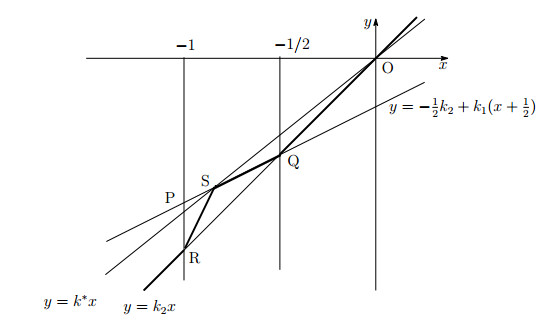
Automated organ segmentation in anatomical sectional images of canines is crucial for clinical applications and the study of sectional anatomy. The manual delineation of organ boundaries by experts is a time-consuming and laborious task. However, semi-automatic segmentation methods have shown low segmentation accuracy. Deep learning-based CNN models lack the ability to establish long-range dependencies, leading to limited segmentation performance. Although Transformer-based models excel at establishing long-range dependencies, they face a limitation in capturing local detail information. To address these challenges, we propose a novel ECA-TFUnet model for organ segmentation in anatomical sectional images of canines. ECA-TFUnet model is a U-shaped CNN-Transformer network with Efficient Channel Attention, which fully combines the strengths of the Unet network and Transformer block. Specifically, The U-Net network is excellent at capturing detailed local information. The Transformer block is equipped in the first skip connection layer of the Unet network to effectively learn the global dependencies of different regions, which improves the representation ability of the model. Additionally, the Efficient Channel Attention Block is introduced to the Unet network to focus on more important channel information, further improving the robustness of the model. Furthermore, the mixed loss strategy is incorporated to alleviate the problem of class imbalance. Experimental results showed that the ECA-TFUnet model yielded 92.63% IoU, outperforming 11 state-of-the-art methods. To comprehensively evaluate the model performance, we also conducted experiments on a public dataset, which achieved 87.93% IoU, still superior to 11 state-of-the-art methods. Finally, we explored the use of a transfer learning strategy to provide good initialization parameters for the ECA-TFUnet model. We demonstrated that the ECA-TFUnet model exhibits superior segmentation performance on anatomical sectional images of canines, which has the potential for application in medical clinical diagnosis.
Citation: Yunling Liu, Yaxiong Liu, Jingsong Li, Yaoxing Chen, Fengjuan Xu, Yifa Xu, Jing Cao, Yuntao Ma. ECA-TFUnet: A U-shaped CNN-Transformer network with efficient channel attention for organ segmentation in anatomical sectional images of canines[J]. Mathematical Biosciences and Engineering, 2023, 20(10): 18650-18669. doi: 10.3934/mbe.2023827
[1] | Zhiyuan Wang, Chu Zhang, Shaopei Xue, Yinjie Luo, Jun Chen, Wei Wang, Xingchen Yan . Dynamic coordinated strategy for parking guidance in a mixed driving parking lot involving human-driven and autonomous vehicles. Electronic Research Archive, 2024, 32(1): 523-550. doi: 10.3934/era.2024026 |
[2] | Xiaoying Zheng, Jing Wu, Xiaofeng Li, Junjie Huang . UAV search coverage under priority of important targets based on multi-location domain decomposition. Electronic Research Archive, 2024, 32(4): 2491-2513. doi: 10.3934/era.2024115 |
[3] | Yu Shen, Hecheng Li . A multi-strategy genetic algorithm for solving multi-point dynamic aggregation problems with priority relationships of tasks. Electronic Research Archive, 2024, 32(1): 445-472. doi: 10.3934/era.2024022 |
[4] | Sida Lin, Lixia Meng, Jinlong Yuan, Changzhi Wu, An Li, Chongyang Liu, Jun Xie . Sequential adaptive switching time optimization technique for maximum hands-off control problems. Electronic Research Archive, 2024, 32(4): 2229-2250. doi: 10.3934/era.2024101 |
[5] | Ismail Ben Abdallah, Yassine Bouteraa, Saleh Mobayen, Omar Kahouli, Ali Aloui, Mouldi Ben Amara, Maher JEBALI . Fuzzy logic-based vehicle safety estimation using V2V communications and on-board embedded ROS-based architecture for safe traffic management system in hail city. Electronic Research Archive, 2023, 31(8): 5083-5103. doi: 10.3934/era.2023260 |
[6] | Jian Gong, Yuan Zhao, Jinde Cao, Wei Huang . Platoon-based collision-free control for connected and automated vehicles at non-signalized intersections. Electronic Research Archive, 2023, 31(4): 2149-2174. doi: 10.3934/era.2023111 |
[7] | Hao Li, Zhengwu Wang, Shuiwang Chen, Weiyao Xu, Lu Hu, Shuai Huang . Integrated optimization of planning and operation of a shared automated electric vehicle system considering the trip selection and opportunity cost. Electronic Research Archive, 2024, 32(1): 41-71. doi: 10.3934/era.2024003 |
[8] | Wenjie Wang, Suzhen Wen, Shen Gao, Pengyi Lin . A multi-objective dynamic vehicle routing optimization for fresh product distribution: A case study of Shenzhen. Electronic Research Archive, 2024, 32(4): 2897-2920. doi: 10.3934/era.2024132 |
