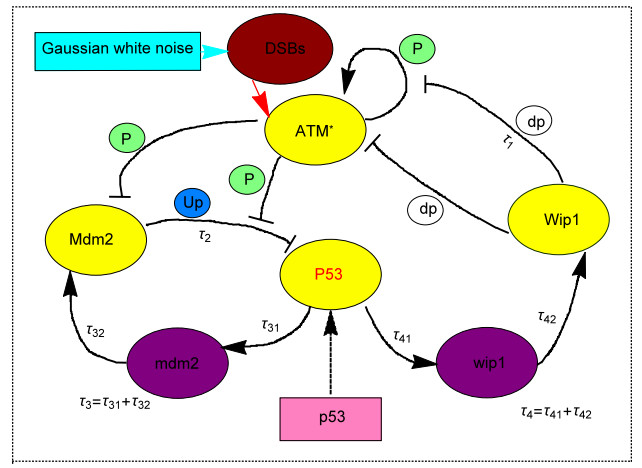
The diffusion of Sulfate ions in concrete is a complex process and affects the performance of concrete. Experiments on the time-dependent distribution of sulfate ions in concrete under the coupling of pressure load, dry-wet circulation, and sulfate attack, and the diffusion coefficient of sulfate ions with various parameters was tested. The applicability of the cellular automata (CA) theory to simulate the diffusion of sulfate ions was discussed. In this paper, a multiparameter cellular automata (MPCA) model was developed to simulate the impacts of load, immersion ways, and sulfate solution concentration for the diffusion of sulfate ions in concrete. The MPCA model was compared with experimental data, considering compressive stress, sulfate solution concentration, and other parameters. The numerical simulations verify the calculation results based on the MPCA model are in good agreement with the test data. Finally, the applicability of the established MPCA model was also discussed.
Citation: Jian Cao, Tao Liu, Ziyang Han, Bin Tu. Sulfate ions diffusion in concrete under coupled effect of compression load and dry-wet circulation[J]. Mathematical Biosciences and Engineering, 2023, 20(6): 9965-9991. doi: 10.3934/mbe.2023437
[1] | Juenu Yang, Fang Yan, Haihong Liu . Dynamic behavior of the p53-Mdm2 core module under the action of drug Nutlin and dual delays. Mathematical Biosciences and Engineering, 2021, 18(4): 3448-3468. doi: 10.3934/mbe.2021173 |
[2] | Changyong Dai, Haihong Liu, Fang Yan . The role of time delays in P53 gene regulatory network stimulated by growth factor. Mathematical Biosciences and Engineering, 2020, 17(4): 3794-3835. doi: 10.3934/mbe.2020213 |
[3] | Tingzhe Sun, Dan Mu . Multi-scale modeling identifies the role of p53-Gys2 negative feedback loop in cellular homeostasis. Mathematical Biosciences and Engineering, 2020, 17(4): 3260-3273. doi: 10.3934/mbe.2020186 |
[4] | Feng Rao, Carlos Castillo-Chavez, Yun Kang . Dynamics of a stochastic delayed Harrison-type predation model: Effects of delay and stochastic components. Mathematical Biosciences and Engineering, 2018, 15(6): 1401-1423. doi: 10.3934/mbe.2018064 |
[5] | B. Spagnolo, D. Valenti, A. Fiasconaro . Noise in ecosystems: A short review. Mathematical Biosciences and Engineering, 2004, 1(1): 185-211. doi: 10.3934/mbe.2004.1.185 |
[6] | Qingwen Hu . A model of regulatory dynamics with threshold-type state-dependent delay. Mathematical Biosciences and Engineering, 2018, 15(4): 863-882. doi: 10.3934/mbe.2018039 |
[7] | Shunyi Li . Hopf bifurcation, stability switches and chaos in a prey-predator system with three stage structure and two time delays. Mathematical Biosciences and Engineering, 2019, 16(6): 6934-6961. doi: 10.3934/mbe.2019348 |
[8] | Peng Feng, Menaka Navaratna . Modelling periodic oscillations during somitogenesis. Mathematical Biosciences and Engineering, 2007, 4(4): 661-673. doi: 10.3934/mbe.2007.4.661 |
[9] | Yifei Wang, Xinzhu Meng . Evolutionary game dynamics of cooperation in prisoner's dilemma with time delay. Mathematical Biosciences and Engineering, 2023, 20(3): 5024-5042. doi: 10.3934/mbe.2023233 |
[10] | Xiao-Wei Ye, Xiao-Peng Zhang, Feng Liu . CSB modulates the competition between HIF-1 and p53 upon hypoxia. Mathematical Biosciences and Engineering, 2019, 16(5): 5274-5262. doi: 10.3934/mbe.2019262 |
The diffusion of Sulfate ions in concrete is a complex process and affects the performance of concrete. Experiments on the time-dependent distribution of sulfate ions in concrete under the coupling of pressure load, dry-wet circulation, and sulfate attack, and the diffusion coefficient of sulfate ions with various parameters was tested. The applicability of the cellular automata (CA) theory to simulate the diffusion of sulfate ions was discussed. In this paper, a multiparameter cellular automata (MPCA) model was developed to simulate the impacts of load, immersion ways, and sulfate solution concentration for the diffusion of sulfate ions in concrete. The MPCA model was compared with experimental data, considering compressive stress, sulfate solution concentration, and other parameters. The numerical simulations verify the calculation results based on the MPCA model are in good agreement with the test data. Finally, the applicability of the established MPCA model was also discussed.
In mammalian cells, the tumor suppressor P53 is activated under various cell pressures and ensures an appropriate response from arrest and repair to the induction of senescence and apoptosis [1]. One of the main defense mechanisms against cellular stress is the activation of the tumor suppressor P53 [2]. However, alterations in the structure and function of the P53 network can lead to serious human diseases, such as cancer [3]. P53 itself is regulated by a feedback loop: in the absence of DNA damage, it is ubiquitinated by the E3 ligase Mdm2 and degraded by the proteasome [1,4,5]. Upon the DNA damage, post-translational modifications of P53 and Mdm2 prevent their interaction and allow P53 to aggregate in the nucleus, where it binds to the target gene promoters and induces their expression [1]. At the moment, a particularly dangerous type of damage is DNA double-strand breaks (DSBs), which can destroy the integrity of the genome and can have harmful consequences if not repaired [6]. In order to combat these injuries, cells will evolve sensitive sensing mechanisms that activate the DNA damage response (DDR) and induce transient cell cycle arrest in the G1 or G2 phase or terminal cell fates, such as apoptosis and senescence [6,7,8].
The tumor suppressor P53 is highly regulated by a complex network of interactions whose dominant characteristics vary by cell stress, cell type and species [9]. Recent studies have shown that the specific function of P53 is also related to its expression level. In normal mammalian cells, P53 is usually maintained at a low level due to the down-regulation of Mdm2, which helps cells avoid premature aging and apoptosis [10,11]. The concentration of P53 changes to a medium-level state when receiving a weak stimulus, thereby inducing cell cycle arrest and avoiding the inheritance of abnormal DNA replication to offspring [12,13]. When the stimulation was eliminated, the expression level of P53 returned to the normal level [14,15]. P53 will have a sustained oscillations state when stimulated by mild or moderate stimulation, which will contribute to cell cycle arrest. However, the concentration of P53 reaches a higher level when subjected to greater stimulation, which in turn induces cell apoptosis [16,17].
