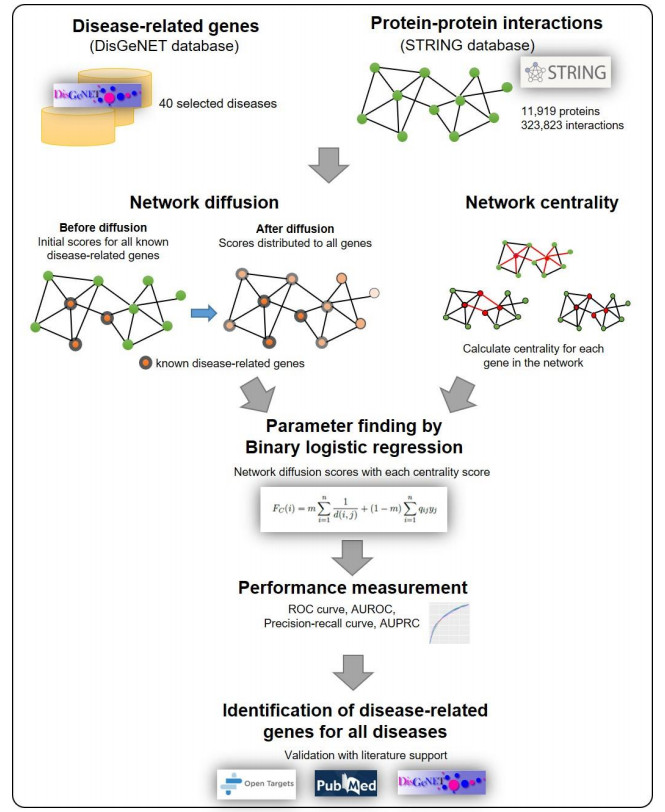
Disease-related gene prioritization is one of the most well-established pharmaceutical techniques used to identify genes that are important to a biological process relevant to a disease. In identifying these essential genes, the network diffusion (ND) approach is a widely used technique applied in gene prioritization. However, there is still a large number of candidate genes that need to be evaluated experimentally. Therefore, it would be of great value to develop a new strategy to improve the precision of the prioritization. Given the efficiency and simplicity of centrality measures in capturing a gene that might be important to the network structure, herein, we propose a technique that extends the scope of ND through a centrality measure to identify new disease-related genes. Five common centrality measures with different aspects were examined for integration in the traditional ND model. A total of 40 diseases were used to test our developed approach and to find new genes that might be related to a disease. Results indicated that the best measure to combine with the diffusion is closeness centrality. The novel candidate genes identified by the model for all 40 diseases were provided along with supporting evidence. In conclusion, the integration of network centrality in ND is a simple but effective technique to discover more precise disease-related genes, which is extremely useful for biomedical science.
Citation: Panisa Janyasupab, Apichat Suratanee, Kitiporn Plaimas. Network diffusion with centrality measures to identify disease-related genes[J]. Mathematical Biosciences and Engineering, 2021, 18(3): 2909-2929. doi: 10.3934/mbe.2021147
[1] | Peter Hinow, Edward A. Rietman, Sara Ibrahim Omar, Jack A. Tuszyński . Algebraic and topological indices of molecular pathway networks in human cancers. Mathematical Biosciences and Engineering, 2015, 12(6): 1289-1302. doi: 10.3934/mbe.2015.12.1289 |
[2] | Yongyin Han, Maolin Liu, Zhixiao Wang . Key protein identification by integrating protein complex information and multi-biological features. Mathematical Biosciences and Engineering, 2023, 20(10): 18191-18206. doi: 10.3934/mbe.2023808 |
[3] | Babak Khorsand, Abdorreza Savadi, Javad Zahiri, Mahmoud Naghibzadeh . Alpha influenza virus infiltration prediction using virus-human protein-protein interaction network. Mathematical Biosciences and Engineering, 2020, 17(4): 3109-3129. doi: 10.3934/mbe.2020176 |
[4] | Fang Niu, Zongwei Liu, Peidong Liu, Hongrui Pan, Jiaxue Bi, Peng Li, Guangze Luo, Yonghui Chen, Xiaoxing Zhang, Xiangchen Dai . Identification of novel genetic biomarkers and treatment targets for arteriosclerosis-related abdominal aortic aneurysm using bioinformatic tools. Mathematical Biosciences and Engineering, 2021, 18(6): 9761-9774. doi: 10.3934/mbe.2021478 |
[5] | Jing Cao, Hui Gan, Han Xiao, Hui Chen, Dan Jian, Ning Jiang, Xuan Zhai . Key protein-coding genes related to microglia in immune regulation and inflammatory response induced by epilepsy. Mathematical Biosciences and Engineering, 2021, 18(6): 9563-9578. doi: 10.3934/mbe.2021469 |
[6] | Ming-Xi Zhu, Tian-Yang Zhao, Yan Li . Insight into the mechanism of DNA methylation and miRNA-mRNA regulatory network in ischemic stroke. Mathematical Biosciences and Engineering, 2023, 20(6): 10264-10283. doi: 10.3934/mbe.2023450 |
[7] | Sufang Wu, Hua He, Jingjing Huang, Shiyao Jiang, Xiyun Deng, Jun Huang, Yuanbing Chen, Yiqun Jiang . FMR1 is identified as an immune-related novel prognostic biomarker for renal clear cell carcinoma: A bioinformatics analysis of TAZ/YAP. Mathematical Biosciences and Engineering, 2022, 19(9): 9295-9320. doi: 10.3934/mbe.2022432 |
[8] | Cicely K. Macnamara, Mark A. J. Chaplain . Spatio-temporal models of synthetic genetic oscillators. Mathematical Biosciences and Engineering, 2017, 14(1): 249-262. doi: 10.3934/mbe.2017016 |
[9] | Ahmed Hammad, Mohamed Elshaer, Xiuwen Tang . Identification of potential biomarkers with colorectal cancer based on bioinformatics analysis and machine learning. Mathematical Biosciences and Engineering, 2021, 18(6): 8997-9015. doi: 10.3934/mbe.2021443 |
[10] | Moxuan Zhang, Quan Zhang, Jilin Bai, Zhiming Zhao, Jian Zhang . Transcriptome analysis revealed CENPF associated with glioma prognosis. Mathematical Biosciences and Engineering, 2021, 18(3): 2077-2096. doi: 10.3934/mbe.2021107 |
