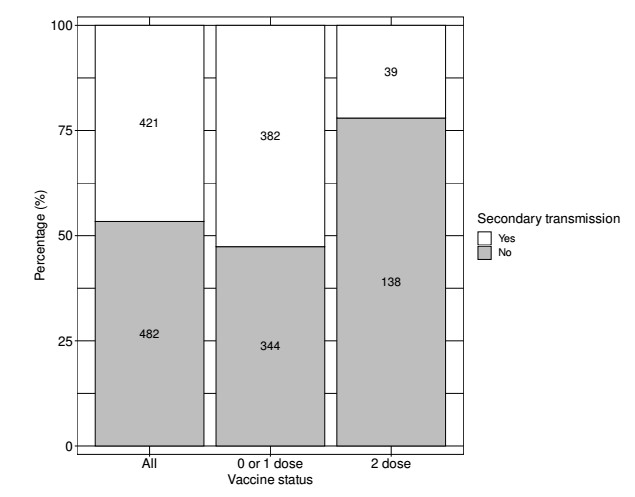
The basic reproduction number, R0, plays a central role in measuring the transmissibility of an infectious disease, and it thus acts as the fundamental index for planning control strategies. In the present study, we apply a branching process model to meticulously observed contact tracing data from Wakayama Prefecture, Japan, obtained in early 2020 and mid-2021. This allows us to efficiently estimate R0 and the dispersion parameter k of the wild-type COVID-19, as well as the relative transmissibility of the Delta variant and relative transmissibility among fully vaccinated individuals, from a very limited data. R0 for the wild type of COVID-19 is estimated to be 3.78 (95% confidence interval [CI]: 3.72–3.83), with k=0.236 (95% CI: 0.233–0.240). For the Delta variant, the relative transmissibility to the wild type is estimated to be 1.42 (95% CI: 0.94–1.90), which gives R0=5.37 (95% CI: 3.55–7.21). Vaccine effectiveness, determined by the reduction in the number of secondary transmissions among fully vaccinated individuals, is estimated to be 91% (95% CI: 85%–97%). The present study highlights that basic reproduction numbers can be accurately estimated from the distribution of minor outbreak data, and these data can provide further insightful epidemiological estimates including the dispersion parameter and vaccine effectiveness regarding the prevention of transmission.
Citation: Minami Ueda, Tetsuro Kobayashi, Hiroshi Nishiura. Basic reproduction number of the COVID-19 Delta variant: Estimation from multiple transmission datasets[J]. Mathematical Biosciences and Engineering, 2022, 19(12): 13137-13151. doi: 10.3934/mbe.2022614
[1] | Fang Wang, Lianying Cao, Xiaoji Song . Mathematical modeling of mutated COVID-19 transmission with quarantine, isolation and vaccination. Mathematical Biosciences and Engineering, 2022, 19(8): 8035-8056. doi: 10.3934/mbe.2022376 |
[2] | Yangyang Yu, Yuan Liu, Shi Zhao, Daihai He . A simple model to estimate the transmissibility of the Beta, Delta, and Omicron variants of SARS-COV-2 in South Africa. Mathematical Biosciences and Engineering, 2022, 19(10): 10361-10373. doi: 10.3934/mbe.2022485 |
[3] | Julijana Gjorgjieva, Kelly Smith, Gerardo Chowell, Fabio Sánchez, Jessica Snyder, Carlos Castillo-Chavez . The Role of Vaccination in the Control of SARS. Mathematical Biosciences and Engineering, 2005, 2(4): 753-769. doi: 10.3934/mbe.2005.2.753 |
[4] | Sarafa A. Iyaniwura, Rabiu Musa, Jude D. Kong . A generalized distributed delay model of COVID-19: An endemic model with immunity waning. Mathematical Biosciences and Engineering, 2023, 20(3): 5379-5412. doi: 10.3934/mbe.2023249 |
[5] | Tahir Khan, Roman Ullah, Gul Zaman, Jehad Alzabut . A mathematical model for the dynamics of SARS-CoV-2 virus using the Caputo-Fabrizio operator. Mathematical Biosciences and Engineering, 2021, 18(5): 6095-6116. doi: 10.3934/mbe.2021305 |
[6] | Kai Wang, Zhenzhen Lu, Xiaomeng Wang, Hui Li, Huling Li, Dandan Lin, Yongli Cai, Xing Feng, Yateng Song, Zhiwei Feng, Weidong Ji, Xiaoyan Wang, Yi Yin, Lei Wang, Zhihang Peng . Current trends and future prediction of novel coronavirus disease (COVID-19) epidemic in China: a dynamical modeling analysis. Mathematical Biosciences and Engineering, 2020, 17(4): 3052-3061. doi: 10.3934/mbe.2020173 |
[7] | Kimihito Ito, Chayada Piantham, Hiroshi Nishiura . Estimating relative generation times and reproduction numbers of Omicron BA.1 and BA.2 with respect to Delta variant in Denmark. Mathematical Biosciences and Engineering, 2022, 19(9): 9005-9017. doi: 10.3934/mbe.2022418 |
[8] | Chentong Li, Jinhu Xu, Jiawei Liu, Yicang Zhou . The within-host viral kinetics of SARS-CoV-2. Mathematical Biosciences and Engineering, 2020, 17(4): 2853-2861. doi: 10.3934/mbe.2020159 |
[9] | Salman Safdar, Calistus N. Ngonghala, Abba B. Gumel . Mathematical assessment of the role of waning and boosting immunity against the BA.1 Omicron variant in the United States. Mathematical Biosciences and Engineering, 2023, 20(1): 179-212. doi: 10.3934/mbe.2023009 |
[10] | Junyuan Yang, Guoqiang Wang, Shuo Zhang . Impact of household quarantine on SARS-Cov-2 infection in mainland China: A mean-field modelling approach. Mathematical Biosciences and Engineering, 2020, 17(5): 4500-4512. doi: 10.3934/mbe.2020248 |
