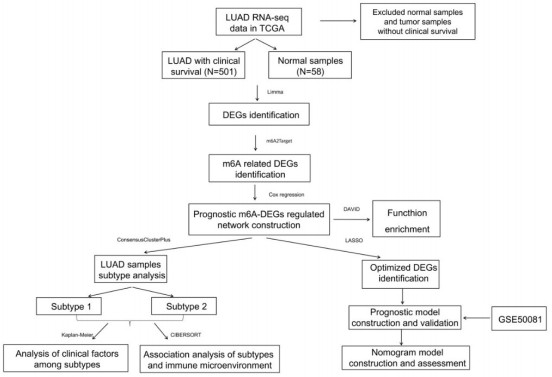
Purpose: Lung adenocarcinoma (LUAD) is a highly lethal subtype of primary lung cancer with a poor prognosis. N6-methyladenosine (m6A), the most predominant form of RNA modification, regulates biological processes and has critical prognostic implications for LUAD. Our study aimed to mine potential target genes of m6A regulators to explore their biological significance in subtyping LUAD and predicting survival. Methods: Using gene expression data from TCGA database, candidate target genes of m6A were predicted from differentially expressed genes (DEGs) in tumor based on M6A2 Target database. The survival-related target DEGs identified by Cox-regression analysis was used for consensus clustering analysis to subtype LUAD. Uni-and multi-variable Cox regression analysis and LASSO Cox-PH regression analysis were used to select the optimal prognostic genes for constructing prognostic score (PS) model. Nomogram encompassing PS score and independent prognostic factors was built to predict 3-year and 5-year survival probability. Results: We obtained 2429 DEGs in tumor tissue, within which, 1267 were predicted to m6A target genes. A prognostic m6A-DEGs network of 224 survival-related target DEGs was established. We classified LUAD into 2 subtypes, which were significantly different in OS time, clinicopathological characteristics, and fractions of 12 immune cell types. A PS model of five genes (C1QTNF6, THSD1, GRIK2, E2F7 and SLCO1B3) successfully split the training set or an independent GEO dataset into two subgroups with significantly different OS time (p < 0.001, AUC = 0.723; p = 0.017, AUC = 0.705).A nomogram model combining PS status, pathologic stage, and recurrence was built, showing good performance in predicting 3-year and 5-year survival probability (C-index = 0.708, 0.723, p-value = 0). Conclusion: Using candidate m6A target genes, we obtained two molecular subtypes and designed a reliable five-gene PS score model for survival prediction in LUAD.
Citation: Xiao Chu, Weiqing Wang, Zhaoyun Sun, Feichao Bao, Liang Feng. An N6-methyladenosine and target genes-based study on subtypes and prognosis of lung adenocarcinoma[J]. Mathematical Biosciences and Engineering, 2022, 19(1): 253-270. doi: 10.3934/mbe.2022013
[1] | Honglei Wang, Wenliang Zeng, Xiaoling Huang, Zhaoyang Liu, Yanjing Sun, Lin Zhang . MTTLm6A: A multi-task transfer learning approach for base-resolution mRNA m6A site prediction based on an improved transformer. Mathematical Biosciences and Engineering, 2024, 21(1): 272-299. doi: 10.3934/mbe.2024013 |
[2] | Shanzheng Wang, Xinhui Xie, Chao Li, Jun Jia, Changhong Chen . Integrative network analysis of N6 methylation-related genes reveal potential therapeutic targets for spinal cord injury. Mathematical Biosciences and Engineering, 2021, 18(6): 8174-8187. doi: 10.3934/mbe.2021405 |
[3] | Pingping Sun, Yongbing Chen, Bo Liu, Yanxin Gao, Ye Han, Fei He, Jinchao Ji . DeepMRMP: A new predictor for multiple types of RNA modification sites using deep learning. Mathematical Biosciences and Engineering, 2019, 16(6): 6231-6241. doi: 10.3934/mbe.2019310 |
[4] | Yong Zhu, Zhipeng Jiang, Xiaohui Mo, Bo Zhang, Abdullah Al-Dhelaan, Fahad Al-Dhelaan . A study on the design methodology of TAC3 for edge computing. Mathematical Biosciences and Engineering, 2020, 17(5): 4406-4421. doi: 10.3934/mbe.2020243 |
[5] | Atefeh Afsar, Filipe Martins, Bruno M. P. M. Oliveira, Alberto A. Pinto . A fit of CD4+ T cell immune response to an infection by lymphocytic choriomeningitis virus. Mathematical Biosciences and Engineering, 2019, 16(6): 7009-7021. doi: 10.3934/mbe.2019352 |
[6] | Tamás Tekeli, Attila Dénes, Gergely Röst . Adaptive group testing in a compartmental model of COVID-19*. Mathematical Biosciences and Engineering, 2022, 19(11): 11018-11033. doi: 10.3934/mbe.2022513 |
[7] | Wenli Cheng, Jiajia Jiao . An adversarially consensus model of augmented unlabeled data for cardiac image segmentation (CAU+). Mathematical Biosciences and Engineering, 2023, 20(8): 13521-13541. doi: 10.3934/mbe.2023603 |
[8] | Tongmeng Jiang, Pan Jin, Guoxiu Huang, Shi-Cheng Li . The function of guanylate binding protein 3 (GBP3) in human cancers by pan-cancer bioinformatics. Mathematical Biosciences and Engineering, 2023, 20(5): 9511-9529. doi: 10.3934/mbe.2023418 |
[9] | Xin Yu, Jun Liu, Ruiwen Xie, Mengling Chang, Bichun Xu, Yangqing Zhu, Yuancai Xie, Shengli Yang . Construction of a prognostic model for lung squamous cell carcinoma based on seven N6-methylandenosine-related autophagy genes. Mathematical Biosciences and Engineering, 2021, 18(5): 6709-6723. doi: 10.3934/mbe.2021333 |
[10] | Tahir Rasheed, Faran Nabeel, Muhammad Bilal, Yuping Zhao, Muhammad Adeel, Hafiz. M. N. Iqbal . Aqueous monitoring of toxic mercury through a rhodamine-based fluorescent sensor. Mathematical Biosciences and Engineering, 2019, 16(4): 1861-1873. doi: 10.3934/mbe.2019090 |
Purpose: Lung adenocarcinoma (LUAD) is a highly lethal subtype of primary lung cancer with a poor prognosis. N6-methyladenosine (m6A), the most predominant form of RNA modification, regulates biological processes and has critical prognostic implications for LUAD. Our study aimed to mine potential target genes of m6A regulators to explore their biological significance in subtyping LUAD and predicting survival. Methods: Using gene expression data from TCGA database, candidate target genes of m6A were predicted from differentially expressed genes (DEGs) in tumor based on M6A2 Target database. The survival-related target DEGs identified by Cox-regression analysis was used for consensus clustering analysis to subtype LUAD. Uni-and multi-variable Cox regression analysis and LASSO Cox-PH regression analysis were used to select the optimal prognostic genes for constructing prognostic score (PS) model. Nomogram encompassing PS score and independent prognostic factors was built to predict 3-year and 5-year survival probability. Results: We obtained 2429 DEGs in tumor tissue, within which, 1267 were predicted to m6A target genes. A prognostic m6A-DEGs network of 224 survival-related target DEGs was established. We classified LUAD into 2 subtypes, which were significantly different in OS time, clinicopathological characteristics, and fractions of 12 immune cell types. A PS model of five genes (C1QTNF6, THSD1, GRIK2, E2F7 and SLCO1B3) successfully split the training set or an independent GEO dataset into two subgroups with significantly different OS time (p < 0.001, AUC = 0.723; p = 0.017, AUC = 0.705).A nomogram model combining PS status, pathologic stage, and recurrence was built, showing good performance in predicting 3-year and 5-year survival probability (C-index = 0.708, 0.723, p-value = 0). Conclusion: Using candidate m6A target genes, we obtained two molecular subtypes and designed a reliable five-gene PS score model for survival prediction in LUAD.
Lung adenocarcinoma (LUAD) is the most common histological subtype of lung primary lung malignancy, approximately accounting for 40% of all cases [1]. LUAD is a leading cause of cancer-related mortality worldwide, with overall survival (OS) time shorter than five years [2]. LUAD frequently involves disseminated metastasis and is highly resistant to conventional radiotherapies and chemotherapies [3]. LUAD patients have high recurrence rate after surgical excision [4]. Therefore, identification of prognostic biomarkers of LUAD is critical for improving long-term survival of LUAD patients and facilitates developing novel effective treatment strategies.
