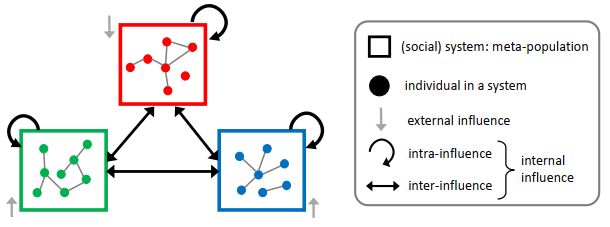
Human interaction patterns on the Web over online social networks vary with the context of communication items (e.g., politics, economics, disasters, celebrities, and etc.), which leads to form unlimited time-evolving curves of information adoption as diffusion proceeds. Online communications often continue to navigate through heterogeneous social systems consisting of a wide range of online media such as social networking sites, blogs, and mainstream news. This makes it very challenging to uncover the underlying causal mechanisms of such macroscopic diffusion. In this respect, we review both top-down and bottom-up approaches to understand the underlying dynamics of an individual item's popularity growth across multiple meta-populations in a complementary way. For a case study, we use a dataset consisting of time-series adopters for over 60 news topics through different online communication channels on the Web. In order to find disparate patterns of macroscopic information propagation, we first generate and cluster the diffusion curves for each target meta-population and then estimate them with two different and complementary approaches in terms of the strength and directionality of influences across the meta-populations. In terms of the strength of influence, we find that synchronous global diffusion is not possible without very strong intra-influence on each population. In terms of the directionality of influence between populations, such concurrent propagation is likely brought by transitive relations among heterogeneous populations. When it comes to social context, controversial news topics in politics and human culture (e.g., political protests, multiculturalism failure) tend to trigger more synchronous than asynchronous diffusion patterns across different social media on the Web. We expect that this study can help to understand dynamics of macroscopic diffusion across complex systems in diverse application domains.
Citation: Minkyoung Kim, Soohwan Kim. Dynamics of macroscopic diffusion across meta-populations with top-down and bottom-up approaches: A review[J]. Mathematical Biosciences and Engineering, 2022, 19(5): 4610-4626. doi: 10.3934/mbe.2022213
[1] | Lu Yu, Yuliang Lu, Yi Shen, Jun Zhao, Jiazhen Zhao . PBDiff: Neural network based program-wide diffing method for binaries. Mathematical Biosciences and Engineering, 2022, 19(3): 2774-2799. doi: 10.3934/mbe.2022127 |
[2] | Simone Göttlich, Stephan Knapp, Dylan Weber . The food seeking behavior of slime mold: a macroscopic approach. Mathematical Biosciences and Engineering, 2020, 17(6): 6631-6658. doi: 10.3934/mbe.2020345 |
[3] | Andriy A. Avramenko, Igor V. Shevchuk . Renormalization group analysis of heat transfer in the presence of endothermic and exothermic chemical reactions. Mathematical Biosciences and Engineering, 2019, 16(4): 2049-2062. doi: 10.3934/mbe.2019100 |
[4] | Karl Peter Hadeler . Structured populations with diffusion in state space. Mathematical Biosciences and Engineering, 2010, 7(1): 37-49. doi: 10.3934/mbe.2010.7.37 |
[5] | Marcelo E. de Oliveira, Luiz M. G. Neto . Directional entropy based model for diffusivity-driven tumor growth. Mathematical Biosciences and Engineering, 2016, 13(2): 333-341. doi: 10.3934/mbe.2015005 |
[6] | Karim El Laithy, Martin Bogdan . Synaptic energy drives the information processing mechanisms in spiking neural networks. Mathematical Biosciences and Engineering, 2014, 11(2): 233-256. doi: 10.3934/mbe.2014.11.233 |
[7] | Elvira Barbera, Giancarlo Consolo, Giovanna Valenti . A two or three compartments hyperbolic reaction-diffusion model for the aquatic food chain. Mathematical Biosciences and Engineering, 2015, 12(3): 451-472. doi: 10.3934/mbe.2015.12.451 |
[8] | József Z. Farkas, Peter Hinow . Physiologically structured populations with diffusion and dynamic boundary conditions. Mathematical Biosciences and Engineering, 2011, 8(2): 503-513. doi: 10.3934/mbe.2011.8.503 |
[9] | Minus van Baalen, Atsushi Yamauchi . Competition for resources may reinforce the evolution of altruism in spatially structured populations. Mathematical Biosciences and Engineering, 2019, 16(5): 3694-3717. doi: 10.3934/mbe.2019183 |
[10] | Christina Surulescu, Nicolae Surulescu . Modeling and simulation of some cell dispersion problems by a nonparametric method. Mathematical Biosciences and Engineering, 2011, 8(2): 263-277. doi: 10.3934/mbe.2011.8.263 |
Human interaction patterns on the Web over online social networks vary with the context of communication items (e.g., politics, economics, disasters, celebrities, and etc.), which leads to form unlimited time-evolving curves of information adoption as diffusion proceeds. Online communications often continue to navigate through heterogeneous social systems consisting of a wide range of online media such as social networking sites, blogs, and mainstream news. This makes it very challenging to uncover the underlying causal mechanisms of such macroscopic diffusion. In this respect, we review both top-down and bottom-up approaches to understand the underlying dynamics of an individual item's popularity growth across multiple meta-populations in a complementary way. For a case study, we use a dataset consisting of time-series adopters for over 60 news topics through different online communication channels on the Web. In order to find disparate patterns of macroscopic information propagation, we first generate and cluster the diffusion curves for each target meta-population and then estimate them with two different and complementary approaches in terms of the strength and directionality of influences across the meta-populations. In terms of the strength of influence, we find that synchronous global diffusion is not possible without very strong intra-influence on each population. In terms of the directionality of influence between populations, such concurrent propagation is likely brought by transitive relations among heterogeneous populations. When it comes to social context, controversial news topics in politics and human culture (e.g., political protests, multiculturalism failure) tend to trigger more synchronous than asynchronous diffusion patterns across different social media on the Web. We expect that this study can help to understand dynamics of macroscopic diffusion across complex systems in diverse application domains.
