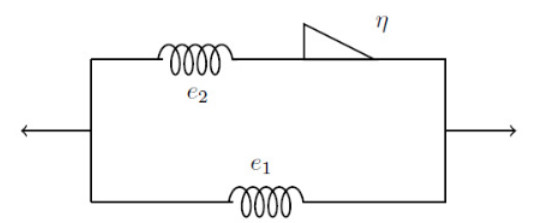
This research deals with formulating a multi-species eco-epidemiological mathematical model when the interacting species compete for the same food sources and the prey species have some infection. It is assumed that infection does not spread vertically. Infectious diseases severely affect the population dynamics of prey and predator. One of the most important factors in population dynamics is the movement of species in the habitat in search of resources or protection. The ecological influences of diffusion on the population density of both species are studied. The study also deals with the analysis of the effects of diffusion on the fixed points of the proposed model. The fixed points of the model are sorted out. The Lyapunov function is constructed for the proposed model. The fixed points of the proposed model are analyzed through the use of the Lyapunov stability criterion. It is proved that coexisting fixed points remain stable under the effects of self-diffusion, whereas, in the case of cross-diffusion, Turing instability exists conditionally. Moreover, a two-stage explicit numerical scheme is constructed, and the stability of the said scheme is found by using von Neumann stability analysis. Simulations are performed by using the constructed scheme to discuss the model's phase portraits and time-series solution. Many scenarios are discussed to display the present study's significance. The impacts of the transmission parameter 𝛾 and food resource f on the population density of species are presented in plots. It is verified that the availability of common food resources greatly influences the dynamics of such models. It is shown that all three classes, i.e., the predator, susceptible prey and infected prey, can coexist in the habitat, and this coexistence has a stable nature. Hence, in the realistic scenarios of predator-prey ecology, the results of the study show the importance of food availability for the interacting species.
Citation: Muhammad Shoaib Arif, Kamaleldin Abodayeh, Asad Ejaz. On the stability of the diffusive and non-diffusive predator-prey system with consuming resources and disease in prey species[J]. Mathematical Biosciences and Engineering, 2023, 20(3): 5066-5093. doi: 10.3934/mbe.2023235
[1] | Maurizio Verri, Giovanna Guidoboni, Lorena Bociu, Riccardo Sacco . The role of structural viscoelasticity in deformable porous media with incompressible constituents: Applications in biomechanics. Mathematical Biosciences and Engineering, 2018, 15(4): 933-959. doi: 10.3934/mbe.2018042 |
[2] | Fang Wang, Jiaming Wang, Mingxin Li, Jun Hu, Kehua Song, Jianguo Zhang, Yubo Fan . Biomechanical study of the effect of traction on elbow joint capsule contracture. Mathematical Biosciences and Engineering, 2023, 20(12): 21451-21466. doi: 10.3934/mbe.2023949 |
[3] | Oualid Kafi, Nader El Khatib, Jorge Tiago, Adélia Sequeira . Numerical simulations of a 3D fluid-structure interaction model for blood flow in an atherosclerotic artery. Mathematical Biosciences and Engineering, 2017, 14(1): 179-193. doi: 10.3934/mbe.2017012 |
[4] | Christophe Prud'homme, Lorenzo Sala, Marcela Szopos . Uncertainty propagation and sensitivity analysis: results from the Ocular Mathematical Virtual Simulator. Mathematical Biosciences and Engineering, 2021, 18(3): 2010-2032. doi: 10.3934/mbe.2021105 |
[5] | Tran Quang-Huy, Phuc Thinh Doan, Nguyen Thi Hoang Yen, Duc-Tan Tran . Shear wave imaging and classification using extended Kalman filter and decision tree algorithm. Mathematical Biosciences and Engineering, 2021, 18(6): 7631-7647. doi: 10.3934/mbe.2021378 |
[6] | Tuoi Vo, William Lee, Adam Peddle, Martin Meere . Modelling chemistry and biology after implantation of a drug-eluting stent. Part Ⅰ: Drug transport. Mathematical Biosciences and Engineering, 2017, 14(2): 491-509. doi: 10.3934/mbe.2017030 |
[7] | Li Cai, Qian Zhong, Juan Xu, Yuan Huang, Hao Gao . A lumped parameter model for evaluating coronary artery blood supply capacity. Mathematical Biosciences and Engineering, 2024, 21(4): 5838-5862. doi: 10.3934/mbe.2024258 |
[8] | Xu Bie, Yuanyuan Tang, Ming Zhao, Yingxi Liu, Shen Yu, Dong Sun, Jing Liu, Ying Wang, Jianing Zhang, Xiuzhen Sun . Pilot study of pressure-flow properties in a numerical model of the middle ear. Mathematical Biosciences and Engineering, 2020, 17(3): 2418-2431. doi: 10.3934/mbe.2020131 |
[9] | Subhadip Paul, Prasun Kumar Roy . The consequence of day-to-day stochastic dose deviation from the planned dose in fractionated radiation therapy. Mathematical Biosciences and Engineering, 2016, 13(1): 159-170. doi: 10.3934/mbe.2016.13.159 |
[10] | Rebecca Vandiver . Effect of residual stress on peak cap stress in arteries. Mathematical Biosciences and Engineering, 2014, 11(5): 1199-1214. doi: 10.3934/mbe.2014.11.1199 |
