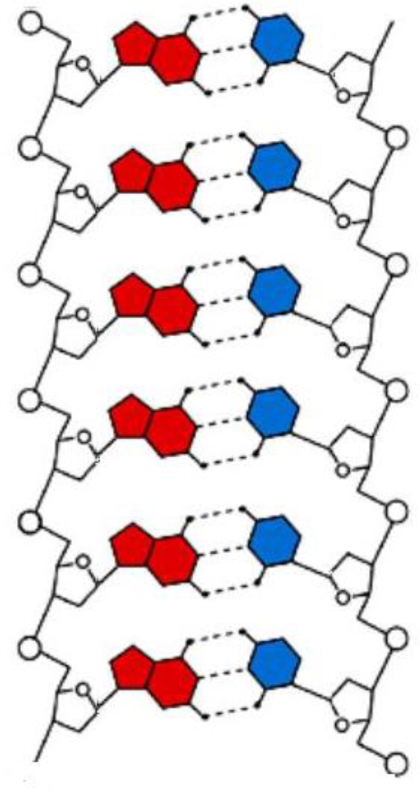
This paper presents a novel teleoperation system using Electroencephalogram (EEG) to control the motion of a wheeled mobile robot (WMR). Different from the other traditional motion controlling method, the WMR is braked with the EEG classification results. Furthermore, the EEG will be induced by using the online BMI (Brain Machine Interface) system, and adopting the non-intrusion induced mode SSVEP (steady state visually evoked potentials). Then, user's motion intention can be recognized by canonical correlation analysis (CCA) classifier, which will be converted into motion commands of the WMR. Finally, the teleoperation technique is utilized to manage the information of the movement scene and adjust the control instructions based on the real-time information. Bezier curve is used to parameterize the path planning of the robot, and the trajectory can be adjusted in real time by EEG recognition results. A motion controller based on error model is proposed to track the planned trajectory by using velocity feedback control, providing excellent track tracking performance. Finally, the feasibility and performance of the proposed teleoperation brain-controlled WMR system are verified using demonstration experiments.
Citation: Su-na Zhao, Yingxue Cui, Yan He, Zhendong He, Zhihua Diao, Fang Peng, Chao Cheng. Teleoperation control of a wheeled mobile robot based on Brain-machine Interface[J]. Mathematical Biosciences and Engineering, 2023, 20(2): 3638-3660. doi: 10.3934/mbe.2023170
[1] | Ludmila V. Yakushevich, Larisa A. Krasnobaeva . Double energy profile of pBR322 plasmid. AIMS Biophysics, 2021, 8(2): 221-232. doi: 10.3934/biophy.2021016 |
[2] | Larisa A. Krasnobaeva, Ludmila V. Yakushevich . DNA kinks behavior in the potential pit-trap. AIMS Biophysics, 2022, 9(2): 130-146. doi: 10.3934/biophy.2022012 |
[3] | Roman Marks . Bubble mediated polymerization of RNA and DNA. AIMS Biophysics, 2022, 9(2): 96-107. doi: 10.3934/biophy.2022009 |
[4] | Audrey Lejart, Gilles Salbert, Sébastien Huet . Cytosine hydroxymethylation by TET enzymes: From the control of gene expression to the regulation of DNA repair mechanisms, and back. AIMS Biophysics, 2018, 5(3): 182-193. doi: 10.3934/biophy.2018.3.182 |
[5] | Roman Marks, Piotr H. Pawłowski . Rotational-electric principles of RNA/DNA and viability. AIMS Biophysics, 2023, 10(3): 385-400. doi: 10.3934/biophy.2023023 |
[6] | Théo Lebeaupin, Hafida Sellou, Gyula Timinszky, Sébastien Huet . Chromatin dynamics at DNA breaks: what, how and why?. AIMS Biophysics, 2015, 2(4): 458-475. doi: 10.3934/biophy.2015.4.458 |
[7] | Yuri M. Moshkin . Chromatin—a global buffer for eukaryotic gene control. AIMS Biophysics, 2015, 2(4): 531-554. doi: 10.3934/biophy.2015.4.531 |
[8] | Arsha Moorthy, Alireza Sarvestani, Whitney Massock, Chamaree de Silva . Twisting and extension: Application of magnetic tweezers to DNA studies. AIMS Biophysics, 2023, 10(3): 317-346. doi: 10.3934/biophy.2023020 |
[9] | Larisa I. Fedoreyeva, Boris F. Vanyushin . Regulation of DNA methyltransferase gene expression by short peptides in nicotiana tabacum regenerants. AIMS Biophysics, 2021, 8(1): 66-79. doi: 10.3934/biophy.2021005 |
[10] | Michael J. Hamilton, Matthew D. Young, Silvia Sauer, Ernest Martinez . The interplay of long non-coding RNAs and MYC in cancer. AIMS Biophysics, 2015, 2(4): 794-809. doi: 10.3934/biophy.2015.4.794 |
