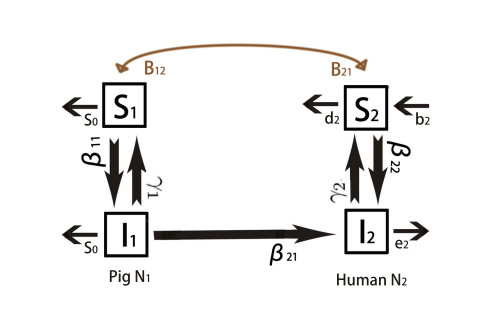
Map-based neuronal models have received much attention due to their high speed, efficiency, flexibility, and simplicity. Therefore, they are suitable for investigating different dynamical behaviors in neuronal networks, which is one of the recent hottest topics. Recently, the memristive version of the Rulkov model, known as the m-Rulkov model, has been introduced. This paper investigates the network of the memristive version of the Rulkov neuron map to study the effect of the memristor on collective behaviors. Firstly, two m-Rulkov neuronal models are coupled in different cases, through electrical synapses, chemical synapses, and both electrical and chemical synapses. The results show that two electrically coupled memristive neurons can become synchronous, while the previous studies have shown that two non-memristive Rulkov neurons do not synchronize when they are coupled electrically. In contrast, chemical coupling does not lead to synchronization; instead, two neurons reach the same resting state. However, the presence of both types of couplings results in synchronization. The same investigations are carried out for a network of 100 m-Rulkov models locating in a ring topology. Different firing patterns, such as synchronization, lagged-phase synchronization, amplitude death, non-stationary chimera state, and traveling chimera state, are observed for various electrical and chemical coupling strengths. Furthermore, the synchronization of neurons in the electrical coupling relies on the network's size and disappears with increasing the nodes number.
Citation: Mahtab Mehrabbeik, Fatemeh Parastesh, Janarthanan Ramadoss, Karthikeyan Rajagopal, Hamidreza Namazi, Sajad Jafari. Synchronization and chimera states in the network of electrochemically coupled memristive Rulkov neuron maps[J]. Mathematical Biosciences and Engineering, 2021, 18(6): 9394-9409. doi: 10.3934/mbe.2021462
[1] | Jing-An Cui, Fangyuan Chen . Effects of isolation and slaughter strategies in different species on emerging zoonoses. Mathematical Biosciences and Engineering, 2017, 14(5&6): 1119-1140. doi: 10.3934/mbe.2017058 |
[2] | Rodolfo Acuňa-Soto, Luis Castaňeda-Davila, Gerardo Chowell . A perspective on the 2009 A/H1N1 influenza pandemic in Mexico. Mathematical Biosciences and Engineering, 2011, 8(1): 223-238. doi: 10.3934/mbe.2011.8.223 |
[3] | Keguo Ren, Xining Li, Qimin Zhang . Near-optimal control and threshold behavior of an avian influenza model with spatial diffusion on complex networks. Mathematical Biosciences and Engineering, 2021, 18(5): 6452-6483. doi: 10.3934/mbe.2021321 |
[4] | Xiaomeng Wang, Xue Wang, Xinzhu Guan, Yun Xu, Kangwei Xu, Qiang Gao, Rong Cai, Yongli Cai . The impact of ambient air pollution on an influenza model with partial immunity and vaccination. Mathematical Biosciences and Engineering, 2023, 20(6): 10284-10303. doi: 10.3934/mbe.2023451 |
[5] | Julien Arino, Fred Brauer, P. van den Driessche, James Watmough, Jianhong Wu . A final size relation for epidemic models. Mathematical Biosciences and Engineering, 2007, 4(2): 159-175. doi: 10.3934/mbe.2007.4.159 |
[6] | Yiwei He, Qianqian Cui, Zengyun Hu . Modeling and analysis of the transmission dynamics of cystic echinococcosis: Effects of increasing the number of sheep. Mathematical Biosciences and Engineering, 2023, 20(8): 14596-14615. doi: 10.3934/mbe.2023653 |
[7] | Ning Bai, Juan Zhang , Li Li, Zhen Jin . Evaluating the effect of virus mutation on the transmission of avian influenza H7N9 virus in China based on dynamical model. Mathematical Biosciences and Engineering, 2019, 16(5): 3393-3410. doi: 10.3934/mbe.2019170 |
[8] | Muntaser Safan, Bayan Humadi . An SIS sex-structured influenza A model with positive case fatality in an open population with varying size. Mathematical Biosciences and Engineering, 2024, 21(8): 6975-7011. doi: 10.3934/mbe.2024306 |
[9] | Haitao Song, Lirong Guo, Zhen Jin, Shengqiang Liu . Modelling and stability analysis of ASFV with swill and the virus in the environment. Mathematical Biosciences and Engineering, 2022, 19(12): 13028-13049. doi: 10.3934/mbe.2022608 |
[10] | Kasia A. Pawelek, Anne Oeldorf-Hirsch, Libin Rong . Modeling the impact of twitter on influenza epidemics. Mathematical Biosciences and Engineering, 2014, 11(6): 1337-1356. doi: 10.3934/mbe.2014.11.1337 |
Map-based neuronal models have received much attention due to their high speed, efficiency, flexibility, and simplicity. Therefore, they are suitable for investigating different dynamical behaviors in neuronal networks, which is one of the recent hottest topics. Recently, the memristive version of the Rulkov model, known as the m-Rulkov model, has been introduced. This paper investigates the network of the memristive version of the Rulkov neuron map to study the effect of the memristor on collective behaviors. Firstly, two m-Rulkov neuronal models are coupled in different cases, through electrical synapses, chemical synapses, and both electrical and chemical synapses. The results show that two electrically coupled memristive neurons can become synchronous, while the previous studies have shown that two non-memristive Rulkov neurons do not synchronize when they are coupled electrically. In contrast, chemical coupling does not lead to synchronization; instead, two neurons reach the same resting state. However, the presence of both types of couplings results in synchronization. The same investigations are carried out for a network of 100 m-Rulkov models locating in a ring topology. Different firing patterns, such as synchronization, lagged-phase synchronization, amplitude death, non-stationary chimera state, and traveling chimera state, are observed for various electrical and chemical coupling strengths. Furthermore, the synchronization of neurons in the electrical coupling relies on the network's size and disappears with increasing the nodes number.