This research deals with formulating a multi-species eco-epidemiological mathematical model when the interacting species compete for the same food sources and the prey species have some infection. It is assumed that infection does not spread vertically. Infectious diseases severely affect the population dynamics of prey and predator. One of the most important factors in population dynamics is the movement of species in the habitat in search of resources or protection. The ecological influences of diffusion on the population density of both species are studied. The study also deals with the analysis of the effects of diffusion on the fixed points of the proposed model. The fixed points of the model are sorted out. The Lyapunov function is constructed for the proposed model. The fixed points of the proposed model are analyzed through the use of the Lyapunov stability criterion. It is proved that coexisting fixed points remain stable under the effects of self-diffusion, whereas, in the case of cross-diffusion, Turing instability exists conditionally. Moreover, a two-stage explicit numerical scheme is constructed, and the stability of the said scheme is found by using von Neumann stability analysis. Simulations are performed by using the constructed scheme to discuss the model's phase portraits and time-series solution. Many scenarios are discussed to display the present study's significance. The impacts of the transmission parameter 𝛾 and food resource f on the population density of species are presented in plots. It is verified that the availability of common food resources greatly influences the dynamics of such models. It is shown that all three classes, i.e., the predator, susceptible prey and infected prey, can coexist in the habitat, and this coexistence has a stable nature. Hence, in the realistic scenarios of predator-prey ecology, the results of the study show the importance of food availability for the interacting species.
The biomechanical characterization of biological soft tissues was initially developed by Y.C. Fung on his classical biomechanical treatments [1,2]. He was one of the firsts, together with Fronek, to used a ``new kind'' of elasticity to describe the mechanical behavior of the soft tissues [3], they called this new behavior as pseudo-elasticity. A few years later a new generation of researchers continued on this sense, one of the most know is Holzapfel that together with Gasser and Ogden proposed a new constitutive framework for arterial wall mechanics behavior [4], basically based in a non linear elastic theory introduced by Ogden [5]. In 2004 this group of research realized a comparison of a multi-layer structural model for arterial walls applying a Fung type model, i.e. viscoelasticity. They explored the problematic that emerge on the material stability, in the convergence sense and others problems relatives to the viscoelastic formulation, then in 2010 Holzapfel and Ogden proposed a constitutive modeling of arteries [6] that originated a whole new constitutive frame, named hyperelasticity. Based on strain-energy functions and that represents a huge step on the task of arteries biomechanical characterization, and a great variety of progress was developed and published, like the modeling of biomechanical effects originated by an aneurysm [7,8], the 3D modelling of the human aorta [9,10] or the visco/hyperelastic model that simulate the nonlinear dynamics of atherosclerotic coronary arteries used to predict the initiation of heart attack [11,12].
No often it is not the original Fung's propose for modeling arteries biomechanical behavior, he originally describe the mechanical behavior of the artery as a viscoelastic material. In general, this behavior may be imagined as a spectrum with elastic deformation in one limit case and viscous flow in the other, with varying combinations of the two spread over the range between. Thus, valid constitutive equations for viscoelastic behavior embody elastic deformation and viscous flow as special cases and at the same time provide for response patterns that characterize behavior blends of the two. Intrinsically, such equations will involve not only stress and strain, but time-rates of both stress and strain as well [13]. As mentioned before this kind of materials models, has a great inconvenient related with the convergence on the finite element method software, frequently used to solve this mathematical models [8,6,14,15]. To avoid this situation we can use a prony series and the relaxation function, but again to obtain an accurate solution we need to use a large number of prony series that elevates the computer time on the task of solution finding.
At recent times the fractional calculus theory has been used to modeling viscoelastic materials [16,17,18], consequently some researchers used to model biological soft tissues [19,20], like the use of Kelvin-Voigt fractional viscoelastic model employed to determinate the biomechanical properties of the human liver tissue or the pancreas by Wex [21,22], using stress relaxation test to articular cartilage [23], and even the human calcaneal fat pad [24] using fractional derivatives kernels. Recently this material models are used to estimate the biological changes of the mechanical behavior due to the presence of tumors [25]. Craiem et al [26,27,28] use a fractional viscoelastic constitutive model to describe the arterial biomechanics response, using uniaxial relaxation test.
One of the greatest advantages consist on that many of the basic viscoelastic ideas can be introduce within the context of a one-dimensional state of stress. Once the relaxation modulus, the creep compliance and the complex modulus are obtained, its functions can be included by a subroutine on a FEM software, with the necessary geometry restrictions [29] and the viscoelastic relaxation modifications, or by an finite element model specially develop for fractional differential and integral operators [30].