N6-methyladenosine (m6A) is the most abundant and prevalent modification in the regulation of splicing, stability, translocation, and translation of eukaryotic mRNAs [5]. The m6A regulators are primarily comprised of "writers" (methyltransferase), such as METTL3, RBM15/15B, "erasers" (demethylase), such as FTO and ALKBH5, and "readers" (m6A-binding proteins that targets the m6A site on mRNA), such as YTHDF1 and YTHDF2 [6]. The aberrantly expressed m6A regulators play a critical role in tumorigenesis [7]. Several pieces of evidences have revealed that m6A regulators have important prognostic implications in LUAD [8,9]. m6A writers, erasers, and readers could serve prognostic biomarkers in LUAD [10]. Moreover, a growing number of studies have developed useful risk scoring systems based on the m6A regulators to predict survival in LUAD [11-13]. However, these studies only characterize prognostic roles of m6A regulators in LUAD, their regulatory mechanisms including downstream target genes and relevant prognostic implications remain elusive.
Here we drew on gene expression data of LUAD from The Cancer Genome Atlas (TCGA) database to identify the potential target genes of m6A methylation regulators and investigate their associations with LUAD subtyping and prognosis prediction. We developed a prognostic score (PS) system based on prognostic target genes to predict survival of patients. This study provided illumulating insights into the biological roles of m6A methylation regulators in LUAD.
Microarray gene expression data of 501 tumor samples and 58 normal samples with corresponding clinical characteristics (Illumina HiSeq 2000 RNA Sequencing platform) were downloaded from TCGA database (https://gdc-portal.nci.nih.gov/) and used as training set.
We launched a search for validation datasets in the Gene Expression Omnibus (GEO) [14] at the National Center for Biotechnology Information (NCBI) (https://www.ncbi.nlm.nih.gov/geo/). The following criteria should be met: gene expression data; tumor tissue samples; histology type information available; all samples no less than 150 and eligible samples no less than 100; survival information available. GSE50081 [15] included 127 tumor samples with corresponding clinical information (GPL570 Affymetrix Human Genome U133 Plus 2.0 Array platform) and was used as the validation dataset.
Differentially expressed genes (DEGs) were screened between normal tissue samples and tumor tissue samples, with FDR < 0.05 and |log2FC| > 1 as the cutoff. Using expression data of top 50 up-regulated DEGs and top 50 down-regulated DEGs according to descending value of |log2FC|, two-way hierarchical clustering analysis was performed using pheatmap version 1.0.8 (https://cran.r-project.org/web/packages/pheatmap/index.html) in R3.6.1 based on centered pearson correlation.
In order to investigate the m6A-related regulatory mechanisms, target genes of m6A regulators were predicted based on M6A2 Target database [16] (http://m6a2target.canceromics.org/) and were then mapped to the identified DEGs. The overlapped genes were subjected to the uni-variate Cox-regression analysis. The significant survival-related target genes and m6A RNA methylation regulators were used to construct a prognostic m6A-DEGs network. The network was visualized and its topological characteristics was analyzed using Cytoscape software [17] (version 3.6.1, https://cytoscape.org/). Gene ontology (GO) function and Kyoto Encyclopedia of Genes and Genomes (KEGG) pathway enrichment analysis was performed for the genes in the network using the Database for Annotation, Visualization and Integrated Discovery (DAVID) tool (version 6.8. https://david.ncifcrf.gov/). The cutoff was set at p-value < 0.05 and the count of significantly enriched genes > 5.
Using gene expression data of the survival-related target genes, consensus clustering analysis was performed by ConsensusClusterPlus Version 1.54.0 to identify subtypes of LUAD. ConsensusClusterPlus implements the consensus clustering method in R that provides quantitative and visual stability evidence for estimating the number of unsupervised classes in a dataset [18]. The optimal clusters were determined according to cumulative distribution function. Overall survival (OS) time, clinical characteristics and fractions of tumor-infiltrating immune cells (TIICs) were compared between different subtypes. CIBERSORT [19] software (https://cibersort.stanford.edu/index.php) was used to estimate proportions of tumor-infiltrating immune cells.
To build a PS model for predicting prognosis in LUAD, firstly, multi-variable Cox regression analysis was performed to identify independent prognostic genes with log-rank p-value < 0.05 from the survival-related target genes. Least absolute shrinkage and selection operator (LASSO) Cox-PH regression model [20] was used to determine the optimal prognostic genes from the independent prognostic genes. Prognostic score (PS) was defined as the linear combination of logarithmically transformed gene expression levels of the optimal prognostic genes weighted by canonical discriminant function coefficients and was used to evaluate mortality risk of a patient. Using expression levels and regression coefficients of the optimal prognostic genes, PS score was calculated for every sample using the following formula:
where Coefgenes represents regression coefficient of a prognostic gene and Expgenes represents expression level of a prognostic gene.
According to median PS score, all samples of the training set or the validation set were separated into a high-risk group and a low-risk group.
Uni-variable and multi-variable Cox regression analysis was performed for all clinical characteristics and PS status. Nomogram is a graphical calculating tool for individualized risk estimation based on use of multiple variables and continuous variables continuously [21]. A nomogram model incorporating all independent prognostic factors was built to predict 3-year and 5-year survival probability. Calibration curves of the nomogram were plotted and concordance index (C-index) [22] was calculated to evaluate its predictive performance.
Survival analysis was performed to compare OS probabilities of different groups using Kaplan-Meier method and log-rank test with the threshold of p-value < 0.05. Receiver Operating Characteristic (ROC) curves were plotted to analyze accuracy of the PS system.
The bioinformatics analyses below were carried out using different packages of R software (version 3.6.1): limma [23] package (version 3.34.7) for differential expression analysis (https://bioconductor.org/packages/release/bioc/html/limma.html); pheatmap [24] package (version 1.0.8, https://cran.r-project.org/web/packages/pheatmap/index.html) for hierarchical clustering analysis; survival [25] package (version 2.41-1, http://bioconductor.org/packages/survivalr/) for uni- and multi- variable Cox regression analysis and survival analysis; ConsensusClusterPlus [18] package (version 1.54.0, http://www.bioconductor.org/packages/release/bioc/html/ConsensusClusterPlus.html) for consensus clustering analysis; lars package (version 1.2) for LASSO Cox-PH regression model; rms package [26] (version 5.1-2, https://cran.r-project.org/web/packages/rms/index.html) for nomogram. P-value < 0.05 suggested significance. Analytical flow chart of this study is shown in Figure 1.
A total of 2429 genes that met the criteria of FDR < 0.05 and |log2FC| > 1 were significantly differentially expressed between tumor samples (N = 501) and normal samples (N = 58, Figure 2A) in the training set. As shown in a heatmap (Figure 2B), expression patterns of top 50 up-regulated DEGs and top 50 down-regulated DEGs were obviously different between tumor samples and normal samples, indicating the DEGs are able to distinguish tumor and normal samples.
Total 1267 DEGs were predicted to be target genes of m6A RNA methylation regulators based on m6A2 Target database and 224 of these were significantly associated with survival in uni-variable Cox regression analysis. Consequently, 12 m6A RNA methylation regulators (writers: RBM15, RBM15B; erasers: FTO; readers: YTHDF1, YTHDF2, YTHDF3, YTHDC1, YTHDC2, HNRNPC, IGF2BP1, IGF2BP2, IGF2BP3) and the 224 survival-related target genes formed a prognostic m6A-DEGs network (Figure 3). Topological analysis showed that IGF2BP1 was the most hub gene with a degree of 145. The other nodes with high degree included YTHDF1, YTHDC1, IGF2BP2 and IGF2BP3 (Table 1). The genes in the network were significantly involved in 26 GO biological processes largely related to cell cycle, and 5 KEGG pathways, such as cell cycle and p53 signaling pathways (Table 2).
