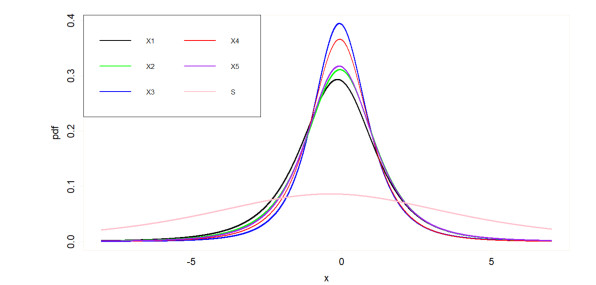
The automatic evaluation of the teaching effect has been a technical problem for many years. Because only video frames are available for it, and the information extraction from such dynamic scenes still remains challenging. In recent years, the progress of deep learning has boosted the application of computer vision in many areas, which can provide much insight into the above issue. As a consequence, this paper proposes a vision sensing-based automatic evaluation method for teaching effects based on deep residual network (DRN). The DRN is utilized to construct a backbone network for sensing from visual features such as attending status, taking notes, playing phones, looking outside, etc. The extracted visual features are further selected as the basis for the evaluation of the teaching effect. We have also collected some realistic course images to establish a real-world dataset for the performance assessment of the proposal. The proposed method is implemented on collected datasets via computer programming-based simulation experiments, so as to obtain accuracy assessment results as measurement. The obtained results show that the proposal can well perceive typical visual features from video frames of courses and realize automatic evaluation of the teaching effect.
Citation: Meijuan Sun. A Vision sensing-based automatic evaluation method for teaching effect based on deep residual network[J]. Mathematical Biosciences and Engineering, 2023, 20(4): 6358-6373. doi: 10.3934/mbe.2023275
[1] | Baishuai Zuo, Chuancun Yin . Stein’s lemma for truncated generalized skew-elliptical random vectors. AIMS Mathematics, 2020, 5(4): 3423-3433. doi: 10.3934/math.2020221 |
[2] | Guangshuai Zhou, Chuancun Yin . Family of extended mean mixtures of multivariate normal distributions: Properties, inference and applications. AIMS Mathematics, 2022, 7(7): 12390-12414. doi: 10.3934/math.2022688 |
[3] | Remigijus Leipus, Jonas Šiaulys, Dimitrios Konstantinides . Minimum of heavy-tailed random variables is not heavy tailed. AIMS Mathematics, 2023, 8(6): 13066-13072. doi: 10.3934/math.2023658 |
[4] | Naif Alotaibi, A. S. Al-Moisheer, Ibrahim Elbatal, Salem A. Alyami, Ahmed M. Gemeay, Ehab M. Almetwally . Bivariate step-stress accelerated life test for a new three-parameter model under progressive censored schemes with application in medical. AIMS Mathematics, 2024, 9(2): 3521-3558. doi: 10.3934/math.2024173 |
[5] | Huifang Yuan, Tao Jiang, Min Xiao . The ruin probability of a discrete risk model with unilateral linear dependent claims. AIMS Mathematics, 2024, 9(4): 9785-9807. doi: 10.3934/math.2024479 |
[6] | Weiwei Ni, Chenghao Xu, Kaiyong Wang . Estimations for aggregate amount of claims in a risk model with arbitrary dependence between claim sizes and inter-arrival times. AIMS Mathematics, 2022, 7(10): 17737-17746. doi: 10.3934/math.2022976 |
[7] | Khaled M. Alqahtani, Mahmoud El-Morshedy, Hend S. Shahen, Mohamed S. Eliwa . A discrete extension of the Burr-Hatke distribution: Generalized hypergeometric functions, different inference techniques, simulation ranking with modeling and analysis of sustainable count data. AIMS Mathematics, 2024, 9(4): 9394-9418. doi: 10.3934/math.2024458 |
[8] | Gunduz Caginalp . Fat tails arise endogenously from supply/demand, with or without jump processes. AIMS Mathematics, 2021, 6(5): 4811-4846. doi: 10.3934/math.2021283 |
[9] | Hamid Reza Safaeyan, Karim Zare, Mohamadreza Mahmoudi, Mohsen Maleki, Amir Mosavi . A Bayesian approach on asymmetric heavy tailed mixture of factor analyzer. AIMS Mathematics, 2024, 9(6): 15837-15856. doi: 10.3934/math.2024765 |
[10] | Yanfang Zhang, Fuchang Wang, Yibin Zhao . Statistical characteristics of earthquake magnitude based on the composite model. AIMS Mathematics, 2024, 9(1): 607-624. doi: 10.3934/math.2024032 |
The automatic evaluation of the teaching effect has been a technical problem for many years. Because only video frames are available for it, and the information extraction from such dynamic scenes still remains challenging. In recent years, the progress of deep learning has boosted the application of computer vision in many areas, which can provide much insight into the above issue. As a consequence, this paper proposes a vision sensing-based automatic evaluation method for teaching effects based on deep residual network (DRN). The DRN is utilized to construct a backbone network for sensing from visual features such as attending status, taking notes, playing phones, looking outside, etc. The extracted visual features are further selected as the basis for the evaluation of the teaching effect. We have also collected some realistic course images to establish a real-world dataset for the performance assessment of the proposal. The proposed method is implemented on collected datasets via computer programming-based simulation experiments, so as to obtain accuracy assessment results as measurement. The obtained results show that the proposal can well perceive typical visual features from video frames of courses and realize automatic evaluation of the teaching effect.
One of the main challenges faced by financial companies is to evaluate market risks in a set of changes of the basic variables such as stock prices, interest rates or exchange rates. In this regard the Value-at-Risk (VaR) introduced by J. P. Morgan in the mid 1990s has become a standard risk measure of financial market risk. Despite its extensive use, the VaR is not a coherent risk measure because it fails to satisfy subadditivity property (see [1]). The VaR can not determine the expected loss of portfolio in q worst case, but it defines the minimum loss. Furthermore, the computation of the VaR is based on the assumption that financial data returns follow the normal distribution. However, as shown in the literatures, the underlying distributions of many financial data exhibit skewness, non-symmetric, heavy tails and excess kurtosis (see [10]). They suggest in particular that large losses occur with much higher probability than the normal distribution would suggest.
The tail conditional expectation (TCE) risk measure shares properties that are considered desirable in all cases. For instance, due to the additivity of expectations, TCE allows venture capital to decompose naturally among its various components.
Consider X to be a loss random variable whose cumulative distribution function (cdf) is denoted by FX(x). The TCE is defined as
TCEp(X)=E(X|X>xp),p∈(0,1), |
where xp=inf{x∈R:FX(x)≥p}=VaRp(X). The TCE has been discussed in many literatures (see e.g., [11,12,15,17]).