In a social system, a "connection" or "relationship" between individuals can be identified by diverse interaction events such as site-specific actions on the Web (e.g., mention, reply, like, retweet), collaborations, friendship, kinship, and even spatio-temporal vicinity [1]. Such interactions collectively generate dynamic communication pathways over heterogeneous social networks constituting complex systems, through which a wide range of (information) items propagate. Most prior work has focused on mutual interactions in a single homogeneous social system and investigated their interaction patterns within the system's border [2,3,4,5]. However, an individual item may reach beyond its originated social system and continue to navigate across different populations. For instance, breaking news reports from mainstream media spread through diverse social networking sites and also blogs [6,7]. Not limited to information, an infectious disease such as dengue and COVID-19 spreads across international borders, leading to pandemic [8,9]. Such far-reaching pathways in the real world bring forward global diffusion over heterogeneous meta-populations and lead to form unlimited adoption or infection curves of affected individuals. In addition, the diffusion patterns vary with the social context [1,10,11], which can be largely categorized into two major trends, synchronous and asynchronous diffusion across meta-populations.
In this respect, we focus on borderless diffusion of individual items, which is beyond local propagation within a single homogeneous social system and across the borders of heterogeneous meta-populations. Such panoramic view helps better understand general dynamics of diffusion, not limited to site-specific social behavior. For the study, we review both top-down and bottom-up approaches to estimate macroscopic diffusion as a complementary way of understanding emergent phenomena in the real world. Top-down and bottom-up approaches have been largely considered as design strategies of knowledge discovery in diverse research communities, where a top-down approach starts with big picture of a complex system and subsequent stepwise refinement while a bottom-up approach begins with pieces of systems bringing about more complex systems [12]. Here, we investigate model-driven methods based on hypotheses of a complex system as top-down approaches and data-driven or model-free methods without any assumptions as bottom-up approaches, whose advantages and disadvantages are discussed.
In addition, we conduct a case study by using a dataset provided by [13], which contains daily adopters for over 60 news topics via different types of online social media such as mainstream news, social networking sites, and blogs. In order to find disparate patterns of macroscopic information propagation, we first generate and cluster the diffusion curves for each meta-population, by considering the different types of online media as heterogeneous meta-populations. We then estimate each cluster with both top-down and bottom-up approaches as complementary methods, based on which underlying dynamics is interpreted in terms of the strength and directionality of influences on each target meta-population. In terms of influence strength, we find that synchronous global diffusion is not possible without very strong intra-influence on each population. In terms of directionality, such concurrent propagation is likely brought by transitive influences among different populations. In other words, different social systems in transitive relations tend to exhibit similar diffusion trends with each other. When it comes to social context, controversial news topics in politics and human culture (e.g., political protests in the Middle East, multiculturalism failure) tend to trigger more synchronous than asynchronous diffusion patterns on the Web. We expect that this study can provide a comprehensive way of understanding general dynamics of macroscopic diffusion across complex systems in diverse application domains.
The rest of the paper is structured as follows. Section 2 introduces a conceptual framework for global diffusion at a macro level, and Section 3 and 4 review top-down and bottom-up approaches to estimate and understand macroscopic diffusion of individual items, whose approaches are compared in Section 5. Section 6 shows a case study with real data by applying the discussed approaches in the previous sections, discusses distinct patterns observed in global diffusion, and interprets underlying dynamics from diverse perspectives. Finally, Section 7 concludes this study with future work.
A variety of individual items spreads beyond a homogeneous social system and across heterogeneous social systems consisting of different meta-populations. For instance, public events are reported by mainstream media first (e.g., CNN, BBC, LeMonde, etc.), and they are shared over online social networks via diverse media channels (e.g., Twitter, Facebook, Instagram, blogs, etc.) or the other way around, since diverse information sources are increasingly accessible than ever before [14]. When it comes to epidemics, disease outbreaks sweep through a nation and spread across international borders as transportation systems accelerate human mobility [9,15,16]. That is, diffusion space is not limited to a homogeneous single social system but expanded to complex systems consisting of multiple meta-populations, as shown in Figure 1.
Traditionally, a diffusion framework mainly consist of external influence outside of a homogeneous social system and internal influence led by mutual and cascading interactions between individuals in the system [17]. However, communication channels have no border and are rather far reaching beyond a single social system [7,9,18]. Thus, our study mainly focuses on macroscopic diffusion with the conceptual framework in Figure 1. Accordingly, internal influence in global diffusion can be divided into intra- and inter-influence. Here, intra-influence implies interactions between members within each social system or meta-population, while inter-influence indicates interactions between members in different social systems or meta-populations.
We first investigate top-down approaches which are based upon assumptions on diffusion space as complex systems, formulating a framework with interpretable model parameters, and thus they are also called model-driven approaches. In other words, we do not have to rely on detailed specifications of biased or incomplete data to estimate macroscopic diffusion. For instance, structural comparisons between different real-world social networks have been studied in order to understand frequent and significant human interaction patterns, by finding subgraphs [19,20] or network motifs [21,22]. However, such topological analysis of networks' current snapshot has limitations in understanding comprehensive human interaction patterns due in part to unknown, incompletely collected, or time-evolving social relationships in the real world. In this regard, we review top-down approaches from a fundamental framework based on ordinary differential equations (ODE), to its extended probabilistic approach, and to a representative point process method. These all approaches estimate the behavior of complex systems at a macro level without detailed topological information of social networks and can provide rich context of underlying diffusion dynamics based on estimated parameter values.
