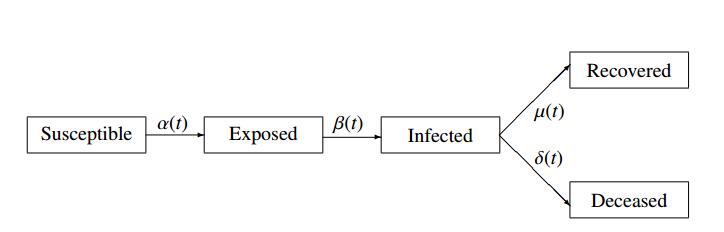
The application of 3D reconstruction technology in building images has been a novel research direction. In such scenes, the reconstruction with proper building details remains challenging. To deal with this issue, I propose a KD-tree and random sample consensus-based 3D reconstruction model for 2D building images. Specifically, the improved KD-tree algorithm with the random sampling consistency algorithm has a better matching rate for the two-dimensional image data extraction of the stadium scene. The number of discrete areas in the stadium scene increases with the increase in the number of images. The sparse 3D models can be transformed into dense 3D models to some extent using the screening method. In addition, we carry out some simulation experiments to assess the performance of the proposed algorithm in this paper in terms of stadium scenes. The results reflect that the error of the proposal is significantly lower than that of the comparison algorithms. Therefore, it is proven that the proposal can be well-suitable for 3D reconstruction in building images.
Citation: Xiaoli Li. A KD-tree and random sample consensus-based 3D reconstruction model for 2D sports stadium images[J]. Mathematical Biosciences and Engineering, 2023, 20(12): 21432-21450. doi: 10.3934/mbe.2023948
[1] | Antonio N. Bojanic, Alejandro Jordán . Modeling the COVID-19 epidemic in Bolivia. Big Data and Information Analytics, 2020, 5(1): 47-57. doi: 10.3934/bdia.2020004 |
[2] | Ugo Avila-Ponce de León, Ángel G. C. Pérez, Eric Avila-Vales . A data driven analysis and forecast of an SEIARD epidemic model for COVID-19 in Mexico. Big Data and Information Analytics, 2020, 5(1): 14-28. doi: 10.3934/bdia.2020002 |
[3] | Nickson Golooba, Woldegebriel Assefa Woldegerima, Huaiping Zhu . Deep neural networks with application in predicting the spread of avian influenza through disease-informed neural networks. Big Data and Information Analytics, 2025, 9(0): 1-28. doi: 10.3934/bdia.2025001 |
[4] | Antonio N. Bojanic . Accounting for the Trump factor in modeling the COVID-19 epidemic: the case of Louisiana. Big Data and Information Analytics, 2021, 6(0): 74-85. doi: 10.3934/bdia.2021006 |
[5] | Jiaqi Ma, Hui Chang, Xiaoqing Zhong, Yueli Chen . Risk stratification of sepsis death based on machine learning algorithm. Big Data and Information Analytics, 2024, 8(0): 26-42. doi: 10.3934/bdia.2024002 |
[6] | Yiwen Tao, Zhenqiang Zhang, Bengbeng Wang, Jingli Ren . Motality prediction of ICU rheumatic heart disease with imbalanced data based on machine learning. Big Data and Information Analytics, 2024, 8(0): 43-64. doi: 10.3934/bdia.2024003 |
[7] | Bill Huajian Yang . Modeling path-dependent state transitions by a recurrent neural network. Big Data and Information Analytics, 2022, 7(0): 1-12. doi: 10.3934/bdia.2022001 |
[8] | Bill Huajian Yang . Resolutions to flip-over credit risk and beyond-least squares estimates and maximum likelihood estimates with monotonic constraints. Big Data and Information Analytics, 2018, 3(2): 54-67. doi: 10.3934/bdia.2018007 |
[9] | Anupama N, Sudarson Jena . A novel approach using incremental under sampling for data stream mining. Big Data and Information Analytics, 2018, 3(1): 1-13. doi: 10.3934/bdia.2017017 |
[10] | Amanda Working, Mohammed Alqawba, Norou Diawara, Ling Li . TIME DEPENDENT ATTRIBUTE-LEVEL BEST WORST DISCRETE CHOICE MODELLING. Big Data and Information Analytics, 2018, 3(1): 55-72. doi: 10.3934/bdia.2018010 |
The application of 3D reconstruction technology in building images has been a novel research direction. In such scenes, the reconstruction with proper building details remains challenging. To deal with this issue, I propose a KD-tree and random sample consensus-based 3D reconstruction model for 2D building images. Specifically, the improved KD-tree algorithm with the random sampling consistency algorithm has a better matching rate for the two-dimensional image data extraction of the stadium scene. The number of discrete areas in the stadium scene increases with the increase in the number of images. The sparse 3D models can be transformed into dense 3D models to some extent using the screening method. In addition, we carry out some simulation experiments to assess the performance of the proposed algorithm in this paper in terms of stadium scenes. The results reflect that the error of the proposal is significantly lower than that of the comparison algorithms. Therefore, it is proven that the proposal can be well-suitable for 3D reconstruction in building images.
