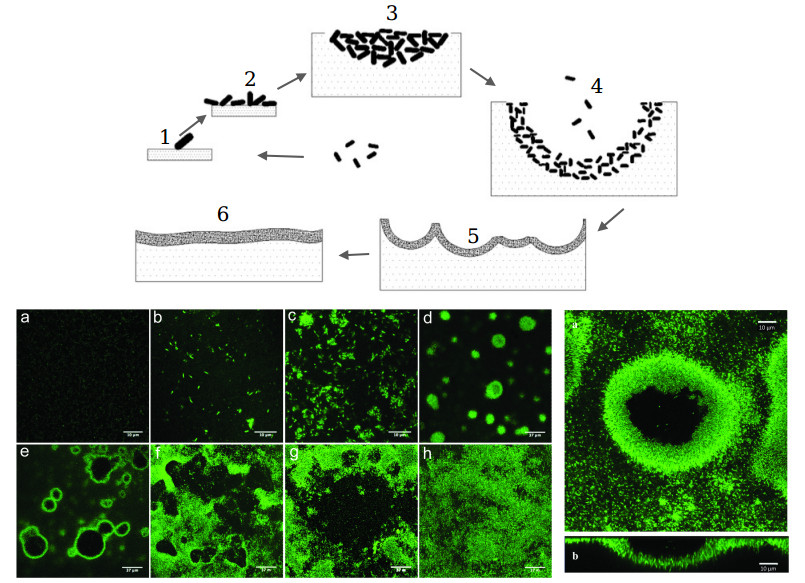
Missing values in the k-NN algorithm are a significant research concern, especially in low-grade tumours and CSF fluid, which are commonly identified in MRI scans. Missing values are usually ignored, but when data is mined, they can lead to bias and errors. In addition, the data is not missing at random. This study improves image accuracy, boosts the efficiency of missing k-NN hybrid values, and develops a research technique for detecting CSF fluid deposits in brain areas separated from non-tumor areas. We also offer a new method for detecting low-grade tumours or cerebrospinal fluid (CSF) formation in its early stages. In this study, we combine the hybrid K-Nearest Neighbor algorithm with the Discrete Fourier transform (DFT), as well as Time-Lagged analysis of four-dimensional (4D) MRI images. These dependencies exist in both space and time, but present techniques do not account for both sequential linkages and numerous types of missingness. To address this, we propose the DFLk-NN imputation method, which combines two imputation approaches based on a hybrid k-NN extension and the DFT to capture time-lag correlations both within and across variables. There are several types of missingness are enables the imputation of missing values across the variable even when all the data for a given time point is missing. The proposed method gives high accuracies of MRI datasets and retrieves the missing data in the images.
Citation: Soobia Saeed, Habibollah Haron, NZ Jhanjhi, Mehmood Naqvi, Hesham A. Alhumyani, Mehedi Masud. Improve correlation matrix of Discrete Fourier Transformation technique for finding the missing values of MRI images[J]. Mathematical Biosciences and Engineering, 2022, 19(9): 9039-9059. doi: 10.3934/mbe.2022420
[1] | Yousef Rohanizadegan, Stefanie Sonner, Hermann J. Eberl . Discrete attachment to a cellulolytic biofilm modeled by an Itô stochastic differential equation. Mathematical Biosciences and Engineering, 2020, 17(3): 2236-2271. doi: 10.3934/mbe.2020119 |
[2] | Blessing O. Emerenini, Stefanie Sonner, Hermann J. Eberl . Mathematical analysis of a quorum sensing induced biofilm dispersal model and numerical simulation of hollowing effects. Mathematical Biosciences and Engineering, 2017, 14(3): 625-653. doi: 10.3934/mbe.2017036 |
[3] | Fadoua El Moustaid, Amina Eladdadi, Lafras Uys . Modeling bacterial attachment to surfaces as an early stage of biofilm development. Mathematical Biosciences and Engineering, 2013, 10(3): 821-842. doi: 10.3934/mbe.2013.10.821 |
[4] | Fabiana Russo, Alberto Tenore, Maria Rosaria Mattei, Luigi Frunzo . Multiscale modelling of the start-up process of anammox-based granular reactors. Mathematical Biosciences and Engineering, 2022, 19(10): 10374-10406. doi: 10.3934/mbe.2022486 |
[5] | Nikodem J. Poplawski, Abbas Shirinifard, Maciej Swat, James A. Glazier . Simulation of single-species bacterial-biofilm growth using the Glazier-Graner-Hogeweg model and the CompuCell3D modeling environment. Mathematical Biosciences and Engineering, 2008, 5(2): 355-388. doi: 10.3934/mbe.2008.5.355 |
[6] | Mudassar Imran, Hal L. Smith . A model of optimal dosing of antibiotic treatment in biofilm. Mathematical Biosciences and Engineering, 2014, 11(3): 547-571. doi: 10.3934/mbe.2014.11.547 |
[7] | Vincenzo Luongo, Maria Rosaria Mattei, Luigi Frunzo, Berardino D'Acunto, Kunal Gupta, Shankararaman Chellam, Nick Cogan . A transient biological fouling model for constant flux microfiltration. Mathematical Biosciences and Engineering, 2023, 20(1): 1274-1296. doi: 10.3934/mbe.2023058 |
[8] | Donna J. Cedio-Fengya, John G. Stevens . Mathematical modeling of biowall reactors for in-situ groundwater treatment. Mathematical Biosciences and Engineering, 2006, 3(4): 615-634. doi: 10.3934/mbe.2006.3.615 |
[9] | Fazal Abbas, Rangarajan Sudarsan, Hermann J. Eberl . Longtime behavior of one-dimensional biofilm models with shear dependent detachment rates. Mathematical Biosciences and Engineering, 2012, 9(2): 215-239. doi: 10.3934/mbe.2012.9.215 |
[10] | Choah Shin, Azhar Alhammali, Lisa Bigler, Naren Vohra, Malgorzata Peszynska . Coupled flow and biomass-nutrient growth at pore-scale with permeable biofilm, adaptive singularity and multiple species. Mathematical Biosciences and Engineering, 2021, 18(3): 2097-2149. doi: 10.3934/mbe.2021108 |
Missing values in the k-NN algorithm are a significant research concern, especially in low-grade tumours and CSF fluid, which are commonly identified in MRI scans. Missing values are usually ignored, but when data is mined, they can lead to bias and errors. In addition, the data is not missing at random. This study improves image accuracy, boosts the efficiency of missing k-NN hybrid values, and develops a research technique for detecting CSF fluid deposits in brain areas separated from non-tumor areas. We also offer a new method for detecting low-grade tumours or cerebrospinal fluid (CSF) formation in its early stages. In this study, we combine the hybrid K-Nearest Neighbor algorithm with the Discrete Fourier transform (DFT), as well as Time-Lagged analysis of four-dimensional (4D) MRI images. These dependencies exist in both space and time, but present techniques do not account for both sequential linkages and numerous types of missingness. To address this, we propose the DFLk-NN imputation method, which combines two imputation approaches based on a hybrid k-NN extension and the DFT to capture time-lag correlations both within and across variables. There are several types of missingness are enables the imputation of missing values across the variable even when all the data for a given time point is missing. The proposed method gives high accuracies of MRI datasets and retrieves the missing data in the images.
Bacterial biofilms are microbial depositions on immersed solid abiotic or biotic surfaces. Once attached, they form a self-secreted matrix of extracellular polymeric substance (EPS) that provides structural stability and an adhesion mechanism to the surface. The EPS is assumed to be mostly composed of polysaccharides, but it may contain nucleic acids and proteins. The bacterial cells become sessile and are protected from anti-microbials and mechanical stress due to the EPS [1,2,3].
The surface to which the biofilm attaches is called the substratum. Some substrata are impenetrable and inert such as rocks or plastic. However, we will be considering substrata that are reactive and can be degraded. These degradable substrata can play a vital role in the biofilm development cycle as they can provide the nutrients needed for biofilm growth and proliferation, which is the main focus of the cellulolytic biofilms we will focus on.
Biofilms require nutrients to grow, which we call substrates. Substrates are either supplied by the substratum (this is the case for cellulolytic biofilms) or by the surrounding environment through diffusion or advection. Depending on the availability, rate of delivery, and local concentrations of substrates, biomass growth may be slow and limited in specific locations, while in other locations, the biomass may be fast-growing and abundant. If we assume the availability of substrates is not a growth-limiting factor, then the metabolic growth rate of the biofilm will determine the generation of biological by-products, and the uptake of substrates [4].
A biofilm community typically consists of many separated sub-colonies, which may merge and form into larger colonies. Macroscopically, biofilm communities are homogeneous, meaning they look like a thin film covering the substratum. However, structural differences become apparent at the mesoscopic level (10μm−1mm in this case). At these scales, the heterogeneity of a biofilm community manifests itself. Factors such as specific growth rate, maximum cell density, and substrate concentration determine the level of heterogeneity of the biofilm community.
The formation of biofilms onto the substratum typically occurs via the physical attachment of free-floating bacteria in the aqueous phase surrounding the substratum by forming a firm physiochemical bond. One possible source of free-floating bacteria is from biomass detachment as a result of shear forces exerted on the biofilm from a fluid [5]. The possibility of adhesion of bacterial cells to a certain position on the substratum can be influenced by many factors, including [6]:
● Bacterial characteristics: surface charge, appendages, hydrophobicity, etc.,
● Environment: temperature, bacterial concentration, chemical treatment, etc.,
● Substratum properties: chemical composition, roughness, physical configuration, etc.,
● Hydrodynamics in the aqueous phase and possibly fluid-structure interaction if the substratum is not rigid.
Hence, the attachment and adhesion of biomass to the substratum depends on numerous parameters. When modelling attachment, this implies that many model parameters are required to capture this phenomenon. Including all of these different parameters in a model will likely make the model too complex. However, one possible mathematical simplification of this process is to consider the attachment phenomenon as a random event at each location of the substratum, where all the underlying mechanisms are lumped together into one driving stochastic process per location on the substratum. In other words, we view the attachment phenomenon as a random behaviour of the cells in the aqueous phase for which the underlying mechanisms of attachment are not considered. This notion is supported by experimental observations in [7,8]. Thus, whatever processes may affect attachment at a given time and with a given set of system parameters are driven by a stochastic process at each inoculation point of the substratum.
The addition of biomass attachment has not readily been used in the modelling literature despite the importance of the process on biofilm growth and formation. Even when attachment is included in models, the approach is typically the addition of a simple deterministic function based on no experimental data, which we discuss at the end of this section. The primary focus of this work is the mathematical modelling of discrete stochastic cell attachment and how this attachment may affect the spatial and temporal evolution of biofilms. The overall goal is to adapt the mathematical framework presented in [9]. The model presented in [9] has two major shortcomings, difficulty in model analysis and slow simulation times. The difficulty in model analysis comes from the Itô stochastic differential equations (SDEs) formalism [10], while the slow simulation times come from small step-sizes caused by the explicit method used. Therefore, we use random ordinary differential equations (RODEs), which allows us to use ODE theory and numerics path-wise. Moreover, RODEs allow to use different stochastic processes and these stochastic processes can appear in nonlinear terms (see Section 3.1).
Barring computational costs, we want our modelling framework to apply to biofilms of different types and morphologies and in any number of spatial dimensions. However, for analysis and simulation purposes, we apply our modelling framework to the case of 2D cellulolytic biofilms. Cellulolytic biofilms mainly arise in the biofuel production industry, and they have specific characteristics that we will exploit. The attachment phenomenon is assumed to be a discrete process and is characterized in terms of the adhesion factors discussed above; however, the attachment phenomenon is not very well-understood.
Biofuels are an alternative fuel source in the transportation sector in place of fossil fuels and can be implemented in the existing infrastructure. A significant benefit of biofuels is that it is an abundant renewable resource and has a net-zero carbon dioxide release into the atmosphere. However, the production of biofuels using food products, such as sugars, starch, fats, and oils, is not sustainable due to high and increasing demand for food [11]. Therefore, it is better to use inedible resources, such as lignocellulose*, to produce biofuels. The annual production of cellulosic ethanol in the US from lignocellulosic resources was estimated at 53 billion gallons in 2016 [12].
*Lignocellulose is composed of carbohydrate polymers (cellulose, hemicellulose), and an aromatic polymer (lignin).
The production of cellulosic ethanol is considered achievable for lignocellulosic biomass. However, a few difficulties arise, including biomass recalcitrance, which is associated with the resistance of cell walls to enzymatic deconstruction, and the heterogeneity of the substrate caused by the complex structure of lignocellulose [13]. Therefore, the lignocellulose biomass needs to be treated and prepared before it can be extracted. In consolidated bioprocessing (CBP), cellulolytic bacteria perform fermentation and hydrolysis of the sugars in solid lignocellulosic substances [13,14]. CBP requires micro-organisms that are highly efficient at fermenting the resultant sugars and producing cellulolytic enzymes. The micro-organisms should also produce little by-product and be able to live in environments with high levels of ethanol.