Domestic pigs originated from the Eurasian wild boar (Sus scrofa), which first appeared about 9000 years ago [1]. They are essential for the transmission of swine influenza. Human beings raise domestic pigs, and then slaughter them for pork [2]. Domestic pigs grow in the food and environment provided by human beings, while human beings get the necessary nutrients by eating them. Consequently, in the breeding process, the swine flu virus is transmitted to human beings through domestic pig-human contact [2,3]. According to this process, a new breed-slaughter model with swine influenza transmission can be proposed as a model (1.1).
{∂S1(x,t)∂t=D1∂2S1(x,t)∂x2+(B12N2(x,t)−ω0N1(x,t))N1(x,t)−s0S1(x,t) −β11I1(x,t)S1(x,t)+γ1I1(x,t),x∈R,t>0,∂I1(x,t)∂t=D1∂2I1(x,t)∂x2+β11I1(x,t)S1(x,t)−(s0+γ1)I1(x,t),x∈R,t>0,∂S2(x,t)∂t=D2∂2S2(x,t)∂x2+(b2−r2N2(x,t)K2+B21N1(x,t))N2(x,t)−d2S2(x,t) −2∑j=1β2jIj(x,t)S2(x,t)+γ2I2(x,t),x∈R,t>0,∂I2(x,t)∂t=D2∂2I2(x,t)∂x2+2∑j=1β2jIj(x,t)S2(x,t)−[e2+γ2+d2]I2(x,t),x∈R,t>0,Ni(x,t)=Si(x,t)+Ii(x,t),i=1,2,x∈R,t>0. | (1.1) |
Domestic pig population N1(x,t) and human population N2(x,t) are assumed to be divided into 2 epidemiological compartments: susceptibles (Si(x,t)) and infectives (Ii(x,t)) at time t and location x, i=1,2. Susceptibles can become infected by means of intra-species or inter-species transmission and then recover as new susceptibles. The notation B12 represents the human breeding parameter for the population growth of domestic pigs, while B21 represents the nutrients from eating domestic pigs to increase the birth rate of human beings. The notation s0 represents the slaughter rate of domestic pigs. It's noteworthy that domestic pigs cannot survive independently without human beings, but human beings can still survive well without the supply of pork [2]. Restrictions on the development of human population mainly come from intra-species competition.
For humans, the notation r2=b2−d2 is the intrinsic growth rate of humans, where b2 and d2 represents the natural natality rate and mortality rate, respectively. K2 is the environmental carrying capacity of human population without domestic pig supply. e2 is the additional mortality rate of humans caused by swine flu. For domestic pigs, ω0 represents the intraspecific competition. During the spread of swine flu, the parameters βij represent the per capita incidence rate from species j to species i, where i,j=1,2. γi denote the recovery rate for domestic animals and humans, i=1,2. D1 and D2 are the diffusion coefficients for domestic animals and humans. It is noteworthy that all parameters mentioned above is positive.
The main purpose of this paper is to propose a new breed-slaughter model with swine influenza transmission, and study the dynamic behavior of it. And then, we focus on the invasion process of infected domestic animals into the habitat of humans. Under certain conditions as in Theorem 2, we construct a propagating terrace linking human habitat to animal-human coexistent habitat, then to swine flu natural foci, which is divided by spreading speeds. Firstly, we calculate the equilibrium points of the model without spatial heterogeneity as a model (1.2) and analyze the existence of them by the persistence theory. Secondly, we discuss their stability by the basic reproduction number. Thirdly, we use these equilibrium points to construct a propagating terrace linking them by spreading speeds.
{dS1(t)dt=(B12N2(t)−ω0N1(t))N1(t)−s0S1(t)−β11I1(t)S1(t)+γ1I1(t),dI1(t)dt=β11I1(t)S1(t)−(s0+γ1)I1(t),dS2(t)dt=(b2−r2N2(t)K2+B21N1(t))N2(t)−d2S2(t)−2∑j=1β2jIj(t)S2(t)+γ2I2(t),dI2(t)dt=2∑j=1β2jIj(t)S2(t)−[e2+γ2+d2]I2(t),Ni(t)=Si(t)+Ii(t),i=1,2. | (1.2) |
In model (1.2), domestic pig population N1(t) and human population N2(t) are assumed to be divided into 2 epidemiological compartments: susceptibles (Si(t)) and infectives (Ii(t)) at time t, i=1,2. Other parameters are the same with model (1.1).
At first, we focus on the breed-slaughter system without swine flu transmission and spatial heterogeneity.
If I1(0)=I2(0)=0, N1(0)>0 and N2(0)>0, model (1.2) turns to a new breed-slaughter system without swine influenza transmission as model (2.1).
{dN1(t)dt=(B12N2(t)−ω0N1(t))N1(t)−s0N1(t),dN2(t)dt=r2(1−N2(t)K2)N2(t)+B21N1(t)N2(t),N1(0)>0,N2(0)>0, | (2.1) |
Similar to the competition system in [4], breed-slaughter system also has abundant dynamic results. For the positive equilibrium point
E∗=(N∗1,N∗2)=(r2(s0−B12K2)B12B21K2−ω0r2,K2(s0B21−ω0r2)B12B21K2−ω0r2) |
of model (2.1), we have three cases:
(a). If B12B21<ω0r2K2 and s0<min{ω0r2B21,B12K2}, the positive equilibrium point E∗ of model (2.1) is stable (Figure 2(a)).
(b). If B12B21>ω0r2K2 and s0>max{ω0r2B21,B12K2}, the positive equilibrium point E∗ of model (2.1) is unstable (Figure 2(b)).
(c). Other than the condition as (a) or (b), the positive equilibrium point E∗ of model (2.1) does not exist.
In order to reflect the effect of interspecific interaction on swine influenza transmission during breeding process as model (1.2), we suppose that B12B21<ω0r2K2 and s0<min{ω0r2B21,B12K2} to guarantee the existence and the stability of the boundary equilibrium point
E3=(r2(s0−B12K2)B12B21K2−ω0r2,0,K2(s0B21−ω0r2)B12B21K2−ω0r2,0) |
with I1(0)=I2(0)=0 in model (1.2).