Viscoelastic fractional models have taken a recent boom in the task of modeling the mechanical behaviour of polymers and soft tissues. Due to the fact that the definition of the fractional derivative provide a new formulation to describe the mechanical behaviour of a material that exhibits a behavior that oscillates between the hooke solids model and the Newtonian fluids [31]. That is one of the principal characteristics of the soft tissues.
The circulatory system is basically composed of the heart and blood vessel system. At the time, the blood vessel system are composing of arteries, arterioles and veins. Arteries are basically conform of three internal layers, known as Tunica Intima, Tunica Media and Tunica Externa or Adventicia, with a semi-cylindrical form and mainly compose of collagen, elastin and muscular fibers [32]. In young humans, the intima is an extremely thin layer (80nm) like a membrane separate to the media for a lay of elastin, the media are form of soft muscular cells merge on a collagen and elastin cellular matrix, finally the externa is the thick layer compose of collagen and fibroblasts [33].
This particular conformation brings the artery a mixed mechanical material behavior know as viscoelasticity [1]. In general, viscoelastic behavior may be imagined as a spectrum with elastic deformation as one limiting case and viscous flow the other extreme case, with varying combinations of the two spread over the range between. Thus, valid constitutive equations for viscoelastic behavior embody elastic deformation and viscous flow as special cases and at the same time provide for response patterns that characterize behavior blends of the two.
Intrinsically, such equations will involve not only stress and strain, but time-rates of both stress and strain as well [13].
We first develop the mathematical and mechanical background that support the present research, with the finality that those readers interested on the topics be familiarized with the basic concepts.
Linear viscoelasticity is a common theory to approximate the time-dependent behaviour of polymers, and materials that exhibit similar characteristics at relatively low temperatures and stress.
The development of the mathematical theory of linear viscoelasticity is based on the principle that the mechanical stress on a certain period of time is directly proportional to the strain rate. In that way, if we have that stress and stress rate are infinitesimal and the stress-strain relation depend on time, that relationship can be expressed by a differential equation with constant coefficients.
The stress-strain relationship can be described, assuming that the Maxwell-Boltzmann principle are satisfied, by the constitutive equation:
σ(t)=t∫−∞G(t−ξ)dε(ξ)dξdξ | (2.1) |
or
ε(t)=t∫−∞J(t−ξ)dσ(ξ)dξdξ | (2.2) |
were G(t) and J(t) are the stress relaxation modulus and the creep compliance respectively. These important functions are commonly employing on material characterization, and are describing above.
The creep test consists of instantaneously subjecting the material to a simple shear stress of magnitude σ0 and maintaining that stress constant thereafter while measuring the shear strain as a function of time. The resulting strain is called the creep. In the stress relaxation test, and instantaneous shear strain of magnitude ε0 is imposed on the material sample and maintained at the value while the resulting stress, is recorded as a function of time. The decrease in the stress values over the duration of the test is referred to as the stress relaxation.
The behavior of viscoelastic materials when are subject to harmonic stress or strain is an important part of the theory of viscoelasticity and sustains a fundamental part of the research. Cyclic experiments are used to identify the mechanical behavior of the material and to determine the values of the elastic and viscous plots of this, maintaining a balance between complexity and simulation capacity of the phenomena. Data processing could be carried out in the same way for a non-cyclic signal, but it would have to be extensive in time to have enough information to fit the model and its processing would be complex.
Consider the response of the material, when is applying a harmonic shear strain of frequency ω as:
ε(t)=ε0sin(ωt) | (2.3) |
At the same time the strain rate changes with the same frequency ω with a translation ϕ with respect to the stress,
σ(t)=σ0sin(ωt+ϕ) | (2.4) |
replacing equation 2.4 on equation 2.1, will be able to obtain the constitutive equation:
σ(t)=ε0(G′sin(ωt)+G′′cos(ωt)) | (2.5) |
with
G′(ω)=ω∫∞0G(t−ξ)sin(ω(t−ξ)) d(t−ξ) | (2.6) |
and
G′′(ω)=ω∫∞0G(t−ξ)cos(ω(t−ξ)) d(t−ξ) | (2.7) |
where G′(ω), G′′(ω) are known as the storage and loss modulus respectively. Expressing the harmonic functions on the complex plane we have
σ∗ε∗=G∗=G′+iG′′ | (2.8) |
where G∗ are define as the complex modulus, and is simply the norm of the loss and storage modulus contributions.
At recent times the fractional calculus theory, has used to formulate a wide range of new models on the biomechanics and mechanobiology field [20], the fractional differential and integral equations have a great development specially in the task of characterize the mechanical behavior of soft tissues [19] like the brain [25], liver [21], arteries [27,28,34] and even the human calcaneal fat pad [24].
