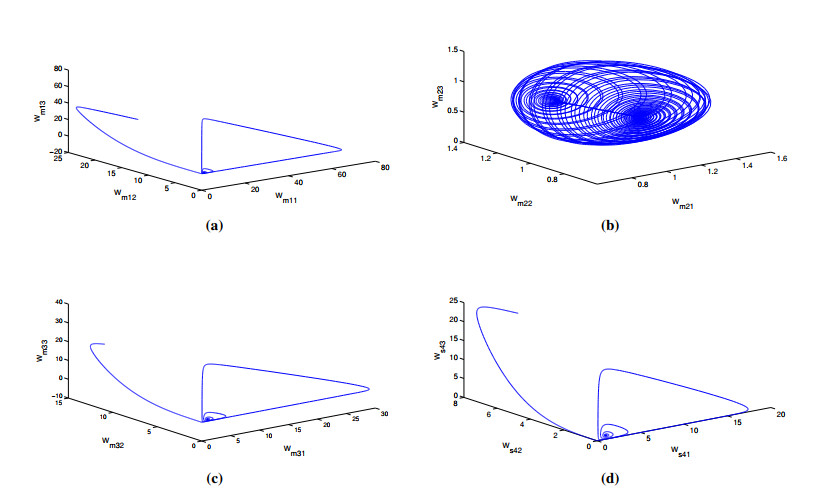
This work deals with a systematic approach for the investigation of compound difference anti-synchronization (CDAS) scheme among chaotic generalized Lotka-Volterra biological systems (GLVBSs). First, an active control strategy (ACS) of nonlinear type is described which is specifically based on Lyapunov's stability analysis (LSA) and master-slave framework. In addition, the biological control law having nonlinear expression is constructed for attaining asymptotic stability pattern for the error dynamics of the discussed GLVBSs. Also, simulation results through MATLAB environment are executed for illustrating the efficacy and correctness of considered CDAS approach. Remarkably, our attained analytical outcomes have been in outstanding conformity with the numerical outcomes. The investigated CDAS strategy has numerous significant applications to the fields of encryption and secure communication.
Citation: Harindri Chaudhary, Mohammad Sajid, Santosh Kaushik, Ali Allahem. Stability analysis of chaotic generalized Lotka-Volterra system via active compound difference anti-synchronization method[J]. Mathematical Biosciences and Engineering, 2023, 20(5): 9410-9422. doi: 10.3934/mbe.2023413
[1] | Xixia Ma, Rongsong Liu, Liming Cai . Stability of traveling wave solutions for a nonlocal Lotka-Volterra model. Mathematical Biosciences and Engineering, 2024, 21(1): 444-473. doi: 10.3934/mbe.2024020 |
[2] | S. Nakaoka, Y. Saito, Y. Takeuchi . Stability, delay, and chaotic behavior in a Lotka-Volterra predator-prey system. Mathematical Biosciences and Engineering, 2006, 3(1): 173-187. doi: 10.3934/mbe.2006.3.173 |
[3] | Pan Yang, Jianwen Feng, Xinchu Fu . Cluster collective behaviors via feedback pinning control induced by epidemic spread in a patchy population with dispersal. Mathematical Biosciences and Engineering, 2020, 17(5): 4718-4746. doi: 10.3934/mbe.2020259 |
[4] | Zigen Song, Jian Xu, Bin Zhen . Mixed-coexistence of periodic orbits and chaotic attractors in an inertial neural system with a nonmonotonic activation function. Mathematical Biosciences and Engineering, 2019, 16(6): 6406-6425. doi: 10.3934/mbe.2019320 |
[5] | Gayathri Vivekanandhan, Hamid Reza Abdolmohammadi, Hayder Natiq, Karthikeyan Rajagopal, Sajad Jafari, Hamidreza Namazi . Dynamic analysis of the discrete fractional-order Rulkov neuron map. Mathematical Biosciences and Engineering, 2023, 20(3): 4760-4781. doi: 10.3934/mbe.2023220 |
[6] | Paul Georgescu, Hong Zhang, Daniel Maxin . The global stability of coexisting equilibria for three models of mutualism. Mathematical Biosciences and Engineering, 2016, 13(1): 101-118. doi: 10.3934/mbe.2016.13.101 |
[7] | Biwen Li, Xuan Cheng . Synchronization analysis of coupled fractional-order neural networks with time-varying delays. Mathematical Biosciences and Engineering, 2023, 20(8): 14846-14865. doi: 10.3934/mbe.2023665 |
[8] | Yuanshi Wang, Donald L. DeAngelis . A mutualism-parasitism system modeling host and parasite with mutualism at low density. Mathematical Biosciences and Engineering, 2012, 9(2): 431-444. doi: 10.3934/mbe.2012.9.431 |
[9] | Xiaomeng Ma, Zhanbing Bai, Sujing Sun . Stability and bifurcation control for a fractional-order chemostat model with time delays and incommensurate orders. Mathematical Biosciences and Engineering, 2023, 20(1): 437-455. doi: 10.3934/mbe.2023020 |
[10] | Sai Zhang, Li Tang, Yan-Jun Liu . Formation deployment control of multi-agent systems modeled with PDE. Mathematical Biosciences and Engineering, 2022, 19(12): 13541-13559. doi: 10.3934/mbe.2022632 |
This work deals with a systematic approach for the investigation of compound difference anti-synchronization (CDAS) scheme among chaotic generalized Lotka-Volterra biological systems (GLVBSs). First, an active control strategy (ACS) of nonlinear type is described which is specifically based on Lyapunov's stability analysis (LSA) and master-slave framework. In addition, the biological control law having nonlinear expression is constructed for attaining asymptotic stability pattern for the error dynamics of the discussed GLVBSs. Also, simulation results through MATLAB environment are executed for illustrating the efficacy and correctness of considered CDAS approach. Remarkably, our attained analytical outcomes have been in outstanding conformity with the numerical outcomes. The investigated CDAS strategy has numerous significant applications to the fields of encryption and secure communication.
