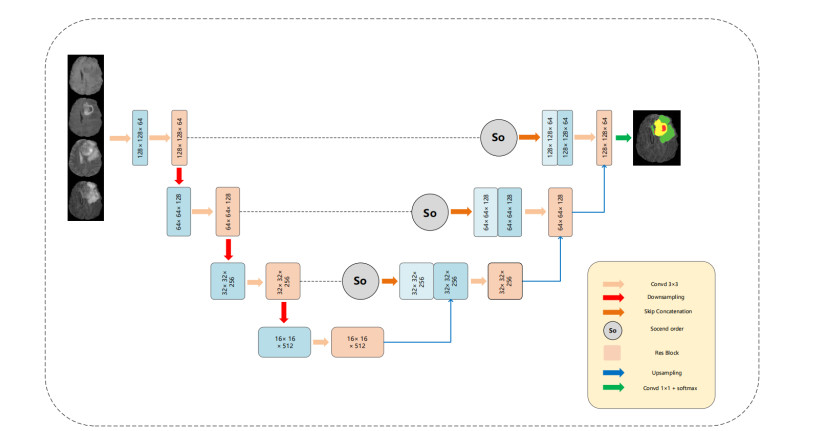
Tumor segmentation using magnetic resonance imaging (MRI) plays a significant role in assisting brain tumor diagnosis and treatment. Recently, U-Net architecture with its variants have become prevalent in the field of brain tumor segmentation. However, the existing U-Net models mainly exploit coarse first-order features for tumor segmentation, and they seldom consider the more powerful second-order statistics of deep features. Therefore, in this work, we aim to explore the effectiveness of second-order statistical features for brain tumor segmentation application, and further propose a novel second-order residual brain tumor segmentation network, i.e., SoResU-Net. SoResU-Net utilizes a number of second-order modules to replace the original skip connection operations, thus augmenting the series of transformation operations and increasing the non-linearity of the segmentation network. Extensive experimental results on the BraTS 2018 and BraTS 2019 datasets demonstrate that SoResU-Net outperforms its baseline, especially on core tumor and enhancing tumor segmentation, illuminating the effectiveness of second-order statistical features for the brain tumor segmentation application.
Citation: Ning Sheng, Dongwei Liu, Jianxia Zhang, Chao Che, Jianxin Zhang. Second-order ResU-Net for automatic MRI brain tumor segmentation[J]. Mathematical Biosciences and Engineering, 2021, 18(5): 4943-4960. doi: 10.3934/mbe.2021251
[1] | Jiajun Zhu, Rui Zhang, Haifei Zhang . An MRI brain tumor segmentation method based on improved U-Net. Mathematical Biosciences and Engineering, 2024, 21(1): 778-791. doi: 10.3934/mbe.2024033 |
[2] | Yuqing Zhang, Yutong Han, Jianxin Zhang . MAU-Net: Mixed attention U-Net for MRI brain tumor segmentation. Mathematical Biosciences and Engineering, 2023, 20(12): 20510-20527. doi: 10.3934/mbe.2023907 |
[3] | Dongwei Liu, Ning Sheng, Tao He, Wei Wang, Jianxia Zhang, Jianxin Zhang . SGEResU-Net for brain tumor segmentation. Mathematical Biosciences and Engineering, 2022, 19(6): 5576-5590. doi: 10.3934/mbe.2022261 |
[4] | Qian Wu, Yuyao Pei, Zihao Cheng, Xiaopeng Hu, Changqing Wang . SDS-Net: A lightweight 3D convolutional neural network with multi-branch attention for multimodal brain tumor accurate segmentation. Mathematical Biosciences and Engineering, 2023, 20(9): 17384-17406. doi: 10.3934/mbe.2023773 |
[5] | Xiaoli Zhang, Kunmeng Liu, Kuixing Zhang, Xiang Li, Zhaocai Sun, Benzheng Wei . SAMS-Net: Fusion of attention mechanism and multi-scale features network for tumor infiltrating lymphocytes segmentation. Mathematical Biosciences and Engineering, 2023, 20(2): 2964-2979. doi: 10.3934/mbe.2023140 |
[6] | Peiqing Lv, Jinke Wang, Xiangyang Zhang, Chunlei Ji, Lubiao Zhou, Haiying Wang . An improved residual U-Net with morphological-based loss function for automatic liver segmentation in computed tomography. Mathematical Biosciences and Engineering, 2022, 19(2): 1426-1447. doi: 10.3934/mbe.2022066 |
[7] | Xiaoyan Zhang, Mengmeng He, Hongan Li . DAU-Net: A medical image segmentation network combining the Hadamard product and dual scale attention gate. Mathematical Biosciences and Engineering, 2024, 21(2): 2753-2767. doi: 10.3934/mbe.2024122 |
[8] | Hong'an Li, Man Liu, Jiangwen Fan, Qingfang Liu . Biomedical image segmentation algorithm based on dense atrous convolution. Mathematical Biosciences and Engineering, 2024, 21(3): 4351-4369. doi: 10.3934/mbe.2024192 |
[9] | Tong Shan, Jiayong Yan, Xiaoyao Cui, Lijian Xie . DSCA-Net: A depthwise separable convolutional neural network with attention mechanism for medical image segmentation. Mathematical Biosciences and Engineering, 2023, 20(1): 365-382. doi: 10.3934/mbe.2023017 |
[10] | Jianhua Song, Lei Yuan . Brain tissue segmentation via non-local fuzzy c-means clustering combined with Markov random field. Mathematical Biosciences and Engineering, 2022, 19(2): 1891-1908. doi: 10.3934/mbe.2022089 |
Tumor segmentation using magnetic resonance imaging (MRI) plays a significant role in assisting brain tumor diagnosis and treatment. Recently, U-Net architecture with its variants have become prevalent in the field of brain tumor segmentation. However, the existing U-Net models mainly exploit coarse first-order features for tumor segmentation, and they seldom consider the more powerful second-order statistics of deep features. Therefore, in this work, we aim to explore the effectiveness of second-order statistical features for brain tumor segmentation application, and further propose a novel second-order residual brain tumor segmentation network, i.e., SoResU-Net. SoResU-Net utilizes a number of second-order modules to replace the original skip connection operations, thus augmenting the series of transformation operations and increasing the non-linearity of the segmentation network. Extensive experimental results on the BraTS 2018 and BraTS 2019 datasets demonstrate that SoResU-Net outperforms its baseline, especially on core tumor and enhancing tumor segmentation, illuminating the effectiveness of second-order statistical features for the brain tumor segmentation application.
Brain tumors are abnormal cells that grow in the brain or skull, including benign and malignant tumors [1]. The incidence of malignant tumors is higher than the former, which seriously endanger human health and lives. The most common malignant brain tumor is glioma, and it can be further divided into high-grade glioma (HGG) and low-grade glioma (LGG) according to the degree of infiltration. Magnetic resource imaging (MRI), as a non-invasive and favorable soft tissue contrast imaging manner, can provide valuable information on the shape, size and location of brain tumors for diagnosis and treatment. Brain tumor segmentation based on MRI, as a essential process in brain tumor diagnosis and treatment [2], has also been receiving wide attention for decades.