After calculation, we summarize that there are at most 6 equilibrium points in R4+ of the system (1.2): E0=(0,0,0,0), E1=(0,0,K2,0), E2=(0,0,¯S2,¯I2), E3=(N∗1,0,N∗2,0), E4=(N′1,0,S′2,I′2), E5=(S∗1,I∗1,S∗2,I∗2), where ¯S2=e2+γ2+d2β22, ¯I2=β22K2−(e2+γ2+d2)β22, N∗1=N′1=r2(s0−B12K2)B12B21K2−ω0r2, N∗2=K2(s0B21−ω0r2)B12B21K2−ω0r2, S′2=e2+γ2+d2β22, I′2=β22K2(1+s0B21−B12B21K2B12B21K2−ω0r2)−(e2+γ2+d2)β22. The exact expression of E5 is unknown. However, under certain conditions as in Theorem 2, we can obtain its existence by persistence theory [5,6,7].
If there is no domestic pigs participation, namely N1(0)=S1(0)=I1(0)=0, The persistence and the stability of boundary equilibrium E2=(0,0,¯S2,¯I2) has been proved in [8]. Similarly, we define R0=β22K1b2+e2+γ2. And then, we can get the following lemma.
Lemma 1. If N1(0)=S1(0)=I1(0)=0 and I2(0)>0, {0}×{0}×R2+ is a invariant set of system (1.2). The trivial equilibrium point E0 in model (1.2) is unstable, and we have following two cases:
(a) If R0≤1, the disease-free equilibrium point E1 of model (1.2) is stable;
(b) If R0>1, model (1.2) has a unique equilibrium point E2 in the interior of {0}×{0}×R2+, which is stable, and E1 is unstable.
Furthermore, we consider the transmission process of human influenza with domestic pigs participating, but not infected from them. Namely I1(0)=0, I2(0)>0 and Ni(0)>0, i=1,2. The persistence and the stability of boundary equilibrium E4=(N′1,0,S′2,I′2) is similar to Lemma 1. Taking E3 as the original point by coordinate translation, we can get the following lemma by the persistence theory [5,9,10], when B12B21<ω0r2K2 and s0<min{ω0r2B21,B12K2}.
According to the definition of basic reproduction number in a single population as [5,11,12], we define R1=β1N∗1s0+γ1 as the basic reproduction number of swine flu transmission in demotic pig population and R2=β22N∗2e2+γ2+d2 as the basic reproduction number of swine flu transmission in human population.
Lemma 2. If N1(0)=S1(0)>0, I1(0)=0 and I2(0)>0, R+×{0}×R2+ is a invariant set of system (1.2). The trivial equilibrium point E0 and the boundary equilibrium point E1, E2 in model (1.2) are unstable when B12B21<ω0r2K2 and s0<min{ω0r2B21,B12K2}, and we have following two cases:
(a) If R2≤1, the disease-free equilibrium point E3 of model (1.2) is stable;
(b) If R2>1, model (1.2) has a unique equilibrium point E4 in the interior of R+×{0}×R2+, which is stable, and E3 is unstable.
Next we focus on the discussion about the existence and the stability of the positive equilibrium point E5=(S∗1,I∗1,S∗2,I∗2). At first, we define Rs=max{R1,R2}. Then, we get the theorem as the following.
Theorem 1. If Ni(0)>0 and Ii(0)>0, i=1,2, R4+ is a invariant set of system (1.2). The trivial equilibrium point E0 and the boundary equilibrium point E1, E2 in model (1.2) are unstable when B12B21<ω0r2K2 and s0<min{ω0r2B21,B12K2}, and we have following three cases:
(a) If Rs≤1, the disease-free equilibrium point E3 of model (1.2) is stable;
(b) If Rs>1, R1<R2 and R1≤1, model (1.2) has a unique equilibrium point E4 except for E0, E1, E2 and E3, which is stable, and E3 is unstable;
(c) If Rs>1 and R1≥R2 (or R2>R1>1) model (1.2) has a unique equilibrium point E5 in the interior of R4+, which is stable, and E3 and E4 are unstable.
Proof. If Rs≤1, E4 and E5 do not exist. Similar to the results of Lemma 2 (a), the disease-free equilibrium point E3 of model (1.2) is stable.
Then we consider the results of system (1.2) when Rs>1 and B12B21<ω0r2K2 and s0<min{ω0r2B21,B12K2}. At first, we define
D={(S1,I1,S2,I2)|0≤Ii≤Si+Ii≤N∗i,i=1,2}, |
D1={(S1,I1,S2,I2)|I1=0 or I2=0 ,0≤Si+Ii≤N∗i,i=1,2}, |
D2=D∖D1,˜D2={(S1,I1,S2,I2)|0<Ii≤Si+Ii≤N∗i,i=1,2}. |
D2 and ˜D2 are forward invariant.
Let Ω∗ consists of equilibria E0, E1, E2, E3 and E4. These equilibria cannot be chained to each other in D1. By analyzing the flow in neighborhood of each equilibrium, it is easy to see that Ω∗ is isolated in D and D1 is a uniform strong repeller for ˜D2.
If x(t)=(S1(t),I1(t),S2(t),I2(t)) stays close to E2, we have two cases: if I1(0)=I2(0)=0, then I1(t)=I2(t)=0; if I1(0)>0 or I2(0)>0, then I2(t)>0. Therefore, E2 is isolated in D. Similarly, we can prove that E0, E1 and E3 are isolated in D.
For E4 and E5, we have two cases: (A). R1<R2 and R1≤1; (B). R1≥R2 or R2>R1>1.
(A). R1<R2 and R1≤1
If R1<R2 and R1≤1, E5 do not exist. Similar to the results of Lemma 2 (b), the boundary equilibrium point E4 of model (1.2) is stable.
(B). R1≥R2 or R2>R1>1
If x(t)=(S1(t),I1(t),S2(t),I2(t)) stays close to E4, we have two cases: if I1(0)=0, then I1(t)=0; if I1(0)>0, then I1(t)>0. Since (S1(t),I1(t),S2(t),I2(t)) satisfying system (1.2) has no invariant subset other than E4 in its neighborhood. E4 is isolated in D and a uniform weak repeller for ˜D2. Therefore, we can prove that E0, E1, E2, E3 and E4 are isolated in D.
Using Proposition 4.3 in [5], we can prove that D1 is a uniform weak repeller for ˜D2; and using Theorem 4.5 in [5], we can prove that D1 is a uniform strong repeller for ˜D2.