The constituent members in a system mainly found in nature can be interacting with each other through cooperation and competition. Demonstrations for such systems involve biological species, countries, businesses, and many more. It's very much intriguing to investigate in a comprehensive manner numerous social as well as biological interactions existent in dissimilar species/entities utilizing mathematical modeling. The predation and the competition species are the most famous interactions among all such types of interactions. Importantly, Lotka [1] and Volterra [2] in the 1920s have announced individually the classic equations portraying population dynamics. Such illustrious equations are notably described as predator-prey (PP) equations or Lotka-Volterra (LV) equations. In this structure, PP/LV model represents the most influential model for interacting populations. The interplay between prey and predator together with additional factors has been a prominent topic in mathematical ecology for a long period. Arneodo et al. [3] have established in 1980 that a generalized Lotka-Volterra biological system (GLVBS) would depict chaos phenomena in an ecosystem for some explicitly selected system parameters and initial conditions. Additionally, Samardzija and Greller [4] demonstrated in 1988 that GLVBS would procure chaotic reign from the stabled state via rising fractal torus. LV model was initially developed as a biological concept, yet it is utilized in enormous diversified branches for research [5,6,7,8]. Synchronization essentially is a methodology of having different chaotic systems (non-identical or identical) following exactly a similar trajectory, i.e., the dynamical attributes of the slave system are locked finally into the master system. Specifically, synchronization and control have a wide spectrum for applications in engineering and science, namely, secure communication [9], encryption [10,11], ecological model [12], robotics [13], neural network [14], etc. Recently, numerous types of secure communication approaches have been explored [15,16,17,18] such as chaos modulation [18,19,20,21], chaos shift keying [22,23] and chaos masking [9,17,20,24]. In chaos communication schemes, the typical key idea for transmitting a message through chaotic/hyperchaotic models is that a message signal is nested in the transmitter system/model which originates a chaotic/ disturbed signal. Afterwards, this disturbed signal has been emitted to the receiver through a universal channel. The message signal would finally be recovered by the receiver. A chaotic model has been intrinsically employed both as receiver and transmitter. Consequently, this area of chaotic synchronization & control has sought remarkable considerations among differential research fields.
Most prominently, synchronization theory has been in existence for over 30 years due to the phenomenal research of Pecora and Carroll [25] established in 1990 using drive-response/master-slave/leader-follower configuration. Consequently, many authors and researchers have started introducing and studying numerous control and synchronization methods [9,26,27,28,29,30,31,32,33,34,35,36] etc. to achieve stabilized chaotic systems for possessing stability. In [37], researchers discussed optimal synchronization issues in similar GLVBSs via optimal control methodology. In [38,39], the researchers studied the adaptive control method (ACM) to synchronize chaotic GLVBSs. Also, researchers [40] introduced a combination difference anti-synchronization scheme in similar chaotic GLVBSs via ACM. In addition, authors [41] investigated a combination synchronization scheme to control chaos existing in GLVBSs using active control strategy (ACS). Bai and Lonngren [42] first proposed ACS in 1997 for synchronizing and controlling chaos found in nonlinear dynamical systems. Furthermore, compound synchronization using ACS was first advocated by Sun et al. [43] in 2013. In [44], authors discussed compound difference anti-synchronization scheme in four chaotic systems out of which two chaotic systems are considered as GLVBSs using ACS and ACM along with applications in secure communications of chaos masking type in 2019. Some further research works [45,46] based on ACS have been reported in this direction. The considered chaotic GLVBS offers a generalization that allows higher-order biological terms. As a result, it may be of interest in cases where biological systems experience cataclysmic changes. Unfortunately, some species will be under competitive pressure in the coming years and decades. This work may be comprised as a step toward preserving as many currently living species as possible by using the proposed synchronization approach which is based on master-slave configuration and Lyapunov stability analysis.