This paper presents a novel teleoperation system using Electroencephalogram (EEG) to control the motion of a wheeled mobile robot (WMR). Different from the other traditional motion controlling method, the WMR is braked with the EEG classification results. Furthermore, the EEG will be induced by using the online BMI (Brain Machine Interface) system, and adopting the non-intrusion induced mode SSVEP (steady state visually evoked potentials). Then, user's motion intention can be recognized by canonical correlation analysis (CCA) classifier, which will be converted into motion commands of the WMR. Finally, the teleoperation technique is utilized to manage the information of the movement scene and adjust the control instructions based on the real-time information. Bezier curve is used to parameterize the path planning of the robot, and the trajectory can be adjusted in real time by EEG recognition results. A motion controller based on error model is proposed to track the planned trajectory by using velocity feedback control, providing excellent track tracking performance. Finally, the feasibility and performance of the proposed teleoperation brain-controlled WMR system are verified using demonstration experiments.
It is generally accepted that the transcription bubbles, which are small, locally untwisted regions (or distortions) of the double helix [1]–[4], are formed at the initial stage of the transcription and then move along the DNA molecule. On the other hand, the DNA molecule is considered by many researchers as a medium in which nonlinear conformational distortions (or solitary waves) can arise and propagate [5],[6]. This amazing property of DNA associates such a purely biological object as DNA with numerous nonlinear, mostly physical systems. Mathematically the movement of the nonlinear distortions are modeled by nonlinear differential equations, in particular, by the nonlinear sine-Gordon equation:
having, among others, one-soliton solutions (kinks) [7],[8]:
where
Beginning with the work of Englander et al. [9], kinks have been actively used to model nonlinear conformational distortions in DNA, of which transcription bubbles are a special case. Currently, to model the dynamics of transcription bubbles, different modifications of the Englander model are used. Usually, they consist of a system of nonlinear differential equations with kink-like solutions. These equations contain many DNA dynamic parameters, such as the moments of inertia of nitrous bases, the distances between base pairs, the distances from the centers of mass of bases to sugar-phosphate chains, the rigidity of the sugar-phosphate backbone and the coefficients characterizing interactions between bases within pairs. Taken together, the equations and their parameters constitute the so-called dimensional model.
To reduce the number of parameters, it is much more convenient and efficient to use a dimensionless analog of a dimensional model. It is believed that the dimensionless analog not only makes it possible to noticeably reduce the number of parameters, but it also significantly simplifies the analysis of equations and methods for finding their solutions. In addition, the results obtained within the dimensionless model are valid not only for DNA, but also for other similar nonlinear media.
In this work, we have constructed a dimensionless analog of the nonlinear differential equations simulating the dynamics of transcription bubbles. We show that carrying out the dimensionless procedure really leads to a decrease in the number of model parameters, as well as to justification of the validity of applying the perturbation theory and the McLaughlin-Scott method [10],[11] based on it, which greatly facilitates finding solutions to model equations, their analysis and understanding the nature of the motion of transcription bubbles.