Time delays generally exist in various systems. From the perspective of dynamic systems, a time delay can cause larger oscillations, which plays an important role in biological systems or gene regulatory networks. It can produce a wealth of dynamic behaviors, such as periodic dynamics and chaotic dynamics [18,19,20,21]. In general, there is an average transcriptional time delay of 10∼20 minutes between the action of a transcription factor on a gene promoter and the presence of the corresponding mature mRNA in the cytoplasm [22]. Similarly, the synthesis of a typical protein from mRNA requires a translation time delay of 1∼3 minutes. In addition, due to the low copy number of the molecule and the fluctuation of the environment [23,24], the interference of noise cannot be avoided in the system. Noise can induce bistability, oscillation, and bifurcation, and it plays a key role in a series of processes including skeleton dynamics, cellular polarization, signal transduction and neural activity. From a dynamic point of view, bistability refers to the phenomenon that there exist two stable equilibrium points under a certain set of parameters of a dynamic system. Different initial values will cause the solution curves to tend to different stable equilibrium points. From a biological point of view, bistability is a common phenomenon of cell behavior, mainly showing the coexistence of two phenotypes that can occur under certain conditions. In order to adapt to the harsh living environment, some organisms often choose appropriate forms of expression according to the changes in the environment. For example, Ozbudak et al. discussed the condition of bistability behavior of the lac operon, which is a gene involved in lactose metabolism in Escherichia coli and its expression level can determine the state of the cells [25]. They found that in the absence of glucose, the lac operon gene was not induced (low expression levels) at low thio-methylgalactoside (TMG) concentrations (< 3 μM), but could be induced (high expression level) at high TMG concentrations (> 3 μM). If starting from the uninduced cells (low TMG concentration), the TMG concentration is steadily raised until the lac operon gene is induced in the cells after more than 30 μM. Conversely, starting from the induced cells (high TMG concentration), the concentration of TMG was gradually reduced until it was lower than 3 μM to turn off the expression of the lac operon gene. Hence, when the concentration of TMG is between two thresholds, the bacteria exhibit bistable dynamics.
Based on the above biological background, this paper proposes an improved model by including noise and time delay and studies the dynamic characteristics, including stability and bifurcation. The bifurcation analysis shows that under the appropriate parameter range P53 oscillation can be induced. Since time delay is common in various systems [20,21], we study the performance of the system by analyzing the influence of time delay. Taking time delay as the bifurcation parameter, the stability of the system and the existence of Hopf bifurcation are studied by using Hopf bifurcation theory. It is found that time delay is not only an important condition for the generation of Hopf bifurcation, but that it also affects the period and amplitude of the Hopf bifurcation. At the same time, the research also shows that the combination of time delay can not only promote the oscillation of the system, but it also has better robustness, which means that the parameter domain in which oscillation occurs has been broadened due to time delay. In order to study the more abundant dynamic characteristics of the system, the impact of time delay and parameter interaction on its stability is also investigated. In addition, because of the low copy number of molecules and the fluctuation of the environment [24], we also consider the impact of noise on the system. It is found that the noise can change the state of the system without delay when the stochastic differential equation is used for numerical simulation. Meanwhile, under the action of a single time delay or multiple time delays, the noise can not only induce the switching of the system state, but it can also promote system oscillation. The above research may help us to further understand the regulation mechanism of P53 in the cell cycle.
In the absence of pressure, ataxia telangiectasia mutated protein (ATM) will form inactive homodimers. After being induced by DSBs, ATM is rapidly activated by the complex formed by Mre11, Rad50 and NBS1 and decomposed into catalytically active monomers through autophosphorylation [26]. The activated ATM (ATM∗) phosphorylates the histone variant H2AX, which acts as a scaffold to recruit more proteins related to DDR and damage repair [7,27]. The negative feedback loop of Mdm2 and Wip1 is an important regulatory element in the P53 network in the model proposed by [7]; the corresponding interaction diagram is shown in Figure 1. P53-Mdm2 is the core negative feedback loop in response to the DNA DSB [7,28]. As a transcription factor, P53 can induce the transcription of Mdm2 [29], whereas normally functioning Mdm2 can be used as an E3-ubiquitin ligase to target P53 for proteasomal-mediated degradation [4,5]. Another feedback loop is P53-Wip1-ATM. The transcription of Wip1 is induced by P53. The phosphatase Wip1 not only directly dephosphorylates ATM∗, but it also inhibits ATM∗ self-activation [7,28,30].
However, many works on P53 oscillation have supposed that transcriptional and translational events are instantaneous, whereas the time needed in these gene expression processes has been poorly noticed. It is well known that gene expression refers to the process of synthesizing genetic information from a gene to a functional gene product that is usually a protein or functional RNA such as miRNA and sRNA. Transcription, transcript splicing and processing and translation are essential steps in this synthesis process. Meanwhile, the existing theoretical results suggest that the time delay can usually lead to oscillation and complex dynamic behaviors of gene regulatory networks. This shows that the delay of protein synthesis is a common phenomenon, and it is reasonable and necessary to include the delay factor in the model of the gene regulation network.
In this paper, we introduced four time delays to the model proposed by M¨onke et al. [7] to study the performance of the P53 gene regulation model. The delayed differential equations are as follows:
{ddtATM∗=A⋅ATM∗2(1+ATM∗2kA)⋅(1+Wip1(t−τ1)kWA)−dA⋅ATM∗−P⋅ATM∗⋅Wip1(t−τ1)+Smax⋅DSBγ+DSB,ddtP53=C−dP⋅P53−g⋅Mdm2(t−τ2)⋅P53kMP+P53⋅(1+R1+ATM∗),ddtmdm2=Tm⋅P53(t−τ3)kPm+P53(t−τ3)−dm⋅mdm2,ddtMdm2=TM⋅mdm2−dM⋅Mdm2−dAM⋅ATM∗⋅Mdm2,ddtwip1=Tω⋅P53(t−τ4)kPω+P53(t−τ4)−dω⋅wip1,ddtWip1=TW⋅wip1−dW⋅Wip1. | (2.1) |
The variables involved in the model are ATM∗, P53, mdm2, Mdm2, wip1 and Wip1. ATM∗ represents the activated ATM. P53 is the tumor suppressor P53 protein; mdm2 and Mdm2 are mdm2 mRNA and mdm2 protein, respectively; wip1 and Wip1 are wip1 mRNA and wip1 protein, respectively. Here, τ1 is the time delay needed in the dephosphorylation of ATM∗ depending on the transport of Wip1. τ2 is the time delay needed in the degradation of P53 depending on the transport of Mdm2. τ3 is the time delay of Mdm2 protein synthesis. τ4 is the time delay of Wip1 protein synthesis. Other parameters of this model were mostly selected according to the literature[7], and all parameter values are shown in Table 1.
Parameter | Description | Value | Reference |
A | Maximum self-activation rate of ATM | 30.5 | [7] |
P | Dephosphorylation rate of ATM by Wip1 | 22 | [28] |
C | Production rate of P53 | 1.4 | [30] |
g | maximum degradation of P53 by Mdm2 | 2.5 | [30] |
dAM | Degradation rate of Mdm2 by ATM∗ | 20 | [28] |
Tm | Mmaximum production rate of mdm2 | 1 | [7] |
TM | Maximum production rate of Mdm2 | 4 | [7] |
Tω | Maximum production rate of wip1 | 1 | [7] |
TW | Maximum production rate of Wip1 | 1 | [7] |
dA | Basic dephosphorylation rate of ATM∗ | 0.16 | [7] |
dP | Basic degradation rate of P53 | 0.1 | [31] |
dm | Basic degradation rate of mdm2 | 1 | [7] |
dM | Basic degradation rate of Mdm2 | 2 | [30] |
dω | Basic degradation rate of wip1 | 1.3 | [7] |
dW | Basic degradation rate of Wip1 | 2.3 | [28] |
kA | Michaelis constant for the ATM∗ self activation | 0.5 | [7] |
kWA | Michaelis constant for the inhibition of the ATM∗ self-activation by Wip1 | 0.14 | [7] |
kMP | Michaelis constant of the degradation for P53 by Mdm2 | 0.15 | [30] |
kPm | Michaelis constant for the production of mdm2 by P53 | 1 | [7] |
kPω | Michaelis constant for the production of wip1 by P53 | 1 | [7] |
R | Strength of the inhibition of the Mdm2 mediated degradation of P53 by ATM∗ | 2 | [7] |
γ | Michaelis contant for the signal strength of the DSB process | 9 | [32] |
Smax | Maximum signal strength of the DSB process | 0.2 | [33] |
DSB | DNA damage in the form of DSBs | 0.1 | Estimate |
τ1 | Time delay needed in the dephosphorylation of ATM∗ depend on the transport of Wip1 | 0∼5 | Estimate |
τ2 | Time delay needed in the degradation of P53 depend on the transport of Mdm2 | 0∼5 | Estimate |
τ3 | Time delay needed in the Mdm2 protein synthesis | 0∼5 | Estimate |
τ4 | Time delay needed in the Wip1 protein synthesis | 0∼5 | Estimate |
In this research, particular attention has been paid to the bistability and oscillations of the P53-Mdm2-Wip1 network. Bistability refers to the ability to achieve two different internal states under different stimulus conditions. It is ubiquitous in natural biomolecular networks and has important biological significance [34,35]. Cells can switch their state between two internal states to accommodate environmental and intercellular conditions owing to regulatory interactions among cellular components. Oscillatory behaviors are produced by the corresponding interactions among genes, proteins, and metabolites. They are used to control various aspects of cell physiology, including cell growth, maturity, division and death [36,37]. Hopf bifurcation, including supercritical and subcritical Hopf bifurcation, is the key principle in the design of biochemical oscillators. Noise is ubiquitous in cells, and it is generated from the fluctuation of the chemical reaction during the transcription and translation process or caused by the fluctuation of the concentration or state of other components in the cell [38]. Moreover, research has shown that noise can often cause bistability, oscillations and bifurcations, even if these behaviors cannot be observed in deterministic models [13,14]. In addition, noise-induced stochastic resonance is widely used in chemical analysis, instrument analysis and signal detection[16,17]. Next, we will investigate these dynamics via numerical simulation and bifurcation analysis by using XPPAUT and Matlab software. To be specific, first of all, the influence of important parameters in the model on the system when there is no time delay is investigated. This part essentially considers the model proposed by M¨onke et al. [7]. Second, the influence of a single time delay on system dynamics is studied. Third, the cooperative effect of multiple time delays is discussed. Furthermore, the cooperative effects of important parameters and multiple time delays are explored. Finally, the influence of noise on the system is researched.