Then we get that there exists an ϵ>0 such that
liminft→∞min{I1(t),I2(t)}>ϵ, |
with Ni(0)>0 and Ii(0)>0, i=1,2.
Therefore, if B12B21<ω0r2K2, s0<min{ω0r2B21,B12K2} and R1≥R2 (R2>R1>1), there exists at least one internal equilibrium of system (1.2) [9,10,13].
Next, we use Theorem 2 in [11] to discuss the basic reproduction number of system (1.2).
The Jacobian matrix of (I1,I2) is
J=(β11S1−(s0+γ1)0β21S2β22S2−(e2+γ2+d2)), |
Let J=F−V, F be the rate of appearance of new infections in compartment I, V be the rate of transfer of individuals out of compartment I. Then, we get
F=(β11S10β21S2β22S2), |
V= diag(s0+γ1e2+γ2+d2). |
We call FV−1 be the next generation matrix for the model (1.2) and set Rs=ρ(FV−1|E3), where ρ(A) denotes the spectral radius of a matrix A.
Then we get
Rs=max{β11N∗1s0+γ1,β22N∗2e2+γ2+d2}. |
Finally, using Theorem 2 in [11], we can prove Theorem 1.
The basic reproduction number is an important threshold value in the research of the epidemic mathematical model, which determines the disease to break out or not. However, it is not sufficient to discuss the breed-slaughter model with interspecific interaction. The main purpose of this paper is to investigate invasion process of infected domestic animal into human habitat. And we construct a propagating terrace linking human habitat E1 to animal-human coexistent habitat E3, then to swine flu natural foci E4 (or E5), which is divided by certain spreading speeds. The propagating terrace can describe the spatio-temporal continuous change of the transmission of swine flu.
Based on the heterogeneity of the population structure and the temporal and spatial continuity of the mammal movement, the population's spatial factor is considered in the spread of swine flu. If the swine flu host populations are distributed differently in space, the diffusion term may change their local population structure, thus change the swine flu epidemic. In order to describe the population invasion process, we set the initial value is zero in the area x∈(−∞,−x0)∪(x0,∞). The area of (−x0,x0) is the original habitat of N, and N will invade to the area of x∈(−∞,−x0)∪(x0,∞) at the spreading speed s [14].
The definition of spreading speed of a single population is the positive value s satisfied with the conditions as follows,
limt→+∞{sup|x|>ctN(x,t)}=0,∀c>s |
and
limt→+∞inf{inf|x|<ctN(x,t)}>0,∀c<s, |
in the model [4.1]
{∂N(x,t)∂t=D∂2N(x,t)∂x2+rN(x,t)(1−N(x,t)K),x∈R,t>0,N(x,0)=N0>0,x∈[−x0,x0],N(x,0)=0,x∈(−∞,x0)∪(x0,∞). | (4.1) |
The biological description of spreading speed s has been shown in the third figure of Figure 3. The value of s approximates the inverse of the slope of the color lines. It is easy to see that the co-effect of diffusion and reproduction leads to the population territory expansion, in which the local diffusion rate D guarantees the population spatial invasion to new areas and the reproduction rate r guarantees its development on occupied areas. The spreading speed of a single population in the model [4.1] is expressed by s:=2√Dr by [14]. However, it is not enough to study the swine flu with more than one host species [15,16,17,18,19,20]. We redefine the spreading speeds at the human-animal interface, as shown below.
s1:=2√D1(B12K2−s0), |
s2:=max{2√D1(β11N∗1−s0−γ1),2√D2(β22N∗2−e2−γ2−d2)}. |
Due to the participation of two populations, some notations need to be redefined. The notations s and x0 are replaced by si, xi, with i=1,2, corresponding to the two swine flu host populations.
Then we construct a propagating terrace linking human habitat E1 to animal-human coexistent habitat E3, then to swine flu natural foci E4 (or E5), which is divided by certain spreading speeds. The propagating terrace can describe the spatio-temporal continuous change of the transmission of swine flu, which can be show in Theorem 2.
Theorem 2. For system (1.1), if B12B21<ω0r2K2, B21N∗1<r2 and s0<min{ω0r2B21,B12K2}, the initial conditions satisfy that 0<S1(x,0)<N∗1, x∈[−x1,x1]; S1(x,0)=0, x∈(−∞,x1)∪(x1,∞), for some x1>0; 0<I1(x,0)<N∗1, x∈[−x2,x2]; I1(x,0)=0, x∈(−∞,x2)∪(x2,∞), for some x2>0; S2(x,0)=K2, I2(x,0)=0, x∈R.
We set
s1:=2√D1(B12K2−s0),s2:=max{2√D1(β11N∗1−s0−γ1),2√D2(β22N∗2−e2−γ2−d2)}. |
Suppose that s1>s2, x1>x2, then there are three cases about the invasion process as following:
(a) Rs≤1,
limt→+∞sup|x|>ct{|S1(x,t)|+|I1(x,t)|+|S2(x,t)−K2|+|I2(x,t)|}=0, ∀c>s1, |
limt→+∞sup|x|<ct{|S1(x,t)−N∗1|+|I1(x,t)|+|S2(x,t)−N∗2|+|I2(x,t)|}=0, ∀c<s1. |
The system (1.1) forms a propagating terrace, linking E1 to E3.
(b) If Rs>1, R1<R2 and R1≤1,
limt→+∞sup|x|>ct{|S1(x,t)|+|I1(x,t)|+|S2(x,t)−K2|+|I2(x,t)|}=0, ∀c>s1, |
limt→+∞supc2t<|x|<c1t{|S1(x,t)−N∗1|+|I1(x,t)|+|S2(x,t)−N∗2|+|I2(x,t)|}=0, ∀s2<c2<c1<s1, |
limt→+∞sup|x|<ct{|S1(x,t)−N′1|+|I1(x,t)|+|S2(x,t)−S′2|+|I2(x,t)−I′2|}=0, ∀c<s2. |
The system (1.1) forms a propagating terrace, linking E1 to E3, then to E4.
