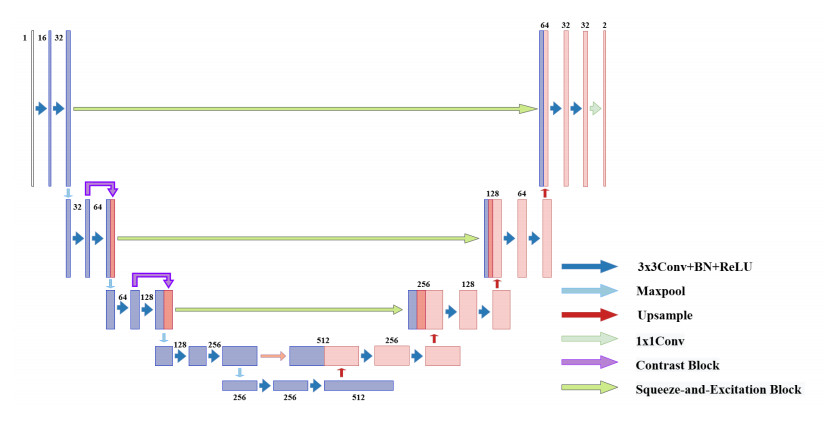
Ischemic heart disease or stroke caused by the rupture or dislodgement of a carotid plaque poses a huge risk to human health. To obtain accurate information on the carotid plaque characteristics of patients and to assist clinicians in the determination and identification of atherosclerotic areas, which is one significant foundation work. Existing work in this field has not deliberately extracted texture information of carotid from the ultrasound images. However, texture information is a very important part of carotid ultrasound images. To make full use of the texture information in carotid ultrasound images, a novel network based on U-Net called Contrast U-Net is designed in this paper. First, the proposed network mainly relies on a contrast block to extract accurate texture information. Moreover, to make the network better learn the texture information of each channel, the squeeze-and-excitation block is introduced to assist in the jump connection from encoding to decoding. Experimental results from intravascular ultrasound image datasets show that the proposed network can achieve superior performance compared with other popular models in carotid plaque detection.
Citation: WenJun Zhou, Tianfei Wang, Yuhang He, Shenghua Xie, Anguo Luo, Bo Peng, Lixue Yin. Contrast U-Net driven by sufficient texture extraction for carotid plaque detection[J]. Mathematical Biosciences and Engineering, 2023, 20(9): 15623-15640. doi: 10.3934/mbe.2023697
[1] | Ran Zhou, Yanghan Ou, Xiaoyue Fang, M. Reza Azarpazhooh, Haitao Gan, Zhiwei Ye, J. David Spence, Xiangyang Xu, Aaron Fenster . Ultrasound carotid plaque segmentation via image reconstruction-based self-supervised learning with limited training labels. Mathematical Biosciences and Engineering, 2023, 20(2): 1617-1636. doi: 10.3934/mbe.2023074 |
[2] | Jun Liu, Zhenhua Yan, Chaochao Zhou, Liren Shao, Yuanyuan Han, Yusheng Song . mfeeU-Net: A multi-scale feature extraction and enhancement U-Net for automatic liver segmentation from CT Images. Mathematical Biosciences and Engineering, 2023, 20(5): 7784-7801. doi: 10.3934/mbe.2023336 |
[3] | Dongwei Liu, Ning Sheng, Tao He, Wei Wang, Jianxia Zhang, Jianxin Zhang . SGEResU-Net for brain tumor segmentation. Mathematical Biosciences and Engineering, 2022, 19(6): 5576-5590. doi: 10.3934/mbe.2022261 |
[4] | Zhenwu Xiang, Qi Mao, Jintao Wang, Yi Tian, Yan Zhang, Wenfeng Wang . Dmbg-Net: Dilated multiresidual boundary guidance network for COVID-19 infection segmentation. Mathematical Biosciences and Engineering, 2023, 20(11): 20135-20154. doi: 10.3934/mbe.2023892 |
[5] | Yue Zhang, Haitao Gan, Furong Wang, Xinyao Cheng, Xiaoyan Wu, Jiaxuan Yan, Zhi Yang, Ran Zhou . A self-supervised fusion network for carotid plaque ultrasound image classification. Mathematical Biosciences and Engineering, 2024, 21(2): 3110-3128. doi: 10.3934/mbe.2024138 |
[6] | Jiajun Zhu, Rui Zhang, Haifei Zhang . An MRI brain tumor segmentation method based on improved U-Net. Mathematical Biosciences and Engineering, 2024, 21(1): 778-791. doi: 10.3934/mbe.2024033 |
[7] | Yuqing Zhang, Yutong Han, Jianxin Zhang . MAU-Net: Mixed attention U-Net for MRI brain tumor segmentation. Mathematical Biosciences and Engineering, 2023, 20(12): 20510-20527. doi: 10.3934/mbe.2023907 |
[8] | Huan Cheng, Jucheng Zhang, Yinglan Gong, Zhaoxia Pu, Jun Jiang, Yonghua Chu, Ling Xia . Semantic segmentation method for myocardial contrast echocardiogram based on DeepLabV3+ deep learning architecture. Mathematical Biosciences and Engineering, 2023, 20(2): 2081-2093. doi: 10.3934/mbe.2023096 |
[9] | Xiaoyan Zhang, Mengmeng He, Hongan Li . DAU-Net: A medical image segmentation network combining the Hadamard product and dual scale attention gate. Mathematical Biosciences and Engineering, 2024, 21(2): 2753-2767. doi: 10.3934/mbe.2024122 |
[10] | Yinlin Cheng, Mengnan Ma, Liangjun Zhang, ChenJin Jin, Li Ma, Yi Zhou . Retinal blood vessel segmentation based on Densely Connected U-Net. Mathematical Biosciences and Engineering, 2020, 17(4): 3088-3108. doi: 10.3934/mbe.2020175 |
Ischemic heart disease or stroke caused by the rupture or dislodgement of a carotid plaque poses a huge risk to human health. To obtain accurate information on the carotid plaque characteristics of patients and to assist clinicians in the determination and identification of atherosclerotic areas, which is one significant foundation work. Existing work in this field has not deliberately extracted texture information of carotid from the ultrasound images. However, texture information is a very important part of carotid ultrasound images. To make full use of the texture information in carotid ultrasound images, a novel network based on U-Net called Contrast U-Net is designed in this paper. First, the proposed network mainly relies on a contrast block to extract accurate texture information. Moreover, to make the network better learn the texture information of each channel, the squeeze-and-excitation block is introduced to assist in the jump connection from encoding to decoding. Experimental results from intravascular ultrasound image datasets show that the proposed network can achieve superior performance compared with other popular models in carotid plaque detection.