Methods for reducing model equations to a dimensionless form are varied [12]–[15]. To simplify calculations, we limited ourselves to the case of homogeneous (synthetic) DNA. In this case, the desired analog can be obtained using a simple transformation from the variables z and t to the new variables ξ and τ:
which is accompanied by additional requirements for the transformation coefficients. We obtained dimensionless model equations, estimated their parameters, found one-soliton solutions (kinks) imitating transcription bubbles and justified the McLaughlin-Scott method, which made it possible to
calculate the dimensionless velocity of transcription bubbles and to plot their trajectories in the {ξ, τ} plane.
Let us consider a homogeneous case when one of the two polynucleotide chains contains only one type of nitrous bases, such as adenines, and the second chain contains only thymines (Figure 1).
In this case, more general model equations proposed in [16] to describe transcription bubbles dynamics take the following form:
Here
Equations (4)–(5) take into account only one type of internal DNA motion, namely, angular displacements of the nitrous bases, which is believed to make the main contribution to the opening base pairs and formation of the transcription babbles. An alternative opinion developed in [17] is that the transverse displacements are more important. The model taking into account only transverse displacements is known as a BP model. In general, a more accurate model imitating the formation dynamics of the transcription bubbles should include both the transverse and angular displacements of the bases, as well as their longitudinal displacements [18].
Despite the limitations of the homogeneous case, equations (4)–(5) contain a fairly large number of dynamic parameters. However, they are only a part of the vast and complex space of parameters used in DNA melting and deformation models [19]–[21]. Therefore, the question of finding methods to reduce the number of parameters seems to be very relevant.
Restricting themselves to the continuum approximation and taking into account the features of the distribution of interactions within the DNA molecule results in the following: the presence of «weak» hydrogen bonds between nitrous bases inside complementary pairs and «strong» valence interactions along the sugar-phosphate chains; equations (4)–(5) can be reduced (in the first approximation) to two independent equations:
Here
Homogeneous sequence type | I×10−44 (kg·m2) | K′×10−18 (N·m) | V×10−20 (J) | β×10−34 (J·s) | M0×10−22 (J) |
poly(A) | 7.61 | 2.35 | 2.09 | 4.25 | 3.12 |
poly(T) | 4.86 | 1.61 | 1.43 | 2.91 | 3.12 |
In a particular case, when the effects of dissipation and the action of a constant torsion moment are small (
Let us write Hamiltonians corresponding to equations (8)–(9):
and exact one-soliton solutions of equations (8)–(9) – kinks:
Here,
The 3D graphs of the two DNA kinks (12)–(13) are presented in Figure 2. They have a canonical kink-like shape, which has been observed in a variety of media, including the mechanical chains of coupled pendulums [24], optical media [25], the chains of Josephson junctions [26]–[29], crystals [30], superfluid media [27], the Earth's crust [31], ferromagnetic and antiferromagnetic materials [32],[33] and biological molecules [34]–[36].
Substituting equation (12) into equation (10) and equation (13) into equation (11), we find formulas for the kink total energies EA and ET:
where EA and ET are the the kink rest energies:
In the case of low velocities (
where
Homogeneous sequence type | d (nm) | E0×10−18 (J) | m×10−25 (kg) |
poly(A) | 3.61 | 1.77 | 2.48 |
poly(T) | 3.61 | 1.21 | 1.58 |
For the general case, when
However, unlike
Here,
Having determined the kink coordinates from the relations
Let us consider the transformation from variables z and t to new variables ξ and τ according to equation (3). To find the transformation coefficients σ and η, substitute equation (22) into equations (6) and (7), respectively:
Next, let us multiply and divide
and require the fulfillment of two conditions:
from which we find the transformation coefficients σ and η:
In the new variables, equations (26) and (27) take the following form:
where
It can be seen that the number of parameters in the first of the two dimensionless model equations has decreased from five to two. In the second equation, the number of parameters has not changed. Taking into account the fact that the torsion moment in both equations is the same, the total number of parameters became equal to six, which is less than the nine parameters of the dimensional model (6)–(7).