Recently, with the great success of deep neural network (DNN) [3,4,5] applied in various computer vision tasks and medical image analysis problems, computer-aided diagnosis of MRI brain tumors based on deep learning has gradually became a hot topic and achieved breakthrough development. Particularly, the Multimodal Brain Tumor Segmentation challenge (BraTS), which has been held since 2012 in conjunction with the International Association for Medical Image Computing and Computer Assisted Intervention (MICCAI) [6], greatly promotes the development of deep learning and brain tumor segmentation methods. Generally speaking, the existing deep neural network based on brain tumor segmentation methods mainly consist of two categories: i.e., convolutional neural network (CNN)-based methods and fully convolutional network (FCN)-based methods. Brain tumor segmentation based on convolutional neural network adopts the classification of small-scale image blocks to design the MRI brain tumor segmentation network, which further divides into single-path and multi-paths models. Pereiraet et al. [7] explore an automatic segmentation method based on a deep convolutional neural network with 3$ \times small cores, efficiently reducing the possible of overfitting. Compared with single-path CNN, multi-paths convolutional neural networks can gain diverse information from different paths and summarize them. For instance, Moeskops et al. [8] employ a CNN network composed of three patch paths with different sizes in order to accept more details and maintain spatial consistency for brain tumor segmentation. However, due to the inefficiency and huge memory burden of the convolutional neural network, FCN-based brain tumor segmentation networks have obtained increasing popularity by researchers. FCN [9] adopts the structure of encoder and decoder for pixel-level classification to solve the problem of semantic image segmentation, which can be considered as an crucial milestone of image semantic segmentation tasks. Then Ronneberger et al. propose an improved version of FCN, i.e., U-Net [10]. The U-Net structure contains the down-sampling layer in the feature learning module, the up-sampling layer in the feature recovery module and horizontal links to perfectly combine them, which enables the excellent adaptation to medical image segmentation and became one of the current mainstream algorithms for brain tumor segmentation [11,12,13]. For example, Fabian et al. [14] only employ the traditional U-Net with improving the process of preprocessing, training and post-processing (NNU-Net), which wins the runner-up of the BraTS 2018 challenge. Thereafter, modified U-Nets with more complex structures have been attempted to probe its deeper potential. The BraTS 2018 champion team from Nvidia [15], adopt a multi-branches structure with a VAE module to significantly improve the performance of network. Jiang et al. [16] devise a novel two-stages cascaded U-Net to segment brain tumors from coarse to fine, which gains the first place in the BraTS 2019 competition.
In view of the complex feature distribution in brain tumors and the limitation of the number of medical image databases, only extracting plain first-order information makes it difficult to meet the demands of tumor segmentation and fully realize the potential of networks [17]. Recently, the concept of high-order has been commonly used in natural images, demonstrating the discriminant representations of global high-order statistics of deep features. Cimpoi et al. [18] propose a new texture descriptor named FV-CNN which combines high-order information with convolutional neural networks and achieves impressive recognition accuracy on slight texture recognition with sufficient labeled data is not available. Lin et al. [19] build a representative bilinear CNN model (BCNN), which captures the pairwise correlation of feature channels by performing an outer product. The output can capture the pairwise correlation among feature channels. In this way, the model could identify the trifling gaps between two objects which are particularly useful for fine-grained categorization. Chen et al. [20] compute complex high-order statistical information through the inner product, capturing small differences between pedestrians by mixing first-order, second-order or even higher-order related information which achieves an excellent outcome. Then, for strengthening the robustness of high-order information, researchers try to combine them with other methods [21,22], which have achieved impressive performance on lots of visual tasks as well, powerfully confirming the effectiveness that second-order modeling could get high-order statistics beyond the first-order. Inspired by this, we try to embed the second-order module into the brain tumor segmentation model to improve the accuracy of the brain tumor segmentation task, and propose a novel second-order residual U-Net model called SoResU-Net. Experimental results on two brain tumor segmentation datasets illuminate the benefit of the second-order module to brain tumor segmentation, especially for small-scale tumors. The overview of our SoResU-Net is shown in Figure 1, and its core module i.e., second-order (so) is presented in Figure 2. The main contributions of this paper are summarized on three aspects: (1) We introduce the high-order concept into brain tumor segmentation tasks, and built a high-order module named second-order. (2) Combining second-order module and residual module with the mainstream model of brain tumor segmentation, i.e., U-Net, to construct a new end-to-end model, named SoResU-Net for MRI brain tumor segmentation tasks. SoResU-Net not only extracts richer high-order semantic information, but also pays more attention to small-scale brain tumors. (3) Extensively evaluating SoResU-Net on the two brain tumor segmentation benchmarks of BraTS 2018 and BraTS 2019, whose results show the competitive performance of our method on brain tumor segmentation. Moreover, to prove the excellent generalization of our module, we also perform experiments of embedding second-order module into the U-Net model which is named SoU-Net. Experimental results show that both two models are better than their first-order baselines (i.e., ResU-Net and U-Net) respectively.
In this section, we first introduce details of SoResU-Net for brain tumor segmentation. Then, the basic theories of second-order and the combined loss function we adopted for our model is described.
On brain tumor segmentation task, the existence of abnormal tissues may be easily detectable in most cases. However, accurate, reproducible segmentation and characterization of anomalies is not straightforward [23], especially for small-scale brain tumors. On one hand, small-scale brain tumors have low presences and blurred boundaries due to the complex morphology and diverse sizes of brain tumors. On the other hand, during the network up-sampling and down-sampling, the successive cascading convolution transformation process will cause the loss of high-order spatial information and position details. Consequently, to solve these challenges, we integrate the second-order module and the residual module into the U-Net architecture to generate a new second-order ResU-Net model, which can effectively attain context-related information and take advantage of the high-order statistical information to compensate for the loss information. Figure 1 demonstrates the overall architecture of SoResU-Net. As shown in Figure 1, SoResU-Net employs a traditional encoder-decoder architecture which consists of an image contracting path (encoder on the left) and an image expanding path (decoder on the right). The input of the network is 128×128×4 where the size of each image is 128×128 and channel is 4.
In the image contracting path, SoResU-Net utilizes three residual blocks to replace the max pooling component used for down-sampling in the U-Net model to avoid gradient disappearance and accelerate network fusion. Concretely speaking, the size of each image will be reduced to half and the channels will extend to double of original when enters the down-sampling layer. Meanwhile, for increasing non-linearity and model convergence speed, each layer of our models contains follow-up activation functions and normalization processes. The image expansion path is structurally consistent with the former. Notably, SoResU-Net utilizes a second-order module in the horizontal connection to replace the original residual connection, which explores the second-order statistics through the inner product operator that receives two activation tensors from two 1×1 convolutional layers performed on input tensors. The intention of 1×1 convolutional layer is to extend the first-order channel information. Additionally, we use an identity shortcut connection to add input tensor and scaled one for strengthening information propagation. Thereby, improved segmentation accuracy of small-scale brain tumors can be obtained. At the last layer of the model, the multi-channels feature map is mapped to the corresponding category via a 1×1 convolution with softmax activation function.
The main function of the second-order module is to model a complex high-order interaction of activation X [20]. For further explanation, we define a high-order statistic linear polynomial predictor for variable X:
f(x)=R∑r=1⟨wr,⊗rx⟩ | (2.1) |
where R is the number of order, ⟨.,.⟩ represents inner product of two same size tensors, x∈RC denotes a local feature and ⊗rx is the r-th order self-outer product of x that can model all the degree-r interactions, and wr is the r-th weight tensor containing degree-r homogeneous polynomial predictor. Obviously, once the value of r in equation (2.1) is too large, wr will produce too many parameters that may cause the model overfitting, so we set r to an appropriate value after weighing. Meanwhile, we suppose that wr can be approximated by Dr rank-1 tensors when r>1 and define wr=∑Drd=1ar,dur,d1⊗⋯⊗ur,dr. For more details, when r>1, where ar,d is the weight of d-th rank-1 tensor, ur,dr is a C−dimensional vector, and ⊗ indicates the outer product. Thus, equation (2.1) can be reformulated as:
f(x)=R∑r=2⟨Dr∑d=1ar,dur,d1⊗⋯⊗ur,dr,⊗rx⟩ | (2.2) |
to facilitate understanding, some operations can be further applied to simplify equation (2.2) as:
f(x)=R∑r=2Dr∑d=1ar,dr∏s=1⟨ur,ds,x⟩=R∑r=2⟨αr,zr⟩ | (2.3) |
where αr=[αr,1,⋯,αr,Dr]T is the weight vector and zr=[zr,1,⋯,zr,Dr]T∈RDr with each entry zr,d=∏rs=1⟨ur,ds,x⟩. If operating it in an inner product form, equation (2.3) can be rewritten as:
f(x)=R∑r=2vT(αr⊙zr) | (2.4) |
where ⊙ is element-wise product and vT is a row vector of ones. In equation (2.4), f(x) represents the high-order statistics, and we normalize the output result and apply the relu activation function to further increase non-linearity:
F(x)=σ(f(x)) | (2.5) |
where σ denotes relu activation function and we use residual short connections to reduce the network degradation problem:
A(x)=(w,x)+F(x) | (2.6) |
where (w,x) indicates a linear predictor on the first-order statistics of X, the + is the element-wise addition that integrates first-order statistics and second-order statistics by an identity shortcut connection. A(x) is the output of second-order module and sequentially feeds to the next convolutional layer.