Atherosclerosis is the main pathogenic process in most cardiovascular diseases [1]. The carotid artery, the main artery leading to the head, gradually ages as the body ages and the constant impact of blood flow causes damage to the carotid lining. The damaged carotid artery lining will continue to produce lipid deposits and when these deposits reach a certain level atherosclerotic plaques will form. The development of atherosclerotic plaque is accompanied by fibrosis and calcium deposition, resulting in stiffening of the vessel, narrowing of the lumen and a reduction in elasticity [2,3]. The risk of stroke increases with the severity of carotid stenosis and the thickening of the carotid artery due to plaque deposition [4,5].
The importance of early detection of carotid artery plaque lies in the potential prevention of life-threatening events such as stroke. When the plaque deposits in the carotid arteries become substantial, they can narrow the arterial lumen and restrict blood flow to the brain. In some cases, pieces of the plaque can rupture and travel to smaller vessels, causing blockages and leading to an ischemic stroke. Detecting and monitoring the progression of carotid artery plaque at its early stages can provide an opportunity for medical professionals to implement preventive measures and reduce the risk of stroke and related complications. Traditionally, carotid artery plaque detection has relied on manual inspection of ultrasound images by experienced radiologists or sonographers. This approach, although effective, is time-consuming and subjective, depending on the expertise of the clinician. To address these limitations, significant advancements have been made in the field of medical imaging, particularly with the integration of deep learning techniques. Deep learning methods have revolutionized the detection and segmentation of carotid artery plaque, enabling automated and accurate identification of plaque regions from ultrasound images. Among the various deep learning architectures, U-Net has emerged as a prominent method for medical image segmentation. The U-Net architecture [6], initially proposed by Ronneberger et al., has demonstrated remarkable success in biomedical image segmentation tasks. It utilizes a U-shaped network structure consisting of an encoder pathway for feature extraction and a decoder pathway for generating segmentation masks. This design allows the network to capture both local details and global context information, leading to precise and accurate segmentation results. Since its introduction, U-Net has been extensively adopted and adapted for various medical image segmentation tasks, including carotid artery plaque detection. Researchers have explored different variations and improvements to enhance the performance of U-Net in this specific domain. Çiçek et al. extended the U-Net architecture to the three-dimension (3D) domain with their work on the 3D U-Net [7]. This variant enabled dense volumetric segmentation with sparse annotations, making it suitable for volumetric medical image analysis. This advancement facilitated more comprehensive and accurate analysis of carotid artery plaques by considering the three-dimensional nature of the data.
To address the challenges of volumetric segmentation, Milletari et al. proposed the V-Net architecture [8]. Built upon the U-Net design, V-Net specifically targeted volumetric medical image segmentation tasks. Its innovative 3D, fully convolutional approach showcased promising results in several applications, including carotid artery plaque segmentation.
Recognizing the need for improved segmentation accuracy, Zhou et al. introduced the UNet++ architecture[9]. This nested U-Net design further enhanced the segmentation performance by incorporating multi-scale context information, enabling more precise identification and localization of carotid artery plaques.
In addition to architectural enhancements, researchers have explored techniques such as self-adaptation and attention mechanisms to optimize U-Net for carotid artery plaque detection. Isensee et al. [10] developed the nnU-Net framework, which facilitated automatic configuration and customization of U-Net for specific datasets. This adaptability made it possible to achieve superior segmentation results for carotid artery plaques.
Moreover, attention mechanisms have been integrated into U-Net architectures. Oktay et al. introduced the Attention U-Net [11], which selectively focuses on important regions during segmentation tasks. This approach has demonstrated its efficacy in accurately identifying and segmenting carotid artery plaques.
The DCAN network proposed by Chen et al. [12] leveraged contour information to improve gland segmentation. While not directly applied to carotid artery plaque detection, this contour-aware network exemplifies the exploration of specialized variations of U-Net for specific segmentation tasks.
These studies represent a subset of the extensive research conducted on U-Net and its variants for medical image segmentation tasks, particularly in the context of carotid artery plaque detection. In the following sections, we will delve into these works in more detail, discussing their methodologies, results, and contributions to the field of carotid artery plaque detection using deep learning techniques. By examining the evolution and advancements of U-Net-based methods, we aim to provide a comprehensive overview of the progress made and the potential for further improvements in automated carotid artery plaque detection.
The main contributions of this work are summarized as follows:
1) We propose a novel network called Contrast U-Net, which is implemented to detect plaque in carotid ultrasound images;
2) We design an efficient contrast block module, which can extract sufficient texture information and with the help of this module the number of parameters and computational complexity caused by ultrasound image information extraction in the network are greatly reduced.
The rest contents are organized as follows. In Section 2 we present the details of the Contrast U-Net. The experimental results are presented in Section 3 and the conclusions are presented in Section 4.