(c) If Rs>1 and R1≥R2 (or R2>R1>1),
limt→+∞sup|x|>ct{|S1(x,t)|+|I1(x,t)|+|S2(x,t)−K2|+|I2(x,t)|}=0, ∀c>s1, |
limt→+∞supc2t<|x|<c1t{|S1(x,t)−N∗1|+|I1(x,t)|+|S2(x,t)−N∗2|+|I2(x,t)|}=0, ∀s2<c2<c1<s1, |
limt→+∞sup|x|<ct{|S1(x,t)−S∗1|+|I1(x,t)−I∗1|+|S2(x,t)−S∗2|+|I2(x,t)−I∗2|}=0, ∀c<s2. |
The system (1.1) forms a propagating terrace, linking E1 to E3, then to E5.
Proof. The epidemic of swine flu originates in the interaction between humans and domestic animals in the breeding process, so the breaking out of swine flu would lag behind this process. Therefore, we first confirm the propagating terrace linking E1 and E3.
The breed-slaughter system without swine flu transmission can be transferred to model (4.2).
{∂N1(x,t)∂t=D1∂2N1(x,t)∂t2+(B12N2(x,t)−ω0N1(x,t))N1(x,t)−s0N1(x,t),∂N2(x,t)∂t=D2∂2N2(x,t)∂t2+r2(1−N2(x,t)K2)N2(t)+B21N1(x,t)N2(x,t). | (4.2) |
Let (N1,N2) be a solution to system (4.2) with the initial condition 0<N1(x,0)<N∗1, x∈[−x1,x1]; N1(x,0)=0, x∈(−∞,x1)∪(x1,∞), for some x1>0; N2(x,0)=K2, x∈R.
If B12B21<ω0r2K2 and s0<min{ω0r2B21,B12K2}, we claim that (N1(x,t),N2(x,t))∈Σ, ∀x∈R,t∈[0,∞), where
Σ:={(N1,N2)∈[0,N∗1]×[0,N∗2]:B12N2(x,t)−ω0N1(x,t)−s0≥0,r2(1−N2(x,t)K2)+B21N1(x,t)≥0}. |
By the strong maximum principle, N1≥0 for t>0. Then we get
∂N2(x,t)∂t≥D2∂2N2(x,t)∂t2+r2(1−N2(x,t)K2)N2(x,t). |
By a comparison, N2≥X, where X is the solution to
{∂X(x,t)∂t=D2∂2X(x,t)∂t2+r2(1−X(x,t)K2)X(x,t),X(x,0)=N2(x,0). | (4.3) |
Then we get the result
limt→+∞infN2(x,t)≥limt→+∞infX(x,t)=K2. |
Set u:=N1 and v:=N2−K2. Then ∂N2(x,t)∂t can be rewritten as
∂v(x,t)∂t=D2∂2v(x,t)∂t2−r2v(x,t)K2(v(x,t)+K2)+B21u(x,t)(v(x,t)+K2). |
Due to u=N1∈[0,N∗1] and v=N2−K2≥0 then
∂v(x,t)∂t≤D2∂2v(x,t)∂t2−(r2−B21N∗1)v(x,t)+B21K2u(x,t). |
By the strong maximum principle, if follows that v≤Y in R×[0,∞), where Y is the solution to
{∂Y(x,t)∂t=D2∂2Y(x,t)∂t2−(r2−B21N∗1)Y(x,t)+B21K2u(x,t),X(x,0)=0,x∈R. | (4.4) |
Then we have
Y(x,t)=B21K2∫t0{e−(r2−B21N∗1)(t−s)∫Re−(x−y)2/[4(t−s)]u(y,s)dy}ds. |
Given ϵ>0, we choose δ>0 small enough such that 2√D1(B12K2−s0+B12δ)<s1+ϵ.
For this δ, we claim that there is τ≫1 such that Y(x,t)<δ+Mu(x,t), ∀x∈R, t≥τ, for some positive constant M. Then it follows that N1 satisfies
∂N1(x,t)∂t≤D1∂2N1(x,t)∂t2+(B12K1−s0+B12δ−(B12M+ω0)N1(x,t))N1(x,t). |
Therefore, according to the comparison principle and the definition of spreading speed [14,15,16,19,21], for any c∈(2√D1(B12K2−s0+B12δ),s1+ϵ), it follows that limt→+∞sup|x|>ctN1(x,t)=0, and then limt→+∞sup|x|>ctN2(x,t)=K2.
Because of the arbitrariness of ϵ, we get
limt→+∞sup|x|>ct{|N1(x,t)|+|N2(x,t)−K2|}=0,∀c>s1. |
Thus, if the swine flu does not break out, namely Rs≤1, for system (1.1),
limt→+∞sup|x|>ct{|S1(x,t)|+|I1(x,t)|+|S2(x,t)−K2|+|I2(x,t)|}=0, ∀c>s1. |
Then we set U:=N∗1−N1 and V:=N∗2−N2. Similar to the proof before, we can get
limt→+∞sup|x|<ct{|N1(x,t)−N∗1|+|N2(x,t)−N∗2|}=0, ∀c<s1. |
If Rs≤1, for system (1.1),
limt→+∞sup|x|<ct{|S1(x,t)−N∗1|+|I1(x,t)|+|S2(x,t)−N∗2|+|I2(x,t)|}=0, ∀c<s1. |
Next we consider the propagating terrace linking E3 to (E4 or E5). Let (S1,I1,S2,I2) be a solution to system (1.1) with the initial condition S1(x,0)=N∗1, S2(x,0)=N∗2, I2(x,0)=0, x∈R. I1(x,0)>0, x∈[−x2,x2]; I1(x,0)=0, x∈(−∞,x2)∪(x2,∞), for some x2>0.
If B12B21<ω0r2K2 and s0<min{ω0r2B21,B12K2}, we claim that (S1(x,t)+I1(x,t),S2(x,t)+I2(x,t))∈Σ, ∀x∈R,t∈[0,∞).
For the spreading speed when Rs>1, comparison principle and strong maximum principle are no longer applicable due to the complexity of system (1.1). However, we can calculate the minimum wave speed from largest eigenvalue of its linearized system at E3 as [22] to link E3 and E4 (or E5).