The tail conditional expectation risk measure shares properties that are considered desirable in a variety of situations. For instance, due to the additivity of expectations, TCE allows for a natural decomposition of risk capital among its various constituents. The conception of capital allocation principle has long been introduced, in which the capital allocated to each risk unit can be expressed as its contribution to the tail conditional expectation of total risk. Risk allocation can not only help to evaluate and compare the performance of individual risk units, but also help to understand the risk contribution of each unit towards the total risk of the portfolio. Landsman and Valdez [17] derived the portfolio risk decomposition with TCE for the multivariate elliptical distribution. In [18], authors derived the portfolio risk decomposition with TCE for the exponential dispersion model, and Kim [14] for the exponential family class. The allocation for the class of exponential marginal was developed in []. The portfolio risk decomposition with TCE was further considered in [19] for the skew-normal distribution. Furman and Landsman [16] for the multivariate Gamma distribution. Cai and Li [2] for the phase-type distribution. Goovaerts et al. [9] and Chiragiev and Landsman [4] have provided the TCE-capital allocation for the multivariate Pareto distribution while Cossette et al. [5] have considered multivariate compound distribution. Ignatieva and Landsman [12] for generalized hyperbolic distribution. Recently, Kim and Kim [15] and Ignatieva and Landsman [12] investigated the TCE allocation for the family of multivariate normal mean-variance mixture distributions and skewed generalized hyperbolic, respectively. The univariate TCE and risk allocation formula for the generalized hyper-elliptical class were available in [13].
Furman and Landsman [8] observed that in many cases the TCE does not provide adequate information about the risks on the right tail. This point can be confirmed by the fact that the TCE does not include the information that the risk deviates from the upper tail expectation. Furman and Landsman [8] introduced the tail variance measure. The tail variance is defined as
TVp(X)=Var(X|X>xp)=E((X−TCEp(X))2|X>xp), |
and it has been discussed in many literatures (see e.g., [8,15]).
In this paper we consider a class of multivariate location-scale mixtures of elliptical (LSME) distributions which is known to be extremely flexible and contains many special cases as its members. Examples include the generalized hyper-elliptical distribution and generalized hyperbolic distribution.
The rest of the paper is organized as follows. Section 2 reviews the definition and properties of the multivariate LSME class, and introduces the generalized hyper-elliptical distribution as a representative subclass. Section 3 presents a theorem and proves the proposed TCE formula for the LSME and in Section 4, the development is extended to the portfolio risk decomposition with TCE for the multivariate LSME. In Section 5, we develop TV formula for univariate LSME. Section 6 deals with the special case of generalized hyperbolic distribution. Numercial illustration is presented in Section 7. Finally, concluding remarks are presented in Section 8.
In this section, we introduce the class of location-scale mixtures of elliptical distributions and some of its properties.
Let Ψn be a class of functions ψ(t):[0,∞)→R such that function ψ(∑ni=1t2i) is an n-dimensional characteristic function.
A random vector Y is said to have a multivariate elliptical distribution, denoted by Y∼En(μ,Σ,ψ), if its characteristic function can be expressed as
φY(t)=exp(itTμ)ψ(12tTΣt), | (2.1) |
for column-vector vector μ, n×n positive definite scale matrix Σ, and for function ψ(t)∈Ψn, which is called the characteristic generator.
In general, a multivariate elliptical distribution may not have a probability density function (pdf), but if its pdf exists then the form will be
fY(y)=cn√|Σ|gn[12(y−μ)TΣ−1(y−μ)], | (2.2) |
for function gn(⋅), which is called the density generator. The condition
∫∞0un2−1gn(u)du<∞ | (2.3) |
guarantees gn(u) to be the density generator ([7]). In addition, the normalizing constant cn is
cn=Γ(n2)(2π)n2(∫∞0un2−1gn(u)du)−1. |
Similarly, the elliptical distribution can also be introduced by the density generator and then written Y∼En(μ,Σ,gn).
From (2.1), it follows that, if Y∼En(μ,Σ,gn) and A is m×n matrix of rank m≤n and b is m-dimensional column-vector, then
AY+b∼Em(Aμ+b,AΣAT,gm). |
The following condition:
∫∞0g1(u)du<∞ |
guarantees the existence of the mean. If, in addition, |ψ′(0)|<∞, the covariance matrix exists and is equal to
Cov(Y)=−ψ′(0)Σ, |
(see [3]).
From (2.2) and (2.3), g1(x) can be a density generator of univariate elliptical distribution of the random variable Y∼E1(μ,σ2,g1) whose pdf can be expressed as
fY(y)=cσg1(12(y−μσ)2), |
where c is the normalizing constant. In this paper, we assume
Var(Z)=σ2Z<∞, | (2.4) |
where Z=Y−μσ is the spherical random variable. The cdf of the random variable Z can be written as the following integration form:
FZ(z)=c∫z−∞g1(12u2)du. |
We can obtain the mean and variance of Z:
μZ=0 |
and
σ2Z=2c∫∞0u2g1(12u2)du=−ψ′(0). |
Landsman and Valdez [17] showed that
fZ∗(z)=1σ2Z¯G(12z2) | (2.5) |
is the density of another spherical random variable Z∗ associated with Z, where
¯G(z)=∫∞zg1(u)du. |
The random vector X∼ LSME n(μ,Σ,γ,gn;Π) has an n-dimensional LSME distribution with location parameter μ, positive definite scale matrix Σ, if
X=m(Θ)+Θ12Σ12Y, | (2.6) |
in distribution, where
(1) Y∼En(0,In,gn), the n-dimensional multivariate elliptical variable;
(2) Non-negative scalar random variable Θ is independent of Y, whose pdf and cdf are π(θ) and Π(θ) respectively;
(3) m(Θ)=μ+Θγ, where μ=(μ1,…,μn)Tandγ=(γ1,…,γn)T are constant vectors in Rn.
The pdf of the LSME can be written as the following integration form:
fX(x)=cn√|Σ|∫∞01√θgn(12θ(x−μ−θγ)TΣ−1(x−μ−θγ))π(θ)dθ,x∈Rn. |
We find that the conditional distribution of X|θ is elliptical, that is
X|Θ=θ∼En(m(θ),θΣ,gn). | (2.7) |
We can obtain the mean and covariance of X:
E(X)=E[E(X|Θ)]=E(m(Θ))=μ+E(Θ)γ |
and
Cov(X)=E[Var(X|Θ)]+Var[E(X|Θ)]=E(ΘΣ)+Var(m(Θ))=E(Θ)Σ+Var(Θ)γγT. |
The characteristic function of X|Θ=θ exists and equals to
φX(t|Θ=θ)=exp(itTμ)exp(iθtTγ)ψ(12θtTΣt). |
Then the characteristic function of the LSME-distributed random vector X can be written as
φX(t)=exp(itTμ)E[exp(iθtTγ)ψ(12θtTΣt)]=exp(itTμ)∫∞0exp(iθtTγ)ψ(12θtTΣt)π(θ)dθ. | (2.8) |
Under the condition (2.2) and from (2.5), we can conclude ¯G(z) is the density generator of the associated elliptical variable Z∗, then
X∗=m(Θ)+√ΘσZ∗ | (2.9) |
is said to have a univariate LSME distributions, denoted by X∗∼LSME1(μ,σ2,γ,Θ,¯G;Π).
Proposition 1. If X∼LSMEn(μ,Σ,γ,gn;Π) and Y=BX+b where B is m×n (m≤n) matrix and b is m-dimensional column-vector, then it holds that Y∼LSMEm(Bμ+b,BΣBT,Bγ,gm;Π).