In recent years, deep learning techniques have been used in various fields with the computer image processing capability continuing to improve. The medical field is also trying to apply deep learning methods in medical imaging processing. In 2015, Evan et al. [13] proposed the FCN network, which can accept input of any size and generate output of corresponding size. Due to its powerful image segmentation ability, it is widely used in medical image segmentation. In 2019, Gu et al. [14] proposed a context encoder network (CE-Net) to capture more high-level information and preserve spatial information for 2D medical image segmentation. CE-Net mainly contains three major components: a feature encoder module, a context extractor and a feature decoder module. In 2015, Ronneberger et al. [6] proposed the U-Net network, which is a convolutional neural network that can be used for medical image segmentation. The network consists of a symmetric encoder and decoder structure, where the encoder downsamples the input image to a feature map while the decoder upsamples the feature map to the same resolution as the input image. The network performs well in several medical image segmentation tasks. In addition, later generations continued to optimize on the basis of U-Net and many more efficient methods were born.
For example, Alom et al. [15] proposed a medical image segmentation method combining ResUNet and RNN. The method implements feature accumulation in recursive residual convolutional layers, improving feature representation in image segmentation tasks. In the same year, Yu et al. [11] proposed a method called Attention U-Net, which combines U-Net and attention mechanism. In this method, an attention mechanism is used to strengthen the network's attention on important features to improve segmentation accuracy. The method was tested on datasets such as ISBI and the results showed that it outperforms the traditional U-Net method. In 2020, Wang et al. [16] proposed a Non-local U-Net to overcome the drawback of local convolution for medical image segmentation. The Non-local U-Net employs the self-attention mechanism and the global aggregation block to extract full image information during the parts of both up-sampling and down-sampling, which can improve the final segmentation accuracy. The Non-local block is a general-purpose block that can be easily embedded in different convolutional neural networks to improve their performance.
In 2017, Dong et al. [17] segmented carotid plaques from MRI images based on a fully convolutional network (FCN). The method uses FCN as the base network architecture, making GoogLeNet, VGG-16, and ResNet-101, respectively, the networks for the feature extraction stage. The highest average segmentation accuracy of 56% can be achieved. In 2020, Meshram et al. [18] performed plaque segmentation on longitudinal carotid ultrasound images based on U-Net and Dilated U-Net. The experimental results show that the segmentation accuracies are 48 and 55% under the automatic segmentation method. In 2020, Xie et al. [19] performed vascular and plaque segmentation of carotid ultrasound images based on U-Net, which proposed dual-decoder convolutional U-Net was used as the segmentation network with one decoder for segmenting blood vessels and the other for segmenting plaques, leading an accuracy of 91% for blood vessel segmentation and 69% for plaque segmentation.One notable contribution in this area is the work by Zhao et al. [20] in 2021. They introduced a comprehensive method for the automatic extraction and evaluation of coronary artery stenosis based on invasive coronary angiogram images. The authors addressed the limitations of manual segmentation and assessment methods, which are time-consuming and prone to errors. In their study, a deep learning model which integrates a feature pyramid with a U-Net++ model was developed to automatically segment coronary arteries in ICAs. A compound loss function which contains Dice loss, dilated Dice loss and L2 regularization was utilized to train the proposed segmentation model. Following the segmentation, an algorithm that extracts vascular centerlines, calculates the diameters and measures the stenotic levels was developed to detect arterial stenosis. Experimental results demonstrated the effectiveness of their method in accurately extracting coronary arteries and reliably evaluating stenosis severity. This work provides valuable insights and tools for the early diagnosis and treatment of coronary artery disease, offering potential benefits in clinical practice
In addition, there are some other methods. GAN networks are also commonly used for image segmentation. Segmentation of COVID-19 infections in medical imaging plays a crucial role in the diagnosis and treatment of the disease. Several studies have focused on developing effective segmentation methods for COVID-19 infections. Notably, He et al. [21] proposed an evolvable adversarial network with a gradient penalty for COVID-19 infection segmentation. However, none of the above methods deliberately extract texture information from the carotid ultrasound image. Aiming to make full use of the sufficient texture information of carotid ultrasound images, we propose a novel network called as Contrast U-Net, which unifies a contrast block module and encoder-decoder deep learning framework for accurate texture information extraction. First, we introduce a contrast block module to calculate the texture features of intravascular ultrasound images. Second, the Contrast U-Net model is proposed by incorporating the contrast block module into the encoder-decoder deep learning framework with the squeeze-and-excitation block [22] to the skip connection for adaptively recalibrating the intensity of feature responses between channels. The experimental results show that the proposed Contrast U-Net model is surprisingly effective in carotid plaque detection. The performance is effectively improved relative to the reference, while the number of parameters and computational effort are substantially reduced.
In this section, we introduce a more efficient variant of U-Net network, called Contrast U-Net, which is an improved version that enhances training efficiency while maintaining prediction accuracy. Our model greatly reduces hardware consumption during training and provides a solid foundation for updating the model during online training. Then, we provide a detailed explanation of contrast block module and squeeze-and-excitation block module, these two blocks incorporated into the U-Net architecture. In the end, we describe the loss function used in the Contrast U-Net model.
The proposed architecture called Contrast U-Net, which is illustrated in Figure 1 and is composed of four key modules: encoder, decoder, contrast block, and squeeze-and-excitation. The encoder and decoder modules are similar to the classic U-Net [23], utilizing convolution and downsampling to extract multi-scale features. To improve computational efficiency and reduce hardware requirements during training, our algorithm reduces the number of convolutional kernels in each layer by half. Since each layer's feature map in our model is only half the size of the U-Net, the computational workload associated with the corresponding convolutions and pooling operations is also reduced by half. Additionally, we have introduced fixed operators, further decreasing the computational burden of the backpropagation process.