[9] | Yineng Ouyang, Zhaotao Liang, Zhihui Ma, Lei Wang, Zhaohua Gong, Jun Xie, Kuikui Gao . A class of constrained optimal control problems arising in an immunotherapy cancer remission process. Electronic Research Archive, 2024, 32(10): 5868-5888. doi: 10.3934/era.2024271 |
[10] | Michael Barg, Amanda Mangum . Statistical analysis of numerical solutions to constrained phase separation problems. Electronic Research Archive, 2023, 31(1): 229-250. doi: 10.3934/era.2023012 |
Automated organ segmentation in anatomical sectional images of canines is crucial for clinical applications and the study of sectional anatomy. The manual delineation of organ boundaries by experts is a time-consuming and laborious task. However, semi-automatic segmentation methods have shown low segmentation accuracy. Deep learning-based CNN models lack the ability to establish long-range dependencies, leading to limited segmentation performance. Although Transformer-based models excel at establishing long-range dependencies, they face a limitation in capturing local detail information. To address these challenges, we propose a novel ECA-TFUnet model for organ segmentation in anatomical sectional images of canines. ECA-TFUnet model is a U-shaped CNN-Transformer network with Efficient Channel Attention, which fully combines the strengths of the Unet network and Transformer block. Specifically, The U-Net network is excellent at capturing detailed local information. The Transformer block is equipped in the first skip connection layer of the Unet network to effectively learn the global dependencies of different regions, which improves the representation ability of the model. Additionally, the Efficient Channel Attention Block is introduced to the Unet network to focus on more important channel information, further improving the robustness of the model. Furthermore, the mixed loss strategy is incorporated to alleviate the problem of class imbalance. Experimental results showed that the ECA-TFUnet model yielded 92.63% IoU, outperforming 11 state-of-the-art methods. To comprehensively evaluate the model performance, we also conducted experiments on a public dataset, which achieved 87.93% IoU, still superior to 11 state-of-the-art methods. Finally, we explored the use of a transfer learning strategy to provide good initialization parameters for the ECA-TFUnet model. We demonstrated that the ECA-TFUnet model exhibits superior segmentation performance on anatomical sectional images of canines, which has the potential for application in medical clinical diagnosis.
We consider the system of Hamilton-Jacobi equations
{λu1(x)+H1(Du1(x))+B1(u1(x),u2(x))=0 in Tn,λu2(x)+H2(Du2(x))+B2(u1(x),u2(x))=0 in Tn, | (1.1) |
where λ>0 is a given constant, the functions Hi:Rn→R and Bi:R2→R, with i=1,2, are given continuous functions, and Tn denotes the n-dimensional flat torus Rn/Zn.
In a recent paper [6], the authors have investigated the vanishing discount problem for a nonlinear monotone system of Hamilton-Jacobi equations
{λu1(x)+G1(x,Du1(x),u1(x),u2(x),…,um(x))=0 in Tn,λu1(x)+G1(x,Du1(x),u1(x),u2(x)⋮λum(x)+Gm(x,Dum(x),u1(x),u2(x),…,um(x))=0 in Tn, | (1.2) |
and established under some hypotheses on the Gi∈C(Tn×Rn×Rm) that, when uλ=(uλ,1,…,uλ,m)∈C(Tn)m denoting the (viscosity) solution of (1.2), the whole family {uλ}λ>0 converges in C(Tn)m to some u0∈C(Tn)m as λ→0+. The constant λ>0 in the above system is the so-called discount factor.
The hypotheses on the system are the convexity, coercivity, and monotonicity of the Gi as well as the solvability of (1.2), with λ=0. Here the convexity of Gi is meant that the functions Rn×Rm∋(p,u)↦Gi(x,p,u) are convex. We refer to [6] for the precise statement of the hypotheses.
Prior to work [6], there have been many contributions to the question about the whole family convergence (in other words, the full convergence) under the vanishing discount, which we refer to [1,3,4,6,8,9,10] and the references therein.
In the case of the scalar equation, B. Ziliotto [11] has recently shown an example of the Hamilton-Jacobi equation having non-convex Hamiltonian in the gradient variable for which the full convergence does not hold. In Ziliotto's approach, the first step is to find a system of two algebraic equations
{λu+f(u−v)=0,λv+g(v−u)=0, | (1.3) |
with two unknowns u,v∈R and with a parameter λ>0 as the discount factor, for which the solutions (uλ,vλ) stay bounded and fail to fully converge as λ→0+. Here, an "algebraic" equation is meant not to be a functional equation. The second step is to interpolate the two values uλ and vλ to get a function of x∈T1 which satisfies a scalar non-convex Hamilton-Jacobi equation in T1.