Disease-related gene prioritization is one of the most well-established pharmaceutical techniques used to identify genes that are important to a biological process relevant to a disease. In identifying these essential genes, the network diffusion (ND) approach is a widely used technique applied in gene prioritization. However, there is still a large number of candidate genes that need to be evaluated experimentally. Therefore, it would be of great value to develop a new strategy to improve the precision of the prioritization. Given the efficiency and simplicity of centrality measures in capturing a gene that might be important to the network structure, herein, we propose a technique that extends the scope of ND through a centrality measure to identify new disease-related genes. Five common centrality measures with different aspects were examined for integration in the traditional ND model. A total of 40 diseases were used to test our developed approach and to find new genes that might be related to a disease. Results indicated that the best measure to combine with the diffusion is closeness centrality. The novel candidate genes identified by the model for all 40 diseases were provided along with supporting evidence. In conclusion, the integration of network centrality in ND is a simple but effective technique to discover more precise disease-related genes, which is extremely useful for biomedical science.
Network medicine is an essential network-based approach that applies appropriate methods in various types of biological networks, making it beneficial for the completion of several tasks [1]. Interactome-based approaches to human diseases have been collated in [2] to highlight the importance of physical interactions within the cell. The types of molecular data and analytical methods utilized in inferring molecular networks have also been discussed [3]. Human interactomes with co-expression networks have been discovered to be capable of predicting novel disease genes and disease modules [4]. Specifically, the key network modules related to chronic obstructive pulmonary disease [5] and glioblastoma [6] have been identified.
To enhance the understanding of disease mechanisms, disease and gene association studies are required in biological science. Several studies have provided associations between diseases and genes using gene prioritization to identify the genes with close relationships to diseases. The identification of those genes can be useful in disease diagnosis and prevention. Given that wet-lab experiments are time consuming and costly, a great number of computational approaches have been formulated to prioritize candidate disease genes [7,8,9]. For example, inflammatory bowel disease-related proteins have been successfully identified using a reverse k-nearest neighbor search [8], while epilepsy-related genes have been recognized using a random walk with a restart algorithm [9]. Moreover, the disease module detection (DIAMOnD) algorithm is able to identify the community of diseases around a set of known disease proteins in a network [10]. Meanwhile, for cancerous diseases, the SWItchMiner (SWIM) uses the concept of nearest neighbor node to identify crucial nodes [11].
Network diffusion (ND) is one of the most promising methods to identify novel targets for many diseases [12,13]. It starts with a set of seed nodes which are known disease-related genes and then disperses the scores throughout the network with an iterative technique and random walks. It is worth noting that with the use of initial scores marked by known disease-related genes, ND results in a good ranking performance, but it may be limited to some topological structures [14] and unknown disease-related genes hidden at crucial central locations in the network. Many modified versions of ND have been proposed. For example, the statistical normalization of input scores has been applied to reduce bias and variance of values throughout the network [14]. An adaptive version of ND, namely, network smoothing index and permutation-adjusted score with network resampling has also been applied in the analysis of enriched disease modules of autism [15] and prostate adenocarcinoma [16]. In addition, it has been later proven by calculating the median degree of output nodes that network propagation tends to return the hub nodes [17]. Interestingly, hub nodes can be identified by various centrality measures.