During downsampling, there are two different convolution blocks in Contrast U-Net model. The first block is similar to U-Net, employing two consecutive convolutions with a kernel of 3×3 and an output channel number twice as large as the input channel number. The second block also has the same first convolution, but its second layer consists of a contrast block module, a convolution with a kernel of 3×3 and the same output channel number as the input channel number. The contrast block and convolution results are then concatenated to extract texture information and expand the channel number. Furthermore, to adaptively recalibrate the feature response strength between channels, we replace the skip connection between the encoder and decoder with a squeeze-and-excitation block.
In this work, we introduce a novel operator called a Contrast Value Operator: CVO into the contrast block module for image processing task. The general definition of CVO is described below. CVO is an effective operator that is sensitive to extract textures of images, as described in our previous work [24].
CVO operator is designed with two component convolutions: the difference component consisting of –1 and 1, and the contrast component consisting of 2 and 1. As shown in Figure 2, CVO operator in different directions is obtained by convolving the differential and contrast components in various directions.
Textures in images often exhibit directional features. By using two different directional operators for convolution, the sensitivity to various directional textures is increased, enabling better capture and emphasis of texture details in the image. Moreover, the different directional operators complement each other during the convolution process. For example, one operator may perform better for certain texture features, while the other operator may be more effective for other texture characteristics. By utilizing two different directional operators, the information from both operators is combined, enhancing the ability to extract various types of textures. In summary, employing two different directional operators for convolution enhances sensitivity to different directional textures, leading to improved capture and emphasis of texture details in the image. Additionally, the mutual supplementation of information between the different directional operators enhances the extraction capability for various texture types. This is why the CVO operator is particularly sensitive for texture extraction. We validated this point in Figure 3, where we successfully extracted surface textures for various materials. Initially, we read the three-channel image and applied the CVO convolution operation to each channel individually. The resulting textures are shown in Figure 3(b). To enhance visibility in the paper, we performed image enhancement by increasing the overall brightness to observe texture details. It is important to note that image enhancement was not applied in the model itself. Figure 3(c) displays the enhanced image, demonstrating the perfect extraction of texture details from the input image.
The proposed contrast block module is obtained by convolving the different directions of CVO operator and computing the gradient as shown in Figure 4. The input image is convolved with CVO operator from various directions before the approximate gradient is calculated.
As introduced in Section 3.2, we utilized the CVO operator to extract texture features of images. However, since the CVO operator is fixed, it does not change with each round of training, which may result in limited sensitivity to certain features such as plaque features. To enhance the saliency of the plaque area, we introduced the squeeze-and-excitation block module in our model, which is designed to be more sensitive to plaque features through multiple rounds of training.
The squeeze-and-excitation block module adaptively recalibrates the strength of feature responses between channels to simulate the interdependence between channels. Specifically, the module is designed as a matrix M consisting of H×W items of size C, where each item represents the weight of the corresponding feature item. The module is divided into two steps: squeeze and excite. In the squeeze step, a globally compressed feature vector is obtained by performing global average pooling. In the excite step, the weight of each channel is obtained through two fully connected layers and the weighted input is used as the output. The module schematic is shown in Figure 5.
The introduction of the squeeze-and-excitation block module is aimed at enhancing the sensitivity of the algorithm to plaque features and improving its overall performance. The module enables the algorithm to adaptively adjust the strength of the feature responses between channels, which is particularly useful in scenarios where the features of interest are complex or subtle. Overall, the incorporation of the squeeze-and-excitation block module represents a significant improvement to our algorithm, and we believe that it has the potential to facilitate the accurate and efficient detection of plaque features in medical imaging applications.
In carotid ultrasound images, there is a very large percentage of background pixels and only a small percentage of plaque. This leads to class imbalance in the images. To facilitate the optimization of the proposed network, focal loss (FL) [25] is used as a loss function to measure the relationship between the predicted mapping and the ground truth. It can be formulated as follows.
FL=(1−pt)γlog(pt), | (3.1) |
where pt reflects the proximity to the ground truth, and γ is a modifiable factor.
Furthermore, we also introduce Lovasz loss (LL) [26] into our loss function, which is based on the convex Lovasz expansion of submodular losses to optimize the mean IoU loss of the neural network. The mean IoU is more in line with the intuition of image comparison. So, Lovasz loss is chosen to assist focal loss to adjust the results. It can be formulated as follows.
LL=ˉΔJ1(m), | (3.2) |
where ˉΔJ1 is Jaccard loss and m is the optimization algorithm.
Finally, the total loss function is designed as:
loss=FL+α⋅LL, | (3.3) |
where α is set as 0.4 in our model.
● Dataset:
The data for this study was provided by the People's Hospital of Sichuan Province, China, consisting of a total of 117 carotid artery ultrasound images. These images are from real patients and are used for clinical research and applications, so the data set is small and not publicly available. Obtaining the initial carotid artery ultrasound images was not difficult, but the corresponding ground truth images of the plaques had to be manually segmented by doctors, which was a labor-intensive task. The carotid artery ultrasound images and GT images are shown in Figure 6. Therefore, the dataset used in this study is smaller compared to the datasets used in segmentation competitions or publicly available datasets.
In our experiments, we used 97 example images as training images. The first row displays some carotid artery ultrasound images, while the second row shows the corresponding ground truth images segmented by experts. Due to the excessive irrelevant information surrounding the carotid artery ultrasound images, they were cropped to remove irrelevant details as Figure 7 shows.