The basic reproduction number, R0, plays a central role in measuring the transmissibility of an infectious disease, and it thus acts as the fundamental index for planning control strategies. In the present study, we apply a branching process model to meticulously observed contact tracing data from Wakayama Prefecture, Japan, obtained in early 2020 and mid-2021. This allows us to efficiently estimate R0 and the dispersion parameter k of the wild-type COVID-19, as well as the relative transmissibility of the Delta variant and relative transmissibility among fully vaccinated individuals, from a very limited data. R0 for the wild type of COVID-19 is estimated to be 3.78 (95% confidence interval [CI]: 3.72–3.83), with k=0.236 (95% CI: 0.233–0.240). For the Delta variant, the relative transmissibility to the wild type is estimated to be 1.42 (95% CI: 0.94–1.90), which gives R0=5.37 (95% CI: 3.55–7.21). Vaccine effectiveness, determined by the reduction in the number of secondary transmissions among fully vaccinated individuals, is estimated to be 91% (95% CI: 85%–97%). The present study highlights that basic reproduction numbers can be accurately estimated from the distribution of minor outbreak data, and these data can provide further insightful epidemiological estimates including the dispersion parameter and vaccine effectiveness regarding the prevention of transmission.
The basic reproduction number, denoted by R0, is an index that represents the expected number of secondary cases from an infected individual during the entire period of infectiousness in a fully susceptible population [1]. This number plays a central role in measuring the transmissibility of infectious diseases and thus acts as the fundamental index for planning control strategies. During the COVID-19 epidemic, a new variant of the severe acute respiratory syndrome coronavirus-2 (SARS-CoV-2), the so-called Delta variant (B.1.617), was detected in Japan in March 2021. Estimating the relative reproduction number of the variant compared with the wild type of the virus then became an urgent task. In the early phase of the Delta variant epidemic, common methodologies to estimate R0, which includes models based on exponential growth rate and generation time distribution, were difficult to implement due to a limited number of observed cases and the progress of variant replacements from Alpha (B.1.1.7) to Delta. Moreover, the spread of the Delta variant occurred in the middle of the very first vaccination campaign, from spring to summer of 2021, making it even more challenging to calculate the variant's basic reproduction number and relative transmissibility. Common methods for estimating R0, as forementioned, rely on major outbreak datasets with an assumption that the population is fully susceptible.
To overcome such problems, the present study focuses on the meticulous observation of secondary transmissions in Wakayama Prefecture of Japan to estimate R0. By applying the branching process model, which has been widely used to model minor outbreaks with non-negligible stochasticity, the study presents a method to efficiently estimate R0 from very limited data on minor outbreaks. The branching process is particularly useful in quantifying the probability of extinction, a property that is supported by quantifying the offspring distribution and final size, i.e., the cumulative incidence of an outbreak. For a realistic representation of the offspring distribution of directly transmitted infectious diseases, the distribution is frequently assumed to follow a negative binomial distribution with two parameters, R0 and the dispersion parameter k [2,3,4,5,6]. The distribution is highly dispersed for k<1, and a very small value of k implies the presence of a super-spreading event [2]. Geometric and Poisson distributions are special cases of the negative binomial distribution when k→∞ and k=1, respectively.
The branching process model has already been employed to model COVID-19, especially in the early stages of the pandemic [6,7,8,9,10]. Published studies clearly indicate the presence of "over-dispersed" secondary transmission, where k is estimated to range from 0.1–0.3 for the wild type of the virus [9,11,12,13]. Preliminary research on the Delta variant has estimated the dispersion parameter to be k=0.23 (95% confidence interval [CI]: 0.18–0.30) [14], which is broadly consistent with that of the wild type, but published evidence remains scarce.
Wakayama Prefecture of Japan is located 50 km south of Osaka, which is the third-largest prefecture in Japan by population. This prefecture continuously experienced epidemic "waves" of COVID-19, just as other prefectures in Japan did; a "wave" is conventionally recognized as a period of the epidemic with upward and downward trends with substantial and sustaining changes [15,16]. The present study focuses on the first and fifth waves caused, respectively, by the wild type and the Delta variant. Each wave is generally recognized as an epidemic around February to May 2020 [17,18] and July to October 2021, respectively. The COVID-19 epidemic was controlled early in Japan, involving 16,741 confirmed cases and 898 deaths until the end of May 2020; the indices are far fewer than in other industrialized countries that experienced population-level epidemics. Because of the low-impact setting, a thorough contact tracing practice, including retrospective (backward) tracing of contacts involving earlier generations, was possible and was continued over time. The practice yielded an empirically observed offspring distribution and the final size of minor outbreaks.