An outbreak of pneumonia initially took place in Wuhan, China, which turned out to be coronavirus disease 2019 (COVID-19), caused by a novel coronavirus (SARS-CoV-2). The pandemic has brought about devastating public health, economic, and political crises. The outbreak has spread to 222 countries and territories, inflicted more than 116.17 million confirmed cases, and claimed more than 2.58 million lives, as reported on March 7th, 2021 [1]. People residing in the United States (US) have paid a high price; the pandemic has caused more than 29.05 million confirmed cases and claimed 527,726 lives, as reported on March 11th, 2021 [2,3]. The emergence of COVID-19 pandemic demonstrates the importance of research on accurately understanding and modeling the transmission and spread of the COVID-19 and SARS-CoV-2 [4,5,6]. It is essential to understand the mathematical mechanism and dynamics of COVID-19 to predict the pandemic better and evaluate the effects of intervention strategies and offer effective policies [7]. This paper is motivated by the need for in-depth investigations.
The COVID-19 is a communicable disease. An exposed person may interact with and transmit the virus to a susceptible individual. An interaction between the exposed person and the susceptible individual may result in the susceptible individual becoming exposed. An exposed individual may experience an incubation period before becoming ill, i.e., infected. Thus, an exposed person is infectious but not yet infected. An infected individual may be lucky enough to recover, at least to some extent, after a period of time. Alternatively, they will die. This process can be described by a dynamical system of susceptible-exposed-infected-recovered-deceased (SEIRD) differential equations, which can connect the numbers of susceptible, exposed, infected, recovered, and deceased individuals. Figure 1 shows the SEIRD model flow diagram of the transmission of COVID-19.
In the Figure 1, α(t) is the contact rate between susceptible and exposed people, β(t) is the incidence rate of the exposed people becoming infected, μ(t) is the recovery rate of the infected individuals, and δ(t) is the mortality rate of the infected individuals. In the literature, a system of susceptible-exposed-infected-recovered (SEIR) differential equations was developed to describe diseases like chickenpox and vector-borne diseases such as dengue hemorrhagic fever [8,9,10,11,12,13,14,15,16,17,18,19,20,21,22,23,24,25,26,27,28,29,30,31,32]. These diseases have a long incubation period, during which time the exposed individual cannot yet transmit the pathogen to others. The SEIR assumes that only an infected individual is infectious and can transmit the virus to a susceptible person and an exposed person is not infectious, as with susceptible-infected-recovered (SIR) models. For the very dangerous COVID-19, an exposed individual is infectious and can transmit the virus to susceptible people rapidly. An infected person is infectious but may be sent to hospitals quickly and separated from the susceptible people, making them unlikely to transmit the virus to susceptible people. Therefore, the existing SEIR and SIR systems cannot be used to analyze the COVID-19, and we need to build new models to fill the gap.
To get an intuitive idea of these rates for the COVID-19 transmission process, we obtain population data from US Census Bureau and COVID-19 daily reports from the Johns Hopkins University website through a GitHub repository [4,5,6]. The data are then combined for a unified analysis. We then calculate the empirical incidence, mortality, and recovered rates. Figure 2 provides trajectories of total numbers of people tested, confirmed cases, deaths, and recovered as well as related linear regression lines for COVID-19 in the US from April 16th to June 30th, 2020.
In Figures 3 and S1, we show trajectories of empirical testing rate, incidence rate, mortality rate, and recovery rate as well as related quadratic and linear regression lines for COVID-19 based on the results of Figure 2, respectively. It can be seen from the Figures 3 and S1 that both the testing rate and recovery rate increase, the incidence rate decreases, and the mortality rate increases at the beginning and then remains relatively stable before decreasing. The features of these rate may be due to people's being inexperienced at the beginning of the pandemic but gradually becoming more experienced at dealing with it and getting better prepared.