For general ultrasound images, there will be a lot of noise, which will have a bad effect on the segmentation effect. To address these limitations, several advanced techniques have been proposed. One notable approach is the Peckle Reducing Bilateral Filter method introduced by [27]. They develop a new bilateral filter for speckle reduction in ultrasound images for follicle segmentation and measurement. Different from the previous bilateral filters, the proposed bilateral filter uses normalized difference in the computation of the Gaussian intensity difference. We also present the results of follicle segmentation after speckle reduction. Experimental results on both synthetic images and real ultrasound images demonstrate the effectiveness of the proposed filter. There is another notable approach is the robust detail preserving anisotropic diffusion (RD-PAD) method introduced by [28]. This method aims to reduce speckle noise while preserving important details in ultrasound images. RD-PAD utilizes anisotropic diffusion, which adapts the diffusion process based on local image features to selectively reduce noise in homogeneous regions while preserving edges and fine structures. By incorporating robust statistics, the proposed method effectively suppresses speckle noise without blurring important image features. The dataset utilized in this study was provided by the Sichuan Provincial People's Hospital and it underwent assessment by medical professionals who determined that the level of noise was not severe. Subsequent experimental results revealed that the proposed model in this paper exhibits a certain degree of noise resilience. Consequently, in the preprocessing stage, only image cropping was performed to eliminate irrelevant textual information.
● Evaluation indicator:
We adopt accuracy (Acc), mean accuracy (MAcc), mean intersection over union (MIoU) and frequency weighted intersection over union (FWIoU) for the quantitative evaluation. The accuracy (Acc) is a basic evaluation indicator for evaluating the performance of the segmentation algorithm. The ratio of the correct classification of pixels in the division result is to the total number of pixels. The calculation method of accuracy is:
Accuracy=TP+TNTP+TN+FP+FN, | (4.1) |
where, TP indicates true positive, that is, the number of pixels that the algorithm will correctly classify; TN indicates true negative, that is, the number of pixels that are correctly classified by the algorithm; FP indicates false positive, that is, the algorithm is classified into the number of pixels with negative errors; FN indicates false negative, that is, algorithm classifies the number of pixels with a positive error into negative. The range of accuracy is [0, 1] and the larger the value, the closer the segmentation result is to the ground truth.
The mean accuracy (MAcc) calculates the segmentation algorithm based on the classification results of pixel levels The accuracy of the average classification can better reflect the overall performance of the segmentation algorithm. The calculation method of the average accuracy is:
MAcc=1NcNc∑i=1TPiTPi+FPi, | (4.2) |
where, NC represents the number of categories of classification, and TPi indicates the number of pixels that should be classified as category i and the correct classification. FPi indicates the number of pixels misclassified as category i. The range of MACC's value is [0, 1] and the larger the value, the division results closer to the real results.
Mean intersection over union (MIoU) is calculated based on pixel -level classification results, the average classification accuracy of the segmentation algorithm and considers the position relationship between pixels. The calculation method of the average interchange ratio is:
MIoU=1NcNc∑i=1TPiTPi+FPi+FNi, | (4.3) |
where, NC represents the number of categories of classification, and TPi indicates the number of pixels classified as category i and the correct classification. FPi indicates the number of pixels misclassified as category i. FNi indicates the number of pixels should be classified as category i but error classified as other category. The range of MIoU's value is [0, 1] and the larger the value, the division results closer to the real results.
Frequency weighted intersection over union (FWIoU) considers the occurrence frequency of each class in the entire dataset. Therefore, for datasets with class imbalance, FWIoU can better reflect the performance of the model. FWIoU is defined as the weighted average of the intersection over union (IoU) for each class, where the weight is the occurrence frequency of that class in the entire dataset. Specifically, assuming that there are K classes in the dataset, the occurrence frequency of the k−th class is fk and the intersection and union of the model on that class are Ik and Uk, respectively, then FWIoU can be represented as:
FWIoU=1∑Kk=1fkK∑k=1fk⋅IkUk+ϵ, | (4.4) |
where, ϵ is a small constant used to avoid division by zero. The value range of FWIoU is [0, 1], and the larger the value, the better the performance of the model.
● Implementation:
1) In our experiments we used 97 examples as training images and enhanced the data by horizontal rotation, vertical rotation, etc. and the remaining 20 examples were used as prediction images.
2) The framework for all experiments was done using PyTorch 1.11.0 on an Nvidia RTX 3070Ti GPU.
To showcase the effectiveness of the contrast block and the loss function, we conducted experiments to demonstrate their individual impacts, and the results are presented in Tables 1 and 2. These tables demonstrate that the addition of contrast block and the proposed loss function has significantly improved various metrics.
Contrast Block | ✓ | |
Acc | 98.23 | 98.53 |
MAcc | 79.75 | 80.33 |
MIoU | 71.95 | 74.52 |
FWIoU | 96.91 | 97.32 |
Loss | FL | LL | FL+LL | FL+0.4LL |
Acc | 98.42 | 97.51 | 98.51 | 98.53 |
MAcc | 68.86 | 49.99 | 78.48 | 80.33 |
MIoU | 67.74 | 48.76 | 73.64 | 74.52 |
FWIoU | 96.90 | 95.11 | 97.27 | 97.32 |
Notably, when compared to using a single FL or LL, the addition of CVO led to a considerable decrease in MAcc and MIoU. Additionally, the one-to-one ratio of various metrics was lower than that of our approach. The experimental results demonstrate the effectiveness of our proposed contrast block and loss function in improving the accuracy of carotid ultrasound image analysis.
We compared our proposed model with several state-of-the-art methods, including U-Net, UNet++ [9], and ResUNet++ [29]. Although these models do not intentionally extract texture information, texture is a crucial aspect of carotid ultrasound images. Hence, our model deliberately extracts texture information and Table 3 demonstrates the benefits of our approach.
