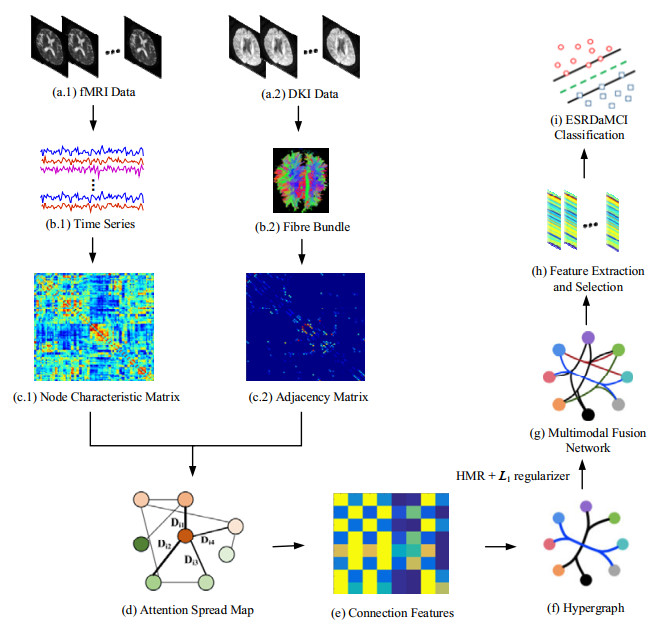
Microfiltration is a widely used engineering technology for fresh water production and water treatment. The major concern in many applications is the formation of a biological fouling layer leading to increased hydraulic resistance and flux decline during membrane operations. The growth of bacteria constituting such a biological layer implicates the formation of a multispecies biofilm and the consequent increase of operational costs for reactor management and cleaning procedures. To predict the biofouling evolution, a mono-dimensional continuous free boundary model describing biofilm dynamics and EPS production in different operational phases of microfiltration systems has been well studied. The biofouling growth is governed by a system of hyperbolic PDEs. Substrate dynamics are modeled through parabolic equations accounting for diffusive and advective fluxes generated during the filtration process. The free boundary evolution depends on both microbial growth and detachment processes. What is not addressed is the interplay between biofilm dynamics, filtration, and water recovery. In this study, filtration and biofilm growth modeling principles have been coupled for the definition of an original mathematical model able to reproduce biofouling evolution in membrane systems. The model has been solved numerically to simulate biologically relevant conditions, and to investigate the hydraulic behavior of the membrane. It has been calibrated and validated using lab-scale data. Numerical results accurately predicted the pressure drop occurring in the microfiltration system. A calibrated model can give information for optimization protocols as well as fouling prevention strategies.
Citation: Vincenzo Luongo, Maria Rosaria Mattei, Luigi Frunzo, Berardino D'Acunto, Kunal Gupta, Shankararaman Chellam, Nick Cogan. A transient biological fouling model for constant flux microfiltration[J]. Mathematical Biosciences and Engineering, 2023, 20(1): 1274-1296. doi: 10.3934/mbe.2023058
[1] | Chaofan Song, Tongqiang Liu, Huan Wang, Haifeng Shi, Zhuqing Jiao . Multi-modal feature selection with self-expression topological manifold for end-stage renal disease associated with mild cognitive impairment. Mathematical Biosciences and Engineering, 2023, 20(8): 14827-14845. doi: 10.3934/mbe.2023664 |
[2] | Yu Lu, Tongqiang Liu, Quan Sheng, Yutao Zhang, Haifeng Shi, Zhuqing Jiao . Predicting the cognitive function status in end-stage renal disease patients at a functional subnetwork scale. Mathematical Biosciences and Engineering, 2024, 21(3): 3838-3859. doi: 10.3934/mbe.2024171 |
[3] | Wei Kong, Feifan Xu, Shuaiqun Wang, Kai Wei, Gen Wen, Yaling Yu . Application of orthogonal sparse joint non-negative matrix factorization based on connectivity in Alzheimer's disease research. Mathematical Biosciences and Engineering, 2023, 20(6): 9923-9947. doi: 10.3934/mbe.2023435 |
[4] | Xia Xu, Song Xu, Liting Han, Xufeng Yao . Coupling analysis between functional and structural brain networks in Alzheimer's disease. Mathematical Biosciences and Engineering, 2022, 19(9): 8963-8974. doi: 10.3934/mbe.2022416 |
[5] | Jiyun Shen, Yiyi Xia, Yiming Lu, Weizhong Lu, Meiling Qian, Hongjie Wu, Qiming Fu, Jing Chen . Identification of membrane protein types via deep residual hypergraph neural network. Mathematical Biosciences and Engineering, 2023, 20(11): 20188-20212. doi: 10.3934/mbe.2023894 |
[6] | Wei Yin, Tao Yang, GuangYu Wan, Xiong Zhou . Identification of image genetic biomarkers of Alzheimer's disease by orthogonal structured sparse canonical correlation analysis based on a diagnostic information fusion. Mathematical Biosciences and Engineering, 2023, 20(9): 16648-16662. doi: 10.3934/mbe.2023741 |
[7] | Dominique Duncan, Thomas Strohmer . Classification of Alzheimer's disease using unsupervised diffusion component analysis. Mathematical Biosciences and Engineering, 2016, 13(6): 1119-1130. doi: 10.3934/mbe.2016033 |
[8] | Sathyanarayanan Gopalakrishnan, Swaminathan Venkatraman . Prediction of influential proteins and enzymes of certain diseases using a directed unimodular hypergraph. Mathematical Biosciences and Engineering, 2024, 21(1): 325-345. doi: 10.3934/mbe.2024015 |
[9] | Xiaowen Jia, Jingxia Chen, Kexin Liu, Qian Wang, Jialing He . Multimodal depression detection based on an attention graph convolution and transformer. Mathematical Biosciences and Engineering, 2025, 22(3): 652-676. doi: 10.3934/mbe.2025024 |
[10] | Sangita Swapnasrita, Joost C de Vries, Carl M. Öberg, Aurélie MF Carlier, Karin GF Gerritsen . Computational modeling of peritoneal dialysis: An overview. Mathematical Biosciences and Engineering, 2025, 22(2): 431-476. doi: 10.3934/mbe.2025017 |
Microfiltration is a widely used engineering technology for fresh water production and water treatment. The major concern in many applications is the formation of a biological fouling layer leading to increased hydraulic resistance and flux decline during membrane operations. The growth of bacteria constituting such a biological layer implicates the formation of a multispecies biofilm and the consequent increase of operational costs for reactor management and cleaning procedures. To predict the biofouling evolution, a mono-dimensional continuous free boundary model describing biofilm dynamics and EPS production in different operational phases of microfiltration systems has been well studied. The biofouling growth is governed by a system of hyperbolic PDEs. Substrate dynamics are modeled through parabolic equations accounting for diffusive and advective fluxes generated during the filtration process. The free boundary evolution depends on both microbial growth and detachment processes. What is not addressed is the interplay between biofilm dynamics, filtration, and water recovery. In this study, filtration and biofilm growth modeling principles have been coupled for the definition of an original mathematical model able to reproduce biofouling evolution in membrane systems. The model has been solved numerically to simulate biologically relevant conditions, and to investigate the hydraulic behavior of the membrane. It has been calibrated and validated using lab-scale data. Numerical results accurately predicted the pressure drop occurring in the microfiltration system. A calibrated model can give information for optimization protocols as well as fouling prevention strategies.
End-stage renal disease (ESRD) is usually accompanied by renal failure, central nervous system abnormalities and multiple organ dysfunction [1]. It may lead to cognitive dysfunction, including abnormal cognitive control, memory impairment and emotional impairment [2]. Cognitive impairment is a common comorbidity in ESRD cases, especially for patients receiving hemodialysis (HD). The impairment of cognitive function is more common in orientation, attention, and executive function [3]. About 30–60% of ESRD patients will develop mild cognitive impairment (MCI) when receiving HD treatment [4]. MCI patients have a high risk of developing dementia, which may significantly reduce the survival rate and prognosis. After cognitive training and rehabilitation treatment, the development of dementia in MCI patients may be delayed, and some may even return to a normal state [5]. However, the exact neuropathological mechanism of ESRD associated with MCI (ESRDaMCI) is still unclear, and hinders the development of effective treatment. Therefore, the diagnosis of neuroimaging changes in ESRD patients with MCI is critical for effective treatment and prognosis improvement in these patients.