The objective of the present study is to interpret the empirically collected epidemic data in a low-impact setting by jointly estimating (ⅰ) the relative transmissibility of the Delta variant compared with the wild type and (ⅱ) the relative transmissibility among vaccinated individuals compared with unvaccinated individuals. Contrary to common methods for estimating R0 that rely on large datasets from major outbreaks in a fully susceptible population, our study presents a method to efficiently estimate R0 from very limited data on minor outbreaks. This exercise estimates the basic reproduction number of the Delta variant.
We used two datasets: (a) the epidemiological tracing results of secondary transmission during the first wave in 2020, caused by the wild type, and (b) the number of cases that produced at least one secondary transmission during the fifth wave in 2021, caused by the Delta variant. Other waves (the second, third, and fourth waves) were excluded from our scope since the focus of the present study was to estimate the basic reproduction number of the Delta variant, for which evidence is yet limited compared to other variants.
The observations were made in Wakayama Prefecture, which has a population of ∼917,000. Despite the proximity to the metropolis of Osaka, the first wave within the prefecture was contained at a relatively low level, i.e., only 64 cumulative cases and 3 cumulative deaths were observed from the emergence of COVID-19 until June 30, 2020, compared with 1833 cases and 86 deaths in Osaka in the same period. In Wakayama, reverse transcription-polymerase chain reaction (RT-PCR) testing was conducted intensively, and cluster interventions were handled through contact tracing (i.e., containment effort). The vaccination program in Japan started in the early part of 2021, after the end of the third wave in February 2021. Initially, health care workers were prioritized (starting on February 17), and then the elderly population (over 65 years old) began to be vaccinated starting from April 12. At the peak of the fifth wave, caused by the Delta variant in Japan (August 26, 2021), 41.63% of the entire population had received two doses of vaccine [19]. More than 99% of vaccines were mRNA vaccines, either BNT162b2 (Pfizer-BioNTech) or mRNA-1273 (Moderna). Even during the fifth wave, cluster interventions were continued in parallel to vaccination. All cases were confirmed either via RT-PCR testing or antigen testing (including both a qualitative test based on immunochromatogaphy and a quantitative test based on the chemiluminescence enzyme immunoassay (CLEIA) method), and if positive, they were consistently notified to the government health authority (Ministry of Health, Labour and Welfare) in accordance with the Infectious Disease Law.
The first dataset from the first wave covered 25 clusters of COVID-19 infection, and each record included the total number of cases that stemmed from a single primary case. Since each cluster was from a minor outbreak contained at a low level, of which most were reported from households, we could assume that the data was not influenced by public health and social measures (PHSMs) and psychological factors. Minor and major outbreaks are types of epidemics in stochastic SIR models that Whittle characterized in 1955 [20]. Minor outbreaks are generally known to have a shorter duration and substantially fewer cases than major outbreaks [21], and hence we temporarily define the threshold of the final size between the minor and major to be 500 cases for convenience of the discussion. We emphasize that the dataset was from small settings where transmission was contained within each cluster and did not lead to major outbreaks where transmission is prevalent in wide community settings. Considering that the overall infections at the population level also contained at a low level and that the vaccination programs did not exist during the first wave, we can interpret our estimates as the approximation the basic reproduction number, capturing the intrinsic transmission dynamics in a completely susceptible population.
The second dataset from the fifth wave contained the respective numbers of cases that did and did not produce secondary transmission. We assumed that we could ignore the effects of PHSMs since no such kinds of policies were implemented in the region during the period. Furthermore, since the contact tracing practices were continued in the same manner as in the first wave, our estimates can be interpreted as the basic reproduction number for the Delta variant, just as for the wild type. The data were categorized into two groups by vaccination status of the primary case: the "all" group included all cases, regardless of vaccination (i.e., either 0, 1, or 2 doses), whereas the "2 doses" group included cases of individuals who had received two vaccine doses. We obtained values for the "0 or 1 dose" group by subtracting the "2 doses" group from the "all" group. The proportions of those who produced secondary transmissions for each group are shown in Figure 1.
The goal of this study was to estimate R0 and k from limited observations of transmission in the first and fifth waves, where the wild type and Delta variant were respectively dominant. We estimated four parameters, i.e., R0 and k for the wild type, the relative transmissibility of the Delta variant to the wild type (γdelta), and the relative transmissibility among fully vaccinated individuals (post 2 doses of vaccination) compared with unvaccinated individuals (γvac). We used a negative binomial branching process model and estimated the parameters using maximum-likelihood estimation (MLE). The 95% CI for the estimated parameters was obtained from a parametric bootstrapping procedure.
With the assumption of R0>1, because the virus caused a global pandemic, we modelled the final size distribution Z using the Galton–Watson (GW) process that involves a negative binomially distributed offspring distribution. Using the probability generating function (p.g.f.) for Z,
GZ(s)=s(1+R0(1−GZ(s))k)−k | (2.1) |
the probability of the final size being z is expressed as follows:
Pr(Z=z;R0,k)=pz=1z!dzdszGZ(s)|s=0 | (2.2) |
Specifically for z=1 and z≥2, the probabilities of the final size being z are the following [5,22].