Model | U-Net | U-Net++ | ResUNet++ | Ours |
Acc | 98.31 | 98.49 | 98.19 | 98.53 |
MAcc | 79.33 | 77.55 | 71.59 | 80.33 |
MIoU | 72.34 | 73.01 | 67.22 | 74.52 |
FWIoU | 97.01 | 97.22 | 96.68 | 97.32 |
In particular, our network employs a contrast block, which uses a fixed convolutional kernel to extract texture information. By doing so, our model does not require computing the convolutional kernel of the contrast block during extensive learning, thereby reducing the number of parameters and computational resources required.
Table 4 presents the number of parameters and computational efforts of each model. Our model has significantly lower parameters and computational requirements than the other models, making it easier for physicians to locate plaques during the detection process. The results of Tables 3 and 4 suggest that the complexity of the model does not necessarily translate to higher accuracy in detecting plaque locations in carotid ultrasound images.
Model | U-Net | U-Net++ | ResUNet++ | Ours |
Params(M) | 7.8 | 26.7 | 54.4 | 1.8 |
MACs(G) | 4.3 | 9.2 | 14.5 | 0.9 |
To further illustrate the effectiveness of Contrast U-Net, we show some qualitative comparison results with state-of-the-art methods as shown in Figure 7. In Figure 8, our model can detect plaques that are difficult for physicians to identify as well as plaques in carotid ultrasound images with poor imaging quality.
Overall, our proposed model is a promising approach for plaque detection in carotid ultrasound images with fine texture extraction and low computational complexity.
After adding the CVO operator, the results met our expectations. The algorithm became more sensitive to the details of the image texture, allowing us to rapidly identify areas of interest such as blood vessels and plaques. By incorporating the squeeze-and-excitation block, we were able to selectively enhance the weight of the desired plaque area while suppressing the weight of other blood vessels that could interfere with our analysis. This allowed us to more accurately identify the plaques area of interest.
In addition, we recorded the changes in the loss function of our proposed model and other models on the training set as shown in Figure 9, the Figure 9(a) shows the change of loss in processing time, the Figure 9(b) shows the change of loss at each epoch and analyzed them. Compared to the classic U-Net model, our model, incorporating a fixed contrast operator, converges faster in the direction we anticipated, even with half the number of parameters. We analyzed that learning the plaque region requires capturing a lot of texture and edge information. The traditional U-Net model gradually learns these contents through multiple rounds of backpropagation. However, by introducing the fixed contrast operator, we essentially provide guidance for the learning direction. The faster convergence of U-Net++ and ResU-Net++ networks is attributed to the utilization of more parameters compared to the traditional U-Net model, while still retaining many shallow features. Many edge and texture features tend to weaken as the network deepens. However, in comparison to these two algorithms, our approach achieves excellent results with minimal parameter usage. This indicates the feasibility of incorporating the fixed operator for specific segmentation applications. Additionally, U-Net++ provides us with insights and in future research we will consider how to preserve more shallow features and integrate the fixed operator into shallow networks.Through Figure 9(b), we can get that, in terms of computational resource requirements, this algorithm is significantly lower compared to the other algorithms, leading to a substantial reduction in training time. As the training dataset size increases, the computational advantage of this model becomes more evident. Furthermore, it provides a foundation for online model updates.
Going forward, we can consider increasing the number of model parameters to further improve its effectiveness or continue to pursue a lightweight design concept to achieve online updating of the predictive model. Theoretically, with the increase in the training dataset through online updates, the recognition accuracy can be improved. Therefore, the requirement for model lightweight design becomes particularly important. This is precisely the significance of our research. In the designed scenario, doctors can make minor adjustments to obtain accurate ground truth regions after observing the areas predicted by the model, thereby continuously expanding the training dataset. During the doctors' rest time, the model can automatically update its training dataset and retrain the predictive model to improve prediction accuracy. This approach of online updating the predictive model is not only applicable to carotid plaque detection but also serves as a promising strategy for private datasets with high manual labeling costs. The utilization of lightweight models, coupled with online updating of the predictive model, proves to be a viable solution.
This paper introduced a novel network called Contrast U-Net, which is based on U-Net network and designed to help clinicians quickly locate carotid plaques. Contrast U-Net is effective in utilizing the texture information of carotid ultrasound images, moreover the addition of the contrast block module helped Contrast U-Net model significantly reduced the number of parameters and the amount of computation required for model training in comparison with other popular models. Experimental results demonstrated that Contrast U-Net model outperformed other models in terms of quality. Overall, the proposed approach offered a promising solution to improve the accuracy and efficiency of plaque detection in carotid ultrasound images.
The authors declare they have not used Artificial Intelligence (AI) tools in the creation of this article.
This work was supported by Natural Science Foundation of Sichuan, China under Grant 2023NSFSC0504. All data in this paper are supported by Sichuan Provincial People's Hospital, China.
The authors declare there is no conflict of interest.