The empirical results shown in Figures 1, 3 and S1 provide us with a rationale for building the models. One noticeable feature is that these rates are not constants but time-dependent. Figures 1, 3 and S1 are based on COVID-19 data in the US from April 16th to June 30th, 2020, in which the sample size is large, and the deterministic SEIRD system is appropriate. The results of Figures 1, 3 and S1 provide a good estimate of the incidence, mortality, and recovery rates of COVID-19. Based on the empirical results, we can consider a few cases of contact, incidence, recovery, and mortality rates to model dynamics of COVID-19.
In this article, we build a system of SEIRD ordinary differential equations to describe relationship among the numbers of susceptible, exposed, infected, recovered, and deceased individuals in a town, state or country based on the empirical rates. The organization of the paper is as follows. In section 2, we build SEIRD models in details. We perform empirical analysis for the SEIRD models in section 3. In section 4, we provide some discussion about the property of the SEIRD models and some thoughts about future research direction.
Consider an independent time variable t which is measured in days. At time t, let s(t) be the number of susceptible individuals in a town, state or country; e(t), the number of exposed individuals that have transmitted the virus; i(t), the number of exposed individuals that are infected; r(t), the number of infected individuals that have recovered; and d(t), the number of infected individuals that have died. To describe the transmission process of COVID-19, consider a SEIRD system of ordinary differential equations
ds(t)dt=−α(t)s(t)e(t),de(t)dt=α(t)s(t)e(t)−β(t)e(t),di(t)dt=β(t)e(t)−μ(t)i(t)−δ(t)i(t),dr(t)dt=μ(t)i(t),dd(t)dt=δ(t)i(t), | (2.1) |
with initial conditions s(0)=S0,e(0)=E0,i(0)=I0,r(0)=0, and d(0)=0. In the system (2.1), α(t) is contact rate, β(t) is incidence rate, μ(t) is recovery rate, and δ(t) is mortality rate displayed in the Figure 1. Note all these rates are non-negative. The first equation in the system (2.1) is called susceptible equation, the second equation in the system (2.1) is called exposed equation, the third is called infected equation, the fourth is called recovery equation, and the fifth is called deceased equation, respectively. The system (2.1) is different from those in the literature of SEIR system as the susceptible and exposed equations are different. In the SEIR models, it is assumed that only an infected individual can transmit the virus to a susceptible person like SIR models and so the first two SEIR equations are
ds(t)dt=−α(t)s(t)i(t),de(t)dt=α(t)s(t)i(t)−β(t)e(t). |
For COVID-19, however, an exposed individual is infectious and may interact with a susceptible individual, thus transmitting the virus. This transmission is described by the susceptible equation in system (2.1). The exposed equation in system (2.1) implies that an exposed individual may contract coronavirus and experience an incubation period before becoming ill, i.e., infected. Once contracting the virus, an exposed person can transmit the virus to a susceptible individual. The infected can either recover with a recovery rate μ(t) or die with a mortality rate δ(t).
Let us denote N=S0+E0+I0. Note
ds(t)dt+de(t)dt+di(t)dt+dr(t)dt+dd(t)dt=0. |
It follows that s(t)+e(t)+i(t)+r(t)+d(t)=N is a constant. Since ds(t)dt=−α(t)s(t)e(t)≤0, the susceptible function s(t) is a decreasing function. Similarly, the recovery function r(t) and deceased function d(t) are increasing functions since dr(t)dt=μ(t)i(t)≥0 and dd(t)dt=δ(t)i(t)≥0, respectively. In the following, we impose conditions on incidence rate β(t), recovery rate μ(t), and mortality rate δ(t) based on the empirical results of the Figures 3 and S1 as follows
Quadratic decline of incidence rate: β(t)=β0−β1t+β2t2≥0,β0>0,β1>0,β2>0,Linear decline of incidence rate: β(t)=β0−β1t≥0,β0>0,β1>0,Linear increase of recovery rate: μ(t)=μ0+μ1t,μ0≥0,μ1≥0,Quadratic mortality rate: δ(t)=δ0+δ1t+δ2t2≥0,δ0≥0,δ1≥0,δ2<0. | (2.2) |
As shown in Figure 3, a declining incidence rate implies that the proportion of infected people is high at the beginning but decreases as time goes by. The empirical mortality rate is assumed to be a quadratic curve. It implies that the mortality rate increases initially, after which it remains stable and eventually decreases. One possible reason for this is that the virus is strongly virulent at the beginning but gradually weakens. Also, healthcare for infected people improves, and this contributes to an eventual decline in mortality. On the other hand, an increasing recovery rate is most likely due to improving healthcare and the fact that people are more experienced at handling the disease as time progresses. We assume that the recovery rate is linearly increasing from the empirical result of COVID-19 in the US, as shown in Figure 3.