We now consider the fractional generalization of the Standard Linear Solid (FSLS), show on Figure 1. For this purpose, is sufficient to replace the first order derivative with the fractional Caputo [35] derivative of order ν∈(0,1) in their constitutive equations. We obtain the following stress-strain relationship and the corresponding material functions are described latter.
The equation 2.9 is basically the same that in integer order, but here we replace the first derivative with the Caputo fractional differential operator
∗0Dνtσ(t)+e2ησ(t)=(e1+e2) ∗0Dνtε(t)+e1e2ηε(t) | (2.9) |
applying the Laplace transform to both sides of the equation 2.9 we obtain,
[sν+e2η]ˉσ(s)=[(e1+e2)sν+(e1e2η)]ˉε(s) | (2.10) |
solving for ˉε(s)
ˉε(s)=sν+α(e1+e2)sν+βˉσ(s) | (2.11) |
where α=e2η and β=e1e2η, applying the Laplace inverse transform and the convolution law, we have the analytical solution for FSLS model,
ε(t)=[δ(t)e1+e2+1(e1+e2)t∞∑n=1(−ζtν)nΓ(nν)+αtν−1e1+e2∞∑n=0(−ζtν)nΓ(ν(n+1))]∗σ(t) | (2.12) |
where δ(t) is the traditional Dirac's delta, ∗ is a convolution and ζ=βe1.
Now we proceed to the implementation of the FSLS to the artery modeling process, first we describe the relaxation modulus, the creep compliance and the complex modulus, all necessary for the mechanical one dimensional characterization on the material [36]. Next we briefly shown the process to the creation of the vectorized image and the exportation to CAD software that aloud to be treating like a solid with the mechanical properties and restrictions.
The values of the constants used on the research are taking for experimental creep relaxation test realized on [27] and are e1=0.68, e2=0.39, η=2.14 and ν=0.23. Now we describe the material model functions like the relaxation modulus, creep compliance and complex modulus. The relaxation modulus for the FSLS has the form,
G(t)=e1+e2⋅Eν[−(e2ηtν)] | (3.1) |
where
Eν,φ[(−e2ηtν)]=∞∑n=0(−e2ηtν)nΓ(νn+φ) | (3.2) |
is the Mittag-Lleffler function [20] with ν,φ∈R+ and e1η∈R. On Figure 2 are plot the relaxation modulus function for four fractional order values, and the constants value mentioned before.
In the same way, we obtain and plot the creep compliance function, for different fractional values ν, the creep compliance function J(t) have the form:
J(t)=μ+(1e1−μ)[1−Eν[−(e1e2μηtν)]] | (3.3) |
where μ=1e1+e2.
The complex modulus, present on Figure 2, complete the set of basic functions required for the mechanical characterization.
The axial dicom images are used to obtain a 2D geometry for every one of the slices, taking care on identify properly witch points generate each one of the segments, to do that its necessary to establish a consistent metric respect the patient's measure and an appropriate Hounsfield scale. The coordinates are localized and saved on a csv file. Now a spline curve can be generated through the geometric pattern, this way the cloud of points on each slice are limit by a close contour. This processes are repeat for the creation of each one of the slices and each one of the respective segments of the artery, the intima, the media and the adventicia. This procedure is illustrated in the left side of Figure 3.
Once the geometric patterns are due in all the set of axial images. Again a spline is applied to the set of slices, now to generate the 3D artery segment representation. Therefore this geometry is save on.iges format and export to CAD software, for the three layer solid representation. Now this is able to function properly in finite element method analysis software as see on the right side of Figure 3.
Like in all the others numerical methods, the precision of the method consist basically on the size of the step. If the element is the sufficient small the method converge to the require solution with minimal error. For that reason we need to do the finest mesh that can be possible in function that the processor is able to work.
The artery have a total volume of 0.2865 cm3 and are meshed with 654,977 eight node brick elements, i.e. the mesh consist on 2,000,000 element for cubic centimeter. This size of the mesh it's necessary to are secure that the finite element method converge to the require solution, because in other way the software can enter on a infinity loop or brings an non sense solution, from this number of elements the convergence is the same. The mechanical properties of the three segments are shown in table 1.
Omega g∗ | Omega g∗ | Omega k∗ | Omega k∗ | Frecuency |
real | imag | real | imag | Hz |
2.02E-11 | 4.32E-06 | 2.18E-11 | 3.94E-06 | 0.001 |
2.02E-09 | 4.32E-05 | 2.18E-09 | 0.000038 | 0.01 |
2.02E-07 | 0.000432 | 2.18E-07 | 0.000389 | 0.1 |
2.01E-05 | 0.004323 | 2.17E-05 | 0.003897 | 1.0 |
-0.056137 | 0.166779 | 0.116504 | -0.018474 | 23.0 |
0.113203 | 0.267329 | 0.313763 | 0.479758 | 100.0 |
0.989947 | 0.032453 | 0.514424 | -0.426401 | 350.0 |
The finite element method software are configured to realize the viscoelastic material routine by the property implementation of the frequency data test. Once the data are introduced on the software, we need to apply a load on the internal intima surface, simulating the pressure caused by a blood flow rate of 120/80 mmHg as shown on Figure 4 [37], and a constant load pressure on the exterior externa surface due to muscular compression originated by the muscles that round the artery, the temperature of the body will be consider constant, the extremes of the aorta are fixed by a constraint option and for last the interaction between the layers is set as a tie restriction.