In consideration of the aforementioned discussions and observations, our primary focus here is to develop a systematic approach for investigating compound difference anti-synchronization (CDAS) approach in 4 similar chaotic GLVBSs via ACS. The considered ACS is a very efficient yet theoretically rigorous approach for controlling chaos found in GLVBSs. Additionally, in view of widely known Lyapunov stability analysis (LSA) [47], we discuss actively designed biological control law & convergence for synchronization errors to attain CDAS synchronized states.
The major attributes for our proposed research in the present manuscript are:
● The proposed CDAS methodology considers four chaotic GLVBSs.
● It outlines a robust CDAS approach based active controller to achieve compound difference anti-synchronization in discussed GLVBSs & conducts oscillation in synchronization errors along with extremely fast convergence.
● The construction of the active control inputs has been executed in a much simplified fashion utilizing LSA & master-salve/ drive-response configuration.
● The proposed CDAS approach in four identical chaotic GLVBSs of integer order utilizing ACS has not yet been analyzed up to now. This depicts the novelty of our proposed research work.
This manuscript is outlined as follows: Section 2 presents the problem formulation of the CDAS scheme. Section 3 designs comprehensively the CDAS scheme using ACS. Section 4 consists of a few structural characteristics of considered GLVBS on which CDAS is investigated. Furthermore, the proper active controllers having nonlinear terms are designed to achieve the proposed CDAS strategy. Moreover, in view of Lyapunov's stability analysis (LSA), we have examined comprehensively the biological controlling laws for achieving global asymptotical stability of the error dynamics for the discussed model. In Section 5, numerical simulations through MATLAB are performed for the illustration of the efficacy and superiority of the given scheme. Lastly, we also have presented some conclusions and the future prospects of the discussed research work in Section 6.
We here formulate a methodology to examine compound difference anti-synchronization (CDAS) scheme viewing master-slave framework in four chaotic systems which would be utilized in the coming up sections.
Let the scaling master system be
˙wm1= f1(wm1), | (2.1) |
and the base second master systems be
˙wm2= f2(wm2), | (2.2) |
˙wm3= f3(wm3). | (2.3) |
Corresponding to the aforementioned master systems, let the slave system be
˙ws4= f4(ws4)+U(wm1,wm2,wm3,ws4), | (2.4) |
where wm1=(wm11,wm12,...,wm1n)T∈Rn, wm2=(wm21,wm22,...,wm2n)T∈Rn, wm3=(wm31,wm32,...,wm3n)T∈Rn, ws4=(ws41,ws42,...,ws4n)T∈Rn are the state variables of the respective chaotic systems (2.1)–(2.4), f1,f2,f3,f4:Rn→Rn are four continuous vector functions, U=(U1,U2,...,Un)T:Rn×Rn×Rn×Rn→Rn are appropriately constructed active controllers.
Compound difference anti-synchronization error (CDAS) is defined as
E=Sws4+Pwm1(Rwm3−Qwm2), |
where P=diag(p1,p2,.....,pn),Q=diag(q1,q2,.....,qn),R=diag(r1,r2,.....,rn),S=diag(s1,s2,.....,sn) and S≠0.
Definition: The master chaotic systems (2.1)–(2.3) are said to achieve CDAS with slave chaotic system (2.4) if
limt→∞‖E(t)‖=limt→∞‖Sws4(t)+Pwm1(t)(Rwm3(t)−Qwm2(t))‖=0. |
We now present our proposed CDAS approach in three master systems (2.1)–(2.3) and one slave system (2.4). We next construct the controllers based on CDAS approach by
Ui= ηisi−(f4)i−KiEisi, | (3.1) |
where ηi=pi(f1)i(riwm3i−qiwm2i)+piwm1i(ri(f3)i−qi(f2)i), for i=1,2,...,n.
Theorem: The systems (2.1)–(2.4) will attain the investigated CDAS approach globally and asymptotically if the active control functions are constructed in accordance with (3.1).
Proof. Considering the error as
Ei= siws4i+piwm1i(riwm3i−qiwm2i),fori=1,2,3,.....,n. |
Error dynamical system takes the form
˙Ei= si˙ws4i+pi˙wm1i(riwm3i−qiwm2i)+piwm1i(ri˙wm3i−qi˙wm2i)= si((f4)i+Ui)+pi(f1)i(riwm3i−qiwm2i)+piwm1i(ri(f3)i−qi(f2)i)= si((f4)i+Ui)+ηi, |
where ηi=pi(f1)i(riwm3i−qiwm2i)+piwm1i(ri(f3)i−qi(f2)i), i=1,2,3,....,n. This implies that
˙Ei= si((f4)i−ηisi−(f4)i−KiEisi)+ηi= −KiEi | (3.2) |
The classic Lyapunov function V(E(t)) is described by
V(E(t))= 12ETE= 12ΣE2i |
Differentiation of V(E(t)) gives
˙V(E(t))=ΣEi˙Ei |
Using Eq (3.2), one finds that
˙V(E(t))=ΣEi(−KiEi)= −ΣKiE2i). | (3.3) |
An appropriate selection of (K1,K1,.......,Kn) makes ˙V(E(t)) of eq (3.3), a negative definite. Consequently, by LSA [47], we obtain
limt→∞Ei(t)=0,(i=1,2,3). |
Hence, the master systems (2.1)–(2.3) and slave system (2.4) have attained desired CDAS strategy.