Symbol | Average Shortest Path Length | Betweenness Centrality | Closeness Centrality | Degree |
IGF2BP1 | 1.7167382 | 0.34083022 | 0.5825 | 145 |
YTHDF1 | 1.89699571 | 0.25043473 | 0.52714932 | 123 |
YTHDC1 | 1.97424893 | 0.27286644 | 0.50652174 | 114 |
IGF2BP2 | 2.07725322 | 0.15502202 | 0.48140496 | 103 |
IGF2BP3 | 2.19742489 | 0.10570453 | 0.45507813 | 90 |
RBM15B | 2.62660944 | 0.01980201 | 0.38071895 | 38 |
YTHDF2 | 2.72961373 | 0.0403311 | 0.3663522 | 26 |
YTHDF3 | 2.77253219 | 0.0139253 | 0.36068111 | 22 |
RBM15 | 2.83261803 | 0.00242151 | 0.3530303 | 14 |
HNRNPC | 2.87553648 | 0.00141667 | 0.34776119 | 11 |
CDCA5 | 1.97424893 | 0.00901307 | 0.50652174 | 9 |
HHIPL2 | 2.03433476 | 0.00668609 | 0.49156118 | 8 |
ASPM | 1.99141631 | 0.00712224 | 0.50215517 | 8 |
ZIC2 | 2 | 0.00689356 | 0.5 | 8 |
PLK1 | 1.99141631 | 0.00648788 | 0.50215517 | 7 |
CDK5R1 | 2 | 0.00649329 | 0.5 | 7 |
FAM83A | 2.43776824 | 0.00945876 | 0.41021127 | 7 |
ZIC5 | 2.04291845 | 0.00450393 | 0.4894958 | 7 |
HCN2 | 1.99141631 | 0.00648788 | 0.50215517 | 7 |
CENPF | 2.02575107 | 0.00463828 | 0.49364407 | 7 |
Category | Term | Count of genes | P-value | FDR |
GO Biology Process | Cell division | 39 | 2.10E-25 | 2.31E-22 |
Mitotic nuclear division | 34 | 5.76E-25 | 3.17E-22 | |
Sister chromatid cohesion | 21 | 7.80E-19 | 2.87E-16 | |
Mitotic sister chromatid segregation | 10 | 8.34E-12 | 2.30E-09 | |
Chromosome segregation | 13 | 3.00E-11 | 6.61E-09 | |
DNA replication | 17 | 6.75E-11 | 1.24E-08 | |
G2/M transition of mitotic cell cycle | 16 | 1.17E-10 | 1.84E-08 | |
G1/S transition of mitotic cell cycle | 13 | 3.88E-09 | 5.34E-07 | |
Mitotic cytokinesis | 8 | 4.38E-08 | 5.37E-06 | |
Mitotic metaphase plate congression | 8 | 2.66E-07 | 2.67E-05 | |
Regulation of cell cycle | 12 | 3.32E-07 | 3.05E-05 | |
Anaphase-promoting complex-dependent catabolic process | 10 | 4.77E-07 | 3.75E-05 | |
Spindle organization | 6 | 9.87E-07 | 7.25E-05 | |
Mitotic spindle assembly checkpoint | 6 | 3.37E-06 | 2.32E-04 | |
Mitotic spindle assembly | 7 | 4.29E-06 | 2.78E-04 | |
Microtubule-based movement | 9 | 6.23E-06 | 3.81E-04 | |
Mitotic cell cycle checkpoint | 6 | 3.89E-05 | 2.14E-03 | |
Cell proliferation | 16 | 4.26E-05 | 2.23E-03 | |
DNA repair | 12 | 1.45E-04 | 6.39E-03 | |
CENP-A containing nucleosome assembly | 6 | 1.67E-04 | 6.80E-03 | |
Cytokinesis | 6 | 2.82E-04 | 1.07E-02 | |
KEGG Pathway | Cell cycle | 20 | 2.67E-16 | 3.71E-14 |
Oocyte meiosis | 13 | 8.39E-09 | 5.83E-07 | |
Progesterone-mediated oocyte maturation | 10 | 1.02E-06 | 4.74E-05 | |
p53 signaling pathway | 7 | 1.63E-04 | 5.66E-03 | |
Fanconi anemia pathway | 4 | 2.74E-02 | 7.61E-01 | |
Note: Count of genes stand for the number of significantly enriched genes. GO, gene ontology; KEGG, Kyoto Encyclopedia of Genes and Genomes. |
Consensus clustering analysis for the 224 survival-related genes led to the identification of two LUAD subtypes (Figure 4A). In the training set, 222 tumor samples belonged to subtype 1, while 279 tumor samples fell into subtype 2. Kaplan-Meier survival curves in Figure 4B showed that subtype 2 had significantly shorter OS time compared to subtype 1 (p-value = 0.00096). Subtype 2 had less patients older than 60 years (p-value = 2.51E-03), less female patients (p-value = 1.15E-03), higher Pathologic N stage (p-value = 4.68E-03), higher Pathologic T stage (p-value = 2.85E-03), higher pathologic stage (p-value = 5.81E-04), and less never-smokers (p-value = 1.33E-02, Table 3) in comparison with subtype 1.
Characteristics | N of cases | Subtype | P-value | |
Subtype 1 (N = 222) | Subtype 2 (N = 279) | |||
Age(years) | ||||
≤ 60 | 157 | 54 | 103 | 2.51E-03 |
➢ 60 | 334 | 164 | 170 | |
Gender | ||||
Male | 231 | 84 | 147 | 1.15E-03 |
Female | 270 | 138 | 132 | |
Pathologic M | ||||
M0 | 333 | 149 | 184 | 8.63E-02 |
M1 | 24 | 6 | 18 | |
Pathologic N | ||||
N0 | 324 | 158 | 166 | 4.68E-03 |
N1 | 94 | 34 | 60 | |
N2 | 72 | 22 | 50 | |
Pathologic T | ||||
T1 | 167 | 93 | 74 | 2.85E-03 |
T2 | 267 | 100 | 167 | |
T3 | 45 | 19 | 26 | |
T4 | 19 | 8 | 11 | |
Pathologic stage | ||||
Stage I | 268 | 140 | 128 | 5.81E-04 |
Stage II | 119 | 42 | 77 | |
Stage III | 81 | 27 | 54 | |
Stage IV | 25 | 7 | 18 | |
Recurrence | ||||
Yes | 151 | 59 | 92 | 8.38E-02 |
No | 275 | 132 | 143 | |
Tobacco smoking history | ||||
Never | 28 | 17 | 11 | 1.33E-02 |
Reform | 127 | 67 | 60 | |
Current | 46 | 14 | 32 |
It has been reported that the m6A genes are important regulators in tumor microenvironment of LUAD and affect TIICs [7]. Therefore, we also analyzed composition of TIICs in the two subtypes. Remarkably, compared to subtype 1, subtype 2 had significantly less memory B cells, more CD8+ T cells, less resting CD4+ memory T cells, more activated CD4+ memory T cells, more Tfh cells, more activated NK cells, less monocytes, more M0 and M1 macrophages, less M2 macrophages, more resting myeloid dendritic cells, less activated mast cells, more resting mast cells, and more Neutrophils (p-value < 0.001, Figure 5).
Total 93 genes in the m6A-DEGs network were found to be independent prognostic factors in multi-variable Cox regression analysis. Five independent prognostic genes [C1QTNF6 (C1q/tumor necrosis factor-related protein 6), THSD1 (Thrombospondin type I domain 1), GRIK2 (Glutamate receptor, ionotropic, kainate 2), E2F7 (E2F transcription factor 7) and SLCO1B3 (solute carrier organic anion transporter family member 1B3)] achieved the optimal lambda value and were selected by LASSO Cox-PH model as the optimal gene panel for prognosis prediction (Table 4 and Figure 6). Kaplan-Meier survival curves in Figure 7 showed that for each of the five optimal prognostic genes, the high expression and low expression samples had significantly different OS time (p < 0.001, p = 0.0052, p = 0.00092, p = 0.00012, and p = 0.0036) in the training set.
Gene name | Hazard Ratio | 95% Confidence Interval | Standard error | Z score | P-value | LASSO Coefficient |
C1QTNF6 | 1.477 | 1.239-1.761 | 0.090 | 4.341 | 1.420E-05 | 0.02171 |
THSD1 | 0.683 | 0.488-0.958 | 0.172 | -2.210 | 2.711E-02 | -0.00642 |
GRIK2 | 1.491 | 1.083-2.052 | 0.163 | 2.451 | 1.423E-02 | 0.00173 |
E2F7 | 1.386 | 1.091-1.759 | 0.122 | 2.679 | 7.390E-03 | 0.00650 |
SLCO1B3 | 1.261 | 1.084-1.466 | 0.077 | 3.002 | 2.680E-03 | 0.00430 |
Based on expression levels and regression coefficients of the five optimal prognostic genes, PS score was calculated for every sample in the training set. With median PS score as the cutoff, the training set was split in a high-risk group and a low-risk group. The high-risk patients had significantly shorter OS time compared to the low-risk patients (p < 0.0001, Figure 8A). AUC for the training set was 0.723 (specificity = 0.560, sensitivity = 0.820, Figure 8B). Similarly, the validation set (GSE50081) was divided by the five-gene PS score into high-risk and low-risk groups with significantly different OS time (p = 0.017, Figure 8A). AUC for the validation set was 0.705 (specificity = 0.711, sensitivity = 0.627, Figure 8B).