Network centrality by itself has been used to prioritize disease genes based on the interaction network. Different types of centralities measure different aspects of calculations to distinguish the role of a certain node in the network related to the network topology. The co-occurrence gene-interaction network has been analyzed to identify the disease-gene associations of cancerous diseases [18]. Recently, Zhao et al. reported that complex disease genes tend to have higher betweenness centrality, smaller average shortest path length, and smaller clustering coefficient; they also have no significance in degree centrality [19]. Moreover, the combination of all five centralities (i.e., degree centrality, eigenvector centrality, closeness centrality, betweenness centrality, and k-clique percolation) is useful in identifying non-cancerous disease-related genes [20].
In this work, we propose the use of a centrality measure together with ND to prioritize and identify novel disease-related genes. The standard calculation of ND was applied, and five commonly used centrality measures, namely, betweenness centrality, closeness centrality, degree centrality, eigenvector centrality, and pagerank centrality, were investigated and evaluated. The trade-off weighting parameters between the diffusion scores and centrality scores were captured with the normalization of the parameters obtained from a binary logistic regression. Various performance measures were applied such as ten-fold cross-validation, ROC curve, and precision-recall curve. Finally, the top-ranking disease-related genes were identified and validated with supporting evidence.
The overview of our workflow is shown in Figure 1. First, a protein-protein interaction (PPI) network of humans was constructed from the STRING database [21] and known disease-related genes were extracted from the DisGeNet database [22]. Afterward, ND score for each disease was calculated to obtain stable scores for all proteins, and the calculation of each centrality was applied to all proteins in the network. The combination of each centrality measure and ND technique was then evaluated and the parameters were tuned using binary logistic regression. The ND and best centrality model were used and evaluated with ten-fold cross-validation technique. Finally, the prioritization scores and the top candidates were selected and reported with supporting evidence.
The PPI network and evidence of their corresponding genes were obtained from the STRING database version 11.0 [21]. The interactions with a confidence score lower than 900 were excluded, isolated nodes were eliminated, and multiple edges were removed. With these filters, we obtained a weighted connected network of 11,919 proteins and 323,823 interactions. All isolated nodes and islands were discarded. The weight values ranging from 900 to 999 were rescaled into the range of [0.1, 1]. Disease-gene associations were retrieved from the DisGeNET database [22]. All disease genes were mapped onto the PPI network. Forty diseases from the database were selected for this study. The list of diseases and the number of genes corresponding to each disease are shown in Supplementary Table T1. Notice that there are approximately 1,200–3,600 proteins associated with a disease. Therefore, there are highly unbalanced data between the known and unknown disease-related genes for each disease.
ND is an effective and widely used technique to identify disease-related genes. Diffusion requires prior data of gene-disease associations to be assigned as initial scores of nodes. Then the score of each node diffuses through the edges.
Let be a graph with nodes and be edge weights connecting between node and . Weight adjacency matrix is an matrix whose entry is defined as if a connection exists between node and ; otherwise, it is 0.
Let be an diagonal matrix with and be the Laplacian of the graph. The ND score (Ds) using regularized Laplacian kernel [23] is defined as
(1) |
where is a kernel and is an initial label vector of length . This label is defined as 1 if gene has an association with a disease and 0 otherwise. is an identity matrix, and is a constant of the diffusion rate.
To find the impact of each node or protein in the PPI network, the centrality measure could be exploited. The five most widely used centrality measures are betweenness, closeness, degree, eigenvector, and pagerank.
Betweenness centrality CB: The betweenness centrality measures the number of times a protein is in-between the shortest path between any two other proteins in the network. We could calculate the betweenness centrality of a protein as
(2) |
where is the number of the shortest path between nodes and and is the number of the shortest path between nodes and that passes through node . As the defined weight is originally derived from the confidence score of the STRING database, this score indicates how much two proteins are likely to interact with each other. In other words, higher weights mean more similarity for those two proteins. However, to calculate the shortest path for betweenness centrality, the weight of the edges should reflect a distance (or difference) between two proteins. Therefore, the reciprocal of the defined weight should be applied as the distance between two proteins in the network which can be used to find the shortest path.
Closeness centrality CC: The closeness centrality is the inverse distances between a protein and all other proteins in the network. It can be calculated as the reciprocal of the sum of the length of the shortest paths between the node and all other nodes in the graph,
(3) |
where is the shortest distance between node and node . Thus, a central node obtains high closeness value. Note that in the same manner, to calculate the shortest path or distance as betweenness centrality, the distance between two proteins is the reciprocal of the originally defined weight.
Degree centrality CD: The degree centrality of a protein in a network is simply the number of connections of that protein to the other proteins in the network. In the biological network, most proteins have a low number of connections and a small number of proteins have a high number of connections. The high-degree proteins are called "hubs" and are important for cell viability. The degree can simply be calculated as
(4) |
Eigenvector centrality CE: The extended version of degree centrality is eigenvector centrality. It takes the global structure of the network together under the assumption that important nodes should be located near the other important ones, and their scores should be nearly the same or the average. A node with high eigenvector centrality has a great number of neighbors, and its neighbors also have a great number of neighbors. Eigenvector centrality can be formulated as
(5) |
where is the largest eigenvalue of .