The hazard function, also called hazard rate, h(x) is the ratio of the probability density function P(x) to the survival function S(x) as:
h(x)=P(x)S(x)=fX(x)1−FX(x), | (3.1) |
where FX(x)=∫x−∞fX(u)du and fX(x)=ddxFX(x). The hazard rate denotes the likelihood that an item will die or fail based on a given age x it has reached. In terms of the diffusion rate, it is governed by a hazard function h(t) as the ratio of new adopters to the number of potential adopters at time t, given a population size n as:
h(t)=a(t)n−A(t)=f(t)1−F(t), | (3.2) |
where A(t) and a(t)=dA(t)/dt denote the number of cumulative adopters and new adopters at time t, respectively. Accordingly, the proportion of the cumulative adopters is denoted by F(t)=A(t)/n and the proportion of new adopters by f(t)=dF(t)/dt=a(t)/n. That is, the hazard rate becomes the diffusion rate implying the likelihood that an individual item will be adopted based on its survival to an earlier time t (i.e., not adopted until time t). This hazard function has been an fundamental framework for diffusion processes, such as the Bass model [23] in economics as:
f(t)1−F(t)=p+qF(t), | (3.3) |
where the parameter p is called the coefficient of innovation, since it does not interact with the cumulative adopter proportion F(t), and q is called the coefficient of imitation, because it represents the internal influence of previous adopters. Equation (3.3) has a closed form solution as:
F(t)=1−e−(p+q)t1+qpe−(p+q)t. | (3.4) |
As Equation (3.3) shows, the fundamental assumption is that a underlying population is not only homogeneous but also fully connected as in the traditional epidemic models [24]. Regardless of such unrealistic assumption, this fundamental diffusion framework has been applied and extended by diverse fields such as marketing, computer science, and operations research, by focusing on either the heterogeneity of populations [25,26], network structures (e.g., cluster density, reachability, and degree distributions) [27,28,29], or both [14].
As shown in Equation (3.3), the hazard rate is defined as a simple linear form of the proportion of the cumulative adopters F(t) until time t. That is, it allows for models in the form of ordinary differential equations for the fraction of individuals (i.e., adopted, infected, purchased, and etc.).
In a probabilistic point of view, the hazard function in Equation (3.1) can be defined as a new adoption probability that an average individual, who has not adopted before, adopts at time t as:
f(t)1−F(t)=P(A=a∣¬a,t), | (3.5) |
where A(={a,¬a}) is a binary random variable for the event of individual's adoption (A=a) or not (A=¬a).
Accordingly, the Dynamic Influence Model (DM) defines the probability in Equation (3.5) as the union of two events, external and internal influences on adoption as [6]:
P(a|¬a,t)=Pext(a|¬a,t)+(1−Pext(a|¬a,t))Pint(a|¬a,t), | (3.6) |
where Pext(a|¬a,t) and Pint(a|¬a,t) denote the new adoption probabilities by external and internal influences, respectively.
In order to deal with the heterogeneity of populations, DM introduces a discrete random variable, i=1,...,m for different types of m meta-populations, and accordingly the adoption probability in Equation (3.6) are constructed for each population i=1,...,m as:
P(A=a|¬a,i,t)=Pext(a|¬a,i,t)+(1−Pext(a|¬a,i,t))Pint(a|¬a,i,t). | (3.7) |
Also, by incorporating the structural connectivity of underlying social networks of size n with a power-law degree distribution in a probabilistic way, the adoption probability by internal influence, Pint(a|¬a,i,t) in Equation (3.7) becomes:
Pint(a∣¬a,i,t)=1−1ζ(α)n−1∑k=1(1−m∑j=1cjiP(a|j,t))kkα, | (3.8) |
where α is the power law coefficient, ζ(α)=∑n−1k=1k−α, and cji∈[0,1] denotes the probability that an individual of type i adopts when it is exposed to a previous adopter of type j in its neighbors (refer to [6] for details). Note that i in Equation (3.8) is an average individual of a meta-population type i who adopts when its neighbors have adopted in a probabilistic way. Here, the degree distribution of an individual is assumed to follow a power-law, since real-world networks are scale-free networks exhibiting power-law distributions [24]. Thus, this model does not require micro-level local structures of contact networks.
This macro-level diffusion model incorporates two main features of underlying networks, heterogeneity and structural connectivity, in a probabilistic way, rather than constructing and measuring the current snapshot of networks. This enables to estimate temporal dynamics of global diffusion in diverse application domains in the real world, by avoiding manual and incomplete construction of underlying networks.
Global diffusion can be considered as point processes of adoption event arrivals, exhibiting collective bursty behaviors in the real world: e.g., abrupt popularity growth of an information item, pandemic, and political protests. A point process is an ordered set of random variables in time, geographical space, or more general spaces [30]. In particular, a temporal point process is a counting process {N(t),t≥0}, where N(t) denotes the number of events that occur up to time t. This temporal point process can be characterized by a conditional intensity function, λ(t) as:
λ(t)=limΔt→0P{N(t+Δ)−N(t)=1∣Ht}Δt, | (3.9) |
where Ht is the history of events that have occurred before time t. This can be interpreted that λ(t) estimates the infinitesimal rate of an event in the immediate future, conditioned on the event history prior to time t.
When the intensity function λ(t) is constant over time, it is called a homogeneous Poisson process, otherwise a nonhomogeneous Poisson process. On the other hand, a Hawkes process [31] is a non-Markovian extension of the Poisson process, which enables clustering the arrival of events [32]. That is, the intensity is likely dependent on the history of event occurrences as:
λ(t)=μ+∑{k:tk<t}g(t−tk), | (3.10) |
where μ denotes a baseline intensity, and g is a triggering kernel affecting clustered inter-event times. There have been large extensions of A Hawkes process by generalizing a baseline intensity μ and the triggering kernel g across diverse research areas [1].
In terms of global diffusion across meta-populations, a Hawkes process in Equation (3.10) can also be constructed for each population i=1,...,m as:
λi(t)=μi+m∑k=1λki(t), | (3.11) |
where the intensity function of population i at time t, λi(t), is defined with its baseline intensity μi and doubly stochastic point processes λki(t) based on the superposition property of Poisson processes [33].