In recent years, multimodal neuroimaging techniques, including structural magnetic resonance imaging (sMRI) [6], fluorodeoxyglucose positron emission tomography (FDG-PET) [7], arterial spin labeling (ASL) [8], and functional magnetic resonance imaging (fMRI) [9], diffusion tensor imaging (DTI) [10] and diffusion kurtosis imaging (DKI) [11], have developed rapidly. Researchers can understand the pathophysiology of brain diseases in different aspects, such as pathological marker deposition, structural connectivity (SC) and functional connectivity (FC). These provide a valuable tool for the studies of potential imaging biomarkers of ESRD associated with neurological complications. Previous studies on voxel-based morphometrics, surface-based morphometrics, and DTI, revealed gray matter volume defects and reduced cortical thickness in ESRD patients, as well as reduced white matter integrity [12]. The Alzheimer's Disease Imaging Initiative reported the possibility of DTI as a biomarker of cognitive impairment [13], whereas, DTI could not accurately reflect the changes of brain tissue microstructure because it could not quantify non-Gaussian diffusion. DKI overcomes these limitations and provides a new way to analyze the microstructure of gray matter and white matter. It has been applied to the studies of nervous systems, including normal brain tissue, cerebral infarction [14], brain trauma [15], glioma [16], and Alzheimer's disease [17], etc.
Great deals of attention have been paid to studying the mechanisms of some brain disorders, such as epilepsy [18], schizophrenia [19], Alzheimer's disease [20], and ESRD-related neurological complications [21]. Brain networks (BN) provide powerful representations on the interaction patterns between various brain regions. Nodes in BN represent brain regions divided by physiological templates, and edges represent interactions between regions extracted from noninvasive imaging techniques. Edges can be classified as FC and SC. FC estimates the consistency of brain activity between different brain regions, which is usually calculated from time series extracted from fMRI or electroencephalogram (EEG) signals. SC describes the physical connection of nerve fibers between brain regions, which can be directly obtained by calculating the number of fiber tracts (FN) in DKI. Many computational models, including sparse learn-based models [22] or graph-theoretic metrics [23], have been applied to search for potential biomarkers from FC or SC for diagnosing brain diseases. Traditional BNs simply takes into account the interactions between pairwise brain regions. This can result in the omission of serviceable information in higher-order relationships between brain regions (that is, broader nodal interactions between more than two brain regions) that are essential for understanding the pathological basis of brain diseases.
Some new methods have been developed fusing FC and SC for BN analysis. Yu et al. [24] described the complex interaction between multiple nodes as hypergraphs. Ji et al. [25] constructed a hypergraph manifold regularization (HMR) framework for brain functional networks. Diffusion processes have been used to analyze multimodal data [26], providing some general learning frameworks. FC provides information about brain activity, while SC directs the flow of information. If SC is directly used as the transition matrix, where the weights are predetermined and cannot solve the classification task. While SC may be helpful in determining interaction links between nodes, link weights between nodes are broadly correlated and complex. The joint feature representation obtained through diffusion is yet in the node level, which is different from the global level connections of BN [27].
In this study, a hypergraph representation method of multimodal BN is proposed. The node representation is optimized by fusing FC and SC to automatically learn interactions between nodes, considering direct and indirect connections. Specifically, firstly, the node feature matrix is constructed according to the time series extracted from fMRI, and the adjacency matrix is constructed according to the number of FN extracted from DKI. On this basis, an attention spread graph is defined to describe the node interaction based on the input node connection graph. It is called the node feature matrix, where the weight (strength) of the node connection can be automatically learned through training. The diffusion process guided by the attention diffusion map (ADM) integrates fMRI and DKI datasets to generate corresponding node representations. Then, the connection features for classification are generated by bilinear pooling and transformed into an optimization model. Finally, the hypergraph is constructed according to the generated node representation and connection features. HMR and L1 norm regularization terms are introduced into the optimization model to obtain the hypergraph representation of multimodal BN (HRMBN) for ESRDaMCI classification.
The major contributions and novelty are as follows. a) A hypergraph representation method is proposed for the first time to integrate fMRI and DKI to construct multimodal BN considering the complementary information of FC and SC of ESRDaMCI; b) It is the first time to construct hypergraphs based on node representations and connection features generated by fMRI and DKI, and the first time to introduce HMR and L1 norm regularization terms into the optimization model constructed by bilinear attention mechanism; c) More desirable results are obtained in the classification of ESRDaMCI, and the key brain regions are identified, which provided a reference for the clinical research and auxiliary diagnosis of ESRDaMCI.
Figure 1 shows the hypergraph representation framework: (a) Preprocessing fMRI data and DKI data obtained by scanning; (b) Extracting time series from fMRI, and tracing fiber tracts from DKI; (c) Constructing the node feature matrix for time series, and constructing the adjacency matrix for FN obtained from fiber bundle tracking; (d) Constructing an ADM based on node features and adjacency matrix to guide the diffusion process to generate node representation; (e) Using bilinear pooling to generate connection features, which is transformed into an optimization model; (f) Constructing hypergraph based on node representation and connection features, and obtain hypergraph Laplacian matrix; (g) Calculating HMR term by hypergraph Laplacian matrix, and introducing HMR and L1 norm regularization terms into the optimization model at the same time to acquire the HRMBN; (h) Taking the network edge weights in BN as features for feature selection to obtain discriminative features; (i) Dividing the selected features into a training set and a test set, and using the former to train the classifier, then classifying the later and evaluating its classification performance.
Fifty-one ESRD patients diagnosed with MCI in Changzhou Second People's Hospital Affiliated to Nanjing Medical University from February 2020 to June 2021 are included in ESRDaMCI group. Their average length of education is (11.25 ± 3.15) years (range, 5–19 years). All of them are right-handed and had no previous cardiovascular disease; No neurological disease; Free from infectious diseases; No diabetes; There are no contraindications of magnetic resonance examination. Meanwhile, thirty-nine healthy volunteers are recruited to the normal group. The average length of education is (9.73 ± 3.85) years (range, 5–19 years). All of them are right-handed; Previously in good health; There are no contraindications of magnetic resonance examination. There are 5 cases in ESRDaMCI group and 3 cases in normal group who are excluded due to excessive head movement. Finally, 51 patients and 39 volunteers are included. This study was approved and supervised by the Ethics Committee of Changzhou Second People's Hospital Affiliated to Nanjing Medical University, with the approval number KY039-01. All subjects voluntarily signed written informed consent.
Montreal Cognitive Assessment (MoCA) includes 11 items that measure multiple cognitive domains, such as memory, language, abstract thinking, executive functioning, visuospatial skills, attention and concentration, computation, and orientation [28]. According to the statistics from Changzhou Second People's Hospital Affiliated to Nanjing Medical University, MoCA has a better completion degree and a higher sensitivity to identify MCI in memory clinic than other cognitive function assessment scales, due to some factors such as patients' short temper and lack of cooperation. Therefore, MoCA is used to evaluate the cognitive function of ESRD patients and normal people. The full score of the MoCA scale is 30 points, with 26 or more as normal, 18–26 as mild, 10–17 as moderate, and less than 10 as severe. The average score of ESRD patients diagnosed with MCI is 21.30 ± 2.75. Table 1 shows the specific demographic information.
Item | ESRDaMCI (n = 51) | Normal (n = 39) | t/χ2 | P |
Age (ˉx±s) | 50.05 ± 7.86 | 48.37 ± 6.59 | 1.079 | 0.251 |
Gender (M/F) | 24/27 | 24/15 | 0.341 | 0.536 |
Education years (ˉx±s) | 11.25 ± 3.15 | 9.73 ± 3.85 | 0.973 | 0.771 |
MoCA score (ˉx±s) | 21.30 ± 2.75 | 27.27 ± 1.24 | -13.728 | 0.000 |
Note: ESRDaMCI: ESRD patients diagnosed with MCI; Normal: Normal people; P < 0.05 is a statistically significant difference. |
In GE Discovery MR 750W 3.0T scanner, A rubber cork was used to fix the head to avoid artifacts caused by head movement during the test. All subjects first underwent conventional MR examination, including T2-weighted (T2WI) and fluid attenuation inversion recovery (FLAIR) sequence T2WI. Two diagnostic imaging physicians ruled out organic brain damage. T1WI high-resolution structural images of the whole brain were acquired by 3D brain volume imaging (3D BRAVO) sequence with repetition time (TR) = 8.2 ms, echo time (TE) = 3.2 ms, layer thickness = 1.2 mm, layer interval = 0 mm, and flip angle = 12°. The matrix size = 256 × 256, the field of view (FOV) = 240 mm × 240 mm, the number of scanning layers = 152, and the scanning time = 3 min 57 s.