PageRank centrality CP: With a definition that is similar to that of eigenvector centrality, pagerank centrality is used for a directed network and for node labelling. In case of an undirected network, the pagerank centrality of a node is defined as
(6) |
where is the set of neighbors of node is the number of neighbors of node , and is a factor used for normalization.
We intend to develop a simple and effective formula to integrate the values of ND scores and centrality. Let and be two variables of centrality and diffusion scores, respectively. Then, the score combination can be written as
(7) |
where is a weight constant that can be found by training a binary logistic regression. According to the ND score in Equation 1, Equation 7 can be rewritten as
(8) |
Let , then . Therefore, our formula for node and disease is
(9) |
The result of selecting is to obtain the matrix which can be considered as matrix of relative forest accessibilities. This matrix provides information on how close any two nodes in the network (or forest) are to each other. represents the close connection. If nodes and are farther from each other, then is small. More details of the calculation can be found in [24]. Therefore, the second term of our model gives a higher score to a node that is close to a disease-related gene node. For a disease , tuning parameter was done by binary logistic regression with 10 random selections of 70% of the data to obtain the coefficient of the model.
According to the proposed model in Equation 8 (general) and Equation 9 (for each disease), binary logistic regression was used to tune the parameter m. This parameter is a key point to assign a proportion between genes that have close connections to a known disease-related gene, that is, genes that are located centrally or importantly in the flow and structure of the whole network. Thus, in the regression, the centrality and diffusion scores were used as factors or predictors of the binary logistic regression whose corresponding outcomes are disease-related genes or not. Finally, the logistic regression yields the probability of the observed data as a function of the unknown parameters. Then, the maximum likelihood estimators were applied to find the best-fit parameters that will maximize this function. Afterward, the obtained parameters were rescaled in the range of 0 and 1 as a representative of a proportion (m) of a centrality and (1 - m) for the diffusion. The same procedure was applied to all diseases via Equation 9 to find the md for each disease.
To formulate a fair performance measurement, 10-fold cross-validation was selected as a validation strategy in our work. The data were divided into 10 parts: one part for testing and nine parts for training. Each part can take a turn to be a testing set iteratively. For each iteration, we set the initial scores of testing samples to zero to demonstrate that there was no association between a gene and a disease in this testing set. Afterward, ND calculation was performed, and the resulting scores were used to make a prediction as to which genes are associated with a disease. Finally, we obtained the association scores for all samples.
Various metrics have been used to measure the performance of the proposed model as highly unbalanced data between known and unknown disease-gene relations is considered as a challenge. The precision and the top newly identified disease-related genes would be of much interest in this prioritization. Ten-fold cross-validation was performed five times to obtain the average performance and to make a final prediction score for each gene of each disease. All of the prediction results for each gene in the five runs were summed and used to calculate an average prediction value for each gene of a disease. Then, the averaged prediction score was used to measure the performance of each disease model.
Confusion matrix: The confusion matrix is shown in Table 1, where positivity and negativity stand for a gene having an association and no association with a disease, respectively. Let known disease-related genes be a positive class and unknown disease-related genes be a negative class. To calculate the values for the confusion matrix, genes whose averaged prediction scores matched and/or ranked above a certain threshold, were predicted to be in the positive class.
Gold Standard | |||
Positive | Negative | ||
Predicted | Positive | True Positive (TP) | False Positive (FP) |
Negative | False Negative (FN) | True Negative (TN) |
True positive rate (TPR) or Sensitivity is calculated as TP/(TP+FN) and false positive rate (FPR) is calculated as TN/(TN+FP).
Area Under the Receiver Operating Characteristic Curve (AUROC): The receiver operating characteristic (ROC) curve is a plot displaying the relation between true positive rate (TPR) and false positive rate (FPR), at any cut point , and the AUROC is defined by . AUROC would be 1 for the best binary classifier and 0.5 for random prediction.
Partial Area Under the ROC Curve (pAUROC): All possible genes in the entire network were analyzed and ranked. To measure the performance precisely, only high-ranked genes were considered. Thus, pAUROC is employed and defined as , . In this study, we selected . It means that only 5% of the FPR are considered.
Area Under the Precision-Recall Curve (AUPRC): The precision-recall curve shows the relation between precision and recall. The AUPRC is defined by for any cut point . Similarly, AUPRC should be 1 for the best binary classifier. However, AUPRC is more informative for an imbalanced dataset.
Top-k: The number of true positives in the top predicted genes are of interest in retrieving a positive and a new positive sample. Top k measures how many genes are correctly predicted in the first k top ranking. Thus, if top is equal to , it implies that all top predicted genes are correctly labelled. In this study, we set k = 100 to measure the percentage of how many true disease-related genes could be detected in the Top 100 ranked genes.