Latent Influence Point Process model (LIPP) extends a multidimensional Hawkes process in Equation (3.11) by incorporating major counter-balancing factors such as exogenous/endogenous influences and a time decay effect [9]. At a macro level, internal dynamics across complex systems drives bursts of events via intra- and inter-system interactions [1]. In this context, LIPP incorporates cross-regional human mobility as macro-level endogenous effects on diffusion, which defines λki(t) in Equation (3.11), the endogenous intensity via mutual excitations across multiple regions, as:
λki(t)=∑ti<tζ(i,k)ξkρkiϕi(t−ti), | (3.12) |
where ζ(⋅,⋅) function controls self-excitations, and ξk>0 represents the latent influence (infectiousness) of a meta-population k on other meta-populations, due in part to population density, social interactivity, transportation hub, and vicinity to virus-endemic regions, embedding socio-economic factors. The third term ρki>0 represents the strength of directed connectivity from k to i based on human mobility(interaction) patterns such that ∑mi=1ρki=1. Finally, the last term ϕi(⋅) represents the time relaxation function for reflecting the effect of time decay on the likelihood of diffusion. Refer to [9] for more details.
In this section, we investigate bottom-up approaches which are independent of any assumptions on a complex system, and thus called model-free or data-driven approaches. Information theory, introduced by Shannon, provides the benefits of quantifying non-linear dynamics of complex systems, such as information of a random variable, a collection of variables, and exchanges between variables [14]. Such quantification makes information theoretic-measures model-free, since top-down approaches are based upon assumptions, i.e., model-driven [34]. In other words, we do not necessarily define ad hoc models for any assumptions on underlying social structures which often depend on site-specific actions (e.g., mentions, retweets, and hashtags in Twitter and like, share, and comments in Facebook).
Information-theoretic measures have been used in predicting user behavioral patterns in social media, which have largely focused on interactions within a homogeneous social system. For instance, the entropy is used for classifying Twitter user behaviors [35] and for predicting mobility in real [36] or virtual [37] human lives, the mutual information is used for predicting individual or group level future interactions [38], and the transfer entropy is used for detecting relationships between Twitter users independent of the knowledge of follower-followee social structures in Twitter [39,40].
A stochastic process is an ordered set of random variables, and thus it can be considered as time-evolving random variables when they are indexed by time [41]. When we consider a discrete-time stochastic process X as:
X:={Xt:t∈T}, | (4.1) |
where T is an ordered set of time t, and Xt is a random variable indexed by time t.
A meta-population produces a wide range of time-series activity sequences such as growth of product purchases over time, daily case reporting of an infections disease, retweet trends of news in Twitter, and so forth. That is, diffusion over a homogeneous population leads to form a time-evolving curve of observations, which can be considered a meta-population's social signal affecting other neighboring meta-populations. In other words, collective behaviors of a social system are attributed to behavioral changes of different systems. For instance, popularity growth of an individual item (e.g., an original content from Netflix, COVID-19, a consumer product, and etc.) in a specific country or continent is contagious worldwide, affecting the growth patterns of the same item in neighboring countries or continents in a similar way.
Accordingly, a source social system generates a signal such as time-series growth rates of adopters in the system, and the signal is encoded and transmitted to a destination social system through a noisy channel. The encoded signal X is noise-corrupted after passing through the communication channel, and its decoded signal Y is received by the destination system. Thus, identifying a social system's signals is important to catch invisible information transfer between stochastic processes. For identifying a macro-level signal of a meta-population in [41], the changes of an individual item's growth rate are captured as acceleration A over time. Three discrete states of a social system as a stochastic process X={Xt:t∈T} as:
Xt={−1(decrease),ifAt∈(−∞,−τ]0(transition),ifAt∈(−τ,τ)+1(increase),ifAt∈[τ,∞), | (4.2) |
where At=f(t)−f(t−1), and the value of τ is determined based on real data so that the probabilities of three states are equally likely (uniformly distributed). Here, f(t) is the proportion of adopters at time t given a meta-population's size n in a social system.
A simplified social signal, defined as a stochastic process in Equation (4.2), enables to estimate information transfer at a macro level [14]. Based on the definition of a stochastic process in Equation (4.1), the information-theoretic measures can estimate macroscopic information transfer between meta-populations. The mutual information cannot capture the directionality of information flow between two random processes due to its symmetric property. On the contrary, the transfer entropy, introduced by Schreiber [42], is defined to consider causal relations between two stochastic processes, X and Y as:
TEY→X=I(Xt;Yht−1|Xkt−1) | (4.3) |
=H(Xt|Xt−1:t−k)−H(Xt|Xt−1:t−k,Yt−1:t−h)=∑P(Xt,X(k)t−1,Y(h)t−1)logP(Xt|X(k)t−1,Y(h)t−1)P(Xt|X(k)t−1), | (4.4) |
where t is a time index, and k and h denote the Markov order for the previous states of X and Y, respectively such that X(k)t={Xt−1,...,Xt−k} and Y(h)t={Yt−1,...,Yt−h}. The transfer entropy TEY→X describes the reduction of uncertainty of the state of Xt, given k previous states of the destination process X, by introducing h previous states of the source process Y. In addition, the transfer entropy can be considered as the conditional mutual information as Equation (4.3), which implies the average information shared between the past (Yht−1) of the source process Y and the next state (Xt) of the destination process X except for X's past (Xkt−1).