In the first step above, Ziliotto constructs f,g based on a game-theoretical and computational argument, and the formula for f,g is of the minimax type and not quite explicit. In [5], the author has reexamined the system given by Ziliotto, with a slight generality, as a counterexample for the full convergence in the vanishing discount.
Our purpose in this paper is to present a system (1.3), with an explicit formula for f,g, for which the solution (uλ,vλ) does not fully converge to a single point in R2. A straightforward consequence is that (1.1), with B1(u1,u2)=f(u1−u2) and B2(u1,u2)=g(u2−u1), has a solution given by
(uλ,1(x),uλ.2(x))=(uλ,vλ) for x∈Tn, |
under the assumption that Hi(x,0)=0 for all x∈Tn, and therefore, gives an example of a discounted system of Hamilton-Jacobi equations, the solution of which fails to satisfy the full convergence as the discount factor goes to zero.
The paper consists of two sections. This introduction is followed by Section 2, the final section, which is divided into three subsections. The main results are stated in the first subsection of Section 2, the functions f,g, the key elements of (1.3), are contstructed in the second subsection, and the final subsection provides the proof of the main results.
Our main focus is now the system
{λu+f(u−v)=0λv+g(v−u)=0, | (2.1) |
where f,g∈C(R,R) are nondecreasing functions, to be constructed, and λ>0 is a constant, to be sent to zero. Notice that (2.1) above is referred as (1.3) in the previous section.
We remark that, due to the monotonicity assumption on f,g, the mapping (u,v)↦(f(u−v),g(v−u)),R2→R2 is monotone. Recall that, by definition, a mapping (u,v)↦(B1(u,v),B2(u,v)),R2→R2 is monotone if, whenever (u1,v1),(u2,v2)∈R2 satisfy u1−u2≥v1−v2 (resp., v1−v2≥u1−u2), we have B1(u1,v1)≥B1(u2,v2) (resp., B2(u1,v1)≥B2(u2,v2)).
Our main results are stated as follows.
Theorem 1. There exist two increasing functions f,g∈C(R,R) having the properties (a)–(c):
(a) For any λ>0 there exists a unique solution (uλ,vλ)∈R2 to (2.1),
(b) the family of the solutions (uλ,vλ) to (2.1), with λ>0, is bounded in R2,
(c) the family {(uλ,vλ)}λ>0 does not converge as λ→0+.
It should be noted that, as mentioned in the introduction, the above theorem has been somewhat implicitly established by Ziliotto [11]. In this note, we are interested in a simple and easy approach to finding functions f,g having the properties (a)–(c) in Theorem 1.
The following is an immediate consequence of the above theorem.
Corollary 2. Let Hi∈C(Rn,R), i=1,2, satisfy H1(0)=H2(0)=0. Let f,g∈C(R,R) be the functions given by Theorem 1, and set B1(u1,u2)=f(u1−u2) and B2(u1,u2)=g(u2−u1) for all (u1,u2)∈R2. For any λ>0, let (uλ,1,uλ,2) be the (viscosity) solution of (1.1). Then, the functions uλ,i are constants, the family of the points (uλ,1,uλ,2) in R2 is bounded, and it does not converge as λ→0+.
Notice that the convexity of Hi in the above corollary is irrelevant, and, for example, one may take Hi(p)=|p|2 for i∈I, which are convex functions.
We remark that a claim similar to Corollary 2 is valid when one replaces Hi(p) by degenerate elliptic operators Fi(x,p,M) as far as Fi(x,0,0)=0, where M is the variable corresponding to the Hessian matrices of unknown functions. (See [2] for an overview on the viscosity solution approach to fully nonlinear degenerate elliptic equations.)
If f,g are given and (u,v)∈R2 is a solution of (2.1), then w:=u−v satisfies
λw+f(w)−g(−w)=0. | (2.2) |
Set
h(r)=f(r)−g(−r) for r∈R, | (2.3) |
which defines a continuous and nondecreasing function on R.
To build a triple of functions f,g,h, we need to find two of them in view of the relation (2.3). We begin by defining function h.
For this, we discuss a simple geometry on xy-plane as depicted in Figure 1 below. Fix 0<k1<k2. The line y=−12k2+k1(x+12) has slope k1 and crosses the lines x=−1 and y=k2x at P:=(−1,−12(k1+k2)) and Q:=(−12,−12k2), respectively, while the line y=k2x meets the lines x=−1 and x=−12 at R:=(−1,−k2) and Q=(−12,−12k2), respectively.
Choose k∗>0 so that 12(k1+k2)<k∗<k2. The line y=k∗x crosses the line y=−12k2+k1(x+12) at a point S:=(x∗,y∗) in the open line segment between the points P=(−12,−12(k1+k2)) and Q=(−12,−12k2). The line connecting R=(−1,−k2) and S=(x∗,y∗) can be represented by y=−k2+k+(x+1), with k+:=y∗+k2x∗+1>k2.