We estimated the values of the coefficients of dimensionless model equations (30)–(31) and presented them in Table 3.
Coefficients | Estimated values |
0.64 | |
0.68 | |
0.68 | |
0.01 | |
0.007 | |
0.01 |
Note that, in the case of dimensional
In the particular case when the effects of dissipation and external action are negligibly small equation (30) takes the form of the classical canonical sine-Gordon equation (1), which has no coefficients:
At the same time equation (31) retains all three coefficients:
Hamiltonians corresponding to equations (32) and (33) have the following form:
To obtain one-soliton solutions of equations (32), (33), we take dimensional solutions (11) and (12) and rewrite them with new variables:
Substituting the solutions into the Hamiltonians (34), (35) we find the total kink energies:
where
In the general case, when
where
In this work, we have constructed the dimensionless analog of the model simulating the dynamics of transcription bubbles and demonstrated the method of constructing in detail. As a basic dimensional model, we used a system of nonlinear differential equations proposed in [16], the one-soliton solutions of which (kinks) were interpreted as mathematical images of transcription bubbles. The main results, including equations of motion, kink-like solutions, kink rest energy, and total kink energy obtained for the dimensionless model, are presented in Table 4.
Parameters | Model characteristics | Kink movement in the poly(A) chain | Kink movement in the poly(T) chain |
Equations of motion | |||
Hamiltonian | |||
Kink-like solution | |||
Total energy | |||
Rest energy | |||
Equations of motion | |||
Kink-like solution | |||
Equation for kink velocity |
For comparison, we present in Table 5, similar results obtained for the corresponding dimensional model.
Parameters | Model characteristics | Kink movement in the poly(A) chain | Kink movement in the poly(T) chain |
Equations of motion | |||
Hamiltonian | |||
Kink-like solution | |||
Total energy | |||
Rest energy | |||
Equations of motion | |||
Kink-like solution | |||
Equation for kink velocity |
It can be seen that the dimensionless model has advantages over the dimensional one. Indeed, carrying out the procedure of transformation of the dimensional model to the dimensionless one leads to the decrease in the number of model parameters from 9 to 6. Moreover, in the framework of the dimensionless analog, it became obvious that the coefficients in the terms simulating the dissipation effects and the action of a constant torsion moment are really small, which proves the validity of the application of the perturbation theory and the method of McLaughlin and Scott.
It should be noted, however, that in order to simplify calculations and to present the procedure of transition to dimensionless form more clear, we limited ourselves to the case of homogeneous (synthetic) DNA. Obviously, the next step in the development of this direction can be the construction of a dimensionless analog for the case of inhomogeneous DNA. This will provide an answer to the question of whether the advantages of the dimensionless model will be preserved in the inhomogeneous case.
One more step could be the improvement if the dimensional model itself by removing the limitations and simplifications detailed in Section 2. Finally, one could consider the issue of DNA kinks being created by mutual DNA-DNA interactions, which were considered theoretically in [38],[39], and experimentally in [40].