Common bacteria used in CBP include Clostridium thermocellum and Caldicellulosiruptor obsidiansis, which consume cellulosic substrate and form cellulolytic biofilms [7,15]. These cellulolytic biofilm colonies are distributed spatially and evolve temporally [8]. Cellulolytic biofilms form very little EPS, attach directly to the substrate, and are typically monolayers [7,8]. These cellulolytic biofilms differ from the traditional biofilms, which grow into the aqueous phase that contains the substrates. These cellulolytic biofilms carve out crater-like structures in the substratum, which are referred to as inverse colonies [8]. Cellulolytic biofilms form and grow through several stages (see Figure 1):
1) Initial cell attachment to the substratum,
2) Cell growth and division,
3) Inverted colony formation,
4) Emergence of crater-like structures due to substrate consumption and substratum degradation,
5) Radial growth of the crater-like depressions and the eventual coalescence of the crater-like structures,
6) Formation of a uniform-thickness biofilm.
The different stages of the spatiotemporal evolution of biofilms formed by C. obsidiansis on cellulose chads are captured by a confocal laser scanning microscope and are visualized in Figure 1 [8]. The crater-like structures that form are provided in Figure 1 [8].
The mathematical modelling of stochastic attachment of cells to cellulolytic biofilms has been addressed in two different works. First, in an ad hoc manner by modifying a deterministic model of cellulolytic biofilms [16]. Secondly, [9] developed a model by first discretizing the deterministic model from [16] and then adding an additional stochastic term to the biomass equations using an attachment factor, which converted the model to an SDE system. Therefore, both models have the same deterministic part that deals with the formation and temporal evolution of cellulolytic biofilms and is described by a highly nonlinear degenerate coupled system of a partial differential equation (PDE) and an ODE for the biomass density and substrate concentration respectively. However, the SDE model in [9] is constructed via a spatial discretization of the PDE-ODE coupled system.
The model in [16] has an ad hoc inclusion of stochastic cell attachment via the addition of an impulse function to the biomass equation. The modelling mechanism behind the impulse function works as follows. If a certain quantity (Q) is greater than a uniform random number over [0,1], then the impulse function becomes non-zero, and a fixed amount of biomass is added to the PDE; otherwise, the value of the impulse function is zero, and hence no biomass is added. The quantity Q is defined based on a few assumptions on the likelihood of an attachment event occurring. An attachment event is less likely to occur if the location has a large quantity of biomass, M, already, or if the substrate concentration, C, is too low, i.e., Q∝(1−M)×C, where we assume that M is bounded by 1. By formulating the attachment phenomenon in this way, paper [16] was able to reproduce the qualitative behaviour of cellulolytic biofilms with cell attachment. This can be seen from the graphical visualization taken from [16] (see Figure 2) and the comparison with the experimental observations given in Figure 1.
Although the approach taken in [16] reproduces the expected qualitative behaviour, there is no mathematical framework and it is not based on a stochastic process. In particular, the model cannot be written as a stochastic or random (partial) differential equation.
The model in [9] seeks to utilize the insight gained from [16] but using a rigorous mathematical framework. In order to capture cellular attachment a similar impulse term is mollified and added to the biomass equation and an attachment factor is introduced for each grid cell of the domain. The attachment factor is a stochastic process based on the construction of Q given above, without drift. The attachment factor was simulated alongside the biomass and substrate equations. The model consists of an Itô SDE with the necessary regularity properties to apply SDE theory. The model in [9] reproduced the qualitative behaviour observed in experiments (see Figure 2). However, there are still significant downsides to this approach. The first being the similar ad hoc approach with an attachment factor, and the second being the numerical implementation. The numerical method for solving this model was the simplest available. Namely, [9] used the Euler-Maruyama method with a time-stepping approach constructed to ensure stability of the system. The downside of this method stems from the fact that discretized diffusion-reaction equations are typically stiff [17], especially with the nonlinear diffusion coefficient in this model. Therefore, the time-step required to maintain stability is quite small.
Other attachment modelling strategies are used in the literature for different types of biofilms. The approaches taken in other models are deterministic. For example, in [18], attachment was added to a 3D biofilm model via a pre-determined function of time. In other models, the attachment of biomass is prescribed as a function of the biomass concentration in the aqueous phase [19,20,21]. Typically the function αu, where α is the attachment rate and u is the concentration of biomass in the aqueous phase, is used. The attachment terms in both cases are not related to any underlying mechanism. This is similar to the approaches for cellulolytic biofilms [16] and to the approach we will present. However, we take into account the stochastic nature of attachment, which might be a more realistic approach.
The model in [16] adapts the model for traditional biofilms proposed in [22] to the case of cellulolytic biofilms. The aqueous phase does not explicitly appear in the model formulation and the nutrients are contained in the substratum. The model assumptions are given below.
1) The local capacity of the substratum for accommodating biomass is limited.
2) The only growth-limiting substrate is carbon. The carbon is only present inside the substratum and is assumed to be immobile and not replenished after consumption.
3) The spatial distribution, production, and movement of biomass only depends on the local availability of space and nutrients in the substratum. The biofilm does not exhibit notable expansion if there is available space.
4) Carbon is consumed by the bacteria and converted into new cells. We assume that biomass growth and carbon degradation are governed by standard saturation kinetics.
5) Cells are lost from the biofilm either by detachment or cell lysis. Both processes are combined into one process and are proportional to the local biomass density. Once carbon becomes depleted, biofilm growth ceases, and the loss of cells becomes the dominant process.
6) No cellular attachment occurs from the aqueous phase.
We will describe the two-dimensional variation of the model presented in [16]. More specifically, we will be considering the limiting case of cellulosic biofilms that spread across a very thin cellulosic substratum, where the depth of the substratum is negligible compared to the length scales of the colonies. This is one of the two cases described in [16].
The model is a coupled system of a PDE describing the spatiotemporal evolution of the biomass density M=M(t,x) and an ODE describing the evolution of the carbon concentration C=C(t,x). We formulate the model in a rectangular spatial domain Ω:=[0,1]×[0,W]⊂R2, with W≤1. All variables and parameters are dimensionless (see [9] for more details). The model is given by
∂tM=∇⋅(D(M)∇M)+F(C)M,(t,x)∈(0,∞)×Ω, | (2.1) |
∂tC=−G(C)M,(t,x)∈(0,∞)×Ω. | (2.2) |
Here D(M) is the biomass diffusion coefficient, F(C) is the net biomass growth rate, and G(C) is the uptake rate of the carbon substrate. The substrate uptake rate is the only driving factor in (2.2) since there is no transport of carbon by model assumption 2.1. This is in contrast to the modelling framework presented in [22]. The actual biofilm consists of the sub-region of the domain Ω defined by
ΩM(t):={x∈Ω∣M(t,x)>0}. |
Following [9,16,22], we define the biomass density dependent diffusion coefficient by
D(M):=δMα(1−M)β,α,β≥1, | (2.3) |
where δ>0 is the motility coefficient. By model assumption 2.1 and the non-dimensionalization given in [9], we assume that the maximum attainable biomass is 1.
The diffusion coefficient exhibits two distinct cases of behaviour: (ⅰ) if the local biomass density is low, then little diffusion will occur, meaning the substratum can accommodate additional biomass (in accordance with model assumption 2.1); (ⅱ) if the local biomass density is close to its maximum value, then the equation for biomass will exhibit super diffusion behaviour and the biomass will move quickly to neighbouring locations (in accordance with model assumption 2.1). For 0≤M≪1, the diffusion coefficient can be approximated by a degenerate power law, which is known from the porous medium equation, i.e., D(M)≈Mα, which approaches 0 as M→0. From simulations in [23], we know that if the initial data has compact support, then so does the solution. Therefore, the degenerate power-law creates an expanding biofilm colony with a sharp front. The exponent α in the diffusion coefficient controls how much biomass can be accommodated locally before a noticeable expansion into neighbouring regions occurs. When 0≪M<1, the biomass density approaches the singularity in the diffusion coefficient and super diffusion dominates, i.e., D(M)≈(1−M)−β. The exponent β determines the severity of the super diffusion. Both diffusion modes are required to ensure expansion with finite speed (due to the degenerate power-law) and to have the local volume filling effect (due to the super diffusion) [16].
The net biomass growth rate is composed of the biomass growth rate and the cell loss rate. By model assumption 2.1, the cell loss rate includes both cell lysis and detachment of viable bacteria into the aqueous phase. By model assumption 2.1, carbon is the only growth-limiting substrate in the system, and by model assumption 2.1 the biomass growth rate is described by Monod kinetics [24]. Therefore, the net growth rate is given by
F(C)=Cκ+C−λ, | (2.4) |
where κ>0 is the half-saturation constant and λ>0 is the cell loss rate. The maximum growth rate is equal to 1 by our non-dimensionalization.
The uptake rate of the carbon substrate is modelled using the standard Monod saturation kinetics [24] by model assumption 2.1 and is given by
G(C)=ΥCκ+C, | (2.5) |
where Υ>0 is the maximum specific consumption rate.
Biomass is restricted to the domain Ω and it is thus natural to impose homogeneous Neumann conditions on (2.1), i.e.,
∂nM(t,x)=0,(t,x)∈[0,∞)×∂Ω, | (2.6) |
where ∂n denotes the outward normal derivative and ∂Ω the boundary of Ω. We also impose the initial conditions
M(0,x)=M0(x),C(0,x)=C0(x),x∈Ω. | (2.7) |
We assume that M0(x)>0 in small subsets of Ω and M0(x)=0 everywhere else and we assume C0(x)>0 in Ω. In general, C0(x) can depend on the spatial position x; however, in most of our simulations we restrict ourselves to the case where C0(x)=C∞=const as in the experiments conducted in [7,8,25], which used homogeneous paper chads.
For simulation purposes and to introduce our attachment term, we spatially discretize the PDE-ODE coupled system. We place an N×K uniform grid N onto the rectangular domain Ω:=[0,1]×[0,W] with W≤1. We use the finite volume scheme as introduced in [26] and described in detail in [9]. This converts our PDE-ODE coupled system into 2NK ODEs for each grid cell (i,j) as
dMi,jdt=1Δx∑σ∈Ni,jJσ+(Ci,jκ+Ci,j−λ)Mi,j, | (2.8) |
dCi,jdt=−ΥCi,jκ+Ci,jMi,j. | (2.9) |
where Ni,j:={(i+1,j),(i−1,j),(i,j+1),(i,j−1)} is the set of neighbouring grid cells and
Jσ={12Δx(D(Mσ)+D(Mi,j))(Mσ−Mi,j)forσ∈N0forσ∉N, | (2.10) |
is the flux out of the grid cell (i,j) into grid cell σ, where Δx=1/N=W/K is the side length of the grid cells. We write the 2NK ODEs in matrix-vector notation as
dMdt=D(M)M+F(C)M,dCdt=−G(C)M, | (2.11) |
after ordering the grid cells by π(i,j)=(i−1)K+j. The matrices D(M), F(C) and G(C) are NK×NK and represent the diffusion, net biomass growth, and carbon uptake, respectively.
Remark 2.1. The matrix D(M) is symmetric and weakly diagonally dominant with non-negative off-diagonal entries and non-positive diagonal entries [9]. Therefore, D(M) is at least negative semi-definite. The matrices F(C) and G(C) are diagonal matrices with diagonal entries fpp=f(Cp) and gpp=G(Cp). If Cp=0, then fpp=−λ, the natural cell loss rate, and gpp=0.
In the next section, we introduce our new approach for modelling cellular attachment via RODEs.
Here we introduce random ordinary differential equations (RODEs), which we will use to model random attachment. We follow the description in [27].
Let (S,F,P) be a probability space, where S is the set of outcomes, F is a σ-algebra on S, and P is a probability measure, and let η:[0,T]×S→Rm be an Rm-valued stochastic process with continuous sample paths. In addition, let g:Rd×Rm→Rd. A RODE in Rd is an equation of the form
dxdt=g(x,ηt(s)),x∈Rd, | (3.1) |
that can be viewed as a non-autonomous ordinary differential equation
dxdt=Gs(t,x):=g(x,ηt(s)) | (3.2) |
for almost every realization s∈S [27]. Since RODEs are just non-autonomous ODEs, we can apply results from ODE theory path-wise (for a given realization s∈S) to gain insight into the dynamics of an RODE, as long as our Gs still satisfies the regularity requirements. The results in our case only require continuity in t, which our stochastic processes satisfy.
In fact, we will use the Wiener process Wt. The Wiener process is a Gaussian process with the following properties [28]:
1) W0=0 almost surely.
2) Wt has independent increments, namely Wt1−Wτ1 and Wt2−Wτ2 are independent random variables for all 0≤τ1<t1≤τ2<t2.
3) The increments Wt−Wτ are normally distributed with E(Wt−Wτ)=0 and Var(Wt−Wτ)=t−τ for all 0≤τ<t.
4) The sample paths are continuous almost surely.