Single excitation plane echo imaging (SS-EPI) sequence was used for DKI scanning, divided into 30 diffused susceptibility directions, b value = 0, 1000, 2000 s/mm2, TR = 6500 ms, TE = 95.8 ms, FOV = 240.0 mm × 240.0 mm, matrix size = 128 × 128, layer thickness = 3.6 mm, the layer spacing = 0, the excitation times = 2, the number of scanning layers = 30, and the scanning time = 14 min 43 s. Gradient-echo plane echo imaging (GRE-EPI) sequence was used for fMRI scanning. The machine scanning parameters are as follows: Repetition time (TR) = 2000 ms, echo time (TE) = 40 ms, field of view (FOV) = 24 cm, flip angle (FA) = 90°, matrix size = 64 × 64, the layer thickness = 6 mm. Complete the MoCA evaluation before scanning.
The DKI data is preprocessed in FSL_5.0 and PANDA of Diffusion Toolkit [29]. Complete the following steps: 1) Format conversion: Transform the original data of DICOM format for 4 DNIFTI format using MRICRON "dcm2nii" in software toolkit; 2) eddy current correction: Remove data with large head movement to ensure the consistency of data; 3) Gradient correction and smooth noise reduction: Correct for subtle differences between brain structures and reduce spatial noise; 4) Acquisition of brain mask image: Extractb0 image, and then obtain the corresponding mask through the b0 image; 5) Calculating tensor, Fractional Anisotropy index (FA), Mean Diffusivity (MD) equivalent; 6) Resampling: Register the image to the standard space of Montreal Neurological Institute (MNI); 7) Gaussian smoothing and transforming the Automated Labeling (AAL) template in MNI space into the node in the brain structural network in individual space; 8) White matter fiber tract tracking: Use deterministic fiber tract tracking algorithm to calculate FN and Fractional Anisotropy (FA) and other indicators between brain regions.
The fMRI data is preprocessed in SPM8 and DPARSF of Matlab 2012b platform [30]. The specific steps are as follows: 1) Format conversion: Use DICOM Import of SPM8 to convert DICOM format into NIFTI format; 2) Slice Timing: Delete the first 10 time points and use the remaining 230 time series for subsequent processing; 3) Realign and normalize: Use the rigid registration for head correction, and normalize the fMRI images to MNI space and then standardize (Bounding Box: [-90, -126, -72; 90, 90,108], Voxel Size: [3 3 3]). 4) Smooth: Use full-width-at-half-maximum to smooth the Gaussian kernel; 5) Detrend; 6) Band pass filtering: Set frequency range from 0.01 to 0.08 Hz; 7) Region partition: Divide a brain into 90 brain regions using AAL standard partition template, and extract the time series for the convenience of subsequent research.
Given a BN G (V, E), V = {v1, v2, …, vn} represents a group of nodes (brain regions), E = {eij} (I, j = 1, 2, …, n) represents a set of edges (connections between nodes). Suppose F = {F1, F2, …, Fn}, Fi ∈ Rn is the feature set of nodes, and S ∈ Rn × n is the corresponding adjacency matrix. F represents a node feature matrix consisting of Pearson's correlation coefficients between fMRI time series. S represents a FN adjacency matrix, and FN can represent the strength of SC in BN. When the FN between two nodes is more than 3, there is a connection [31]. The adjacency matrix of FN is normalized by Minmax to improve the speed and accuracy of iterative solution and eliminate the dimensional difference of variables.
The diffusion process is randomly transferred on a graph, and the transition matrix can be expressed as:
P={pij}(i,j=1,2,…,n) | (1) |
where pij represents the predetermined transition probability of moving from one node to another in a one-step diffusion.
In the scenario of BN analysis, the diffusion process can be affected by the node characteristics defined by FC. Adding attention mechanism can solve this concern [32]. The attention diffusion process (ADP) can generate node representations by considering both node features and SC, thus naturally fusing SC and FC.
The node interaction is computationally represented by using the node feature matrix F and the adjacency matrix S. Specifically, the connections of nodes are determined by SC, and the weight of node interactions may be influenced by node features. This is due to the realization that the SC of the brain is fixed, whereas the FC of the brain is not fixed and depends on different nodal interactions between different brain systems. In this way, the weight of interaction strength between node i and node j is denoted as:
cij=f(Wc⋅(WαFi||WαFj)+bc) | (2) |
where || means to concatenate two vectors, Wc ∈ R1 × 2d is a trainable weights matrix; Wα ∈ Rd × n is a learnable linear projection matrix to improve the expression ability of the original features. bc is the bias term, a constant, and it learns with the whole model; f is an activation function.
Since C is not symmetric, the influence is directional and can reflect the influence degree of node i on node j. The weight matrix C is normalized to construct the attention spread map D for consistency comparison between different nodes. D is formulated as:
Dij=softmax(cij)=exp(cij)∑k∈Niexp(cik) | (3) |
where Ni represents the set of adjacent elements of element i of the adjacency matrix S.
Only Ni is considered for normalization to reflect SC between brain regions. If multistep diffusion is performed, it is no longer reasonable to use the power series of the attention spread graph D. This is because the attention mechanism changes with diffusion, reflecting the dynamic characteristics of BN. Therefore, an independent attention mechanism is applied to calculate the weight of the diffusion process and normalize it:
Dtij=exp(f(Wtc⋅(WtαFi||WtαFj)+btc))∑k∈Ntiexp(f(Wtc⋅(WtαFi||WtαFk)+btc)) | (4) |
where Nit represents the set of adjacent elements of the element i of the adjacency matrix S in the t-step diffusion, namely St=S...S⏟t.
Nodes of a multimodal BN can be represented as Zt = {Z1t, Z2t, …, Znt}, and after the t-step ADP Zit ∈ Rd can be formulated as:
Zti=∑k∈NiDtikWtαFi | (5) |
where Dt ∈ Rn × n is the ADM in the t-step diffusion, representing node interactions in t-step diffusion. The advantage of multistep diffusion is that it can capture potential connections between nodes, which is sensitive to the identification of brain diseases. Ni represents the set of neighboring nodes of node i according to the adjacency matrix S. Wαt ∈ Rd × n learns along with the entire model. Since the training process is guided by the label (patient is 1, normal is -1), the Wα learning matrix in Eq (5) can improve the recognition ability of the original features.
Node representation is enhanced by fusing the functionality and structure, as well as leveraging other node information. Nevertheless, these are still node-level features that reflect only local information. Thus, we need to generate connection features from the enhanced node representations. Bilinear pooling is used to extract the node representation information in the entire network to generate connection features:
Btij=ZtTi⋅Ztj | (6) |
where Bt ∈ Rn × n is bilinear feature. There are at least two benefits to using these features. First, these features can be viewed as connecting features that generate an overall representation of BN. Second, these features can improve classification sensitivity [33].
After centralization and standardization of Btij, the BN in the t-step diffusion is expressed as Bt=ZtT⋅Zt, which is transformed into the optimized form as follows:
minBt‖Bt−ZtT⋅Zt‖2F | (7) |
For the constructed BN, a hypergraph G (V, E, W) can describe the attribute relations between the BN. The set of nodes is denoted as V, the set of hyperedges is denoted as E, and the set of weights of each hyperedge is denoted as W. In hypergraph G, the node of each BN corresponds to node v∈V in the hypergraph, and each hyperedge e∈E contains more than two nodes to represent the simultaneous interaction of multiple BN nodes.
Let H∈R|V|×|E| be the point-edge incidence matrix of hypergraph G, the values of matrix elements are defined as follows.
h(v,e)={1,v∈e0,v∉e | (8) |
where v∈V is a node of hypergraph G, and e∈E is a hyperedge of hypergraph G.
In the incidence matrix H, the node degree d(vi) of each node and the edge degree δ(ei) of each hyperedge are respectively expressed as:
d(vi)=∑ei∈Ew(ei)h(vi,ei),i=1,...,m | (9) |
δ(ei)=∑vi∈Vh(vi,ei),i=1,...,m | (10) |
where ei is the i-th hyperedge, w(ei) is the weight of the hyperedge, vi is the i-th node, m denotes the number of hyperedges.
Laplacian matrix is often used in the matrix representation of graphs because it can reflect the intrinsic geometric structure of graphs. The Laplacian matrix of hypergraph can better reflect the higher-order relationship between nodes. It is expressed as
L=Dv−Θ | (11) |
where Θ=HWD−1eHT is the adjacency matrix of the hypergraph, Dv is the node degree matrix with diagonal elements d(vi), and De is the hyperedge degree matrix with diagonal elements δ(ei).
The standardized Laplacian matrix of a hypergraph is deduced and calculated according to the calculation method of the Laplacian matrix of a simple graph, as shown in Eq (12):
Lh=I−Dv−12HWDe−1HTDv−12 | (12) |
where Lh is the normalized Laplacian matrix of the hypergraph and I is the identity matrix. Dv is the hypergraph node degree matrix, De is the hypergraph hyperedge degree matrix, W is the diagonal matrix with hyperedge weights, and H∈R|V|×|E| is the point-edge incidence matrix of the hypergraph G.