[1] |
Z. Li, W. Yuan, S. Zhao, Z. Yu, Y. Kang, C. P. Chen, Brain-actuated control of dual-arm robot manipulation with relative motion, IEEE Trans. Cognit. Dev. Syst., 11 (2017), 51–62. https://doi.org/10.1109/TCDS.2017.2770168 doi: 10.1109/TCDS.2017.2770168
![]() |
[2] |
Y. Yuan, W. Su, Z. Li, G. Shi, Brain-computer interface-based stochastic navigation and control of a semiautonomous mobile robot in indoor environments, IEEE Trans. Cognit. Dev. Syst., 11 (2018), 129–141. https://doi.org/10.1109/TCDS.2018.2885774 doi: 10.1109/TCDS.2018.2885774
![]() |
[3] |
Y. Chae, J. Jeong, S. Jo, Toward brain-actuated humanoid robots: asynchronous direct control using an EEG-based BCI, IEEE Trans. Rob., 28 (2012), 1131–1144. https://doi.org/10.1109/TRO.2012.2201310 doi: 10.1109/TRO.2012.2201310
![]() |
[4] |
L. Tonin, F. C. Bauer, J. D. R. Millan, The role of the control framework for continuous teleoperation of a brain-machine interface-driven mobile robot, IEEE Trans. Rob., 36 (2019), 78–91. https://doi.org/10.1109/TRO.2019.2943072 doi: 10.1109/TRO.2019.2943072
![]() |
[5] |
S. Zhao, Z. Li, R. Cui, Y. Kang, F. Sun, R. Song, Brain-machine interfacing-based teleoperation of multiple coordinated mobile robots, IEEE Trans. Ind. Electron., 64 (2016), 5161–5170. https://doi.org/10.1109/TIE.2016.2606089 doi: 10.1109/TIE.2016.2606089
![]() |
[6] |
X. Deng, Z. Yu, C. Lin, Z. Gu, Y. Li, A bayesian shared control approach for wheelchair robot with brain machine interface, IEEE Trans. Neural Syst. Rehabil. Eng., 28 (2019), 328–338. https://doi.org/10.1109/TNSRE.2019.2958076 doi: 10.1109/TNSRE.2019.2958076
![]() |
[7] |
Z. Li, S. Zhao, J. Duan, C. Y. Su, C. Yang, X. Zhao, Human cooperative wheelchair with brain-machine interaction based on shared control strategy, IEEE/ASME Trans. Mechatron., 22 (2016), 185–195. https://doi.org/10.1109/TMECH.2016.2606642 doi: 10.1109/TMECH.2016.2606642
![]() |
[8] |
B. Rebsamen, E. Burdet, C. Guan, H. Zhang, C. Teo, Q. Zeng, et al., Controlling a wheelchair indoors using thought, IEEE Intell. Syst., 22 (2007), 18–24. https://doi.org/10.1109/MIS.2007.26 doi: 10.1109/MIS.2007.26
![]() |
[9] |
E. Yin, Z. Zhou, J. Jiang, Y. Yu, D. Hu, A dynamically optimized SSVEP brain-computer interface (BCI) speller, IEEE Trans. Biomed. Eng., 62 (2014), 1147–1456. https://doi.org/10.1109/TBME.2014.2320948 doi: 10.1109/TBME.2014.2320948
![]() |
[10] |
T. Vouga, K. Z. Zhuang, J. Olivier, M. A. Lebedev, M. A. Nicolelis, M. Bouri, et al., EXiO-A brain-controlled lower limb exoskeleton for rhesus macaques, IEEE Trans. Neural Syst. Rehabil. Eng., 25 (2017), 131–141. https://doi.org/10.1109/TNSRE.2017.2659654 doi: 10.1109/TNSRE.2017.2659654
![]() |
[11] |
F. Janabi-Sharifi, I. Hassanzadeh, Experimental analysis of mobile-robot teleoperation via shared impedance control, IEEE Trans. Syst. Man Cybern. Part B Cybern., 41 (2010), 591–606. https://doi.org/10.1109/TSMCB.2010.2073702 doi: 10.1109/TSMCB.2010.2073702
![]() |
[12] |