Similarly to [9], we add discrete random attachment events. Attachment is the process by which cells in the aqueous phase attach to either the substratum or the pre-existing biomass. In many controlled experiments, no bacteria are present at the inflow, and thus, bacteria are only present in the aqueous phase if they have been previously detached from the biofilm. This is an example of the experiments done in [7,29], on which the models presented in [9,16] are based. Similar to [9], we make the simplifying assumption that attachment and detachment can be modelled independently. This is supported by [7] as they found that the availability of cells in the aqueous phase was not a limiting factor for attachment. Therefore, we assume that there is an unlimited supply of available cells for attachment. We also assume that cells generally do not attach to areas that have low carbon concentration or areas with high biomass density. This is consistent with the assumptions in [9,16].
We add a mollified impulse function to each biomass Eq (2.8) with a mutually independent Wiener process Wt as argument for each grid cell (i,j). As in [9], we use the Fermi-Dirac distribution. It allows us to easily and independently control the location of the impulse, the height of the impulse, and how well the mollification approximates a true discontinuous impulse function. The Fermi-Dirac distribution with parameters a,b,σ is given by
f(ϕ)=1eϕ−bσ+1−1eϕ−aσ+1. | (3.3) |
The parameters a<b determines the location of the impulse, while the parameter σ controls how close the approximation is to the true impulse function. In particular, as σ→0 the Fermi-Dirac distribution converges to an impulse function supported on [a,b] turning on at ϕ=a and off at ϕ=b.
We now add to each biomass equation the term
γg(Mi,j)h(Ci,j)f(W(i,j)t), | (3.4) |
where 0<γ≪1 controls the intensity of bacterial attachment per unit time and W(i,j)t is an independent standard Wiener process for each grid cell (i,j). Here we see that the stochastic processes appear in the nonlinear function f. The functions g and h satisfy the attachment assumptions depending on M and C, i.e., g(1)=0=h(0), both g(0),h(1)≤1, g is decreasing and h is increasing, and these functions are sufficiently smooth. As in [9], we take functions of the form
g(M)={(1−M)ν1,M<1,0,M≥1,,h(C)=Cν2. | (3.5) |
The exponents ν1,ν2>0 are included for generality. Hence, from (2.8) we obtain
dMi,jdt=1Δx∑σ∈Ni,jJσ+(Ci,jκ+Ci,j−λ)Mi,j+γg(Mi,j)h(Ci,j)f(W(i,j)t). | (3.6) |
Using the same ordering as before, our model becomes a system of 2NK RODEs given by
dMdt=D(M)M+F(C)M+T(M,C)F(Wt),dCdt=−G(C)M, | (3.7) |
where D(M), F(C), and G(C) are the same as in (2.11). Moreover, T(M,C) is an NK×NK matrix with entries calculated based on (3.4) and F(Wt) is an NK vector with components equal to (3.3).
Remark 3.1. The matrix T(M,C) is a diagonal matrix with diagonal entries ipp=γg(Mp)h(Cp). These entries vanish if either Mp=1 or Cp=0 and attain their maximum value if both Mp=0 and Cp=1. Therefore, 0≤ipp≪1. The pth entry of F is given by Fp(Wt)=f(Wpt)=(eWpt−bσ+1)−1−(eWpt−aσ+1)−1. Finally, by construction, 0≤Fp≤1.
As in previous works [9,26,30], we regularize the diffusion coefficient and consider
Dϵ(M)={0,M<0,δMα(1−M)β,0≤M≤1−ϵ,δϵ−β(1−ϵ)α,M>1−ϵ, | (3.8) |
for ϵ>0 sufficiently small. This leads to the regularized, approximate system
dMϵdt=Dϵ(Mϵ)Mϵ+F(Cϵ)Mϵ+T(Mϵ,Cϵ)F(Wt),dCϵdt=−G(Cϵ)Mϵ. | (3.9) |
We perform analysis pathwise, i.e., for a given realization of the Wiener processes. We will show solutions are non-negative and bounded using comparison theorems and the tangent criterion from [31]. We will also study the long-term behaviour of our system. We first state a lemma that follows from standard Calculus results.
Lemma 3.2. Consider the scalar ODE
dudt=−au+ρ(t) |
with a>0, and ρ(t)≥0 and continuous for all t≥0 with ρ(t)→ρ∞ as t→∞. Then,
lim |
Proposition 3.3. Let \boldsymbol{{M}}^\epsilon and \boldsymbol{{C}}^\epsilon be path-wise solutions to (3.9) and suppose that the initial data satisfy {\bf{0}}\leq \boldsymbol{{M}}^\epsilon(0)\leq\zeta{\bf{1}} with \zeta\in(0, 1) and {\bf{0}}\leq\boldsymbol{{C}}^\epsilon(0)\leq{\bf{1}} . Then for almost every realization of the Wiener processes there exists a unique solution and the corresponding solution satisfies
\begin{align*} &{\bf{0}}\leq\boldsymbol{{M}}^\epsilon\leq \mu{{\bf{1}}}\quad {{for\; all}}\;t\geq0, \\ &{\bf{0}}\leq \boldsymbol{{C}}^\epsilon\leq{\bf{1}}\quad {{for \;all}}\;t\geq0, \end{align*} |
where the constant \mu > 0 depends on the initial value and model parameters.
Proof. To prove the non-negativity, we invoke the tangent criterion [31]. First we consider the substrate equation. From Remark 2.1, the matrix \mathscr{G}(\boldsymbol{C}^\epsilon) is diagonal and thus the pth component of the substrate equation is
\begin{align*} -(\mathscr{G}(\boldsymbol{C}^\epsilon)\boldsymbol{M}^\epsilon)_p = -\Upsilon\frac{C_p^\epsilon M_p^\epsilon}{\kappa+C_p^\epsilon}. \end{align*} |
For C_p^\epsilon = 0 , the above vanishes and since -\mathscr{G}(\boldsymbol{C}^\epsilon)\boldsymbol{M}^\epsilon satisfies a Lipschitz condition, it follows from the tangent criterion that \boldsymbol{C}^\epsilon\geq0 [31].
The equation for the biomass consists of three components. From Remarks 2.1 and 3.1 we have
● pth component of \mathscr{T}(\boldsymbol{M}^\epsilon, \boldsymbol{C}^\epsilon)\boldsymbol{F}\left(\boldsymbol{W_t}\right)
\begin{align*} \mathfrak{if}_p = \gamma g(M_p^\epsilon)h(C_p^\epsilon)f(W_t^p), \end{align*} |
● pth component of \mathscr{F}(\boldsymbol{{C^\epsilon}})\boldsymbol{{M^\epsilon}}
\begin{align*} \mathfrak{fm}_p = \left(\frac{C_p^\epsilon}{\kappa+C_p^\epsilon}-\lambda\right)M_p^\epsilon, \end{align*} |
● pth component of \mathscr{D^\epsilon}(\boldsymbol{{M^\epsilon}})\boldsymbol{M}^\epsilon
\begin{align*} \mathfrak{dm}_p = &\frac{1}{2\Delta x^2}[(D^\epsilon(M^\epsilon_{p-K})+D^\epsilon(M^\epsilon_p))M^\epsilon_{p-K} + (D^\epsilon(M^\epsilon_{p-1})+D^\epsilon(M^\epsilon_p))M^\epsilon_{p-1}\\ &-(D^\epsilon(M^\epsilon_{p-K})+D^\epsilon(M^\epsilon_{p-1})+4D^\epsilon(M^\epsilon_p)+D^\epsilon(M^\epsilon_{p+1})+D^\epsilon(M^\epsilon_{p+K}))M^\epsilon_p\\ &+(D^\epsilon(M^\epsilon_{p+1})+D^\epsilon(M^\epsilon_p))M^\epsilon_{p+1} + (D^\epsilon(M^\epsilon_{p+K})+D^\epsilon(M^\epsilon_p))M^\epsilon_{p+K}], \end{align*} |
where M^\epsilon_i = 0 , whenever the grid cell does not exist.
If M^\epsilon_p = 0 , then \mathfrak{if}_p\geq0 , \mathfrak{fm}_p = 0 , and
\begin{align*} \mathfrak{dm}_p = {\frac{1}{2\Delta x^2}}[D^\epsilon(M_{p-M}^\epsilon)M_{p-M}^\epsilon+D^\epsilon(M_{p-1}^\epsilon)M_{p-1}^\epsilon+D^\epsilon(M_{p+1}^\epsilon)M_{p+1}^\epsilon+D^\epsilon(M_{p+M}^\epsilon)M_{p+M}^\epsilon]\geq0 \end{align*} |
if M_{p-M}^\epsilon, \ M_{p-1}^\epsilon, \ M_{p+1}^\epsilon, \ M_{p+M}^\epsilon are all non-negative. Therefore, \boldsymbol{{M}}^\epsilon is non-negative.
The non-negativity of \boldsymbol{M}^\epsilon and \boldsymbol{C}^\epsilon now implies that \boldsymbol{C}^\epsilon is bounded by 1, as C^\epsilon is non-increasing. We finish by proving the upper bound for \boldsymbol{{M}}^\epsilon . Since d\boldsymbol{C}^\epsilon / dt\leq0 , {\bf{0}}\leq\boldsymbol{C}^\epsilon\leq{{\bf{1}}} , and -\mathscr{G}(\boldsymbol{C}^\epsilon)\boldsymbol{M}^\epsilon satisfies a Lipschitz condition, we can conclude that
\begin{align} \lim\limits_{t\to\infty}\frac{d\boldsymbol{C}^\epsilon}{dt} = 0. \end{align} | (3.10) |
Therefore, each component of d\boldsymbol{C}^\epsilon / dt\leq0 must converge to 0. Let
\begin{align} \boldsymbol{\eta}(t): = \mathscr{G}(\boldsymbol{{C^\epsilon}})\boldsymbol{{M^\epsilon}} = -\frac{d\boldsymbol{C}^\epsilon}{dt}, \end{align} | (3.11) |
and define \eta_1(t): = {{\bf{1}}}^T\boldsymbol{\eta}(t) . By (3.10), we know \eta_1(t)\to0 as t\to\infty . Now we can consider the ODE system
\begin{align} \begin{aligned} \frac{d\boldsymbol{{\hat M^\epsilon}}}{dt}& = \mathscr{D^\epsilon}(\boldsymbol{{\hat M^\epsilon}})\boldsymbol{{\hat M^\epsilon}}+\mathscr{F}(\boldsymbol{{\hat C^\epsilon}})\boldsymbol{{\hat M^\epsilon}}+\gamma{\bf{1}}, \\ \frac{d\boldsymbol{{\hat C^\epsilon}}}{dt}& = -\mathscr{G}(\boldsymbol{{\hat C^\epsilon}})\boldsymbol{{\hat M^\epsilon}}. \end{aligned} \end{align} | (3.12) |
Let \boldsymbol{{M}}_{tot} = \sum_{p = 1}^{NK} \hat M^\epsilon_p . Then, we can construct a differential inequality for \boldsymbol{M}_{tot} by summing up all components of the \boldsymbol{{\hat M^\epsilon}} DE in (3.12). This gives
\begin{align} \frac{d\boldsymbol{{M}}_{tot}}{dt}& = \sum\limits_{p = 1}^{NK}F(\hat C^\epsilon_p)\hat M^\epsilon_p + NK\gamma = \sum\limits_{p = 1}^{NK}\left[\frac{\hat C^\epsilon_p}{\kappa+\hat C^\epsilon_p}-\lambda\right]\hat M^\epsilon_p + NK\gamma\leq-\lambda\boldsymbol{M}_{tot}+\frac{\eta_1(t)}{\Upsilon}+NK\gamma. \end{align} | (3.13) |
From this we define the barrier ODE
\begin{align} \frac{d\boldsymbol{{\overline M}}_{tot}}{dt}+\lambda\boldsymbol{{\overline M_{tot}}} = \frac{\eta_1(t)}{\Upsilon}+NK\gamma, \end{align} | (3.14) |
which we know is an upper bound on \boldsymbol{M}_{tot} by comparison theorems [31]. By Lemma 3.2, we know
\begin{align} \lim\limits_{t\to\infty}\boldsymbol{{\overline M_{tot}}}(t) = \frac{NK\gamma}{\lambda}. \end{align} | (3.15) |
The ODE (3.14), is a standard linear ODE, and thus the solution does not tend to infinity in finite time and since \boldsymbol{M}_{tot}\geq0 , we have
\begin{align} \boldsymbol{{\overline M_{tot}}} \leq \mu \end{align} | (3.16) |
for some \mu\geq 0 that depends on model parameters and initial data. Since
\begin{align} \mathfrak{if}_p = \gamma g(M^\epsilon_p)h(C^\epsilon_p)f(W_t^p)\leq\gamma, \end{align} | (3.17) |
we observe that the solutions to (3.12) are upper bounds for the solutions to our RODE model (3.9), which implies the biomass density is bounded.
Since the Wiener process is pathwise continuous and the right-hand side of (3.9) satisfies a local Lipschitz condition, we have a unique local solution, and by the boundedness condition, we have a unique global solution [31].
We now move on to showing the long-term behaviour of (3.9).