{p1=1(1+R0k)kpz=Πz−2j=0(jk+z)z!(kR0+k)kz(R0kR0+k)z−1 | (2.3) |
Because the dataset consists of a series of minor outbreaks, we conditioned the final size distribution on extinction. Thus, under the assumption that the offspring distribution follows a negative binomial distribution with dispersion parameter k, the probability of the final size being z was normalized by the probability of extinction π [5].
Pr(Z=z;minor outbreak)=p′z=1πpz | (2.4) |
π=1(1+R0(1−π)k)k | (2.5) |
The likelihood function for estimating the parameters R0 and k for the wild type, given j complete observations each with a final size of zi, is expressed as follows:
L1(R0,k;zi)=j∏i=1p′z1(1−p′1)=j∏i=1pziπ11−(1π(1+R0k)k)=j∏i=1pzi(1+R0k)kπ(1+R0k)k−1 | (2.6) |
We excluded all observations of z=1, which are sporadic and terminal cases, to adjust for the under-ascertainment of such cases, i.e., compared with the presence of secondary transmission, sporadic primary cases may be far harder to ascertain, especially during the first wave [5,6]. We estimated k indirectly via its reciprocal α=1k, as numerous studies on the estimation of negative binomial parameters have shown that this is better than using k. The sampling distribution for α tends to be more symmetric than that for k, which ensures a faster approach to asymptotic normality [2,3].
Given 14 data points of final size z from the first dataset, from which all observations of z=1 were excluded, we estimated parameters R0 and k. Using the Nelder-Mead method with intial values R0=1.5 and k=0.15, we obtained parameter estimates that maximizes the likelihood function (Eq (2.6)). The optimization was conducted using the R function "optim".
We conducted parametric bootstrapping to obtain the 95% CI of R0 and k. Firstly, we derived the covariance matrix from the Hessian matrix that was returned as part of the optimization result in "optim" function. Using the covariance matrix, we generated 3000 pairs of multivariate normal distributions for R0 and k and calculated the 95% CI with the bootstrap percentile method.
We let the reproduction numbers of the Delta variant in the "0 or 1 dose" group and the "2 doses" group to be:
{R0delta=γdelta⋅R0,R0delta_vac=γvac⋅γdelta⋅R0. | (2.7) |
Here, γdelta expresses the relative transmissibility of the Delta variant compared with that of the wild type, and γvac represents the reduced transmissibility among fully vaccinated individuals compared with unvaccinated individuals. R0 is the basic reproduction number of the wild type. The vaccine effectiveness (VE), in terms of preventing secondary transmission, can be calculated as 100(1−γvac)%. We note that γdelta and γvac are coefficients that represent relative changes in the number of secondary transmissions produced but not the risk of being infected.
Under the assumption that the offspring distribution Y follows a negative binomial distribution, the p.g.f. for having y offspring is as follows.:
GY(s)=(1+R0i(1−s)k)−k | (2.8) |
Using such p.g.f., the probability of having no offspring (y=0) [22] is derived as:
Pr(Y=0)=py=GY(0)=1(1+R0ik)k | (2.9) |
where R0i represents R0delta or R0delta_vac for i=1 and 2, respectively.
The likelihood function for estimating γdelta and γvac is as follows.:
L2(γdelta,γvac;R0,k,ni,mi)=2∏i=1(nimi)pymii(1−pyi)ni−mi+1=2∏i=1(nimi)(1(1+R0ik)k)mi(1−1(1+R0ik)k)ni−mi+1 | (2.10) |
Here, i represents different groups based on vaccine status, where i=1 represents the group with 0 or 1 vaccine doses, and i=2 represents fully vaccinated individuals. ni refers to the total number of people in the category, and mi refers to number of those who had no offspring (y=0). For i=1 in the 0 or 1 dose vaccination group, n1 and m1 had values of 177 and 138, respectively. For i=2 in the fully vaccinated group, n2 and m2 were 726 and 344, respectively.
Given the secondary transmission dataset from the fifth wave and 3000 bootstrap pairs of pre-estimated R0 and k, we estimated parameters γdelta and γvac that maximizes the likelihood function. Using the Nelder-Mead method with intial parameter values γdelta=3 and γvac=0.2, we obtained 3000 pairs of parameter estimates that maximizes the likelihood function. The optimization was conducted using the R function "optim". By the repeating parametric bootstrap for each pairs in the same manner as R0 and k, we obtained 95% CIs for γdelta and γvac.
We assumed that the dispersion parameter k is consistent across SARS-CoV-2. Thus, we conducted a sensitivity analysis of the estimated reproduction number for the Delta variant with different values of the dispersion parameter k. We repeated the estimation for γdelta and γvac using different values of k, ranging between 0.05 and 1, which were minimum and maximum values found either as estimates or the bounds of the CI in prior research [6,7,10,11,12,23,24,25,26,27]. Although there is not yet much evidence for the dispersion parameter of the Delta variant, preliminary research estimates k=0.23 (95% CI: 0.18–0.30) [14], which is within the range of the dispersion parameter for the wild type.
Reproduction numbers of the Delta variant in populations with different vaccination statues were calculated by substituting estimated γdelta and γvac into Eq (2.7). 95% CIs for reproduction numbers were also calculated with the bootstrap percentile method.