Note that the mutual information I(X;Y) is the Kullback-Leibler divergence [43] of the joint distribution P(X,Y) from the product distribution P(X)P(Y). Accordingly, the transfer entropy is the Kullback-Leibler divergence between two conditional probability distributions P(Xt|X(k)t−1) and P(Xt|X(k)t−1,Y(h)t−1) as:
TEY→X=DKL(P(Xt|X(k)t−1)∥P(xt|X(k)t−1,Y(h)t−1)). |
We need consider distinct adoption behaviors between meta-populations in terms of time-delay and memory effects. For instance, news diffusion in social media exhibits different growth patterns: relatively faster in mainstream news and social networking sites than blogs [14]. When it comes to disease spread, viruses tend to be rapidly transmitted in central cities than peripheral or rural areas due in part to dominant population fluxes via transportation systems [9]. In this context, the transfer entropy in Equation (4.4) can be modified by incorporating the length of time-delay d and memory k,h so that we can analyze the effect of the d time-shifted recent h or k states of a meta-population on the future state of the other meta-population. The transfer entropy with time-delay (d) and memory effects (k,h) is defined as:
TEY→X=I(Xt;Yht−d∣Xkt−d)=H(Xt|Xt−d:t−k−d+1)−H(Xt|Xt−d:t−k−d+1,Yt−d:t−h−d+1)=∑P(Xt,X(k)t−d,Y(h)t−d)logP(Xt|X(k)t−d,Y(h)t−d)P(Xt|X(k)t−d), | (4.5) |
where d denotes the length of time-delay, and it is the only difference between Equation (4.4) and (4.5). For simplicity, we can assume the Markov orders of the X's past and Y's past are same (k=l). These time-delay and memory effects cannot be estimated by top-down approaches discussed in the previous section, and thus this bottom-up approach can provide new perspectives on macro-level diffusion.
The discussed fundamental framework of top-down approaches is based on ordinary differential equations by defining the hazard rate as a linear form of the proportion of cumulative adopters. This is a simple and robust approach for predicting an individual item's popularity in a homogeneous and fully mixing social system. However, underlying populations in the real world exhibit heterogeneous and scale-free network structures. Accordingly, the Dynamic Influence Model (DM) extends this fundamental framework by incorporating the heterogeneity of complex systems and structural connectivity with a power-law degree distribution so that it can mimic real-world social networks. Thus, DM enables to estimate macroscopic diffusion across different populations with the consideration of degree distributions. Its limitation is that the system of partial derivative equations for DM is not mathematically tractable, which requires to solve it numerically to get the adoption probabilities of each meta-population. That is, the more meta-populations likely increase parameter estimation errors, leading to wrong interpretations of dynamics. While DM defines the hazard function as a new adoption probability that an average individual, who has not adopted before, adopts at specific time, the Latent Influence Point Process model (LIPP) is non-Markovian extension of the Poisson process conditioned on the history of event occurrences with time decay effects. That is, DM considers the adoption history but ignores time decay of previous events. LIPP is based on a point process, where spatiotemporal events are well realized due to its flexible consideration of lasting impact of bursty behaviors rather than a current snapshot. However, LIPP needs information of structural connectivity between meta-populations, and thus it is advantageous when estimating bursty and clustered events in diffusion processes with known structures of meta-populations such as transportation routes, cities, and countries.
Information-theoretic measures as the fundamental framework of bottom-up approaches can directly quantify non-linear dynamics of complex systems without any assumptions, which makes them model-free. As each meta-population produces a time-series activity sequence, it can be considered a social signal and defined as a stochastic process, i.e., time-ordered set of random variables. That is, the identification of a stochastic process and the quantification of exchanges between identified processes are not affected by the number of meta-populations, in contrast to parameter errors in top-down approaches. While the mutual information cannot capture the directionality of influence between meta-populations due to its symmetric property, transfer entropy can estimate causal relations between two stochastic processes by quantifying reduced uncertainty of a destination process state by introducing previous states of a source process. Incorporating time-delay and memory effects into transfer entropy makes it possible to characterize each population with responsiveness and persistency, respectively.
The discussed top-down approaches can estimate macroscopic diffusion without detailed network topologies in the complex environment and can provide rich context of dynamics in terms of strength and directionality of intra- and inter-influences within and between meta-populations. However, the methods start with assumptions on a complex system, such as a power-law degree distribution in DM and network structures among meta-populations in LIPP. On the other hand, the introduced bottom-up approaches do not require any assumptions on such structural connectivity by using information-theoretic measures. These non-parametric statistics provide benefits of quantifying non-linear dynamics of complex systems, but a small sample size of each meta-population leads to a biased probability distribution of a target random variable. Thus, the two approaches in conjunction can help to obtain more significant diffusion patterns by comparing the estimation results from the approaches and maximizing the similarity between them. When it comes to interpretation of underlying diffusion dynamics, the top-down approaches enable to reveal the strength and directionality of influence within and between meta-populations as well as external influence outside each population. The bottom-up approach also provides the strength and directionality of inter-influence between different populations except for intra-influence and external influence. However, the time-delay and memory effects of the transfer entropy can additionally provide behavioral characteristics of each population.
All in all, these two different approaches together provide a complementary macroscopic picture of diffusion dynamics by filling the gap which either approach cannot provide alone, and thus they can help better understand real-world diffusion in a comprehensive way. They also suggest alternative options to choose a more appropriate approach in accordance to data availability and experimental conditions of different application domains.
In this section, we conduct a case study with real data consisting of time-evolving information adopters for each meta-population. We first cluster time-series curves and find distinct patterns so that we can better understand real-world diffusion in a more principled way. We then estimate each clustered curve with both top-down and bottom-up approaches and interpret dynamics of macroscopic diffusion on the Web in a complementary way.
For an exemplary case study of global diffusion, we target news propagation across different types of online social media by using the dataset provided by [13]. This dataset consists of time-series adopters for over 60 news topics via mainstream news media (News), social networking sites (SNS), and personal blogs (Blog), during a one month period.
For extracting distinct diffusion patterns, we clustered the cumulative diffusion rates (F) of all news topics for each social media (News, SNS, and Blog) as a representative meta-population on the Web. As a clustering method, we use the simple and robust k-means clustering [44] which is a centroid-based algorithm finding a fixed number (k) of clusters in a dataset by calculating Euclidean distances between two vectors (F) and minimizing within-cluster variances (squared Euclidean distances). In this way, the time-series adoption sequences are partitioned into k similar diffusion patterns. By varying the value of k from k=2 to k=10, we obtained five distinct clusters. Figure 2 shows the obtained five clusters of macro-level diffusion across different social media types (News, SNS, Blog), where each plot exhibits the averaged cumulative diffusion rates F for each media. As shown in the figure, mainly four disparate patterns (EAG, ESG, LAG, and LSG) are disclosed, which will be discussed in the next subsection.