Proposition 3.4. All solutions to (3.7), with non-negative initial data, satisfy
\begin{align} \lim\limits_{t\to\infty} \boldsymbol{M}^\epsilon(t) = {\bf{0}} \quad {{and}} \quad \lim\limits_{t\to\infty} \boldsymbol{C}^\epsilon(t) = \boldsymbol{{\tilde C}}, \end{align} | (3.18) |
where {\bf{0}}\leq \boldsymbol{{\tilde C}}\leq {{\bf{1}}} .
Proof. For notational simplicity, we denote by \boldsymbol{M} = \boldsymbol{M}(t) and \boldsymbol{C} = \boldsymbol{C}(t) the solutions to (3.9). By (3.10) in the previous proof
\begin{align} \lim\limits_{t\to\infty}-{\mathscr{G}}(\boldsymbol{C})\boldsymbol{M} = {\bf{0}}. \end{align} | (3.19) |
We also know that
\begin{align} (\mathscr{G}(\boldsymbol{C})\boldsymbol{M})_p = \Upsilon\frac{C_p}{\kappa+C_p}M_p, \end{align} | (3.20) |
and hence, \mathscr G(\boldsymbol{C})\boldsymbol{M} = {\bf{0}} if and only if C_pM_p = 0 for all p = 1, ..., NK . Therefore, since we know \boldsymbol{M} is bounded by a constant, we have
\begin{align} (\boldsymbol{M}^T\mathscr{T}(\boldsymbol{M}, \boldsymbol{C})\boldsymbol{F}(\boldsymbol{W_t}))_p = \gamma g(M_p)M_p C_p^{\nu_2}f(W_t^p)\to0 \end{align} | (3.21) |
as t\to\infty for all p . Therefore, \boldsymbol{M}^T\mathscr{G}(\boldsymbol{{C}})\boldsymbol{{M}}/\Upsilon+\boldsymbol{M}^T\mathscr{T}(\boldsymbol{M}, \boldsymbol{C})\boldsymbol{F}(\boldsymbol{W_t})\to0 as t\to\infty . Since we have the existence of a unique solution to (3.9) by Proposition 3.3, we know \boldsymbol{M}^T\mathscr{G}(\boldsymbol{{C}})\boldsymbol{{M}}/\Upsilon+\boldsymbol{M}^T\mathscr{T}(\boldsymbol{M}, \boldsymbol{C})\boldsymbol{F}(\boldsymbol{W_t}) is continuous. To this end we define
\begin{align} \eta(t): = \frac{1}{\Upsilon}\boldsymbol{M}^T\mathscr{G}(\boldsymbol{{C}})\boldsymbol{{M}}+\boldsymbol{M}^T\mathscr{T}(\boldsymbol{M}, \boldsymbol{C})\boldsymbol{F}(\boldsymbol{W_t}), \end{align} | (3.22) |
where we know \eta(t) is continuous for all t > 0 and \eta(t) converges to \eta_\infty: = 0 as t\to\infty . We will now use a discrete energy estimate type argument to show die-out of biomass. Let
\begin{align} E(t):& = \left\lVert{\boldsymbol{M}(t)}\right\rVert_2^2. \end{align} | (3.23) |
Then if we multiply the biomass equation in (3.9) by 2\boldsymbol{M}^T on the left we get
\begin{align} \frac{dE}{dt}& = 2\boldsymbol{M}^T\frac{d\boldsymbol{M}}{dt} = 2\boldsymbol{M}^T\mathscr D_\epsilon(\boldsymbol{M})\boldsymbol{M}+2\boldsymbol{M}^T{\mathscr G}(\boldsymbol{C})\boldsymbol{M}+2\boldsymbol{M}^T \mathscr{T}(\boldsymbol{M}, \boldsymbol{C})\boldsymbol{F}(\boldsymbol{W_t}). \end{align} | (3.24) |
By Remark 2.1, we know \mathscr{D}(\boldsymbol{M}) is negative semi-definite. The same reasoning applies to the regularized diffusion matrix and so \mathscr{D}_\epsilon(\boldsymbol{M}) is also negative semi-definite. Therefore, \boldsymbol{M}^T\mathscr{D}_\epsilon(\boldsymbol{M})\boldsymbol{M}\leq0 . So,
\begin{align} \begin{aligned} \frac{dE}{dt}&\leq-2\lambda E+2\eta(t). \end{aligned} \end{align} | (3.25) |
since \mathscr G(C) = \Upsilon(\mathscr F(C)+\lambda I) , where I is the identity matrix. From this we define the barrier ODE
\begin{align} \frac{d\overline{E}}{dt}+2\lambda\overline{E} = 2\eta(t), \end{align} | (3.26) |
which we know is an upper bound on (3.24) by comparison theorems [31]. By Lemma 3.2, we know that
\begin{align} \lim\limits_{t\to\infty}\overline{E}(t) = \frac{\eta_\infty}{\lambda} = 0. \end{align} | (3.27) |
Since, by definition, E is non-negative, we know that E\to0 as t\to\infty . Therefore, \boldsymbol{M}(t)\to 0 as t\to\infty since E(t) = \left\lVert{\boldsymbol{M}(t)}\right\rVert_2^2 = 0 if and only if \boldsymbol{M}(t) = 0 .
Remark 3.5. We cannot prove that the biomass is strictly bounded by 1, which is an underlying assumption of the model. For generic parameters and initial data, it can occur that M\geq1 in finite time. This issue is due to the homogeneous Neumann boundary conditions, which imply that no biomass can leave the domain and biomass decays if and only if C is sufficiently small. We can determine the critical substrate concentration for a given grid cell, such that biomass loss will start dominating locally. This occurs when C < \lambda\kappa/(1-\lambda) = :C_{crit} . We always assume \lambda < 1 ; otherwise, cell loss would always dominate growth. Strictly speaking, our model is only valid as long as M < 1 . However, during all simulations that we will present, the biomass concentration never reached 1, i.e. C dropped below C_{crit} before M could reach unity. Therefore, the restriction of our model to the case where M < 1 has no practical effect.
For numerical integration of the RODE system (3.7) we use the ODE trapezoid method with constant time-stepping. We use ODE numerics as the traditional SDE and RODE numerics [32,33] led to instability, negative values or excessive simulation runtime, and were not remedied by the techniques found in [34]. To solve the non-linear system of equations, we use a fixed-point iteration scheme to convert the non-linear system into a sequence of linear systems. Namely, let U be either state vairable, then U^k denotes the solution at the current time step and U^{k+1} the solution at the next time step. We denote by U^{(p)} and U^{(p+1)} the current and next iterate in our fixed point iteration in the current timestep. To simplify notation we omit here the time step counter k . Then, in each time step we iterate
\begin{align} \begin{split} \left[I-\frac{1}{2}\left(\Delta t\mathscr{D}(\boldsymbol{M}^{(p)})+\Delta t\mathscr{F}(\boldsymbol{C}^{(p)})\right)\right]\boldsymbol{{M}}^{(p+1)} = &\boldsymbol{M}^k+\frac{\Delta t}{2}\left(\mathscr{T}(\boldsymbol{M}^{(p)}, \boldsymbol{C}^{(p)})\boldsymbol{F}\left(\boldsymbol{W}_{t_{k+1}}\right)\right.\\ &+\mathscr{D}(\boldsymbol{M}^{k})\boldsymbol{M}^{k} +\mathscr{F}(\boldsymbol{C}^{k})\boldsymbol{M}^{k}\\ &\left. +\mathscr{T}(\boldsymbol{M}^{k}, \boldsymbol{C}^{k})\boldsymbol{F}\left(\boldsymbol{W}_{t_{k}}\right)\right), \end{split} \end{align} | (4.1) |
\begin{align} \boldsymbol{C}^{(p+1)} = &\boldsymbol{C}^k-\frac{\Delta t}{2}\left(\mathscr{G}(\boldsymbol{C}^{(p+1)})\boldsymbol{M}^{(p+1)}+\mathscr{G}(\boldsymbol{C}^{k})\boldsymbol{M}^{k}\right), \end{align} | (4.2) |
over p until the 1- norm of successive iterations is less than some pre-specified tolerance. For our simulations we use a tolerance of 10^{-6} . Once convergence is reached we set \boldsymbol{M}^{k+1} = \boldsymbol{M}^{(p+1)} and \boldsymbol{C}^{k+1} = C^{(p+1)} . Each linear system for \boldsymbol{M}^{(p+1)} is solved using the Conjugate Gradient method [35], since the resulting system is symmetric positive definite [36]. See [36] for more details.
Simulations were conducted using Julia 1.5.3 [37]. To simulate the independent Wiener processes, we use randn , which is a Guassian random number generator built into Julia. Namely, given the previous value of the Wiener process W_{t_k} at time t_k , we calculate the next value W_{t_{k+1}} at time t_{k+1} , via W{t_{k+1}} = W_{t_k}+\Delta W_{t_k} , where \Delta W_{t_k} is a Guassian random number with mean 0 and variance \Delta t = t_{k+1}-t_k . We control the seeding of the random number generation by the Random.seed! function.
We parallelize the construction of the linear systems, the pseudo-random number generation (PRNG), the array copying, and the convergence calculations. We used two different parallelization techniques in Julia. The first is the macro @threads , which is placed in front of for loops, while the second is the vmapntt function, which provides vectorized parallel execution of a scalar function. For both methods, the '{\tt -t } n ' runtime flag was used to control the number of threads available, where n is the number of threads. For more details see [36].
We ran all of our simulations on two separate computers. The first is a custom-built workstation with an AMD Ryzen Threadripper 3955WX (3.9 GHz, 16 cores, 32 threads) and 64 GB RAM running Ubuntu 20.10 (Groovy Gorilla). The second workstation is a custom-built Intel Xeon E5-1660 v4 (3.2 GHz, 6 cores, 12 threads) with 32 GB RAM running Ubuntu 16.04.7 LTS (Xenial Xerus).
We begin by showcasing several simulations to illustrate the efficacy of our model and numerical method. The first set is with an initially clean domain, i.e., the initial biomass density is zero everywhere. The second set investigates the model with an initially colonized domain, which starts with a small circular inoculation of biomass to see how the random attachment events interact with pre-existing biomass.
Here we simulate (3.7) with the initial conditions M_0\equiv0 and C_0\equiv1 everywhere in \Omega . Between the two simulations, we controlled the emerging characteristics of the biomass by the impulse interval [a, b] . Both simulations use the parameter values given in Table 1 with Simulation 1 having an attachment interval [a, b] = [0.99999, 1.0] (see Figure 3) while Simulation 2 has an attachment interval [a, b] = [0.9999, 1.0] (see Figure 4). As the simulations progress temporally, we see small colonies form across the domain; in both simulations, this occurs at around t = 20 . These colonies grow rapidly, and once they reach saturation, they expand over to neighbouring colonies. The simulations manifest the crater-like structures (see Figure 1) resembling the colonies formed in cellulolytic biofilms (see Figure 1). As the colonies continue to spread, some colonies coalesce and form communities. These communities continue to coalesce and form until the substratum is fully degraded. Once the substrate in the substratum becomes limited, the biomass begins to decay until no substrate is present and, in turn, no biomass.
Parameter | Symbol | Value | Reference |
Motility Coefficient | \tilde\delta | 10^{-6} | [16] |
Diffusion Coefficient Exponent 1 | \alpha | 4.0 | [16] |
Diffusion Coefficient Exponent 2 | \beta | 4.0 | [16] |
Maximum Consumption Rate | \tilde\Upsilon | 0.4 | [16] |
Half Saturation Concentration | \tilde\kappa | 0.01 | [16] |
Cell Loss Rate | \tilde\lambda | 0.42 | [16] |
Control Parameter of f | \sigma | 0.000001 | assumed |
Shift Parameters of f | a & b | Varied | assumed |
Density of an Attached Cell per Time Step | \gamma | 0.01 | assumed |
Biomass Attachment Exponents | \nu_{1/2} | 2.0 | assumed |
Domain | \Omega | [0, 1]\times[0, 1] | assumed |
Grid Size | N\times K | 2^{9}\times 2^{9} | assumed |
Step Size | \Delta t | 10^{-2} | assumed |
Seed | - | 1 | assumed |
Figures 3 and 4 show subtle differences in the colony formation. In Figure 3, we have fewer attachment events taking place, which is expected due to the smaller impulse interval [a, b] . At time t = 20 we have slightly more established attachment events in Figure 4 compared to Figure 3. If an attachment event occurs in the simulation depicted in Figure 3, then it must occur in the simulation depicted in Figure 4 since [0.99999, 1]\subset[0.9999, 1] and Wiener processes have continuous sample paths. The simulation ends earlier for the larger impulse interval. Meaning the substrate and biomass reaching zero (or close to 0) everywhere. On Figure 3 we see that the simulation finishes shortly after t = 50 , while in Figure 4 it finishes at t = 45 . This is caused by the slightly sooner attachment events and the larger total biomass resulting from more attachment events. More biomass in the system, especially spread out across more grid cells, means quicker substrate degradation. More substrate degradation means faster biomass death and detachment, as discussed in Remark 3.5.