All of the analysis in the present study was conducted using R, version 3.6.3 [28].
The present study examined publicly available data that do not contain any personally identifiable information. As such, ethical approval was not required for the present study.
For the wild type of COVID-19, R0 was estimated to be 3.78 (95% CI: 3.72–3.83). This value is higher than suggested by previous research, with meta-analysis and a systematic review concluding that R0 lies in the range from 2–3 [29,30]. The value of k for the wild type was estimated to be 0.236 (95% CI: 0.233–0.240), which is broadly consistent with prior findings [6,8,9,10,12,13,26]. The final size distribution given by the estimated R0 and k is shown in Figure 2, which compares observed data (line) and the fitted model (bars). While the model captures the overall trend of frequency, the frequencies for z=2 and z=3 are underestimated. The fit may be biased by a cluster observed at z=15.
Using the estimated parameters of the wild type, we then estimated the relative transmissibility of the Delta variant and the relative transmissibility among fully vaccinated individuals. The relative transmissibility of the Delta variant to the wild type, γdelta, was estimated to be 1.42 (95% CI: 0.94–1.90). The relative effect on transmissibility from two-dose vaccination, γvac, was estimated to be 0.09 (95% CI: 0.03–0.14). This indicates that VE for preventing secondary transmission among a fully vaccinated population, compared with unvaccinated individuals, was 91% (95% CI: 85%–97%).
By substituting the estimated parameters into Eq (2.7), the basic reproduction number of the Delta variant, R0delta, was calculated as 5.37 (95% CI: 3.55–7.21). This value is consistent with prior research findings, which indicate a mean value of 5.08 [31]. The reproduction number of the Delta variant among fully vaccinated individuals, R0delta_vac, was calculated to be 0.44 (95% CI: 0.20–0.69). This result highlights that the reproduction number in the fully vaccinated population was well below 1.
The offspring distribution of the Delta variant was calculated using the mean of the estimated reproduction number for both the 0 or 1 dose population and the 2 doses population (Figure 3). The dispersion parameter k was assumed to be identical to the estimated value for the wild type (k=0.236). The left panel shows the result for the population with vaccination status of either 0 or 1 dose, while the right panel shows the result for 2 doses. The dotted horizontal line identifies the observed frequency of those who did not cause a secondary infection (y=0). For the 2 doses population, the estimated frequency from the model matches that of the observed data.
The results of sensitivity analysis with various values of the dispersion parameter k are shown in Figure 4. Dotted vertical lines indicate the value of the dispersion parameter (k=0.23) estimated from the data for the wild type. At k=0.05, the Delta variant is estimated to have an R0 value of 2878.75 (95% CI 1248.96–4458.64), while at k=1.0, R0 is 1.11 (95% CI 0.95–1.28) (Panel C). As shown in Panel B, the relative transmissibility among fully vaccinated individuals is reduced at smaller k values, meaning that there is a greater reduction in the number of secondary transmissions. The reproduction number in fully vaccinated individuals was estimated to be less than 1 for all k values greater than 0.15.
Using meticulously observed contact tracing data from Wakayama prefecture in early 2020 and mid-2021, we estimated the basic reproduction number R0 and the dispersion parameter k of the wild-type COVID-19, as well as the relative transmissibility of the Delta variant and relative transmissibility among fully vaccinated individuals. For the wild type, R0 was estimated to be 3.78, and k was estimated to be 0.24. For the Delta variant, we estimated that R0 is 5.37, which is in line with preliminary findings reporting a mean of 5.08 [31]. Our estimates also indicated that the number of secondary transmissions was reduced by 91% among fully vaccinated individuals. To the best of our knowledge, no previous research has quantified the R0 value of the COVID-19 Delta variant from minor outbreak data while accounting for the relative transmissibility among fully vaccinated individuals.
The present study highlights that the basic reproduction numbers of variants can efficiently be estimated by applying the branching process model to the distribution of minor outbreak data. We emphasize that our method provides "relative transmissibility" of emerging variants on the basic reproduction number, not the effective reproduction number. This provides the basic reproduction numbers of new variants that work as fundamental indices for controlling pandemics (i.e., in planning strategies of vaccination programs). Our methods can be applied if epidemiological surveys are being conducted at a constant level, and the relative susceptibility within the population remains at a comparable level. Moreover, we emphasize that the data from minor outbreaks, which are a type of epidemic that has a small number of cases, can provide further insightful epidemiological estimates, including the dispersion parameter and VE in terms of preventing transmission. While the estimated R0 of the wild type was higher than the commonly agreed value, it was within the upper range of published CIs [29,30]. Furthermore, we have proved that VE in terms of preventing secondary transmission can also be estimated from limited observed data. Finally, the 95% CI for the estimated R0 of the Delta variant overlaps with values reported in existing studies [31,32].
The strength of the present study lies in the successful estimation of the basic reproduction number R0 of the Delta variant from minimal observed data of minor outbreaks. Understanding R0 is essential in planning effective strategies to contain the spread of the virus, particularly when it is known to be growing with the emergence of new variants. Furthermore, estimations regarding the Delta variant were made possible by assuming that the dispersion parameter is equivalent to that of the wild type, which we estimated from another dataset collected in the same geographic region. We could have separately estimated the dispersion parameter if either the final size or offspring distribution had also been observed for the Delta variant.
