construted a Vietnamese-Chinese CLS dataset. Additionally, to further evaluate the generalization of TFLCLS, we synthesized two pseudo low-resource datasets. Systematic experiments on three datasets demonstrated the effectiveness and generalization ability of TFLCLS, which bring new insights into future research of CLS. We released our dataset and code at https://github.com/Zhangkaixiongyyds/TFLCLS.
The authors declare they have not used Artificial Intelligence (AI) tools in the creation of this article.
This research was supported by National Natural Science Foundation of China (U21B2027, 61972186, 62266027, 62266028, 62302201), Yunnan provincial major science and technology special plan projects (202302AD080003, 202202AD080003), Yunnan Fundamental Research Projects (202301AT070393, 202301AT070471), Kunming University of Science and Technology's "Double First-rate" construction joint project (202201BE070001-021).
The authors declare there is no conflict of interest.
[1] | X. Wan, Using bilingual information for cross-language document summarization, in Proceedings of the 49th Annual Meeting of the Association for Computational Linguistics: Human Language Technologies, (2011), 1546–1555. |
[2] |
J. Zhang, Y. Zhou, C. Zong, Abstractive cross-language summarization via translation model enhanced predicate argument structure fusing, IEEE/ACM Trans. Audio Speech Language Process., 24 (2016), 1842–1853. https://doi.org/10.1109/TASLP.2016.2586608 doi: 10.1109/TASLP.2016.2586608
![]() |
[3] | X. Wan, H. Li, J. Xiao, Cross-language document summarization based on machine translation quality prediction, in Proceedings of the 48th Annual Meeting of the Association for Computational Linguistics, (2010), 917–926. |
[4] | J. Zhu, Q. Wang, Y. Wang, Y. Zhou, J. Zhang, S. Wang, et al., NCLS: Neural cross-lingual summarization, in Proceedings of the 2019 Conference on Empirical Methods in Natural Language Processing and the 9th International Joint Conference on Natural Language Processing (EMNLP-IJCNLP), (2019), 3054–3064. https://doi.org/10.18653/v1/D19-1302 |
[5] |
G. Erkan, D. R. Radev, Lexrank: Graph-based lexical centrality as salience in text summarization, J. Artif. Int. Res., 22 (2004), 457–479. https://doi.org/10.1613/jair.1523 doi: 10.1613/jair.1523
![]() |
[6] | R. Mihalcea, P. Tarau, TextRank: Bringing order into text, in Proceedings of the 2004 Conference on Empirical Methods in Natural Language Processing, (2004), 404–411. |
[7] |
J. Zhao, L. Yang, X. Cai, Hettreesum: A heterogeneous tree structure-based extractive summarization model for scientific papers, Expert Syst. Appl., 210 (2022), 118335. https://doi.org/10.1016/j.eswa.2022.118335 doi: 10.1016/j.eswa.2022.118335
![]() |
[8] | R. Nallapati, B. Zhou, C. dos Santos, Ç. Gulçehre, B. Xiang, Abstractive text summarization using sequence-to-sequence RNNs and beyond, in Proceedings of the 20th SIGNLL Conference on Computational Natural Language Learning, (2016), 280–290. https://doi.org/10.18653/v1/K16-1028 |
[9] | U. Khandelwal, K. Clark, D. Jurafsky, L. Kaiser, Sample efficient text summarization using a single pre-trained transformer, preprint, arXiv: 1905.08836. |
[10] |