Proof. Using the characteristic function (2.8), we write
φY(t)=E(eitT(BX+b))=exp(itTb)φX(BTt)=exp(itTb)exp(i(BTt)Tμ)∫∞0exp(iθ(BTt)Tγ)ψ(12θ(BTt)TΣ(BTt))π(θ)dθ=exp(itT(Bμ+b))∫∞0exp(iθtTBγ)ψ(12θtTBΣBTt)π(θ)dθ, | (2.10) |
i.e., Y∼LSMEm(Bμ+b,BΣBT,Bγ,gm;Π).
Example2.1 (Generalized hyper-elliptical distribution). The GHE distribution is constructed by mixing a generalized inverse Gaussian distribution with elliptical distribution. A positive random variable Θ is said to have a generalized inverse Gaussian distribution, denoted by Θ∼GIG(λ,a,b), if its pdf is given by
π(θ;λ,a,b)=a−λ(√ab)λ2Kλ(√ab)θλ−1exp(−12(aθ−1+bθ)),θ>0, | (2.11) |
where parameters follow
{a≥0andb>0,ifλ>0,a>0andb≥0,ifλ<0,a>0andb>0,ifλ=0 |
and Kλ(⋅) denotes the modified Bessel function of the second kind with index λ∈R. A random vector X∼GHEn(μ,Σ,γ,gn,λ,a,b) has an n-dimensional GHE distribution, if there exists a random vector Y follows (2.6) such that
X=m(Θ)+Θ12Σ12Y, | (2.12) |
where Θ∼GIG(λ,a,b).
The univariate LSME variable is given by n=1 in the multivariate definition. That is, the univariate LSME variable X∼LSME1(μ,σ2,γ,g1;Π) satisfies
X=m(Θ)+√ΘσZ, |
where Z∼E1(0,1,g1) is the standard elliptical variable, and non-negative scalar random variable Θ with pdf π(θ) is independent of Z. From (2.7), we have
X|θ∼E1(m(θ),θσ2,g1). | (3.1) |
Assuming that both the conditional distribution and the mixed distribution are continuous, the pdf of X produced by the mixed distribution can be written as
fX(x)=∫Ωθf(x|θ)π(θ)dθ, | (3.2) |
where f(x|θ) is the pdf of X|θ and Ωθ is the support of π(θ). Now let xp be the quantile of the LSME variable X. Then the TCE of X can be expressed as
E(X|X>xp)=11−p∫∞xpxfX(x)dx=11−p∫Ωθ∫∞xpxf(x|θ)dxπ(θ)dθ=11−p∫ΩθEX|θ(X|X>xp)¯FX|θ(xp)π(θ)dθ. | (3.3) |
The TCE formula for a univariate elliptical distribution is introduced by [17], and equals to
TCEp(X|θ)=EX|θ(X|X>xp)=m(θ)+1√θσfZ∗(κ(xp;θ))¯FZ(κ(xp;θ))σ2Zθσ2=m(θ)+1√θσfZ∗(κ(xp;θ))¯FZ(κ(xp;θ))Var(X|θ), | (3.4) |
where
κ(x;θ)=x−m(θ)√θσ. |
We now give a general TCE formula for the univariate LSME distributions.
Theorem 1. Let X∼LSME1(μ,σ2,γ,g1;Π) and π′(θ)=(c′)−1θπ(θ) be a mixing pdf with c′=E(Θ)<∞. Then the TCE of X can be computed by:
E(X|X>xp)=μ+γc′1−p¯FLSME,1(xp;μ,σ2,γ,g1;Π′)+c′σ2σ2Z1−pfLSME,1(xp;μ,σ2,γ,¯G;Π′), | (3.5) |
where Π′ is the cdf corresponding to the pdf π′.
Proof. From (3.3) and (3.4), we have
E(X|X>xp)=11−p∫∞0[m(θ)+1√θσfZ∗(κ(xp;θ))¯FZ(κ(xp;θ))Var(X|θ)]¯FX|θ(xp)π(θ)dθ=11−p∫∞xp∫∞0m(θ)√θσfZ(κ(x;θ))π(θ)dθdx+σ2σ2Z1−p∫∞0fZ∗(κ(xp;θ))√θσθπ(θ)dθ=11−p∫∞xpμf(x)dx+γ1−p∫∞xp∫∞0f(x|θ)θπ(θ)dθdx+σ2σ2Z1−p∫∞0fZ∗(κ(xp;θ))√θσθπ(θ)dθ. | (3.6) |
From the definition of LSME distributions and (3.2), we have
∫∞0f(x|θ)θπ(θ)dθ=c′∫∞0f(x|θ)π′(θ)dθ=c′fLSME,1(x;μ,σ2,γ,g1;Π′). |
As a result, (3.6) can be further simplified
E(X|X>xp)=μ+γc′1−p∫∞xp∫∞0f(x|θ)π′(θ)dθdx+c′σ2σ2Z1−p∫∞0fZ∗(κ(xp;θ))√θσπ′(θ)dθ=μ+γc′1−p∫∞xpfLSME,1(x;μ,σ2,γ,g1;Π′)dx+c′σ2σ2Z1−p∫∞0fZ∗(κ(xp;θ))√θσπ′(θ)dθ=μ+γc′1−p¯FLSME,1(xp;μ,σ2,γ,g1;Π′)+c′σ2σ2Z1−pfLSME,1(xp;μ,σ2,γ,¯G;Π′). |
Corollary 1. Let X∼GHE1(μ,σ2,γ,g1,λ,a,b). Assume the conditions in Theorem 1 are satisfied, then the TCE of GHE can be computed by:
TCEp(X)=μ+γ1−p√abKλ+1(√ab)Kλ(√ab)¯FGHE,1(xp;μ,σ2,γ,g1,λ+1,a,b)+σ2σ2Z1−p√abKλ+1(√ab)Kλ(√ab)fGHE,1(xp;μ,σ2,γ,¯G,λ+1,a,b). | (3.7) |
Proof. From the GIG density in (2.11), we conclude
θπ(θ;λ,a,b)=√abKλ+1(√ab)Kλ(√ab)π(θ;λ+1,a,b), |
by setting
c′=√abKλ+1(√ab)Kλ(√ab), |
then
π′(θ)=(c′)−1θπ(θ)=π(θ;λ+1,a,b), |
which also is the GIG density. Using (3.5) we can directly obtain (3.7).
Consider a risk vector Y=(Y1,…,Yn)T and S=Y1+…+Yn. We denote sp as the p-quantile of S, then
E(S|S>sp)=n∑i=1E(Yi|S>sp), |
where E(Yi|S>sp) is the contribution of the i-th risk to the aggregated risks.
Let Y=(Y1,…,Yn)∼En(μ,Σ,gn) and S=Y1+…+Yn, then ([6])
E(Yi|S=s)=∫∞−∞yif(yi|s)dyi=E(Yi)+Cov(Yi,S)Var(S)(s−E(S)). |
The contribution of risk Yi,1≤i≤n, to the total TCE can be expressed as
E(Yi|S>sp)=∫∞spE(Yi|S=s)dFS(s|S>sp)=∫∞spE(Yi|S=s)fS(s)1−FS(sp)ds=11−p∫∞spE(Yi|S=s)fS(s)ds. |
We now exploit this formulation to the multivariate LSME to obtain its portfolio risk decomposition with TCE.