[1] |
G. K. Hansson, Inflammation, atherosclerosis, and coronary artery disease, N. Engl. J. Med., 352 (2005), 1685–1695. http://doi.org/10.1056/NEJMra043430 doi: 10.1056/NEJMra043430
![]() |
[2] |
R. Virmani, F. D. Kolodgie, A. P. Burke, A. V. Finn, H. K. Gold, T. N. Tulenko, et al., Atherosclerotic plaque progression and vulnerability to rupture: angiogenesis as a source of intraplaque hemorrhage, Arterioscler. Thromb. Vasc. Biol., 25 (2005), 2054–2061. http://doi.org/10.1090/S0894-0347-1992-1124979-1 doi: 10.1090/S0894-0347-1992-1124979-1
![]() |
[3] |
B. Smitha, Joseph K. Paul, Fractal and multifractal analysis of atherosclerotic plaque in ultrasound images of the carotid artery, Chaos Solitons Fractals, 123 (2019), 91–100, https://doi.org/10.1016/j.chaos.2019.03.041 doi: 10.1016/j.chaos.2019.03.041
![]() |
[4] |
M. Biswas, L. Saba, T. Omerzu, A. M. Johri, N. N. Khanna, K. Viskovic, et al., A review on joint carotid intima-media thickness and plaque area measurement in ultrasound for cardiovascular/stroke risk monitoring: Artificial intelligence framework, J Digit Imaging, 34 (2021), 581–604. http://doi.org/10.1007/s10278-021-00461-2 doi: 10.1007/s10278-021-00461-2
![]() |
[5] |
Y. Li, S. Zheng, J. Zhang, F. Wang, X. Liu, W. He, Advance ultrasound techniques for the assessment of plaque vulnerability in symptomatic and asymptomatic carotid stenosis: A multimodal ultrasound study, Cardiovasc. Diagn. Ther., 11 (2021), 28. http://doi.org/10.21037/cdt-20-876 doi: 10.21037/cdt-20-876
![]() |
[6] | O. Ronneberger, P. Fischer, T. Brox, U-net: Convolutional networks for biomedical image segmentation, in Medical Image Computing and Computer-Assisted Intervention – MICCAI 2015, 9351 (2015). http://doi.org/10.1007/978-3-319-24574-4_28 |
[7] | Ö. Çiçek, A. Abdulkadir, S. S. Lienkamp, T. Brox, O. Ronneberger, 3d u-net: Learning dense volumetric segmentation from sparse annotation, in Medical Image Computing and Computer-Assisted Intervention–MICCAI 2016: 19th International Conference, (2016), 424–432. https://doi.org/10.48550/arXiv.1606.06650 |
[8] | F. Milletari, N. Navab, S. A. Ahmadi, V-net: Fully convolutional neural networks for volumetric medical image segmentation, in 2016 fourth international conference on 3D vision (3DV), (2016), 565–571. https://doi.org/10.1109/3DV.2016.79 |
[9] | Z. Zhou, M. M. Rahman Siddiquee, N. Tajbakhsh, J. Liang, Unet++: A nested u-net architecture for medical image segmentation, in Deep learning in medical image analysis and multimodal learning for clinical decision support, Springer, (2018), 3–11. http://doi.org/10.1007/978-3-030-00889-5_1 |
[10] | F. Isensee, J. Petersen, A. Klein, D. Zimmerer, P. F. Jaeger, S. Kohl, et al., nnu-net: Self-adapting framework for u-net-based medical image segmentation, preprint, arXiv: 1809.10486. |
[11] | O. Oktay, J. Schlemper, L. L. Folgoc, M. J. Lee, M. P. Heinrich, K. Misawa, et al., Attention u-net: Learning where to look for the pancreas, preprint, arXiv: 1804.03999. |
[12] | H. Chen, X. Qi, L. Yu, P. A. Heng, Dcan: deep contour-aware networks for accurate gland segmentation, in Proceedings of the IEEE conference on Computer Vision and Pattern Recognition, (2016), 2487–2496. https://doi.org/10.1016/j.media.2016.11.004 |
[13] |
E. Shelhamer, J. Long, T. Darrell, Fully convolutional networks for semantic segmentation, IEEE Trans. Pattern Anal. Mach. Intell., 39 (2017), 640–651. http://doi.org/10.1109/TPAMI.2016.2572683 doi: 10.1109/TPAMI.2016.2572683
![]() |
[14] |
Z. Gu, J. Cheng, H. Fu, K. Zhou, H. Hao, Y. Zhao, et al., Ce-net: Context encoder network for 2d medical image segmentation, IEEE Trans. Med. Imaging., 38 (2019), 2281–2292. http://doi.org/10.1109/TMI.2019.2903562 doi: 10.1109/TMI.2019.2903562
![]() |
[15] | M. Z. Alom, M. Hasan, C. Yakopcic, T. M. Taha, V. K. Asari, Recurrent residual convolutional neural network based on u-net (r2u-net) for medical image segmentation, preprint, arXiv: 1802.06955. |
[16] | Z. Wang, N. Zou, D. Shen, S. Ji, Non-local u-nets for biomedical image segmentation, in Proceedings of the AAAI Conference on Artificial Intelligence, (2020), 6315–6322. http://doi.org/10.48550/arXiv.1812.04103 |
[17] | Y. Dong, Y. Pan, X. Zhao, R. Li, C. Yuan, W. Xu, Identifying carotid plaque composition in mri with convolutional neural networks, in 2017 IEEE International Conference on Smart Computing (SMARTCOMP), (2017), 1–8. http://doi.org/10.1109/SMARTCOMP.2017.7947015 |
[18] |
N. H. Meshram, C. C. Mitchell, S. Wilbrand, R. J. Dempsey, T. Varghese, Deep learning for carotid plaque segmentation using a dilated u-net architecture, Ultrasonic Imaging, 42 (2020), 221–230. http://dx.doi.org/10.1177/0161734620951216 doi: 10.1177/0161734620951216