We set
ψ(x)={k2x for x∈(−∞,−1]∪[−1/2,∞),min{−k2+k+(x+1),−12k2+k1(x+12)} for x∈(−1,−12). |
It is clear that ψ∈C(R) and increasing on R. The building blocks of the graph y=ψ(x) are three lines whose slopes are k1<k2<k+. Hence, if x1>x2, then ψ(x1)−ψ(x2)≥k1(x1−x2), that is, the function x↦ψ(x)−k1x is nondecreasing on R.
Next, we set for j∈N,
ψj(x)=2−jψ(2jx) for x∈R. |
It is clear that for all j∈N, ψj∈C(R), the function x↦ψj(x)−k1x is nondecreasing on R, and
ψj(x){>k2x for all x∈(−2−j,−2−j−1),=k2x otherwise. |
We set
η(x)=maxj∈Nψj(x) for x∈R. |
It is clear that η∈C(R) and x↦η(x)−k1x is nondecreasing on R. Moreover, we see that
η(x)=k2x for all x∈(−∞,−12]∪[0,∞), |
and that if −2−j<x<−2−j−1 and j∈N,
η(x)=ψj(x)>k2x. |
Note that the point S=(x∗,y∗) is on the graph y=ψ(x) and, hence, that for any j∈N, the point (2−jx∗,2−jy∗) is on the graph y=η(x). Similarly, since the point S=(x∗,y∗) is on the graph y=k∗x and for any j∈N, the point (2−jx∗,2−jy∗) is on the graph y=k∗x. Also, for any j∈N, the point (−2−j,−k22−j) lies on the graphs y=η(x) and y=k2x.
Fix any d≥1 and define h∈C(R) by
h(x)=η(x−d). |
For the function h defined above, we consider the problem
λz+h(z)=0. | (2.4) |
Lemma 3. For any λ≥0, there exists a unique solution zλ∈R of (2.4).
Proof. Fix λ≥0. The function x↦h(x)+λx is increasing on R and satisfies
limx→∞(h(x)+λx)=∞ and limx→−∞(h(x)+λx)=−∞. |
Hence, there is a unique solution of (2.4).
For any λ≥0, we denote by zλ the unique solution of (2.4). Since h(d)=0, it is clear that z0=d.
For later use, observe that if λ>0, k>0, and (z,w)∈R2 is the point of the intersection of two lines y=−λx and y=k(x−d), then w=−λz=k(z−d) and
z=kdk+λ. | (2.5) |
Lemma 4. There are sequences {μj} and {νj} of positive numbers converging to zero such that
zμj=k2dk2+μj and zνj=k∗dk∗+νj. |
Proof. Let j∈N. Since (−2−j,−k22−j) is on the intersection of the graphs y=k2x and y=η(x), it follows that (−2−j+d,−k22−j) is on the intersection of the graphs y=k2(x−d) and y=h(x). Set
μj=k22−jd−2−j, | (2.6) |
and note that μj>0 and that
−μj(d−2−j)=−k22−j, |
which says that the point (d−2−j,−k22−j) is on the line y=−μjx. Combining the above with
−k22−j=h(d−2−j) |
shows that d−2−j is the unique solution of (2.4). Also, since (d−2−j,−μj(d−2−j))=(d−2−j,−k22−j) is on the line y=k2(x−d), we find by (2.5) that
zμj=k2dk2+μj. |
Similarly, since (2−jx∗,2−jy∗) is on the intersection of the graphs y=k∗x and y=η(x), we deduce that if we set
νj:=−2−jy∗d+2−jx∗=2−j|y∗|d−2−j|x∗|, | (2.7) |
then
zνj=k∗dk∗+νj. |
It is obvious by (2.6) and (2.7) that the sequences {μj}j∈N and {νj}j∈N are decreasing and converge to zero.
We fix k0∈(0,k1) and define f,g∈C(R) by f(x)=k0(x−d) and
g(x)=f(−x)−h(−x). |
It is easily checked that g(x)−(k1−k0)x is nondecreasing on R, which implies that g is increasing on R, and that h(x)=f(x)−g(−x) for all x∈R. We note that
f(d)=h(d)=g(−d)=0. | (2.8) |
We fix f,g,h as above, and consider the system (2.1).
Lemma 5. Let λ>0. There exists a unique solution of (2.1).
The validity of the above lemma is well-known, but for the reader's convenience, we provide a proof of the lemma above.