In uni-variable Cox regression analysis, Pathologic M (p = 5.361E-03), Pathologic N (p = 3.993E-11), Pathologic T (p = 3.102E-06), Pathologic stage (p = 2.942E-14) and recurrence (p = 2.438E-07) were significantly related to OS time. Pathologic stage (p = 3.850E-02, HR (95% CI) = 1.919[1.035-3.559]), recurrence (p = 1.510E-05, HR (95% CI) = 2.496[1.649-3.778]) and PS status (p = 3.550E-03, HR (95% CI) = 1.584[1.032-2.433]) were further found to be independent prognostic factors in multi-variable Cox regression analysis (Figure 9A, Table 5). In order to improve predictive performance of the five-gene PS score and facilitate its application, we combined pathologic stage, recurrence and PS status to build a nomogram for predicting 3-year and 5-year survival probability (Figure 9B). Calibration plots for the nomogram showed good consistence between the predicted and actual 3-year and 5-year survival probabilities (C-index = 0.708, 0.723, p-value = 0, Figure 9C), suggesting good predictive accuracy of the composite nomogram based on pathologic stage, recurrence and PS status. All these results demonstrated that the five-gene PS score was a useful prognostic tool in LUAD.
Clinical characteristics | TCGA(N = 501) | Uni-variable cox | Multi-variable cox | ||
HR (95% CI) | P value | HR (95% CI) | P value | ||
Age(years, mean ± sd) | 65.28 ± 10.05 | 1.009[0.994-1.024] | 2.640E-01 | - | - |
Gender(Male/Female) | 231/270 | 1.060[0.792-1.418] | 6.954E-01 | - | - |
Pathologic M(M0/M1/-) | 333/24/144 | 2.111[1.232-3.616] | 5.361E-03 | 0.304[0.061-1.511] | 1.455E-01 |
Pathologic N(N0/N1/N2/-) | 324/94/72/11 | 1.782[1.493-2.128] | 3.993E-11 | 0.965[0.552-1.685] | 8.998E-01 |
Pathologic T(T1/T2/T3/T4/-) | 167/267/45/19/3 | 1.550[1.289-1.863] | 3.102E-06 | 1.221[0.823-1.531] | 4.672E-01 |
Pathologic stage(I / II / III / IV /-) | 268/119/81/25/8 | 1.679[1.463-1.928] | 2.942E-14 | 1.919[1.035-3.559] | 3.850E-02 |
Recurrence(Yes/No/-) | 151/275/75 | 2.392[1.700-3.367] | 2.438E-07 | 2.496[1.649-3.778] | 1.510E-05 |
Smoking history(Never/Reform/Current/-) | 28/127/46/300 | 0.765[0.542-1.081] | 1.287E-01 | - | - |
PS status(High/Low) | 250/251 | 2.201[1.625-2.981] | 1.805E-07 | 1.584[1.032-2.433] | 3.550E-03 |
Note: PS status stands for prognostic score status. |
m6A RNA methylation is a critical player in tumor initiation and progression through affecting gene expression and various cellular processes [27]. m6A genes have been increasingly acknowledged as potential prognostic biomarkers of LUAD [28]. However, candidate target genes of m6A modification regulators and their prognostic significance remain poorly studied. Our present study predicted 1267 DEGs in LUAD tumor as candidate target genes of m6A regulators based on m6A2 Target database. Using the 224 survival-related target genes, we obtained a prognostic m6A-DEGs network and two molecular subtypes (subtype 1 and 2) of LUAD with significantly different OS time, distinct clinical characteristics and composition of immune cells. Moreover, we developed and validated a robust five-gene PS system for survival prediction in LUAD. This study deepens our understanding on the regulatory mechanisms underlying the roles of m6A RNA methylation in LUAD, contributing to improvement of prognosis prediction and aiding in individualized genome-based therapy in LUAD.
LUAD has various histological subtypes ranged from pre-invasive lesion to aggressive adenocarcinoma [2]. Our study identified two molecular subtypes of LUAD (subtype 1 and 2) using consensus clustering analysis based on expression data of the survival-related target genes. Subtype 2 had poorer survival then subtype 1. Subtype 2 was prone to be at more advanced stage and behave more aggressively and invasively. These results suggest that these survival-related target genes are important determinants in LUAD progression in consistence with a previous report [29]. Besides, subtype 2 is more likely to be observed in young male smokers, while subtype 1 has a greater propensity to be found in older female never-smokers. TIICs have a close association with LUAD progression and prognosis [30]. Our study found that subtype 1 and 2 were significantly different in proportions of 14 types of immune cells. These findings together demonstrate diverse clinicopathological characteristics and varied composition of immune cells between the two subtypes. Identification of the two m6A methylation-related molecular subtypes may have important clinical implications and lend support to targeted therapies to combat LUAD.
The present study showed that our PS model was a reliable and robust tool for survival prediction in LUAD, manifested in several levels. Firstly, the PS score could discriminate high-risk patients from low-risk patients in the training set. Secondly, prognostic performance of our PS model was successfully verified in an independent dataset from GEO database, supporting the generalizability of the PS model. Thirdly, AUC values of ROC curves for the two sets were larger than 0.7, providing evidence for predictive accuracy of the PS model. Fourthly, PS status had been identified as an independent prognostic factor of LUAD. Finally, the nomogram model incorporating PS status, pathologic stage and recurrence performed well in predicting 3-year and 5-year survival probability of LUAD patients (C-index > 0.7).
Our PS model was developed based on five optimal prognostic genes (C1QTNF6, THSD1, GRIK2, E2F7 and SLCO1B3). According to the HR values, 4 genes (C1QTNF6, GRIK2, E2F7 and SLCO1B3) were associated with increased risk of LUAD, while the expression level of one gene (TNSD1) was associated with reduced risk of LUAD. C1QTNF6 overexpression is involved in cell proliferation, migration and invasion and may be an independent prognostic factor in LUAD [31,32]. Inhibition of C1QTNF6 could attenuate cell proliferation, migration, and invasion and promote apoptosis, indicating C1QTNF6 might be a new perspective in treating non-small-cell lung cancer [33]. GRIK2 belongs to an ionotropic glutamate receptor and is identified as a novel epigenetic target in gastric cancer [34]. Its high expression is related to a poor prognosis in urinary tract carcinoma [35]. E2F7 is an E2F transcription factor, which plays an essential role in regulation of cell cycle progression [36]. E2F7 is highly upregulated in non-small-cell lung cancer samples, and aberrantly allow the cells to enter into S phase of cell cycle [37]. High expression of E2F7 is associated with short OS time of LUAD patients [38]. SLCO1B3 is mainly expressed in the basement membrane of liver cells and plays important roles in transporting endogenous compounds into cells [39]. It has been recognized as a positive prognostic biomarker of breast cancer [40]. SLCO1B3 exerts an oncogenic effect through promoting epithelial-mesenchymal transition in progression of non-small cell lung cancer [41]. Nagai et al. showed that SLCO1B3 is expressed in lung cancer tissues but not in normal tissues [42]. Taken together, C1QTNF, GRIK2, E2F7 and SLCO1B3 are highly expressed in cancer samples, which are consistent with our results. THSD1 is a novel tumor suppressor gene mapping to 13q14. It is demonstrated to be downregulated in esophageal squamous cell carcinoma, which might be related with promoter hypermethylation [43]. In LUAD, THSD1 is found being aberrantly methylated and its expression is significantly correlates with prognosis of LUAD patients [44]. Our result found that TNSD1 was associated with reduced risk of LUAD, further suggesting its role as a tumor suppressor gene. Nevertheless, molecular mechanism and prognostic significance of GRIK2 in LUAD remains poorly understood. The molecular and cellular mechanisms of different caners share similarities, such as mutations in proto-oncogenes and tumor suppressors, exposures to chemicals and discordant regulation or activities of many critical signaling pathways [45]. The dysregulation or association with prognosis of the identified predictive genes in other neoplastic conditions might provide evidence of their role in LUAD carcinogenesis. However, downstream analysis on the roles of them in carcinogenesis broadly are still warranted to identify cross-talk and pleiotropic mechanisms as well as potential adjuvant therapies and repurposable drugs across different neoplastic conditions.