For the contact rate α(t), we consider an exponential declining rate: α(t)=α0exp(−α1t),α0>0,α1>0. The rationale for a declining contact rate is that people may contact each other as normal at the beginning stage of the pandemic but reduce contact quickly as time moves on. The exponential decline means that the contact reduces to a minimum fast.
From the susceptible equation and the exposed equation in the system (2.1), we have
de(t)ds(t)=−1+β(t)α(t)s(t). |
Integrating the above equation gives
e(t)=E0+S0−s(t)+∫t0β(u)α(u)s(u)ds(u). |
If α(t)=α0 and β(t)=β0 are constants, the above equation provides an exact solution
e(t)=E0+S0−s(t)+1qln(s(t)/S0),q=α0β0. | (2.3) |
In addition, de(t)ds(t)=−1+β0α0s(t)=0 implies that e(t) reaches its maximum when s(t)=1/q. Hence, the maximal number of exposed individual is given by
emax=E0+S0−1q(1+ln(qS0)), if α(t)=α0 and β(t)=β0. | (2.4) |
Under an assumption of constant contacting rate α(t)=α0 and constant incidence rate β(t)=β0, we obtain the exposed function e(t) and the maximal number of exposed individual emax in relations (2.3) and (2.4). In general, the contact rates, incidence rates, recovery rates, and mortality rates are functions of time variable t. An exact solutions of SEIRD system (2.1) is not possible. Instead, a numerical approximation procedure can provide us a solution via Euler's method [33,34,35]. Taking a small time interval Δt and increment Δs(t)=s(t+Δt)−s(t), we may make an approximation ds(t)dt=Δs(t)Δt. Similarly, we may approximate di(t)dt=Δi(t)Δt, where Δi(t)=i(t+Δt)−i(t), etc. Then, we may obtain a system of recurrence equations as follows to approximate the numbers of susceptible, exposed, infected, recovered, and deceased
s(t+Δt)=s(t)−α(t)s(t)e(t)Δt,e(t+Δt)=e(t)+[α(t)s(t)e(t)−β(t)e(t)]Δt,i(t+Δt)=i(t)+[β(t)e(t)−μ(t)i(t)−δ(t)i(t)]Δt,r(t+Δt)=r(t)+μ(t)i(t)Δt,d(t+Δt)=d(t)+δ(t)i(t)Δt. | (3.1) |
Using the recurrence system (3.1) and initial conditions s(0)=S0,e(0)=E0,i(0)=I0,r(0)=0, and d(0)=0, we perform numerical approximations for a few cases of the rates defined in relations (2.2).
By using the rate functions provided in the Figure 3, we perform numerical calculation for approximate numbers of susceptible, exposed, infected, and deceased. The incidence, recovery, and mortality rates are from Figure 3: β(t)=0.01(21.0−0.30t+0.0018t2), μ(t)=0.01(14.65+0.188t), and δ(t)=0.01(5.25+0.045t−0.00067t2). The initial conditions are given by S0=106,E0=10, and I0=2. We consider four exponential decline contact functions α(t)=1.2×10−6exp(α1t): (a) α1=−0.01, (b) α1=−0.025, (c) α1=−0.05, and (d) α1=−0.065, respectively. Thus, the exponential declining rates of the four contact functions decrease from −0.01 to −0.065. The results are plotted in Figure 4.
It can be seen from Figure 4(a), (b) that the number of susceptible individuals rapidly decreases to zero when α1=−0.01 and α1=−0.025. If α1=−0.05, the number of susceptible individuals decreases and stabilizes around 100,000 when the time t is about 30 days (Figure 4 (c)); if α1=−0.065, the number of susceptible individuals decreases and stabilizes around 500,000 when the time t is about 30 days (Figure 4 (d)). For each plot, the numbers of exposed and infected start low and then increase. Once they reach their peak, they decrease to low numbers again. When α1=−0.01 and α1=−0.025, both the numbers of exposed and infected reach high levels when the time t is about 15 days, while fewer people are exposed and infected when α1=−0.05 and α1=−0.065, respectively. The numbers of recovered and deceased individuals increase. The numbers of recovered and deceased decrease as the exponential declining rate decreases exponentially from –0.01 to –0.065, e.g., the curve of recovered in Figure 4(a) is higher than that in Figure 4(d).