Proof. By choice of f,g, the functions f,g are nondecreasing on R. We show first the comparison claim: if (u1,v1),(u2,v2)∈R2 satisfy
λu1+f(u1−v1)≤0,λv1+g(v1−u1)≤0, | (2.9) |
λu2+f(u2−v2)≥0,λv2+g(v2−u2)≥0, | (2.10) |
then u1≤u2 and v1≤v2. Indeed, contrary to this, we suppose that max{u1−u2,v1−v2}>0. For instance, if max{u1−u2,v1−v2}=u1−u2, then we have u1−v1≥u2−v2 and u1>u2, and moreover
0≥λu1+f(u1−v1)≥λu1+f(u2−v2)>λu2+f(u2−v2), |
yielding a contradiction. The other case when max{u1−u2,v1−v2}=v1−v2, we find a contradiction, 0>λv2+g(v2−u2), proving the comparison.
From the comparison claim, the uniqueness of the solutions of (2.1) follows readily.
Next, we may choose a constant C>0 so large that (u1,v1)=(−C,−C) and (u2,v2)=(C,C) satisfy (2.9) and (2.10), respectively. We write S for the set of all (u1,u2)∈R2 such that (2.9) hold. Note that (−C,−C)∈S and that for any (u,v)∈S, u≤C and v≤C. We set
u∗=sup{u:(u,v)∈S for some v},v∗=sup{v:(u,v)∈S for some u}. |
It follows that −C≤u∗,v∗≤C. We can choose sequences
{(u1n,v1n)}n∈N,{(u2n,v2n)}n∈N⊂S |
such that {u1n},{v2n} are nondecreasing,
limn→∞u1n=u∗ and limn→∞v2n=v∗. |
Observe that for all n∈N, u2n≤u∗, v1n≤v∗, and
0≥λu1n+f(u1n−v1n)≥λu1n+f(u1n−v∗), |
which yields, in the limit as n→∞,
0≥λu∗+f(u∗−v∗). |
Similarly, we obtain 0≥λv∗+g(v∗−u∗). Hence, we find that (u∗,v∗)∈S.
We claim that (u∗,v∗) is a solution of (2.1). Otherwise, we have
0>λu∗+f(u∗−v∗) or 0>λv∗+g(v∗−u∗). |
For instance, if the former of the above inequalities holds, we can choose ε>0, by the continuity of f, so that
0>λ(u∗+ε)+f(u∗+ε−v∗). |
Since (u∗,v∗)∈S, we have
0≥λv∗+g(v∗−u∗)≥λv∗+g(v∗−u∗−ε). |
Accordingly, we find that (u∗+ε,v∗)∈S, which contradicts the definition of u∗. Similarly, if 0>λv∗+g(v∗−u∗), then we can choose δ>0 so that (u∗,v∗+δ)∈S, which is a contradiction. Thus, we conclude that (u∗,v∗) is a solution of (2.1).
Theorem 6. For any λ>0, let (uλ,vλ) denote the unique solution of (2.1). Let {μj},{νj} be the sequences of positive numbers from Lemma 2.4. Then
limj→∞uμj=k0dk2 and limj→∞uνj=k0dk∗. |
In particular,
lim infλ→0uλ≤k0dk2<k0dk∗≤lim supλ→0uλ. |
With our choice of f,g, the family of solutions (uλ,vλ) of (2.1), with λ>0, does not converge as λ→0.
Proof. If we set zλ=uλ−vλ, then zλ satisfies (2.4). By Lemma 4, we find that
zμj=k2dk2+μj and zνj=k∗dk∗+νj. |
Since uλ satisfies
0=λuλ+f(zλ)=λuλ+k0(zλ−d), |
we find that
uμj=−k0(zμj−d)μj=−k0dμj(k2k2+μj−1)=−k0dμj−μjk2+μj=k0dk2+μj, |
which shows that
limj→∞uμj=k0dk2. |
A parallel computation shows that
limj→∞uνj=k0dk∗. |
Recalling that 0<k∗<k2, we conclude that
lim infλ→0uλ≤k0dk2<k0dk∗≤lim supλ→0uλ. |
We remark that, since
limλ→0zλ=d and vλ=uλ−zλ, |
limj→∞vμj=k0dk2−d and limj→∞vνj=k0dk∗−d. |
We give the proof of Theorem 1.
Proof of Theorem 1. Assertions (a) and (c) are consequences of Lemma 5 and Theorem 6, respectively.
Recall (2.8). That is, we have f(d)=h(d)=g(−d)=0. Setting (u2,v2)=(d,0), we compute that for any λ>0,
λu2+f(u2−v2)>f(d)=0 and λv2+g(v2−u2)=g(−d)=0. |
By the comparison claim, proved in the proof of Lemma 5, we find that uλ≤d and vλ≤0 for any λ>0. Similarly, setting (u1,v1)=(0,−d), we find that for any λ>0,
λu1+f(u1−v1)=f(d)=0 and λv1+g(v1−u1)≤g(v1−u1)=g(−d)=0, |
which shows by the comparison claim that uλ≥0 and vλ≥−d for any λ>0. Thus, the sequence {(uλ,vλ)}λ>0 is bounded in R2, which proves assertion (b).