The segmentation task of brain tumors suffers from a serious class imbalance problem. Overall, about 98.46% part of the voxels belong to healthy tissue, only 0.23% voxels belong to necrosis and non-enhancing, edema account for 1.02% and enhancing tumors are 0.29%, respectively. Therefore, we additionally employ generalized dice loss (GDL) [24] and weighted cross entropy loss (WCE) [25] for better estimating and predicting tasks, the combined loss function Loss can be defined as follows:
Loss=LGDL+LWCE | (2.7) |
LGDL represents generalized dice loss (GDL), which is a commonly used medical image segmentation loss function, allowing the model to focus on learning samples that are difficult to learn. In this way, our combined loss could relatively enlarge the gradient of difficult-to-classify samples as well as relatively reduce the gradient of easy-to-classify samples, alleviating the problem of category imbalance to a certain extent. where LWCE represents the weighted cross entropy loss (WCE), which is considered as a good solution to the problem of multi-task imbalance, decreasing the difference between the training samples and evaluation metric. The two parameters are defined as follows:
LGDL=1−2∑Liwigipi∑Liwi(gi+pi) | (2.8) |
LWCE=−L∑iwigilog(pi) | (2.9) |
where L indicates the total number of labels, wi is the weight allocated to the i-th label, pi and gi denote the pixel value of the segmented binary image and the binary ground truth image, respectively.
In this section, we first introduce the two brain tumor segmentation datasets adopted for the model evaluation. After that, the data preprocessing, evaluation metrics and implementation details are briefly described.
We utilize the BraTS 2018 dataset and the BraTS 2019 dataset to evaluate the performance of our model. The BraTS 2018 dataset consists of 285 training cases containing 210 high grade glioma (HGG) cases and 75 low grade glioma (LGG) cases, and the validation includes 66 validation cases of unknown grade. With respect to the BraTS 2019 dataset, the organizers of the competition have greatly expanded it which contains 335 training cases, including 259 HGG patients and 75 LGG patients, while its validation contains 125 patient cases with unknown grade, thus we pay more attention on the BraTS 2019 dataset as well as conduct core experiments on it. All brain images are stripped of the skull and have the same orientation. For each patient case, there are four MRI modalities, i.e., Flair, T1, T1ce, and T2. To homogenize these data, all modalities are registered together to the T1c sequence and re-sampled to 1 mm isotropic resolution on a normalized axis which uses a linear interpolator. The labels are divided into four classes named as healthy tissues (label 0), necrosis and non-enhancing (label 1), edema (label 2), and enhancing tumor (label 4). Figure 3 illuminates a typical case of MRI brain image along with the ground truth. Note that the ground truth of the validation dataset is not provided. Therefore, researchers have to upload their predicted results to the online website and obtain the final evaluation result from the competition, which well ensures the fairness and authority of evaluation result.
As shown in Figure 3, the ground truth of each image is marked by the manual segmentation result given by the expert. The basic labels include four types, which are called healthy parts (label 0), necrotic and non-enhancing tumors (label 1), edema around the tumor (label 2), and GD enhancing tumors (label 4). According to these labels, three main assessment contents can be drawn, named the whole tumor (the combined area of labels 1, 2, and 4), the core tumor (the combined area of labels 1, 4) and the enhancing tumor (the area of label 4).
Due to the uncertainty of brain tumor morphology, location, blurring of boundaries, and manual annotation deviation, data processing of brain tumor images is particularly important. In this work, we adopt multi-modality 3D MRI brain to scan two years BraTS datasets, in brain tumor images, the size of each 3D MRI image data is 240×240×155. These pictures contain valid information and a lot of useless background parts, so we first reduce the size of the 3D image to 146×192×152 to eliminate some unwanted background areas and reduce the amount of calculation. However, for multi-label brain tumors, healthy pixels account for 98% and abnormal pixels account for only 2%, this serious category imbalance requires data to be further refined. Secondly, we clip the highest 1% voxels and the lowest 1% voxels of the 3D image to eliminate the influence of some extreme values. Next, each 3D image is sliced into multiple 2D images on which we extract 128x128 size pixels so as to increase the ratio of effective pixels. Moreover, in order to reduce the influence of different institutions, scanners, and data collected by different protocols, we use the z-score method to regularize the unstandardized brain tumors. The z-score normalization applies the average and standard deviation to process each images, the calculation formula is as follows:
z′=z−μδ | (3.1) |
where z is the input image, z′ is the normalized image. μ and δ denote the mean value and standard deviation of the input image, respectively.
In order to evaluate the effectiveness of our models, we utilise the Dice Similarity Coefficient (DSC) and Hausdorff distance (HD) which are commonly used for brain tumor segmentation to estimate its segmentation effect. DSC and Hausdorff95 metric are defined as follows:
DSC=2TPFP+2TP+FN | (3.2) |
HD(T,P)=max{supt∈Tinfp∈Pd(t,p),supp∈Pinft∈Td(t,p)} | (3.3) |
In definitions (3.2), The parameters TP, FP, TN and FN represents the number of false negative, true negative, true positive and false positive voxels, respectively. In the other word, TP is the total number of pixels correctly classified as brain tumor by the deep learning model, while FP is the number of pixels incorrectly classified. TN and FN are defined as the total number of pixels correctly and incorrectly classified as non-brain tumor by the model. The DSC is a Metric of ensemble similarity. It is usually used to calculate the similarity of two samples. The value range is 0-1. The best segmentation result is 1 and the worst is 0.
Another outstanding indicator is Hausdorff95, in definitions (3.3), t and p represent the points on the surface T of the ground truth regions and the surface P of the predicted regions, respectively. d(t, p) is the function that computes the distance between points of t and p. Hausdorff95 is a metric of Hausdorff distance to measure the 95% quantile of the surface distance, which is very sensitive to the divided boundary and often used to assist DSC indicator to measure the performance of the model.
Our models are conducted in Keras 2.2.4 with the Tensorflow as the backend. Running on a cluster equipped with 32 GB RAM and Tesla V100 GPU. Every model uses stochastic gradient descent (SGD) algorithm as the optimizer, its initial learning rate is 0.085, momentum is 0.95, and the weight decay is 5e−6. In addition, we utilize the batch size of patch slices for training and use a combined loss function. The size of each input block is 128×128×4. The size of each input block is 1 pixels. Training from the beginning of the network with a batch size is 10, and set 5 cycles to ensure that best experimental results are used.
At first, we divided the experiment into four parts, i.e., using BraTS 2019 training dataset, BraTS 2019 validation dataset and the BraTS 2018 validation dataset as a supplementary experiment to evaluate our model, Finally, in order to better verify the generalization of our module, we conduct experiments on two datasets which replace the second-order module with the residual module in ResU-Net. It is worth noting that we apply a five-fold cross-validation method on the BraTS 2019 training dataset. We used 80% for training, the remaining 20% for validation and repeat this process five times. At the same time, the experimental results of BraTS 2018 and BraTS 2019 validation are gained through the BraTS online website to ensure the authority and validity of our conclusions. Finally, we conduct ablation experiments to discuss our essential module, i.e., second-order module, verifying the influence of the number of channels for the module.
Firstly, we compare our models with two baseline models, i.e., U-Net and ResU-Net, on the BraTS 2019 training dataset by using five-fold cross-validation. More specifically, we split the 2019 BraTS training dataset into five fixed sub-datasets, where four sub-datasets (including 80% images) are utilized to train segmentation network and the remaining 20% images are for model validation, and the training and validation processes are repeated for five times using various sub-datasets to achieve the statistical results. We report the compared results on this dataset in Table 1.