The five clusters are first estimated by DM as a top-down approach, since structural connectivity among the target meta-populations are not only unknown but also adoption events are collected on a daily basis without detailed web posting time. Based on parameter estimations, the strength and directionality of influences in macroscopic news diffusion across the media are illustrated in Figure 3.
● Cluster #1. EAG (Early and Abrupt Growth): In Figure 2(a), this cluster exhibits the early and abrupt growth patterns in news diffusion. As the figure shows, the growth rate in SNS is even faster than News. Example news topics are "Brazil Floods" and "Golden Globe Awards" each of which has drawn wide attention from the public. As shown in Figure 3(a), News and SNS show very strong intra-influences, and they also strongly influence with each other. On the other hand, Blog shows relatively weak intra-influence, but it is both externally and internally influenced in a balanced way.
● Cluster #2. ESG (Early and Slow Growth): In Figure 2(b), this cluster exhibits the early but slow growth patterns in news diffusion, illustrating concave-shaped diffusion curves in News and SNS rather than traditional S-curves. This can be interpreted that innovators or early adopters [17] are eager to introduce news at an early stage, but such efforts are hardly move their contact networks to keep spreading the news (i.e., narrower attention from the public than Cluster #1). Relevant news items are mostly on famous public figures (e.g., "Actress Zsa Zsa Gabor", "Google's outgoing CEO Schmitdt", and "Support for Julian Assange") or even a popular product (e.g., iPad). In Figure 3(b), external influences on News and SNS are stronger even than its own intra-influences except for Blog.
● Cluster #3: ESG (Early and Slow Growth): The large proportion (50%) of the selected news topics belong to this pattern. In Figure 2(c), this cluster also exhibits the early and slow growth patterns in news diffusion but with different dynamics compared to Cluster #2. As shown in Figure 3(b), News shows stronger external influence than intra-relationship. On the contrary, SNS and News show stronger intra-relationships, but they are relatively weak compared to other clusters. As shown in Figure 2(c), SNS and Blog produce relatively flat S-curves compared with Cluster #1, #4, and #5, and News shows the concave-shaped curve as in the Pattern #2. This can be interpreted that mainstream news sites are eager to introduce new information, but their choices are not always successful to draw wide attentions from the public.
● Cluster #4. LAG (Late and Abrupt Growth): This pattern shows the most highly synchronous diffusion curves across the different types of social media, as shown in Figure 2(d). In Figure 3(d), intra-relationships of News, SNS, and Blog are all balanced and the strongest among the clusters, while external influences are ignorable and inter-relationships between the media are relatively balanced compared to other clusters. Related news items are on disputable topics in culture such as "Multiculturalism Failure", and "Muslim-Christian Conflicts". Such controversial issues are not normally expected but draw abrupt and wide attentions from the public when the issues occurred, which more likely leads to synchronous diffusion patterns across heterogeneous social systems.
● Cluster #5. LSG (Late and Steady Growth): In Figure 2(e), News, SNS, and Blog exhibit similar S-curves, showing concurrent diffusion patterns across the systems. In Figure 3(e), News, SNS, and Blog all show stronger intra-influences than inter-influences, but weaker intra-influence than Cluster #1 and #4. At first external influences are weak, but intra-influences and inter-relationships between the media become gradually stronger. This can be interpreted that the political protests in the Middle East gradually and steadily affect neighboring countries throughout diverse social media channels.
Traditionally, news media have been considered as separated external sources, but Cluster #1(EAG), #4(LAG), and #5(LSG) show that they interact with each other as if they were in a social network. In other words, such collective behaviors of news media reflect the topic or context of news items. Pattern #4(LAG) and #5(LSG) shows similar diffusion patterns across the different types of media, which are driven by both strong intra- and inter-influences in a balanced way. The big difference between these asynchronous and synchronous is the strength of intra-influences. That is, stronger intra-relationships more likely reflect influences from other social media and fuel diffusion within each media, leading to a more synchronous and simultaneous diffusion across online social media.
The five clusters in Figure 2 are also estimated by Transfer Entropy with time-delay and memory effects as a bottom-up approach, and Figure 4 shows macroscopic information pathways between the media. As shown in the figure, Cluster #1(EAG), #4(LAG), and #5(LSG) exhibit transitive relations, and particularly Cluster #4(LAG) and #5(LSG) present the same unique structure. In other words, different social systems in transitive relations tend to exhibit similar diffusion trends with each other. In terms of time-delay (the level of recency) and memory effects (the length of adoption histories), more recent and longer stochastic processes more likely influence others. The strongest memory effect on diffusion is observed in Blog, which means that Blog is more influenced by longer adoption trends in News and SNS. Regarding news topics, culture and disaster news categories exhibit the strongest memory effects, while the science and celebrity category shows the weakest. It can be interpreted that people tend to pay longer attention to controversial and life-related events than scientific and celebrity news topics. Such estimations imply that the bottom-up approach can provide behavioral characteristics of meta-populations, which cannot be provided by the top-down approaches.
Synchronous diffusion is shown in transitive relations among meta-populations, but it is not possible without strong and balanced intra-influences across the complex system. For instance, both balanced and strong intra-influences of Cluster #4(LAG) exhibit the most synchronous and simultaneous diffusion across the media, while the balanced but weaker intra-influence of Cluster #5(LSG) shows a relatively less synchronous diffusion patterns than Pattern #4(LAG). Unbalanced intra-influences of Cluster #1(EAG) show the least synchronous diffusion compared to these three clusters, even though it shows the most interactive unique structure among the possible network motifs. When it comes to social context, controversial news topics in politics and human culture (e.g., political protests in the Middle East, multiculturalism failure) tend to trigger more synchronous than asynchronous diffusion patterns on the Web.