Our results imply that contact tracing is a critical factor that can enhance future data collection during the pandemic. Our findings prove that contact tracing data contribute to estimations of transmissibility and VE. To further understand the nature of the virus and its mechanisms of transmission, surveillance should be customized to include information as follows: vaccination status, number of secondary transmissions (not only whether they did or did not cause secondary transmission), detailed demographic and epidemiological attributes (i.e., sex and age group for each reported case). This information allows a more explicit and detailed analysis of the transmission dynamics specific to particular population groups and settings.
We must address some limitations of our study. First, the data on secondary transmission were identified through epidemiological investigation, which relied on the local capacity of contact tracing and patient cooperation in the tracing practice. The tracing method means that there is always the possibility of recall bias, which may lead to the presence of unascertained cases. However, because backward tracing was combined with forward tracing in Japan, it is likely that most secondary transmission, including asymptomatic cases, was captured. More active testing strategies, including daily PCR testing, may capture more comprehensive transmission dynamics. Second, the sample size of the final size distribution was limited to only 25 clusters. While it is still very rare for data to be observed, the estimates for R0 and k could have been more consistent with existing research if more reports were available. A serological survey could have been conducted so that the actual final size could be determined without the possibility of any biases. Third, the fifth wave dataset lacked a detailed offspring distribution, but it instead included the respective frequencies of those who did or did not cause secondary transmissions. If the full distribution of secondary transmission per person was observed (e.g., y=1,2,3,…), R0 and k for the Delta variant could have been separately estimated with greater precision. While modeling from a limited dataset is still possible under certain assumptions, a detailed offspring distribution is essential in understanding the full nature of the transmission dynamics of infectious diseases. Finally, the waning of vaccine-induced immunity was ignored. Because the fifth wave occurred amid the vaccination program, we believe it was not essential to meticulously measure the time since vaccination at an individual level.
In conclusion, the present study has demonstrated a method to estimate the basic reproduction number of a new variant, denoted by the relative transmissibility from an existing type of virus, through the application of branching process models on very limited data. We emphasize that our method does not rely on exponential growth rate and generation time distribution models that require major outbreak datasets from fully susceptible populations. Using the final size and offspring distribution of minor outbreaks aggregated based on intensive contact tracing, we have estimated that the Delta variant is 1.42 times as transmissive as the wild type of COVID-19, which results in R0 of 5.37. The VE in the reduction in the number of secondary transmissions was estimated to be 91% among fully vaccinated individuals compared with unvaccinated individuals.
The study has also presented the importance of collecting pieces of information on minor outbreaks, where transmissions are contained in small settings without spreading to a wide range of communities in the population. We emphasize that offspring and final size distributions accumulated based on intensive contact tracing practices or serological surveys can significantly contribute to determining the fundamental indices for understanding and controlling outbreaks.
H. N. received funding from Health and Labour Sciences Research Grants (20CA2024, 20HA2007, 21HB1002 and 21HA2016), the Japan Agency for Medical Research and Development (JP20fk0108140, JP20fk0108535, and JP21fk0108612), the Japan Society for the Promotion of Science (JSPS) KAKENHI (21H03198), the Environment Research and Technology Development Fund (JPMEERF20S11804) of the Environmental Restoration and Conservation Agency of Japan, and the Japan Science and Technology Agency SICORP program (JPMJSC20U3 and JPMJSC2105). We thank local governments, public health centers, and institutes for surveillance, laboratory testing, epidemiological investigations, and data collection. We thank Stuart Jenkinson, PhD, from Edanz (https://jp.edanz.com/ac) for editing a draft of this manuscript.