In this section, the model of M¨onke et al. (let τ1=τ2=τ3=τ4=0 in Eq (2.1)) is adopted to clarify the influence of different model parameters on the dynamic behavior of the P53 system. Here, we mainly study the influence of six parameters, including P, dm, Tω, C, TM and TW that respectively refer to the dephosphorylation rate of ATM by Wip1, the basic degradation rate of mdm2, the maximum production rate of wip1, the production rate of P53, the maximum production rate of Mdm2 and the maximum production rate of Wip1. It should be noted that in the bifurcation diagram below, the red and black lines respectively represent stable and unstable steady states, green dots are the maxima and minima of the oscillation state corresponding to the stable limit cycles and the blue open circles denote the maxima and minima of the oscillation state corresponding to the unstable limit cycles.
Experiments have shown that the activation of ATM will induce sustained oscillation of P53. Next, we will study how the level of ATM affects the expression level of P53. For this reason, we draw the bifurcation diagram of P53 concentration versus P which is the dephosphorylation rate of ATM by Wip1, as shown in Figure 2(A). When the dephosphorylation rate of ATM by Wip1 is very small, the concentration of P53 is at a high level, which promotes cell apoptosis. With the increase of the dephosphorylation rate of ATM by Wip1, the P53 system appears bistable approximately in the interval (4.708,13.13) and a subcritical Hopf bifurcation point appears at P≈13.13, which leads to a series of sustained oscillations in the P53 system. These oscillations disappear when the dephosphorylation rate of ATM by Wip1 increases and exceeds the bistable region. Finally, the concentration of P53 enters a low state, which helps cells avoid premature senescence and apoptosis [20,28,39].
It can be seen in Figure 2(B) that the expression level of P53 is dependent on dm which is the basic degradation rate of mdm2. Specifically, when the basic degradation rate of mdm2 is small enough, there exists a unique stable steady state. With the basic degradation rate of mdm2 increasing gradually, the bistability of the system appears approximately in the interval (0.1062,0.2931) and a Hopf bifurcation point appears at dm≈0.2931, which leads to sustained oscillations of the P53 system. Interestingly, in the bistable region, the high steady state and the low steady state coexist. However, the concentration of P53 enters a low state when the basic degradation rate of mdm2 increases and exceeds the bistable region, which means that the cell is in a dormant state.
As can be seen in Figure 3(A), when Tω, i.e., the maximal production rate of wip1, is low, the concentration of p53 is in a high state. As the maximal production rate of wip1 increases gradually, bistability occurs in the range starting from Tω≈0.2609 to Tω≈0.7303 and a Hopf bifurcation point appears at Tω≈0.7303, which leads to a series of sustained oscillations of the system. When the maximal production rate of wip1 increases to a certain extent, the concentration of P53 is in a low stable steady state, which means that the cells enter a normal state. A bifurcation point (HB) appears when the maximal production rate of wip1 takes an appropriate value, which indicates that the maximal production rate of wip1 is very important for the dynamics of P53.
As observed in Figure 3(B), the concentration of P53 is located at a high state if C (the production rate of P53) is small enough. As the production rate of P53 increases gradually, bistability occurs approximately in the interval (0.1549,0.7123). In particular, a Hopf bifurcation point occurs at C≈0.7123, which means that the system generates sustained oscillations. When the production rate of P53 increases to a certain extent, the concentration of P53 enters a low state, which corresponds to the normal cellular state.
TM and TW denoted the maximum productivity of Mdm2 and the maximum productivity of Wip1, respectively. Because the concentration of Wip1 and Mdm2 can affect the concentration of P53, we also studied the influence of TM and TW on the P53 dynamics, which may help to further understand the significance of the model parameters in the dynamic behavior of P53. To show this, the bifurcation diagrams of the P53 concentration versus TM and TW are given in Figure 4.
Figure 4(A) reveals that when the value of the maximum production rate of Mdm2 changes, the concentration of P53 also changed. It can be seen that there exists a unique stable steady state if the maximum production rate of Mdm2 is small enough. With the maximum production rate of Mdm2 increasing gradually, the system appears bistable approximately in the interval (12.01,37.79). In this range, the high steady state and the low steady state coexist. The concentration of P53 enters a high state when the maximum production rate of Mdm2 rises and exceeds the bistable region, which means that the cell undergoes apoptosis. Figure 4(B) shows that with the increase of TW, there is a certain change in the concentration level of P53. When the maximum production rate of Wip1 is low, the bistability of the P53 concentration occurs. Then, as the increase of the maximum production rate of Wip1 further exceeds the bistable region, the concentration of P53 enters a low stable state, which indicates that the cells eventually enter a dormant state. Through numerical simulation, it is found that the proper parameter values can cause system oscillation. Therefore, we can change the dynamic properties of the system by regulating important parameters, and then we can control the dynamic characters of P53.
Negative feedback is the most fundamental requirement for the generation of biological oscillations. However, for a network with one node, a negative feedback loop alone can not lead to biological oscillations unless it is coupled with other elements including time delays. The negative feedback loop among a network with two or more nodes can generate oscillation under appropriate parameter conditions, but if time delay is incorporated, it can enhance the robustness of the period and amplitude of the oscillation [40,41,42,43]. Here, we study the performance of the system by analyzing the effect of time delay. We have theoretically deduced that time delay is an important condition to induce Hopf bifurcation. Because this process is very tedious, for the convenience of reading, it has been placed in the supplementary material, and only the numerical analysis is given in the main text. In order to study the effect of time delay τ2 on the system, we assume that the values of time delay τ1, τ3 and τ4 are zero. It is easy to verify that the system has a unique positive equilibrium E∗≈(0.00164,0.19733, 0.16481,0.32428,0.12678,0.05512).
Figure 5 displays the time course of the P53 system. Clearly, the positive equilibrium point is stable if there are no time delays in the system, which can be seen in Figure 5(A). If there is a time delay in the system, its influence on P53 is shown as in Figure 5(B)–(D). The positive equilibrium point E∗ of the system is asymptotically stable when τ2=1 and unstable for τ2=2. This result indicates that as the time delay increases and crosses through the critical value, the equilibrium of the system loses its stability and the system undergoes a Hopf bifurcation. The critical value of time delay for the Hopf bifurcation was calculated to be τ02≈1.20245.
Figure 6(A) is the time evolution diagram of the system under the action of time delay τ1. Obviously, the positive equilibrium point E∗ of the system is stable if τ1=0. And as the time delay τ1 increases, the system is still in a stable state. In Figure 6(B), we can see that the system generates a series of sustained oscillations when the value of the delay τ2 is greater than the critical value, and that the period and amplitude of the oscillations increase with the increase of τ2. It can be seen in Figure 6(C) that the positive equilibrium point E∗ of the system is stable when the time delay τ3=0. The positive equilibrium point of the system is asymptotically stable when the time delay τ3=1. However, the positive equilibrium E∗ of the system (2.1) is unstable when the time delay τ3=1.5 or τ3=2. Figure 6(D) shows that the system is in a stable state no matter whether it is under the effect of the time delay τ4 or without the time delay τ4. This indicates that, for the parameter values shown in Table 1, the time delays τ2 and τ3 can induce system oscillation alone, while the time delays τ1 and τ4 can not do this.