Referring to the research of Shao et al. [34], the K-Nearest Neighbor (KNN) algorithm is adopted to construct the hypergraph according to the connection features generated by Eq (7), and the node degree and edge degree of the hypergraph are calculated, so as to obtain the regularization term of the hypergraph manifold, and the L1 norm regularization term is added. Inspired by Huang et al. [32], the ADP is combined with bilinear pooling to take FC as the node. Finally, the multimodal BN is constructed with SC as edges to obtain a HRMBN. The objective function of this method is as follows:
minBt‖Bt−ZtT⋅Zt‖2F+λ‖Bt‖1+βtr(BtTLhBt) | (13) |
where Bt is the hypergraph representation of multimodal in the diffusion at t-step, λ and β represent the regularization parameters of the L1 norm regularization term and the hypergraph manifold regularization term, respectively, and Lh is the normalized Laplacian matrix of the hypergraph.
After constructing multimodal BN, we determine the features to be used for classification. Two methods are commonly used to identify cognitive impairment. One is to extract features based on some graph metrics, such as local clustering coefficients. The other is to directly take network edge weights as features [35]. This study adopts the second method, which can reduce the impact of the difference of extracted features on the verification of BN itself. The simplest t-test method is used to select features to avoid the confounding effect of feature extraction.
Support vector machine (SVM) has good generalization performance [36]. It uses kernel function to map samples to high-dimensional space during classification, thus simplifying linearly separable problems in high-dimensional space. Linear kernel SVM, with the advantages of easy comparison of experimental results, has been extensively used in small-sample classification problems. Therefore, SVM is selected for this study with only 90 subjects. The discriminative features are selected by t-test, and then SVM is trained to identify patients by using the selected features. The optimization objective function of SVM classifier is as follows:
L(w,β,a)=n∑p=1ap−12n∑p,q=1ypyqapaqK⟨xp,xq⟩s.t.{K⟨xp,xq⟩=xTpxqw=l∑p=1apypxpl∑p=1apyp=00≤ap≤C,p=1,…,n | (14) |
where L is the Lagrange function, w is the normal vector of the fitted hyperplane, β is the displacement term determining the distance between the fitted hyperplane and the origin, and a is the fitting error, K ⟨xp, xq⟩ is a linear kernel, x is the value of BN features, y ∈ {-1, +1} represents labels, n is the number of training samples, p is the sample number in the training set, q is the sample number in the test set, and the parameter C = 1.
A linear transformation transfers the original node features to a low-dimensional space. Equation (2) is used to refine the interaction weights, and the modified linear unit (Relu) is selected as its activation function. These interaction weights are normalized according to Eq (3) to construct an ADM, which guides the diffusion process to generate a joint node representation. Bilinear pooling is used to extract the connected features to build hypergraph, t-test is used to select the features, and SVM is used for classification. In the classification, the label of ESRDaMCI group is set to 1 and the label of normal group is set to -1. Because of the limited number of samples, leave-one-out cross-validation is adopted to evaluate the performance of HRMBN. In short, one is tested and the others are tested in the training model. The effects of different parameters on the classification performance of ESRDaMCI are discussed to determine the optimal parameters of HRMBN. It is compared with seven of the most advanced multimodal BN construction methods.
The range of the number of nearest neighbors k is set as [1,20], and the range of the L1 norm regularization parameter λ and the HMR parameter β are set as [2-5, 25]. The significance level of t-test features is set to 0.05, and the linear kernel classifier parameter C is set to 1. Because the proposed model has multiple parameters, the grid search method cannot find the optimal parameters directly. The strategy of this study is to use leave-one-out cross-validation method to gradually calculate the optimal parameter values of the model, that is, first, the number of neighbors k is determined based on the hypergraph generated by the node representation and connection features, and then the regularization parameters λ and β are determined. The test results are averaged to measure the performance of the model. The model is repeatedly trained to determine the optimal hyperparameter, and the original samples are used to test the optimal hyperparameter model to avoid data leakage. Classification accuracy (ACC), area under ROC curve (AUC), sensitivity (SEN) and specificity (SPE) are used to evaluate the classification performance [37].
KNN algorithm is utilized to construct hypergraphs according to the generated node representation and connection features [38]. Specifically, we select k nearest vertices to generate hyperedges. As shown in Figure 2, k = 1 means that no hypergraph is formed. By comparing the values of various classification indexes between normal group and ESRDaMCI group under different k, it can be seen that when the value of k is 8, the best ACC is 86.6457%. When the value of k changes from small to large, the classification performance increases first and then decreases. The cause might be that for small values of k it describes features that are too dense. When the value of k is large, the hypergraph depicts the overall structural features of BN rather than local features, and many nodes on the hyperedge may belong to different categories, so it is difficult to well reflect the structural features of BN. If the hypergraph is not constructed (that is, when k value is 1) and the classification is performed directly, the effect is unsatisfactory, indicating that the hypergraph with moderate k value is helpful to improve the classification performance. This is basically consistent with the research conclusion of Shao et al. [34].
As the L1 norm regular term is not derivable, the proximal operator method [38] is used to optimize and solve it. Firstly, the gradient descent method is used to update Bt in Eq (13) m times, with a step of αm. Then the nearest neighbor operator of the L1 norm regularization term is computed to impose soft threshold operations on the elements in Bt. After the completion of each gradient descent calculation, the nearest neighbor operator method is used to calculate Bt's nearest neighbor operator, and it is put into the next iteration for updating. So repeatedly, when the objective function in Eq (13) converges, the optimal solution of Bt is obtained, that is, the HRMBN. The L1 norm regularization term plays a role in removing redundant features and making the fused BN sparser. HMR term retains the discriminative information of each subject, so as to induce more discriminative features. In general, the regularization parameters λ and β are important to regulate the complexity of building the fused BN. Figure 3 shows the classification accuracy values of normal group and ESRDaMCI group with different regularization parameters.
Since there is no parameter selection task involved in determining the regularization parameters, we calculated ACC based on the results of leave-one-out cross-validation for all subjects only. The final result is extremely sensitive to the regularization parameter, and the appropriate combination of the L1 norm regularization parameter λ and the HMR parameter β can help to improve the classification performance. In particular, when λ = 2-3 and β = 21, the best ACC (91.0891%) is achieved. As the values of λ and β change, especially when λ ≥ 2-1, ACC has an obvious downward trend. To sum up, the number of neighbors is set as 8, and the regularization parameter λ = 2-3 and β = 21 are applied into construct the multimodal BN.
It is compared with two single-modal network methods and seven of the most advanced multi-modal network methods to verify its effectiveness. In the unimodal method, FN matrix of DKI normalized by Minmax is used as the network and Pearson's correlation coefficient extracted from fMRI time series by Pearson's correlation is used as the network. Multi-model methods include multi-kernel [39], multi-linear principal component Analysis (MPCA) [40], kernelized Support Tensor Machine (KSTM) [41], Joint Structural Functional Connectivity (JFSC) [42], Multi-view Graph Convolutional Network (MVGCN) [43], Diffusion Convolutional Neural Network (DCNN) [44] and Attention Diffusion Bilinear Neural Network (ADBNN) [32]. According to the model inputs, the fusion methods of these multimodal fusion methods can be divided into two ways. Post-fusion (Multi-kernel, MPCA, KSTM) and pre-fusion (JFSC, MVGCN, DCNN, ADBNN). Post fusion is to fuses at the decision level. Pre-fusion to fuse the information of the two modes together by setting the model in the input stage. The method in this study belongs to pre-fusion. The above methods all use t-test method for feature selection and use linear kernel SVM classifier for classification. Their classification performance for ESRDaMCI is evaluated by leave-one-out cross-validation, as shown in Table 2. The optimal classification results are indicated in bold.