In the supplementary materials, we consider another set of incidence, recovery, and mortality rates from Figure S1: β(t)=0.01(19.25−0.17t),μ(t)=0.01(14.65+0.20t), and δ(t)=0.01(5.25+0.045t−0.001t2). In this set of rates, the incidence rate is fitted by linear regression, instead of a quadratic regression in Figure 3. Again, the initial conditions are given by S0=106,E0=10, and I0=2. Using the rates, approximate numbers of susceptible, exposed, infected, and deceased are given in Figure S2, which provides similar results as Figure 4.
In Figure 5, we use another set of rates to calculate approximate numbers of susceptible, exposed, infected, and deceased: β(t)=0.01(14.5−0.25t),μ(t)=0.01(8.5+0.30t), and δ(t)=0.01(4.5−0.05t−0.001t2). The features of the numbers of susceptible, recovered, and deceased are similar to that of Figure 4. However, the number of exposed is still high and does no reach a low level when time t is around 50 days, and the number of infected does not stabilize when time t is around 50 days. Hence, this set of rate functions leads to a good fit for the transmissions and progressions of US COVID-19 pandemic.
In this article, we build SEIRD differential equations to describe relationships among the numbers of susceptible, exposed, infected, recovered, and deceased individuals. Based on the empirical rates, empirical analyses for the SEIRD models are carried out to explore the applications and implications of the models. For COVID-19, a susceptible individual may interact with an exposed person and contract the virus. Hence, an exposed individual is infectious and can transmit the virus to susceptible people rapidly. The SEIRD system differs from SEIR and SIR differential equations in the literature. In these literature models, only an infected individual is infectious and can transmit the virus to a susceptible person. The proposed SEIRD system can directly describe the transmission of COVID-19 in a susceptible population.
Using the US empirical incidence, mortality, and recovery rate functions of COVID-19, we calculate approximate numbers of susceptible, exposed, infected, recovered, and deceased. The incidence, recovery, and mortality rate functions are functions of time in days and are not constant. We consider four exponential decline contact functions to investigate the impact of contact rate on the transmission of COVID-19. An exponential declining contact rate implies that people have contact with each other as usual at the beginning, with a reduction in contact quickly later on. Our empirical results show that a very rapid decline in contact significantly reduces the numbers of exposed, infected, and deceased. Therefore, it makes sense to wear masks and reduce social activities during the pandemic.
In the literature, the SEIR models were extended to be a susceptible-exposed-infected-recovered-susceptible (SEIRS) models, where recovered people may become susceptible again, i.e., recovery does not confer lifelong immunity [25]. For example, rotavirus and malaria are diseases with long incubation periods, and recovery only confers temporary immunity. In the SEIRD system (2.1), we assume immunity for COVID-19 and that recovery confers lifelong immunity. The models can be extended to remove this assumption. As time progresses, we may have empirical data supporting the fact that recovery does not confer lifelong immunity for COVID-19; SEIRD system (2.1) can then be extended to accommodate the finding. Another extension of SEIRD system (2.1) allows for immunity by vaccination. Because vaccine rollout began in December 2020, its effectiveness and influence can be evaluated and provide us with data to help us develop an extension of the SEIRD system that will accommodate immunity by vaccination.
In this article, we only consider a deterministic system of SEIRD differential equations which can not capture random fluctuations of the numbers of susceptible, exposed, infected, recovered, and deceased individuals. The deterministic models can be extended to include stochastic components to describe random fluctuations of the virus transmissions of COVID-19 and viral phylodynamics of SARS-CoV-2 [36,37,38,39,40,41,42,43,44,45]. More research is needed for further investigations.
This study was supported by U.S. National Science Foundation grant DMS-1915904 (Ruzong Fan). One anonymous reviewer and Dr. Jianhong Wu, provided very good and insightful comments for us to improve the manuscript. Mr. Marion L. Hartley helped us to edit the paper. Ruzong Fan conceives the project; Shuqi Wang, Wen Tang, Liyan Xiong, Menyu Fang, and Chi-Yang Chiu provide technical support for the project.