C. Escolano, J. M. Antelis, J. Minguez, A telepresence mobile robot controlled with a nonoimvasive brain-computer interface, IEEE Trans. Syst. Man Cybern. Part B Cybern., 42 (2011), 793–804. https://doi.org/10.1109/TSMCB.2011.2177968 doi: 10.1109/TSMCB.2011.2177968
![]() |
[13] |
I. Iturrate, J. M. Antelis, A. Kubler, J. Minguez, A noninvasive brain-actuated wheelchair based on a P300 neurophysiological protocol and automated navigation, IEEE Trans. Rob., 25 (2009), 614–627. https://doi.org/10.1109/TRO.2009.2020347 doi: 10.1109/TRO.2009.2020347
![]() |
[14] |
A. Kelly, N. Chan, H. Herman, D. Huber, R. Meyers, P. Rander, et al., Real-time photorealistic virtualized reality interface for remote mobile robot control, Int. J. Rob. Res., 30 (2011), 384–404. https://doi.org/10.1177/0278364910383724 doi: 10.1177/0278364910383724
![]() |
[15] |
M. Lepetic, G. Klancar, I. Skrjanc, D. Matko, B. Potocnik, Time optimal path planning considering acceleration limits, Rob. Auton. Syst., 45 (2003), 199–210. https://doi.org/10.1016/j.robot.2003.09.007 doi: 10.1016/j.robot.2003.09.007
![]() |
[16] |
T. C. Liang, J. S. Liu, G. T. Hung, Y. Z. Chang, Practical and flexible path planning for car-like mobile robot using maximal-curvature cubic spiral, Rob. Auton. Syst., 52 (2005), 312–335. https://doi.org/10.1016/j.robot.2005.05.001 doi: 10.1016/j.robot.2005.05.001
![]() |
[17] |
E. Papadopoulos, I. Papadimitriou, I. Poulakakis, Polynomial-based obstacle avoidance techniques for nonholonomic mobile manipulator systems, Rob. Auton. Syst., 51 (2005), 229–247. https://doi.org/10.1016/j.robot.2005.03.006 doi: 10.1016/j.robot.2005.03.006
![]() |
[18] |
L. Fowler, J. Rogers, Bzier curve path planning for parafoil terminal guidance, J. Aerosp. Inf. Syst., 11 (2014), 300–315. https://doi.org/10.2514/1.I010124 doi: 10.2514/1.I010124
![]() |
[19] |
J. R. Wolpaw, N. Birbaumer, D. J. McFarland, G. Pfurtscheller, T. M. Vaughan, Brain-computer interfaces for communication and control, Clin. Neurophysiol., 113 (2002), 767–791. https://doi.org/10.1016/S1388-2457(02)00057-3 doi: 10.1016/S1388-2457(02)00057-3
![]() |
[20] |
E. Candes, J. Romberg, T. Tao, Robust uncertainty principles: Exact signal reconstruction from highly incomplete frequency information, IEEE Trans. Inf. Theory, 52 (2006), 489–509. https://doi.org/10.1109/TIT.2005.862083 doi: 10.1109/TIT.2005.862083
![]() |
[21] |
J. W. Choi, R. E. Curry, G. H. Elkaim, Continuous curvature path generation based on Bzier curves for autonomous vehicles, IAENG Int. J. Appl. Math., 40 (2010), 91–101. https://doi.org/10.1016/j.cej.2005.12.011 doi: 10.1016/j.cej.2005.12.011
![]() |
[22] |
K. G. Jolly, R. S. Kumar, R. vijayakumar, A Bezier curve based path planning in a multi-agent robot soccer system without violating the acceleration limits, Rob. Auton. Syst., 57 (2009), 23–33. https://doi.org/10.1016/j.robot.2008.03.009 doi: 10.1016/j.robot.2008.03.009
![]() |
[23] |
Z. Sun, F. Li, X. Duan, L. Jin, Y. Lian, S. Liu, et al., A novel adaptive iterative learning control approach and human-in-the-loop control pattern for lower limb rehabilitation robot in disturbances environment, Auton. Rob., 45 (2021), 595–610. https://doi.org/10.1007/s10514-021-09988-3 doi: 10.1007/s10514-021-09988-3