Y. Huang, S. Hou, G. Li, Z. Yu, Abstractive summary of public opinion news based on element graph attention, Information, 14. https://doi.org/10.3390/info14020097 doi: 10.3390/info14020097
![]() |
[11] | J. Zhu, Y. Zhou, J. Zhang, C. Zong, Attend, translate and summarize: An efficient method for neural cross-lingual summarization, in Proceedings of the 58th Annual Meeting of the Association for Computational Linguistics, (2020), 1309–1321. https://doi.org/10.18653/v1/2020.acl-main.121 |
[12] | Y. Qin, G. Neubig, P. Liu, Searching for effective multilingual fine-tuning methods: A case study in summarization, preprint, arXiv: 2212.05740. |
[13] | J. Wang, F. Meng, T. Zhang, Y. Liang, J. Xu, Z. Li, et al., Understanding translationese in cross-lingual summarization, preprint, arXiv: 2212.07220. |
[14] | J. Wang, F. Meng, D. Zheng, Y. Liang, Z. Li, J. Qu, et al., Towards unifying multi-lingual and cross-lingual summarization, in Proceedings of the 61st Annual Meeting of the Association for Computational Linguistics, (2023), 15127–15143. https://doi.org/10.18653/v1/2023.acl-long.843 |
[15] | D. Taunk, S. Sagare, A. Patil, S. Subramanian, M. Gupta, V. Varma, Xwikigen: Cross-lingual summarization for encyclopedic text generation in low resource languages, in Proceedings of the ACM Web Conference, (2023), 1703–1713. https://doi.org/10.1145/3543507.3583405 |
[16] | Y. Bai, Y. Gao, H. Huang, Cross-lingual abstractive summarization with limited parallel resources, in Proceedings of the 59th Annual Meeting of the Association for Computational Linguistics and the 11th International Joint Conference on Natural Language Processing, (2021), 6910–6924. https://doi.org/10.18653/v1/2021.acl-long.538 |
[17] | T. T. Nguyen, A. T. Luu, Improving neural cross-lingual abstractive summarization via employing optimal transport distance for knowledge distillation, in Proceedings of the AAAI Conference on Artificial Intelligence, 36 2022, 11103–11111. https://doi.org/10.1609/aaai.v36i10.21359 |
[18] |
Y. Liu, J. Gu, N. Goyal, X. Li, S. Edunov, M. Ghazvininejad, et al., Multilingual denoising pre-training for neural machine translation, Trans. Assoc. Comput. Linguist., 8 (2020), 726–742. https://doi.org/10.1162/tacl_a_00343 doi: 10.1162/tacl_a_00343
![]() |
[19] | L. Liebel, M. Körner, Auxiliary tasks in multi-task learning, preprint, arXiv: 1805.06334. |
[20] | J. Zhu, H. Li, T. Liu, Y. Zhou, J. Zhang, C. Zong, MSMO: Multimodal summarization with multimodal output, in Proceedings of the 2018 Conference on Empirical Methods in Natural Language Processing, (2018), 4154–4164. https://doi.org/10.18653/v1/D18-1448 |
[21] | C. Y. Lin, ROUGE: A package for automatic evaluation of summaries, in Text Summarization Branches Out, Association for Computational Linguistics, Barcelona, Spain, (2004), 74–81. |
[22] | R. Cipolla, Y. Gal, A. Kendall, Multi-task learning using uncertainty to weigh losses for scene geometry and semantics, in Proceedings of the IEEE Conference on Computer Vision and Pattern Recognition (CVPR), (2018), 7482–7491. |
[23] | S. Kiritchenko, S. Mohammad, Best-worst scaling more reliable than rating scales: A case study on sentiment intensity annotation, in Proceedings of the 55th Annual Meeting of the Association for Computational Linguistics, (2017), 465–470. https://doi.org/10.18653/v1/P17-2074 |