All authors declare no conflicts of interest in this paper.
In the supplementary materials, we consider another set of incidence, recovery, and mortality rates from Figure S1: β(t)=0.01(19.25−0.17t),μ(t)=0.01(14.65+0.20t), and δ(t)=0.01(5.25+0.045t−0.001t2). In this set of rates, the incidence rate is fitted by linear regression, instead of a quadratic regression in Figure 3. Again, the initial conditions are given by S0=106,E0=10, and I0=2. Using the rates, approximate numbers of susceptible, exposed, infected, and deceased are given in Figure S2, which provides similar results as Figure 4.
[1] |
B. Ahmad, P. A. Floor, I. Farup, Ø. Hovde, 3D reconstruction of gastrointestinal regions using single-view methods, IEEE Access, 11 (2023), 61103–61117. https://doi.org/10.1109/ACCESS.2023.3286937 doi: 10.1109/ACCESS.2023.3286937
![]() |
[2] |
Z. Cui, J. Feng, J. Zhou, Monocular 3D fingerprint reconstruction and unwarping, IEEE Trans. Pattern Anal. Mach. Intell., 45 (2023), 8679–8695. https://doi.org/10.1109/TPAMI.2022.3233898 doi: 10.1109/TPAMI.2022.3233898
![]() |
[3] |
H. Choi, M. Lee, J. Kang, D. Lee, Online 3D edge reconstruction of wiry structures from monocular image sequences, IEEE Rob. Autom. Lett., 8 (2023), 7479–7486. https://doi.org/10.1109/LRA.2023.3320022 doi: 10.1109/LRA.2023.3320022
![]() |
[4] |
Y. Ding, Z. Chen, Y. Ji, J. Yu, J. Ye, Light field-based underwater 3D reconstruction via angular re-sampling, IEEE Trans. Comput. Imaging, 9 (2023), 881–893. https://doi.org/10.1109/TCI.2023.3319983 doi: 10.1109/TCI.2023.3319983
![]() |
[5] |
M. Pistellato, F. Bergamasco, A. Torsello, F. Barbariol, J. Yoo, J. Y. Jeong, et al., A physics-driven CNN model for real-time sea waves 3D reconstruction, Remote Sens., 13 (2021), 3780. https://doi.org/10.3390/rs13183780 doi: 10.3390/rs13183780
![]() |
[6] |
Y. Liang, X. Fan, Y. Yang, D. Li, T. Cui, Oblique view selection for efficient and accurate building reconstruction in rural areas using large-scale UAV images, Drones, 6 (2022), 175. https://doi.org/10.3390/drones6070175 doi: 10.3390/drones6070175
![]() |
[7] |
Z. Hu, Y. Hou, P. Tao, J. Shan, IMGTR: Image-triangle based multi-view 3D reconstruction for urban scenes, ISPRS J. Photogramm. Remote Sens., 181 (2021), 191–204. https://doi.org/10.1016/j.isprsjprs.2021.09.009 doi: 10.1016/j.isprsjprs.2021.09.009
![]() |
[8] |
J. Pan, L. Li, H. Yamaguchi, K. Hasegawa, F. I. Thufail, Brahmantara, et al., 3D reconstruction of Borobudur reliefs from 2D monocular photographs based on soft-edge enhanced deep learning, ISPRS J. Photogramm. Remote Sens., 183 (2022), 439–450. https://doi.org/10.1016/j.isprsjprs.2021.11.007 doi: 10.1016/j.isprsjprs.2021.11.007
![]() |
[9] |
J. Zhang, L. Zhao, K. Yu, G. Min, A. Y. Al-Dubai, A. Y. Zomaya, A novel federated learning scheme for generative adversarial networks, IEEE Trans. Mob. Comput., 2023 (2023), 1–17. https://doi.org/10.1109/TMC.2023.3278668 doi: 10.1109/TMC.2023.3278668
![]() |
[10] |
Q. Hu, B. Yang, L. Xie, S. Rosa, Y. Guo, Z. Wang, et al., Learning semantic segmentation of large-scale point clouds with random sampling, IEEE Trans. Pattern Anal. Mach. Intell., 44 (2021), 8338–8354. https://doi.org/10.1109/TPAMI.2021.3083288 doi: 10.1109/TPAMI.2021.3083288
![]() |
[11] |