Proof of Corollary 2. For any λ>0, let (uλ,vλ)∈R2 be the unique solution of (2.1). Since H1(0)=H2(0)=0, it is clear that the constant function (uλ,1(x),uλ,2(x)):=(uλ,vλ) is a classical solution of (1.1). By a classical uniqueness result (see, for instance, [7,Theorem 4.7]), (uλ,1,uλ,2) is a unique viscosity solution of (1.1). The rest of the claims in Corollary 2 is an immediate consequence of Theorem 1.
Some remarks are in order. (ⅰ) Following [11], we may use Theorem 6 as the primary cornerstone for building a scalar Hamilton-Jacobi equation, for which the vanishing discount problem fails to have the full convergence as the discount factor goes to zero.
(ⅱ) In the construction of the functions f,g∈C(R,R) in Theorem 6, the author has chosen d to satisfy d≥1, but, in fact, one may choose any d>0. In the proof, the core step is to find the function h(x)=f(x)−g(−x), with the properties: (a) the function x↦h(x)−εx is nondecreasing on R for some ε>0 and (b) the curve y=h(x), with x<d, meets the lines y=p(x−d) and y=q(x−d), respectively, at Pj and Qj for all j∈N, where p,q,d are positive constants such that ε<p<q, and the sequences {Pj}j∈N,{Qj}j∈N converge to the point (d,0). Obviously, such a function h is never left-differentiable at x=d nor convex in any neighborhood of x=d. Because of this, it seems difficult to select f,g∈C(R,R) in Theorem 1, both smooth everywhere. In the proof of Theorem 6, we have chosen ε=k0, p=k∗, q=k2, Pj=(uνj,k∗(uνj−d)), and Qj=(uμj,k2(uμj−d))
Another possible choice of h among many other ways is the following. Define first η:R→R by η(x)=x(sin(log|x|)+2) if x≠0, and η(0)=0 (see Figure 2). Fix d>0 and set h(x)=η(x−d) for x∈R. we remark that η∈C∞(R∖{0}) and h∈C∞(R∖{d}). Note that if x≠0,
η′(x)=sin(log|x|)+cos(log|x|)+2∈[2−√2,2+√2], |
and that if we set xj=−exp(−2πj) and ξj=−exp(−2πj+π2), j∈N, then
η(xj)=2xj and η(ξj)=3ξj. |
The points Pj:=(xj+d,2xj) are on the intersection of two curves y=h(x) and y=2(x−d), while the points Qj:=(d+ξj,3ξj) are on the intersection of y=h(x) and y=3(x−d). Moreover, limPj=limQj=(d,0).
The author would like to thank the anonymous referees for their careful reading and useful suggestions. He was supported in part by the JSPS Grants KAKENHI No. 16H03948, No. 20K03688, No. 20H01817, and No. 21H00717.
The author declares no conflict of interest.
[1] |
K. Karasawa, M. Oda, T. Kitasaka, K. Misawa, M. Fujiwara, C. W. Chu, et al., Multi-atlas pancreas segmentation: Atlas selection based on vessel structure, Med. Image Anal., 39 (2017), 18–28. https://doi.org/10.1016/j.media.2017.03.006 doi: 10.1016/j.media.2017.03.006
![]() |
[2] |
P. F. Li, P. Liu, C. L. Chen, H. Duan, W. J. Qiao, O. H. Ognami, The 3D reconstructions of female pelvic autonomic nerves and their related organs based on MRI: a first step towards neuronavigation during nerve-sparing radical hysterectomy, Eur. Radiol., 28 (2018), 4561–4569. https://doi.org/10.1007/s00330-018-5453-8 doi: 10.1007/s00330-018-5453-8
![]() |
[3] |
H. S. Park, D. S. Shin, D. H. Cho, Y. W. Jung, J. S. Park, Improved sectioned images and surface models of the whole dog body, Ann. Anat., 196 (2014), 352–359. https://doi.org/10.1016/j.aanat.2014.05.036 doi: 10.1016/j.aanat.2014.05.036
![]() |
[4] |
J. S. Park, Y. W. Jung, Software for browsing sectioned images of a dog body and generating a 3D model, Anat. Rec., 299 (2016), 81–87. https://doi.org/10.1002/ar.23200 doi: 10.1002/ar.23200
![]() |
[5] |
K. Czeibert, G. Baksa, A. Grimm, S. A. Nagy, E. Kubinyi, Ö. Petneházy, MRI, CT and high resolution macro-anatomical images with cryosectioning of a Beagle brain: creating the base of a multimodal imaging atlas, PLoS One, 14 (2019), e0213458. https://doi.org/10.1371/journal.pone.0213458 doi: 10.1371/journal.pone.0213458
![]() |
[6] |
X. Shu, Y. Y. Yang, B. Y. Wu, A neighbor level set framework minimized with the split Bregman method for medical image segmentation, Signal Process., 189 (2021), 108293. https://doi.org/10.1016/j.sigpro.2021.108293 doi: 10.1016/j.sigpro.2021.108293