Lastly, although it is difficult to see in these visualisations, the attachment events that contribute to substantial additional biomass, i.e. when \gamma g(M)h(C)f(W_t)\approx\gamma , cease in the grid locations that have high biomass density or low substrate concentration.
Here we simulated (3.7) with the initial biomass density given by
\begin{align} M_0(x, y) = \begin{cases} -\frac{h}{d^2}\left((x-0.5)^2+(y-0.5)^2\right)+h, &\;{\rm{if }}\; (x-0.5)^2+(y-0.5)^2 < d^2, \\ 0 & \text{otherwise}, \end{cases} \end{align} | (5.1) |
with h = 0.1 , d = 5/127 , and C_0\equiv1 everywhere in \Omega . The goal of these simulations is to examine the effects of attachment when a substantial bacterial colony is already present in the system.
We present two separate simulations. Both using the default simulation parameters in Table 1 with attachment intervals [a, b] = [0.99999, 1.0] (see Figure 5) and [a, b] = [0.9999, 1] (see Figure 6).
From Figures 5 and 6 we see that before attachment events start taking place, the central colony expands radially outward. The characteristic for cellulolytic biofilms craterlike depressions form. Initially they have a rim of approximately constant thickness in the wake of which eventually all biomass decays upon depletion of all nutrients. Eventually after this crater like structure merges with other colonies, the biomass vanishes as it attempts to expand into regions in which nutrients already have been depleted, i.e., into regions in which biomass loss terms dominate over growth. This is in agreement with experimental observations reported in [25] based on microscopy and online CO _2 measurements that monitor microbial activity. Already the deterministic model in [16], on which our work is based, and the SDE model in [9], upon which we aim to improve, showed this behavior. At around t = 20 in both simulations, we see attached colonies start to form, which is consistent with the initially clean domain simulations as we use the same seeding for the stochastic processes. However, unlike the previous simulations, we see that no attachment occurs on or inside the central colony, which is caused by the lack of substrate and/or high biomass densities at these locations, and therefore unfavorable conditions for attachment per our model assumptions.
Like in the previous simulations, for larger impulse intervals, more attachment events occur, and when the impulse interval is closer to 0, attachment events occur slightly sooner. In both cases, the colonies that form due to attachment have ample time to grow and expand before they merge with the central colony. They consume the majority of the substrate in the sections of the domain which they occupy. As a result, the central colony cannot reach the edges of the domain before becoming completely distorted by the attached biomass. Unsurprisingly, the simulation with the larger attachment interval (see Figure 6) consumes the substrate quicker, distorts the central colony much sooner and to a greater degree when compared to the simulation with the smaller attachment interval (see Figure 5).
When comparing our simulations illustrated in Figures 3–6 to the empirical imaging in Figure 1 we see that Figures 3 and 5, which both use the attachment interval [a, b] = [0.99999, 1.0] , best capture the amount of biomass deposited onto these domains.
We performed a spatial grid refinement study to investigate the variations in our attachment model (3.7) as we refine the grid. Due to the stochastic nature of the model, we ran an ensemble of simulations for each grid size. We use the generally accepted notion that 30 samples are sufficient to apply the Central Limit Theorem† and thus, we perform 30 simulations for each grid size [38]. To compare the results between the grid resolutions, we keep the size of the attachment site constant. This preserves the added energy in the system.
†The use of the Central Limit Theorem will become more apparent when we discuss the stability of travelling wave solutions. We kept the number of simulations the same across different experiments for consistency sake.
We generate two statistics, the sample mean (expectation) and the standard error of the sample mean, given by
\begin{align} \text{sample mean}&: = E[X] = \frac{1}{n}\sum\limits_{i = 1}^nX_i, \end{align} | (5.2) |
\begin{align} \text{standard error of the sample mean}&: = SEM[X] = \frac{\sqrt{\frac{1}{n-1}\sum\limits_{i = 1}^n\left(X_i-E[X]\right)^2}}{\sqrt{n}}. \end{align} | (5.3) |
where X = (X_1, ..., X_n) denotes the samples and n is the number of realizations (samples).
The quantities of interest (QI) we will use are the total biomass and carbon,
\begin{align} M_{TOT}(t)&: = \int_{\Omega}M(t, x)dx = \Delta x^2\sum\limits_{p = 1}^{NK} M_p(t), \end{align} | (5.4) |
\begin{align} C_{TOT}(t)&: = \int_{\Omega}C(t, x)dx = \Delta x^2\sum\limits_{p = 1}^{NK} C_p(t), \end{align} | (5.5) |
where \Omega is the spatial domain, NK the number of grid cells, and M_p(t) , C_p(t) denote the p th component of the vectors \boldsymbol{M}(t) and \boldsymbol{C}(t) . The occupancy of the biomass and carbon,
\begin{align} M_{A}(t)&: = \int_{\Omega_M'(t)}dx = \Delta x^2\sum\limits_{p = 1}^{NK} U^M_p(t), \end{align} | (5.6) |
\begin{align} C_{A}(t)&: = \int_{\Omega_C'(t)}dx = \Delta x^2\sum\limits_{p = 1}^{NK} U^C_p(t), \end{align} | (5.7) |
where \Omega_{M}'(t): = \{x\in\Omega\mid M(t, x) > 10^{-2}\} and \Omega_{C}'(t): = \{x\in\Omega\mid C(t, x) > 10^{-2}\} , and the points U^L_p(t) for L = M, C are 1 if L_p(t) > 10^{-2} and zero otherwise. We use 10^{-2} as an approximation to 0 to avoid numerical noise. Lastly, we investigate the number of distinct biofilm colonies and depleted substrate colonies, which we denote by M_{CC} = M_{CC}(t) and C_{CC} = C_{CC}(t) for biomass and carbon respectively. To calculate the number of different colonies we determine the number of connected components using \Omega_{M/C}'(t) with the near-nearest neighbour formulation [39].
We also calculate the relative distance between the expectation curves given by
\begin{align} dist_N = \frac{\left\lVert{U_N-U_{1024}}\right\rVert_p}{\left\lVert{U_{1024}}\right\rVert_p} \end{align} | (5.8) |
with p = 1, 2, \infty denoting the usual p -norms and U_N denoting the expectation time series for the N\times N grid.
For all grid refinement simulations, we used the default parameter values given in Table 1 and an attachment interval of [a, b] = [0.99, 1.0] . We use a larger attachment interval for the grid refinement study since the number of Wiener processes is less. We set the initial conditions to M\equiv 0 and C\equiv 1 in order to simulate an initially clean domain.
The time series of the sample means of the total biomass and substrate, the occupancy, and the number of communities are given in Figures 7, 9 and 11 with the associated standard error plots given in Figures 8, 10 and 12. The relative distances between the grid sizes are given in Table 2.
Biomass Density (M) | Carbon Concentration (C) | |||||
Grid Resolution | \left\lVert{\cdot}\right\rVert_1 | \left\lVert{\cdot}\right\rVert_2 | \left\lVert{\cdot}\right\rVert_\infty | \left\lVert{\cdot}\right\rVert_1 | \left\lVert{\cdot}\right\rVert_2 | \left\lVert{\cdot}\right\rVert_\infty |
Total Mass | ||||||
64\times64 | 0.0757 | 0.0861 | 0.1135 | 0.01 | 0.0222 | 0.0648 |
128\times128 | 0.0491 | 0.0542 | 0.068 | 0.0067 | 0.0144 | 0.0401 |
256\times256 | 0.0255 | 0.0277 | 0.0338 | 0.0036 | 0.0075 | 0.0204 |
512\times512 | 0.0095 | 0.0102 | 0.0123 | 0.0014 | 0.0028 | 0.0075 |
Occupancy | ||||||
64\times64 | 0.0508 | 0.0932 | 0.2535 | 0.0125 | 0.0309 | 0.107 |
128\times128 | 0.0328 | 0.058 | 0.1483 | 0.0081 | 0.0187 | 0.0606 |
256\times256 | 0.0174 | 0.0299 | 0.0692 | 0.0042 | 0.0094 | 0.0294 |
512\times512 | 0.0067 | 0.0113 | 0.0249 | 0.0016 | 0.0035 | 0.0106 |
Communities | ||||||
64\times64 | 0.0853 | 0.1148 | 0.1761 | 0.577 | 0.6319 | 0.7099 |
128\times128 | 0.0526 | 0.0763 | 0.1179 | 0.4011 | 0.4203 | 0.4707 |
256\times256 | 0.0274 | 0.0417 | 0.0651 | 0.2106 | 0.2175 | 0.2442 |
512\times512 | 0.0111 | 0.0174 | 0.0282 | 0.0829 | 0.0822 | 0.0916 |
We see that for finer grid resolutions, the mean of the total biomass reaches a smaller peak value and the mean of the total biomass and carbon decay slower as a result, which is similar to the decay of biomass and carbon occupancy. This is interesting since \Delta x only appears in the diffusion coefficient, which plays no role for the total biomass. We also see that the mean biomass occupancy increases slower as the grid is refined. The smaller peaks and slower increase of the total mass and occupancy plots are in contrast to the colonies plots where we see higher biomass and depleted carbon colonies across the time series for finer grid resolutions. This is also reflected in the colonies standard error plots. We see variability in the number of colonies whenever they are above 0, which is to be expected based on the calculation of the statistics. The standard error plots for total mass and occupancy of biomass show an interesting two node behaviour. The two nodes correspond to the mass and occupancy of biomass increasing and decreasing whereas the valley is where the two quantities are maximized. The standard error plots for total mass and occupancy of carbon show a single peak which roughly corresponds to the maximum rate of decay in the carbon substrate.
From all the figures and tables presented in this section, we can see that as the grid resolution is refined, we have the expectation graphs and the standard error graphs approaching the associated graphs of 1024\times 1024 more closely. However, we do not see the graphs converging as closely as we may wish, but due to the computational cost of simulating 30 realizations on a 2048\times 2048 grid, we cannot include any more grid refinement. Nevertheless, we still get relatively adequate convergence results, as seen in the figures and tables.
In this section, we investigate the effects of the attachment interval on the three quantities of interest given in Section 5.2, namely the total biomass and carbon, the occupancy of biomass and carbon, and the number of biofilm colonies and fully depleted carbon colonies. In order to examine the effects of the attachment interval, we perform sensitivity analysis by varying the location of the attachment interval on the real line while keeping the length of the interval the same. For our purposes, we fix the length of the attachment interval as 0.00001 and translate the interval by \xi > 0 . We assume the base interval is [0.99999, 1.0] and generate our new interval as
\begin{align} [a, b] = [0.99999+\xi, 1.0+\xi]. \end{align} | (5.9) |
Since the initial value for each Wiener process is 0, we expect that as [a, b] deviates from 0 , the number of attachment events will decrease and the first attachment events will occur later due to the continuity of the Wiener process.
For all simulations, we set the initial conditions to M_0\equiv 0 and C_0\equiv 1 in order to simulate an initially clean domain. We use 30 realizations to generate the same test statistics as in Section 5.2. However, since we are not interested in investigating the convergence of the sample statistics, we only include a plot of the expectation time-series and include error bars at each point equal to \pm one standard deviation. We opt to use the standard deviation ( SEM(X)\sqrt{n} ) in this setting since the standard error in the sample mean is too small to detect at the current image size. The simulation results are summarized in Figures 13–15.
In all three figures, we can see that increasing \xi leads to slower initial changes in the three quantities of interest, smaller peak values and slower decay. This is caused by the longer time required for attachment events to occur and the lower number of attachment events when increasing \xi . We see from Figures 13 and 14 that the spatially integrated and occupancy plots follow each other quite closely in terms of shape, which is unsurprising due to the similarity of what they measure. The number of colonies graph, however, differs quite greatly from the other two. We see that the number of depleted carbon colonies increases rapidly shortly after the number of biomass colonies starts to decrease. This corresponds to the dominance of the -\lambda M term in the biomass equation, which occurs once the carbon has become significantly depleted.
Although it is difficult to see in the plots of the total mass and occupancy, as we increase \xi , we see that the error bars in the mass and occupancy plots increase in length, which suggests there is more variability across the given realizations. This observation of increasing error bars is following what was found in [9]. Therefore, when more attachment events occur, the deviation in how much biomass is present and how much total biomass is occupying the domain decreases. However, when we look at the colonies' plots, we typically see more variability in the biomass plots when more attachment occurs. This indicates that the number of colonies can vary more greatly when we have a higher chance of attachment events occurring.
Evidence of the existence of a 1D travelling wave solution for the deterministic models (2.1) and (2.2) has been given in [40] using a very similar numerical method to ours except they used the Implicit Euler method for the biomass equation. Furthermore, the existence of a travelling wave solution to the 1D PDE-ODE coupled system along with a linear stability result in the 2D case, has been recently shown analytically in [41]. However, there has been no analytical investigation into the stability of such a travelling wave solution under random perturbations. This is a challenging open problem and left for future research. So far, only few results have been obtained concerning the existence and stability of travelling waves for stochastic reaction-diffusion equations or PDE-ODE couplings, e.g. see [42,43,44] and the references therein. We are not aware of results for stochastic systems with degenerate diffusion.