Let us assume X=(X1,…,Xn)T∼LSMEn(μ,Σ,γ,gn;Π). Denote the (i,j) element of Σ by σij, define
S=X1+…+Xn. |
Then, E(Xi|S=s) can be further expanded by conditioning on θ as follows:
E(Xi|S=s)=∫∞−∞xif(xi|s)dxi=∫∞−∞xif(xis)dxifS(s)=1fS(s)∫∞−∞xi∫∞0f(xi,s|θ)dπ(θ)dxi=1fS(s)∫∞−∞xi∫∞0f(xi|s,θ)f(s|θ)π(θ)dθdxi=1fS(s)∫∞0[∫∞−∞xif(xi|s,θ)dxi]f(s|θ)π(θ)dθ. | (4.1) |
To deal with the inner integral, we define a matrix Bi of size 2×n:
Bi=[00…010…011……………1]. | (4.2) |
The first row vector has 1 in the ith position. If we keep the general form
m(θ)=(m1(θ),…,mn(θ))T, |
we have
BiX|θ=(Xi,S|θ)T=Bim(θ)+θ12BiΣ12Y, |
here (Xi,S|θ)T stands for a random column vector of size 2×1, with each element being Xi|θ and S|θ, respectively. Thus, the joint distribution of (Xi,S) under the condition of Θ=θ is a bivariate elliptical distribution
(Xi,S|θ)T∼E2(Bim(θ),θBiΣBTi,g2), |
where the mean vector and convariance matrix of (Xi,S|θ) are given by
E(BiX|θ)=Bim(θ)=(E(Xi|θ),E(S|θ))T=[mi(θ)∑nj=1mj(θ)], |
Cov(BiX|θ)=−ψ′(0)θBiΣBTi=−ψ′(0)[θσiiθ∑nj=1σijθ∑nj=1σijθσ2S], |
where
σ2S=1TΣ1=n∑i=1n∑j=1σij. |
Therefore, if we impose another condition on S, we see that f(xi|s,θ) is an elliptical density. In particular
∫∞−∞xif(xi|s,θ)dxi=E(Xi|S=s,Θ=θ)=E(Xi|θ)+Cov(Xi,S|θ)Var(S|θ)(s−E(S|θ))=mi(θ)+−ψ′(0)∑nj=1σij−ψ′(0)σ2S(s−n∑j=1mj(θ))=mi(θ)+∑dj=1σijσ2S(s−n∑j=1mj(θ)). |
Consequently
E(Xi|S=s)=1fS(s)∫∞0[∫∞−∞xif(xi|s,θ)dxi]f(s|θ)π(θ)dθ=1fS(s)∫∞0[mi(θ)+∑nj=1σijσ2S(s−n∑j=1mj(θ))]×1√θσSfZ(s−∑nj=1mj(θ)√θσS)π(θ)dθ. | (4.3) |
Eventually
E(Xi|S>sp)=11−p∫∞spE(Xi|S=s)fS(s)ds=11−p∫∞sp∫∞0[mi(θ)+∑nj=1σijσ2S(s−n∑j=1mj(θ))]×1√θσSfZ(s−∑nj=1mj(θ)√θσS)π(θ)dθds. | (4.4) |
This expression, though complex, can produce a closed-form quantity to properly select π(θ) and mj(θ).
The portfolio risk decomposition with TCE is additive, that is, the sum of all portfolio risk decomposition must amount to the TCE for S. We can verify this
n∑i=1E(Xi|S>sp)=11−pn∑i=1∫∞sp∫∞0[mi(θ)+∑nj=1σijσ2S(s−n∑j=1mj(θ))]1√θσSfZ(s−∑nj=1mj(θ)√θσS)π(θ)dθds=11−p∫∞sp∫∞0[n∑i=1mi(θ)+∑ni=1∑nj=1σijσ2S(s−n∑j=1mj(θ))]1√θσSfZ(s−∑nj=1mj(θ)√θσS)π(θ)dθds=11−p∫∞sp∫∞0[n∑i=1mi(θ)+(s−n∑j=1mj(θ))]1√θσSfZ(s−∑nj=1mj(θ)√θσS)π(θ)dθds=11−p∫∞sp∫∞0s1√θσSfZ(s−∑nj=1mj(θ)√θσS)π(θ)dθds=E(S|S>sp), |
as required. Now the general portfolio risk decomposition with TCE formula for the multivariate LSME distributions class in presented is a more concrete and compact manner when m(θ) is linear in θ.
Theorem 2. Let X=(X1,X2,⋯,Xn)T∼LSMEn(μ,Σ,γ,gn;Π) and denote the pdf of S=1TX by fS(s). Let π′(θ)=(c′)−1θπ(θ) be a mixing pdf with c′=E(Θ)<∞.
Then the portfolio risk decomposition with TCE for the i-th marginal variable is given by
E(Xi|S>sp)=b0,i+b1,iE(S|S>sp)+b2,i1−pc′¯FLSME,1(sp;1Tμ,1TΣ1,1Tγ,g1;Π′), | (4.5) |
where Π′ is the cdf corresponding to the pdf π′, the coefficients b0,i,b1,i, and b2,i are defined as
b0,i=μi−b1,in∑j=1μj;b1,i=n∑j=1σijσ2S;b2,i=γi−b1,in∑j=1γj, |
and sp is the p-quantile of S.
Proof. Let mi(θ)=μi+θγi, and from (4.3) we have
E(Xi|S=s)=1fS(s)∫∞0[μi+∑nj=1σijσ2S(s−n∑j=1μj)+(γi−n∑j=1γj∑nj=1σijσ2S)θ]×1√θσSfZ(s−∑nj=1μj−θ∑nj=1γj√θσS)π(θ)dθ=1fS(s)∫∞0[b0,i+b1,is+b2,iθ]1√θσSfZ(s−∑nj=1μj−θ∑nj=1γj√θσS)π(θ)dθ=1fS(s)[b0,ifS(s)+b1,isfS(s)+b2,ic′fLSME,1(sp;1Tμ,1TΣ1,1Tγ,g1;Π′)]=b0,i+b1,is+b2,ic′fLSME,1(sp;1Tμ,1TΣ1,1Tγ,g1;Π′)fS(s). |
By inserting this into the portfolio risk decomposition with TCE formulation (4.4), we complete the proof as
E(Xi|S>sp)=∫∞spE(Xi|S=s)f(s|S>sp)ds=∫∞spE(Xi|S=s)fS(s)1−pds=11−p∫∞sp(b0,i+b1,is)fS(s)ds+11−pb2,ic′∫∞spfLSME,1(s;1Tμ,1TΣ1,1Tγ,g1;Π′)ds=b0,i+b1,iE(S|S>sp)+b2,i1−pc′¯FLSME,1(sp;1Tμ,1TΣ1,1Tγ,g1;Π′). | (4.6) |
Notice that ∑ni=1b0,i=0,∑ni=1b1,i=1, and ∑ni=1b2,i=0, which can be used to verify that the sum of these portfolio risk decomposition amounts to E(S|S>sp).