We first constructed a PPI network based on the STRING database (see Materials and Methods). The weighted PPI network consists of 11,919 proteins and 323,823 interactions with edge weights ranging from 0.1 to 1. The network follows the power-law distribution [25] (Figure 2), in which a large number of nodes have a low degree and a small number of nodes have a high degree. The list of diseases and the number of genes corresponding to each disease are shown in Supplementary Table T1.
The betweenness, closeness, degree, eigenvector, and pagerank centralities were calculated for each gene in the network. Note that each centrality was calculated once for all diseases, while ND was calculated for each disease separately with a change of the initial scores for known disease-related genes. When comparing the scores of each method to the known disease-related genes for each disease, we found that betweenness and closeness centralities yield higher performance than the others. This result is in an agreement with the study of Zhao et al. [20], which indicated that disease genes tend to have high betweenness centrality and small average shortest path length, which refers to the meaning of closeness. Table 2 shows the mean and standard deviations of each performance of each centrality for all 40 diseases. Figure 3 shows the AUROC of the centrality measures for all 40 diseases. The plot of the means and standard deviations of AUROC, pAUROC, AUPRC, and Top 100 performances for each centrality show the same results and can be found in Supplementary Figure F1.
AUROC | pAUROC | AUPRC | Top 100 | |
Betweenness centrality | 0.6532 ± 0.0150 | 0.1027 ± 0.0065 | 0.2754 ± 0.0633 | 57.6000 ± 9.7212 |
Closeness centrality | 0.6645 ± 0.0335 | 0.0940 ± 0.0173 | 0.2840 ± 0.0773 | 61.8000 ± 13.0977 |
Degree | 0.6048 ± 0.0164 | 0.0380 ± 0.0130 | 0.1970 ± 0.0591 | 22.2250 ± 6.8444 |
Eigenvector centrality | 0.5862 ± 0.0265 | 0.0125 ± 0.0033 | 0.1750 ± 0.0584 | 9.5750 ± 5.8172 |
PageRank centrality | 0.6128 ± 0.0137 | 0.0784 ± 0.0122 | 0.2366 ± 0.0613 | 50.0500 ± 9.9123 |
In Table 2, closeness centrality provides the best performance in AUROC of approximately 66%, while betweenness centrality and pagerank centrality come in second and third, respectively. Interestingly, betweenness centrality yields the best pAUROC. As we set p = 0.05, 5% of false positive rate was considered. It indicates to what level the high scores could represent disease-related genes. Closeness centrality also provides the best performance for AUPRC and Top 100. On the average, nearly 62% of all Top 100 detected genes are true positives or true disease-related genes.
Since ND requires an initial score for the seed nodes, which are the disease-related genes in our study, the ranking performance using the ND score is higher than network centrality, which does not use disease-gene association data. Network centrality was calculated directly based on the structure of the network without the information of known disease-related genes, while ND had prior information on some known disease-related genes and their calculations based on both the structure of the network and prior knowledge on which genes are related with a disease. Therefore, the prediction results from ND are better than those from network centrality. However, the use of network centrality that can capture how topologically important a node is in the network can be used to improve the performance of ND. The combination of the ND and centrality for each disease can be formulated as shown in Equation 9 (see Materials and Methods). The weight parameter was learned using binary logistic regression for a disease . Two terms of rescaled network centrality and ND were used as features and the initial score was used as a class. The rescaled coefficients were then used as parameter md for each disease, as presented in Supplementary Table T2.
Table 3 shows the mean values and the standard deviations of the performances of AUROC, pAUROC, AUPRC, and Top 100 for all 40 diseases. Note that ND with pagerank centrality yields the best result in the Top 100 rank hits. However, it is good in terms of accuracy. Most of the centralities combined with ND exhibit better performances in several aspects than using ND only. However, ND with eigenvector centrality yields the lowest result for pAUPRC and Top 100 performances and makes a worse prediction than ND. This result indicates that disease-related genes do not necessarily have high connections to other high-connection genes. Degree centrality is not very helpful in improving the performance of ND. To better compare how well betweenness centrality and closeness centrality could improve the predictions, the number of tested diseases in which the model could yield high performance was counted (Table 4). Although betweenness centrality exhibits good performance in AUROC values, it is only one disease better than closeness centrality. In another round, closeness centrality offers much better pAUROC, AUPRC, and Top 100 performances. Therefore, closeness centrality is suitable for integration into ND for our model.