Methods | ACC (%) | SEN (%) | SPE (%) | AUC |
DKI (FN) | 69.8113 ± 4.2871 | 72.5490 ± 1.4290 | 53.8462 ± 2.5617 | 0.6953 ± 3.3493 |
fMRI (PC) | 72.4314 ± 2.8581 | 71.4435 ± 3.1146 | 66.7949 ± 1.4570 | 0.7504 ± 3.4192 |
Multi-kernel [39] | 73.5821 ± 5.7912 | 69.8234 ± 3.3247 | 75.4712 ± 2.6145 | 0.7231 ± 1.0767 |
MPCA [40] | 67.5541 ± 1.5424 | 66.6715 ± 2.8952 | 79.1628 ± 5.4774 | 0.7671 ± 0.2130 |
KSTM [41] | 70.0756 ± 3.1433 | 80.1643 ± 6.3478 | 61.5717 ± 3.3541 | 0.7982 ± 4.2041 |
JSFC [42] | 63.4320 ± 3.5114 | 73.9417 ± 3.2264 | 60.6984 ± 2.6427 | 0.6864 ± 3.6714 |
MVGCN [43] | 81.6488 ± 4.6481 | 86.8074 ± 1.4194 | 77.5132 ± 4.2438 | 0.8081 ± 4.0278 |
DCNN [44] | 79.4045 ± 2.2725 | 74.1611 ± 0.5384 | 80.9744 ± 3.9925 | 0.7937 ± 0.8421 |
ADBNN [32] | 86.7439 ± 1.4567 | 82.7921 ± 4.5711 | 84.7233 ± 3.7440 | 0.8874 ± 2.1534 |
HRMBN | 91.0891 ± 2.5417 | 86.6461 ± 2.8941 | 89.7436 ± 2.1513 | 0.9023 ± 1.1164 |
Obviously, our method outperforms other methods for ESRDaMCI except SEN, and achieves the best classification effect. The best ACC, SPE and AUC are 91.0891% ± 2.5417%, 89.7436% ± 2.1513% and 0.9023 ± 1.1164, respectively. Compared with other methods, the error range of the proposed method is smaller, indicating that HRMBN is more stable and the classification performance is better. Compared with the suboptimal ADBNN method, the average ACC, SEN, SPE and AUC are improved by 4.3452, 3.8540, 5.0203 and 0.0149, respectively. In addition, it is found that most of the classification tasks using multimodality-based methods performed better than the single modality methods using only fMRI or DKI. This validates that combining two types of brain connections can provide complementary information and thus improve classification performance. For example, the accuracy of multi-kernel using simple combination strategy is at least 1.1507% higher than that of fMRI only method. Although JSFC combines fMRI and DKI, the accuracy obtained by JSFC is lower than that obtained by fMRI-based methods. This result may be due to the fact that most fMRI information does not involve the SC reconstruction process.
In this study, the connection in BN is used as a characteristic to identify patients with ESRDaMCI. Multimodal BN hypergraph representation is constructed based on the best classification performance, and then features with significant differences are selected (p < 0.05) to find some biomarkers for ESRDaMCI diagnosis. We calculate the number of brain regions involved in these connections and count the brain regions that appeared more frequently in the cross validation, and finally obtain 103 of the most discriminative features in the ESRDaMCI classification task. It is worth noting that there are 73 features selected from the brain functional network based on Pearson's correlation. It is clear that our HRMBN method has more discriminative features, and the generated network representation is better than traditional methods. Figure 4 shows the visualized discriminative features.
The thickness of each arc is inversely proportional to the corresponding p value, representing the discriminative power of the corresponding feature (not its actual connectivity strength). Since the brain regions selected in each cross validation are not the same, we count the top 15 brain regions most frequently selected in ESRDaMCI group and normal group classification as discriminative brain regions. From the connection in Figure 4, It can be seen that these brain regions are left middle frontal gyrus (Frontal_Mid_L), left middle frontal gyrus (Frontal_Mid_Orb_L), left inferior frontal gyrus (Frontal_Inf_Orb_L), left central sulcus cover (Rolandic_Oper_L), and left supplementary motor area (Supp_Motor_Area_L), left medial superior frontal gyrus (Frontal_Sup_Medial_L), left gyrus rectus muscle (Rectus_L), right hippocampus (Hippocampus_R), left superior occipital gyrus (Occipital_Sup_L), left middle occipital gyrus (Occipital_Mid_L), left Occipital gyrus (Occipital_Inf_L), right occipital gyrus (Occipital_Inf_R), left angular gyrus (Angular_L), left middle temporal gyrus (Temporal_Mid_L), right temporal pole: middle temporal gyrus (Temporal_Pole_Mid_R). Map to the ICBMl52 template space using BrainNet Viewer toolbox, as shown in Figure 5.
Most of the selected brain regions, such as left middle temporal gyrus (Temporal_Mid_L), right hippocampus (Hippocampus_R), and right temporal pole: middle temporal gyrus (Temporal_Pole_Mid_R), are consistent with those brain regions with significant differences in topological attributes between the ESRD patients and the normal people in the previous study of Wu et al. [45]. The discriminative brain regions selected are mainly in the left side of the brain, which is thought to be involved in logical task processing, language and analytical thinking. For example, the left middle frontal gyrus (Frontal_Mid_L) in the frontal lobe, the left middle frontal gyrus (Frontal_Mid_Orb_L), the left inferior frontal gyrus (Frontal_Inf_Orb_L), the left medial superior frontal gyrus (Frontal_Sup_Medial_L), etc. [46], Mainly related to creative and advanced spiritual activities such as thinking and consciousness; The right hippocampus (Hippocampus_R) [47] plays a significant role in helping human short-term memory, long-term memory and spatial orientation; The left supplementary motor area (Supp_Motor_Area_L) [48] is mainly related to the voluntary motor control of trunk muscles. The left angular gyrus (Angular_L) is the visual language center (reading center). After the injury, people who have been able to read become unable to read (unable to understand the meaning of the copied words), although their vision are not affected. Occipital lobe includes left superior occipital gyrus (Occipital_Sup_L), left middle occipital gyrus (Occipital_Mid_L), left inferior occipital gyrus (Occipital_Inf_L) and right inferior occipital gyrus (Occipital_Inf_R) [49], which are responsible for vision, image recognition and image perception. left middle temporal gyrus (Temporal_Mid_L) and right temporal pole: middle temporal gyrus (Temporal_Pole_Mid_R) [50] are closely related to short-term memory, balance and emotion, etc. If these brain regions are damaged, personality changes will be caused. The multimodal BN hypergraph representation method can also identify the left rectus gyrus muscle (Rectus_L) region located on the orbital surface of the frontal lobe. Wee et al. [51] showed that morphological changes in the rectus muscle would affect the progression of MCI. These brain regions are selected, indicating that ESRDaMCI group had changes in memory, language, spatial visual processing and other aspects compared with normal group.
Some of the selected discriminative brain regions, including the right hippocampus (Hippocampus_R) and the left angular gyrus (Angular_L), belonging to the default mode network (DMN), are related to memory and visual language, respectively. DMN plays a key role in cognitive function and neuro-regulation. These results indicate that our study may provide the most discriminative features and brain regions that cause ESRDaMCI, and provide a reference for clinical diagnosis.
In recent years, more and more attention has been paid to the study of some diseases associated with MCI. The borderline of such concomitant diseases remains a challenge. As the development of new neuroimaging techniques and the introduction of new research methods, these challenges may turn into opportunities for further insight into human brains. To date, there are relatively few focuses on ESRDaMCI classification. Most studies of BN depend on the properties of the unimodal data itself and only consider the pairwise relationship between brain regions, without taking into account the complementary information of FC and SC. In this study, a hypergraph representation method of multimodal BN is proposed to integrate FC and SC to construct BN, and it is applied to the classification of ESRDaMCI. Compared with the general network, this method naturally links the structural network and the functional network, and provides more feature information.
Current studies have shown that compared with only using a single mode, better classification accuracy can be obtained by fusing information from multiple modes [52]. The biggest difference between multimodal BN analysis and traditional multimodal data analysis lies in the natural connection between structural network and functional network. Structure can often affect function, so the correlation information between two modes can be further explored. Previous studies have modeled multimodal fusion. Mišić et al. [53] analyzed the connection between the two by partial least squares method, and found a number of closely related sub-networks and important nodes. Goñi et al. [54] directly used the connecting edge strength of SC to predict FC and found that there was a high degree of consistency in some brain regions. Nevertheless, these methods do not consider how to integrate two modes of information, but directly use the single mode data itself to directly fuse. Therefore, how to describe the correlation information between brain regions is very important. The method in this study automatically learns node interaction from FC and SC instead of using SC directly. The hypergraph constructed according to the generated node representation and connection features, whose multiple vertices are on the same hyperedge, can well reflect this potential correlation information.
It is worth mentioning that we generate a joint node representation of FC and SC by using diffusion processes, rather than simply treating them as two patterns to be spliced together as in the existing methods. FC and SC are also integrated in ADM which guides the diffusion process. In the map, the connections of nodes are determined by SC, and the connection weights between nodes are learned based on FC. The direct and indirect connections (that is, the diffusion step and two step diffusion) do not share each step of the parameters. For that reason, multi-step diffusion helps to increase the risk of excessive fitting. The HMR and the regularization term of L1 norm are introduced to increase the prior information appropriately, so as to reduce the complexity of the model, enhance the robustness and prevent overfitting. Moreover, unlike the traditional approach of representing node interactions using non-directed joins, our approach learns directed node interactions. As seen from ADM constructed by Eqs (2) and (3), the directed node interactions contain weight information and direction information. The directional information of this diffusion map is exactly similar to the effective connections describing effective interactions between brain regions. Experimental results in Table 2 verify that the proposed method performs better than the traditional combination method.