1. | Jiushun Ma, Yuxin Huang, Linqin Wang, Xiang Huang, Hao Peng, Zhengtao Yu, Philip Yu, Augmenting Low-Resource Cross-Lingual Summarization with Progression-Grounded Training and Prompting, 2024, 23, 2375-4699, 1, 10.1145/3675167 | |
2. | Shaohuan Cheng, Wenyu Chen, Yujia Tang, Mingsheng Fu, Hong Qu, Unified Training for Cross-Lingual Abstractive Summarization by Aligning Parallel Machine Translation Pairs, 2024, 12, 2227-7390, 2107, 10.3390/math12132107 |
Vi2ZhLow | train | valid | test | En2ZhLow | train | valid | test | Zh2EnLow | train | valid | test |
#Docs | 5,000 | 3,000 | 3,000 | #Docs | 5,000 | 3,000 | 3,000 | #Docs | 5,000 | 3,000 | 3,000 |
#AvgW (S) | 715 | 715 | 717 | #AvgW (S) | 755 | 760 | 745 | #AvgC (S) | 104 | 104 | 104 |
#AvgW (R) | 48 | 48 | 48 | #AvgW (R) | 55 | 55 | 55 | #AvgC (R) | 18 | 18 | 18 |
#AvgC (R) | 53 | 53 | 54 | #AvgC (R) | 96 | 96 | 95 | #AvgW (R) | 14 | 14 | 14 |
S represents source texts, R represents reference summaries. AvgW (S) represents the average number of Vietnamese/English words in the source texts. AvgW (R) represents the average number of words in the Vietnamese/English summaries. AvgC (S) represents the average number of Chinese characters in the source texts. AvgC (R) represents the average number of characters in Chinese summaries. |
Model | # of training samples | Vi2ZhLow | En2ZhLow | Zh2EnLow | ||||||||
R-1 | R-2 | R-L | R-1 | R-2 | R-L | R-1 | R-2 | R-L | ||||
NCLS | 1000 | 23.362 | 3.161 | 20.889 | 20.093 | 2.221 | 17.954 | 13.723 | 1.122 | 12.920 | ||
3000 | 24.753 | 4.429 | 21.296 | 21.999 | 3.037 | 19.769 | 14.279 | 1.329 | 13.729 | |||
5000 | 25.234 | 5.015 | 21.828 | 22.345 | 3.285 | 19.715 | 15.564 | 1.555 | 14.265 | |||
NCLS+MS | 1000 | 22.777 | 3.740 | 20.057 | 13.148 | 1.974 | 11.772 | 15.419 | 1.169 | 13.785 | ||
3000 | 23.916 | 4.079 | 20.695 | 18.536 | 3.043 | 15.660 | 13.788 | 1.245 | 12.272 | |||
5000 | 24.892 | 4.477 | 22.145 | 20.284 | 3.034 | 17.770 | 14.437 | 1.463 | 12.876 | |||
MCLAS | 1000 | 24.395 | 7.467 | 19.760 | 22.625 | 4.609 | 14.383 | 10.364 | 1.456 | 9.270 | ||
3000 | 27.598 | 9.853 | 22.430 | 23.872 | 6.257 | 16.522 | 13.376 | 2.748 | 11.960 | |||
5000 | 28.086 | 10.288 | 22.875 | 29.031 | 9.459 | 19.690 | 16.642 | 3.785 | 14.701 | |||
Nguyen et al. | 1000 | 25.262 | 8.064 | 20.287 | 23.930 | 5.045 | 15.354 | 10.894 | 2.037 | 10.106 | ||
3000 | 27.617 | 9.846 | 22.405 | 25.880 | 7.212 | 17.587 | 15.003 | 3.356 | 13.615 | |||
5000 | 29.030 | 10.665 | 23.503 | 31.147 | 10.577 | 20.998 | 16.552 | 4.147 | 14.939 | |||
mBART-CLS | 1000 | 26.557 | 7.553 | 21.875 | 26.912 | 7.839 | 19.004 | 16.575 | 3.271 | 14.275 | ||
3000 | 28.816 | 9.310 | 24.035 | 30.233 | 10.632 | 21.383 | 19.782 | 4.755 | 16.891 | |||
5000 | 29.386 | 9.664 | 24.407 | 32.122 | 12.251 | 22.968 | 21.197 | 5.631 | 18.139 | |||
TFLCLS | 1000 | 28.192 | 8.439 | 23.292 | 28.555 | 9.219 | 20.502 | 18.182 | 4.065 | 15.599 | ||
3000 | 30.600 | 10.540 | 25.436 | 32.177 | 12.483 | 23.107 | 21.384 | 5.542 | 18.217 | |||
5000 | 31.627 | 11.489 | 26.568 | 33.234 | 13.808 | 24.272 | 22.645 | 6.583 | 19.609 |
Model | # of training samples | Vi2ZhLow | En2ZhLow | Zh2EnLow | ||||||||