In the previous subsection, we separately investigated the effect of the time delays τ1, τ2, τ3 and τ4 on the dynamic behavior of the system. We found that, with the selected parameter values, adjusting the time delays τ2 and τ3 not only induces system oscillations, but also changes the amplitude and period of the oscillations. However, adjusting the time delays τ1 and τ4 in the selected parameter values did not induce system oscillations. In order to better study the influence of time delay on the system, we will study the influence of the combination of multiple time delays on the dynamic behavior of the system.
As shown in Figure 7(A), (C), the system is in a sustained oscillatory state when only the action of τ2 is considered. However, when considering the influence of the time delays τ1 and τ4, the state of the system does not change significantly, which indicates that the system is not sensitive to the time delays τ1 and τ4. As shown in Figure 7(B), the system is in a low amplitude oscillation state when only the action of the time delay τ2 is considered. But the system is in an oscillation state with a higher amplitude when the effect of the time delay τ3 is considered, and the amplitude increases with the increase of τ3, which indicates that the time delay τ3 plays a role in promoting the oscillation of the system. Figure 8 can further verify the above conclusion. In general, the relative magnitude of these four time delays may have an effect on the stability, period and amplitude of the system.
In the previous study, we found that the dephosphorylation rate of ATM by Wip1, the basic degradation rate of mdm2, the maximum production rate of wip1, the production rate of P53, the maximum production rate of Mdm2 and the maximum production rate of Wip1 could cause changes in the P53 concentration. At the same time, it is also mentioned in literature[16,17] that the parameters in the mathematical model have a great influence on system dynamics. In order to further understand the dynamics of the P53 system, we will study the dynamic behavior under the combinatorial effects of parameters and time delays. To achieve this, we fixed the time delay and selected different parameter values for numerical simulation.
As shown in Figure 9(A), with the increase of C, i.e., the production rate of P53, the system is always in a stable state. As can be seen in Figure 9(B), the system changes from a stable state to an oscillating state with the increase of the production rate of P53, which means that the system state can be changed by changing the value of the production rate of P53. Figure 9(C) reveals that when C=0.2, the system is in a stable state. With the increase of C, the state of the system changes from a stable state to a state of sustained oscillation, and its period and amplitude increase with the production rate of P53. In Figure 9(D), it can be seen that the system is always in a stable state when the production rate of P53 increases.
In Figure 10(A), (D), we can see that with the increase of dm, i.e., the basic degradation rate of mdm2, the system lies in a stable state. However, Figure 10(B), (C) indicate that as the basic degradation rate of mdm2 increases, the state of the system transitions from a steady state to an oscillating state. It can be seen in Figure 10 that the four time delays have some differences in regulating the oscillation dynamics of the system.
Figure 11 plots the time evolution diagram of P53 concentration when the time delay is combined with TM, i.e., the maximum production rate of Mdm2. As shown in Figure 11(A), (D), the state of the system did not change significantly with the increase of TM. However, in Figure 11(B), (C), we can see that as the maximum production rate of Mdm2 increases, the system changes from an oscillation state to a stable state. Therefore, we can regulate the state of the system by using parameters and time delay.
Physical, chemical and biological systems may be disturbed by the noise generated by the random birth and death of a single molecule or the fluctuation of biochemical reactions [23,38,44,45]. It can not only cause bistability but also oscillation and bifurcation [34,46]. These behaviors play an important role in biological systems [24,34,46]. In order to better understand the dynamics of the P53 model, we introduce random perturbations into the deterministic model to reveal richer and more complex dynamics. Various researchers have used a binomial τ-leap algorithm, Monte Carlo and other methods to study the properties of noise; it was found that the noise will affect the state of the system[35,47,48,49,50]. Here, we established stochastic models of P53-Wip1 and P53-Mdm2 networks and use the Wiener process to deal with the noise term, which focuses on the impact of noise and time delay on the system.
According to the randomness of DSB dynamics[7,51], we consider the influence of external noise on the interaction between ATM and P53; then, the system becomes as follows:
{ddtATM∗=A⋅ATM∗2(1+ATM∗2kA)⋅(1+Wip1(t−τ1)kWA)−dA⋅ATM∗−P⋅ATM∗⋅Wip1(t−τ1)+Smax⋅DSBγ+DSB+σ⋅ξ(t),ddtP53=C−dP⋅P53−g⋅Mdm2(t−τ2)⋅P53kMP+P53⋅(1+R1+ATM∗)+σ⋅ξ(t),ddtmdm2=Tm⋅P53(t−τ3)kPm+P53(t−τ3)−dm⋅mdm2,ddtMdm2=TM⋅mdm2−dM⋅Mdm2−dAM⋅ATM∗⋅Mdm2,ddtwip1=Tω⋅P53(t−τ4)kPω+P53(t−τ4)−dω⋅wip1,ddtWip1=TW⋅wip1−dW⋅Wip1, | (3.1) |
where ξ(t) is Gaussian white noise, which has the properties E[ξ(t)]=0 and E[ξ(t)ξ(t+ι)]=2σδ(ι) and σ is the noise intensity. Here, the influence of noise on the system is represented by the Wiener process. For the noise term, we mainly focus on noise intensity.
From the previous numerical simulation, it is known that all states of the molecules in the studied system are synchronous, which refers to the qualitative properties including oscillation, and that the steady state of the six molecules are concordant. In this paper, we mainly focus on the switch between oscillation and steady state. Therefore, to study if oscillations of the system occur, one only needs to discuss the oscillation dynamics of one of six molecules. In the following study, we only choose the variable P53 for numerical simulation. The time evolution of P53 under three different noise intensities is shown in Figure 12(A). We have found that the noise can not only cause the oscillation of the system but also induce the state switching without time delay. When the noise intensity σ=0, the concentration gradually approaches a certain stable value [24]. At a noise intensity σ=0.02, the system has a slight oscillation. Interestingly, oscillation with a large amplitude occurs when the noise intensity σ=0.12.
Figure 12(B) shows the phase plane diagram of the system (3.1) without time delay. When the noise intensity σ=0, the phase trajectory is represented by the magenta curve and the system is in a steady state. When the noise intensity σ=0.02, the motion track of the system is represented by a black curve. At this time, the track has obvious deviation, which was obviously caused by noise. When the noise intensity σ=0.12, the phase trajectory of the system is represented by the cyan curve, which shows that the system is in an oscillation state. Thus, Figure 12(B) further visually captured the phenomenon in Figure 12(A).
In order to further understand the dynamics of the P53 model, we will study the dynamics of P53 under the combined action of time delay and noise to reveal richer and more complex dynamics of P53. The time history diagram of P53 under the influence of a single time delay and noise is shown in Figure 13. Figure 13(A), (D) shows that when the time delay fails to induce system oscillation, adding noise with positive intensity to the system can cause the system to oscillate. In Figure 13(B), (C), we can see that the system is in a state of low amplitude oscillation under the action of a single time delay, while the system is in a state of high amplitude oscillation when noise with a positive intensity is added to the system. This indicates that noise can promote the oscillation of the system.
The time evolution process of P53 under the influence of multiple time delays and noise is shown in Figures 14–16. It can be seen in Figures 14(A), (B) and (D) that when σ=0, the system is in a low-amplitude sustained oscillation state. While σ=0.12, the system is in a state of sustained oscillation with high amplitude. This means that noise can enhance the oscillation of the system. Figure 14(C) shows that the system is in a stable state when σ=0, while the system is in an oscillation state when σ=0.12, which indicates that the noise can cause the system to oscillate.
From Figures 15 and 16, it is found that when σ=0, the system is in a low-amplitude oscillation state, and when the noise intensity increases to σ=0.12, the system is in a high-amplitude oscillation state, which indicates that the noise plays a role in promoting the oscillation under the action of multiple time delays. The above numerical results show that the introduction of noise into the system can not only cause system oscillation, but it can also enlarge its amplitude. The effect of noise on the dynamics of the P53 system has been studied in several studies [47,48,49]. In [47], the random behavior of the P53-Mdm2 network is described by using the Langevin equation; also, both the intrinsic noise and the extrinsic noise are considered. The Monte Carlo simulation results show that the two kinds of noises can cause system oscillation. In [48], random simulations using the binomial τ-leap algorithm showed that noise is critical to system oscillation. In [49], it is found that the noise will induce sustained oscillations with random excursions in the larger cycle corresponding to spikes. In [50], it is pointed out that the mechanism of oscillation induced by noise maybe occurs via a slowly varying Ornstein–Uhlenbeck process and three numerical examples are showed to explain it. The above articles introduce the methods of dealing with noise from different perspectives, and the focus of this paper is to pay attention to the impact of noise and time delay on the system. More specifically, time delay and noise can induce oscillation alone under certain parameter ranges. Although the emphasis is different, the results of these papers are consistent with that of this paper.