We now describe GLVBS as the scaling master system:
{˙wm11=wm11−wm11wm12+b3w2m11−b1w2m11wm13,˙wm12=−wm12+wm11wm12,˙wm13=b2wm13+b1w2m11wm13, | (4.1) |
where (wm11,wm12,wm13)T∈R3 is state vector of (4.1). Also, wm11 represents the prey population and wm12, wm13 denote the predator populations. For parameters b1=2.9851, b2=3, b3=2 and initial conditions (27.5,23.1,11.4), scaling master GLVBS displays chaotic/disturbed behaviour as depicted in Figure 1(a).
The base master systems are the identical chaotic GLVBSs prescribed respectively as:
{˙wm21=wm21−wm21wm22+b3w2m21−b1w2m21wm23,˙wm22=−wm22+wm21wm22,˙wm23=b2wm23+b1w2m21wm23, | (4.2) |
where (wm21,wm22,wm23)T∈R3 is state vector of (4.2). For parameter values b1=2.9851, b2=3, b3=2, this base master GLVBS shows chaotic/disturbed behaviour for initial conditions (1.2,1.2,1.2) as displayed in Figure 1(b).
{˙wm31=wm31−wm31wm32+b3w2m31−b1w2m31wm33,˙wm32=−wm32+wm31wm32,˙wm33=b2wm33+b1w2m31wm33, | (4.3) |
where (wm31,wm32,wm33)T∈R3 is state vector of (4.3). For parameters b1=2.9851, b2=3, b3=2, this second base master GLVBS displays chaotic/disturbed behaviour for initial conditions (2.9,12.8,20.3) as shown in Figure 1(c).
The slave system, represented by similar GLVBS, is presented by
{˙ws41=ws41−ws41ws42+b3w2s41−b1w2s41ws43+U1,˙ws42=−ws42+ws41ws42+U2,˙ws43=b2ws43+b1w2s41ws43+U3, | (4.4) |
where (ws41,ws42,ws43)T∈R3 is state vector of (4.4). For parameter values, b1=2.9851, b2=3, b3=2 and initial conditions (5.1,7.4,20.8), the slave GLVBS exhibits chaotic/disturbed behaviour as mentioned in Figure 1(d).
Moreover, the detailed theoretical study for (4.1)–(4.4) can be found in [4]. Further, U1, U2 and U3 are controllers to be determined.
Next, the CDAS technique has been discussed for synchronizing the states of chaotic GLVBS. Also, LSA-based ACS is explored & the necessary stability criterion is established.
Here, we assume P=diag(p1,p2,p3), Q=diag(q1,q2,q3), R=diag(r1,r2,r3), S=diag(s1,s2,s3). The scaling factors pi,qi,ri,si for i=1,2,3 are selected as required and can assume the same or different values.
The error functions (E1,E2,E3) are defined as:
{E1=s1ws41+p1wm11(r1wm31−q1wm21),E2=s2ws42+p2wm12(r2wm32−q2wm22),E3=s3ws43+p3wm13(r3wm33−q3wm23). | (4.5) |
The major objective of the given work is the designing of active control functions Ui,(i=1,2,3) ensuring that the error functions represented in (4.5) must satisfy
limt→∞Ei(t)=0for(i=1,2,3). |
Therefore, subsequent error dynamics become
{˙E1=s1˙ws41+p1˙wm11(r1wm31−q1wm21)+p1wm11(r1˙wm31−q1˙wm21),˙E2=s2˙ws42+p2˙wm12(r2wm32−q2wm22)+p2wm12(r2˙wm32−q2˙wm22),˙E3=s3˙ws43+p3˙wm13(r3wm33−q3wm23)+p3wm13(r3˙wm33−q3˙wm23). | (4.6) |
Using (4.1), (4.2), (4.3), and (4.5) in (4.6), the error dynamics simplifies to
{˙E1=s1(ws41−ws41ws42+b3w2s41−b1w2s41ws43+U1)+p1(wm11−wm11wm12+b3w2m11−b1w2m11wm13)(r1wm31−q1wm21)+p1wm11(r1(wm31−wm31wm32+b3w2m31−b1w2m31wm33)−q1(wm21−wm21wm22+b3w2m21−b1w2m21wm23),˙E2=s2(−ws42+ws41ws42+U2)+p2(−wm12+wm11wm12)(r2wm32−q2wm22)+p2wm12(r2(−wm32+wm31wm32)−q2(−wm22+wm21wm22)),˙E3=s3(b2ws43+b1w2s41ws43+U3)+p3(b2wm13+b1w2m11wm13)(r3wm33−q3wm23)+p3wm13(r3(b2wm33+b1w2m31wm33)−q3(b2wm23+b1w2m21wm23)). | (4.7) |
Let us now choose the active controllers:
U1= η1s1−(f4)1−K1E1s1, | (4.8) |
where η1=p1(f1)1(r1wm31−q1wm21)+p1wm11(r1(f3)1−q1(f2)1), as described in (3.1).