The result of the solid's deformation is shown on top of Figure 5, where the simulation exhibit a tendency or pattern of the deformation route and not necessarily the real deformation, the behavior showed by the artery concord with those founded and predicted previously by [24,19].
In Figure 5 the efforts of von Mises also known as equivalent efforts are show since these are obtained from a relationship that combines the main efforts in an equivalent effort that can be used to compare with the effort of transfer of the analyzed material. The values of the von Mises stress obtained in this research are consistent with previously developed investigations in the experimental field where values of 0.213 MPa for blood pressure of 120mmHg are reported, which coincides with the results obtained by simulation using the viscoelastic fractional method.
In previous research it is founded that the area where the maximum stress values are presented, is distributed in the intimate layer of the artery and is located in the place where it changes its geometry, that is, where there is a change in artery curvature [40].
Finally, Figure 6 shows the distribution of internal pressure caused by blood flow where 0.03MPa pressure zones are identified in general and some 0.098MP maximum pressure zones, which is consistent with the results previously published by Holzapfel [41]
The results obtained were compared with experiments carried out in 2014, where fractional models have been used to characterize various soft tissues, showing that the parameters determined in the research are within the range of those previously found [24,25,19].
The stress distribution and the maximum values funded in the research concords with those previously reported by Holzapfel [40,41], using an hyperelastic model with prony series.
First we obtained the reconstruction of a segment of the aortic artery based on medical images obtained from a computerized axial tomography scanner, using the Hounsfield scale we could identify each of its three constituent layers (intima, media and adventitia). In addition, the process of exporting the medical image in a vectorized geometry was carried out with which it was possible to export to a solid form, that could be manipulated in a finite element software.
Compared with previous works where simulations of the biomechanical effects of the artery were performed using geometric idealizations, considering the layers of the artery as perfect cylinders, it was observed that when doing this what is had for the state of stress consists of a distribution perfectly symmetrical of the stresses, and in the case of the state of deformations in the same way there is a constant deformation in all directions of the solid. However, the geometry of the artery does not consist of a series of cylinders, so it was found in the development of the investigation that the distribution of stress has its local maximum, speaking of von Mises stress, at the point where the artery presents a change in curvature that generates a great deformation at that point, unlike to a uniform deformation, will end up affecting more to one end of the artery.
In this paper we shown that viscoelastic fractional models represents properly the mechanical behavior of the aortic artery, based on a uniaxial simple model.
In addition, it has been proven that with the viscoelastic fractional model, values similar to those previously provided in the literature are obtained without the use of prony series, which considerably reduces the computation time required.
We want to thankful the institutions that supported the present research project, Tecnológico Nacional de México / Instituto Tecnológico Superior de Cajeme, the Biomechanics Investigation Group from Universidad Tecnológica de la Habana, La Habana, Cuba and the Pontificia Universidad Católica de Valparaíso, Chile.
All authors declare no conflicts of interest in this paper.
[1] |
Y. Huang, F. Chen, L. Zhong, Stability analysis of a prey predator model with Holling type III response function incorporating a prey refuge, Appl. Math. Comput., 182 (2006), 672–683. https://doi.org/10.1016/j.amc.2006.04.030 doi: 10.1016/j.amc.2006.04.030