AUROC | pAUROC | AUPRC | Top 100 | |
Network diffusion (ND) | 0.7279 ± 0.0175 | 0.1411 ± 0.0164 | 0.3458 ± 0.0680 | 49.7917 ± 9.9291 |
ND + Betweenness centrality | 0.7322 ± 0.0181 | 0.1600 ± 0.0163 | 0.3641 ± 0.0668 | 57.0167 ± 9.4824 |
ND + Closeness centrality | 0.7316 ± 0.0189 | 0.1822 ± 0.0192 | 0.3797 ± 0.0622 | 60.8500 ± 8.7981 |
ND + Degree | 0.7170 ± 0.0164 | 0.1446 ± 0.0156 | 0.3405 ± 0.0670 | 57.6500 ± 9.0467 |
ND + Eigenvector centrality | 0.7185 ± 0.0169 | 0.0935 ± 0.0129 | 0.3045 ± 0.0686 | 18.2083 ± 7.9535 |
ND + PageRank centrality | 0.7241 ± 0.0176 | 0.1569 ± 0.0164 | 0.3561 ± 0.0648 | 61.0333 ± 9.1980 |
Network diffusion (ND) | ND + Betweenness centrality | ND + Closeness centrality | ||
AUROC | ≥ 0.75 | 4 | 6 | 5 |
≥ 0.70 | 35 | 37 | 36 | |
≥ 0.65 | 40 | 40 | 40 | |
pAUROC | ≥ 0.20 | 0 | 1 | 6 |
≥ 0.15 | 8 | 30 | 39 | |
≥ 0.10 | 40 | 40 | 40 | |
AUPRC | ≥ 0.50 | 2 | 2 | 3 |
≥ 0.40 | 7 | 8 | 11 | |
≥ 0.30 | 29 | 35 | 39 | |
Top 100 | ≥ 70 | 2 | 4 | 7 |
≥ 60 | 7 | 12 | 18 | |
≥ 50 | 16 | 31 | 37 |
Table 3 and Table 4 confirm the results in Table 2 and Figure 3 that betweenness centrality and closeness centrality are of interest to be combined with the ND scores. Figures 4 and 5 represents the performance plots for each disease when applying each centrality to the ND. We found that for 40 diseases, on the average, ND with betweenness centrality performs a bit better than that with closeness centrality, as shown in Table 3. There is no difference in the performance AUROC when either betweenness or closeness centrality is used. However, when considering the other performances, which are pAUROC, AUPRC, and Top 100 measures, it turns out that ND with closeness centrality yields a better performance, as shown in Table 4, which presents the number of diseases whose performances were greater than a certain threshold. Note that this indicates that it has better precision in recognizing disease-related genes for most diseases. Furthermore, for the calculation of the shortest paths, closeness centrality can be calculated faster than betweenness centrality. Therefore, we propose the final model for a disease d based on the closeness centrality, which can be calculated as
(10) |
to be our prioritization method to identify the disease-related genes for each disease. The obtained coefficients with p-value are shown in Supplementary Table T3. Note that all disease models have p-values that are less than the significance level of 0.05, implying that both predictors are meaningful.
We also compared our result with those generated by the state-of-the art-methods in [12]. First, the baseline method is applied for each disease using none of the disease-gene associations. "Random" permutes the input score of labels or randomly identifies the gene relation to each disease with the same number of genes as the goal standard. "Randomraw" also permutes the input score of labels and then computes the diffusion score to represent the effect from the network. "PageRank with a uniform prior (pr)" [26] equally diffuses the score from each node. "Extending Guilt by Association' by Degree (EGAD)" [27] uses the PPI network and assesses how well it groups known sets of genes. Next, we also compared our result with those of semi-supervised learning methods. The "K-nearest neighbors (KNN)" and "Weighted Sum with Linear Decay (wlsd)" [28] use techniques of label propagation and random walk algorithms, choosing parameter and coefficient of linear decay .
Focusing on the diffusion approaches in this work, we already included the definition of raw diffusion (raw) in the methodology. However, the method needs improvement, and several studies have developed raw diffusion. "GeneMania-based weights (gm)" [29] is similar to raw diffusion, defining -1 on negative, 1 on positive, and 0 on unknown. "Monte Carlo normalized scores (mc)" [30] uses the concept of statistical normalization to compute the probability that the score will be greater than when randomly permuted. The p-value is computed using 100 permutation times. "Z-scores (z)" [30] reduces the computational time of mc using expectation and variance. Using a different kernel, "Personalized PageRank (ppr)" [31] also diffuses the score from the initial labels.
We showed that the diffusion approaches, especially our combination method, yields better performance than both the baseline method and semi-supervised learning method (Figs. 6–9). For the baseline method, EGAD performs best, followed by pr, when validated with AUROC and AUPRC. However, the result generated by EGAD when validated with pAUROC and Top 100 is similar to that of random and randomraw, which are unsatisfied. Moreover, pagerank is categorized as inappropriate for ranking when compared with the other centrality measures. Thus, using only network interaction is not sufficient for identifying disease-gene relations.
The developed methods using the association between disease and gene or semi-supervised learning methods are also considered. The results of KNN and WSLD are better than that of the baseline method, but still need improvement.