However, there are some improvements in the proposed method. The multi-head attention mechanism will be explored and integrated into our model. It is likely to help improve classification performance. Node representations are generated in the model using only the spatial attention mechanism. Given that fMRI data can represent time by measuring fluctuations, temporal information should also be taken into account during representation learning. For the next stage, we plan to explore how to joint temporal and spatial factors to characterize the temporal and spatial characteristics of BN [55]. Furthermore, the dataset in this study has a limited number of samples, so it is necessary to add more samples and then train diffusion through two steps and above to increase the range of interactions. The brain regions mentioned above are defined using the AAL template, and only 90 brain regions are selected to construct the BN. Several other available templates should be considered for dividing the brain into finer brain regions.
In this study, a hypergraph representation method of multimodal BN is proposed to construct BN (i.e., HRMBN) to identify patients with ESRDaMCI. This method effectively integrates FC and SC DKI and fMRI data into a learning framework, where the characteristics of network nodes are refined based on automatic learning node graph. The second order statistics of the features of these nodes can be further extracted by bilinear pooling for classification, so as to better understand the potential correlation between brain function and structure, and provide a new method for brain analysis. The entire network is trained in an end-to-end manner and shows better performance on real ESRDaMCI datasets, with ACC, SEN, SPE and AUC reaching 91.0891, 86.6461, 89.7436% and 0.9023, respectively. In addition, the discriminative brain regions are identified according to the selected features to better reflect the pathogenesis of ESRDaMCI. The HRMBN method is effective in identifying ESRDaMCI with functional or structural abnormalities.
This work was supported by National Natural Science Foundation of China (grant No. 51877013), and Jiangsu Provincial Key Research and Development Program (grant No. BE2021636). This work was also sponsored by Qing Lan Project of Jiangsu Province.
The authors declare there is no conflict of interest.
[1] |
R. V. Linares, A. Wexler, S. S. Bucs, C. Dreszer, A. Zwijnenburg, H. C. Flemming, et al., Compaction and relaxation of biofilms, Desalin. Water Treat., 57 (2016), 12902–12914. https://doi.org/10.1080/19443994.2015.1057036 doi: 10.1080/19443994.2015.1057036
![]() |
[2] |
K. Martin, D. Bolster, N. Derlon, E. Morgenroth, R. Nerenberg, Effect of fouling layer spatial distribution on permeate flux: a theoretical and experimental study, J. Membr. Sci., 471 (2014), 130–137. https://doi.org/10.1016/j.memsci.2014.07.045 doi: 10.1016/j.memsci.2014.07.045
![]() |
[3] |
A. Venezuela, J. Pérez-Guerrero, S. Fontes, Hybrid modeling of convective laminar flow in a permeable tube associated with the cross-flow process, Commun. Nonlinear Sci. Numer. Simul., 14 (2009), 795–810. https://doi.org/10.1016/j.cnsns.2007.11.009 doi: 10.1016/j.cnsns.2007.11.009
![]() |
[4] |
S. F. Anis, R. Hashaikeh, N. Hilal, Microfiltration membrane processes: a review of research trends over the past decade, J. Water Process Eng., 32 (2019), 100941. https://doi.org/10.1016/j.jwpe.2019.100941 doi: 10.1016/j.jwpe.2019.100941
![]() |
[5] |
I. Ivanovic, T. Leiknes, The biofilm membrane bioreactor (bf-mbr)—a review, Desalin. Water Treat., 37 (2012), 288–295. https://doi.org/10.1080/19443994.2012.661283 doi: 10.1080/19443994.2012.661283
![]() |
[6] |
B. D'Acunto, L. Frunzo, V. Luongo, M. R. Mattei, Invasion moving boundary problem for a biofilm reactor model, Eur. J. Appl. Math., 29 (2018), 1079–1109. https://doi.org/10.1017/S0956792518000165 doi: 10.1017/S0956792518000165
![]() |
[7] |
B. D'Acunto, L. Frunzo, V. Luongo, M. R. Mattei, Modeling heavy metal sorption and interaction in a multispecies biofilm, Mathematics, 7 (2019), 781. https://doi.org/10.3390/math7090781 doi: 10.3390/math7090781
![]() |
[8] |
A. Trucchia, M. Mattei, V. Luongo, L. Frunzo, M. Rochoux, Surrogate-based uncertainty and sensitivity analysis for bacterial invasion in multi-species biofilm modeling, Commun. Nonlinear Sci. Numer. Simul., 73 (2019), 403–424. https://doi.org/10.1016/j.cnsns.2019.02.024 doi: 10.1016/j.cnsns.2019.02.024
![]() |
[9] |
C. Dreszer, J. S. Vrouwenvelder, A. H. Paulitsch-Fuchs, A. Zwijnenburg, J. C. Kruithof, H. C. Flemming, Hydraulic resistance of biofilms, J. Membr. Sci., 429 (2013), 436–447. https://doi.org/10.1016/j.memsci.2012.11.030 doi: 10.1016/j.memsci.2012.11.030
![]() |
[10] |
S. Kang, W. Lee, S. Chae, H. Shin, Positive roles of biofilm during the operation of membrane bioreactor for water reuse, Desalination, 202 (2007), 129–134. https://doi.org/10.1016/j.desal.2005.12.048 doi: 10.1016/j.desal.2005.12.048
![]() |
[11] |
S. Kerdi, A. Qamar, A. Alpatova, N. Ghaffour, An in-situ technique for the direct structural characterization of biofouling in membrane filtration, J. Membr. Sci., 583 (2019), 81–92. https://doi.org/10.1016/j.memsci.2019.04.051 doi: 10.1016/j.memsci.2019.04.051
![]() |
[12] |
W. Bowen, J. Calvo, A. Hernandez, Steps of membrane blocking in flux decline during protein microfiltration, J. Membr. Sci., 101 (1995), 153–165. https://doi.org/https://doi.org/10.1016/0376-7388(94)00295-A doi: 10.1016/0376-7388(94)00295-A
![]() |
[13] |
S. Chellam, W. Xu, Blocking laws analysis of dead-end constant flux microfiltration of compressible cakes, J. Colloid Interface Sci., 301 (2006), 248–257. https://doi.org/10.1016/j.jcis.2006.04.064 doi: 10.1016/j.jcis.2006.04.064
![]() |
[14] |
N. Cogan, S. Chellam, Incorporating pore blocking, cake filtration, and eps production in a model for constant pressure bacterial fouling during dead-end microfiltration, J. Membr. Sci., 345 (2009), 81–89. https://doi.org/10.1016/j.memsci.2009.08.027 doi: 10.1016/j.memsci.2009.08.027
![]() |
[15] |
M. Imran, H. L. Smith, A model of optimal dosing of antibiotic treatment in biofilm, Math. Biosci. Eng., 11 (2014), 547. https://doi.org/10.3934/mbe.2014.11.547 doi: 10.3934/mbe.2014.11.547
![]() |
[16] |
C. Laspidou, L. Spyrou, N. Aravas, B. Rittmann, Material modeling of biofilm mechanical properties, Math. Biosci., 251 (2014), 11–15. https://doi.org/10.1016/j.mbs.2014.02.007 doi: 10.1016/j.mbs.2014.02.007
![]() |
[17] |