![]() |
[2] |
J. D. Reeve, Environmental variability, migration, and persistence in host-parasitoid systems, Am. Nat., 132 (1988), 810–836. https://doi.org/10.1086/284891 doi: 10.1086/284891
![]() |
[3] |
W. W. Murdoch, C. J. Briggs, R. M. Nisbet, W. S. C. Gurney, A. Stewart-Oaten, Aggregation and stability in met population models, Am. Nat., 140 (1992), 41–58. https://doi.org/10.1086/285402 doi: 10.1086/285402
![]() |
[4] |
M. M. Myerscough, M. Darwen, W. Hogarth, Stability, persistence and structural stability in a classical predator-prey model, Ecol. Model., 89 (1996), 31–42. https://doi.org/10.1016/0304-3800(95)00117-4 doi: 10.1016/0304-3800(95)00117-4
![]() |
[5] |
Q. L. Peng, L. S. Chen, Asymptotic behavior of the nonautonomous two-species Lotka-Volterra competition models, Comput. Math. Appl., 27 (1994), 53–60. https://doi.org/10.1016/0898-1221(94)90085-X doi: 10.1016/0898-1221(94)90085-X
![]() |
[6] |
F. Chen, Positive periodic solutions of neutral Lotka Volterra system with feedback control, Appl. Math. Comput., 162 (2005), 1279–1302. https://doi.org/10.1016/j.amc.2004.03.009 doi: 10.1016/j.amc.2004.03.009
![]() |
[7] |
B. Dubey, A prey-predator model with a reserved area, Nonlinear Anal. Model. Control, 12 (2007), 479–494. https://doi.org/10.15388/NA.2007.12.4.14679 doi: 10.15388/NA.2007.12.4.14679
![]() |
[8] |
B. Dubey, P. Chandra, P. Sinha, A model for fishery resource with reserve area, Nonlinear Anal. Real World Appl., 4 (2003), 625–637. https://doi.org/10.1016/S1468-1218(02)00082-2 doi: 10.1016/S1468-1218(02)00082-2
![]() |
[9] | C. M. Roberts, N. V. Polunin, Marine reserves: simple solutions to managing complex fisheries?, Ambio, 1993 (1993), 363–368. |
[10] |
Y. Takeuchi, N. Adachi, Existence and bifurcation of stable equilibrium in two-prey, one-predator communities, Bull. Math. Biol., 45 (1983), 877–900. https://doi.org/10.1016/S0092-8240(83)80067-6 doi: 10.1016/S0092-8240(83)80067-6
![]() |
[11] |
N. Wang, M. Zhao, H. Yu, C. Dai, B. Wang, P. Wang, Bifurcation behavior analysis in a predator-prey model, Discrete Dyn. Nat. Soc., 2016 (2016), 3565316. https://doi.org/10.1155/2016/3565316 doi: 10.1155/2016/3565316
![]() |
[12] |
J. Wang, J. Shi, J. Wei, Predator prey system with strong Allee effect in prey, J. Math. Biol., 62 (2011), 291–331. https://doi.org/10.1007/s00285-010-0332-1 doi: 10.1007/s00285-010-0332-1
![]() |
[13] |
S. R. Zhou, Y. F. Liu, G. Wang, The stability of predator prey systems subject to the Allee effects, Theor. Popul. Biol., 67 (2005), 23–31. https://doi.org/10.1016/j.tpb.2004.06.007 doi: 10.1016/j.tpb.2004.06.007
![]() |
[14] |
M. Yavuz, N. Sene, Stability analysis and numerical computation of the fractional predator prey model with the harvesting rate, Fractal Fractional, 4 (2020), 35. https://doi.org/10.3390/fractalfract4030035 doi: 10.3390/fractalfract4030035
![]() |
[15] |
M. Haque, A predator prey model with disease in the predator species only, Nonlinear Anal. Real World Appl., 11 (2010), 2224–2236. https://doi.org/10.1016/j.nonrwa.2009.06.012 doi: 10.1016/j.nonrwa.2009.06.012
![]() |
[16] |
L. Fang, J. Wang, The global stability and pattern formations of a predator prey system with consuming resource, Appl. Math. Lett., 58 (2016), 49–55. https://doi.org/10.1016/j.aml.2016.01.020 doi: 10.1016/j.aml.2016.01.020
![]() |
[17] |
B. E. Ainseba, M. Bendahmane, A. Noussair, A reaction diffusion system modeling predator prey with prey-taxis, Nonlinear Anal. Real World Appl., 9 (2008), 2086–2105. https://doi.org/10.1016/j.nonrwa.2007.06.017 doi: 10.1016/j.nonrwa.2007.06.017
![]() |
[18] |
S. Chen, J. Yu, Stability and bifurcation on predator-prey systems with nonlocal prey competition, Discrete Contin. Dyn. Syst., 38 (2018), 43. https://doi.org/10.3934/dcds.2018002 doi: 10.3934/dcds.2018002
![]() |
[19] |
S. Djilali, Pattern formation of a diffusive predator prey model with herd behavior and nonlocal prey competition, Math. Methods Appl. Sci., 43 (2020), 2233–2250. https://doi.org/10.1002/mma.6036 doi: 10.1002/mma.6036
![]() |
[20] |