For the following eigenvalue problem
1λAληλ=cηλ, |
where
Aλ=diag(Diλ2)+J|E3. |
J is the jacobian matrix,
J=(B12N2−2ω0N1−s0−β11I1−β11S1+γ1B12N10β11I1β11S1−(s0+γ1)00B12N2−β21S2r2(1−2N2K2)+B21N1−(β21I1+β22I2)−β22S2+γ20β21S2β21I1+β22I2β22S2−(e2+γ2+d2)). |
For λ≥0, the eigenvalues of the matrix
Aλ=(D1λ2−ω0N∗1−β11N∗1+γ1B12N∗100D1λ2+β11N∗1−(s0+γ1)00B12N∗2−β21N∗2D2λ2−r2N∗2K2−β22N∗2+γ20β21N∗20D2λ2+β22N∗2−(e2+γ2+d2)). |
are D1λ2+β11N∗1−(s1+γ1), D2λ2+β22N∗2−(e2+γ2+d2) and other two impossible results, which cannot define positive wave speed.
Thus, the minimum wave speed can be defined as follows, which can be divided the propagating terrace, linking E3 to E4 (or E5).
s2=max{infλ>0D1λ2+β11N∗1−(s0+γ1)λ,infλ>0D2λ2+β22N∗2−(e2+γ2+d2)λ}=max{2√D1(β11N∗1−s0−γ1),2√D2(β22N∗2−e2−γ2−d2)}. |
If Rs>1, there are two cases: (A). R1<R2 and R1≤1; (B). R1≥R2 or R2>R1>1.
(A). If R1<R2 and R1≤1, then E5 does not exist. s2=2√D2(β22N∗2−e2−γ2−d2), then we get
limt→+∞sup|x|>c2t+x2{|S1(x,t)−N∗1|+|I1(x,t)|+|S2(x,t)−N∗2|+|I2(x,t)|}=0, ∀c>s2, |
limt→+∞sup|x|<ct+x2{|S1(x,t)−N′1|+|I1(x,t)|+|S2(x,t)−S′2|+|I2(x,t)−I′2|}=0, ∀c<s2. |
Combining the results before, linking E1 to E3, then
limt→+∞sup|x|>ct+x1{|S1(x,t)|+|I1(x,t)|+|S2(x,t)−K2|+|I2(x,t)|}=0, ∀c>s1, |
limt→+∞supc2t+x2<|x|<c1t+x1{|S1(x,t)−N∗1|+|I1(x,t)|+|S2(x,t)−N∗2|+|I2(x,t)|}=0, ∀s2<c2<c1<s1, |
limt→+∞sup|x|<ct+x2{|S1(x,t)−N′1|+|I1(x,t)|+|S2(x,t)−S′2|+|I2(x,t)−I′2|}=0, ∀c<s2. |
The system (1.1) forms a propagating terrace, linking E1 to E3, then to E4.
(B). If R1≥R2 or R2>R1>1, set s2=max{2√D1(β11N∗1−s0−γ1),2√D2(β22N∗2−e2−γ2−d2)}. then we get
limt→+∞sup|x|>ct+x1{|S1(x,t)|+|I1(x,t)|+|S2(x,t)−K2|+|I2(x,t)|}=0, ∀c>s1, |
limt→+∞supc2t+x2<|x|<c1t+x1{|S1(x,t)−N∗1|+|I1(x,t)|+|S2(x,t)−N∗2|+|I2(x,t)|}=0, ∀s2<c2<c1<s1, |
limt→+∞sup|x|<ct+x2{|S1(x,t)−S∗1|+|I1(x,t)−I∗1|+|S2(x,t)−S∗2|+|I2(x,t)−I∗2|}=0, ∀c<s2. |
The system (1.1) forms a propagating terrace, linking E1 to E3, then to E5.
If s1>s2, x1>x2 and Rs>1, R1>R2>1, then in Figure 4, the blue area represents the original habitat area of humans at the population size of E1. After domesticating pigs, the red part will be shared with the two species at E3. While after swine flu transmitting between domestic pigs and humans, the internal red part will be shared again with two populations at E5 with swine flu transmission. It is a biological description of propagating terrace of humans with swine flu transmission, which is the local spacial variation of the population.
If s1>s2,x1>x2 and Rs≤1, then in Figure 5, the blue area represents the original habitat area of humans at the population size of E1. After domesticating pigs, the red part will be shared with the two species at E3. Because Rs≤1, there is no swine flu transmission during the breed and slaughter process. Then the propagating terrace links unstable equilibrium E1 and stable equilibrium E2.
We establish a new swine flu mathematical model to reflect the dynamic process of swine flu transmission with interspecific action between domestic pigs and humans, in which the roles of different species will no longer be at the same level. Domestic pigs cannot survive independently without human beings, but human beings can still survive well without the supply of pork. By our new swine flu model, we find that the human-animal interface has promoted the cross-species transmission of swine flu and resulted in the prevalence of flu in humans. In addition, the threshold values of population development and disease transmission are also discussed in order to provide a scientific basis for future health decision makers in swine flu prevention and control. We propose the zoonotic basic reproduction number Rs, which is more applicable to the study of swine flu transmission. Then, it is analyzed that the spreading speed of different species forming propagating terraces is influenced by the intrinsic growth rate r and diffusion rate D.
In this paper, the equilibrium points of the model are calculated and we analyze the existence of the equilibrium points by the persistence theory. Then we discuss their stability by the basic reproduction number. In addition, after redefining the spreading speed, we divide the propagating terrace with two populations, which is an unprecedented task. We concern with the invasion process of infected domestic animals into the habitat of humans. Under certain conditions as in Theorem 2, we construct a propagating terrace linking human habitat to animal-human coexistent habitat, then to swine flu natural foci, which is divided by spreading speeds.
This work is supported by the National Natural Science Foundation of China, 11771044.
The authors declared that they have no conflicts of interest to this work.