Collective interactions in complex systems have no border and generate communication pathways over heterogeneous social networks. A wide range of individual items propagates through dynamic pathways which often reflect the social context of the items. This study focuses on top-down and bottom-up approaches to estimate global diffusion and ultimately understand underlying dynamics among meta-populations at a macro level. Top-down approaches assume underlying networks without the need to construct and measure complete structures and can provide rich context; we can interpret of diffusion dynamics in terms of external and internal influences. While bottom-up approaches are independent of any assumptions on networks and allow quantification of information pathways in terms of the strength and directionality of influences between meta-populations with time-delay and memory effects. These two approaches can be complementary to conduct more robust estimation and help better understand global diffusion.
From the case study with real data, we try to discover general dynamics of global diffusion by clustering distinct diffusion curves, investigating their context leading to common or different dynamics with both top-down and bottom-up approaches, and analyzing the discovered patterns in terms of synchronous and asynchronous global diffusion across the representative online social media (News, SNS, and Blog). In common, strong and balanced internal influences across the systems more likely drive synchronous diffusion. In general, transitive relations have more opportunities to bring about similar diffusion patterns across the systems, but the strength as well as balance of intra-influences are also significant to synchronous global diffusion. That is, concurrent propagation is likely driven by strong intra-relationships as momentum turning a system's state into a critical mass, which is triggered by transitive relationships among social systems. Also, synchronous diffusion are observed in controversial news items such as multiculturalism and political protests, which implies that synchronous and asynchronous diffusion is topic sensitive. Dynamic influence with different strength and directionality leads to form unlimited diffusion patterns, but from the generated curves we can now draw an high-level picture of diffusion dynamics among meta-populations. We expect that this study can help obtain underlying dynamics of global diffusion across complex systems in diverse application domains. As future work, we plan to combine the both top-down and bottom-up approaches into a unified framework and improve the performance of estimation.
This work was supported by the National Research Foundation of Korea(NRF) grant funded by the Korea government(MSIT) (No. NRF-2020R1G1A1011097).
The author declare no conflict of interest. The funders had no role in the design of the study; in the collection, analyses, or interpretation of data; in the writing of the manuscript, or in the decision to publish the results
[1] |
M. Kim, D. Paini, R. Jurdak, Real-world diffusion dynamics based on point process approaches: A review, Artific. Intell. Rev., 53 (2020), 321–350. https://doi.org/10.1007/s10462-018-9656-9 doi: 10.1007/s10462-018-9656-9
![]() |
[2] | S. Gao, J. Ma, Z. Chen, Modeling and predicting retweeting dynamics on microblogging platforms, in Proc. 8th ACM Int'l Conf. Web Search Web Data Mining, (2015), 107–116. https://doi.org/10.1145/2684822.2685303 |
[3] | H. Shen, D. Wang, C. Song, A.-L. Barabási, Modeling and predicting popularity dynamics via reinforced poisson processes, in Proc. 28th AAAI Conf. Artific. Intell, (2014), 291–297. |
[4] |
D. Wang, C. Song, A.-L. Barabási, Quantifying long-term scientific impact, Science, 342 (2013), 127–132. https://doi.org/10.1126/science.1237825 doi: 10.1126/science.1237825
![]() |
[5] | Q. Zhao, M. A. Erdogdu, H. Y. He, A. Rajaraman, J. Leskovec, Seismic: A self-exciting point process model for predicting tweet popularity, in Proc. ACM SIGKDD Int'l Conf. Knowl. Disc. Data Mining, (2015), 1513–1522. https://doi.org/10.1145/2783258.2783401 |
[6] |
M. Kim, D. Newth, P. Christen, Modeling dynamics of diffusion across heterogeneous social networks: News diffusion in social media, Entropy, 15 (2013), 4215–4242. https://doi.org/10.3390/e15104215 doi: 10.3390/e15104215
![]() |
[7] | M. Kim, L. Xie, P. Christen, Event diffusion patterns in social media, in Proc. 6th Int'l AAAI Conf. Weblogs Soc. Media, (2012), 178–185. |
[8] |
L. M. Aiello, D. Quercia, K. Zhou, M. Constantinides, S. Šćepanović, S. Joglekar, How epidemic psychology works on Twitter: Evolution of responses to the COVID-19 pandemic in the U.S., Humanit. Soc. Sci. Commun., 8 (2021), 1–15. https://doi.org/10.1057/s41599-021-00861-3 doi: 10.1057/s41599-021-00861-3
![]() |
[9] |
M. Kim, D. Paini, R. Jurdak, Modeling stochastic processes in disease spread across a heterogeneous social system, The Proc. Nat. Acad. Sci., 116 (2019), 401–406. https://doi.org/10.1073/pnas.1801429116 doi: 10.1073/pnas.1801429116
![]() |
[10] |
R. Crane, D. Sornette, Robust dynamic classes revealed by measuring the response function of a social system, The Proc. Nat. Acad. Sci., 105 (2008), 15649–15653. https://doi.org/10.1073/pnas.0803685105 doi: 10.1073/pnas.0803685105
![]() |
[11] |
M. Kim, Understanding time-evolving citation dynamics across fields of sciences, Appl. Sci., 10 (2020), 5846. https://doi.org/10.3390/app10175846 doi: 10.3390/app10175846
![]() |