Corollary 2. Let X=(X1,X2,⋯,Xn)T∼GHEn(μ,Σ,γ,gn,λ,a,b). The portfolio risk decomposition with TCE for the i-th marginal variable is given by
E(Xi|S>sp)=μi+σ2Zn∑j=1σij1−p√abKλ+1(√ab)Kλ(√ab)fGHE,1(sp;1Tμ,1TΣ1,1Tγ,¯G;λ+1,a,b)+γi1−p√abKλ+1(√ab)Kλ(√ab)¯FGHE,1(sp;1Tμ,1TΣ1,1Tγ,g1,λ+1,a,b). | (4.7) |
Proof. We can know S∼GHE1(sp;1Tμ,1TΣ1,1Tγ,g1,λ+1,a,b) by using Proposition 1. Using (4.5), we see that TCE of S is given by
E(S|S>sp)=μi+σ2Zn∑j=1σij1−p√abKλ+1(√ab)Kλ(√ab)fGHE,1(sp;1Tμ,1TΣ1,1Tγ,¯G,λ+1,a,b)+γi1−p√abKλ+1(√ab)Kλ(√ab)¯FGHE,1(sp;1Tμ,1TΣ1,1Tγ,g1,λ+1,a,b). |
Therefore
E(Xi|S>sp)=μi+b1,iσ2Sσ2Z1−p√abKλ+1(√ab)Kλ(√ab)fGHE,1(sp;1Tμ,1TΣ1,1Tγ,¯G,λ+1,a,b)+γi1−p√abKλ+1(√ab)Kλ(√ab)¯FGHE,1(sp;1Tμ,1TΣ1,1Tγ,g1,λ+1,a,b)=μi+σ2Z∑nj=1σij1−p√abKλ+1(√ab)Kλ(√ab)fGHE,1(sp;1Tμ,1TΣ1,1Tγ,¯G,λ+1,a,b)+γi1−p√abKλ+1(√ab)Kλ(√ab)¯FGHE,1(sp;1Tμ,1TΣ1,1Tγ,g1,λ+1,a,b). |
The TV of the univariate elliptical distribution is introduced by [8]. From (3.1), We can write the TV for X|θ as
TVp(X|θ)=Var(X|θ)[r(κ(xp;θ))+hZ,Z∗(κ(xp;θ))(κ(xp;θ)−hZ,Z∗(κ(xp;θ))σ2Z)], |
where κ(x;θ) is the same as in (3.4),
r(z)=¯FZ∗(z)¯FZ(z) |
is the distorted ratio function, and
hZ,Z∗(z)=fZ∗(z)¯FZ(z) |
is the distorted hazard function.
TV can be rewritten as:
TVp(X)=Var(X|X>xp)=E[(X−TCEp(X))2|X>xp]=E(X2|X>xp)−[TCEp(X)]2. | (5.1) |
Consequently, we need to derive the second order conditional tail moment E(X2|X>xp). We now provide its analytic expression in the following result.
Proposition 2. Assume a random variable X∼LSME1(μ,σ2,γ,g1;Π). Let π′(θ)=(c′)−1θπ(θ) and π″(θ)=(c″)−1θ2π(θ) be two different mixing pdfs with c′=E(Θ)<∞ and c″=E(Θ2)<∞ respectively. Then
E(X2|X>xp)=σ2σ2Z1−p[(xp+μ)c′fLSME,1(xp;μ,σ2,γ,¯G;Π′)+γc″fLSME,1(xp;μ,σ2,γ,¯G;Π″)+c′¯FLSME,1(xp;μ,σ2,γ,¯G;Π′)]+γ1−p[2μc′¯FLSME,1(xp;μ,σ2,γ,g1;Π′)+γc″¯FLSME,1(xp;μ,σ2,γ,g1;Π″)]+μ2, | (5.2) |
where Π′ and Π″ are two cdfs corresponding to the two different pdfs π′ and π″, respectively.
Proof. From observing
E(X2|X>xp)=11−p∫∞xpx2fX(x)dx=11−p∫∞0∫∞xpx2fX|θ(x)π(θ)dxdθ=11−p∫∞0EX|θ(X2|X>xp)¯FX|θ(xp)π(θ)dθ. |
To deal with the second order conditional tail moment in the integration, we write it as
EX|θ(X2|X>xp)=TVp(X|θ)+[TCEp(X|θ)]2. | (5.3) |
From [17], we know
TCEp(X|θ)=m(θ)+hZ,Z∗(κ(xp;θ))Var(X|θ)√θσ, |
taking Var(X|θ)=θσ2σ2Z into consideration, then (5.3) becomes
EX|θ(X2|X>xp)=Var(X|θ)[r(κ(xp;θ))+hZ,Z∗(κ(xp;θ))(κ(xp;θ)−hZ,Z∗(κ(xp;θ))σ2Z)]+(m(θ)+hZ,Z∗(κ(xp;θ))Var(X|θ)√θσ)2=Var(X|θ)r(κ(xp;θ))+Var(X|θ)hZ,Z∗(κ(xp;θ))xp−m(θ)√θσ−Var(X|θ)(hZ,Z∗(κ(xp;θ)))2σ2Z+m2(θ)+2m(θ)hZ,Z∗(κ(xp;θ))Var(X|θ)√θσ+(hZ,Z∗(κ(xp;θ)))2θσ2σ2ZVar(X|θ)θσ2=m2(θ)+Var(X|θ)(r(κ(xp;θ))+hZ,Z∗(κ(xp;θ))xp+m(θ)√θσ). |
As a result
E(X2|X>xp)=11−p∫∞0EX|θ(X2|X>xp)¯FX|θ(xp)π(θ)dθ=11−p∫∞0Var(X|θ)[xp+m(θ)√θσhZ,Z∗(κ(xp;θ))+r(κ(xp;θ))]ׯFX|θ(xp)π(θ)dθ+11−p∫∞0m2(θ)¯FX|θ(xp)π(θ)dθ=11−p∫∞0Var(X|θ)[xp+m(θ)√θσfZ∗(κ(xp;θ))+¯FZ∗(κ(xp;θ))]×π(θ)dθ+11−p∫∞0¯FX|θ(xp)π(θ)(μ2+2μθγ+θ2γ2)dθ=σ2σ2Z1−p[(xp+μ)c′fLSME,1(xp;μ,σ2,γ,¯G;Π′)+γc″fLSME,1(xp;μ,σ2,γ,¯G;Π″)+c′¯FLSME,1(xp;μ,σ2,γ,¯G;Π′)]+γ1−p[2μc′¯FLSME,1(xp;μ,σ2,γ,g1;Π′)+γc″¯FLSME,1(xp;μ,σ2,γ,g1;Π″)]+μ2. |
Theorem 3. Under assumptions of Proposition 2, the TV of X is given by
TVp(X)=σ2σ2Z1−p[(xp−μ)c′fLSME,1(xp;μ,σ2,γ,¯G;Π′)+γc″fLSME,1(xp;μ,σ2,γ,¯G;Π″)+c′¯FLSME,1(xp;μ,σ2,γ,¯G;Π′)]+γ21−pc″¯FLSME,1(xp;μ,σ2,γ,g1;Π″)−(c′1−p)2[γ¯FLSME,1(xp;μ,σ2,γ,g1;Π′)+σ2σ2ZfLSME,1(xp;μ,σ2,γ,¯G;Π′)]2, | (5.4) |
where Π′ and Π″ are two cdfs corresponding to the two different pdfs π′ and π″, respectively.