There are some limitations in our study. First, we have identified two subtypes of LUAD based on the expression level of 222 DEGs that were significantly related with survival. These subtypes might be clinically meaningful. However, sub-types need to be widely validated before they could be agreed upon as acceptable. Second, downstream analyses on the roles of the identified predictive genes are still warranted. Third, clinical information of some patients in the training set and validation set is lacking, which might lead to bias in Cox regression analysis.
Using predicted target genes of m6A regulators, we obtained two molecular subtypes of LUAD with differential survival time, distinct clinicopathological and immunological features. Based on five prognostic target genes, we developed and validated a PS model for risk in LUAD. This study contributes to better tumor classification and supplies a reference for individualized survival estimation and targeted treatment strategy for LUAD.
The study was funded by Talent Development Plan funded by Shanghai Fifth People's Hospital, Fudan University (Award Number 2020WYRCJY06).
The authors declare that they have no conflict of interest.
[1] |
B. C. Bade, C. S. D. Cruz, Lung cancer 2020: epidemiology, etiology, and prevention, Clin. Chest. Med., 41 (2020), 1-24. doi: 10.1016/j.ccm.2019.10.001. doi: 10.1016/j.ccm.2019.10.001
![]() |
[2] |
B. D. Hutchinson, G. S. Shroff, M. T. Truong, J. P. Ko, Spectrum of lung adenocarcinoma, Semin. Ultrasound CT MR, 40 (2019), 255-264. doi: 10.1053/j.sult.2018.11.009. doi: 10.1053/j.sult.2018.11.009
![]() |
[3] |
T. V. Denisenko, I. N. Budkevich, B. Zhivotovsky, Cell death-based treatment of lung adenocarcinoma, Cell Death Dis., 9 (2018), 117. doi: 10.1038/s41419-017-0063-y. doi: 10.1038/s41419-017-0063-y
![]() |
[4] |
F. R. Hirsch, G. V. Scagliotti, J. L. Mulshine, R. Kwon, W. J. C. Jr, Y. Wu, et al., Lung cancer: current therapies and new targeted treatments, Lancet, 389 (2017), 299-311. doi: 10.1016/S0140-6736(16)30958-8. doi: 10.1016/S0140-6736(16)30958-8
![]() |
[5] |
L. He, H. Li, A. Wu, Y. Peng, G. Shu, G. Yin, Functions of N6-methyladenosine and its role in cancer, Mol. Cancer, 18 (2019), 176. doi: 10.1186/s12943-019-1109-9. doi: 10.1186/s12943-019-1109-9
![]() |
[6] |
D. Dai, H. Wang, L. Zhu, H. Jin, X. Wang, N6-methyladenosine links RNA metabolism to cancer progression, Cell Death Dis., 9 (2018), 124.doi: 10.1038/s41419-017-0129-x. doi: 10.1038/s41419-017-0129-x
![]() |
[7] |
Y. Li, J. Gu, F. Xu, Q. Zhu, Y. Chen, D. Ge, et al., Molecular characterization, biological function, tumor microenvironment association and clinical significance of m6A regulators in lung adenocarcinoma, Brief Bioinform., 22 (2020), bbaa225. doi: 10.1093/bib/bbaa225. doi: 10.1093/bib/bbaa225
![]() |
[8] |
Y. Zhang, X. Liu, L. Liu, J. Li, Q. Hu, R. Sun, Expression and prognostic significance of m6A-related genes in lung adenocarcinoma, Med. Sci. Monit., 26 (2020), e919644.doi: 10.12659/MSM.919644. doi: 10.12659/MSM.919644
![]() |
[9] |
F. Li, H. Wang, H. Huang, L. Zhang, D. Wang, Y. Wan, m6A RNA methylation regulators participate in the malignant progression and have clinical prognostic value in lung adenocarcinoma, Front. Genet., 11 (2020), 994. doi: 10.3389/fgene.2020.00994. doi: 10.3389/fgene.2020.00994
![]() |
[10] |
H. Wang, X. Zhao, Z. Lu, m6A RNA methylation regulators act as potential prognostic biomarkers in lung adenocarcinoma, Front. Genet., 12 (2021), 622233. doi: 10.3389/fgene.2021.622233. doi: 10.3389/fgene.2021.622233
![]() |
[11] |
J. Zhu, M. Wang, D. Hu, Deciphering N6-methyladenosine-related genes signature to predict survival in lung adenocarcinoma, Biomed Res. Int., 2020 (2020), 2514230. doi: 10.1155/2020/2514230. doi: 10.1155/2020/2514230
![]() |
[12] |
C. Gao, J. Zhuang, H. Li, C. Liu, C. Zhou, L. Liu, et al., Gene signatures of 6-methyladenine regulators in women with lung adenocarcinoma and development of a risk scoring system: a retrospective study using the cancer genome atlas database, Aging (Albany NY), 13 (2021), 3957-3968. doi: 10.18632/aging.202364. doi: 10.18632/aging.202364
![]() |
[13] |
Y. Liu, X. Guo, M. Zhao, H. Ao, X. Leng, M. Liu, et al., Contributions and prognostic values of m6A RNA methylation regulators in non-small-cell lung cancer, J. Cell. Physiol., 235 (2020), 6043-6057. doi: 10.1002/jcp.29531. doi: 10.1002/jcp.29531
![]() |
[14] |
E. Clough, T. Barrett, The gene expression omnibus database, Methods Mol. Biol., 1418 (2016), 93-110. doi: 10.1007/978-1-4939-3578-9_5. doi: 10.1007/978-1-4939-3578-9_5
![]() |
[15] |
S. D. Der, J. Sykes, M. Pintilie, C. Q. Zhu, D. Strumpf, N. Liu, et al., Validation of a histology-independent prognostic gene signature for early-stage, non-small-cell lung cancer including stage IA patients, J. Thorac. Oncol., 9 (2014), 59-64. doi: 10.1097/jto.0000000000000042. doi: 10.1097/jto.0000000000000042
![]() |
[16] |
S. Deng, H. Zhang, K. Zhu, X. Li, Y. Ye, R. Li, et al., M6A2target: a comprehensive database for targets of m6A writers, erasers and readers, Brief. Bioinform., 22 (2020). doi: 10.1093/bib/bbaa055. doi: 10.1093/bib/bbaa055
![]() |
[17] |
M. Kohl, S. Wiese, B. Warscheid, Cytoscape: software for visualization and analysis of biological networks, Methods Mol. Biol. (Clifton, N. J.), 696 (2011), 291-303. doi: 10.3390/ijms18091880. doi: 10.3390/ijms18091880
![]() |
[18] |
M. D. Wilkerson, D. N. Hayes, ConsensusClusterPlus: a class discovery tool with confidence assessments and item tracking, Bioinformatics, 26 (2010), 1572-1573. doi: 10.1093/bioinformatics/btq170. doi: 10.1093/bioinformatics/btq170
![]() |
[19] |
B. Chen, M. S. Khodadoust, C. L. Liu, A. M. Newman, A. A. Alizadeh, Profiling tumor infiltrating immune cells with CIBERSORT, Methods Mol. Biol., 1711 (2018), 243-259. doi: 10.1007/978-1-4939-7493-1_12. doi: 10.1007/978-1-4939-7493-1_12