J. D. Ferreira, S. H. Da Silva, V. S. H. Rao, Stability analysis of predator prey models involving cross-diffusion, Phys. D Nonlinear Phenom., 400 (2019), 132141. https://doi.org/10.1016/j.physd.2019.06.007 doi: 10.1016/j.physd.2019.06.007
![]() |
[21] |
S. Kant, V. Kumar, Stability analysis of predator prey system with migrating prey and disease infection in both species, Appl. Math. Model., 42 (2017), 509–539. https://doi.org/10.1016/j.apm.2016.10.003 doi: 10.1016/j.apm.2016.10.003
![]() |
[22] |
K. M. Owolabi, Numerical approach to chaotic pattern formation in diffusive predator prey system with Caputo fractional operator, Numer. Methods Partial Differ. Equations, 37 (2021), 131–151. https://doi.org/10.1002/num.22522 doi: 10.1002/num.22522
![]() |
[23] |
D. Song, C. Li, Y. Song, Stability and cross-diffusion-driven instability in a diffusive predator prey system with hunting cooperation functional response, Nonlinear Anal. Real World Appl., 54 (2020), 103106. https://doi.org/10.1016/j.nonrwa.2020.103106 doi: 10.1016/j.nonrwa.2020.103106
![]() |
[24] |
T. Zhang, Y. Xing, H. Zong, M. Han, Spatio-temporal dynamics of a reaction-diffusion system for a predator prey model with hyperbolic mortality, Nonlinear Dyn., 78 (2014), 265–277. https://doi.org/10.1007/s11071-014-1438-6 doi: 10.1007/s11071-014-1438-6
![]() |
[25] |
U. Ghosh, S. Pal, M. Banerjee, Memory effect on Bazykin prey-predator model: Stability and bifurcation analysis, Chaos Solitons Fractals, 143 (2021), 110531. https://doi.org/10.1016/j.chaos.2020.110531 doi: 10.1016/j.chaos.2020.110531
![]() |
[26] |
K. M. OWolabi, Computational dynamics of predator-prey model with the power-law kernel, Results Phys., 21 (2021), 103810. https://doi.org/10.1016/j.rinp.2020.103810 doi: 10.1016/j.rinp.2020.103810
![]() |
[27] |
W. Shatanawi, A. Raza, M. S. Arif, M. Rafiq, M. Bibi, M. Mohsin, Essential features preserving dynamics of stochastic Dengue model, Comput. Model. Eng. Sci., 126 (2021), 201–215. https://doi.org/10.32604/cmes.2021.012111 doi: 10.32604/cmes.2021.012111
![]() |
[28] |
W. Shatanawi, A. Raza, M. S. Arif, K. Abodayeh, M. Rafiq, M. Bibi, Design of nonstandard computational method for stochastic susceptible–infected–treated–recovered dynamics of coronavirus model, Adv. Differ. Equations, 2020 (2020), 1–15. https://doi.org/10.1186/s13662-019-2438-0 doi: 10.1186/s13662-019-2438-0
![]() |
[29] |
M. S. Arif, A. Raza, M. Rafiq, M. Bibi, J. N. Abbasi, A. Nazeer, U. Javed, Numerical simulations for stochastic computer virus propagation model, Comput. Mater. Contin., 62 (2020), 61–77. https://doi.org/10.32604/cmc.2020.08595 doi: 10.32604/cmc.2020.08595
![]() |
[30] |
S. A. Pasha, Y. Nawaz, M. S. Arif, The modified homotopy perturbation method with an auxiliary term for the nonlinear oscillator with discontinuity, J. Low Freq. Noise Vib. Active Control, 38 (2019), 1363–1373. https://doi.org/10.1177/0962144X18820454 doi: 10.1177/0962144X18820454
![]() |
[31] |
S. X. Wu, X. Y. Meng, Dynamics of a delayed predator-prey system with fear effect, herd behavior and disease in the susceptible prey, AIMS Math., 6 (2021), 3654–3685. https://doi.org/10.3934/math.2021218 doi: 10.3934/math.2021218
![]() |
[32] |
B. Ghanbari, On approximate solutions for a fractional prey–predator model involving the Atangana–Baleanu derivative, Adv. Differ. Equations, 2020 (2020), 1–24. https://doi.org/10.1186/s13662-019-2438-0 doi: 10.1186/s13662-019-2438-0
![]() |
[33] |
B. Ghanbari, On the modeling of the interaction between tumor growth and the immune system using some new fractional and fractional-fractal operators, Adv. Differ. Equations, 2020 (2020), 1–32. https://doi.org/10.1186/s13662-019-2438-0 doi: 10.1186/s13662-019-2438-0
![]() |
[34] |
B. Ghanbari, A fractional system of delay differential equation with nonsingular kernels in modeling hand-foot-mouth disease, Adv. Differ. Equation, 2020 (2020), 536. https://doi.org/10.1186/s13662-020-02993-3 doi: 10.1186/s13662-020-02993-3
![]() |
[35] |
B. Ghanbari, On novel non differentiable exact solutions to local fractional Gardner's equation using an effective technique, Math. Methods Appl. Sci., 44 (2021), 4673–4685. https://doi.org/10.1002/mma.7060 doi: 10.1002/mma.7060
![]() |
[36] |