![]() |
[24] |
L. Jin, J. Li, Z. Sun, J. Lu, F. Wang, Neural dynamics for computing perturbed nonlinear equations applied to ACP-based lower limb motion intention recognition, IEEE Trans. Syst. Man Cybern.: Syst., 52 (2021), 5105–5113. https://doi.org/10.1109/TSMC.2021.3114213 doi: 10.1109/TSMC.2021.3114213
![]() |
[25] |
Z. Sun, G. Wang, L. Jin, C. Cheng, B. Zhang, J. Yu, Noise-suppressing zeroing neural network for online solving time-varying matrix square roots problems: A control-theoretic approach, Expert Syst. Appl., 192 (2022), 116272. https://doi.org/10.1016/j.eswa.2021.116272 doi: 10.1016/j.eswa.2021.116272
![]() |
[26] |
Z. Sun, T. Shi, L. Jin, B. Zhang, Z. Pang, J. Yu, Discrete-time zeroing neural network of O(τ4) pattern for online solving time-varying nonlinear optimization problem: Application to manipulator motion generation, J. Franklin Inst., 358 (2021), 7203–7220. https://doi.org/10.1016/j.jfranklin.2021.07.006 doi: 10.1016/j.jfranklin.2021.07.006
![]() |
[27] |
W. Qi, H. Su, A cybertwin based multimodal network for ecg patterns monitoring using deep learning, IEEE Trans. Ind. Inf., 18 (2022), 6663–6670. https://doi.org/10.1109/TII.2022.3159583 doi: 10.1109/TII.2022.3159583
![]() |
[28] |
K. Liu, Y. Liu, Y. Zhang, L. Wei, Z. Sun, L. Jin, Five-step discrete-time noise-tolerant zeroing neural network model for time-varying matrix inversion with application to manipulator motion generation, Eng. Appl. Artif. Intell., 103 (2021), 104306. https://doi.org/10.1016/j.engappai.2021.104306 doi: 10.1016/j.engappai.2021.104306
![]() |
[29] |
H. Su, Y. Hu, H. R. Karimi, A. Knoll, G. Ferrigno, E. D. Momi, Improved recurrent neural network-based manipulator control with remote center of motion constraints: Experimental results, Neural Networks, 131 (2020), 291–299. https://doi.org/10.1016/j.neunet.2020.07.033 doi: 10.1016/j.neunet.2020.07.033
![]() |
[30] |
H. Su, W. Qi, Y. Schmirander, S. E. Ovur, S. Cai, X. Xiong, A human activity-aware shared control solution for medical human–robot interaction, Assem. Autom., 42 (2022), 388–394. https://doi.org/10.1108/AA-12-2021-0174 doi: 10.1108/AA-12-2021-0174
![]() |
[31] |
H. Su, A. Marian, S. E. Ovur, A. Menciassi, G. Ferrigno, E. D. Momi, Toward teaching by demonstration for robot-assisted minimally invasive surgery, IEEE Trans. Autom. Sci. Eng., 18 (2021), 484–494. https://doi.org/10.1109/TASE.2020.3045655 doi: 10.1109/TASE.2020.3045655
![]() |
[32] |
J. Chen, H. Qiao, Motor-cortex-like recurrent neural network and multitask learning for the control of musculoskeletal systems, IEEE Trans. Cognit. Dev. Syst., 14 (2020), 424–436. https://doi.org/10.1109/TCDS.2020.3045574 doi: 10.1109/TCDS.2020.3045574
![]() |
[33] |
H. Su, S. E. Ovur, X. Zhou, W. Qi, G. Ferrigno, E. D. Momi, Depth vision guided hand gesture recognition using electromyographic signals, Adv. Rob., 34 (2020), 985–997. https://doi.org/10.1080/01691864.2020.1713886 doi: 10.1080/01691864.2020.1713886