![]() |
[20] |
S. Huang, C. Yee, T. Ching, H. Yu, L. X. Garmire, A novel model to combine clinical and pathway-based transcriptomic information for the prognosis prediction of breast cancer, PLoS Comput. Biol., 10 (2014), e1003851. doi: 10.1371/journal.pcbi.1003851. doi: 10.1371/journal.pcbi.1003851
![]() |
[21] |
S. Wang, L. Yang, B. Ci, M. Maclean, D. E. Gerber, G. Xiao, Development and validation of a nomogram prognostic model for SCLC patients, J. Thorac. Oncol., 13 (2018), 1338-1348. doi: 10.1016/j.jtho.2018.05.037. doi: 10.1016/j.jtho.2018.05.037
![]() |
[22] |
E. Longato, M. Vettoretti, B. Di Camillo, A practical perspective on the concordance index for the evaluation and selection of prognostic time-to-event models, J. Biomed. Inform., 108 (2020), 103496. doi: 10.1016/j.jbi.2020.103496. doi: 10.1016/j.jbi.2020.103496
![]() |
[23] |
M. E. Ritchie, B. Phipson, D. Wu, Y. Hu, C. W. Law, W. Shi, Limma powers differential expression analyses for RNA-sequencing and microarray studies, Nucleic Acids Res., 43 (2015), e47-e47. doi: 10.1093/nar/gkv007. doi: 10.1093/nar/gkv007
![]() |
[24] |
L. Yang, L. Xu, Q. Wang, M. Wang, G. An, Dysregulation of long non-coding RNA profiles in human colorectal cancer and its association with overall survival, Oncol. Lett., 12 (2016), 4068-4074. doi: 10.3892/ol.2016.5138. doi: 10.3892/ol.2016.5138
![]() |
[25] |
P. Wang, Y. Wang, B. Hang, X. Zou, J. H. Mao, A novel gene expression-based prognostic scoring system to predict survival in gastric cancer, Oncotarget, 7 (2016), 55343-55351. doi: 10.18632/oncotarget.10533. doi: 10.18632/oncotarget.10533
![]() |
[26] |
Z. Zhang, M. W. Kattan, Drawing nomograms with R: applications to categorical outcome and survival data, Ann. Transl. Med., 5 (2017). doi: 10.21037/atm.2017.04.01. doi: 10.21037/atm.2017.04.01
![]() |
[27] |
Q. Lan, P. Y. Liu, J. Haase, J. L. Bell, S. Hüttelmaier, T. Liu, The critical role of RNA m6A methylation in cancer, Cancer Res., 79 (2019), 1285-1292. doi: 10.1158/0008-5472.CAN-18-2965. doi: 10.1158/0008-5472.CAN-18-2965
![]() |
[28] |
R. Xu, G. Pang, Q. Zhao, L. Yang, S. Chen, L. Jiang, The momentous role of N6-methyladenosine in lung cancer, J. Cell. Physiol., 236 (2021), 3244-3256. doi: 10.1002/jcp.30136. doi: 10.1002/jcp.30136
![]() |
[29] |
S. Ma, C. Chen, X. Ji, J. Liu, Q. Zhou, G. Wang, et al., The interplay between m6A RNA methylation and noncoding RNA in cancer, J. Hematol. Oncol., 12 (2019), 121. doi: 10.1186/s13045-019-0805-7. doi: 10.1186/s13045-019-0805-7
![]() |
[30] |
Q. Wang, M. Li, M. Yang, Y. Yang, F. Song, W. Zhang, et al., Analysis of immune-related signatures of lung adenocarcinoma identified two distinct subtypes: implications for immune checkpoint blockade therapy, Aging (Albany NY), 12 (2020), 3312-3339. doi: 10.18632/aging.102814. doi: 10.18632/aging.102814
![]() |
[31] |
M. Han, B. Wang, M. Zhu, Y. Zhang, C1QTNF6 as a novel biomarker regulates cellular behaviors in A549 cells and exacerbates the outcome of lung adenocarcinoma patients, in vitro Cell. Dev. Biol. Animal, 55 (2019), 614-621. doi: 10.1007/s11626-019-00377-w. doi: 10.1007/s11626-019-00377-w
![]() |
[32] |
W. Zhang, Y. Shen, G. Feng, Predicting the survival of patients with lung adenocarcinoma using a four-gene prognosis risk model, Oncol. Lett., 18 (2019), 535-544. doi: 10.3892/ol.2019.10366. doi: 10.3892/ol.2019.10366
![]() |
[33] |
W. Zhang, G. Feng, C1QTNF6 regulates cell proliferation and apoptosis of NSCLC in vitro and in vivo, Biosci. Rep., 41 (2021). doi: 10.1042/BSR20201541. doi: 10.1042/BSR20201541
![]() |
[34] |
C. S. Wu, Y. J. Lu, H. P. Li, C. Hsueh, C. Y. Lu, Y. Leu, et al., Glutamate receptor, ionotropic, kainate 2 silencing by DNA hypermethylation possesses tumor suppressor function in gastric cancer, Int. J. Cancer, 126 (2010), 2542-2552. doi: 10.1002/ijc.24958. doi: 10.1002/ijc.24958
![]() |
[35] |
R. Inoue, Y. Hirohashi, H. Kitamura, S. Nishida, A. Murai, A. Takaya, GRIK2 has a role in the maintenance of urothelial carcinoma stem-like cells, and its expression is associated with poorer prognosis, Oncotarget, 8 (2017), 28826-28839. doi: 10.18632/oncotarget.16259. doi: 10.18632/oncotarget.16259
![]() |
[36] |
L. Di Stefano, M. R. Jensen, K. Helin, E2F7, a novel E2F featuring DP-independent repression of a subset of E2F-regulated genes, Embo J., 22 (2003), 6289-6298. doi: 10.1093/emboj/cdg613. doi: 10.1093/emboj/cdg613
![]() |
[37] |
N. S. Moon, N. Dyson, E2F7 and E2F8 keep the E2F family in balance, Dev. Cell., 14 (2008), 1-3. doi: 10.1016/j.devcel.2007.12.017. doi: 10.1016/j.devcel.2007.12.017
![]() |
[38] |
X. Wu, Z. Sui, H. Zhang, Y. Wang, Z. Yu, Integrated analysis of lncRNA-mediated ceRNA network in lung adenocarcinoma, Front. Oncol., 10 (2020), 554759. doi: 10.3389/fonc.2020.554759. doi: 10.3389/fonc.2020.554759
![]() |
[39] |
B. Hagenbuch, P. J. Meier, The superfamily of organic anion transporting polypeptides, Biochim. Bioph. Acta, 1609 (2003), 1-18. doi: 10.1016/s0005-2736(02)00633-8. doi: 10.1016/s0005-2736(02)00633-8
![]() |
[40] |
T. Tang, G. Wang, S. Liu, Z, Zhang, C. Liu, F. Li, et al., Highly expressed SLCO1B3 inhibits the occurrence and development of breast cancer and can be used as a clinical indicator of prognosis, Sci. Rep., 11 (2021), 631. doi: 10.1038/s41598-020-80152-0. doi: 10.1038/s41598-020-80152-0
![]() |
[41] |
H. Hase, M. Aoki, K. Matsumoto, S. Nakai, T. Nagata, A. Takeda, et al., Cancer type-SLCO1B3 promotes epithelial-mesenchymal transition resulting in the tumour progression of non-small cell lung cancer, Oncol. Rep., 45 (2021), 309-316. doi: 10.3892/or.2020.7839. doi: 10.3892/or.2020.7839
![]() |
[42] |
M. Nagai, T. Furihata, S. Matsumoto, S. Ishii, S. Motohashi, I. Yoshino, et al., Identification of a new organic anion transporting polypeptide 1B3 mRNA isoform primarily expressed in human cancerous tissues and cells, Biochem. Bioph. Res. Commun., 418 (2012), 818-823. doi: 10.1016/j.bbrc.2012.01.115. doi: 10.1016/j.bbrc.2012.01.115