The authors declare there is no conflict of interest.
[1] |
O. Diekmann, J. A. P. Heesterbeek, J. A. J. Metz, On the definition and the computation of the basic reproduction ratio R0 in models for infectious diseases in heterogeneous populations, J. Math. Biol., 28 (1990), 365–382. https://doi.org/10.1007/BF00178324 doi: 10.1007/BF00178324
![]() |
[2] |
J. O. Lloyd-Smith, S. J. Schreiber, P. E. Kopp, W. M. Getz, Superspreading and the effect of individual variation on disease emergence, Nature, 438 (2005), 355–359. https://doi.org/10.1038/nature04153 doi: 10.1038/nature04153
![]() |
[3] |
J. O. Lloyd-Smith, Maximum likelihood estimation of the negative binomial dispersion parameter for highly overdispersed data, with applications to infectious diseases, PLOS ONE, 2 (2007), e180. https://doi.org/10.1371/journal.pone.0000180 doi: 10.1371/journal.pone.0000180
![]() |
[4] |
S. Blumberg, J. O. Lloyd-Smith, Inference of R0 and transmission heterogeneity from the size distribution of stuttering chains, PLoS Comput. Biol., 9 (2013), e1002993. https://doi.org/10.1371/journal.pcbi.1002993 doi: 10.1371/journal.pcbi.1002993
![]() |
[5] |
H. Nishiura, P. Yan, C. K. Sleeman, C. J. Mode, Estimating the transmission potential of supercritical processes based on the final size distribution of minor outbreaks, J. Theor. Biol., 294 (2012), 48–55. https://doi.org/10.1016/j.jtbi.2011.10.039 doi: 10.1016/j.jtbi.2011.10.039
![]() |
[6] |
S. Zhao, M. Shen, S. S. Musa, Z. Guo, J. Ran, Z. Peng, et al., Inferencing superspreading potential using zero-truncated negative binomial model: Exemplification with COVID-19, BMC Med. Res. Methodol, 21 (2021), 30. https://doi.org/10.1186/s12874-021-01225-w doi: 10.1186/s12874-021-01225-w
![]() |
[7] |
D. C. Adam, P. Wu, J. Y. Wong, E. H. Y. Lau, T. K. Tsang, S. Cauchemez, et al., Clustering and superspreading potential of SARS-CoV-2 infections in Hong Kong, Nat. Med., 26 (2020), 1714–1719. https://doi.org/10.1038/s41591-020-1092-0 doi: 10.1038/s41591-020-1092-0
![]() |
[8] |
A. Endo, Centre for the Mathematical Modelling of Infectious Diseases COVID-19 Working Group, S. Abbott, A. J. Kucharski, S. Funk, Estimating the overdispersion in COVID-19 transmission using outbreak sizes outside China, Wellcome Open Res., 5 (2020), 67. https://doi.org/10.12688/wellcomeopenres.15842.3 doi: 10.12688/wellcomeopenres.15842.3
![]() |
[9] |
H. Lee, C. Han, J. Jung, S. Lee, Analysis of superspreading potential from transmission clusters of COVID-19 in South Korea, Int. J. Environ. Res. Public Health, 18 (2021), 12893. https://doi.org/10.3390/ijerph182412893 doi: 10.3390/ijerph182412893
![]() |
[10] |
Y. Zhang, Y. Li, L. Wang, M. Li, X. Zhou, Evaluating transmission heterogeneity and super-spreading event of COVID-19 in a metropolis of China, Int. J. Environ. Res. Public Health, 17 (2020), 3705. https://doi.org/10.3390/ijerph17103705 doi: 10.3390/ijerph17103705
![]() |
[11] |
A. Tariq, Y. Lee, K. Roosa, S. Blumberg, P. Yan, S. Ma, et al., Real-time monitoring the transmission potential of COVID-19 in Singapore, March 2020, BMC Medicine, 18 (2020), 166. https://doi.org/10.1186/s12916-020-01615-9 doi: 10.1186/s12916-020-01615-9
![]() |
[12] |
L. Wang, X. Didelot, J. Yang, G. Wong, Y. Shi, W. Liu, et al., Inference of person-to-person transmission of COVID-19 reveals hidden super-spreading events during the early outbreak phase, Nat. Commun., 11 (2020), 5006. https://doi.org/10.1038/s41467-020-18836-4 doi: 10.1038/s41467-020-18836-4
![]() |
[13] |
K. Nakajo, H. Nishiura, Transmissibility of asymptomatic COVID-19: Data from Japanese clusters, Int. J. Infect. Dis., 105 (2021), 236–238. https://doi.org/10.1016/j.ijid.2021.02.065 doi: 10.1016/j.ijid.2021.02.065
![]() |
[14] |
H. Hwang, J. S. Lim, S. A. Song, C. Achangwa, W. Sim, G. Kim, et al., Transmission dynamics of the Delta variant of SARS-CoV-2 infections in South Korea, J. Infect. Dis., 225 (2022), 793–799. https://doi.org/10.1093/infdis/jiab586 doi: 10.1093/infdis/jiab586
![]() |
[15] |
J. Middleton, H. Lopes, K. Michelson, J. Reid, Planning for a second wave pandemic of COVID-19 and planning for winter: A statement from the Association of Schools of Public Health in the European Region, Int. J. Public Health, 65 (2020), 1525–1527. https://doi.org/10.1007/s00038-020-01455-7 doi: 10.1007/s00038-020-01455-7
![]() |
[16] |
S. X. Zhang, F. Arroyo Marioli, R. Gao, S. Wang, A second wave? What do people mean by COVID waves? – a working definition of epidemic waves, RMHP, 14 (2021), 3775–3782. https://doi.org/10.2147/RMHP.S326051 doi: 10.2147/RMHP.S326051
![]() |
[17] |
R. Kinoshita, S. M. Jung, T. Kobayashi, A. R. Akhmetzhanov, H. Nishiura, Epidemiology of coronavirus disease 2019 (COVID-19) in Japan during the first and second waves, Math. Biosci. Eng., 19 (2022), 6088–6101. https://doi.org/10.3934/mbe.2022284 doi: 10.3934/mbe.2022284