U2= η2s2−(f4)2−K2E2s2, | (4.9) |
where η2=p2(f1)2(r2wm32−q2wm22)+p2wm12(r2(f3)2−q2(f2)2).
U3= η3s3−(f4)3−K3E3s3, | (4.10) |
where η3=p3(f1)3(r3wm33−q3wm23)+p3wm13(r3(f3)3−q3(f2)3) and K1>0,K2>0,K3>0 are gaining constants.
By substituting the controllers (4.8), (4.9) and (4.10) in (4.7), we obtain
{˙E1=−K1E1,˙E2=−K2E2,˙E3=−K3E3. | (4.11) |
Lyapunov function V(E(t)) is now described by
V(E(t))= 12[E21+E22+E23]. | (4.12) |
Obviously, the Lyapunov function V(E(t)) is +ve definite in R3. Therefore, the derivative of V(E(t)) as given in (4.12) can be formulated as:
˙V(E(t))= E1˙E1+E2˙E2+E3˙E3. | (4.13) |
Using (4.11) in (4.13), one finds that
˙V(E(t))= −K1E21−K2E22−K3E23<0, |
which displays that ˙V(E(t)) is -ve definite.
In view of LSA [47], we, therefore, understand that CDAS error dynamics is globally as well as asymptotically stable, i.e., CDAS error E(t)→0 asymptotically for t→∞ to each initial value E(0)∈R3.
This section conducts a few simulation results for illustrating the efficacy of the investigated CDAS scheme in identical chaotic GLVBSs using ACS. We use 4th order Runge-Kutta algorithm for solving the considered ordinary differential equations. Initial conditions for three master systems (4.1)–(4.3) and slave system (4.4) are (27.5,23.1,11.4), (1.2,1.2,1.2), (2.9,12.8,20.3) and (14.5,3.4,10.1) respectively. We attain the CDAS technique among three masters (4.1)–(4.3) and corresponding one slave system (4.4) by taking pi=qi=ri=si=1, which implies that the slave system would be entirely anti-synchronized with the compound of three master models for i=1,2,3. In addition, the control gains (K1,K2,K3) are taken as 2. Also, Figure 2(a)–(c) indicates the CDAS synchronized trajectories of three master (4.1)–(4.3) & one slave system (4.4) respectively. Moreover, synchronization error functions (E1,E2,E3)=(51.85,275.36,238.54) approach 0 as t tends to infinity which is exhibited via Figure 2(d). Hence, the proposed CDAS strategy in three masters and one slave models/systems has been demonstrated computationally.
In this work, the investigated CDAS approach in similar four chaotic GLVBSs using ACS has been analyzed. Lyapunov's stability analysis has been used to construct proper active nonlinear controllers. The considered error system, on the evolution of time, converges to zero globally & asymptotically via our appropriately designed simple active controllers. Additionally, numerical simulations via MATLAB suggest that the newly described nonlinear control functions are immensely efficient in synchronizing the chaotic regime found in GLVBSs to fitting set points which exhibit the efficacy and supremacy of our proposed CDAS strategy. Exceptionally, both analytic theory and computational results are in complete agreement. Our proposed approach is simple yet analytically precise. The control and synchronization among the complex GLVBSs with the complex dynamical network would be an open research problem. Also, in this direction, we may extend the considered CDAS technique on chaotic systems that interfered with model uncertainties as well as external disturbances.
The authors gratefully acknowledge Qassim University, represented by the Deanship of Scientific Research, on the financial support for this research under the number 10163-qec-2020-1-3-I during the academic year 1441 AH/2020 AD.
The authors declare there is no conflict of interest.