![]() |
[43] |
J. M. Ko, P. L. Chan, W. L. Yau, H. K. Chan, K. C. Chan, Z. Y. Yu, et al., Monochromosome transfer and microarray analysis identify a critical tumor-suppressive region mapping to chromosome 13q14 and THSD1 in esophageal carcinoma, Mol. Cancer Res., 6 (2008), 592-603. doi: 10.1158/1541-7786.MCR-07-0154. doi: 10.1158/1541-7786.MCR-07-0154
![]() |
[44] |
W. He, D. Ju, Z. Jie, A. Zhang, X. Xing, Q. Yang, Aberrant CpG-methylation affects genes expression predicting survival in lung adenocarcinoma, Cancer Med., 7 (2018), 5716-5726. doi: 10.1002/cam4.1834. doi: 10.1002/cam4.1834
![]() |
[45] |
A. D. Patterson, F. J. Gonzalez, G. H. Perdew, J. M. Peters, Molecular regulation of carcinogenesis: friend and foe, Toxicol. Sci., 165 (2018), 277-283. doi: 10.1093/toxsci/kfy185. doi: 10.1093/toxsci/kfy185
![]() |
1. | Muhammad Zubair Mehboob, Arslan Hamid, Jeevotham Senthil Kumar, Xia Lei, Comprehensive characterization of pathogenic missense CTRP6 variants and their association with cancer, 2025, 25, 1471-2407, 10.1186/s12885-025-13685-0 |
Symbol | Average Shortest Path Length | Betweenness Centrality | Closeness Centrality | Degree |
IGF2BP1 | 1.7167382 | 0.34083022 | 0.5825 | 145 |
YTHDF1 | 1.89699571 | 0.25043473 | 0.52714932 | 123 |
YTHDC1 | 1.97424893 | 0.27286644 | 0.50652174 | 114 |
IGF2BP2 | 2.07725322 | 0.15502202 | 0.48140496 | 103 |
IGF2BP3 | 2.19742489 | 0.10570453 | 0.45507813 | 90 |
RBM15B | 2.62660944 | 0.01980201 | 0.38071895 | 38 |
YTHDF2 | 2.72961373 | 0.0403311 | 0.3663522 | 26 |
YTHDF3 | 2.77253219 | 0.0139253 | 0.36068111 | 22 |
RBM15 | 2.83261803 | 0.00242151 | 0.3530303 | 14 |
HNRNPC | 2.87553648 | 0.00141667 | 0.34776119 | 11 |
CDCA5 | 1.97424893 | 0.00901307 | 0.50652174 | 9 |
HHIPL2 | 2.03433476 | 0.00668609 | 0.49156118 | 8 |
ASPM | 1.99141631 | 0.00712224 | 0.50215517 | 8 |
ZIC2 | 2 | 0.00689356 | 0.5 | 8 |
PLK1 | 1.99141631 | 0.00648788 | 0.50215517 | 7 |
CDK5R1 | 2 | 0.00649329 | 0.5 | 7 |
FAM83A | 2.43776824 | 0.00945876 | 0.41021127 | 7 |
ZIC5 | 2.04291845 | 0.00450393 | 0.4894958 | 7 |
HCN2 | 1.99141631 | 0.00648788 | 0.50215517 | 7 |
CENPF | 2.02575107 | 0.00463828 | 0.49364407 | 7 |
Category | Term | Count of genes | P-value | FDR |
GO Biology Process | Cell division | 39 | 2.10E-25 | 2.31E-22 |
Mitotic nuclear division | 34 | 5.76E-25 | 3.17E-22 | |
Sister chromatid cohesion | 21 | 7.80E-19 | 2.87E-16 | |
Mitotic sister chromatid segregation | 10 | 8.34E-12 | 2.30E-09 | |
Chromosome segregation | 13 | 3.00E-11 | 6.61E-09 | |
DNA replication | 17 | 6.75E-11 | 1.24E-08 | |
G2/M transition of mitotic cell cycle | 16 | 1.17E-10 | 1.84E-08 | |
G1/S transition of mitotic cell cycle | 13 | 3.88E-09 | 5.34E-07 | |
Mitotic cytokinesis | 8 | 4.38E-08 | 5.37E-06 | |
Mitotic metaphase plate congression | 8 | 2.66E-07 | 2.67E-05 | |
Regulation of cell cycle | 12 | 3.32E-07 | 3.05E-05 | |
Anaphase-promoting complex-dependent catabolic process | 10 | 4.77E-07 | 3.75E-05 | |
Spindle organization | 6 | 9.87E-07 | 7.25E-05 | |
Mitotic spindle assembly checkpoint | 6 | 3.37E-06 | 2.32E-04 | |
Mitotic spindle assembly | 7 | 4.29E-06 | 2.78E-04 | |
Microtubule-based movement | 9 | 6.23E-06 | 3.81E-04 | |
Mitotic cell cycle checkpoint | 6 | 3.89E-05 | 2.14E-03 | |
Cell proliferation | 16 | 4.26E-05 | 2.23E-03 | |
DNA repair | 12 | 1.45E-04 | 6.39E-03 | |
CENP-A containing nucleosome assembly | 6 | 1.67E-04 | 6.80E-03 | |
Cytokinesis | 6 | 2.82E-04 | 1.07E-02 | |
KEGG Pathway | Cell cycle | 20 | 2.67E-16 | 3.71E-14 |
Oocyte meiosis | 13 | 8.39E-09 | 5.83E-07 | |
Progesterone-mediated oocyte maturation | 10 | 1.02E-06 | 4.74E-05 | |
p53 signaling pathway | 7 | 1.63E-04 | 5.66E-03 | |
Fanconi anemia pathway | 4 | 2.74E-02 | 7.61E-01 | |
Note: Count of genes stand for the number of significantly enriched genes. GO, gene ontology; KEGG, Kyoto Encyclopedia of Genes and Genomes. |
Characteristics | N of cases | Subtype | P-value | |
Subtype 1 (N = 222) | Subtype 2 (N = 279) | |||
Age(years) | ||||
≤ 60 | 157 | 54 | 103 | 2.51E-03 |
➢ 60 | 334 | 164 | 170 | |
Gender | ||||
Male | 231 | 84 | 147 | 1.15E-03 |
Female | 270 | 138 | 132 | |
Pathologic M | ||||
M0 | 333 | 149 | 184 | 8.63E-02 |
M1 | 24 | 6 | 18 | |
Pathologic N | ||||
N0 | 324 | 158 | 166 | 4.68E-03 |
N1 | 94 | 34 | 60 | |
N2 | 72 | 22 | 50 | |
Pathologic T | ||||
T1 | 167 | 93 | 74 | 2.85E-03 |
T2 | 267 | 100 | 167 | |
T3 | 45 | 19 | 26 | |
T4 | 19 | 8 | 11 | |
Pathologic stage | ||||
Stage I | 268 | 140 | 128 | 5.81E-04 |
Stage II | 119 | 42 | 77 | |
Stage III | 81 | 27 | 54 | |
Stage IV | 25 | 7 | 18 | |
Recurrence | ||||
Yes | 151 | 59 | 92 | 8.38E-02 |
No | 275 | 132 | 143 | |
Tobacco smoking history | ||||
Never | 28 | 17 | 11 | 1.33E-02 |
Reform | 127 | 67 | 60 | |
Current | 46 | 14 | 32 |
Gene name | Hazard Ratio | 95% Confidence Interval | Standard error | Z score | P-value | LASSO Coefficient |
C1QTNF6 | 1.477 | 1.239-1.761 | 0.090 | 4.341 | 1.420E-05 | 0.02171 |
THSD1 | 0.683 | 0.488-0.958 | 0.172 | -2.210 | 2.711E-02 | -0.00642 |
GRIK2 | 1.491 | 1.083-2.052 | 0.163 | 2.451 | 1.423E-02 | 0.00173 |
E2F7 | 1.386 | 1.091-1.759 | 0.122 | 2.679 | 7.390E-03 | 0.00650 |
SLCO1B3 | 1.261 | 1.084-1.466 | 0.077 | 3.002 | 2.680E-03 | 0.00430 |
Clinical characteristics | TCGA(N = 501) | Uni-variable cox | Multi-variable cox | ||
HR (95% CI) | P value | HR (95% CI) | P value | ||
Age(years, mean ± sd) | 65.28 ± 10.05 | 1.009[0.994-1.024] | 2.640E-01 | - | - |
Gender(Male/Female) | 231/270 | 1.060[0.792-1.418] | 6.954E-01 | - | - |
Pathologic M(M0/M1/-) | 333/24/144 | 2.111[1.232-3.616] | 5.361E-03 | 0.304[0.061-1.511] | 1.455E-01 |
Pathologic N(N0/N1/N2/-) | 324/94/72/11 | 1.782[1.493-2.128] | 3.993E-11 | 0.965[0.552-1.685] | 8.998E-01 |