Proof. From the Theorem 1, the TCE formula is
E(X|X>xp)=μ+γc′1−p¯FLSME,1(xp;μ,σ2,γ,g1;Π′)+c′σ2σ2Z1−pfLSME,1(xp;μ,σ2,γ,¯G;Π′). |
Hence, the result can be derived by using Proposition 2 and (5.1).
Corollary 3. Let X∼GHE1(μ,σ2,γ,g1,λ,a,b). Assume the conditions in Theorem 3 are satisfied, then the TV for GHE can be computed by:
TVp(X)=σ2σ2Z1−p[(xp−μ)√abKλ+1(√ab)Kλ(√ab)fGHE,1(xp;μ,σ2,γ,¯G;λ+1,a,b)+γabKλ+2(√ab)Kλ(√ab)fGHE,1(xp;μ,σ2,γ,¯G;λ+2,a,b)+√abKλ+1(√ab)Kλ(√ab)¯FGHE,1(xp;μ,σ2,γ,¯G;λ+1,a,b)]+γ21−pabKλ+2(√ab)Kλ(√ab)¯FGHE,1(xp;μ,σ2,γ,g1,λ+2,a,b)−(11−p√abKλ+1(√ab)Kλ(√ab))2[γ¯FGHE,1(xp;μ,σ2,γ,g1,λ+1,a,b)+σ2σ2ZfGHE,1(xp;μ,σ2,γ,¯G;λ+1,a,b)]2. | (5.5) |
Proof. From the GIG density in (2.11), we conclude
θπ(θ;λ,a,b)=√abKλ+1(√ab)Kλ(√ab)π(θ;λ+1,a,b) |
and
θ2π(θ;λ,a,b)=abKλ+2(√ab)Kλ(√ab)π(θ;λ+2,a,b). |
By setting
c′=√abKλ+1(√ab)Kλ(√ab),c″=abKλ+2(√ab)Kλ(√ab), |
the two pdfs can be presented as
π′(θ)=(c′)−1θπ(θ)=π(θ;λ+1,a,b) |
and
π″(θ)=(c″)−1θ2π(θ)=π(θ;λ+2,a,b), |
which also are two GIG pdfs. Using (5.4) we can directly obtain (5.5).
Example6.1 (Generalized hyperbolic distribution). If μ=0, Σ=In and density generator g(u)=e−u in (2.2), then the elliptical vector Y is said to have a multivariate normal distribution, denoted by Y∼Nn(0,In). Letting Y∼Nn(0,In) in (2.6), then the random vector X∼GHn(μ,Σ,γ,λ,a,b) is an n-dimensional generalized hyperbolic (GH) distribution. Therefore, the pdf of the GH distribution is (see [15])
fGHn(x,μ,Σ,γ,λ,a,b)=cKλ−(n/2)(√(a+(x−μ)TΣ−1(x−μ))(b+γTΣ−1γ))e(x−μ)TΣ−1γ(a+(x−μ)TΣ−1(x−μ))(b+γTΣ−1γ))n4−λ2, |
where the normalizing constant is
c=(ab)−λ2bλ(b+γTΣ−1γ)(n/2)−λ(2π)n/2|Σ|1/2Kλ√ab. |
From Corollary 1, TCE of GH distribution is given by
TCEp(X)=μ+γ1−p√abKλ+1(√ab)Kλ(√ab)¯FGH,1(xp;μ,σ2,γ,λ+1,a,b)+σ21−p√abKλ+1(√ab)Kλ(√ab)fGH,1(xp;μ,σ2,γ,λ+1,a,b). |
From Corollary 2, portfolio risk decomposition with TCE for the i-th marginal of GH distribution is given by
E(Xi|S>sp)=μi+n∑j=1σij1−p√abKλ+1(√ab)Kλ(√ab)fGH,1(sp;1Tμ,1TΣ1,1Tγ,λ+1,a,b)+γi1−p√abKλ+1(√ab)Kλ(√ab)¯FGH,1(sp;1Tμ,1TΣ1,1Tγ,λ+1,a,b). |
From Corollary 3, TV of GH distribution is given by
TVp(X)=σ21−p[(xp−μ)√abKλ+1(√ab)Kλ(√ab)fGH,1(xp;μ,σ2,γ,λ+1,a,b)+γabKλ+2(√ab)Kλ(√ab)fGH,1(xp;μ,σ2,γ,λ+2,a,b)+√abKλ+1(√ab)Kλ(√ab)¯FGH,1(xp;μ,σ2,γ,λ+1,a,b)]+γ21−pabKλ+2(√ab)Kλ(√ab)¯FGH,1(xp;μ,σ2,γ,λ+2,a,b)−(11−p√abKλ+1(√ab)Kλ(√ab))2[γ¯FGH,1(xp;μ,σ2,γ,λ+1,a,b)+σ2fGH,1(xp;μ,σ2,γ,λ+1,a,b)]2. |
In this section we discuss the TV of five stocks (Amazon, Goldman Sachs, IBM, Google, and Apple) and aggregate portfolio covering a time frame from the 1st of January 2015 to the 1st of January 2017. Ignatieva and Landsman [12] fitted a GH model to five stocks and aggregate portfolio, and obtained the following parameter set based on the maximum likelihood technique.
λ=−1.18336,a=1.272016,ψ=0.348483,μ=(−0.09977−0.04555−0.09355−0.03669−0.10367),γ=(−0.08626−0.008030.07928−0.052300.08534), |
Σ=(3.3871.4071.1031.8281.3541.4073.0141.2881.2091.4341.1031.2881.8701.0611.1551.8281.2091.0612.1711.2201.3541.4341.1551.2202.891). |
For the risk analysis, we denote five stocks as X1,⋯,X5. We also consider aggregate portfolio S where each stock has equal weight for simplicity, so that the aggregate portfolio is defined as S=X1+⋯+X5. Figure 1 shows the densities for five stocks Xi,i=1,⋯,5 and aggregate portfolio S. The pdf of S has the largest variance, and Amazon has the largest dispersion among five stocks. IBM has the smallest dispersion. Figure 2 presents the TV for five stocks Xi,i=1,⋯,5 and aggregate portfolio S. All the risk measures increase over the quantile with the TV. Also Figure 2 shows the differences in the TV measure along with five stocks and aggregate portfolio. For the same quantile, the TV of Apple is the largest one and the TV of IBM is the smallest one among the five stocks.