Methods | DSC | Hausdorff95 | ||||
Whole | Core | Enhancing | Whole | Core | Enhancing | |
U-Net | 0.872 | 0.774 | 0.689 | 8.79 | 7.62 | 3.65 |
SoU-Net | 0.875 | 0.786 | 0.698 | 8.59 | 7.35 | 3.57 |
ResU-Net | 0.877 | 0.782 | 0.694 | 8.59 | 7.36 | 3.67 |
SoResU-Net | 0.881 | 0.796 | 0.707 | 8.40 | 7.06 | 3.48 |
It can be seen that SoResU-Net gains the highest score among the four models listed in Table 1, which achieves DSC of 0.881, 0.796, 0.707 on the whole tumor, core tumor and enhancing tumor, respectively, exceeding its first-order baseline, i.e., ResU-Net 0.4%, 1.4% and 1.3%. Similarly, for another set of experiments, SoU-Net and U-Net, SoU-Net achieves DSC sores of 0.875, 0.786, 0.698 on the whole tumor, core tumor and enhancing tumor segmentation respectively and increases by 0.3%, 1.2% and 0.9% comparing with the basic model U-Net. As for Hausdorff95, relative to the original model, both improved models reduce a certain value. The results illuminate that embedding a second-order module into ResU-Net and U-Net can benefit the brain tumor segmentation owing to rich statistical information of high-order module.
Moreover, we also visualize the brain tumor segmentation results of the four models by applying various colors to represent different tumor classes for the brain tumor segmented images and covering on the original brain image. Figure 4 illuminates several typical samples with corresponding segmentation results. In Figure 4, the red regions are necrosis and non-enhancing, the green regions indicate edema, and the yellow regions represent enhancing tumor. Meanwhile, images from left to right are Ground Truth, U-Net, SoU-Net, ResU-Net and SoResU-Net segmentation results overlaid on flair image, respectively. As can be observed, we can conclude that SoResU-Net achieves the best segmentation results of brain tumors among our models.
Here, we perform compared experiments with two baselines on the BraTS 2019 validation dataset, which will further demonstrate the effectiveness of the second-order statistics on this medical image analysis application. Table 2 lists the comparison results on the BraTS 2019 validation dataset.
Methods | DSC | Hausdorff95 | ||||
Whole | Core | Enhancing | Whole | Core | Enhancing | |
U-Net | 0.865 | 0.748 | 0.678 | 12.73 | 14.16 | 7.25 |
SoU-Net | 0.867 | 0.766 | 0.693 | 12.30 | 12.73 | 7.08 |
ResU-Net | 0.871 | 0.766 | 0.701 | 9.71 | 12.21 | 6.14 |
SoResU-Net | 0.875 | 0.788 | 0.724 | 9.35 | 11.47 | 5.97 |
It can be observed from Table 2 that SoResU-Net still shows the best performance among the four models, which achieves DSC of 0.875, 0.788, 0.724 on the whole tumor, core tumor and enhancing tumor, respectively, superior to the basic model ResU-Net by 0.40%, 2.20% and 2.30%. For another set of compared models, SoU-Net and U-Net, SoU-Net achieves DSC sores of 0.867, 0.766, and 0.693 for the whole tumor, core tumor and enhancing tumor segmentation, respectively. Comparing with the the performance of basic model U-Net, SoU-Net increases by 0.20%, 1.80% and 1.50% on the whole tumor, core tumor and enhancing tumor segmentation, respectively. In particular, the obvious promotion obtained by our two improved models on the core tumors and enhancing tumors reaches 2.20% and 2.30%, 1.80% and 1.50%, respectively, demonstrating the effectiveness of second-order module on small-scale tumors. In addition, Hausdorff95 index also shows that embedding our module can improve the competitiveness of model and sensitivity to boundaries. For more evident comparisons, Figure 5 shows the bar plots of the DSC and Hausdorff95 scores for the three tumor regions on BraTS 2019 validation dataset.
Besides, we compare the results of the BraTS 2019 validation with some typical methods. Table 3 shows the compared results, which indicate our SoResU-Net model could acquire competitive performance on DSC scores. Considering the limitation of the memory sizes of the GPU device, we only utilize the 2D slice of the brain image to segment the brain tumor, which might reduce our model performance to some extent and explain the reason for our SoResU-Net ranking second place on core tumor segmentation that slightly weaker than the methods of Tai et al. [27]. It is worth noting that our 2D SoResU-Net can achieve competitive performance compared with the some other 3D models and obtain the highest result on enhancing tumor segmentation on DSC metric. Similarly, despite the mediocre performance of our 2D model on whole tumor segmentation and core tumor segmentation on Hausdorff95, SoResU-Net still gains the lowest value on enhancing tumor segmentation. The above comparisons prove the effectiveness of second-order, confirming that SoResU-Net could dramatically improve the segmentation performance of small-scale tumors.
Methods | DSC | Hausdorff95 | ||||
Whole | Core | Enhancing | Whole | Core | Enhancing | |
Bhalerao et al. [26] | 0.852 | 0.709 | 0.666 | 8.07 | 9.57 | 7.27 |
Tai et al. [27] | 0.843 | 0.804 | 0.684 | - | - | - |
Baid et al. [28] | 0.87 | 0.77 | 0.70 | 13.36 | 12.71 | 6.45 |
Rehman et al. [29] | 0.869 | 0.775 | 0.708 | - | - | - |
Jiang et al. [30] | 0.870 | 0.777 | 0.709 | 9.07 | 11.40 | 6.49 |
Amian et al. [31] | 0.86 | 0.77 | 0.71 | 8.42 | 11.55 | 6.92 |
ours | 0.875 | 0.788 | 0.724 | 9.35 | 11.47 | 5.97 |
To further demonstrate the effectiveness of our modules, we also employ the BraTS 2018 validation dataset to compare SoResU-Net, SoU-Net with their baselines, and the compared experimental results on this validation dataset are described in Table 4.
Methods | DSC | Hausdorff95 | ||||
Whole | Core | Enhancing | Whole | Core | Enhancing | |
U-Net | 0.865 | 0.782 | 0.743 | 11.31 | 15.34 | 3.79 |
SoU-Net | 0.868 | 0.797 | 0.755 | 10.81 | 13.94 | 3.65 |
ResU-Net | 0.871 | 0.790 | 0.751 | 8.89 | 11.71 | 3.51 |
SoResU-Net | 0.876 | 0.811 | 0.771 | 8.42 | 10.56 | 3.22 |
From Table 4, we can see that SoResU-Net performs best among the four models. SoResU-Net achieves DSC scores of 0.876, 0.811, and 0.771 for the whole tumor, core tumor and enhancing tumor segmentation, respectively. Comparing with its baseline, the performance of SoResU-Net on the whole tumor, core tumor and enhancing tumor segmentation increases by 0.50%, 2.10% and 2.00%, respectively. At the same time, our second-order module also shows a certainly enhanced performance in SoU-Net, and achieves average DSC scores of 0.868, 0.797, and 0.755 on the whole tumor, core tumor and enhancing tumor segmentation, which is respectively outperforms U-Net by 0.30%, 1.50% and 1.20%. Furthermore, The performance of the two sets of comparative experiments on Hausdorff95 can also prove that high-order statistical information is beneficial to improve the robustness of the model. In a word, the results of these comparisons clarify the effectiveness of our second-order module embedded in original ResU-Net model and U-Net model for brain tumor segmentation, especially to small-scale brain tumors.
Compared results on BraTS 2018 with other typical methods are given in Table 5. It can be obviously found that SoResU-Net gains an advantage in enhancing tumor of DSC scores while core tumor is slightly lower than the methods of Baid et al. [35]. For the indicator of Hausdorff95, consistent with BraTS 2019 validation, SoResU-Net obtains an excellent segmentation capability on enhancing tumor. In general, the compared results show the competitive performance of our 2D SoResU-Net and the effectiveness for segmenting small-scale brain tumors. At the same time, it can be concluded that embedding the second-order module into our networks could effectively improve segmentation performance.