Pathologic T(T1/T2/T3/T4/-) | 167/267/45/19/3 | 1.550[1.289-1.863] | 3.102E-06 | 1.221[0.823-1.531] | 4.672E-01 |
Pathologic stage(I / II / III / IV /-) | 268/119/81/25/8 | 1.679[1.463-1.928] | 2.942E-14 | 1.919[1.035-3.559] | 3.850E-02 |
Recurrence(Yes/No/-) | 151/275/75 | 2.392[1.700-3.367] | 2.438E-07 | 2.496[1.649-3.778] | 1.510E-05 |
Smoking history(Never/Reform/Current/-) | 28/127/46/300 | 0.765[0.542-1.081] | 1.287E-01 | - | - |
PS status(High/Low) | 250/251 | 2.201[1.625-2.981] | 1.805E-07 | 1.584[1.032-2.433] | 3.550E-03 |
Note: PS status stands for prognostic score status. |
Symbol | Average Shortest Path Length | Betweenness Centrality | Closeness Centrality | Degree |
IGF2BP1 | 1.7167382 | 0.34083022 | 0.5825 | 145 |
YTHDF1 | 1.89699571 | 0.25043473 | 0.52714932 | 123 |
YTHDC1 | 1.97424893 | 0.27286644 | 0.50652174 | 114 |
IGF2BP2 | 2.07725322 | 0.15502202 | 0.48140496 | 103 |
IGF2BP3 | 2.19742489 | 0.10570453 | 0.45507813 | 90 |
RBM15B | 2.62660944 | 0.01980201 | 0.38071895 | 38 |
YTHDF2 | 2.72961373 | 0.0403311 | 0.3663522 | 26 |
YTHDF3 | 2.77253219 | 0.0139253 | 0.36068111 | 22 |
RBM15 | 2.83261803 | 0.00242151 | 0.3530303 | 14 |
HNRNPC | 2.87553648 | 0.00141667 | 0.34776119 | 11 |
CDCA5 | 1.97424893 | 0.00901307 | 0.50652174 | 9 |
HHIPL2 | 2.03433476 | 0.00668609 | 0.49156118 | 8 |
ASPM | 1.99141631 | 0.00712224 | 0.50215517 | 8 |
ZIC2 | 2 | 0.00689356 | 0.5 | 8 |
PLK1 | 1.99141631 | 0.00648788 | 0.50215517 | 7 |
CDK5R1 | 2 | 0.00649329 | 0.5 | 7 |
FAM83A | 2.43776824 | 0.00945876 | 0.41021127 | 7 |
ZIC5 | 2.04291845 | 0.00450393 | 0.4894958 | 7 |
HCN2 | 1.99141631 | 0.00648788 | 0.50215517 | 7 |
CENPF | 2.02575107 | 0.00463828 | 0.49364407 | 7 |
Category | Term | Count of genes | P-value | FDR |
GO Biology Process | Cell division | 39 | 2.10E-25 | 2.31E-22 |
Mitotic nuclear division | 34 | 5.76E-25 | 3.17E-22 | |
Sister chromatid cohesion | 21 | 7.80E-19 | 2.87E-16 | |
Mitotic sister chromatid segregation | 10 | 8.34E-12 | 2.30E-09 | |
Chromosome segregation | 13 | 3.00E-11 | 6.61E-09 | |
DNA replication | 17 | 6.75E-11 | 1.24E-08 | |
G2/M transition of mitotic cell cycle | 16 | 1.17E-10 | 1.84E-08 | |
G1/S transition of mitotic cell cycle | 13 | 3.88E-09 | 5.34E-07 | |
Mitotic cytokinesis | 8 | 4.38E-08 | 5.37E-06 | |
Mitotic metaphase plate congression | 8 | 2.66E-07 | 2.67E-05 | |
Regulation of cell cycle | 12 | 3.32E-07 | 3.05E-05 | |
Anaphase-promoting complex-dependent catabolic process | 10 | 4.77E-07 | 3.75E-05 | |
Spindle organization | 6 | 9.87E-07 | 7.25E-05 | |
Mitotic spindle assembly checkpoint | 6 | 3.37E-06 | 2.32E-04 | |
Mitotic spindle assembly | 7 | 4.29E-06 | 2.78E-04 | |
Microtubule-based movement | 9 | 6.23E-06 | 3.81E-04 | |
Mitotic cell cycle checkpoint | 6 | 3.89E-05 | 2.14E-03 | |
Cell proliferation | 16 | 4.26E-05 | 2.23E-03 | |
DNA repair | 12 | 1.45E-04 | 6.39E-03 | |
CENP-A containing nucleosome assembly | 6 | 1.67E-04 | 6.80E-03 | |
Cytokinesis | 6 | 2.82E-04 | 1.07E-02 | |
KEGG Pathway | Cell cycle | 20 | 2.67E-16 | 3.71E-14 |
Oocyte meiosis | 13 | 8.39E-09 | 5.83E-07 | |
Progesterone-mediated oocyte maturation | 10 | 1.02E-06 | 4.74E-05 | |
p53 signaling pathway | 7 | 1.63E-04 | 5.66E-03 | |
Fanconi anemia pathway | 4 | 2.74E-02 | 7.61E-01 | |
Note: Count of genes stand for the number of significantly enriched genes. GO, gene ontology; KEGG, Kyoto Encyclopedia of Genes and Genomes. |
Characteristics | N of cases | Subtype | P-value | |
Subtype 1 (N = 222) | Subtype 2 (N = 279) | |||
Age(years) | ||||
≤ 60 | 157 | 54 | 103 | 2.51E-03 |
➢ 60 | 334 | 164 | 170 | |
Gender | ||||
Male | 231 | 84 | 147 | 1.15E-03 |
Female | 270 | 138 | 132 | |
Pathologic M | ||||
M0 | 333 | 149 | 184 | 8.63E-02 |
M1 | 24 | 6 | 18 | |
Pathologic N | ||||
N0 | 324 | 158 | 166 | 4.68E-03 |
N1 | 94 | 34 | 60 | |
N2 | 72 | 22 | 50 | |
Pathologic T | ||||
T1 | 167 | 93 | 74 | 2.85E-03 |
T2 | 267 | 100 | 167 | |
T3 | 45 | 19 | 26 | |
T4 | 19 | 8 | 11 | |
Pathologic stage | ||||
Stage I | 268 | 140 | 128 | 5.81E-04 |
Stage II | 119 | 42 | 77 | |
Stage III | 81 | 27 | 54 | |
Stage IV | 25 | 7 | 18 | |
Recurrence | ||||
Yes | 151 | 59 | 92 | 8.38E-02 |
No | 275 | 132 | 143 | |
Tobacco smoking history | ||||
Never | 28 | 17 | 11 | 1.33E-02 |
Reform | 127 | 67 | 60 | |
Current | 46 | 14 | 32 |
Gene name | Hazard Ratio | 95% Confidence Interval | Standard error | Z score | P-value | LASSO Coefficient |
C1QTNF6 | 1.477 | 1.239-1.761 | 0.090 | 4.341 | 1.420E-05 | 0.02171 |
THSD1 | 0.683 | 0.488-0.958 | 0.172 | -2.210 | 2.711E-02 | -0.00642 |
GRIK2 | 1.491 | 1.083-2.052 | 0.163 | 2.451 | 1.423E-02 | 0.00173 |
E2F7 | 1.386 | 1.091-1.759 | 0.122 | 2.679 | 7.390E-03 | 0.00650 |
SLCO1B3 | 1.261 | 1.084-1.466 | 0.077 | 3.002 | 2.680E-03 | 0.00430 |
Clinical characteristics | TCGA(N = 501) | Uni-variable cox | Multi-variable cox | ||
HR (95% CI) | P value | HR (95% CI) | P value | ||
Age(years, mean ± sd) | 65.28 ± 10.05 | 1.009[0.994-1.024] | 2.640E-01 | - | - |
Gender(Male/Female) | 231/270 | 1.060[0.792-1.418] | 6.954E-01 | - | - |
Pathologic M(M0/M1/-) | 333/24/144 | 2.111[1.232-3.616] | 5.361E-03 | 0.304[0.061-1.511] | 1.455E-01 |
Pathologic N(N0/N1/N2/-) | 324/94/72/11 | 1.782[1.493-2.128] | 3.993E-11 | 0.965[0.552-1.685] | 8.998E-01 |
Pathologic T(T1/T2/T3/T4/-) | 167/267/45/19/3 | 1.550[1.289-1.863] | 3.102E-06 | 1.221[0.823-1.531] | 4.672E-01 |
Pathologic stage(I / II / III / IV /-) | 268/119/81/25/8 | 1.679[1.463-1.928] | 2.942E-14 | 1.919[1.035-3.559] | 3.850E-02 |
Recurrence(Yes/No/-) | 151/275/75 | 2.392[1.700-3.367] | 2.438E-07 | 2.496[1.649-3.778] | 1.510E-05 |
Smoking history(Never/Reform/Current/-) | 28/127/46/300 | 0.765[0.542-1.081] | 1.287E-01 | - | - |
PS status(High/Low) | 250/251 | 2.201[1.625-2.981] | 1.805E-07 | 1.584[1.032-2.433] | 3.550E-03 |
Note: PS status stands for prognostic score status. |