![]() |
[34] |
H. Su, W. Qi, C. Yang, J. Sandoval, G. Ferrigno, E. De Momi, Deep neural network approach in robot tool dynamics identification for bilateral teleoperation, IEEE Rob. Autom. Lett., 5 (2020), 2943–2949. https://doi.org/10.1109/LRA.2020.2974445 doi: 10.1109/LRA.2020.2974445
![]() |
[35] |
J. Chen, H. Qiao, Muscle-synergies-based neuromuscular control for motion learning and generalization of a musculoskeletal system, IEEE Trans. Syst. Man Cybern.: Syst., 51 (2020), 3993–4006. https://doi.org/10.1109/TSMC.2020.2966818 doi: 10.1109/TSMC.2020.2966818
![]() |
1. | Mikhail I. Drobotenko, Oksana M. Lyasota, Jose Luis Hernandez-Caceres, Roberto Rodriguez Labrada, Alexandr A. Svidlov, Аnna A. Dorohova, Mikhail G. Baryshev, Yury D. Nechipurenko, Luis Velázquez Pérez, Stepan S. Dzhimak, Abnormal open states patterns in the ATXN2 DNA sequence depends on the CAG repeats length, 2024, 276, 01418130, 133849, 10.1016/j.ijbiomac.2024.133849 |
Homogeneous sequence type | I×10−44 (kg·m2) | K′×10−18 (N·m) | V×10−20 (J) | β×10−34 (J·s) | M0×10−22 (J) |
poly(A) | 7.61 | 2.35 | 2.09 | 4.25 | 3.12 |
poly(T) | 4.86 | 1.61 | 1.43 | 2.91 | 3.12 |
Homogeneous sequence type | d (nm) | E0×10−18 (J) | m×10−25 (kg) |
poly(A) | 3.61 | 1.77 | 2.48 |
poly(T) | 3.61 | 1.21 | 1.58 |
Coefficients | Estimated values |
0.64 | |
0.68 | |
0.68 | |
0.01 | |
0.007 | |
0.01 |
Parameters | Model characteristics | Kink movement in the poly(A) chain | Kink movement in the poly(T) chain |
Equations of motion | |||
Hamiltonian | |||
Kink-like solution | |||
Total energy | |||
Rest energy | |||
Equations of motion | |||
Kink-like solution | |||
Equation for kink velocity |
Parameters | Model characteristics | Kink movement in the poly(A) chain | Kink movement in the poly(T) chain |
Equations of motion | |||
Hamiltonian | |||
Kink-like solution | |||
Total energy | |||
Rest energy | |||
Equations of motion | |||
Kink-like solution | |||
Equation for kink velocity |
Homogeneous sequence type | I×10−44 (kg·m2) | K′×10−18 (N·m) | V×10−20 (J) | β×10−34 (J·s) | M0×10−22 (J) |
poly(A) | 7.61 | 2.35 | 2.09 | 4.25 | 3.12 |
poly(T) | 4.86 | 1.61 | 1.43 | 2.91 | 3.12 |
Homogeneous sequence type | d (nm) | E0×10−18 (J) | m×10−25 (kg) |
poly(A) | 3.61 | 1.77 | 2.48 |
poly(T) | 3.61 | 1.21 | 1.58 |
Coefficients | Estimated values |
0.64 | |
0.68 | |
0.68 | |
0.01 | |
0.007 | |
0.01 |
Parameters | Model characteristics | Kink movement in the poly(A) chain | Kink movement in the poly(T) chain |
Equations of motion | |||
Hamiltonian | |||
Kink-like solution | |||
Total energy | |||
Rest energy | |||
Equations of motion | |||
Kink-like solution | |||
Equation for kink velocity |
Parameters | Model characteristics | Kink movement in the poly(A) chain | Kink movement in the poly(T) chain |
Equations of motion | |||
Hamiltonian | |||
Kink-like solution | |||
Total energy | |||
Rest energy | |||
Equations of motion | |||
Kink-like solution | |||
Equation for kink velocity |