Methods | DSC | Hausdorff95 | ||||
Whole | Core | Enhancing | Whole | Core | Enhancing | |
Hu et al. [32] | 0.882 | 0.748 | 0.718 | 12.60 | 9.62 | 5.69 |
Marcinkiewicz et al. [33] | 0.862 | 0.768 | 0.723 | - | - | - |
Chandra et al. [34] | 0.872 | 0.795 | 0.741 | 5.04 | 9.59 | 5.58 |
Baid et al. [35] | 0.878 | 0.826 | 0.748 | 16.81 | 11.20 | 7.29 |
Çiçek et al. [36] | 0.885 | 0.718 | 0.760 | 17.10 | 11.60 | 6.04 |
ours | 0.876 | 0.811 | 0.771 | 8.42 | 10.56 | 3.22 |
Due to the more cases of BraTS 2019 validation dataset and the results are more comparative, we explore the relationship between the number of channels of 1 × 1 convolutional with our second-order module on BraTS 2019 validation dataset. Considering that too many channels will cause over-fitting and excessive parameters, we respectively multiply the number of channel of 1× 1 convolutional filter by 1 time, 4 times, by 8 times, by 16 times. The bar graph result is shown in the Figure 6 and compared results are shown in Table 6.
Channels | Whole | Core | Enhancing |
1×channel | 0.873 | 0.778 | 0.702 |
4×channel | 0.872 | 0.784 | 0.709 |
8×channel | 0.873 | 0.786 | 0.715 |
16×channel | 0.875 | 0.788 | 0.724 |
The experimental results in Table 6 show that our methods can obtain the highest accuracy based on the number of channels that multiplies by 16 times, so the core part of our second-order module default to multiply the number of channels by 16 times.
Finally, to authenticate the generalization and effectiveness of our second-order module, we execute a set of supplementary experiments, that is, add the second-order module to the residual block in ResU-Net up-sampling and down-sampling to generate a new integrated model called ReSoU-Net. The experimental results are shown in Table 7.
Methods | Dataset | DSC | Hausdorff95 | ||||
Whole | Core | Enhancing | Whole | Core | Enhancing | ||
ResU-Net | BraTS 2018 | 0.871 | 0.790 | 0.751 | 8.89 | 11.71 | 3.51 |
ReSoU-Net | 0.873 | 0.808 | 0.766 | 8.78 | 10.94 | 3.22 | |
ResU-Net | BraTS 2019 | 0.871 | 0.766 | 0.701 | 9.71 | 12.21 | 6.14 |
ReSoU-Net | 0.876 | 0.782 | 0.717 | 8.75 | 11.29 | 5.24 |
As shown in Table 7, embedding the second-order module in the up-sampling and down-sampling processes of ResU-Net encourage better performance on both DSC and Hausdorff95 scores. On the BraTS 2018 validation dataset, ReSoU-Net achieves DSC scores of 0.873, 0.808 and 0.766 on the whole tumor, core tumor and enhancing tumor segmentation, outperforms ResU-Net by 0.20%, 1.80% and 1.50%, respectively. Moreover, in the BraTS 2019 validation dataset, ReSoU-Net achieves DSC scores of 0.876, 0.782, and 0.717 on the whole tumor, core tumor, and enhancing tumor segmentation, higher than the basic model by 0.50%, 1.60%, and 1.60%, respectively. When it comes to Hausdorff95 metric results, equivalently, ReSoU-Net makes progress on three areas. The results show that the second-order module can produce similar positive effects on ResU-Net no matter the embedded locations, proving the strong generalization of our second-order module.
In this article, we mainly exploit the effectiveness of the second-order module for brain tumor segmentation tasks and propose a SoResU-Net model. SoResU-Net replaces the horizontal link part of the baseline network with a second-order module to adapt the complex feature distribution of the brain tumor images. The second-order module enables our network to focus on small-scale tumors, which has certain significance for clinical practice. We evaluate the new model on two authoritative brain tumor datasets of BraTS 2018-2019. The experimental results show that SoResU-Net is better than its baseline, i.e., ResU-Net. However, since the 2D U-Net model and the ResU-Net model have a limitation in using the 3D information of MRI data, especially during the slicing process, a large amount of context information and local information between different slices will be lost. In the future, we will try to explore the 3D network architecture to improve the segmentation performance of SoU-Net or SoResU-Net, and expand the improved architecture to more datasets to show its generalization.
This work was partially supported by the National Natural Science Foundation of China (61972062), the Key R & D Program of Liaoning Province (2019 JH2/10100030), the Young and Middle-aged Talents Program of the National Civil Affairs Commission, the Liaoning BaiQianWan Talents Program, the University-Industry Collaborative Education Program (201902029013), and the Henan Provincial Department of Science and Technology Research Project (202102210168).
The authors have no conflict of interest.
[1] |
J. Liu, M. Li, J. Wang, F. Wu, Y. Pan, A survey of MRI-based brain tumor segmentation methods, Tsinghua Sci. Technol., 19 (2014), 578-595. doi: 10.1109/TST.2014.6961028
![]() |
[2] |
P. Y. Wen, D. R. Macdonald, D. A. Reardon, Updated response assessment criteria for high-grade gliomas: Response assessment in neuro-oncology working group, J. Clin. Oncol., 28 (2010), 1963-1972. doi: 10.1200/JCO.2009.26.3541
![]() |
[3] | K. Simonyan, A. Zisserman, Very deep convolutional networks for large-scale image recognition, preprint, arXiv: 1409.1556. |
[4] | K. He, X. Zhang, S. Ren, J. Sun, Deep residual learning for image recognition, in 2016 IEEE Conference on Computer Vision and Pattern Recognition, CVPR 2016, IEEE Computer Society, (2016), 770-778. |
[5] | G. Huang, Z. Liu, L. van der Maaten, K. Q. Weinberger, Densely connected convolutional networks, in 2017 IEEE Conference on Computer Vision and Pattern Recognition, CVPR 2017, IEEE Computer Society, (2017), 2261-2269. |
[6] | B. H. Menze, A. Jakab, S. Bauer, J. Kalpathy-Cramer, K. Farahani, J. Kirby, et al., The multimodal brain tumor image segmentation benchmark (BRATS), IEEE Transact. Med. Imag., 34 (2014), 1993-2024. |
[7] |
S. Pereira, A. Pinto, V. Alves, C. A. Silva, Brain tumor segmentation using convolutional neural networks in MRI images, IEEE Transact. Med. Imag., 35 (2016), 1240-1251. doi: 10.1109/TMI.2016.2538465
![]() |
[8] |
P. Moeskops, M. A. Viergever, A. M. Mendrik, L. S. De Vries, M. J. Benders, I. Išgum, Automatic segmentation of MR brain images with a convolutional neural network, IEEE Transact. Med. Imag., 35 (2016), 1252-1261. doi: 10.1109/TMI.2016.2548501