Z. Guo, K. Yu, Z. Lv, K. K. R. Choo, P. Shi, J. J. P. C. Rodrigues, Deep federated learning enhanced secure POI microservices for cyber-physical systems, IEEE Wireless Commun., 29 (2022), 22–29. http://doi.org/10.1109/MWC.002.2100272 doi: 10.1109/MWC.002.2100272
![]() |
[12] |
L. Bai, Y. Li, M. Cen, F. Hu, 3D instance segmentation and object detection framework based on the fusion of Lidar remote sensing and optical image sensing, Remote Sens., 13 (2021), 3288. https://doi.org/10.3390/rs13163288 doi: 10.3390/rs13163288
![]() |
[13] |
J. Yang, L. Jia, Z. Guo, Y. Shen, X. Li, Z. Mou, et al., Prediction and control of water quality in Recirculating Aquaculture System based on hybrid neural network, Eng. Appl. Artif. Intell., 121 (2023), 106002. https://doi.org/10.1016/j.engappai.2023.106002 doi: 10.1016/j.engappai.2023.106002
![]() |
[14] |
J. Huang, F. Yang, C. Chakraborty, Z. Guo, H. Zhang, L. Zhen, et al., Opportunistic capacity based resource allocation for 6G wireless systems with network slicing, Future Gener. Comput. Syst., 140 (2023), 390–401. https://doi.org/10.1016/j.future.2022.10.032 doi: 10.1016/j.future.2022.10.032
![]() |
[15] |
B. Gecer, S. Ploumpis, I. Kotsia, S. Zafeiriou, Fast-GANFIT: Generative adversarial network for high fidelity 3D face reconstruction, IEEE Trans. Pattern Anal. Mach. Intell., 44 (2021), 4879–4893. https://doi.org/10.1109/TPAMI.2021.3084524 doi: 10.1109/TPAMI.2021.3084524
![]() |
[16] |
G. Hou, W. Zhang, B. Wu, R. He, 3D reconstruction and positioning of surface features based on a monocular camera and geometric constraints, Appl. Opt., 61 (2022), C27–C36. https://doi.org/10.1364/AO.436234 doi: 10.1364/AO.436234
![]() |
[17] |
X. Zhu, F. Ma, F. Ding, Z. Guo, J. Yang, K. Yu, A low-latency edge computation offloading scheme for trust evaluation in finance-level artificial intelligence of things, IEEE Internet Things J., 2023. https://doi.org/10.1109/JIOT.2023.3297834 doi: 10.1109/JIOT.2023.3297834
![]() |
[18] |
Z. Guo, Q. Zhang, F. Ding, X. Zhu, K. Yu, A novel fake news detection model for context of mixed languages through multiscale transformer, IEEE Trans. Comput. Social Syst., 2023 (2023), 1–11. https://doi.org/10.1109/TCSS.2023.3298480 doi: 10.1109/TCSS.2023.3298480
![]() |
[19] |
J. Yang, Z. Guo, J. Luo, Y. Shen, K. Yu, Cloud-edge-end collaborative caching based on graph learning for cyber-physical virtual reality, IEEE Syst. J., 2023 (2023), 1–12. https://doi/org/10.1109/JSYST.2023.3262255 doi: 10.1109/JSYST.2023.3262255
![]() |
[20] |
Z. Shen, F. Ding, Y. Yao, A. Bhardwaj, Z. Guo, K. Yu, A privacy-preserving social computing framework for health management using federated learning, IEEE Trans. Comput. Social Syst., 10 (2023), 1666–1678. https://doi.org/10.1109/TCSS.2022.3222682 doi: 10.1109/TCSS.2022.3222682
![]() |
[21] |
Z. Zheng, T. Yu, Y. Liu, Q. Dai, Pamir: Parametric model-conditioned implicit representation for image-based human reconstruction, IEEE Trans. Pattern Anal. Mach. Intell., 44 (2022), 3170–3184. https://doi.org/10.1109/TPAMI.2021.3050505 doi: 10.1109/TPAMI.2021.3050505
![]() |
[22] |
D. Meng, Y. Xiao, Z. Guo, A. Jolfaei, L. Qin, X. Lu, et al., A data-driven intelligent planning model for UAVs routing networks in mobile Internet of Things, Comput. Commun., 179 (2021), 231–241. https://doi.org/10.1016/j.comcom.2021.08.014 doi: 10.1016/j.comcom.2021.08.014
![]() |
[23] |