This section is broken into two parts. First, we show that our numerical methods can accurately reproduce a travelling wave solution for the deterministic model (3.7) with \mathscr{T}(\boldsymbol{M}, \boldsymbol{C})\boldsymbol{F}\left(\boldsymbol{W_t}\right) = 0 . We then use our random attachment model to introduce random perturbations in the system, which we use to provide evidence for the stability of the travelling wave. All simulations presented in this section use the parameter values in Table 1 unless otherwise stated.
We ran a simulation of (3.7) with \mathscr{T}(\boldsymbol{M}, \boldsymbol{C})\boldsymbol{F}\left(\boldsymbol{W_t}\right) = 0 . The initial wave profile for both M and C was verified using the method described below. Figure 16 provides a summary of the simulation.
We note by the wave interface plot in Figure 17 that the 1D structure is preserved throughout the simulation. Therefore, we can stick to a given x-coordinate. To show that we have a 1D travelling wave solution, we need to show that the solution propagates at a fixed wave speed and does not change shape. We can do this graphically by horizontally translating the solution at different time points by c(t_0-{t_n}) , where c is the wave speed, t_0 is the reference time point, and t_n are the times where that solution exists pre-translation. If the translated solutions coincide, then we can conclude that there is evidence of a travelling wave solution. Therefore, we need to first estimate the wave speed of our simulation. To do this, we calculate the location of interface of the biomass wave at the time points generated throughout the simulation. To calculate the location of the wave interface, we take the largest y -coordinate that has a biomass density larger than 10^{-2} . Once we have the location of the wave interface, we can estimate the wave speed by fitting a linear function through these data points. To fit a linear model to the data set, we use the built-in function, fit , from GNUPLOT. This fits the model f(x) = cx+b to our data, where c is the estimate of the wave speed. The results are plotted in Figure 17.
With our calculated wave speed, we can now investigate whether the shape of the wave is preserved. To do this we plot the solutions M(x-c(t_0-t_n)) , where t_0 = 25.0 is the reference time point, for which we translate the waves onto (see Figure 17). The values of t_n are the time points for the other solutions. By translating the solutions by a factor of c(t_0-t_n) , we see that the solutions agree almost exactly, barring the thickness of the plotted lines. Therefore, we have sufficient evidence to suggest that the deterministic model, with our numerical method, can produce a travelling wave solution.
To start, we investigate one simulation with attachment to illustrate that the wave speed likely persists and the wave profile re-establishes. Due to the small spatial domain and time scale required for a travelling wave to establish, we start our simulation with the wave profile from Figure 16 both for the biomass density and the carbon concentration.
To simulate random perturbations of the wave, we apply attachment events only to the section of the domain where y\in[256\Delta x, 341\Delta x] , which corresponds to the y grid cells between K/2 and (2K-1)/3 , where K = 512 . The impulse interval used in this next simulation is given by [a, b] = [0.4999, 0.5] . We chose this impulse interval and the specified grid cells as we get significant attachment, but the attachment sites do not form enough biomass to destroy the wave. Figure 18 illustrates this simulation. The colours chosen in the 2D colour map are different from the previous simulations to give a higher contrast for the attachment events.
From the snapshots given in Figure 18, we see that our travelling wave seems to persist through the attachment events. We now further investigate how the wave speed, interface, and profile changes after the attachment events.
We fit our linear model to all interface values after t = 30 . Figure 19 illustrates the calculation of the wave speed. Knowing the wave speed, we can investigate whether we have the same wave profile across multiple time steps. We proceed as before. Using a reference time step t_0 = 33.0 . Figure 19 illustrates the translated wave profiles. The translation of wave profiles gets slightly more complicated as the attachment does jump the wave profile forward, which means if we take the fitted line before and after the attachment sites, the y-intercepts are not the same. Therefore, if we translate the waves by the same factor c(t_0-t_n) , our wave profiles will not necessarily coincide. We need to account for the difference in the y-intercepts. Taking the line of best fit over the interface points up to t = 15 gives us a y -intercept value for the pre-attachment wave of b_1 = 0.3101 while the y -intercept value post attachment is b_2 = 0.3099 . If we translate the first set of points 33, 34, 35 which are post-attachment by a factor of c(t_0-t_n) while translating the pre-attachment time points 5, 10, 15 by a factor of c(t_0-t_n)+b_2-b_1 we get the plot given in Figure 19.
As with our previous deterministic travelling wave simulations, we see that the profiles coincide completely across the time points, barring the thickness of the plotted curves, which suggests that the travelling wave is stable. We lastly provide a plot of the wave interface at various time points to illustrate that the interface returns to one dimension after re-establishing itself. The wave interface at various time points is given in Figure 19. The plot of the wave interface is calculated in the same manner as in the deterministic case. At a given time point t , we find the largest y -coordinate for each x -coordinate that has M(t, x, y) > 10^{-2} . Then we plot the (x, y) point. The distortions that appear in the region y\in[0.5, 0.7] are caused by the attachment events distorting the wave interface. We see that after clearing the attachment region the wave interface re-establishes.
To give a more rigorous investigation into the stability of the travelling wave, we ran a hypothesis test to investigate whether or not the wave speed changes significantly post attachment. We ran 30 simulations with different initial seeds for the pseudo-random number generation used in the updates of the Wiener process.
With the calculation of the wave speed by linear model fitting, we get an error with the model and the associated parameters, along with the associated errors that arise from the numerical method and spatial discretization. We can also get differing wave speeds depending on which values we use for our linear fitting. If we start fitting at t = 30 for the simulation described in Figure 17, then we get a wave speed of c = 0.01958 , which differs from the speed calculated from Figure 17. Therefore, we cannot expect more than 4 digits of accuracy in our wave speed calculations. Furthermore, the travelling wave in an attachment simulation typically re-establishes itself at around t = 30 . Therefore, for consistency sake, we choose c = 0.01958 as our true wave speed‡ and calculate all post-attachment wave speeds for time points after t = 30 and round to the nearest 10^{-5} . The wave speeds are given in Table 3.
‡The wave speed of the deterministic simulation calculated for interface values after t = 30 and rounded to the nearest 10^{-5} .
Seed | Wave Speed | Seed | Wave Speed | Seed | Wave Speed |
1 | 0.01959 | 11 | 0.01958 | 21 | 0.01958 |
2 | 0.01959 | 12 | 0.01957 | 22 | 0.01958 |
3 | 0.01957 | 13 | 0.01959 | 23 | 0.01957 |
4 | 0.01959 | 14 | 0.01958 | 24 | 0.01959 |
5 | 0.01958 | 15 | 0.01958 | 25 | 0.01957 |
6 | 0.01959 | 16 | 0.01958 | 26 | 0.01959 |
7 | 0.01959 | 17 | 0.01957 | 27 | 0.01957 |
8 | 0.01959 | 18 | 0.01958 | 28 | 0.01959 |
9 | 0.01957 | 19 | 0.01958 | 29 | 0.01956 |
10 | 0.01959 | 20 | 0.01959 | 30 | 0.01957 |
With these data, we can conduct a hypothesis test to determine if there is a significant statistical difference between computed post-attachment wave speeds and the deterministic wave speed. We assert the null hypothesis that the true mean wave speed post attachment is the same as the true wave speed, and use a two-sided alternative hypothesis. To generate the statistics, we use the OneSampleTTest function from the Hypothesis Tests package in Julia. Given our computed post attachment wave speeds c_i , we get the following statistics:
\begin{align} \overline c = 0.0195813, \quad SEM(\overline c)\approx 1.64235\times 10^{-6}, \quad t_{\overline c}\approx 0.811844, \quad p\approx0.4235. \end{align} | (5.10) |
Given our large p -value of 0.4235 , we fail to reject the null hypothesis that the true mean wave speeds are the same post attachment. Constructing a 95% confidence interval about the sample mean gives (0.019578, 0.019585) . Given our previous discussion on the associated errors from the numerical methods and the linear fitting, we conclude that our calculated post attachment wave speeds are within the margin of error of the deterministic wave speed. Thus, concluding that we have significant evidence in favour of a stable travelling wave solution.
The main objective of this work was to develop a new mathematical modelling framework for discrete stochastic attachment that improved on the drawbacks of the previous attempts in [9,16]. Namely, a lack of a rigorous mathematical framework in [16] and expensive simulation runtime in [9]. Through applying our framework to the PDE-ODE coupled system for cellulolytic biofilm growth we gained more theoretical insight into our model while also greatly improving the simulation runtime when compared to the model presented in [9].
The nature of stochastic cellular attachment, along with the lack of experimental data, makes it difficult to propose a cellular attachment framework based on underlying mechanisms in the system. This makes the stochastic approach used here adequate for now. However, if future experiments and analyses can provide a mechanism for cellular attachment in a fully deterministic way, the resulting model will likely capture the system behaviour in a more concise manner.
The use of the RODE framework lent itself better to capturing attachment events when compared to the Itô SDE framework used in [9]. Our framework omits the addition of an extra NK DEs used to model the attachment signal; we were able to lump that into a stochastic process for each grid cell. This modification simplifies the mathematical theory we can rely on since RODEs are path-wise ODEs. We were able to show the global existence and uniqueness of a regularized version of our model and the long-term behaviour of solutions, which was unattainable with the SDE framework.
The elimination of the extra NK DEs from the model in [9] does come with some notable disadvantages, mostly related to the fitting of the model. The attachment factor used in [9] had additional parameters and depended on state variables, a mechanism that is not present in our formulation. In our model, if the biomass was close to unity or the substrate was close to zero, the Wiener process was still simulated and thus, could still cause an attachment event to take place. Now, since the biomass and substrate would hinder the amount of biomass attached (by a factor of g(M)h(C) ), we would not have much biomass attached; however, there would still be some. However, with the model from [9], the attachment factor was also controlled by the state variables in a similar manner as the attachment term in our model. Therefore, when the biomass and carbon were inhibiting attachment, they were also inhibiting the attachment factor, which for most realizations meant no attachment would occur instead of the possibility for attachment events.
For simplicity, we used the Wiener process, as we can simply use a random number generator to simulate it. Moreover, the qualitative behaviour of the model is as desired. We tried other stochastic processes, including the Ornstein-Uhlenbeck process and found that the qualitative behaviour of the model did not change; however, the simulation time did increase. It is an interesting question to see how different stochastic processes can affect the model behaviour. However, this is outside the scope of our research.
The use of an implicit time integrator for our model allowed us to drastically improve simulation times when compared to the explicit solver used in [9] (by a factor of over 300%). Unfortunately, some of the traditional disadvantages of an implicit method became apparent in our situation. The major issue arises with larger time steps; we can lose out on some of the attachment events if the computed values of the Wiener process bracket the impulse interval without being in the interval, which means we introduce more errors. The lengths of the attachment intervals used in Section 5.1 are 0.0001 and 0.00001. When we simulate the Wiener process, we use the formula W_{k+1} = W_k+\sqrt{\Delta t}\Delta W_k where \Delta W_k is a standard normal random variable. The probability that |\Delta W_k| > 1 is roughly 31.8%. Therefore, to avoid missing attachment events 68.2% of the time, we need \Delta t to be either 10^{-8} or 10^{-10} , which is way too low for an implicit time step and is much closer to the time steps used in [9]. This is the main reason why changing the impulse interval in Section 5.1 lead to significantly more attachment events since the Wiener processes more likely attained a value within the interval when the interval is larger. To mitigate this issue, we could use a different stochastic process, preferably one with less variance, so that the increments are scaled by a smaller number, which would lower the likelihood of missing attachment events. We could have also used a finer step size to simulate the Wiener process. Meaning, if \Delta t is our implicit time step, then we could use the time step \Delta t/N to simulate the Wiener process. This does come with some challenges though. For example, if we have an attachment event occurring on some fraction of \Delta t , then it is unclear how much should be attached at the \Delta t time step. Moreover, we need to decide how to handle the case where a Wiener process enters and exits the attachment interval more than once throughout a \Delta t time step. Lastly, in order to ensure that we have an appropriate number of attachment events we will need to shrink the size of our attachment interval. However, shrinking the size of the interval implies a higher chance of missing attachment events. For these reasons, we simulated our Wiener processes using the \Delta t time step as it gave us the desired qualitative behaviour.
From a practical standpoint, the large time step does not affect our model in a meaningful way. Since we have no experimental data to calibrate our model to, we cannot currently use our model to predict quantitative behaviour. Furthermore, spatio-temporally resolved experimental studies are very difficult and the current microscopy is not able to give the spatial and dynamic resolution that would be needed for a direct calibration. However, if more experimental data were available or in cases where we wished to use our framework in a more sophisticated system, we may have concerns with missing attachment events. These concerns also manifest themselves when we consider the reduced control we have over the rate of attachment events, as we have less chance of properly calibrating our model or properly capturing system behaviour in complex systems. A more complex system where these concerns will likely matter is any system where we have bulk liquid surrounding the biofilm such that the availability of free cells is determined by this liquid.