![]() |
[9] | J. Long, E. Shelhamer, T. Darrell, Fully convolutional networks for semantic segmentation, in IEEE Conference on Computer Vision and Pattern Recognition, CVPR 2015, IEEE Computer Society, (2015), 3431-3440. |
[10] | O. Ronneberger, P. Fischer, T. Brox, U-net: Convolutional networks for biomedical image segmentation, in Medical Image Computing and Computer-Assisted Intervention - MICCAI 2015-18th International Conference Munich, Lecture Notes in Computer Science, 9351, Springer, (2015), 234-241. |
[11] | H. Dong, G. Yang, F. Liu, Y. Mo, Y. Guo, Automatic brain tumor detection and segmentation using u-net based fully convolutional networks, in Medical Image Understanding and Analysis, 21st Annual Conference, MIUA 2017, Communications in Computer and Information Science, 723, Springer, (2017), 506-517. |
[12] |
N. M. Aboelenein, P. Songhao, A. Koubaa, A. Afifi, HTTU-Net: Hybrid Two Track U-Net for automatic brain tumor segmentation, IEEE Access, 8 (2020), 101406-101415. doi: 10.1109/ACCESS.2020.2998601
![]() |
[13] | X. Cheng, Z. Jiang, Q. Sun, Memory-Efficient Cascade 3D U-Net for Brain Tumor Segmentation, in Brainlesion: Glioma, Multiple Sclerosis, Stroke and Traumatic Brain Injuries 5th International Workshop, BrainLes 2019, Held in Conjunction with MICCAI 2019, Shenzhen, China, October 17, 2019, Revised Selected Papers, Part I, Lecture Notes in Computer Science, Springer, Cham, (2019), 242-253. |
[14] | F. Isensee, P. Kickingereder, W. Wick, M. Bendszus, K. H. Maier-Hein, No new-net, in Brainlesion: Glioma, Multiple Sclerosis, Stroke and Traumatic Brain Injuries - 4th International Workshop, BrainLes 2018, Held in Conjunction with MICCAI 2018, Granada, Spain, September 16, 2018, Revised Selected Papers, Part II, Lecture Notes in Computer Science, 11384, Springer, (2018), 234-244. |
[15] | A. Myronenko, 3D MRI brain tumor segmentation using autoencoder regularization, in Brainlesion: Glioma, Multiple Sclerosis, Stroke and Traumatic Brain Injuries--4th International Workshop, BrainLes 2018, Held in Conjunction with MICCAI 2018, Granada, Spain, September 16, 2018, Revised Selected Papers, Part II, Lecture Notes in Computer Science, 11384, Springer, (2018), 311-320. |
[16] | Z. Jiang, C. Ding, M. Liu, Two-Stage Cascaded U-Net: 1st Place Solution to BraTS Challenge 2019 Segmentation Task, in Brainlesion: Glioma, Multiple Sclerosis, Stroke and Traumatic Brain Injuries 5th International Workshop, BrainLes 2019, Held in Conjunction with MICCAI 2019, Shenzhen, China, October 17, 2019, Revised Selected Papers, Part I, Lecture Notes in Computer Science, 11992, Springer, (2019), 231-241. |
[17] | P. Li, J. Xie, Q. Wang, Is second-order information helpful for large-scale visual recognition?, in IEEE International Conference on Computer Vision, ICCV 2017, IEEE Computer Society, (2017), 2070-2078. |
[18] | M. Cimpoi, S. Maji, A. Vedaldi, Deep filter banks for texture recognition and segmentation, in IEEE Conference on Computer Vision and Pattern Recognition, CVPR 2015, IEEE Computer Society, (2015), 3828-3836. |
[19] | T. Y. Lin, A. Roy Chowdhury, S. Maji, Bilinear CNN models for fine-grained visual recognition, in IEEE Conference on Computer Vision and Pattern Recognition, CVPR 2015, IEEE Computer Society, (2015), 1449-1457. |
[20] | B. Chen, W. Deng, J. Hu, Mixed high-order attention network for person re-identification, in IEEE/CVF International Conference on Computer Vision, ICCV 2019, IEEE, (2019), 371-381. |
[21] | Q. Wang, P. Li, L. Zhang, G2DeNet: Global Gaussian distribution embedding network and its application to visual recognition, in 2017 IEEE Conference on Computer Vision and Pattern Recognition, CVPR2017, IEEE Computer Society, (2017), 2730-2739. |
[22] | A. Cherian, P. Koniusz, S. Gould, Higher-order pooling of CNN features via kernel linearization for action recognition in 2017 IEEE Winter Conference on Applications of Computer Vision (WACV), IEEE, (2017), 130-138. |
[23] |
N. Gordillo, E. Montseny, P. Sobrevilla, State of the art survey on MRI brain tumor segmentation, Magnet. Reson. Imag., 31 (2013), 1426-1438. doi: 10.1016/j.mri.2013.05.002
![]() |
[24] | C. H. Sudre, W. Li, T. Vercauteren, S. Ourselin, M. J. Cardoso, Generalised Dice Overlap as a Deep Learning Loss Function for Highly Unbalanced Segmentations, in Deep Learning in Medical Image Analysis and Multimodal Learning for Clinical Decision Support - Third International Workshop, DLMIA 2017, and 7th International Workshop, ML-CDS 2017, Held in Conjunction with MICCAI 2017, Lecture Notes in Computer Science, 10553, Springer, (2017), 240-248. |
[25] | A. Kermi, I. Mahmoudi, M. T. Khadir, Deep convolutional neural networks using U-Net for automatic brain tumor segmentation in multimodal MRI volumes, in Brainlesion: Glioma, Multiple Sclerosis, Stroke and Traumatic Brain Injuries - 4th International Workshop, Brain-Les 2018, Held in Conjunction with MICCAI 2018, Revised Selected Papers, Part II, Lecture Notes in Computer Science, 11384, Springer, (2018), 37-48. |
[26] | M. Bhalerao, S. Thakur, Brain Tumor Segmentation Based on 3D Residual U-Net, in Brainlesion: Glioma, Multiple Sclerosis, Stroke and Traumatic Brain Injuries 5th International Workshop, BrainLes 2019, Held in Conjunction with MICCAI 2019, Revised Selected Papers, Part II, Lecture Notes in Computer Science, 11993, Springer, (2019), 218-225. |
[27] |
Y. L. Tai, S. J. Huang, C. C. Chen, H. S. Lu, Computational Complexity Reduction of Neural Networks of Brain Tumor Image Segmentation by Introducing Fermi-Dirac Correction Functions, Entropy, 23 (2021), 223. doi: 10.3390/e23020223
![]() |
[28] | U. Baid, N. A. Shah, S. Talbar, Brain Tumor Segmentation with Cascaded Deep Convolutional Neural Network, in Brainlesion: Glioma, Multiple Sclerosis, Stroke and Traumatic Brain Injuries 5th International Workshop, BrainLes 2019, Held in Conjunction with MICCAI 2019, Revised Selected Papers, Part II, Lecture Notes in Computer Science, 11993. Springer, (2019), 90-98. |
[29] |
M. U. Rehman, S. B. Cho, J. Kim, K. T. Chong, BrainSeg-Net: Brain Tumor MR Image Segmentation via Enhanced Encoder-Decoder Network, Diagnostics, 11 (2021), 169. doi: 10.3390/diagnostics11020169