M. Mattei, L. Frunzo, B. D'Acunto, Y. Pechaud, F. Pirozzi, G. Esposito, Continuum and discrete approach in modeling biofilm development and structure: a review, J. Math. Biol., 76 (2018), 945–1003. https://doi.org/10.1007/s00285-017-1165-y doi: 10.1007/s00285-017-1165-y
![]() |
[18] |
A. Tenore, M. Mattei, L. Frunzo, Modelling the ecology of phototrophic-heterotrophic biofilms, Commun. Nonlinear Sci. Numer. Simul., 94 (2021), 105577. https://doi.org/10.1016/j.cnsns.2020.105577 doi: 10.1016/j.cnsns.2020.105577
![]() |
[19] |
A. Tenore, F. Russo, M. Mattei, B. D'Acunto, G. Collins, L. Frunzo, Multiscale modelling of de novo anaerobic granulation, Bull. Math. Biol., 83 (2021), 1–50. https://doi.org/10.1007/s11538-021-00951-y doi: 10.1007/s11538-021-00951-y
![]() |
[20] |
M. Jafari, N. Derlon, P. Desmond, M. C. van Loosdrecht, E. Morgenroth, C. Picioreanu, Biofilm compressibility in ultrafiltration: a relation between biofilm morphology, mechanics and hydraulic resistance, Water Res., 157 (2019), 335–345. https://doi.org/10.1016/j.watres.2019.03.073 doi: 10.1016/j.watres.2019.03.073
![]() |
[21] | H. Vrouwenvelder, C. Dreszer, R. V. Linares, J. Kruithof, C. Mayer, H. Flemming, Why and how biofilms cause biofouling–the "hair-in-sink"-effect, in The Perfect Slime: Microbial Extracellular Polymeric Substances (EPS), (2016), 193–206. |
[22] |
G. Tierra, J. P. Pavissich, R. Nerenberg, Z. Xu, M. S. Alber, Multicomponent model of deformation and detachment of a biofilm under fluid flow, J. R. Soc. Interface, 12 (2015), 20150045. https://doi.org/10.1098/rsif.2015.0045 doi: 10.1098/rsif.2015.0045
![]() |
[23] |
M. Li, K. Matouš, R. Nerenberg, Predicting biofilm deformation with a viscoelastic phase-field model: modeling and experimental studies, Biotechnol. Bioeng., 117 (2020), 3486–3498. https://doi.org/10.1002/bit.27491 doi: 10.1002/bit.27491
![]() |
[24] |
M. Rahimi, S. Madaeni, M. Abolhasani, A. A. Alsairafi, Cfd and experimental studies of fouling of a microfiltration membrane, Chem. Eng. Process. Process Intensif., 48 (2009), 1405–1413. https://doi.org/10.1016/j.cep.2009.07.008 doi: 10.1016/j.cep.2009.07.008
![]() |
[25] |
A. Tenore, J. Vieira, L. Frunzo, V. Luongo, M. Fabbricino, Calibration and validation of an activated sludge model for membrane bioreactor wastewater treatment plants, Environ. Technol., 41 (2020), 1923–1936. https://doi.org/10.1080/09593330.2018.1551940 doi: 10.1080/09593330.2018.1551940
![]() |
[26] |
M. Zare, F. Z. Ashtiani, A. Fouladitajar, Cfd modeling and simulation of concentration polarization in microfiltration of oil–water emulsions; application of an eulerian multiphase model, Desalination, 324 (2013), 37–47. https://doi.org/10.1016/j.desal.2013.05.022 doi: 10.1016/j.desal.2013.05.022
![]() |
[27] |
C. Picioreanu, J. Vrouwenvelder, M. Van Loosdrecht, Three-dimensional modeling of biofouling and fluid dynamics in feed spacer channels of membrane devices, J. Membr. Sci., 345 (2009), 340–354. https://doi.org/10.1016/j.memsci.2009.09.024 doi: 10.1016/j.memsci.2009.09.024
![]() |
[28] |
J. Shin, K. Kim, J. Kim, S. Lee, Development of a numerical model for cake layer formation on a membrane, Desalination, 309 (2013), 213–221. https://doi.org/10.1016/j.desal.2012.10.018 doi: 10.1016/j.desal.2012.10.018
![]() |
[29] |
S. Chellam, N. Cogan, Colloidal and bacterial fouling during constant flux microfiltration: comparison of classical blocking laws with a unified model combining pore blocking and eps secretion, J. Membr. Sci., 382 (2011), 148–157. https://doi.org/10.1016/j.memsci.2011.08.001 doi: 10.1016/j.memsci.2011.08.001
![]() |
[30] |
N. Cogan, J. Li, A. R. Badireddy, S. Chellam, Optimal backwashing in dead-end bacterial microfiltration with irreversible attachment mediated by extracellular polymeric substances production, J. Membr. Sci., 520 (2016), 337–344. https://doi.org/10.1016/j.memsci.2016.08.001 doi: 10.1016/j.memsci.2016.08.001
![]() |
[31] |
B. D'Acunto, L. Frunzo, V. Luongo, M. Mattei, A. Tenore, Free boundary problem for the role of planktonic cells in biofilm formation and development, Z. Angew. Math. Phys., 72 (2021), 1–17. https://doi.org/10.1007/s00033-021-01561-3 doi: 10.1007/s00033-021-01561-3
![]() |
[32] |
Y. Rohanizadegan, S. Sonner, H. J. Eberl, Discrete attachment to a cellulolytic biofilm modeled by an itô stochastic differential equation, Math. Biosci. Eng., 17 (2020), 2236–2271. https://doi.org/10.3934/mbe.2020119 doi: 10.3934/mbe.2020119
![]() |
[33] |
F. Russo, A. Tenore, M. R. Mattei, L. Frunzo, Multiscale modelling of the start-up process of anammox-based granular reactors, Math. Biosci. Eng., 19 (2022), 10374–10406. https://doi.org/10.3934/mbe.2022486 doi: 10.3934/mbe.2022486
![]() |
[34] |
O. Wanner, W. Gujer, A multispecies biofilm model, Biotechnol. Bioeng., 28 (1986), 314–328. https://doi.org/10.1002/bit.260280304 doi: 10.1002/bit.260280304
![]() |
[35] |
B. D'Acunto, L. Frunzo, M. Mattei, On a free boundary problem for biosorption in biofilms, Nonlinear Anal. Real World Appl., 39 (2018), 120–141. https://doi.org/10.1016/j.nonrwa.2017.06.010 doi: 10.1016/j.nonrwa.2017.06.010
![]() |
[36] |
B. D'Acunto, L. Frunzo, I. Klapper, M. Mattei, Modeling multispecies biofilms including new bacterial species invasion, Math. Biosci., 259 (2015), 20–26. https://doi.org/10.1016/j.mbs.2014.10.009 doi: 10.1016/j.mbs.2014.10.009
![]() |
[37] |
J. M. Hughes, H. J. Eberl, S. Sonner, A mathematical model of discrete attachment to a cellulolytic biofilm using random des, Math. Biosci. Eng., 19 (2022), 6582–6619, https://doi.org/10.3934/mbe.2022310 doi: 10.3934/mbe.2022310
![]() |
[38] |
C. S. Laspidou, B. E. Rittmann, Non-steady state modeling of extracellular polymeric substances, soluble microbial products, and active and inert biomass, Water Res., 36 (2002), 1983–1992. https://doi.org/10.1016/S0043-1354(01)00414-6 doi: 10.1016/S0043-1354(01)00414-6
![]() |
[39] |
F. Abbas, R. Sudarsan, H. J. Eberl, Longtime behavior of one-dimensional biofilm models with shear dependent detachment rates, Math. Biosci. Eng., 9 (2012), 215–239. https://doi.org/10.3934/mbe.2012.9.215 doi: 10.3934/mbe.2012.9.215
![]() |
[40] |
E. Morgenroth, P. A. Wilderer, Controlled biomass removal—the key parameter to achieve enhanced biological phosphorus removal in biofilm systems, Water Sci. Technol., 39 (1999), 33–40. https://doi.org/10.1016/S0273-1223(99)00147-X doi: 10.1016/S0273-1223(99)00147-X
![]() |
[41] |
D. Dubber, N. F. Gray, Replacement of chemical oxygen demand (cod) with total organic carbon (toc) for monitoring wastewater treatment performance to minimize disposal of toxic analytical waste, J. Environ. Sci. Health A, 45 (2010), 1595–1600. https://doi.org/10.1080/10934529.2010.506116 doi: 10.1080/10934529.2010.506116