Compared with other diffusion methods, ppr demonstrates the lowest performance in AUROC. The use of the regularized Laplacian matrix might be effective. As we considered raw diffusion, the improved method of gm is similar in this work since we also considered two classes, i.e., known and unknown. However, the use of mc and z yields similar result as that of raw diffusion, which is not appropriate for application in our work and is too time consuming for statistical normalization.
The proposed combination method performs best based on AUROC, pAUROC and AUPRC. However, its performance in the Top 100 identification is still limited.
To identify the disease-related genes, our final model with closeness centrality in Equation 10 (see Results) was used for all 40 diseases. Five instances ten-fold cross-validation were performed for each disease. The genes that were found in the Top 50 predicted genes for each disease in all five rounds were selected as the most likely to be disease-related genes for a certain disease. Based on this criterion, the list of our proposed disease-related genes is shown in Table 5. Genes that are not found in the DisGeNET database are proposed as new disease-related genes. Meanwhile, some genes were verified through a literature search on the PubMed database. To search for literature support, the easyPubMed R package was applied for all proposed genes of each disease. Afterward, manual curation was performed to select only the most relevant literature. In Table 5, the newly proposed genes based on our method were marked as "*" to indicate one to four literature support and "**" to indicate five or more literature support. For example, SPINK6 and STK39 have been reported to be related to non-small cell type lung cancer (NSCLC), including adenocarcinoma [32,33,34]. Moreover, our approach found that AKTIP and CNTFR are related to Alzheimer's disease, in agreement with previous research [35,36,37,38,39]. The full list of all disease-related genes for all 40 diseases, as well as, the literature support, can be found in Supplementary Table T4 and T5.
Disease | Proposed disease-related genes that are not found in DisGeNET | |
1 | Adenocarcinoma | SPINK6*, CYP2R1*, GSTM5*, SCGB1D2*, CLDN12*, JAML, FJX1*, PTGDS*, GLO1**, CLDN23*, METAP1* |
2 | Glioblastoma | PCSK2, NANP, JAML, CD6, PRDM14, PMAIP1*, IL17RE, ZBTB33, XBP1**, PFKFB2*, MITF*, BID*, IL20RA |
3 | Schizophrenia | GABRA2**, DLGAP4*, GABRA3*, NLGN3*, HOMER3 |
4 | Alzheimer's Disease | TBXAS1, CNTFR*, AKTIP*, FTS**, FAM160A2, FCN1, MAG*, ZBTB33* |
5 | Rheumatoid Arthritis | IL20RB*, SPN*, TNFRSF4**, JAML, SELPLG*, IL20RA*, TNFSF8* |
6 | Malignant neoplasm of breast | IL17RA*, PRSS57, HSD17B3* |
7 | Malignant tumor of colon | JAML, PRDM14*, S100PBP, POR*, UGT1A10**, PMAIP1*, TBXAS1*, RAG2* |
8 | Diabetes Mellitus | IL17RA**, DUOXA2, NKX6-1**, SCG5**, SGNE1*, CIDEA**, POGZ, TRPM8*, PPRC1, FABP6*, TFR2**, TBXAS1*, BTLA* |
9 | Melanoma | NCR3**, DIABLO*, JAML, STAT5A*, POGZ, TP53BP2*, TIGAR* |
10 | Neoplasm Metastasis | MFAP2*, MFAP5, CLDN12* |
11 | Obesity | TNFSF4, TNLG2B, EPHX1**, HSD17B2* |
12 | Prostatic Neoplasms | HSD17B2**, HSD17B6*, CYP11B1*, AKR1C2**, CYP2R1*, AKR1C4*, S100PBP, AKR1D1, CD6, HGFAC, WT1*, ZBTB33*, HSD11B1*, PTGES**, GSTM2* |
13 | Malignant neoplasm of lung | CHRNB2, GSTM5*, JAML, DIABLO*, MAP3K4*, FJX1*, GSR*, HEL-75 |
14 | Malignant neoplasm of prostate | AKR1C1**, CYP11B1*, AKR1D1, TXN2, PTGIS*, HSD11B1* |
15 | Prostate carcinoma | CYP11B2*, CYP11B1*, AKR1C1*, AKR1D1*, DNAJC1, ASCC3 |
16 | Breast Carcinoma | HSD3B2*, HSD17B3*, UGT1A4** |
17 | Liver carcinoma | HSD17B2*, HSD17B7*, HSD3B1* |
18 | Squamous cell carcinoma | PKP2*, CYP2B6, DSC1*, PKP3, JAML, TBXAS1, CYP3A4*, CYP2C9**, CD6* |
19 | Colorectal Carcinoma | UGT1A4*, RTCB, UGT1A8*, UGT1A3*, C2orf49, ZNRF3*, TBXAS1* |
20 | Leukemia | DUOXA2, HOXA6*, HOXB5*, HOXB6*, FBXO45, TCF12* |