![]() |
[30] |
J. Zhang, Z. Jiang, J. Dong, Attention Gate ResU-Net for automatic MRI brain tumor segmentation, IEEE Access, 8 (2020), 58533-58545. doi: 10.1109/ACCESS.2020.2983075
![]() |
[31] | M. Amian, M. Soltaninejad, Multi-resolution 3D CNN for MRI Brain Tumor Segmentation and Survival Prediction, inBrainlesion: Glioma, Multiple Sclerosis, Stroke and Traumatic Brain Injuries 5th International Workshop, BrainLes 2019, Held in Conjunction with MICCAI 2019, Revised Selected Papers, Part II, Lecture Notes in Computer Science, 11993, Springer, (2019), 221-230. |
[32] |
K. Hu, Q. Gan, Y. Zhang, S. Deng, F. Xiao, W. Huang et al., Brain tumor segmentation using multi-Cascaded Convolutional Neural Networks and Conditional Random Field, IEEE Access, 7 (2019), 92615-92629. doi: 10.1109/ACCESS.2019.2927433
![]() |
[33] | M. Marcinkiewicz, J. Nalepa, P. R. Lorenzo, W. Dudzik, G. Mrukwa, Automatic Brain Tumor Segmentation Using a Two-Stage Multi-Modal FCNN, in Brainlesion: Glioma, Multiple Sclerosis, Stroke and Traumatic Brain Injuries, (2018), 13-24. |
[34] | S. Chandra, M. Vakalopoulou, L. Fidon, E. Battistella, T. Estienne, R. Sunet, et al., Context aware 3D CNNs for brain tumor segmentation, in Brainlesion: Glioma, Multiple Sclerosis, Stroke and Traumatic Brain Injuries - 4th International Workshop, BrainLes 2018, Held in Conjunction with MICCAI 2018, Revised Selected Papers, Part II, Lecture Notes in Computer Science, 11384, Springer, (2018), 299-310. |
[35] | U. Baid, S. Talbar, S. Rane, S. Gupta, M. H. Thakur, A. Moiyadi, et al., A novel approach for fully automatic intra-tumor segmentation with 3D U-Net architecture for gliomas, Frontiers Comput. Neurosci., 14 (2020), 10. |
[36] | Ö. Çiçek, A. Abdulkadir, S. S. Lienkamp, T. Brox, O. Ronneberger, 3D U-Net: Learning Dense Volumetric Segmentation from Sparse Annotation, in MICCAI: International Conference on Medical Image Computing and Computer-Assisted Intervention, 19th International Conference, Athens, 2016, Proceedings, Part II, Lecture Notes in Computer Science, 9901, Springer, (2016), 424-432. |
1. | Emin GÖKÇE, Mehmet Fatih DEMİRAL, Ali Hakan ISIK, Mehmet BİLEN, Evrişimli Sinir Ağlarında Beyin Tümörü Segmentasyonu, 2022, 2148-3736, 10.31202/ecjse.1141335 | |
2. | Necip Cinar, Alper Ozcan, Mehmet Kaya, A hybrid DenseNet121-UNet model for brain tumor segmentation from MR Images, 2022, 76, 17468094, 103647, 10.1016/j.bspc.2022.103647 | |
3. | Haojia Wang, Xicheng Chen, Rui Yu, Zeliang Wei, Tianhua Yao, Chengcheng Gao, Yang Li, Zhenyan Wang, Dong Yi, Yazhou Wu, E-DU: Deep neural network for multimodal medical image segmentation based on semantic gap compensation, 2022, 151, 00104825, 106206, 10.1016/j.compbiomed.2022.106206 | |
4. | Thong Vo, Pranjal Dave, Gaurav Bajpai, Rasha Kashef, Naimul Khan, 2022, Brain Tumor Segmentation in MRI Images Using A Modified U-Net Model, 978-1-6654-8149-6, 29, 10.1109/ICDH55609.2022.00012 | |
5. | Guangyuan Zhang, Xiaonan Gao, Zhenfang Zhu, Fengyv Zhou, Dexin Yu, Determination of the location of the needle entry point based on an improved pruning algorithm, 2022, 19, 1551-0018, 7952, 10.3934/mbe.2022372 | |
6. | Yuan Cao, Weifeng Zhou, Min Zang, Dianlong An, Yan Feng, Bin Yu, MBANet: A 3D convolutional neural network with multi-branch attention for brain tumor segmentation from MRI images, 2023, 80, 17468094, 104296, 10.1016/j.bspc.2022.104296 | |
7. | Xiangbin Liu, Shufen Hou, Shuai Liu, Weiping Ding, Yudong Zhang, Attention-based Multimodal Glioma Segmentation with Multi-attention Layers for Small-intensity Dissimilarity, 2023, 13191578, 10.1016/j.jksuci.2023.03.011 | |
8. | Jyotismita Chaki, Marcin Woźniak, A deep learning based four-fold approach to classify brain MRI: BTSCNet, 2023, 85, 17468094, 104902, 10.1016/j.bspc.2023.104902 | |
9. | Muqing Zhang, Dongwei Liu, Qiule Sun, Yutong Han, Bin Liu, Jianxin Zhang, Mingli Zhang, Augmented Transformer network for MRI brain tumor segmentation, 2024, 36, 13191578, 101917, 10.1016/j.jksuci.2024.101917 | |
10. | Md Kamrul Hasan Khan, Wenjing Guo, Jie Liu, Fan Dong, Zoe Li, Tucker A Patterson, Huixiao Hong, Machine learning and deep learning for brain tumor MRI image segmentation, 2023, 1535-3702, 10.1177/15353702231214259 | |
11. | Ahmed Hechri, Ahmed Boudaka, Abdelrahman Hamed, Improved brain tumour segmentation using modified U-Net model with inception and attention modules on multimodal MRI images, 2024, 21, 1448-837X, 48, 10.1080/1448837X.2024.2309427 | |
12. | Jing Wang, Liang Yin, Chi Lin, Chang Wu Yu, Ning Wang, CNN-based glioma detection in MRI: A deep learning approach, 2024, 32, 09287329, 4965, 10.3233/THC-240158 | |
13. | Tongxue Zhou, M2GCNet: Multi-Modal Graph Convolution Network for Precise Brain Tumor Segmentation Across Multiple MRI Sequences, 2024, 33, 1057-7149, 4896, 10.1109/TIP.2024.3451936 | |
14. | Pandapotan Siagian, Riyanarto Sarno, Chastine Fatichah, Muhammad Ibadurrahman Arrasyid Supriyanto, Aziz Fajar, 2023, 3D Patch Spatially Localized Network Tiles Enables for 3D Brain Segmentation, 979-8-3503-6110-0, 1, 10.1109/ICT60153.2023.10374062 | |
15. | Ziaur Rahman, Ruihong Zhang, Jameel Ahmed Bhutto, A Symmetrical Approach to Brain Tumor Segmentation in MRI Using Deep Learning and Threefold Attention Mechanism, 2023, 15, 2073-8994, 1912, 10.3390/sym15101912 | |
16. | Tongxue Zhou, Multi-modal brain tumor segmentation via disentangled representation learning and region-aware contrastive learning, 2024, 149, 00313203, 110282, 10.1016/j.patcog.2024.110282 | |
17. | P.S. Tejashwini, J. Thriveni, K.R. Venugopal, A novel SLCA-UNet architecture for automatic MRI brain tumor segmentation, 2025, 100, 17468094, 107047, 10.1016/j.bspc.2024.107047 | |
18. | Tongxue Zhou, Shan Zhu, Uncertainty quantification and attention-aware fusion guided multi-modal MR brain tumor segmentation, 2023, 163, 00104825, 107142, 10.1016/j.compbiomed.2023.107142 | |
19. | Hao Luo, Dongmei Zhou, Yongjian Cheng, Siqi Wang, MPEDA-Net: A lightweight brain tumor segmentation network using multi-perspective extraction and dense attention, 2024, 91, 17468094, 106054, 10.1016/j.bspc.2024.106054 | |
20. | P. Ramadevi, Motamarri Jaya Naga Venkata Sai, P Y Sai Srinivas, 2024, Segmentation of Brain Tumor with Deep Learning Models, 979-8-3503-8166-5, 1, 10.1109/ICDSIS61070.2024.10594009 | |