Since we investigated the effects of the attachment interval, knowing that we likely missed attachment events, we provide some validation to our methods here. We chose to fix the length of the attachment interval and vary its position on the real line as we conjectured that preserving the length of the attachment interval would lead to roughly the same proportion of missed attachment events. So, here we investigate that claim. We took twelve different attachment intervals of the form
\begin{align*} [a, b] = [0.49999+\xi, 0.5+\xi] \end{align*} |
with an integer \xi in the range 0 – 11 and simulated 512\times 512 Wiener process over 200 time units with a time step of 10^{-2} . We counted the number of attachment events along with the number of missed attachment events§ and took the ratio of missed attachment events over counted attachment events. The data are presented in Table 4. As we can see, we roughly miss 8000 attachment events for every 1 attachment event we count. However, across all twelve \xi values, we see that the proportion of missed events is roughly equal, which suggests that our investigation into qualitative effects of the attachment interval is justified.
§Missed attachment events are when the current and next value of the Wiener process bracket the impulse interval.
\xi | Ratio | \xi | Ratio | \xi | Ratio | \xi | Ratio |
0.0 | 7915.35 | 1.0 | 7946.69 | 2.0 | 7903.99 | 3.0 | 7921.85 |
4.0 | 8280.92 | 5.0 | 8030.46 | 6.0 | 8085.27 | 7.0 | 7709.58 |
8.0 | 8176.43 | 9.0 | 8178.81 | 10.0 | 8177.22 | 11.0 | 7821.86 |
Throughout Section 5 we often ran 30 simulations with the same parameter values to generate different sample statistics or to run hypothesis tests. As outlined in that chapter, we chose 30 simulations as that is the unwritten rule to apply the Central Limit Theorem. However, the Central Limit Theorem typically applies when we are interested in hypothesis testing, but we chose 30 simulations for consistency's sake. Here we provide further justification for the use of 30 realizations, which is summarized in Figure 20. To generate these plots we ran 100 simulations with the parameter values in Table 1 with [a, b] = [0.99999, 1.0] and calculated the 1-norm of the standard deviation time-series for each quantity of interest in Chapter 5. The simulations were run until t = 70 . We see that the standard deviation does not change drastically after 30 samples, supporting our decision.
In our work, the deterministic model is the spatial discretized PDE-ODE coupled system (2.11). We could argue that our deterministic model is the PDE-ODE coupled systems (2.1) and (2.2). The reason why we work with the discretized version is the discrete nature of attachment. PDEs work under the assumption of infinitesimals, which does not lend well to the spatially discrete attachment. However, if we fixed the size of an attachment event, we likely could generalize our model to a PDE via the use of non-local effects. We could take the original PDE-ODE coupled system and add some linear combination of convolutions to add attachment to all locations in a grid cell via the influence of a Wiener process. This construction was outside the scope of this work; however, it would be very interesting to develop and analyze such a model and see if our spatially discrete system (3.7) converges as \Delta x\to0 .
Discretized deterministic continuous biofilm models can be extended into RODEs in order to capture discrete stochastic attachment events via an impulse function with a standard stochastic process as input. We see that this formulation preserves the non-negativity of the system, lends itself well to extending results on the deterministic model, and improves simulation runtime greatly when compared to earlier formulations. Our attachment model (3.7) along with the proposed numerical methods, can also be used to investigate the model behaviour of the underlying deterministic model. Namely, we gave evidence to suggest that there exists a stable 1D travelling wave solution to the PDE-ODE coupled systems (2.1) and (2.2).
The model developed in this paper is for the particular case of cellulosic biofilms. We think this modelling framework could be applied to other two or three dimensional continuum models, including traditional biofilm models where nutrients are supplied via diffusion from the aqueous phase.
This work was supported by a Natural Sciences and Engineering Research Council of Canada (NSERC) CGS-M awarded to JMH, and by an NSERC Discovery Grant (RGPIN-2019-05003) and NSERC Research Tools and Infrastructure Grant (RTI-2016-00080) awarded to HJE. It was supported by the Nederlands Organisatie voor Wetenschappelijke Onderzoek (NWO) with grant OCENW.KLEIN.358, awarded to SS.
No conflicts are declared.
[1] | B. Suthar, H. Patel, A. Goswami, A survey: classification of imputation methods in data mining, Int. J. Emerging Technol. Adv. Eng. , 2 (2012), 309–312. |
[2] | R. Houari, A. Bounceur, A. K. Tari, M. T. Kecha, Handling missing data problems with sampling methods, in 2014 International Conference on Advanced Networking Distributed Systems and Applications, (2014), 99–104. http://doi.org/10.1109/INDS.2014.25 |
[3] | P. E. McKnight, K. M. McKnight, S. Sidani, A. J. Figueredo, Missing Data: A Gentle Introduction, Guilford Press, 2007. |
[4] |
O. F. Ayilara, L. Zhang, T. T. Sajobi, R. Sawatzky, E. Bohm, L. M. Lix, Impact of missing data on bias and precision when estimating the change in patient-reported outcomes from a clinical registry, Health Qual. Life Outcomes, 17 (2019), 106. http://doi.org/10.1186/s12955-019-1181-2 doi: 10.1186/s12955-019-1181-2
![]() |
[5] |
H. Kang, The prevention and handling of the missing data, Korean J. Anesthesiol. , 64 (2013), 402–409. http://doi.org/10.4097/kjae.2013.64.5.402 doi: 10.4097/kjae.2013.64.5.402
![]() |
[6] |
T. Donders, G. Van Der Heijden, T. Stijnen, K. Moons, A gentle introduction to imputation of missing values, J. Clin. Epidemiol. , 59 (2006), 1087–1091. http://doi.org/10.1016/j.jclinepi.2006.01.014 doi: 10.1016/j.jclinepi.2006.01.014
![]() |
[7] |
J. Ludbrook, Outlying observations and missing values: how should they be handled?, Clin. Exp. Pharmacol. Physiol. , 35 (2008), 670–678. http://doi.org/10.1111/j.1440-1681.2007.04860.x. doi: 10.1111/j.1440-1681.2007.04860.x
![]() |
[8] |
Z. Zhang, Missing values in big data research: some basic skills, Ann. Trans. Med. , 3 (2015), 75–84. https://doi.org/10.3978/j.issn.2305-5839.2015.12.11 doi: 10.3978/j.issn.2305-5839.2015.12.11
![]() |
[9] |
D. Langkamp, A. Lehman, S. Lemeshow, Techniques for handling missing data in secondary analyses of large surveys, Acad. Pediatr. , 10 (2010), 205–210. https://doi.org/doi:10.1016/j.acap.2010.01.005 doi: 10.1016/j.acap.2010.01.005
![]() |
[10] | J. T. Shawe, N. Cristianini, Kernel Methods for Pattern Analysis, Cambridge University Press, United Kingdom, 2004. |
[11] |
J. W. Graham, Missing data analysis: Making it work in the real world, Ann. Rev. Psychol. , 60 (2009), 549–576. http://doi.org/10.1146/annurev.psych.58.110405.085530 doi: 10.1146/annurev.psych.58.110405.085530
![]() |
[12] |
A. N. Baraldi, C. K. Enders, An introduction to modern missing data analyses, J. Sch. Psychol. , 48 (2010), 5–37. https://doi.org/10.1016/j.jsp.2009.10.001 doi: 10.1016/j.jsp.2009.10.001
![]() |
[13] |
I. Muhammad, Z. Yan, Supervised machine learning approaches: A survey, ICTACT J. Soft Comput. , 5 (2015), 115–119. http://doi.org/10.21917/ijsc.2015.0133 doi: 10.21917/ijsc.2015.0133
![]() |
[14] | C. Nithya, V. Saravanan, A study of machine learning techniques in data mining, Int. Sci. Refereed Res. J. , 1 (2018), 31–38. |
[15] |
I. B. Aydilek, A. Arslan, A hybrid method for imputation of missing values using optimized fuzzy c-means with support vector regression and a genetic algorithm, Inf. Sci., 233 (2013), 25–35. https://doi.org/10.1016/j.ins.2013.01.021 doi: 10.1016/j.ins.2013.01.021
![]() |
[16] |
J. Lin, N. Li, M. A. Alam, Y. Ma, Data-driven missing data imputation in cluster monitoring system based on deep neural network, Appl. Intell., 50 (2020), 860–877. http://doi.org/10.1007/s10489-019-01560-y doi: 10.1007/s10489-019-01560-y
![]() |
[17] | A. Choudhury, M. Kosorok, Missing data imputation for classification problems, preprint, arxiv: 2002.10709. |
[18] |
B. Al-Helali, Q. Chen, B. Xue, M. Zhang, A new imputation method based on genetic programming and weighted K-NN for symbolic regression with incomplete data, Soft Comput., 25 (2021), 5993–6012. http://doi.org/10.1007/s00500-021-05590-y doi: 10.1007/s00500-021-05590-y
![]() |
[19] |
D. Peng, M. Zou, C. Liu, J. Lu, RESI: A region-splitting imputation method for different types of missing data, Exp. Syst. Appl., 168 (2021), 114425. https://doi.org/10.1016/j.eswa.2020.114425 doi: 10.1016/j.eswa.2020.114425
![]() |
[20] |
J. Fan, F. Han, H. Liu, Challenges of big data analysis, Nat. Sci. Rev., 1 (2014), 293–314. https://doi.org/10.1093/nsr/nwt032 doi: 10.1093/nsr/nwt032
![]() |
[21] |
J. Qiu, Q. Wu, G. Ding, Y. Xu, S. Feng, A survey of machine learning for big data processing, EURASIP J. Adv. Signal Proc., 2016 (2016), 67. https://doi.org/10.1186/s13634-016-0355-x doi: 10.1186/s13634-016-0355-x
![]() |
[22] |
T. Hao, Z. Huang, L. Liang, B. Tang, Biomedical and health information processing and analysis, Math. Biosci. Eng., 17 (2020), 5147–5149. http://doi.org/10.3934/mbe.2020278 doi: 10.3934/mbe.2020278
![]() |
[23] | K. Machhale, H. B. Nandpuru, V. Kapur, L. Kosta, MRI brain cancer classification using hybrid classifier (SVM-KNN), in 2015 International Conference on Industrial Instrumentation and Control (ICIC), (2015). https://doi.org/10.1109/SCEECS.2014.6804439 |
[24] |
E. Dritsas, M. Trigka, P. Gerolymatos, S. Sioutas, Trajectory clustering and k-NN for robust privacy preserving spatiotemporal databases, Algorithms, 11 (2020), 207–210. https://doi.org/10.3390/a11120207 doi: 10.3390/a11120207
![]() |
[25] | L. Beretta, A. Santaniello, Nearest neighbour imputation algorithms: a critical evaluation, in The 5th Translational Bioinformatics Conference (TBC 2015), (2015), 1–7. http://doi.org/10.1186/s12911-016-0318-z |
[26] | J. Derrac, F. Chiclana, S. Garcia, F. Herrera, An interval-valued k-nearest neighbor's classifier, in 16th World Congress of the International Fuzzy Systems Association (IFSA), (2015), 378–384. https://doi.org/10.2991/ifsa-eusflat-15.2015.55 |