[12] | H. A. Simon, The Sciences of the Artificial, Reissue of The Third Edition With A New Introduction by John Laird, The MIT Press, 2019. |
[13] | M. Kim, D. Newth, P. Christen, Modeling dynamics of meta-populations with a probabilistic approach: Global diffusion in social media, in Proc. 22nd ACM Int. Conf. Info. Knowl. Manag., (2013), 489–498. https://doi.org/10.1145/2505515.2505583 |
[14] | M. Kim, Dynamics of Information Diffusion, Ph.D. thesis, The Australian National University, 2015. |
[15] | M. Kim, R. Jurdak, Heterogeneous social signals capturing real-world diffusion processes, in Proc. 2nd Int. Wksp. Soc. Sens., (2017), 95. https://doi.org/10.1145/3055601.3055617 |
[16] | K. Zhang, M. Kim, R. Jurdak, D. Paini, Predictability of irregular human mobility, arXiv: 1709.08486. https://doi.org/10.48550/arXiv.1709.08486 |
[17] | E. Rogers, Diffusion of Innovations, Free Press of Glencoe, New York, 1962. |
[18] | M. Kim, D. A. McFarland, J. Leskovec, Modeling affinity based popularity dynamics, in Proc. ACM Conf. Info. Knowl. Manag., (2017), 477–486. https://doi.org/10.1145/3132847.3132923 |
[19] | S. Goel, D. Watts, D. Goldstein, The structure of online diffusion networks, in Proc. 13th ACM Conf. Electr. Comm., (2012), 623–638. https://doi.org/10.1145/2229012.2229058 |
[20] | J. Leskovec, A. Singh, J. Kleinberg, Patterns of influence in a recommendation network, in Proc. Pacific-Asia Conf. Knowl. Disc. Data Mining, (2006), 380–389. https://doi.org/10.1007/11731139_44 |
[21] |
R. Milo, S. Itzkovitz, N. Kashtan, R. Levitt, S. Shen-Orr, I. Ayzenshtat, et al., Superfamilies of evolved and designed networks, Science, 303 (2004), 1538–1542. https://doi.org/10.1126/science.1089167 doi: 10.1126/science.1089167
![]() |
[22] |
C. M. Schneider, V. Belik, T. Couronné, Z. Smoreda, M. C. González, Unravelling daily human mobility motifs, J. Roy. Soc. J. Royal Soc. Interf., 10 (2013), 20130246. https://doi.org/10.1098/rsif.2013.0246 doi: 10.1098/rsif.2013.0246
![]() |
[23] |
F. M. Bass, Comments on "a new product growth for model consumer durables: the Bass model", Manag. Sci., 50 (2004), 1833–1840. https://doi.org/10.1287/mnsc.1040.0300 doi: 10.1287/mnsc.1040.0300
![]() |
[24] | M. E. Newman, Networks: An Introduction, Oxford University Press, 2010. https://doi.org/10.1093/acprof:oso/9780199206650.001.0001 |
[25] | V. Kumar, T. V. Krishnan, Multinational diffusion models: An alternative framework, Mktg. Sci., 21 (2002), 318–330. |
[26] |
W. Putsis Jr, S. Balasubramanian, E. Kaplan, S. Sen, Mixing behavior in cross-country diffusion, Mktg. Sci., 16 (1997), 354–369. https://doi.org/10.1287/mksc.16.4.354 doi: 10.1287/mksc.16.4.354
![]() |
[27] |
M. Kuperman, G. Abramson, Small world effect in an epidemiological model, Phys. Rev. Lett., 86 (2001), 2909–2912. https://doi.org/10.1103/PhysRevLett.86.2909 doi: 10.1103/PhysRevLett.86.2909
![]() |
[28] | M. Luu, E. Lim, T. Hoang, F. Chua, Modeling diffusion in social networks using network properties, in Proc. 6th Int'l AAAI Conf. Weblogs Soc. Media (2012), 218–225. |
[29] | M. Schilling, C. Phelps, Interfirm collaboration networks: The impact of large-scale network structure on firm innovation, Manag. Sci., 53 (2007), 1113–1126. |
[30] | D. J. Daley, D. Vere-Jones, An Introduction to the Theory of Point Processes – Volume II: General Theory and Structure, Springer, 2008. |
[31] |
A. G. Hawkes, Spectra of some self-exciting and mutually exciting point processes, Biometrika, 58 (1971), 83–90. https://doi.org/10.2307/2334319 doi: 10.2307/2334319
![]() |
[32] | J. Møller, J. G. Rasmussen, Perfect simulation of hawkes processes, Adv. Appl. Probab., 37 (2005), 629–646. |
[33] | E. Cinlar, Introduction to Stochastic Processes, Courier Corporation, 2013. |
[34] | T. M. Cover, J. A. Thomas, Elements of Information Theory, 2nd Edition, John Wiley & Sons, 2012. |
[35] | R. Ghosh, T. Surachawala, K. Lerman, Entropy-based classification of 'retweeting' activity on Twitter, arXiv: 1106.0346 (2011). |
[36] |
C. Song, Z. Qu, N. Blumm, A.-L. Barabási, Limits of predictability in human mobility, Science, 327 (2010), 1018–1021. https://doi.org/10.1126/science.1177170 doi: 10.1126/science.1177170
![]() |
[37] |
R. Sinatra, M. Szell, Entropy and the predictability of online life, Entropy, 16 (2014), 543–556. https://doi.org/10.3390/e16010543 doi: 10.3390/e16010543
![]() |
[38] | C. Wang, B. A. Huberman, How random are online social interactions?, Sci. Rep., 2 (2012). https://doi.org/10.1038/srep00633 |
[39] | G. Ver Steeg, A. Galstyan, Information transfer in social media, in Proc. 21st Int'l Conf. World Wide Web, (2012), 509–518. https://doi.org/10.1145/2187836.2187906 |
[40] | G. Ver Steeg, A. Galstyan, Information-theoretic measures of influence based on content dynamics, in Proc. 6th ACM Int'l Conf. Web Search Web Data Mining (2013), 3–12. https://doi.org/10.1145/2433396.2433400 |
[41] |
M. Kim, D. Newth, P. Christen, Macro-level information transfer in social media: Reflections of crowd phenomena, Neurocomputing, 172 (2016), 84–99. https://doi.org/10.1016/j.neucom.2014.12.107 doi: 10.1016/j.neucom.2014.12.107
![]() |
[42] |
T. Schreiber, Measuring information transfer, Phys. Rev. Lett. 85 (2000), 461–464. https://doi.org/10.1103/PhysRevLett.85.461 doi: 10.1103/PhysRevLett.85.461
![]() |
[43] | D. J. MacKay, Information Theory, Inference, and Learning Algorithms, Cambridge University Press, 2003. |
[44] |
J. A. Hartigan, M. A. Wong, Algorithm AS 136: A k-means clustering algorithm, J. Roy. Stat. Soc. Ser. C (Appl. Stat.), 28 (1979), 100–108. https://doi.org/10.2307/2346830 doi: 10.2307/2346830
![]() |