![]() |
[19] | M. Xie, Y. Li, Y. Xue, L. Huntress, W. Beckerman, S. A. Rahimi, et al., Two-stage and dual-decoder convolutional u-net ensembles for reliable vessel and plaque segmentation in carotid ultrasound images, in 2020 19th IEEE International Conference on Machine Learning and Applications (ICMLA), (2020), 1376–1381. http://doi.org/10.1109/ICMLA51294.2020.00214 |
[20] |
C. Zhao, A. Vij, S. Malhotra, J. Tang, H. Tang, D. Pienta, et al., Automatic extraction and stenosis evaluation of coronary arteries in invasive coronary angiograms, Comput. Biol. Med., 136 (2021), 104667. https://doi.org/10.1016/j.compbiomed.2021.104667 doi: 10.1016/j.compbiomed.2021.104667
![]() |
[21] |
J. He, Q. Zhu, K. Zhang, P. Yu, J. Tang, An evolvable adversarial network with gradient penalty for covid-19 infection segmentation, Appl. Soft Comput., 113 (2021), 107947. http://doi.org/10.1016/j.asoc.2021.107947 doi: 10.1016/j.asoc.2021.107947
![]() |
[22] | J. Hu, L. Shen, G. Sun, squeeze-and-excitation networks, in Proceedings of the IEEE Conference on Computer Vision and Pattern Recognition, (2018), 7132–7141. http://dx.doi.org/10.48550/arXiv.1709.01507 |
[23] | O. Ronneberger, P. Fischer, T. Brox, U-net: Convolutional networks for biomedical image segmentation, in International Conference on Medical image computing and computer-assisted intervention, Springer, (2015), 234–241. http://doi.org/10.1007/978-3-319-24574-4_28 |
[24] | Y. He, S. Xiang, W. Zhou, B. Peng, R. Wang, L. Li, A novel contrast operator for robust object searching, in 2021 17th International Conference on Computational Intelligence and Security (CIS), (2021), 309–313. http://doi.org/10.1109/CIS54983.2021.00071 |
[25] | T. Y. Lin, P. Goyal, R. Girshick, K. He, P. Dollár, Focal loss for dense object detection, in Proceedings of the IEEE international conference on computer vision, (2017), 2980–2988. http://doi.org/10.48550/arXiv.1708.02002 |
[26] | M. Berman, A. R. Triki, M. B. Blaschko, The lovász-softmax loss: A tractable surrogate for the optimization of the intersection-over-union measure in neural networks, in Proceedings of the IEEE conference on computer vision and pattern recognition, (2018), 4413–4421. http://doi.org/10.48550/arXiv.1705.08790 |
[27] |
J. Tang, S. Guo, Q. Sun, Y. Deng, D. Zhou, Speckle reducing bilateral filter for cattle follicle segmentation, BMC Genomics, 11 (2010), 1–9. http://doi.org/10.1186/1471-2164-11-S2-S9 doi: 10.1186/1471-2164-11-S2-S9
![]() |
[28] |
X. Liu, J. Liu, X. Xu, L. Chun, J. Tang, Y. Deng, A robust detail preserving anisotropic diffusion for speckle reduction in ultrasound images, BMC Genomics, 12 (2011), 1–10. http://doi.org/10.1186/1471-2164-12-S5-S14 doi: 10.1186/1471-2164-12-S5-S14
![]() |
[29] | D. Jha, P. H. Smedsrud, M. A. Riegler, D. Johansen, T. De Lange, P. Halvorsen, et al., Resunet++: An advanced architecture for medical image segmentation, in 2019 IEEE International Symposium on Multimedia (ISM), (2019), 225–2255. http://doi.org/10.1109/ISM46123.2019.00049 |
1. | Enxu Zhang, Ning Zhang, Fei Li, Guowei Qi, 2023, Texture-based Fabric Defect Detection Method, 979-8-3503-8287-7, 1, 10.1109/ICEMCE60359.2023.10490814 | |
2. | Bolin Xiao, Wenjun Zhou, Tianfei Wang, Quan Zhang, Bo Peng, Infrared small target detection via contrast-enhanced dual-branch network, 2025, 159, 10512004, 104988, 10.1016/j.dsp.2025.104988 |
Contrast Block | ✓ | |
Acc | 98.23 | 98.53 |
MAcc | 79.75 | 80.33 |
MIoU | 71.95 | 74.52 |
FWIoU | 96.91 | 97.32 |
Loss | FL | LL | FL+LL | FL+0.4LL |
Acc | 98.42 | 97.51 | 98.51 | 98.53 |
MAcc | 68.86 | 49.99 | 78.48 | 80.33 |
MIoU | 67.74 | 48.76 | 73.64 | 74.52 |
FWIoU | 96.90 | 95.11 | 97.27 | 97.32 |
Model | U-Net | U-Net++ | ResUNet++ | Ours |
Acc | 98.31 | 98.49 | 98.19 | 98.53 |
MAcc | 79.33 | 77.55 | 71.59 | 80.33 |
MIoU | 72.34 | 73.01 | 67.22 | 74.52 |
FWIoU | 97.01 | 97.22 | 96.68 | 97.32 |
Model | U-Net | U-Net++ | ResUNet++ | Ours |
Params(M) | 7.8 | 26.7 | 54.4 | 1.8 |
MACs(G) | 4.3 | 9.2 | 14.5 | 0.9 |
Contrast Block | ✓ | |
Acc | 98.23 | 98.53 |
MAcc | 79.75 | 80.33 |
MIoU | 71.95 | 74.52 |
FWIoU | 96.91 | 97.32 |
Loss | FL | LL | FL+LL | FL+0.4LL |
Acc | 98.42 | 97.51 | 98.51 | 98.53 |
MAcc | 68.86 | 49.99 | 78.48 | 80.33 |
MIoU | 67.74 | 48.76 | 73.64 | 74.52 |
FWIoU | 96.90 | 95.11 | 97.27 | 97.32 |
Model | U-Net | U-Net++ | ResUNet++ | Ours |
Acc | 98.31 | 98.49 | 98.19 | 98.53 |
MAcc | 79.33 | 77.55 | 71.59 | 80.33 |
MIoU | 72.34 | 73.01 | 67.22 | 74.52 |
FWIoU | 97.01 | 97.22 | 96.68 | 97.32 |
Model | U-Net | U-Net++ | ResUNet++ | Ours |
Params(M) | 7.8 | 26.7 | 54.4 | 1.8 |
MACs(G) | 4.3 | 9.2 | 14.5 | 0.9 |