In this paper we generalize the tail risk measure and portfolio risk decomposition with TCE formula derived by [15] for the class of multivariate normal mean-variance mixture distributions to the larger class of multivariate elliptical location-scale mixtures distributions. A prominent member in the normal mean-variance mixture class is the generalized hyperbolic (GH) distribution, which itself can construct a Lˊevy process. The GH is a special case of normal mean-variance mixture random variable with X∼Nn(0,In) and the distribution of Θ given by a generalized inverse Gaussian (GIG) distribution with three parameters (see [12,15] for details). Prominent member in the elliptical location-scale mixtures class is the generalized hyper-elliptical (GHE) distribution. The GHE distribution provides excellent fit to univariate and multivariate data, allowing to capture a long right tail in the distribution of losses even more effectively than the GH distribution considered in [12]. And GHE is a special case of elliptical location-scale mixtures random variable with X∼Nn(0,In) and the distribution of Θ given by a generalized inverse Gaussian (GIG) distribution with three parameters. Although the univariate TCE and portfolio risk decomposition with TCE formula for the GHE class was available in [13], it can be derived more efficiently and seen as a special case of TCE for the unified location-scale mixtures of elliptical distributions and risk allocation formula in Theorems 1 and 2, respectively. And the univariate TV formula for the GHE class can be derived efficiently and seen as a special case of TV for the unified location-scale mixtures of elliptical distributions in Theorem 3.
The research was supported by the National Natural Science Foundation of China (No. 12071251).
The authors declare no conflict of interest.
[1] |
Z. Guo, K. Yu, Z. Lv, K. K. R. Choo, P. Shi, J. Rodrigues, Deep federated learning enhanced secure poi microservices for cyber-physical systems, IEEE Wireless Commun., 29 (2022), 22–29. https://doi.org/10.1109/MWC.002.2100272 doi: 10.1109/MWC.002.2100272
![]() |
[2] |
S. Xia, Z. Yao, G. Wu, Y. Li, Distributed offloading for cooperative intelligent transportation under heterogeneous networks, IEEE Trans. Intell. Transp. Syst., 23 (2022), 16701–16714. https://doi.org/10.1109/TITS.2022.3190280 doi: 10.1109/TITS.2022.3190280
![]() |
[3] |
Z. Guo, K. Yu, A. Jolfaei, F. Ding, N. Zhang, Fuz-spam: label smoothing-based fuzzy detection of spammers in internet of things, IEEE Trans. Fuzzy Syst., 30 (2022), 4543–4554. https://doi.org/10.1109/TFUZZ.2021.3130311 doi: 10.1109/TFUZZ.2021.3130311
![]() |
[4] |
L. Zhao, Z. Yin, K. Yu, X. Tang, L. Xu, Z. Guo, et al., A fuzzy logic based intelligent multi-attribute routing scheme for two-layered sdvns, IEEE Trans. Network Serv. Manage., 2022 (2022). https://doi.org/10.1109/TNSM.2022.3202741 doi: 10.1109/TNSM.2022.3202741
![]() |
[5] |
Z. Zhou, X. Dong, Z. Li, K. Yu, C. Ding, Y. Yang, Spatio-yemporal feature encoding for traffic accident detection in vanet environment, IEEE Trans. Intell. Transp. Syst., 23 (2022), 19772–19781. https://doi.org/10.1109/TITS.2022.3147826 doi: 10.1109/TITS.2022.3147826
![]() |
[6] |
S. Zhang, H. Gu, K. Chi, L. Huang, K. Yu, S. Mumtaz, Drl-based partial offloading for maximizing sum computation rate of wireless powered mobile edge computing network, IEEE Trans. Wireless Commun., 21 (2022), 10934–10948. https://doi.org/10.1109/TWC.2022.3188302 doi: 10.1109/TWC.2022.3188302
![]() |
[7] |
D. Peng, D. He, Y. Li, Z. Wang, Integrating terrestrial and satellite multibeam systems toward 6G: techniques and challenges for interference mitigation, IEEE Wireless Commun., 29 (2022), 24–31. https://doi.org/10.1109/MWC.002.00293 doi: 10.1109/MWC.002.00293
![]() |
[8] |
A. Büyükkarci, M. Müldür, digital storytelling for primary school mathematics teaching: product and process evaluation, Educ. Inf. Technol., 27 (2022), 5365–5396. https://doi.org/10.1007/s10639-021-10813-8 doi: 10.1007/s10639-021-10813-8
![]() |
[9] |
Z. Guo, K. Yu, A. K. Bashir, D. Zhang, Y. D. Al-Otaibi, M. Guizani, Deep information fusion-driven POI scheduling for mobile social networks, IEEE Network, 36 (2022), 210–216. https://doi.org/10.1109/MNET.102.2100394 doi: 10.1109/MNET.102.2100394
![]() |
[10] |
A. Cahyadi, Hendryadi, S. Widyastuti, Suryani, Covid-19, emergency remote teaching evaluation: the case of Indonesia, Educ. Inf. Technol., 27 (2022), 2165–2179. https://doi.org/10.1007/s10639-021-10680-3 doi: 10.1007/s10639-021-10680-3
![]() |
[11] |
Y. Lu, L. Yang, S. X. Yang, Q. Hua, A. K. Sangaiah, T. Guo, et al., An intelligent deterministic scheduling method for ultra-low latency communication in edge enabled industrial internet of things, IEEE Trans. Ind. Inf., 19 (2023), 1756–1767. https://doi.org/10.1109/TII.2022.3186891 doi: 10.1109/TII.2022.3186891
![]() |
[12] | B. Huang, K. Wang, An improved BP neural network-based quality evaluation model for Chinese international education teaching courses, in ICCDA 2022: The 6th International Conference on Compute and Data Analysis, (2022), 122–127. https://doi.org/10.1145/3523089.3523109 |
[13] |
Q. Zhang, K. Yu, Z. Guo, S. Garg, J. Rodrigues, M. M. Hassan, et al., Graph neural network-driven traffic forecasting for the connected internet of vehicles, IEEE Trans. Network Sci. Eng., 9 (2022), 3015–3027. https://doi.org/10.1109/TNSE.2021.3126830 doi: 10.1109/TNSE.2021.3126830
![]() |
[14] |
C. Hou, J. Ai, Y. Lin, C. Guan, J. Li, W. Zhu, Evaluation of online teaching quality based on facial expression recognition, Future Internet, 14 (2022). https://doi.org/10.3390/fi14060177 doi: 10.3390/fi14060177
![]() |
[15] |
S. Qi, L. Liu, B. S. Kumar, A. Prathik, An english teaching quality evaluation model based on gaussian process machine learning, Expert Syst. J. Knowl. Eng., 39 (2022). https://doi.org/10.1111/exsy.12861 doi: 10.1111/exsy.12861
![]() |
[16] | H. Shu, English teaching effect evaluation based on data association mining, in CIPAE 2021: 2nd International Conference on Computers, Information Processing and Advanced Education, (2021), 1223–1226. https://doi.org/10.1145/3456887.3457494 |
[17] |
P. Gao, VIKOR method for intuitionistic fuzzy multi-attribute group decision-making and its application to teaching quality evaluation of college english, J. Intell. Fuzzy Syst., 42 (2022), 5189–5197. https://doi.org/10.3233/JIFS-211749 doi: 10.3233/JIFS-211749