21. | Wadhah Ayadi, Wajdi Elhamzi, Mohamed Atri, A deep conventional neural network model for glioma tumor segmentation, 2023, 33, 0899-9457, 1593, 10.1002/ima.22892 | |
22. | Maoyi Zhang, Changqing Ding, 2023, Transformer-stage based segmentation of empty sella and peripheral arteries, 979-8-3503-4754-8, 849, 10.1109/ICCEA58433.2023.10135429 | |
23. | Qian Wu, Yuyao Pei, Zihao Cheng, Xiaopeng Hu, Changqing Wang, SDS-Net: A lightweight 3D convolutional neural network with multi-branch attention for multimodal brain tumor accurate segmentation, 2023, 20, 1551-0018, 17384, 10.3934/mbe.2023773 | |
24. | Agnesh Chandra Yadav, Maheshkumar H. Kolekar, Mukesh Kumar Zope, Modified Recurrent Residual Attention U-Net model for MRI-based brain tumor segmentation, 2025, 102, 17468094, 107220, 10.1016/j.bspc.2024.107220 | |
25. | Tongxue Zhou, Zheng Wang, Xiaohui Liu, Weibo Liu, Shan Zhu, Learning deep feature representations for multi-modal MR brain tumor segmentation, 2025, 638, 09252312, 130162, 10.1016/j.neucom.2025.130162 | |
26. | Tongxue Zhou, Boundary-aware and cross-modal fusion network for enhanced multi-modal brain tumor segmentation, 2025, 165, 00313203, 111637, 10.1016/j.patcog.2025.111637 |
Methods | DSC | Hausdorff95 | ||||
Whole | Core | Enhancing | Whole | Core | Enhancing | |
U-Net | 0.872 | 0.774 | 0.689 | 8.79 | 7.62 | 3.65 |
SoU-Net | 0.875 | 0.786 | 0.698 | 8.59 | 7.35 | 3.57 |
ResU-Net | 0.877 | 0.782 | 0.694 | 8.59 | 7.36 | 3.67 |
SoResU-Net | 0.881 | 0.796 | 0.707 | 8.40 | 7.06 | 3.48 |
Methods | DSC | Hausdorff95 | ||||
Whole | Core | Enhancing | Whole | Core | Enhancing | |
U-Net | 0.865 | 0.748 | 0.678 | 12.73 | 14.16 | 7.25 |
SoU-Net | 0.867 | 0.766 | 0.693 | 12.30 | 12.73 | 7.08 |
ResU-Net | 0.871 | 0.766 | 0.701 | 9.71 | 12.21 | 6.14 |
SoResU-Net | 0.875 | 0.788 | 0.724 | 9.35 | 11.47 | 5.97 |
Methods | DSC | Hausdorff95 | ||||
Whole | Core | Enhancing | Whole | Core | Enhancing | |
Bhalerao et al. [26] | 0.852 | 0.709 | 0.666 | 8.07 | 9.57 | 7.27 |
Tai et al. [27] | 0.843 | 0.804 | 0.684 | - | - | - |
Baid et al. [28] | 0.87 | 0.77 | 0.70 | 13.36 | 12.71 | 6.45 |
Rehman et al. [29] | 0.869 | 0.775 | 0.708 | - | - | - |
Jiang et al. [30] | 0.870 | 0.777 | 0.709 | 9.07 | 11.40 | 6.49 |
Amian et al. [31] | 0.86 | 0.77 | 0.71 | 8.42 | 11.55 | 6.92 |
ours | 0.875 | 0.788 | 0.724 | 9.35 | 11.47 | 5.97 |
Methods | DSC | Hausdorff95 | ||||
Whole | Core | Enhancing | Whole | Core | Enhancing | |
U-Net | 0.865 | 0.782 | 0.743 | 11.31 | 15.34 | 3.79 |
SoU-Net | 0.868 | 0.797 | 0.755 | 10.81 | 13.94 | 3.65 |
ResU-Net | 0.871 | 0.790 | 0.751 | 8.89 | 11.71 | 3.51 |
SoResU-Net | 0.876 | 0.811 | 0.771 | 8.42 | 10.56 | 3.22 |
Methods | DSC | Hausdorff95 | ||||
Whole | Core | Enhancing | Whole | Core | Enhancing | |
Hu et al. [32] | 0.882 | 0.748 | 0.718 | 12.60 | 9.62 | 5.69 |
Marcinkiewicz et al. [33] | 0.862 | 0.768 | 0.723 | - | - | - |
Chandra et al. [34] | 0.872 | 0.795 | 0.741 | 5.04 | 9.59 | 5.58 |
Baid et al. [35] | 0.878 | 0.826 | 0.748 | 16.81 | 11.20 | 7.29 |
Çiçek et al. [36] | 0.885 | 0.718 | 0.760 | 17.10 | 11.60 | 6.04 |
ours | 0.876 | 0.811 | 0.771 | 8.42 | 10.56 | 3.22 |
Channels | Whole | Core | Enhancing |
1×channel | 0.873 | 0.778 | 0.702 |
4×channel | 0.872 | 0.784 | 0.709 |
8×channel | 0.873 | 0.786 | 0.715 |
16×channel | 0.875 | 0.788 | 0.724 |
Methods | Dataset | DSC | Hausdorff95 | ||||
Whole | Core | Enhancing | Whole | Core | Enhancing | ||
ResU-Net | BraTS 2018 | 0.871 | 0.790 | 0.751 | 8.89 | 11.71 | 3.51 |
ReSoU-Net | 0.873 | 0.808 | 0.766 | 8.78 | 10.94 | 3.22 | |
ResU-Net | BraTS 2019 | 0.871 | 0.766 | 0.701 | 9.71 | 12.21 | 6.14 |
ReSoU-Net | 0.876 | 0.782 | 0.717 | 8.75 | 11.29 | 5.24 |
Methods | DSC | Hausdorff95 | ||||
Whole | Core | Enhancing | Whole | Core | Enhancing | |
U-Net | 0.872 | 0.774 | 0.689 | 8.79 | 7.62 | 3.65 |
SoU-Net | 0.875 | 0.786 | 0.698 | 8.59 | 7.35 | 3.57 |
ResU-Net | 0.877 | 0.782 | 0.694 | 8.59 | 7.36 | 3.67 |
SoResU-Net | 0.881 | 0.796 | 0.707 | 8.40 | 7.06 | 3.48 |
Methods | DSC | Hausdorff95 | ||||
Whole | Core | Enhancing | Whole | Core | Enhancing | |
U-Net | 0.865 | 0.748 | 0.678 | 12.73 | 14.16 | 7.25 |
SoU-Net | 0.867 | 0.766 | 0.693 | 12.30 | 12.73 | 7.08 |
ResU-Net | 0.871 | 0.766 | 0.701 | 9.71 | 12.21 | 6.14 |
SoResU-Net | 0.875 | 0.788 | 0.724 | 9.35 | 11.47 | 5.97 |
Methods | DSC | Hausdorff95 | ||||
Whole | Core | Enhancing | Whole | Core | Enhancing | |
Bhalerao et al. [26] | 0.852 | 0.709 | 0.666 | 8.07 | 9.57 | 7.27 |
Tai et al. [27] | 0.843 | 0.804 | 0.684 | - | - | - |
Baid et al. [28] | 0.87 | 0.77 | 0.70 | 13.36 | 12.71 | 6.45 |
Rehman et al. [29] | 0.869 | 0.775 | 0.708 | - | - | - |
Jiang et al. [30] | 0.870 | 0.777 | 0.709 | 9.07 | 11.40 | 6.49 |
Amian et al. [31] | 0.86 | 0.77 | 0.71 | 8.42 | 11.55 | 6.92 |
ours | 0.875 | 0.788 | 0.724 | 9.35 | 11.47 | 5.97 |
Methods | DSC | Hausdorff95 | ||||
Whole | Core | Enhancing | Whole | Core | Enhancing | |
U-Net | 0.865 | 0.782 | 0.743 | 11.31 | 15.34 | 3.79 |
SoU-Net | 0.868 | 0.797 | 0.755 | 10.81 | 13.94 | 3.65 |
ResU-Net | 0.871 | 0.790 | 0.751 | 8.89 | 11.71 | 3.51 |
SoResU-Net | 0.876 | 0.811 | 0.771 | 8.42 | 10.56 | 3.22 |
Methods | DSC | Hausdorff95 | ||||
Whole | Core | Enhancing | Whole | Core | Enhancing | |
Hu et al. [32] | 0.882 | 0.748 | 0.718 | 12.60 | 9.62 | 5.69 |
Marcinkiewicz et al. [33] | 0.862 | 0.768 | 0.723 | - | - | - |
Chandra et al. [34] | 0.872 | 0.795 | 0.741 | 5.04 | 9.59 | 5.58 |
Baid et al. [35] | 0.878 | 0.826 | 0.748 | 16.81 | 11.20 | 7.29 |
Çiçek et al. [36] | 0.885 | 0.718 | 0.760 | 17.10 | 11.60 | 6.04 |
ours | 0.876 | 0.811 | 0.771 | 8.42 | 10.56 | 3.22 |
Channels | Whole | Core | Enhancing |
1×channel | 0.873 | 0.778 | 0.702 |
4×channel | 0.872 | 0.784 | 0.709 |
8×channel | 0.873 | 0.786 | 0.715 |
16×channel | 0.875 | 0.788 | 0.724 |
Methods | Dataset | DSC | Hausdorff95 | ||||
Whole | Core | Enhancing | Whole | Core | Enhancing | ||
ResU-Net | BraTS 2018 | 0.871 | 0.790 | 0.751 | 8.89 | 11.71 | 3.51 |
ReSoU-Net | 0.873 | 0.808 | 0.766 | 8.78 | 10.94 | 3.22 | |
ResU-Net | BraTS 2019 | 0.871 | 0.766 | 0.701 | 9.71 | 12.21 | 6.14 |
ReSoU-Net | 0.876 | 0.782 | 0.717 | 8.75 | 11.29 | 5.24 |