In recent years, a large number of studies have pointed out that P53 is mutated in cancer cells, which affects the fate of cancer cells. The P53 in normal cells can induce the repair or apoptosis of damaged cells, thereby inhibiting cancer, while the mutated P53 does not have such a function, which will lead to the occurrence of cancer. The important and interesting function of P53 is achieved by regulating the cell cycle process [1]. Due to the inherent complexity of biological systems and the importance of P53 in cell fate decisions, it is particularly important to consider the dynamic behavior of the transcription factor P53 under the influence of time delay and noise. In particular, M¨onke et al. [7] combined quantitative single cell data with methods from dynamical systems theory and proposed a network architecture and the corresponding mathematical model based on positive and negative feedback loops that reproduced experimentally measured P53 dynamics over a wide range of conditions.
In this paper, four time delays were introduced to the model proposed by M¨onke et al. [7]. Hopf bifurcation and numerical simulation of the model were used to study the dynamic characteristics of the P53 system under the influence of time delay and noise, including stability and bifurcation. First, we performed bifurcation analysis on the parameters P, dm, Tω, C, TW and TM in the original model proposed by M¨onke et al. [7], and the results showed that important parameters could induce P53 oscillation in an appropriate range. Second, the time delay was found to be ubiquitous in all kinds of systems. We studied the performance of the P53 system by analyzing the influence of time delay. By employing Hopf bifurcation theory, the stability of the system and the existence of Hopf bifurcation were studied by using time delay as the bifurcation parameter. It was found that time delay plays a key role in inducing Hopf bifurcation and adjusting the period and amplitude of oscillation. At the same time, it was also found that the combination of time delays can not only promote the oscillation of the system, but it also offers better robustness.
Then, we studied the influence of important parameters and time delay on the dynamic behavior of the system. The results showed that the P53 system changed from a stable state to an oscillating state with the increase of the production rate of P53 and the basal degradation rate of mdm2 under a certain time delay, which leads to cell cycle arrest and the repair of DNA damage. However, with the increase of the maximal production rate of Mdm2, the P53 system changes from an oscillating state to a stable state, which indicates that appropriately changing the parameter values can change the system state. In addition, due to the low molecular copy number and the fluctuation of the environment, we also considered the influence of noise on the system. Through numerical simulation, we found that the noise can change the state of the system without time delay. Under the action of a single or multiple time delays, the noise can not only change the state of the system, but it can also enlarge its amplitude.
In summary, τ2 and τ3 can induce P53 oscillations alone, while τ1 and τ4 cannot, but they can fine-tune the oscillations. τ2 refers to the time delay required for P53 degradation that is dependent on the transport of Mdm2, τ3 refers to the time delay required for Mdm2 protein synthesis, τ1 refers to the time delay required for dephosphorylation of the ATM∗ that is dependent on the transport of Wip1 and τ4 refers to the time delay required for Wip1 protein synthesis. This suggests that the P53-mdm2 core negative feedback loop plays an essential role in the induction of P53 oscillations, while the P53-Wip1-ATM negative feedback loop plays an auxiliary role [52,53,54]. Second, in the absence of time delay, noise can also cause P53 oscillations, which reveals that, when the DNA suffers a DSB, intracellular molecular movement is violent and noise is strengthened, thereby activating the P53 signaling pathway and initiating the program of repairing DNA damage [55,56]. In addition, important parameters involved in the system, such as the basic production rate of P53, the basic degradation rate of mdm2 RNA, and the maximum transcription rate of mdm2 RNA, can change the critical value of the Hopf bifurcation induced by time delay. This suggests that important parameters, time delay and noise, cooperate to influence the oscillatory behavior of the P53 signaling pathway. Biological facts show that Pp53 oscillations contribute to the repair of damaged cells, while high levels of P53 promote the apoptosis of irreparable cells or cancer cells, avoiding the transmission of damage information to the next generation of daughter cells. Therefore, we can modulate the dynamics of the P53 system by modulating these important parameters, time delays and noise, resulting in cell cycle arrest or apoptosis and thereby preventing tumor development.
The authors express gratitude to the anonymous referee for his/her helpful suggestions and the partial supports of the National Natural Science Foundation of China (12162032/12062027), the Scholarship for Academic Leader (2019HB015), Ten Thousand Talent Plans for Young Top-notch Talents, the Science and Technology Innovation Team about Modern Applied Mathematics and Systems Biology of Yunnan Education Department, and Applied Basic Research Foundation (LS21005) of Yunnan Province.
The authors declare that there is no conflict of interest.
The characteristic equation of System (2.1) expanded at the equilibrium point E=(x∗1,x∗2,x∗3,x∗4,x∗5,x∗6) is
λ6+k1λ5+k2λ4+k3λ3+k4λ2+k5λ+k6+(k7λ3+k8λ2+k9λ+k10)e−λτ2=0, | (A.1) |
where
k1=−a11−a22−a32−a43−a52−a62,k2=a11a22+a11a32+a22a32+a11a43+a22a43+a32a43+a11a52+a22a52+a32a52+a43a52+a11a62+a22a62+a32a62+a43a62+a52a62,k3=−a11a22a32−a11a22a43−a11a32a43−a22a32a43−a11a22a52−a11a32a52−a22a32a52−a11a43a52−a22a43a52−a32a43a52−a11a22a62−a11a32a62−a22a32a62−a11a43a62−a22a43a62−a32a43a62−a11a52a62−a22a52a62−a32a52a62−a43a52a62,k4=a11a22a32a43+a11a22a32a52+a11a22a43a52+a11a32a43a52+a22a32a43a52−a12a21a51a61+a11a22a32a62+a11a22a43a62+a11a32a43a62+a22a32a43a62+a11a22a52a62+a11a32a52a62+a22a32a52a62+a11a43a52a62+a22a43a52a62+a32a43a52a62,k5=−a11a22a32a43a52+a12a21a32a51a61+a12a21a43a51a61−a11a22a32a43a62−a11a22a32a52a62−a11a22a43a52a62−a11a32a43a52a62−a22a32a43a52a62,k6=−a12a21a32a43a51a61+a11a22a32a43a52a62,k7=−a23a31a42,k8=a11a23a31a42+a23a31a42a52+a23a31a42a62,k9=−a11a23a31a42a52−a12a23a41a51a61−a11a23a31a42a62−a23a31a42a52a62,k10=a12a23a32a41a51a61+a11a23a31a42a52a62, |
and
a11=−dA−Px∗6+(2Ax∗1k2AkWA)((x∗12+kA)2(kWA+x∗6)),a12=x∗1(−P−(Ax∗1kAkWA)((x∗12+kA)(kWA+x∗6)2)),a21=(gx∗4x∗2R)((1+x∗1)2(kMP+x∗2)),a22=−(((1+x∗1)dp(kMP+x∗2)2+gkMPx∗4(1+x∗1+R))((1+x∗1)(kMP+x∗2)2)),a23=−((gx∗2(1+x∗1+R))((1+x∗1)(kMP+x∗2))),a31=(kPmTm)(kPm+x∗2)2,a32=−dm, a41=−dAMx∗4, a42=TM,a43=−x∗1dAM−dM, a51=(kPω∗Tω)(kPω+x∗2)2,a52=−dω, a61=TW, a62=−dW. |
Assuming that ±ωi is the root of Eq (A.1), we can get
−ω6+ik1ω5+k2ω4−ik3ω3−k4ω2+ik5ω+k6+(−ik7ω3−k8ω2+ik9ω+k10)(cosωτ2−isinωτ2)=0. | (A.2) |
Separating the real and imaginary parts, we can get
{(k6−k4ω2+k2ω4−ω6)cos(τ2ω)+(−k5ω+k3ω3−k1ω5)sin(τ2ω)+k10−k8ω2=0,(k5ω−k3ω3+k1ω5)cos(τ2ω)+(k6−k4ω2+k2ω4−ω6)sin(τ2ω)+k9ω−k7ω3=0. | (A.3) |
Through calculation, the following equations are obtained
{cos(ωτ2)=−A11(k9ω−k7ω3)−A12(k10−k8ω2)A11(k5ω−k3ω3+k1ω5)−A212,sin(ωτ2)=−−k10k5+k6k9+A21ω2+A22ω4+A23A24ω2+A25ω4+A26ω6+A27ω8+A28, | (A.4) |
where
A11=(−k5ω+k3ω3−k1ω5),A12=(k6−k4ω2+k2ω4−ω6),A21=(k10k3−k6k7+k5k8−k4k9),A22=(−k1k10+k4k7−k3k8+k2k9),A23=(−k2k7+k1k8−k9)ω6+k7ω8,A24=(k25−2k4k6),A25=(k24−2k3k5+2k2k6),A26=(k23−2k2k4+2k1k5−2k6),A27=(k22−2k1k3+2k4),A28=k26+(k21−2k2)ω10+ω12. |
Noticing the formula cos(ωτ2)2+sin(ωτ2)2=1, we can get
H(ω)=h0+h1ω2+h2ω4+h3ω6+h4ω8+h5ω10+ω12=0, | (A.5) |
where
h0=−k210+k26,h1=k25−2k4k6+2k10k8−k29,h2=k24−2k3k5+2k2k6−k28+2k7k9,h3=k23−2(k2k4−k1k5+k6)−k27,h4=k22−2k1k3+2k4,h5=k21−2k2. |
Assume h5>0, h4>0, h3>0, h2>0 and h1>0. If h0<0, then Eq (7.5) has a unique positive root ω0, and the corresponding critical value of time delay is
τ02=1ω0arccos[−A11(k9ω0−k7ω30)−A12(k10−k8ω20)A11(k5ω0−k3ω30+k1ω50)−A211]. | (A.6) |
Therefore, when τ2=τ02, Eq (A.1) has a pair of pure imaginary roots iω0, and all other roots have negative real parts.