![]() |
[18] |
D. Wei, Y. Rong, H. Garg, J. Liu, An extended WASPAS approach for teaching quality evaluation based on pythagorean fuzzy reducible weighted maclaurin symmetric mean, J. Intell. Fuzzy Syst., 42 (2022), 3121–3152. https://doi.org/10.3233/JIFS-210821 doi: 10.3233/JIFS-210821
![]() |
[19] |
M. Li, Multidimensional analysis and evaluation of college english teaching quality based on an artificial intelligence model, J. Sens., 2022 (2022), 1–13. https://doi.org/10.1155/2022/1314736 doi: 10.1155/2022/1314736
![]() |
[20] |
S. Zeng, Y. Pan, H. Jin, Online teaching quality evaluation of business statistics course utilizing fermatean fuzzy analytical hierarchy process with aggregation operator, Systems, 10 (2022). https://doi.org/10.3390/systems10030063 doi: 10.3390/systems10030063
![]() |
[21] |
B. Feng, Dynamic analysis of college physical education teaching quality evaluation based on network under the big data, Comput. Intell. Neurosci., 2021 (2021). https://doi.org/10.1155/2021/5949167 doi: 10.1155/2021/5949167
![]() |
[22] |
X. Xu, F. Liu, Optimization of online education and teaching evaluation system based on GA-BP neural network, Comput. Intell. Neurosci., 2021 (2021). https://doi.org/10.1155/2021/8785127 doi: 10.1155/2021/8785127
![]() |
[23] |
J. Heo, S. Han, The mediating effect of literacy of LMS between self-evaluation online teaching effectiveness and self-directed learning readiness, Educ. Inf. Technol., 26 (2021), 6097–6108. https://doi.org/10.1007/s10639-021-10590-4 doi: 10.1007/s10639-021-10590-4
![]() |
[24] |
R. Tárraga-Mínguez, C. S. Guerrero, P. Sanz-Cervera, Digital teaching competence evaluation of pre-service teachers in spain: a review study, IEEE Rev. Iberoam. Tecnol. Aprendizaje, 16 (2021), 70–76. https://doi.org/10.1109/RITA.2021.3052848 doi: 10.1109/RITA.2021.3052848
![]() |
[25] |
Y. V. Tsekhmister, T. Konovalova, B. Y. Tsekhmister, A. Agrawal, D. Ghosh, Evaluation of virtual reality technology and online teaching system for medical students in ukraine during COVID-19 pandemic, Int. J. Emerging Technol. Learn., 16 (2021). https://doi.org/10.3991/ijet.v16i23.26099 doi: 10.3991/ijet.v16i23.26099
![]() |
[26] |
Y. Wang, S. Li, B. Zhao, J. Zhang, Y. Yang, B. Li, A resnet-based approach for accurate radiographic diagnosis of knee osteoarthritis, CAAI Trans. Intell. Technol., 7 (2022), 512–521. https://doi.org/10.1049/cit2.12079 doi: 10.1049/cit2.12079
![]() |
[27] |
Y. Wang, C. Sun, Y. Guo, A multi-attribute fuzzy evaluation model for the teaching quality of physical education in colleges and its implementation strategies, Int. J. Emerging Technol. Learn., 16 (2021). https://doi.org/10.3991/ijet.v16i02.19725 doi: 10.3991/ijet.v16i02.19725
![]() |
[28] |
S. Qiao, S. Pang, G. Luo, S. Pan, Z. Yu, T. Chen, et al., RLDS: an explainable residual learning diagnosis system for fetal congenital heart disease, Future Gener. Comput. Syst., 128 (2022), 205–218. https://doi.org/10.1016/j.future.2021.10.001 doi: 10.1016/j.future.2021.10.001
![]() |
[29] |
S. Qiao, S. Pang, G. Luo, S. Pan, T. Chen, Z. Lv, FLDS: an intelligent feature learning detection system for visualizing medical images supporting fetal four-chamber views, IEEE J. Biomed. Health Inf., 26 (2022), 4814–4825. https://doi.org/10.1109/JBHI.2021.3091579 doi: 10.1109/JBHI.2021.3091579
![]() |
[30] |
S. Qiao, S. Pang, Y. Sun, G. Luo, W. Yin, Y. Zhao, et al., Sprechd: four-chamber semantic parsing network for recognizing fetal congenital heart disease in medical Metaverse, IEEE J. Biomed. Health. Inf., (2022), 1–11. https://doi.org/10.1109/JBHI.2022.3218577 doi: 10.1109/JBHI.2022.3218577
![]() |
[31] |
Y. Zhang, The development of an evaluation model to assess the effect of online english teaching based on fuzzy mathematics, Int. J. Emerging Technol. Learn., 16 (2021). https://doi.org/10.3991/ijet.v16i12.23325 doi: 10.3991/ijet.v16i12.23325
![]() |
[32] |
Y. Han, Evaluation of english online teaching based on remote supervision algorithms and deep learning, J. Intell. Fuzzy Syst., 40 (2021), 7097–7108. https://doi.org/10.3233/JIFS-189539 doi: 10.3233/JIFS-189539
![]() |
[33] |
H. Liang, Role of artificial intelligence algorithm for taekwondo teaching effect evaluation model, J. Intell. Fuzzy Syst., 40 (2021), 3239–3250. https://doi.org/10.3233/JIFS-189364 doi: 10.3233/JIFS-189364
![]() |
[34] |
Y. Liu, Evaluation algorithm of teaching work quality in colleges and universities based on deep denoising autoencoder network, Mobile Inf. Syst., 2021 (2021). https://doi.org/10.1155/2021/8161985 doi: 10.1155/2021/8161985
![]() |
[35] |
G. Li, F. Liu, Y. Wang, Y. Guo, L. Xiao, L. Zhu, A convolutional neural network (CNN) based approach for the recognition and evaluation of classroom teaching behavior, Sci. Program., 2021 (2021). https://doi.org/10.1155/2021/6336773 doi: 10.1155/2021/6336773
![]() |
[36] |
P. Liu, X. Wang, F. Teng, Online teaching quality evaluation based on multi-granularity probabilistic linguistic term sets, J. Intell. Fuzzy Syst., 40 (2021), 9915–9935. https://doi.org/10.3233/JIFS-202543 doi: 10.3233/JIFS-202543
![]() |
[37] |
Q. Wang, Research on teaching quality evaluation of college english based on the CODAS method under interval-valued intuitionistic fuzzy information, J. Intell. Fuzzy Syst., 41 (2021), 1499–1508. https://doi.org/10.3233/JIFS-210366 doi: 10.3233/JIFS-210366
![]() |
[38] |
H. Yu, Online teaching quality evaluation based on emotion recognition and improved aprioritid algorithm, J. Intell. Fuzzy Syst., 40 (2021), 7037–7047. https://doi.org/10.3233/JIFS-189534 doi: 10.3233/JIFS-189534
![]() |
[39] | Y. Yu, English teaching ability evaluation algorithm based on big data fuzzy k-means clustering, Advances in Intelligent Systems and Computing, Springer, (2021), 557–564. https://doi.org/10.1007/978-3-030-69999-4_77 |
[40] |
A. Amelio, G. Bonifazi, F. Cauteruccio, E. Corradini, M. Marchetti, D. Ursino, et al., Representation and compression of residual neural networks through a multilayer network based approach, Expert Syst. Appl., 215 (2023). https://doi.org/10.1016/j.eswa.2022.119391 doi: 10.1016/j.eswa.2022.119391
![]() |
1. | Mengxin He, Zhong Li, Dynamic behaviors of a Leslie-Gower predator-prey model with Smith growth and constant-yield harvesting, 2024, 32, 2688-1594, 6424, 10.3934/era.2024299 |