[1] |
B. Ibarz, J. M. Casado, M. A. Sanjuán, Map-based models in neuronal dynamics, Phys. Rep., 501 (2011), 1-74. doi: 10.1016/j.physrep.2010.12.003
![]() |
[2] |
J. Ma, J. Tang, A review for dynamics in neuron and neuronal network, Nonlinear Dyn., 89 (2017), 1569-1578. doi: 10.1007/s11071-017-3565-3
![]() |
[3] |
A. L. Hodgkin, A. F. Huxley, A quantitative description of membrane current and its application to conduction and excitation in nerve, J. Physiol., 117 (1952), 500. doi: 10.1113/jphysiol.1952.sp004764
![]() |
[4] | J. L. Hindmarsh, R. Rose, A model of neuronal bursting using three coupled first order differential equations, Proc. R. Soc. London Series B. Biol. Sci., 221 (1984), 87-102. |
[5] |
N. F. Rulkov, Modeling of spiking-bursting neural behavior using two-dimensional map, Phys. Rev. E, 65 (2002), 041922. doi: 10.1103/PhysRevE.65.041922
![]() |
[6] |
E. M. Izhikevich, Simple model of spiking neurons, IEEE Trans. Neural Networks, 14 (2003), 1569-1572. doi: 10.1109/TNN.2003.820440
![]() |
[7] | K. Rajagopal, S. Panahi, M. Chen, S. Jafari, B. Bao, Suppressing spiral wave turbulence in a simple fractional-order discrete neuron map using impulse triggering, Fractals, 29 (2021), 2140030. |
[8] | H. Bao, A. Hu, W. Liu, B. Bao, Hidden bursting firings and bifurcation mechanisms in memristive neuron model with threshold electromagnetic induction, IEEE Trans. Neural Networks Learn. Syst., 31 (2019), 502-511. |
[9] | K. Li, H. Bao, H. Li, J. Ma, Z. Hua, B. C. Bao, Memristive Rulkov neuron model with magnetic induction effects, IEEE Trans. Ind. Inf., 2021. |
[10] |
M. Lv, C. Wang, G. Ren, J. Ma, X. Song, Model of electrical activity in a neuron under magnetic flow effect, Nonlinear Dyn., 85 (2016), 1479-1490. doi: 10.1007/s11071-016-2773-6
![]() |
[11] |
K. Rajagopal, I. Moroz, B. Ramakrishnan, A. Karthikeyn, P. Duraisamy, Modified Morris-Lecar neuron model: effects of very low frequency electric fields and of magnetic fields on the local and network dynamics of an excitable media, Nonlinear Dyn., 104 (2021), 4427-4443. doi: 10.1007/s11071-021-06494-0
![]() |
[12] |
K. Usha, P. Subha, Hindmarsh-Rose neuron model with memristors, Biosystems, 178 (2019), 1-9. doi: 10.1016/j.biosystems.2019.01.005
![]() |
[13] |
X. Hu, C. Liu, Dynamic property analysis and circuit implementation of simplified memristive Hodgkin-Huxley neuron model, Nonlinear Dyn., 97 (2019), 1721-1733. doi: 10.1007/s11071-019-05100-8
![]() |
[14] | H. Bao, Z. Hua, H. Li, M. Chen, B. Bao, Discrete memristor hyperchaotic maps, IEEE Trans. Circuits Syst. I, 2021. |
[15] |
H. Li, Z. Hua, H. Bao, L. Zhu, M. Chen, B. Bao, Two-dimensional memristive hyperchaotic maps and application in secure communication, IEEE Trans. Ind. Electron., 68 (2021), 9931-9940. doi: 10.1109/TIE.2020.3022539
![]() |
[16] |
I. Hussain, S. Jafari, D. Ghosh, M. Perc, Synchronization and chimeras in a network of photosensitive FitzHugh-Nagumo neurons, Nonlinear Dyn., 104 (2021), 2711-2721. doi: 10.1007/s11071-021-06427-x
![]() |
[17] |
A. Bahramian, F. Parastesh, V. T. Pham, T. Kapitaniak, S. Jafari, M. Perc, Collective behavior in a two-layer neuronal network with time-varying chemical connections that are controlled by a Petri net, Chaos: Interdiscip. J. Nonlinear Sci., 31 (2021), 033138. doi: 10.1063/5.0045840
![]() |
[18] |
A. E. Pereda, Electrical synapses and their functional interactions with chemical synapses, Nat. Rev. Neurosci., 15 (2014), 250-263. doi: 10.1038/nrn3708
![]() |
[19] |
H. Sun, H. Cao, Complete synchronization of coupled Rulkov neuron networks, Nonlinear Dyn., 84 (2016), 2423-2434. doi: 10.1007/s11071-016-2654-z
![]() |
[20] |
D. Hu, H. Cao, Stability and synchronization of coupled Rulkov map-based neurons with chemical synapses, Commun. Nonlinear Sci. Numer. Simul., 35 (2016), 105-122. doi: 10.1016/j.cnsns.2015.10.025
![]() |
[21] |
S. Rakshit, A. Ray, B. K. Bera, D. Ghosh, Synchronization and firing patterns of coupled Rulkov neuronal map, Nonlinear Dyn., 94 (2018), 785-805. doi: 10.1007/s11071-018-4394-8
![]() |
[22] |
M. Perc, Thoughts out of noise, Eur. J. Phys., 27 (2006), 451. doi: 10.1088/0143-0807/27/2/026
![]() |
[23] |
X. Sun, M. Perc, Q. Lu, J. Kurths, Effects of correlated Gaussian noise on the mean firing rate and correlations of an electrically coupled neuronal network, Chaos: Interdiscip. J. Nonlinear Sci., 20 (2010), 033116. doi: 10.1063/1.3483876
![]() |
[24] |
Q. Wang, M. Perc, Z. Duan, G. Chen, Synchronization transitions on scale-free neuronal networks due to finite information transmission delays, Phys. Rev. E, 80 (2009), 026206. doi: 10.1103/PhysRevE.80.026206
![]() |
[25] |
S. Boccaletti, J. Kurths, G. Osipov, D. Valladares, C. Zhou, The synchronization of chaotic systems, Phys. Rep., 366 (2002), 1-101. doi: 10.1016/S0370-1573(02)00137-0
![]() |
[26] |
A. Arenas, A. Díaz-Guilera, J. Kurths, Y. Moreno, C. Zhou, Synchronization in complex networks, Phys. Rep., 469 (2008), 93-153. doi: 10.1016/j.physrep.2008.09.002
![]() |
[27] |