![]() |
[7] |
X. Shu, Y. Y. Yang, J. Liu, X. J. Chang, B. Y. Wu, ALVLS: Adaptive local variances-Based levelset framework for medical images segmentation, Pattern Recogn., 136 (2023), 109257. https://doi.org/10.1016/j.patcog.2022.109257 doi: 10.1016/j.patcog.2022.109257
![]() |
[8] |
S. K. Zhou, H. Greenspan, C. Davatzikos, J. S. Duncan, B. Van Ginneken, A. Madabhushi, et al., A review of deep learning in medical imaging: Imaging traits, technology trends, case studies with progress highlights, and future promises, Proc. IEEE, 109 (2021), 820–838. https://doi.org/10.1109/JPROC.2021.3054390 doi: 10.1109/JPROC.2021.3054390
![]() |
[9] | A. Majumdar, L. Brattain, B. Telfer, C. Farris, J. Scalera, Detecting intracranial hemorrhage with deep learning, in 2018 40th Annual International Conference of the IEEE Engineering in Medicine and Biology Society (EMBC), IEEE, (2018), 583–587. https://doi.org/10.1109/EMBC.2018.8512336 |
[10] | J. Long, E. Shelhamer, T. Darrell, Fully convolutional networks for semantic segmentation, in Proceedings of the IEEE Conference on Computer Vision and Pattern Recognition, (2015), 3431–3440. https://doi.org/10.1109/CVPR.2015.7298965 |
[11] | G. Huang, Z. Liu, L. Van Der Maaten, K. Q. Weinberger, Densely connected convolutional networks, in Proceedings of the IEEE Conference on Computer Vision and Pattern Recognition, (2017), 4700–4708. https://doi.org/10.1109/CVPR.2017.243 |
[12] | L. C. Chen, Y. Zhu, G. Papandreou, F. Schroff, H. Adam, Encoder-decoder with atrous separable convolution for semantic image segmentation, in Proceedings of the European Conference on Computer Vision (ECCV), (2018), 801–818. |
[13] | O. Ronneberger, P. Fischer, T. Brox, U-net: Convolutional networks for biomedical image segmentation, in International Conference on Medical Image Computing and Computer-assisted Intervention, Springer, (2015), 234–241. https://doi.org/10.1007/978-3-319-24574-4_28 |
[14] |
D. Schmid, V. B. Scholz, P. R. Kircher, I. E. Lautenschlaeger, Employing deep convolutional neural networks for segmenting the medial retropharyngeal lymph nodes in CT studies of dogs, Vet. Radiol. Ultrasound, 63 (2022), 763–770. https://doi.org/10.1111/vru.13132 doi: 10.1111/vru.13132
![]() |
[15] |
J. Park, B. Choi, J. Ko, J. Chun, I. Park, J. Lee, et al., Deep-learning-based automatic segmentation of head and neck organs for radiation therapy in dogs, Front. Vet. Sci., 8 (2021), 721612. https://doi.org/10.3389/fvets.2021.721612 doi: 10.3389/fvets.2021.721612
![]() |
[16] | H. Cao, Y. Wang, J. Chen, D. Jiang, X. Zhang, Q. Tian, et al., Swin-unet: Unet-like pure transformer for medical image segmentation, in European Conference on Computer Vision, (2021), 205–218. https://doi.org/10.1007/978-3-031-25066-8_9 |
[17] |
Y. Xu, X. He, G. Xu, G. Qi, K. Yu, L. Yin, et al., A medical image segmentation method based on multi-dimensional statistical features, Front. Neurosci., 16 (2022), 1009581. https://doi.org/10.3389/fnins.2022.1009581 doi: 10.3389/fnins.2022.1009581
![]() |
[18] | A. Dosovitskiy, L. Beyer, A. Kolesnikov, D. Weissenborn, X. Zhai, T. Unterthiner, et al., An image is worth 16x16 words: Transformers for image recognition at scale, preprint, arXiv: 2010.11929. |
[19] | N. Carion, F. Massa, G. Synnaeve, N. Usunier, A. Kirillov, S. Zagoruyko, End-to-end object detection with transformers, in European Conference on Computer Vision, Springer, (2020), 213–229. https://doi.org/10.1007/978-3-030-58452-8_13 |
[20] | S. Zheng, J. Lu, H. Zhao, X. Zhu, Z. Luo, Y. Wang, et al., Rethinking semantic segmentation from a sequence-to-sequence perspective with transformers, in Proceedings of the IEEE/CVF Conference on Computer Vision and Pattern Recognition, (2021), 6881–6890. https://doi.org/10.1109/CVPR46437.2021.00681 |
[21] | J. Chen, Y. Lu, Q. Yu, X. Luo, E. Adeli, Y. Wang, et al., Transunet: Transformers make strong encoders for medical image segmentation, preprint, arXiv: 2102.04306. |