![]() |
[42] |
I. Douterelo, J. B. Boxall, P. Deines, R. Sekar, K. E. Fish, C. A. Biggs, Methodological approaches for studying the microbial ecology of drinking water distribution systems, Water Res., 65 (2014), 134–156. https://doi.org/10.1016/j.watres.2014.07.008 doi: 10.1016/j.watres.2014.07.008
![]() |
[43] |
M. E. Sieracki, T. L. Cucci, J. Nicinski, Flow cytometric analysis of 5-cyano-2, 3-ditolyl tetrazolium chloride activity of marine bacterioplankton in dilution cultures, Appl. Environ. Microbiol., 65 (1999), 2409–2417. https://doi.org/10.1128/AEM.65.6.2409-2417.1999 doi: 10.1128/AEM.65.6.2409-2417.1999
![]() |
[44] |
B. Merkey, B. Rittmann, D. Chopp, Modeling how soluble microbial products (SMP) support heterotrophic bacteria in autotroph-based biofilms, J. Theor. Biol., 259 (2009), 670–683. https://doi.org/10.1016/j.jtbi.2009.05.010 doi: 10.1016/j.jtbi.2009.05.010
![]() |
[45] |
W. Guo, H. H. Ngo, J. Li, A mini-review on membrane fouling, Bioresour. Technol., 122 (2012), 27–34. https://doi.org/10.1016/j.biortech.2012.04.089 doi: 10.1016/j.biortech.2012.04.089
![]() |
[46] |
J. Mansouri, S. Harrisson, V. Chen, Strategies for controlling biofouling in membrane filtration systems: challenges and opportunities, J. Mater. Chem., 20 (2010), 4567–4586. https://doi.org/10.1039/B926440J doi: 10.1039/B926440J
![]() |
[47] |
N. Cogan, S. Chellam, A method for determining the optimal back-washing frequency and duration for dead-end microfiltration, J. Membr. Sci., 469 (2014), 410–417. https://doi.org/10.1016/j.memsci.2014.06.052 doi: 10.1016/j.memsci.2014.06.052
![]() |
1. | Chaofan Song, Tongqiang Liu, Haifeng Shi, Zhuqing Jiao, HCTMFS: A multi-modal feature selection framework with higher-order correlated topological manifold for ESRDaMCI, 2024, 243, 01692607, 107905, 10.1016/j.cmpb.2023.107905 | |
2. | Xidong Fu, Shengchang Shan, Chun Liu, Yu Lu, Zhuqing Jiao, Constructing brain functional networks with adaptive manifold regularization for early mild cognitive impairment, 2024, 34, 0899-9457, 10.1002/ima.23053 | |
3. | Jie Chen, Tongqiang Liu, Haifeng Shi, Cota Navin Gupta, End-stage renal disease accompanied by mild cognitive impairment: A study and analysis of trimodal brain network fusion, 2024, 19, 1932-6203, e0305079, 10.1371/journal.pone.0305079 | |
4. | Chaofan Song, Tongqiang Liu, Huan Wang, Haifeng Shi, Zhuqing Jiao, Multi-modal feature selection with self-expression topological manifold for end-stage renal disease associated with mild cognitive impairment, 2023, 20, 1551-0018, 14827, 10.3934/mbe.2023664 | |
5. | Daniel M. Ripley, Terence Garner, Adam Stevens, Developing the ‘omic toolkit of comparative physiologists, 2024, 52, 1744117X, 101287, 10.1016/j.cbd.2024.101287 | |
6. | Rupu Zhang, Xidong Fu, Chaofan Song, Haifeng Shi, Zhuqing Jiao, Characterizing Topological Properties of Brain Functional Networks Using Multi-Threshold Derivative for End-Stage Renal Disease with Mild Cognitive Impairment, 2023, 13, 2076-3425, 1187, 10.3390/brainsci13081187 | |
7. | Xidong Fu, Chaofan Song, Rupu Zhang, Haifeng Shi, Zhuqing Jiao, Multimodal Classification Framework Based on Hypergraph Latent Relation for End-Stage Renal Disease Associated with Mild Cognitive Impairment, 2023, 10, 2306-5354, 958, 10.3390/bioengineering10080958 | |
8. | Alexander N. Pisarchik, Natalia Peña Serrano, Rider Jaimes-Reátegui, 2024, Brain Connectivity Hypergraphs, 979-8-3503-9083-4, 190, 10.1109/DCNA63495.2024.10718518 | |
9. | Manman Yuan, Weiming Jia, Xiong Luo, Jiazhen Ye, Peican Zhu, Junlin Li, 2024, MHSA: A Multi-scale Hypergraph Network for Mild Cognitive Impairment Detection via Synchronous and Attentive Fusion, 979-8-3503-8622-6, 2808, 10.1109/BIBM62325.2024.10822410 |
Item | ESRDaMCI (n = 51) | Normal (n = 39) | t/χ2 | P |
Age (ˉx±s) | 50.05 ± 7.86 | 48.37 ± 6.59 | 1.079 | 0.251 |
Gender (M/F) | 24/27 | 24/15 | 0.341 | 0.536 |
Education years (ˉx±s) | 11.25 ± 3.15 | 9.73 ± 3.85 | 0.973 | 0.771 |
MoCA score (ˉx±s) | 21.30 ± 2.75 | 27.27 ± 1.24 | -13.728 | 0.000 |
Note: ESRDaMCI: ESRD patients diagnosed with MCI; Normal: Normal people; P < 0.05 is a statistically significant difference. |
Methods | ACC (%) | SEN (%) | SPE (%) | AUC |
DKI (FN) | 69.8113 ± 4.2871 | 72.5490 ± 1.4290 | 53.8462 ± 2.5617 | 0.6953 ± 3.3493 |
fMRI (PC) | 72.4314 ± 2.8581 | 71.4435 ± 3.1146 | 66.7949 ± 1.4570 | 0.7504 ± 3.4192 |
Multi-kernel [39] | 73.5821 ± 5.7912 | 69.8234 ± 3.3247 | 75.4712 ± 2.6145 | 0.7231 ± 1.0767 |
MPCA [40] | 67.5541 ± 1.5424 | 66.6715 ± 2.8952 | 79.1628 ± 5.4774 | 0.7671 ± 0.2130 |
KSTM [41] | 70.0756 ± 3.1433 | 80.1643 ± 6.3478 | 61.5717 ± 3.3541 | 0.7982 ± 4.2041 |
JSFC [42] | 63.4320 ± 3.5114 | 73.9417 ± 3.2264 | 60.6984 ± 2.6427 | 0.6864 ± 3.6714 |
MVGCN [43] | 81.6488 ± 4.6481 | 86.8074 ± 1.4194 | 77.5132 ± 4.2438 | 0.8081 ± 4.0278 |
DCNN [44] | 79.4045 ± 2.2725 | 74.1611 ± 0.5384 | 80.9744 ± 3.9925 | 0.7937 ± 0.8421 |
ADBNN [32] | 86.7439 ± 1.4567 | 82.7921 ± 4.5711 | 84.7233 ± 3.7440 | 0.8874 ± 2.1534 |
HRMBN | 91.0891 ± 2.5417 | 86.6461 ± 2.8941 | 89.7436 ± 2.1513 | 0.9023 ± 1.1164 |
Item | ESRDaMCI (n = 51) | Normal (n = 39) | t/χ2 | P |
Age (ˉx±s) | 50.05 ± 7.86 | 48.37 ± 6.59 | 1.079 | 0.251 |
Gender (M/F) | 24/27 | 24/15 | 0.341 | 0.536 |
Education years (ˉx±s) | 11.25 ± 3.15 | 9.73 ± 3.85 | 0.973 | 0.771 |
MoCA score (ˉx±s) | 21.30 ± 2.75 | 27.27 ± 1.24 | -13.728 | 0.000 |
Note: ESRDaMCI: ESRD patients diagnosed with MCI; Normal: Normal people; P < 0.05 is a statistically significant difference. |
Methods | ACC (%) | SEN (%) | SPE (%) | AUC |
DKI (FN) | 69.8113 ± 4.2871 | 72.5490 ± 1.4290 | 53.8462 ± 2.5617 | 0.6953 ± 3.3493 |
fMRI (PC) | 72.4314 ± 2.8581 | 71.4435 ± 3.1146 | 66.7949 ± 1.4570 | 0.7504 ± 3.4192 |
Multi-kernel [39] | 73.5821 ± 5.7912 | 69.8234 ± 3.3247 | 75.4712 ± 2.6145 | 0.7231 ± 1.0767 |
MPCA [40] | 67.5541 ± 1.5424 | 66.6715 ± 2.8952 | 79.1628 ± 5.4774 | 0.7671 ± 0.2130 |
KSTM [41] | 70.0756 ± 3.1433 | 80.1643 ± 6.3478 | 61.5717 ± 3.3541 | 0.7982 ± 4.2041 |
JSFC [42] | 63.4320 ± 3.5114 | 73.9417 ± 3.2264 | 60.6984 ± 2.6427 | 0.6864 ± 3.6714 |
MVGCN [43] | 81.6488 ± 4.6481 | 86.8074 ± 1.4194 | 77.5132 ± 4.2438 | 0.8081 ± 4.0278 |
DCNN [44] | 79.4045 ± 2.2725 | 74.1611 ± 0.5384 | 80.9744 ± 3.9925 | 0.7937 ± 0.8421 |
ADBNN [32] | 86.7439 ± 1.4567 | 82.7921 ± 4.5711 | 84.7233 ± 3.7440 | 0.8874 ± 2.1534 |
HRMBN | 91.0891 ± 2.5417 | 86.6461 ± 2.8941 | 89.7436 ± 2.1513 | 0.9023 ± 1.1164 |