[1] | A. J. Lotka, Elements of physical biology, Sci. Prog., 21 (1926), 341–343. |
[2] |
F. M. Scudo, Vito Volterra and theoretical ecology, Theor. Popul. Biol., 2 (1971), 1–23. https://doi.org/10.1016/0040-5809(71)90002-5 doi: 10.1016/0040-5809(71)90002-5
![]() |
[3] |
A. Arneodo, P. Coullet, C. Tresser, Occurence of strange attractors in three-dimensional Volterra equations, Phys. Lett. A, 79 (1980), 259–263. https://doi.org/10.1016/0375-9601(80)90342-4 doi: 10.1016/0375-9601(80)90342-4
![]() |
[4] |
N. Samardzija, L. D. Greller, Explosive route to chaos through a fractal torus in a generalized Lotka-Volterra model, Bull. Math. Biol., 50 (1988), 465–491. https://doi.org/10.1007/BF02458847 doi: 10.1007/BF02458847
![]() |
[5] |
P. Gatabazi, J. Mba, E. Pindza, C. Labuschagne, Grey Lotka-Volterra models with application to cryptocurrencies adoption, Chaos Solitons Fractals, 122 (2019), 47–57. https://doi.org/10.1016/j.chaos.2019.03.006 doi: 10.1016/j.chaos.2019.03.006
![]() |
[6] |
A. Hening, D. H. Nguyen, Stochastic Lotka-Volterra food chains, J. Math. Biol., 77 (2018), 135–163. https://doi.org/10.1007/s00285-017-1192-8 doi: 10.1007/s00285-017-1192-8
![]() |
[7] |
B. H. Tsai, C. J. Chang, C. H. Chang, Elucidating the consumption and Co2 emissions of fossil fuels and low-carbon energy in the united states using Lotka-Volterra models, Energy, 100 (2016), 416–424. ttps://doi.org/10.1016/j.energy.2015.12.045 doi: 10.1016/j.energy.2015.12.045
![]() |
[8] |
J. Xiong, X. Li, H. Wang, The survival analysis of a stochastic Lotka-Volterra competition model with a coexistence equilibrium, Math. Biosci., 16 (2019), 2717–2737. https://doi.org/10.3934/mbe.2019135 doi: 10.3934/mbe.2019135
![]() |
[9] |
A. Khan, U. Nigar, H. Chaudhary, Secure communication and synchronization dynamics in chaotic Chua's system via adaptive sliding mode control technique, Int. J. Appl. Comput. Math., 8 (2022), 1–20. https://doi.org/10.1007/s40819-022-01378-2 doi: 10.1007/s40819-022-01378-2
![]() |
[10] |
P. Muthukumar, Secure audio signal encryption based on triple compound-combination synchronization of fractional-order dynamical systems, Int. J. Dyn. Control, 10 (2022), 2053–2071. https://doi.org/10.1007/s40435-022-00942-4 doi: 10.1007/s40435-022-00942-4
![]() |
[11] |
X. J. Tong, M. Zhang, Z. Wang, Y. Liu, J. Ma, An image encryption scheme based on a new hyperchaotic finance system, Optik, 126 (2015), 2445–2452. https://doi.org/10.1016/j.ijleo.2015.06.018 doi: 10.1016/j.ijleo.2015.06.018
![]() |
[12] |
B. Sahoo, S. Poria, The chaos and control of a food chain model supplying additional food to top-predator, Chaos Solitons Fractals, 58 (2014), 52–64. https://doi.org/10.1016/j.chaos.2013.11.008 doi: 10.1016/j.chaos.2013.11.008
![]() |
[13] |
B. Patle, D. Parhi, A. Jagadeesh, S. K. Kashyap, Matrix-binary codes based genetic algorithm for path planning of mobile robot, Comput. Electr. Eng., 67 (2018), 708–728. https://doi.org/10.1016/j.compeleceng.2017.12.011 doi: 10.1016/j.compeleceng.2017.12.011
![]() |
[14] |
K. Bouallegue, A new class of neural networks and its applications, Neurocomputing, 249 (2017), 28–47. https://doi.org/10.1016/j.neucom.2017.03.006 doi: 10.1016/j.neucom.2017.03.006
![]() |
[15] |
K. Fallahi, H. Leung, A chaos secure communication scheme based on multiplication modulation, Commun. Nonlinear Sci. Numer. Simul., 15 (2010), 368–383. https://doi.org/10.1016/j.cnsns.2009.03.022 doi: 10.1016/j.cnsns.2009.03.022
![]() |
[16] |
J. He, J. Cai, J. Lin, Synchronization of hyperchaotic systems with multiple unknown parameters and its application in secure communication, Optik, 127 (2016), 2502–2508. https://doi.org/10.1016/j.ijleo.2015.11.055 doi: 10.1016/j.ijleo.2015.11.055
![]() |
[17] |
A. Khan, U. Nigar, Combination projective synchronization in fractional-order chaotic system with disturbance and uncertainty, Int. J. Appl. Comput. Math., 6 (2020), 1–22. https://doi.org/10.1007/s40819-019-0748-2 doi: 10.1007/s40819-019-0748-2