[22] |
B. Li, S. Liu, F. Wu, G. Li, M. Zhong, X. Guan, RT‐Unet: An advanced network based on residual network and transformer for medical image segmentation, Int. J. Intell. Syst., 37 (2022), 8565–8582. https://doi.org/10.1002/int.22956 doi: 10.1002/int.22956
![]() |
[23] | H. Wang, P. Cao, J. Wang, O. R. Zaiane, Uctransnet: rethinking the skip connections in u-net from a channel-wise perspective with transformer, in Proceedings of the AAAI Conference on Artificial Intelligence, 36 (2022), 2441–2449. https://doi.org/10.1609/aaai.v36i3.20144 |
[24] | Q. Wang, B. Wu, P. Zhu, P. Li, W. Zuo, Q. Hu, ECA-Net: Efficient channel attention for deep convolutional neural networks, in 2020 IEEE/CVF Conference on Computer Vision and Pattern Recognition (CVPR), (2020), 11534–11542. https://doi.org/10.1109/CVPR42600.2020.01155 |
[25] |
A. E. Kavur, N. S. Gezer, M. Barış, S. Aslan, P. H. Conze, V. Groza, et al., CHAOS challenge-combined (CT-MR) healthy abdominal organ segmentation, Med. Image Anal. , 69 (2021), 101950. https://doi.org/10.1016/j.media.2020.101950 doi: 10.1016/j.media.2020.101950
![]() |
[26] | K. He, X. Zhang, S. Ren, J. Sun, Deep residual learning for image recognition, in Proceedings of the IEEE Conference on Computer Vision and Pattern Recognition, (2016), 770–778. |
[27] | A. Vaswani, N. Shazeer, N. Parmar, J. Uszkoreit, L. Jones, A. N. Gomez, et al., Attention is all you need, in Advances in Neural Information Processing Systems, 30 (2017). |
[28] | H. Zhao, J. Shi, X. Qi, X. Wang, J. Jia, Pyramid scene parsing network, in Proceedings of the IEEE Conference on Computer Vision and Pattern Recognition, (2017), 2881–2890. https://doi.org/10.1109/CVPR.2017.660 |
[29] | J. Fu, J. Liu, H. Tian, Y. Li, Y. Bao, Z. Fang, et al., Dual attention network for scene segmentation, in Proceedings of the IEEE/CVF Conference on Computer Vision and Pattern Recognition, (2019), 3146–3154. |
[30] | Y. Cao, J. Xu, S. Lin, F. Wei, H. Hu, Gcnet: Non-local networks meet squeeze-excitation networks and beyond, in Proceedings of the IEEE/CVF International Conference on Computer Vision Workshops, 2019. https://doi.org/10.1109/ICCVW.2019.00246 |
[31] | Y. Yuan, X. Chen, J. Wang, Object-contextual representations for semantic segmentation, in European Conference on Computer Vision, Springer, (2020), 173–190. https://doi.org/10.1007/978-3-030-58539-6_11 |
[32] | Z. Liu, Y. Lin, Y. Cao, H. Hu, Y. Wei, Z. Zhang, et al., Swin transformer: Hierarchical vision transformer using shifted windows, in Proceedings of the IEEE/CVF International Conference on Computer Vision, (2021), 10012–10022. https://doi.org/10.1109/ICCV48922.2021.00986 |
[33] | E. Z. Xie, W. H. Wang, Z. D. Yu, A. Anandkumar, J. M. Alvarez, P. Luo, SegFormer: Simple and efficient design for semantic segmentation with transformers, in Advances in Neural Information Processing Systems, 34 (2021), 12077–12090. |
[34] |
M. D. Alahmadi, Medical image segmentation with learning semantic and global contextual representation, Diagnostics, 12 (2022), 1548. https://doi.org/10.3390/diagnostics12071548 doi: 10.3390/diagnostics12071548
![]() |
[35] |
J. Fang, C. Yang, Y. Shi, N. Wang, Y. Zhao, External attention based TransUNet and label expansion strategy for crack detection, IEEE Trans. Intell. Transp. Syst., 23 (2022), 19054–19063. https://doi.org/10.1109/TITS.2022.3154407 doi: 10.1109/TITS.2022.3154407
![]() |
[36] | M. H. Guo, C. Z. Lu, Q. Hou, Z. Liu, M. M. Cheng, S. M. Hu, SegNeXt: Rethinking convolutional attention design for semantic segmentation, in Advances in Neural Information Processing Systems, 35 (2022), 1140–1156. |
[37] | H. Bao, L. Dong, S. Piao, F. Wei, BEiT: BERT pre-training of image transformers, preprint, arXiv: 2106.08254. |
1. | Yuandong Chen, Jinhao Pang, Yuchen Gou, Zhiming Lin, Shaofeng Zheng, Dewang Chen, Research on the A* Algorithm for Automatic Guided Vehicles in Large-Scale Maps, 2024, 14, 2076-3417, 10097, 10.3390/app142210097 |