K. Rajagopal, S. Jafari, A. Karthikeyan, A. Srinivasan, Effect of magnetic induction on the synchronizability of coupled neuron network, Chaos: Interdiscip. J. Nonlinear Sci., 31 (2021), 083115. doi: 10.1063/5.0061406
![]() |
[28] |
J. Fell, N. Axmacher, The role of phase synchronization in memory processes, Nat. Rev. Neurosci., 12 (2011), 105-118. doi: 10.1038/nrn2979
![]() |
[29] | G. Arnulfo, S. H. Wang, V. Myrov, B. Toselli, J. Hirvonen, M. Fato, et al., Long-range phase synchronization of high-frequency oscillations in human cortex, Nat. Commun., 11 (2020), 1-15. |
[30] |
C. A. Bosman, C. S. Lansink, C. M. Pennartz, Functions of gamma‐band synchronization in cognition: From single circuits to functional diversity across cortical and subcortical systems, Eur. J. Neurosci., 39 (2014), 1982-1999. doi: 10.1111/ejn.12606
![]() |
[31] |
C. A. Bosman, J. M. Schoffelen, N. Brunet, R. Oostenveld, A. M. Bastos, T. Womelsdorf, et al., Attentional stimulus selection through selective synchronization between monkey visual areas, Neuron, 75 (2012), 875-888. doi: 10.1016/j.neuron.2012.06.037
![]() |
[32] |
P. Jiruska, M. De Curtis, J. G. Jefferys, C. A. Schevon, S. J. Schiff, K. Schindler, Synchronization and desynchronization in epilepsy: controversies and hypotheses, J. Physiol., 591 (2013), 787-797. doi: 10.1113/jphysiol.2012.239590
![]() |
[33] | T. Wang, H. Liao, Y. Zi, M. Wang, Z. Mao, Y. Xiang, et al., Distinct changes in global brain synchronization in early-onset vs. late-onset Parkinson disease, Front. Aging Neurosci., 12 (2020). |
[34] | F. Parastesh, S. Jafari, H. Azarnoush, Z. Shahriari, Z. Wang, S. Boccaletti, Chimeras, Phys. Rep., 2020. |
[35] |
A. zur Bonsen, I. Omelchenko, A. Zakharova, E. Schöll, Chimera states in networks of logistic maps with hierarchical connectivities, Eur. Phys. J. B, 91 (2018), 1-12. doi: 10.1140/epjb/e2017-80535-3
![]() |
[36] |
E. Rybalova, T. Vadivasova, G. Strelkova, V. S. Anishchenko, A. Zakharova, Forced synchronization of a multilayer heterogeneous network of chaotic maps in the chimera state mode, Chaos: Interdiscip. J. Nonlinear Sci., 29 (2019), 033134. doi: 10.1063/1.5090184
![]() |
[37] |
M. Winkler, J. Sawicki, I. Omelchenko, A. Zakharova, V. Anishchenko, E. Schöll, Relay synchronization in multiplex networks of discrete maps, EPL, 126 (2019), 50004. doi: 10.1209/0295-5075/126/50004
![]() |
[38] | Y. Kuramoto, D. Battogtokh, Coexistence of coherence and incoherence in nonlocally coupled phase oscillators, Nonlinear Phenom. Complex Syst., 5 (2002), 380-385. |
[39] |
S. Nkomo, M. R. Tinsley, K. Showalter, Chimera and chimera-like states in populations of nonlocally coupled homogeneous and heterogeneous chemical oscillators, Chaos: Interdiscip. J. Nonlinear Sci., 26 (2016), 094826. doi: 10.1063/1.4962631
![]() |
[40] |
L. V. Gambuzza, A. Buscarino, S. Chessari, L. Fortuna, R. Meucci, M. Frasca, Experimental investigation of chimera states with quiescent and synchronous domains in coupled electronic oscillators, Phys. Rev. E, 90 (2014), 032905. doi: 10.1103/PhysRevE.90.032905
![]() |
[41] |
B. K. Bera, D. Ghosh, M. Lakshmanan, Chimera states in bursting neurons, Phys. Rev. E, 93 (2016), 012205. doi: 10.1103/PhysRevE.93.012205
![]() |
[42] |
S. Majhi, M. Perc, D. Ghosh, Chimera states in uncoupled neurons induced by a multilayer structure, Sci. Rep., 6 (2016), 1-11. doi: 10.1038/s41598-016-0001-8
![]() |
[43] |
I. A. Shepelev, T. E. Vadivasova, A. Bukh, G. Strelkova, V. Anishchenko, New type of chimera structures in a ring of bistable FitzHugh-Nagumo oscillators with nonlocal interaction, Phys. Lett. A, 381 (2017), 1398-1404. doi: 10.1016/j.physleta.2017.02.034
![]() |
[44] | V. Dos Santos, F. S. Borges, K. C. Iarosz, I. L. Caldas, J. Szezech, R. L. Viana, et al., Basin of attraction for chimera states in a network of Rössler oscillators, Chaos: Interdiscip. J. Nonlinear Sci., 30 (2020), 083115. |
[45] |
B. K. Bera, S. Majhi, D. Ghosh, M. Perc, Chimera states: effects of different coupling topologies, EPL, 118 (2017), 10001. doi: 10.1209/0295-5075/118/10001
![]() |
[46] |
U. K. Verma, G. Ambika, Amplitude chimera and chimera death induced by external agents in two-layer networks, Chaos: Interdiscip. J. Nonlinear Sci., 30 (2020), 043104. doi: 10.1063/5.0002457
![]() |
[47] |
B. K. Bera, D. Ghosh, T. Banerjee, Imperfect traveling chimera states induced by local synaptic gradient coupling, Phys. Rev. E, 94 (2016), 012215. doi: 10.1103/PhysRevE.94.012215
![]() |
[48] |
I. A. Shepelev, A. V. Bukh, S. S. Muni, V. S. Anishchenko, Quantifying the transition from spiral waves to spiral wave chimeras in a lattice of self-sustained oscillators, Regular Chaotic Dyn., 25 (2020), 597-615. doi: 10.1134/S1560354720060076
![]() |
[49] |
G. R. Simo, P. Louodop, D. Ghosh, T. Njougouo, R. Tchitnga, H. A. Cerdeira, Traveling chimera patterns in a two-dimensional neuronal network, Phys. Lett. A, 409 (2021), 127519. doi: 10.1016/j.physleta.2021.127519
![]() |
[50] |
G. R. Simo, T. Njougouo, R. Aristides, P. Louodop, R. Tchitnga, H. A. Cerdeira, Chimera states in a neuronal network under the action of an electric field, Phys. Rev. E, 103 (2021), 062304. doi: 10.1103/PhysRevE.103.062304
![]() |