![]() |
[18] |
X. Wu, Z. Fu, J. Kurths, A secure communication scheme based generalized function projective synchronization of a new 5d hyperchaotic system, Phys. Scr., 90 (2015), 045210. https://doi.org/10.1088/0031-8949/90/4/045210 doi: 10.1088/0031-8949/90/4/045210
![]() |
[19] |
J. S. Lin, C. F. Huang, T. L. Liao, J. J. Yan, Design and implementation of digital secure communication based on synchronized chaotic systems, Digital Signal Process., 20 (2010), 229–237. https://doi.org/10.1016/j.dsp.2009.04.006 doi: 10.1016/j.dsp.2009.04.006
![]() |
[20] |
B. Naderi, H. Kheiri, Exponential synchronization of chaotic system and application in secure communication, Optik, 127 (2016), 2407–2412. https://doi.org/10.1016/j.ijleo.2015.11.175 doi: 10.1016/j.ijleo.2015.11.175
![]() |
[21] |
E. M. Ngouonkadi, H. Fotsin, P. L. Fotso, Implementing a memristive Van der pol oscillator coupled to a linear oscillator: synchronization and application to secure communication, Phys. Scr., 89 (2014), 035201. https://doi.org/10.1088/0031-8949/89/03/035201 doi: 10.1088/0031-8949/89/03/035201
![]() |
[22] |
H. Dedieu, M. P. Kennedy, M. Hasler, Chaos shift keying: modulation and demodulation of a chaotic carrier using self-synchronizing Chua's circuits, IEEE Trans. Circuits Syst. II, 40 (1993), 634–642. https://doi.org/10.1109/81.222795 doi: 10.1109/81.222795
![]() |
[23] |
Y. Y. Hou, H. C. Chen, J. F. Chang, J. J. Yan, T. L. Liao, Design and implementation of the sprott chaotic secure digital communication systems, Appl. Math. Comput., 218 (2012), 11799–11805. https://doi.org/10.1016/j.amc.2012.04.076 doi: 10.1016/j.amc.2012.04.076
![]() |
[24] |
X. Wu, H. Wang and H. Lu, Modified generalized projective synchronization of a new fractional-order hyperchaotic system and its application to secure communication, Nonlinear Anal. Real World Appl., 13 (2012), 1441–1450. https://doi.org/10.1016/j.nonrwa.2011.11.008 doi: 10.1016/j.nonrwa.2011.11.008
![]() |
[25] |
L. M. Pecora, T. L. Carroll, Synchronization in chaotic systems, Phys. Rev. Lett., 64 (1990), 821. https://doi.org/10.1103/PhysRevLett.64.821 doi: 10.1103/PhysRevLett.64.821
![]() |
[26] |
A. Khan, N. Khan, H. Chaudhary, U. Nigar, Analysis and control of complex variable hyper-chaotic robinovich system with fractional derivative, Int. J. Appl. Comput. Math., 8 (2022), 1–23. https://doi.org/10.1007/s40819-022-01463-6 doi: 10.1007/s40819-022-01463-6
![]() |
[27] |
J. Ma, L. Mi, P. Zhou, Y. Xu, T. Hayat, Phase synchronization between two neurons induced by coupling of electromagnetic field, Appl. Math. Comput., 307 (2017), 321–328. https://doi.org/10.1016/j.amc.2017.03.002 doi: 10.1016/j.amc.2017.03.002
![]() |
[28] |
D. Li, X. Zhang, Impulsive synchronization of fractional order chaotic systems with time-delay, Neurocomputing, 216 (2016), 39–44. https://doi.org/10.1016/j.neucom.2016.07.013 doi: 10.1016/j.neucom.2016.07.013
![]() |
[29] |
H. Qiu, X. Xu, Z. Jiang, K. Sun, C. Cao, Dynamical behaviors, circuit design, and synchronization of a novel symmetric chaotic system with coexisting attractors, Sci. Rep., 13 (2023), 1893. https://doi.org/10.1038/s41598-023-28509-z doi: 10.1038/s41598-023-28509-z
![]() |
[30] |
S. Vaidyanathan, S. Sampath, Anti-synchronization of four-wing chaotic systems via sliding mode control, Int. J. Autom. Comput., 9 (2012), 274–279. https://doi.org/10.1007/s11633-012-0644-2 doi: 10.1007/s11633-012-0644-2
![]() |
[31] | Y. Wang, C. Sun, Z. Wang, J. Sun, Projection synchronization of three-dimensional chaotic systems with active control based on dna strand displacement, IEEE Trans. NanoBioscience, 2023 (2023). https://doi.org/10.1109/TNB.2023.3241652 |
[32] |
V. K. Yadav, G. Prasad, M. Srivastava, S. Das, Triple compound synchronization among eight chaotic systems with external disturbances via nonlinear approach, Differ. Equations Dyn. Syst., 2019 (2019), 1–24. https://doi.org/10.1080/2058802X.2019.1674539 doi: 10.1080/2058802X.2019.1674539