In addition, let λ(τ2)=υ(τ2)+iω(τ2) be the root of Eq (A.1), and let it satisfy υ(τ02)=0 and ω(τ02)=ω(0), we can get
[d(Reλ)dτ]∣τ=τ02>0. | (A.7) |
By Eq (A.1), the derivative of λ with respect to τ2 can be obtained
(dλdτ2)−1=(6λ5+5k1λ4+4k2λ3+3k3λ2+2k4λ+k5)eλτ2(k10λ+k9λ2+k8λ2+k7λ3)+(k9+2k8λ+3k7λ2)(k10λ+k9λ2+k8λ2+k7λ3)−τ2λ. | (A.8) |
So there is
(dReλ(τ2)dτ2)−1∣τ2=τ02=ω20H′(ω20)Δ, | (A.9) |
where
H′(ω20)=h1+2h2ω20+3h3ω40+4h4ω60+5h5ω80+6ω100,Δ=(k7ω40−k9ω20)2+(k10ω0−k8ω30)2. |
Given that h5>0, h4>0, h3>0, h2>0 and h1>0, H′(ω20)>0. At the same time, we have
sign(dReλ(τ2)dτ2)−1∣τ2=τ02=sign(dRe(λ)dτ)∣τ2=τ02>0. | (A.10) |
The results show that the P53-Mdm2-Wip1 network is asymptotically stable for 0≤τ2<τ02, but unstable for τ2>τ02 at the equilibrium point E∗. So, the system undergoes Hopf bifurcation when the time delay τ2=τ02. Therefore, it is very important to judge the critical value τ02 of the P53-Mdm2-Wip1 network.
[1] |
Y. Chen, P. Liu, Z. W. Yu, Study on degradation of macro performances and microstructure of concrete attacked by sulfate under artificial simulated environment, Constr. Build. Mater., 260 (2020), 119951. https://doi.org/10.1016/j.conbuildmat.2020.119951 doi: 10.1016/j.conbuildmat.2020.119951
![]() |
[2] |
N. Zhao, S. L. Wang, X. Y. Quan, F. Xu, K. N. Liu, Y. Liu, Behavior of polyvinyl alcohol fiber reinforced geopolymer composites under the coupled attack of sulfate and freeze-thaw in a marine environment, Ocean Eng., 238 (2021), 109734. https://doi.org/10.1016/j.oceaneng.2021.109734 doi: 10.1016/j.oceaneng.2021.109734
![]() |
[3] |
Y. Chen, J. F. Davalos, I. Ray, H. Y. Kim, Accelerated aging tests for evaluations of durability performance of FRP reinforcing bars for concrete structures, Compos. Struct., 78 (2007), 101–111. https://doi.org/10.1016/j.compstruct.2005.08.015 doi: 10.1016/j.compstruct.2005.08.015
![]() |
[4] |
A. E. Idiart, C. M. López, I. Carol, Chemo-mechanical analysis of concrete cracking and degradation due to external sulfate attack: A meso-scale model, Cem. Concr. Compos., 33 (2011), 411–423. https://doi.org/10.1016/j.cemconcomp.2010.12.001 doi: 10.1016/j.cemconcomp.2010.12.001
![]() |
[5] |
D. D. Sun, K. Wu, H. S. Shi, S. Miramini, L. H. Zhang, Deformation behaviour of concrete materials under the sulfate attack, Constr. Build. Mater., 210 (2019), 232–241. https://doi.org/10.1016/j.conbuildmat.2019.03.050 doi: 10.1016/j.conbuildmat.2019.03.050
![]() |
[6] |
J. Marchand, E. Samson, Y. Maltais, J. J. Beaudoin, Theoretical analysis of the effect of weak sodium sulfate solutions on the durability of concrete, Cem. Concr. Compos., 243 (2002), 317–329. https://doi.org/10.1016/S0958-9465(01)00083-X doi: 10.1016/S0958-9465(01)00083-X
![]() |
[7] |
R. Tixier, B. Mobasher, Modeling of damage in cement-based materials subjected to external sulfate attack, I: Formulation, J. Mater. Civ. Eng., 15 (2003), 305–313. https://doi.org/10.1061/(ASCE)0899-1561(2003)15:4(305) doi: 10.1061/(ASCE)0899-1561(2003)15:4(305)
![]() |
[8] |
R. Tixier, B. Mobasher, Modeling of damage in cement-based materials subjected to external sulfate attack, Ⅱ: Comparision with experiments, J. Mater. Civ. Eng., 15 (2003), 314–322. https://doi.org/10.1061/(ASCE)0899-1561(2003)15:4(314) doi: 10.1061/(ASCE)0899-1561(2003)15:4(314)
![]() |
[9] |
B. Bary, Simplified coupled chemo-mechanical modeling of cement pastes behavior subjected to combined leaching and external sulfate attack, Int. J. Numer. Anal. Meth. Geomech, 32 (2008), 1791–1816. https://doi.org/10.1002/nag.696| doi: 10.1002/nag.696
![]() |
[10] |
S. Sarkar, S. Mahadevan, J. C. L. Meeussen, H. van der Sloot, D. S. Kosson, Numerical simulation of cementitious materials degradation under external sulfate attack, Cem. Concr. Compos., 32 (2010), 241–252. https://doi.org/10.1016/j.cemconcomp.2009.12.005 doi: 10.1016/j.cemconcomp.2009.12.005
![]() |
[11] |
C. Sun, J. K. Chen, J. Zhu, M. H. Zhang, J. Ye, A new diffusion model of sulfate ions in concrete, Constr. Build. Mater., 39 (2013), 39–45. https://doi.org/10.1016/j.conbuildmat.2012.05.022 doi: 10.1016/j.conbuildmat.2012.05.022
![]() |
[12] |
T. Ikumi, S. H. P. Cavalaro, I. Segura, A. Fuente, A. Aguado, Simplified methodology to evaluate the external sulfate attack in concrete structures, Mater. Des., 89 (2016), 1147–1160. https://doi.org/10.1016/j.matdes.2015.10.084 doi: 10.1016/j.matdes.2015.10.084
![]() |
[13] |
D. D. Sun, K. Wu, H. S. Shi, L. T. Zhang, L. H. Zhang, Effect of interfacial transition zone on the transport of sulfate ions in concrete, Constr. Build. Mater., 192 (2018), 28–37. https://doi.org/10.1016/j.conbuildmat.2018.10.140 doi: 10.1016/j.conbuildmat.2018.10.140
![]() |
[14] |
Z. Chen, L. Y. Wu, V. Bindiganavile, C. F. Yi, Coupled models to describe the combined diffusion-reaction behaviour of chloride and sulphate ions in cement-based systems, Constr. Build. Mater., 243 (2020), 118232. https://doi.org/10.1016/j.conbuildmat.2020.118232 doi: 10.1016/j.conbuildmat.2020.118232
![]() |
[15] |
Y. W. Zhou, H. Tian, L. L. Sui, F. Xing, N. X. Han, Strength deterioration of concrete in sulfate environment: An experimental study and theoretical modeling, Adv. Mater. Sci. Eng., 2015 (2015), 1–13. https://doi.org/10.1155/2015/951209 doi: 10.1155/2015/951209
![]() |
[16] |
P. Liu, Y. Chen, Z. W. Yu, Z. H. Lu, Effect of sulfate solution concentration on the deterioration mechanism and physical properties of concrete, Constr. Build. Mater., 227 (2019), 116641. https://doi.org/10.1016/j.conbuildmat.2019.08.022 doi: 10.1016/j.conbuildmat.2019.08.022
![]() |
[17] |
S. W. Tang, Y. Yao, C. Andrade, Z. J. Li, Recent durability studies on concrete structure, Cem. Concr. Res., 78 (2015), 143–154. https://doi.org/10.1016/j.cemconres.2015.05.021 doi: 10.1016/j.cemconres.2015.05.021
![]() |
[18] |
M. Santhanam, M. Otieno, Deterioration of concrete in the marine environment, Marine Concr. Struct., (2016), 137–149. https://doi.org/10.1016/B978-0-08-100081-6.00005-2 doi: 10.1016/B978-0-08-100081-6.00005-2
![]() |
[19] |