R-1 | R-2 | R-L | R-1 | R-2 | R-L | R-1 | R-2 | R-L | ||||
TFLCLSbase | 1000 | 28.376 | 8.579 | 23.494 | 28.573 | 8.842 | 20.041 | 17.935 | 4.036 | 15.522 | ||
3000 | 30.420 | 10.259 | 25.414 | 32.399 | 12.776 | 23.351 | 20.943 | 5.478 | 17.888 | |||
5000 | 31.411 | 11.380 | 26.334 | 33.009 | 13.652 | 24.243 | 22.587 | 6.542 | 19.568 | |||
TFLCLSHU | 1000 | 28.097 | 8.178 | 23.088 | 28.426 | 8.896 | 20.032 | 17.954 | 4.131 | 15.422 | ||
3000 | 30.472 | 10.189 | 25.333 | 32.204 | 12.804 | 23.381 | 21.088 | 5.468 | 17.951 | |||
5000 | 31.126 | 11.064 | 26.022 | 33.151 | 13.628 | 24.201 | 22.628 | 6.525 | 19.468 | |||
TFLCLSRHU | 1000 | 28.156 | 8.303 | 23.303 | 28.670 | 8.920 | 20.257 | 18.077 | 4.028 | 15.569 | ||
3000 | 30.439 | 10.278 | 25.363 | 32.194 | 12.571 | 23.116 | 21.106 | 5.519 | 17.975 | |||
5000 | 31.366 | 11.379 | 26.325 | 33.772 | 13.753 | 24.201 | 22.296 | 6.484 | 19.360 | |||
TFLCLS | 1000 | 28.192 | 8.439 | 23.292 | 28.555 | 9.219 | 20.502 | 18.182 | 4.065 | 15.599 | ||
3000 | 30.600 | 10.540 | 25.436 | 32.177 | 12.483 | 23.107 | 21.384 | 5.542 | 18.217 | |||
5000 | 31.627 | 11.489 | 26.568 | 33.234 | 13.808 | 24.272 | 22.645 | 6.583 | 19.609 |
Model | 1000 training samples | 3000 training samples | 5000 training samples | ||||||||
IF | CC | FL | IF | CC | FL | IF | CC | FL | |||
NCLS | –0.365 | –0.503 | –0.382 | –0.354 | –0.423 | –0.400 | –0.383 | –0.503 | –0.423 | ||
NCLS+MS | –0.331 | –0.342 | –0.314 | –0.331 | –0.440 | –0.463 | –0.394 | –0.434 | –0.377 | ||
MCLAS | –0.154 | –0.057 | –0.240 | –0.171 | –0.051 | –0.068 | –0.143 | –0.046 | –0.074 | ||
Nguyen et al. | –0.137 | –0.029 | –0.137 | –0.114 | –0.051 | –0.040 | –0.040 | –0.023 | –0.103 | ||
TFLCLS | 0.148 | 0.171 | 0.200 | 0.297 | 0.326 | 0.251 | 0.331 | 0.337 | 0.394 | ||
Reference | 0.851 | 0.800 | 0.771 | 0.737 | 0.703 | 0.657 | 0.708 | 0.629 | 0.566 |
Vi2ZhLow | train | valid | test | En2ZhLow | train | valid | test | Zh2EnLow | train | valid | test |
#Docs | 5,000 | 3,000 | 3,000 | #Docs | 5,000 | 3,000 | 3,000 | #Docs | 5,000 | 3,000 | 3,000 |
#AvgW (S) | 715 | 715 | 717 | #AvgW (S) | 755 | 760 | 745 | #AvgC (S) | 104 | 104 | 104 |
#AvgW (R) | 48 | 48 | 48 | #AvgW (R) | 55 | 55 | 55 | #AvgC (R) | 18 | 18 | 18 |
#AvgC (R) | 53 | 53 | 54 | #AvgC (R) | 96 | 96 | 95 | #AvgW (R) | 14 | 14 | 14 |
S represents source texts, R represents reference summaries. AvgW (S) represents the average number of Vietnamese/English words in the source texts. AvgW (R) represents the average number of words in the Vietnamese/English summaries. AvgC (S) represents the average number of Chinese characters in the source texts. AvgC (R) represents the average number of characters in Chinese summaries. |
Model | # of training samples | Vi2ZhLow | En2ZhLow | Zh2EnLow | ||||||||
R-1 | R-2 | R-L | R-1 | R-2 | R-L | R-1 | R-2 | R-L | ||||
NCLS | 1000 | 23.362 | 3.161 | 20.889 | 20.093 | 2.221 | 17.954 | 13.723 | 1.122 | 12.920 | ||
3000 | 24.753 | 4.429 | 21.296 | 21.999 | 3.037 | 19.769 | 14.279 | 1.329 | 13.729 | |||
5000 | 25.234 | 5.015 | 21.828 | 22.345 | 3.285 | 19.715 | 15.564 | 1.555 | 14.265 | |||
NCLS+MS | 1000 | 22.777 | 3.740 | 20.057 | 13.148 | 1.974 | 11.772 | 15.419 | 1.169 | 13.785 | ||