![]() |
[33] |
S. H. Yuningsih, E. Rusyaman, S. Vaidyanathan, A. Sambas, Investigation of chaos behavior and integral sliding mode control on financial risk model, AIMS Math., 7 (2022), 18377–18392. https://doi.org/10.3934/math.20221012 doi: 10.3934/math.20221012
![]() |
[34] |
R. Zhang, X. Xi, H. Tian, Z. Wang, Dynamical analysis and finite-time synchronization for a chaotic system with hidden attractor and surface equilibrium, Axioms, 11 (2022), 579. https://doi.org/10.3390/axioms11110579 doi: 10.3390/axioms11110579
![]() |
[35] |
H. Chaudhary, A. Khan, U. Nigar, S. Kaushik, M. Sajid, An effective synchronization approach to stability analysis for chaotic generalized Lotka-Volterra biological models using active and parameter identification methods, Entropy, 24 (2022), 529. https://doi.org/10.3390/e24040529 doi: 10.3390/e24040529
![]() |
[36] |
H. Chaudhary, A. Khan, M. Sajid, An investigation on microscopic chaos controlling of identical chemical reactor system via adaptive controlled hybrid projective synchronization, Eur. Phys. J. Spec. Top., 231 (2022), 453–463. https://doi.org/10.1140/epjs/s11734-021-00404-6 doi: 10.1140/epjs/s11734-021-00404-6
![]() |
[37] |
A. El-Gohary, M. Yassen, Optimal control and synchronization of Lotka-Volterra model, Chaos Solitons Fractals, 12 (2001), 2087–2093. https://doi.org/10.1016/S0960-0779(00)00023-0 doi: 10.1016/S0960-0779(00)00023-0
![]() |
[38] | S. Vaidyanathan, Hybrid synchronization of the generalized Lotka-Volterra three-species biological systems via adaptive control, Int. J. PharmTech Res., 9 (2016), 179–192. |
[39] | S. Vaidyanathan, Adaptive biological control of generalized Lotka-Volterra three-species biological system, Int. J. PharmTech Res., 8 (2015), 622–631. |
[40] |
T. Khan, H. Chaudhary, Estimation and identifiability of parameters for generalized Lotka-Volterra biological systems using adaptive controlled combination difference anti-synchronization, Differ. Equations Dyn. Syst., 28 (2020), 515–526. https://doi.org/10.1007/s12591-020-00534-8 doi: 10.1007/s12591-020-00534-8
![]() |
[41] |
T. Khan, H. Chaudhary, Controlling and synchronizing combined effect of chaos generated in generalized Lotka-Volterra three species biological model using active control design, Appl. Appl. Math., 15 (2020), 25. https://doi.org/10.12968/nuwa.2020.15.25 doi: 10.12968/nuwa.2020.15.25
![]() |
[42] |
E. W. Bai, K. E. Lonngren, Synchronization of two Lorenz systems using active control, Chaos Solitons Fractals, 8 (1997), 51–58. https://doi.org/10.1016/S0960-0779(96)00060-4 doi: 10.1016/S0960-0779(96)00060-4
![]() |
[43] |
J. Sun, Y. Shen, Q. Yin, C. Xu, Compound synchronization of four memristor chaotic oscillator systems and secure communication, Chaos Interdiscip. J. Nonlinear Sci., 23 (2013), 013140. https://doi.org/10.1063/1.4794794 doi: 10.1063/1.4794794
![]() |
[44] |
A. Khan, P. Trikha, Compound difference anti-synchronization between chaotic systems of integer and fractional order, SN Appl. Sci., 757 (2019), 1–13. https://doi.org/10.1007/s42452-019-0776-x doi: 10.1007/s42452-019-0776-x
![]() |
[45] |
K. Ojo, A. Njah, O. Olusola, Compound-combination synchronization of chaos in identical and different orders chaotic systems, Arch. Control Sci., 25 (2015), 463–490. https://doi.org/10.1515/acsc-2015-0030 doi: 10.1515/acsc-2015-0030
![]() |
[46] |
J. Sun, Y. Wang, Y. Wang, G. Cui, Y. Shen, Compound-combination synchronization of five chaotic systems via nonlinear control, Optik, 127 (2016), 4136–4143. https://doi.org/10.1016/j.ijleo.2016.01.018 doi: 10.1016/j.ijleo.2016.01.018
![]() |
[47] | L. Perko, Differential Equations and Dynamical Systems, Springer Science & Business Media, 2013. |
1. | Cheng-Jun 成俊 Xie 解, Xiang-Qing 向清 Lu 卢, Finite time hybrid synchronization of heterogeneous duplex complex networks via time-varying intermittent control, 2025, 34, 1674-1056, 040601, 10.1088/1674-1056/adacc6 |