![]() |
[18] |
T. Kuniya, Evaluation of the effect of the state of emergency for the first wave of COVID-19 in Japan, Infect. Dis. Model., 5 (2020), 580–587. https://doi.org/10.1016/j.idm.2020.08.004 doi: 10.1016/j.idm.2020.08.004
![]() |
[19] | Digital Agency, Japan, [COVID-19 vaccination status], 2022. Avaliable from: https://info.vrs.digital.go.jp/dashboard/ |
[20] |
P. Whittle, The outcome of a stochastic epidemic —a note on Bailey's paper, Biometrika, 42 (1955), 116–122. https://doi.org/10.1093/biomet/42.1-2.116 doi: 10.1093/biomet/42.1-2.116
![]() |
[21] |
W. Tritch, L. J. Allen, Duration of a minor epidemic, Infect. Dis. Model., 3 (2018), 60–73. https://doi.org/10.1016/j.idm.2018.03.002 doi: 10.1016/j.idm.2018.03.002
![]() |
[22] | P. Yan, G. Chowell, Quantitative Methods for Investigating Infectious Disease Outbreaks, Springer, 2019. |
[23] |
K. Sneppen, B. F. Nielsen, R. J. Taylor, L. Simonsen, Overdispersion in COVID-19 increases the effectiveness of limiting nonrepetitive contacts for transmission control, Proc. Natl. Acad. Sci., 118 (2021), e2016623118. https://doi.org/10.1073/pnas.2016623118 doi: 10.1073/pnas.2016623118
![]() |
[24] |
Q. Bi, Y. Wu, S. Mei, C. Ye, X. Zou, Z. Zhang, et al., Epidemiology and transmission of COVID-19 in 391 cases and 1286 of their close contacts in Shenzhen, China: A retrospective cohort study, Lancet Infect. Dis., 20 (2020), 911–919. https://doi.org/10.1016/S1473-3099(20)30287-5 doi: 10.1016/S1473-3099(20)30287-5
![]() |
[25] |
R. Laxminarayan, B. Wahl, S. R. Dudala, K. Gopal, C. Mohan B, S. Neelima, et al., Epidemiology and transmission dynamics of COVID-19 in two Indian states, Science, 370 (2020), 691–697. https://doi.org/10.1126/science.abd7672 doi: 10.1126/science.abd7672
![]() |
[26] |
K. Sun, W. Wang, L. Gao, Y. Wang, K. Luo, L. Ren, et al., Transmission heterogeneities, kinetics, and controllability of SARS-CoV-2, Science, 371 (2021), eabe2424. https://doi.org/10.1126/science.abe2424 doi: 10.1126/science.abe2424
![]() |
[27] |
J. Riou, C. L. Althaus, Pattern of early human-to-human transmission of Wuhan 2019 novel coronavirus (2019-nCoV), December 2019 to January 2020, Eurosurveillance, 25 (2020), 2000058, https://doi.org/10.2807/1560-7917.ES.2020.25.4.2000058 doi: 10.2807/1560-7917.ES.2020.25.4.2000058
![]() |
[28] | R Core Team, R: A Language and Environment for Statistical Computing, R Foundation for Statistical Computing, Vienna, Austria, 2020. Available from: https://www.R-project.org/. |
[29] |
M. A. Billah, M. M. Miah, M. N. Khan, Reproductive number of coronavirus: A systematic review and meta-analysis based on global level evidence, PLOS ONE, 15 (2020), e0242128. https://doi.org/10.1371/journal.pone.0242128 doi: 10.1371/journal.pone.0242128
![]() |
[30] |
Y. Liu, A. A. Gayle, A. Wilder-Smith and J. Rocklöv, The reproductive number of COVID-19 is higher compared to SARS coronavirus, Journal of Travel Medicine, 27 (2020), taaa021. https://doi.org/10.1093/jtm/taaa021. doi: 10.1093/jtm/taaa021
![]() |
[31] |
Y. Liu, J. Rocklöv, The reproductive number of the Delta variant of SARS-CoV-2 is far higher compared to the ancestral SARS-CoV-2 virus, J. Travel Med., 28 (2021), taab124. https://doi.org/10.1093/jtm/taab124 doi: 10.1093/jtm/taab124
![]() |
[32] |
M. Zhang, J. Xiao, A. Deng, Y. Zhang, Y. Zhuang, T. Hu, et al., Transmission dynamics of an outbreak of the COVID-19 Delta variant B.1.617.2 — Guangdong Province, China, May–June 2021, CCDCW, 3 (2021), 584–586. https://doi.org/10.46234/ccdcw2021.148 doi: 10.46234/ccdcw2021.148
![]() |
1. | Olusegun Michael Otunuga, Alexandra Yu, Vaccine breakthrough and rebound infections modeling: Analysis for the United States and the ten U.S. HHS regions, 2023, 8, 24680427, 717, 10.1016/j.idm.2023.05.010 | |
2. | Tobhin Kim, Hyojung Lee, Sungchan Kim, Changhoon Kim, Hyunjin Son, Sunmi Lee, Improved time-varying reproduction numbers using the generation interval for COVID-19, 2023, 11, 2296-2565, 10.3389/fpubh.2023.1185854 | |
3. | Andreas Baumann, Søren Wichmann, Lexical innovations are rarely passed on during one’s lifetime: Epidemiological perspectives on estimating the basic reproductive ratio of words, 2024, 19, 1932-6203, e0312336, 10.1371/journal.pone.0312336 | |
4. | Xuan Li, Ling Yin, Kang Liu, Kemin Zhu, Yunduan Cui, Deep-reinforcement-learning-based optimization for intra-urban epidemic control considering spatiotemporal orderliness, 2024, 1365-8816, 1, 10.1080/13658816.2024.2431904 | |
5. | Huaiyu Teng, Toshikazu Kuniya, The Optimal Vaccination Strategy to Control COVID‐19, 2025, 0170-4214, 10.1002/mma.10908 |