3000 | 23.916 | 4.079 | 20.695 | 18.536 | 3.043 | 15.660 | 13.788 | 1.245 | 12.272 | |||
5000 | 24.892 | 4.477 | 22.145 | 20.284 | 3.034 | 17.770 | 14.437 | 1.463 | 12.876 | |||
MCLAS | 1000 | 24.395 | 7.467 | 19.760 | 22.625 | 4.609 | 14.383 | 10.364 | 1.456 | 9.270 | ||
3000 | 27.598 | 9.853 | 22.430 | 23.872 | 6.257 | 16.522 | 13.376 | 2.748 | 11.960 | |||
5000 | 28.086 | 10.288 | 22.875 | 29.031 | 9.459 | 19.690 | 16.642 | 3.785 | 14.701 | |||
Nguyen et al. | 1000 | 25.262 | 8.064 | 20.287 | 23.930 | 5.045 | 15.354 | 10.894 | 2.037 | 10.106 | ||
3000 | 27.617 | 9.846 | 22.405 | 25.880 | 7.212 | 17.587 | 15.003 | 3.356 | 13.615 | |||
5000 | 29.030 | 10.665 | 23.503 | 31.147 | 10.577 | 20.998 | 16.552 | 4.147 | 14.939 | |||
mBART-CLS | 1000 | 26.557 | 7.553 | 21.875 | 26.912 | 7.839 | 19.004 | 16.575 | 3.271 | 14.275 | ||
3000 | 28.816 | 9.310 | 24.035 | 30.233 | 10.632 | 21.383 | 19.782 | 4.755 | 16.891 | |||
5000 | 29.386 | 9.664 | 24.407 | 32.122 | 12.251 | 22.968 | 21.197 | 5.631 | 18.139 | |||
TFLCLS | 1000 | 28.192 | 8.439 | 23.292 | 28.555 | 9.219 | 20.502 | 18.182 | 4.065 | 15.599 | ||
3000 | 30.600 | 10.540 | 25.436 | 32.177 | 12.483 | 23.107 | 21.384 | 5.542 | 18.217 | |||
5000 | 31.627 | 11.489 | 26.568 | 33.234 | 13.808 | 24.272 | 22.645 | 6.583 | 19.609 |
Model | # of training samples | Vi2ZhLow | En2ZhLow | Zh2EnLow | ||||||||
R-1 | R-2 | R-L | R-1 | R-2 | R-L | R-1 | R-2 | R-L | ||||
TFLCLSbase | 1000 | 28.376 | 8.579 | 23.494 | 28.573 | 8.842 | 20.041 | 17.935 | 4.036 | 15.522 | ||
3000 | 30.420 | 10.259 | 25.414 | 32.399 | 12.776 | 23.351 | 20.943 | 5.478 | 17.888 | |||
5000 | 31.411 | 11.380 | 26.334 | 33.009 | 13.652 | 24.243 | 22.587 | 6.542 | 19.568 | |||
TFLCLSHU | 1000 | 28.097 | 8.178 | 23.088 | 28.426 | 8.896 | 20.032 | 17.954 | 4.131 | 15.422 | ||
3000 | 30.472 | 10.189 | 25.333 | 32.204 | 12.804 | 23.381 | 21.088 | 5.468 | 17.951 | |||
5000 | 31.126 | 11.064 | 26.022 | 33.151 | 13.628 | 24.201 | 22.628 | 6.525 | 19.468 | |||
TFLCLSRHU | 1000 | 28.156 | 8.303 | 23.303 | 28.670 | 8.920 | 20.257 | 18.077 | 4.028 | 15.569 | ||
3000 | 30.439 | 10.278 | 25.363 | 32.194 | 12.571 | 23.116 | 21.106 | 5.519 | 17.975 | |||
5000 | 31.366 | 11.379 | 26.325 | 33.772 | 13.753 | 24.201 | 22.296 | 6.484 | 19.360 | |||
TFLCLS | 1000 | 28.192 | 8.439 | 23.292 | 28.555 | 9.219 | 20.502 | 18.182 | 4.065 | 15.599 | ||
3000 | 30.600 | 10.540 | 25.436 | 32.177 | 12.483 | 23.107 | 21.384 | 5.542 | 18.217 | |||
5000 | 31.627 | 11.489 | 26.568 | 33.234 | 13.808 | 24.272 | 22.645 | 6.583 | 19.609 |
Model | 1000 training samples | 3000 training samples | 5000 training samples | ||||||||
IF | CC | FL | IF | CC | FL | IF | CC | FL | |||
NCLS | –0.365 | –0.503 | –0.382 | –0.354 | –0.423 | –0.400 | –0.383 | –0.503 | –0.423 | ||
NCLS+MS | –0.331 | –0.342 | –0.314 | –0.331 | –0.440 | –0.463 | –0.394 | –0.434 | –0.377 | ||
MCLAS | –0.154 | –0.057 | –0.240 | –0.171 | –0.051 | –0.068 | –0.143 | –0.046 | –0.074 | ||
Nguyen et al. | –0.137 | –0.029 | –0.137 | –0.114 | –0.051 | –0.040 | –0.040 | –0.023 | –0.103 | ||
TFLCLS | 0.148 | 0.171 | 0.200 | 0.297 | 0.326 | 0.251 | 0.331 | 0.337 | 0.394 | ||
Reference | 0.851 | 0.800 | 0.771 | 0.737 | 0.703 | 0.657 | 0.708 | 0.629 | 0.566 |