A.M. Neville, Recovery of creep and observations on the mechanism of creep of concrete, Appl. Sci. Res., 9 (1960). https://doi.org/10.1007/BF00382191 doi: 10.1007/BF00382191
![]() |
[20] |
J. P. Li, F. Xie, G. W. Zhao, L. Li, Experimental and numerical investigation of cast-in-situ concrete under external sulfate attack and drying-wetting cycles, Constr. Build. Mater., 249 (2020), 118789. https://doi.org/10.1016/j.conbuildmat.2020.118789 doi: 10.1016/j.conbuildmat.2020.118789
![]() |
[21] |
P. J. Tumidajski, G. Chan, K. E. Philipose, An effective diffusivity for sulfate transport into concrete, Cem. Concr. Res., 25 (1995), 1159–1163. https://doi.org/10.1016/0008-8846(95)00108-O doi: 10.1016/0008-8846(95)00108-O
![]() |
[22] |
P. N. Gospodinov, Numerical simulation of 3D sulfate ion diffusion and liquid push out of the material capillaries in cement composites, Cem. Concr. Res., 35 (2005), 520–526. https://doi.org/10.1016/j.cemconres.2004.07.005 doi: 10.1016/j.cemconres.2004.07.005
![]() |
[23] |
G. J. Yin, X. B. Zuo, Y. J Tang, O. Ayinde, J. L. Wang, Numerical simulation on time-dependent mechanical behavior of concrete under coupled axial loading and sulfate attack, Ocean Eng., 142 (2017), 115–124. https://doi.org/10.1016/j.oceaneng.2017.07.016 doi: 10.1016/j.oceaneng.2017.07.016
![]() |
[24] |
W. Sun, X. B. Zuo, Numerical simulation of sulfate diffusivity in concrete under combination of mechanical loading and sulfate environments, J. Sustainable Cem.-Based Mater., 1 (2012), 46–55. https://doi.org/10.1080/21650373.2012.728564 doi: 10.1080/21650373.2012.728564
![]() |
[25] | J. Von Neumann, Theory of self-reproducing automata, University of Illinois press, Urbana, 1966. |
[26] |
S. Wolfram, Statistical mechanics of cellular automata, Rev. Mod. Phys., 55 (1983), 601–644. https://doi.org/10.1103/RevModPhys.55.601 doi: 10.1103/RevModPhys.55.601
![]() |
[27] |
A. Talaminos-Barroso, J. Reina-Tosina, L. M. Roa-Romero, Models based on cellular automata for the analysis of biomedical systems, Control Appl. Biomed. Eng. Syst., (2020), 405–445. https://doi.org/10.1016/B978-0-12-817461-6.00014-7 doi: 10.1016/B978-0-12-817461-6.00014-7
![]() |
[28] |
W. C. Huang, B. W. Zhou, Y. C. Yu, D. Z. Yin, Vulnerability analysis of road network for dangerous goods transportation considering intentional attack: Based on cellular automata, Reliab. Eng. Syst. Saf., 214 (2021), 107779. https://doi.org/10.1016/j.ress.2021.107779 doi: 10.1016/j.ress.2021.107779
![]() |
[29] |
S. Ghosh, S. Bhattacharya, Computational model on COVID-19 pandemic using probabilistic cellular automata, SN Comput. Sci., 230 (2021). https://doi.org/10.1007/s42979-021-00619-3 doi: 10.1007/s42979-021-00619-3
![]() |
[30] |
Y. Z. X. Liu, J. Q. Guo, J. Taplin, Y. B. Wang, Characteristic analysis of mixed traffic flow of regular and autonomous vehicles using cellular automata, J. Adv. Transp., 2017 (2017), 1–10. https://doi.org/10.1155/2017/8142074 doi: 10.1155/2017/8142074
![]() |
[31] |
K. Małecki, A computer simulation of traffic flow with on-street parking and drivers' behaviour based on cellular automata and a multi-agent system, J. Comput. Sci., 28 (2018), 32–42. https://doi.org/10.1016/j.jocs.2018.07.005 doi: 10.1016/j.jocs.2018.07.005
![]() |
[32] | F. Biondini, F. Bontempi, D. M. Frangopol, P. G. Malerba, Durability analysis of deteriorating concrete structures due to diffusion processes: Application to box-girder bridges, in Proceedings of the International Conference on Short and Medium Span Bridges, 2 (2002), 745–752. |
[33] |
F. Biondini, F. Bontempi, D. M. Frangopol, P. G. Malerba, Cellular automata approach to durability analysis of concrete structures in aggressive environments, J. Struct. Eng., 130 (2004), 1724–1737. https://doi.org/10.1061/(ASCE)0733-9445(2004)130:11(1724) doi: 10.1061/(ASCE)0733-9445(2004)130:11(1724)
![]() |
[34] | F. Biondini, F. Bontempi, D. M. Frangopol, A cellular automata finite element formulation for lifetime assessment of concrete structures. in Proceedings of the Structural Engineers World Congress, 2002. |
[35] |
F. Biondini, D. M. Frangopol, P. G. Malerba, Uncertainty effects on lifetime structural performance of cable-stayed bridges, Probabilistic Eng. Mech., 23 (2008). https://doi.org/10.1016/j.probengmech.2008.01.008 doi: 10.1016/j.probengmech.2008.01.008
![]() |
[36] | F. Biondini, D. M. Frangopol, Probabilistic life-cycle optimization of concrete structures, Engineering, 2008. |
[37] | J. Podroužek, Importance sampling strategy for oscillatory stochastic processes, in 5th International Conference on Reliable Engineering Computing, 2012. |
[38] |
F. Biondini, D. M. Frangopol, Probabilistic limit analysis and lifetime prediction of concrete structures, Struct. Infrastruct. Eng., 4 (2008), 399–412. https://doi.org/10.1080/15732470701270157 doi: 10.1080/15732470701270157
![]() |
[39] |
D. Vořechovská, J. Podroužek, M. Chromá, P. Rovnaníková, B. Teplý, Modeling of chloride concentration effect on reinforcement corrosion, Comput-Aided. Civ. Inf., 24 (2009), 446–458. https://doi.org/10.1111/j.1467-8667.2009.00602.x| doi: 10.1111/j.1467-8667.2009.00602.x
![]() |
[40] |
J. Cao, Y. F. Wang, K. P. Li, Y. S. Ma, Modeling the diffusion of chloride ion in concrete using cellular automaton, J. Mater. Civ. Eng., 24 (2012), 783–788. https://doi.org/10.1061/(ASCE)MT.1943-5533.0000440 doi: 10.1061/(ASCE)MT.1943-5533.0000440
![]() |
[41] |
F. Biondini, A. Palermo, G. Toniolo, Seismic performance of concrete structures exposed to corrosion: case studies of low-rise precast buildings, Struct. Infrastruct. Eng., 7 (2011), 109–119. https://doi.org/10.1080/15732471003588437 doi: 10.1080/15732471003588437
![]() |
[42] |
A. Titi, F. Biondini, Probabilistic seismic assessment of multistory precast concrete frames exposed to corrosion, Bull Earthquake Eng., 12 (2014), 2665–2681. https://doi.org/10.1007/s10518-014-9620-2 doi: 10.1007/s10518-014-9620-2
![]() |
[43] |
F. Biondini, E. Camnasio, A. Palermo, Lifetime seismic performance of concrete bridges exposed to corrosion. Struct. Infrastruct. Eng., 10 (2014), 880–900. https://doi.org/10.1080/15732479.2012.761248 doi: 10.1080/15732479.2012.761248