[27] | R. Armina, A. Zain, N. Ali, R. Sallehuddin, A review on missing value estimation using imputation algorithm, in International Conference on Materials Physics and Mechanics, (2017), 892–895. http://doi.org/10.1088/1742-6596/892/1/012004 |
[28] |
H. Nezamabadi-pour, E. Kabir, Concept learning by fuzzy k-NN classification and relevance feedback for efficient image retrieval, Exp. Syst. Appl., 36 (2009), 5948–5954. https://doi.org/10.1016/j.eswa.2008.07.008 doi: 10.1016/j.eswa.2008.07.008
![]() |
[29] |
A. L. Green, A. Arnaud, J. Batiller, S. Eljamel, J. Gauld, P. Jones, et al., A multi-centre, prospective, randomized controlled study to evaluate the use of a fibrin sealant as an adjunct to sutured dural repair, Br. J. Neurosurg., 29 (2015), 11–17. http://doi.org/10.3109/02688697.2014.948808 doi: 10.3109/02688697.2014.948808
![]() |
[30] |
W. I. Schievink, M. M. Maya, S. Jean-Pierre, M. Nuño, R.S. Prasad, F. G. Moser, A classification system of spontaneous spinal CSF leaks, Neurology, 87 (2016), 673–679. https://doi.org/10.1212/WNL.0000000000002986 doi: 10.1212/WNL.0000000000002986
![]() |
[31] |
S. Chowdhary, S. Damlo, M. C. Chamberlain, Cerebrospinal fluid dissemination and neoplastic meningitis in primary brain tumors, J. Moffitt Cancer Center, 24 (2017), 1–16. https://doi.org/10.1177/107327481702400118 doi: 10.1177/107327481702400118
![]() |
[32] |
B. Srinivas, R. G. Sasibhushana, A hybrid CNN-KNN model for MRI brain tumor classification, Int. J. Recent Technol. Eng., 8 (2019), 5230–5235. https://doi.org/10.35940/ijrte.B1051.078219 doi: 10.35940/ijrte.B1051.078219
![]() |
[33] |
L. Po, N. Bikakis, F. Desimoni, G. Papastefanatos, Linked data visualization: Techniques, tools, and big data, Synth. Lect. Semantic Web Theory Technol., 10 (2020), 1–157. https://doi.org/10.2200/S00967ED1V01Y201911WBE019 doi: 10.2200/S00967ED1V01Y201911WBE019
![]() |
[34] | E. Chu, Discrete and continuous Fourier transforms: analysis, applications and fast algorithms, in Chapman and Hall/CRC, (2008), 424–458. |
[35] | G. Niranjana, D. Chatterjee, Security and privacy issues in biomedical AI systems and potential solutions, in Handbook of Artificial Intelligence in Biomedical Engineering, Apple Academic Press, 2021. |
[36] |
Y. Zhang, S. Wang, J. Yang, Z. Zhang, P. Phillips, P. Sun, et al., A comprehensive survey on fractional Fourier transform, Fundam. Inf., 151 (2017), 1–48. https://doi.org/10.3233/FI-2017-1477 doi: 10.3233/FI-2017-1477
![]() |
[37] |
S. Saeed, A. Abdullah, N. Z. Jhanjhi, Implementation of Fourier transformation with brain cancer and CSF images, Indian J. Sci. Technol., 12 (2019), 1–9. https://doi.org/10.17485/ijst/2019/v12i37/146151. doi: 10.17485/ijst/2019/v12i37/146151
![]() |
[38] |
A. Q. Khan, I. Ahmad, H. S. Alayachi, M. S. Noorani, A. Khaliq, Discrete-time predator-prey model with flip bifurcation and chaos control, Math. Biosci. Eng., 17 (2020), 5944–5960. http://doi.org/10.3934/mbe.2020317 doi: 10.3934/mbe.2020317
![]() |
[39] | Alsisi A, Eftimie R, Trucu D. Nonlocal multiscale modelling of tumour-oncolytic viruses interactions within a heterogeneous fibrous/non-fibrous extracellular matrix, preprint, arXiv: 2112.09732. |
[40] |
S. Wang, M. Celebi, Y. Zhang, X. Yu, S. Lu, X. Yao, et al., Advances in data preprocessing for biomedical data fusion: an overview of the methods, challenges, and prospects, Inf. Fusion, 76 (2021), 376–421. https://doi.org/10.1016/j.inffus.2021.07.001 doi: 10.1016/j.inffus.2021.07.001
![]() |
[41] |
Y. Zhang, Z. Dong, S. Wang, X. Yu, X. Yao, Q. Zhou, et al., Advances in multimodal data fusion in neuroimaging: overview, challenges, and novel orientation, Inf. Fusion, 64 (2020), 149–187. https://doi.org/10.1016/j.inffus.2020.07.006 doi: 10.1016/j.inffus.2020.07.006
![]() |
[42] | D. G. Dansereau, B. Girod, G. Wetzstein, Supplementary material LiFF: Light field features in scale and depth, in Proceeding: IEEE/CVF Conference on Computer Vision and Pattern Recognition (CVPR), (2019). https://doi.org/10.1109/CVPR.2019.00823 |
[43] | A. Silva, Light field processor: a lytro illum imaging application, Ph. D thesis, University Institute of Lisbon, 2016. |
1. | Vincenzo Luongo, Maria Rosaria Mattei, Luigi Frunzo, Berardino D'Acunto, Kunal Gupta, Shankararaman Chellam, Nick Cogan, A transient biological fouling model for constant flux microfiltration, 2022, 20, 1551-0018, 1274, 10.3934/mbe.2023058 | |
2. | K. Mitra, J. M. Hughes, S. Sonner, H. J. Eberl, J. D. Dockery, Travelling Waves in a PDE–ODE Coupled Model of Cellulolytic Biofilms with Nonlinear Diffusion, 2023, 1040-7294, 10.1007/s10884-022-10240-4 | |
3. | J. Vincent, A. Tenore, M.R. Mattei, L. Frunzo, Modelling drinking water biofilms: Bacterial adhesion and Legionella pneumophila necrotrophic growth, 2024, 128, 10075704, 107639, 10.1016/j.cnsns.2023.107639 |
Parameter | Symbol | Value | Reference |
Motility Coefficient | \tilde\delta | 10^{-6} | [16] |
Diffusion Coefficient Exponent 1 | \alpha | 4.0 | [16] |
Diffusion Coefficient Exponent 2 | \beta | 4.0 | [16] |
Maximum Consumption Rate | \tilde\Upsilon | 0.4 | [16] |
Half Saturation Concentration | \tilde\kappa | 0.01 | [16] |
Cell Loss Rate | \tilde\lambda | 0.42 | [16] |
Control Parameter of f | \sigma | 0.000001 | assumed |
Shift Parameters of f | a & b | Varied | assumed |
Density of an Attached Cell per Time Step | \gamma | 0.01 | assumed |
Biomass Attachment Exponents | \nu_{1/2} | 2.0 | assumed |
Domain | \Omega | [0, 1]\times[0, 1] | assumed |
Grid Size | N\times K | 2^{9}\times 2^{9} | assumed |
Step Size | \Delta t | 10^{-2} | assumed |
Seed | - | 1 | assumed |
Biomass Density (M) | Carbon Concentration (C) | |||||
Grid Resolution | \left\lVert{\cdot}\right\rVert_1 | \left\lVert{\cdot}\right\rVert_2 | \left\lVert{\cdot}\right\rVert_\infty | \left\lVert{\cdot}\right\rVert_1 | \left\lVert{\cdot}\right\rVert_2 | \left\lVert{\cdot}\right\rVert_\infty |
Total Mass | ||||||
64\times64 | 0.0757 | 0.0861 | 0.1135 | 0.01 | 0.0222 | 0.0648 |
128\times128 | 0.0491 | 0.0542 | 0.068 | 0.0067 | 0.0144 | 0.0401 |
256\times256 | 0.0255 | 0.0277 | 0.0338 | 0.0036 | 0.0075 | 0.0204 |
512\times512 | 0.0095 | 0.0102 | 0.0123 | 0.0014 | 0.0028 | 0.0075 |
Occupancy | ||||||
64\times64 | 0.0508 | 0.0932 | 0.2535 | 0.0125 | 0.0309 | 0.107 |
128\times128 | 0.0328 | 0.058 | 0.1483 | 0.0081 | 0.0187 | 0.0606 |
256\times256 | 0.0174 | 0.0299 | 0.0692 | 0.0042 | 0.0094 | 0.0294 |
512\times512 | 0.0067 | 0.0113 | 0.0249 | 0.0016 | 0.0035 | 0.0106 |
Communities | ||||||
64\times64 | 0.0853 | 0.1148 | 0.1761 | 0.577 | 0.6319 | 0.7099 |
128\times128 | 0.0526 | 0.0763 | 0.1179 | 0.4011 | 0.4203 | 0.4707 |
256\times256 | 0.0274 | 0.0417 | 0.0651 | 0.2106 | 0.2175 | 0.2442 |
512\times512 | 0.0111 | 0.0174 | 0.0282 | 0.0829 | 0.0822 | 0.0916 |
Seed | Wave Speed | Seed | Wave Speed | Seed | Wave Speed |
1 | 0.01959 | 11 | 0.01958 | 21 | 0.01958 |
2 | 0.01959 | 12 | 0.01957 | 22 | 0.01958 |
3 | 0.01957 | 13 | 0.01959 | 23 | 0.01957 |
4 | 0.01959 | 14 | 0.01958 | 24 | 0.01959 |
5 | 0.01958 | 15 | 0.01958 | 25 | 0.01957 |
6 | 0.01959 | 16 | 0.01958 | 26 | 0.01959 |
7 | 0.01959 | 17 | 0.01957 | 27 | 0.01957 |
8 | 0.01959 | 18 | 0.01958 | 28 | 0.01959 |
9 | 0.01957 | 19 | 0.01958 | 29 | 0.01956 |
10 | 0.01959 | 20 | 0.01959 | 30 | 0.01957 |
\xi | Ratio | \xi | Ratio | \xi | Ratio | \xi | Ratio |
0.0 | 7915.35 | 1.0 | 7946.69 | 2.0 | 7903.99 | 3.0 | 7921.85 |
4.0 | 8280.92 | 5.0 | 8030.46 | 6.0 | 8085.27 | 7.0 | 7709.58 |
8.0 | 8176.43 | 9.0 | 8178.81 | 10.0 | 8177.22 | 11.0 | 7821.86 |
Parameter | Symbol | Value | Reference |
Motility Coefficient | \tilde\delta | 10^{-6} | [16] |
Diffusion Coefficient Exponent 1 | \alpha | 4.0 | [16] |
Diffusion Coefficient Exponent 2 | \beta | 4.0 | [16] |
Maximum Consumption Rate | \tilde\Upsilon | 0.4 | [16] |
Half Saturation Concentration | \tilde\kappa | 0.01 | [16] |
Cell Loss Rate | \tilde\lambda | 0.42 | [16] |
Control Parameter of f | \sigma | 0.000001 | assumed |
Shift Parameters of f | a & b | Varied | assumed |
Density of an Attached Cell per Time Step | \gamma | 0.01 | assumed |
Biomass Attachment Exponents | \nu_{1/2} | 2.0 | assumed |
Domain | \Omega | [0, 1]\times[0, 1] | assumed |
Grid Size | N\times K | 2^{9}\times 2^{9} | assumed |
Step Size | \Delta t | 10^{-2} | assumed |
Seed | - | 1 | assumed |
Biomass Density (M) | Carbon Concentration (C) | |||||
Grid Resolution | \left\lVert{\cdot}\right\rVert_1 | \left\lVert{\cdot}\right\rVert_2 | \left\lVert{\cdot}\right\rVert_\infty | \left\lVert{\cdot}\right\rVert_1 | \left\lVert{\cdot}\right\rVert_2 | \left\lVert{\cdot}\right\rVert_\infty |
Total Mass | ||||||
64\times64 | 0.0757 | 0.0861 | 0.1135 | 0.01 | 0.0222 | 0.0648 |
128\times128 | 0.0491 | 0.0542 | 0.068 | 0.0067 | 0.0144 | 0.0401 |
256\times256 | 0.0255 | 0.0277 | 0.0338 | 0.0036 | 0.0075 | 0.0204 |
512\times512 | 0.0095 | 0.0102 | 0.0123 | 0.0014 | 0.0028 | 0.0075 |
Occupancy | ||||||
64\times64 | 0.0508 | 0.0932 | 0.2535 | 0.0125 | 0.0309 | 0.107 |
128\times128 | 0.0328 | 0.058 | 0.1483 | 0.0081 | 0.0187 | 0.0606 |
256\times256 | 0.0174 | 0.0299 | 0.0692 | 0.0042 | 0.0094 | 0.0294 |
512\times512 | 0.0067 | 0.0113 | 0.0249 | 0.0016 | 0.0035 | 0.0106 |
Communities | ||||||
64\times64 | 0.0853 | 0.1148 | 0.1761 | 0.577 | 0.6319 | 0.7099 |
128\times128 | 0.0526 | 0.0763 | 0.1179 | 0.4011 | 0.4203 | 0.4707 |
256\times256 | 0.0274 | 0.0417 | 0.0651 | 0.2106 | 0.2175 | 0.2442 |
512\times512 | 0.0111 | 0.0174 | 0.0282 | 0.0829 | 0.0822 | 0.0916 |
Seed | Wave Speed | Seed | Wave Speed | Seed | Wave Speed |
1 | 0.01959 | 11 | 0.01958 | 21 | 0.01958 |
2 | 0.01959 | 12 | 0.01957 | 22 | 0.01958 |
3 | 0.01957 | 13 | 0.01959 | 23 | 0.01957 |
4 | 0.01959 | 14 | 0.01958 | 24 | 0.01959 |
5 | 0.01958 | 15 | 0.01958 | 25 | 0.01957 |
6 | 0.01959 | 16 | 0.01958 | 26 | 0.01959 |
7 | 0.01959 | 17 | 0.01957 | 27 | 0.01957 |
8 | 0.01959 | 18 | 0.01958 | 28 | 0.01959 |
9 | 0.01957 | 19 | 0.01958 | 29 | 0.01956 |
10 | 0.01959 | 20 | 0.01959 | 30 | 0.01957 |
\xi | Ratio | \xi | Ratio | \xi | Ratio | \xi | Ratio |
0.0 | 7915.35 | 1.0 | 7946.69 | 2.0 | 7903.99 | 3.0 | 7921.85 |
4.0 | 8280.92 | 5.0 | 8030.46 | 6.0 | 8085.27 | 7.0 | 7709.58 |
8.0 | 8176.43 | 9.0 | 8178.81 | 10.0 | 8177.22 | 11.0 | 7821.86 |