Q. Zhang, Z. Guo, Y. Zhu, P. Vijayakumar, A. Castiglione, B. B. Gupta, A deep learning-based fast fake news detection model for cyber-physical social services, Pattern Recognit. Lett., 168 (2023), 31–38. https://doi.org/10.1016/j.patrec.2023.02.026 doi: 10.1016/j.patrec.2023.02.026
![]() |
[24] |
J. Chen, W. Wang, K. Yu, X. Hu, M. Cai, M. Guizani, Node connection strength matrix-based graph convolution network for traffic flow prediction, IEEE Trans. Veh. Technol., 72 (2023), 12063–12074. https://doi.org/10.1109/TVT.2023.3265300 doi: 10.1109/TVT.2023.3265300
![]() |
[25] |
X. Yuan, H. Tian, Z. Zhang, Z. Zhao, L. Liu, A. K. Sangaiah, et al., A MEC offloading strategy based on improved DQN and simulated annealing for internet of behavior, ACM Trans. Sens. Netw., 19 (2023), 1–20. https://doi.org/10.1145/3532093 doi: 10.1145/3532093
![]() |
[26] |
S. Han, L. Huo, Y. Wang, J. Zhou, H. Li, Rapid reconstruction of 3D structural model based on interactive graph cuts, Buildings, 12 (2022), 22. https://doi.org/10.3390/buildings12010022 doi: 10.3390/buildings12010022
![]() |
[27] |
L. Yang, F. Zhang, F. Yang, P. Qian, Q. Wang, Y. Wu, et al., Generating topologically consistent BIM models of utility tunnels from point clouds, Sensors, 23 (2023), 6503. https://doi.org/10.3390/s23146503 doi: 10.3390/s23146503
![]() |
[28] |
Y. Yin, G. Liu, S. Li, Z. Zheng, Y. Si, Y. Wang, A method for predicting canopy light distribution in cherry trees based on fused point cloud data, Remote Sens., 15 (2023), 2516. https://doi.org/10.3390/rs15102516 doi: 10.3390/rs15102516
![]() |
[29] |
Y. Peng, S. Lin, H. Wu, G. Cao, Point cloud registration based on fast point feature histogram descriptors for 3D reconstruction of trees, Remote Sens., 15 (2023), 3775. https://doi.org/10.3390/rs15153775 doi: 10.3390/rs15153775
![]() |
[30] |
A. Vong, J. P. Matos-Carvalho, P. Toffanin, D. Pedro, F. Azevedo, F. Moutinho, et al., How to build a 2D and 3D aerial multispectral map? –– all steps deeply explained, Remote Sens., 13 (2021), 3227. https://doi.org/10.3390/rs13163227 doi: 10.3390/rs13163227
![]() |
1. | Marius‐F. Danca, Controlling the dynamics of a COVID‐19 mathematical model using a parameter switching algorithm, 2023, 0170-4214, 10.1002/mma.9014 | |
2. | Mohammad Masum, M.A. Masud, Muhaiminul Islam Adnan, Hossain Shahriar, Sangil Kim, Comparative study of a mathematical epidemic model, statistical modeling, and deep learning for COVID-19 forecasting and management, 2022, 80, 00380121, 101249, 10.1016/j.seps.2022.101249 | |
3. | Javaid Ali, Ali Raza, Nauman Ahmed, Ali Ahmadian, Muhammad Rafiq, Massimiliano Ferrara, Evolutionary optimized Padé approximation scheme for analysis of covid-19 model with crowding effect, 2021, 8, 22147160, 100207, 10.1016/j.orp.2021.100207 | |
4. | N. Raza, A. Bakar, A. Khan, C. Tunç, Numerical Simulations of the Fractional-Order SIQ Mathematical Model of Corona Virus Disease Using the Nonstandard Finite Difference Scheme, 2022, 16, 1823-8343, 391, 10.47836/mjms.16.3.01 | |
5. | Shimelis Bekele Zerefe, Tigabu Kasie Ayele, Surafel Luleseged Tilahun, Mehmet Ünver, Mathematical Modeling of COVID‐19 Disease Dynamics With Contact Tracing: A Case Study in Ethiopia, 2024, 2024, 1110-757X, 10.1155/2024/5556734 | |
6. | Anuradha Bhattacharjee, Kshama Jain, 2024, 3139, 0094-243X, 120002, 10.1063/5.0224325 |