![]() |
[44] |
A. Titi, F. Biondini, On the accuracy of diffusion models for life-cycle assessment of concrete structures, Struct. Infrastruct. Eng., 12 (2015), 1202–1215. https://doi.org/10.1080/15732479.2015.1099110 doi: 10.1080/15732479.2015.1099110
![]() |
[45] |
X. Ruan, Y. Li, X. Y. Zhou, Z. R. Jin, Z. Y. Yin, Simulation method of concrete chloride ingress with mesoscopic cellular automata, Constr. Build. Mater., 249 (2020), 118778. https://doi.org/10.1016/j.conbuildmat.2020.118778 doi: 10.1016/j.conbuildmat.2020.118778
![]() |
[46] |
J. J. Ma, P. Z. Lin, Simulation approach for random diffusion of chloride in concrete under sustained load with cellular automata, Materials, 15 (2022), 4384. https://doi.org/10.3390/ma15134384 doi: 10.3390/ma15134384
![]() |
[47] |
M. L. Berndt, Properties of sustainable concrete containing fly ash, slag and recycled concrete aggregate, Constr. Build. Mater., 23 (2009), 2606–2613. https://doi.org/10.1016/j.conbuildmat.2009.02.011 doi: 10.1016/j.conbuildmat.2009.02.011
![]() |
[48] | Ministry of housing and urban-rural development of the People's Republic of China, Standard for Test Methods of Long-term Performance and Durability of Ordinary Concrete, China Architecture & Building Press, Beijing, 2009. |
[49] |
F. Biondini, A. Nero, Cellular finite beam element for nonlinear analysis of concrete structures under fire, J. Struct. Eng., 137 (2011). https://doi.org/10.1061/(ASCE)ST.1943-541X.0000307 doi: 10.1061/(ASCE)ST.1943-541X.0000307
![]() |
[50] | J. Podroužek, B. Teplý, Modelling of chloride transport inconcrete by cellular automata, Eng. Mech., 15 (2008), 213–222. |
[51] |
Q. Fu, Z. R. Zhang, Z. H. Wang, J. Q. He, D. T. Niu, Erosion behavior of ions in lining concrete incorporating fly ash and silica fume under the combined action of load and flowing groundwater containing composite salt, Case Stud. Constr. Mater., 17 (2022), e01659. https://doi.org/10.1016/j.cscm.2022.e01659 doi: 10.1016/j.cscm.2022.e01659
![]() |
[52] |
F. Liu, Z. P. You, Z. P. A. Diab, Z. Z. Liu, C. Zhang, S. C. Guo, External sulfate attack on concrete under combined effects of flexural fatigue loading and drying-wetting cycles, Constr. Build. Mater., 249 (2020), 118224. https://doi.org/10.1016/j.conbuildmat.2020.118224 doi: 10.1016/j.conbuildmat.2020.118224
![]() |
Parameter | Description | Value | Reference |
A | Maximum self-activation rate of ATM | 30.5 | [7] |
P | Dephosphorylation rate of ATM by Wip1 | 22 | [28] |
C | Production rate of P53 | 1.4 | [30] |
g | maximum degradation of P53 by Mdm2 | 2.5 | [30] |
dAM | Degradation rate of Mdm2 by ATM∗ | 20 | [28] |
Tm | Mmaximum production rate of mdm2 | 1 | [7] |
TM | Maximum production rate of Mdm2 | 4 | [7] |
Tω | Maximum production rate of wip1 | 1 | [7] |
TW | Maximum production rate of Wip1 | 1 | [7] |
dA | Basic dephosphorylation rate of ATM∗ | 0.16 | [7] |
dP | Basic degradation rate of P53 | 0.1 | [31] |
dm | Basic degradation rate of mdm2 | 1 | [7] |
dM | Basic degradation rate of Mdm2 | 2 | [30] |
dω | Basic degradation rate of wip1 | 1.3 | [7] |
dW | Basic degradation rate of Wip1 | 2.3 | [28] |
kA | Michaelis constant for the ATM∗ self activation | 0.5 | [7] |
kWA | Michaelis constant for the inhibition of the ATM∗ self-activation by Wip1 | 0.14 | [7] |
kMP | Michaelis constant of the degradation for P53 by Mdm2 | 0.15 | [30] |
kPm | Michaelis constant for the production of mdm2 by P53 | 1 | [7] |
kPω | Michaelis constant for the production of wip1 by P53 | 1 | [7] |
R | Strength of the inhibition of the Mdm2 mediated degradation of P53 by ATM∗ | 2 | [7] |
γ | Michaelis contant for the signal strength of the DSB process | 9 | [32] |
Smax | Maximum signal strength of the DSB process | 0.2 | [33] |
DSB | DNA damage in the form of DSBs | 0.1 | Estimate |
τ1 | Time delay needed in the dephosphorylation of ATM∗ depend on the transport of Wip1 | 0∼5 | Estimate |
τ2 | Time delay needed in the degradation of P53 depend on the transport of Mdm2 | 0∼5 | Estimate |
τ3 | Time delay needed in the Mdm2 protein synthesis | 0∼5 | Estimate |
τ4 | Time delay needed in the Wip1 protein synthesis | 0∼5 | Estimate |
Parameter | Description | Value | Reference |
A | Maximum self-activation rate of ATM | 30.5 | [7] |
P | Dephosphorylation rate of ATM by Wip1 | 22 | [28] |
C | Production rate of P53 | 1.4 | [30] |
g | maximum degradation of P53 by Mdm2 | 2.5 | [30] |
dAM | Degradation rate of Mdm2 by ATM∗ | 20 | [28] |
Tm | Mmaximum production rate of mdm2 | 1 | [7] |
TM | Maximum production rate of Mdm2 | 4 | [7] |
Tω | Maximum production rate of wip1 | 1 | [7] |
TW | Maximum production rate of Wip1 | 1 | [7] |
dA | Basic dephosphorylation rate of ATM∗ | 0.16 | [7] |
dP | Basic degradation rate of P53 | 0.1 | [31] |
dm | Basic degradation rate of mdm2 | 1 | [7] |
dM | Basic degradation rate of Mdm2 | 2 | [30] |
dω | Basic degradation rate of wip1 | 1.3 | [7] |
dW | Basic degradation rate of Wip1 | 2.3 | [28] |
kA | Michaelis constant for the ATM∗ self activation | 0.5 | [7] |
kWA | Michaelis constant for the inhibition of the ATM∗ self-activation by Wip1 | 0.14 | [7] |
kMP | Michaelis constant of the degradation for P53 by Mdm2 | 0.15 | [30] |
kPm | Michaelis constant for the production of mdm2 by P53 | 1 | [7] |
kPω | Michaelis constant for the production of wip1 by P53 | 1 | [7] |
R | Strength of the inhibition of the Mdm2 mediated degradation of P53 by ATM∗ | 2 | [7] |
γ | Michaelis contant for the signal strength of the DSB process | 9 | [32] |
Smax | Maximum signal strength of the DSB process | 0.2 | [33] |
DSB | DNA damage in the form of DSBs | 0.1 | Estimate |
τ1 | Time delay needed in the dephosphorylation of ATM∗ depend on the transport of Wip1 | 0∼5 | Estimate |
τ2 | Time delay needed in the degradation of P53 depend on the transport of Mdm2 | 0∼5 | Estimate |
τ3 | Time delay needed in the Mdm2 protein synthesis | 0∼5 | Estimate |
τ4 | Time delay needed in the Wip1 protein synthesis | 0∼5 | Estimate |