B. Ghanbari, A new model for investigating the transmission of infectious diseases in a prey-predator system using a non-singular fractional derivative, Math. Methods Appl. Sci., 2021 (2021), forthcoming. https://doi.org/10.1002/mma.7412 doi: 10.1002/mma.7412
![]() |
[37] |
B. Ghanbari, A. Atangana, Some new edge detecting techniques based on fractional derivatives with non-local and non-singular kernels, Adv. Differ. Equation, 435 (2020). https://doi.org/10.1186/s13662-020-02890-9. doi: 10.1186/s13662-020-02890-9
![]() |
[38] |
S. Saha, A. Maiti, G. P. Samanta, A Michaelis–Menten predator–prey model with strong Allee effect and disease in prey incorporating prey refuge, Int. J. Bifurcation Chaos, 28 (2018), 1850073. https://doi.org/10.1142/S0218127418500736 doi: 10.1142/S0218127418500736
![]() |
[39] |
S. Saha, G. P. Samanta, Analysis of a predator–prey model with herd behavior and disease in prey incorporating prey refuge, Int. J. Biomath., 12 (2019), 1950007. https://doi.org/10.1142/S1793524519500074 doi: 10.1142/S1793524519500074
![]() |
[40] |
S. Saha, G. P. Samanta, A prey–predator system with disease in prey and cooperative hunting strategy in predator, J. Phys. A Math. Theor., 53 (2020), 485601. https://doi.org/10.1088/1751-8121/abbc7b doi: 10.1088/1751-8121/abbc7b
![]() |
[41] |
A. Mondal, A. K. Pal, G. P. Samanta, On the dynamics of evolutionary Leslie-Gower predator-prey eco-epidemiological model with disease in predator, Ecol. Genet. Genomics, 10 (2019), 100034. https://doi.org/10.1016/j.egg.2018.11.002 doi: 10.1016/j.egg.2018.11.002
![]() |
[42] |
S. Sharma, G. P. Samanta, Analysis of a two prey one predator system with disease in the first prey population, Int. J. Dyn. Control, 3 (2015), 210–224. https://doi.org/10.1007/s40435-014-0107-4 doi: 10.1007/s40435-014-0107-4
![]() |
[43] |
S. Sharma, G. P. Samanta, A Leslie–Gower predator–prey model with disease in prey incorporating a prey refuge, Chaos Solitons Fractals, 70 (2015), 69–84. https://doi.org/10.1016/j.chaos.2014.11.010 doi: 10.1016/j.chaos.2014.11.010
![]() |
[44] |
B. Ghanbari, Chaotic behaviors of the prevalence of an infectious disease in a prey and predator system using fractional derivatives, Math. Methods Appl. Sci., 44 (2021), 9998–10013. https://doi.org/10.1002/mma.7386 doi: 10.1002/mma.7386
![]() |
[45] |
A. Ejaz, Y. Nawaz, M. S. Arif, D. S. Mashat, K. Abodayeh, Stability analysis of predator-prey system with consuming resource and disease in predator species, CMES Comput. Model. Eng. Sci., 132 (2022), 489–506. https://doi.org/10.32604/cmes.2022.019440 doi: 10.32604/cmes.2022.019440
![]() |
[46] | M. S. Arif, K. Abodayeh, A. Ejaz, Computational modeling of reaction-diffusion COVID-19 model having isolated compartment, CMES Comput. Model. Eng. Sci., 2022 (2022), 1–25. |
[47] |
Y. Peng, G. Zhang, Dynamics analysis of a predator prey model with herd behavior and nonlocal prey competition, Math. Comput. Simul., 170 (2020), 366–378. https://doi.org/10.1016/j.matcom.2019.11.012 doi: 10.1016/j.matcom.2019.11.012
![]() |
1. | Qiyin Lv, Yuan Zhang, Ping He, Yao Chen, Yiwen Wang, Negative Poisson’s ratio artificial blood vessel skeleton multi-objective optimization design and numerical modelling, 2025, 0954-4062, 10.1177/09544062241312888 |
Omega g∗ | Omega g∗ | Omega k∗ | Omega k∗ | Frecuency |
real | imag | real | imag | Hz |
2.02E-11 | 4.32E-06 | 2.18E-11 | 3.94E-06 | 0.001 |
2.02E-09 | 4.32E-05 | 2.18E-09 | 0.000038 | 0.01 |
2.02E-07 | 0.000432 | 2.18E-07 | 0.000389 | 0.1 |
2.01E-05 | 0.004323 | 2.17E-05 | 0.003897 | 1.0 |
-0.056137 | 0.166779 | 0.116504 | -0.018474 | 23.0 |
0.113203 | 0.267329 | 0.313763 | 0.479758 | 100.0 |
0.989947 | 0.032453 | 0.514424 | -0.426401 | 350.0 |
Omega g∗ | Omega g∗ | Omega k∗ | Omega k∗ | Frecuency |
real | imag | real | imag | Hz |
2.02E-11 | 4.32E-06 | 2.18E-11 | 3.94E-06 | 0.001 |
2.02E-09 | 4.32E-05 | 2.18E-09 | 0.000038 | 0.01 |
2.02E-07 | 0.000432 | 2.18E-07 | 0.000389 | 0.1 |
2.01E-05 | 0.004323 | 2.17E-05 | 0.003897 | 1.0 |
-0.056137 | 0.166779 | 0.116504 | -0.018474 | 23.0 |
0.113203 | 0.267329 | 0.313763 | 0.479758 | 100.0 |
0.989947 | 0.032453 | 0.514424 | -0.426401 | 350.0 |