21 | Malignant neoplasm of stomach | POGZ, PORCN*, TBXAS1, PAX9*, NAXE, FJX1, HGFAC* |
22 | Neuroblastoma | DCT, POGZ*, PANX1*, METAP1, PMEL, MITF*, RAG2, CNTFR*, NEUROG3*, BID*, MSRB2, DIABLO*, AIFM1*, BCL2L1*, PAX9* |
23 | Pancreatic carcinoma | IL17RA, IL25, S100P**, RAG1, DHPS*, NKX6-1, C1GALT1, BID, BTLA |
24 | Malignant neoplasm of pancreas | IL17RA, TRPA1*, ASCC2, DHPS, BTLA*, C1GALT1* |
25 | Carcinogenesis | IL17RE, IL17B, UGT1A10*, TXN2*, TBXAS1*, UGT1A9*, TCF3*, GSTM5* |
26 | Carcinoma of lung | SLC24A5, JAML, STK3*, TRPV1*, ZBTB33*, NEIL1*, QTRT2, CD6, CYP2C8* |
27 | Colon Carcinoma | UGT1A4*, UGT1A10*, UGT1A3*, HCST, PRDM14, JAML, UGT1A8*, DIABLO*, S100PBP, CD6, APPL1, HAVCR2* |
28 | Stomach Carcinoma | CLDN12*, POGZ, LHPP, WNT4, PTGIS, PORCN, WNT8A |
29 | Central neuroblastoma | POGZ, METAP1, PMEL, MITF, PRDX1, ABCD4, BCL2L1*, BID, IL17RE |
30 | Primary malignant neoplasm of lung | GSTM5, TNFRSF8, TXN2, SFTPC*, PCSK2*, CD6, UGT1A3, UGT1A10, NEIL1* |
31 | Mammary Neoplasms | EPHX1*, TBXAS1*, HSD3B2*, SRD5A1*, TNFRSF18, RERE*, HPGDS*, JAML, CYP4A11*, CYP11B2*, HSD17B3*, CD6* |
32 | Colorectal Cancer | UGT1A3*, RTCB, ZBTB8OS, UGT1A8*, C2orf49, RSPO1*, ZNRF3*, TBXAS1*, UGT1A4* |
33 | Non-Small Cell Lung Carcinoma | SPINK6, PUM2*, PTGDS*, DHPS, DIABLO*, PRDM14*, UGT1A3*, TSNAX, UGT1A10*, DOHH, BBC3* |
34 | Diabetes Mellitus, Non-Insulin-Dependent | NPPC, SCG5*, SGNE1*, ATP2A1*, HSD17B6, SELPLG*, CYP24A1* |
35 | Glioma | PCSK2, MLANA*, IL17RE, LINGO1*, POGZ, SPINT1*, ZBTB33*, BID, BCL2L11* |
36 | Leukemia, Myelocytic, Acute | HOXA6*, HOXC6*, HOXC5*, JAML*, TNFRSF8*, TNFRSF4*, LGALS9*, SECTM1*, DRG1, BID |
37 | Ovarian Carcinoma | DNAJC1, MFAP2, JAML, POGZ, ZBTB33, FASLG, TNFSF6 |
38 | Tumor Progression | HCST, JAML, TRPV1*, NCR3*, DHPS*, MYOCD, PANX1*, IL22RA1*, FBLN2, METAP1* |
39 | Malignant neoplasm of ovary | DNAJC1, MFAP2, CYP2R1*, MST1*, DHPS, PSTPIP1, TNFSF9*, TNLG5A |
40 | Intellectual Disability | ALG1, PIGP*, PIGM*, KCNJ8* |
Graph-based techniques such as ND and network centrality, are widely and commonly used to prioritize and identify disease-related genes. ND has an advantage in terms of the use of initial scores from known disease-related genes while network centrality focuses on the essentiality of a node based on its connections and locations that reflect the topological community of the network. In this work, applying ND alone yielded an acceptable performance that was obviously better than using centrality alone. However, the combination of ND and network centrality improved the prediction performance substantially, indicating its efficacy in identifying new disease-related genes.
Disparate aspects were noted for the different centrality measures. Closeness centrality works best in explaining disease-related genes in the PPI network in our study. Closeness centrality determines that a certain gene is in the central position, that most of the genes in the network are close to it, and that they can reach it in the shortest way compared with the others. It reflects that disease-related genes are more likely to be reachable in the network community. The second-best centrality is betweenness centrality, which explains the load of the shortest paths for a node in the network. This centrality measure also manifests better performance when combined with ND. However, it could not improve precision much because its high scores not only reflect the load but also account for high connections, as measured by degree centrality. Most of the high-connection nodes also have high loads of the shortest path, but high-load nodes do not necessarily have high connections. As expected, ND with degree centrality provides better performance, but the different is not that notable. Since the constructed PPI network follows the power-law distribution that is common in many real-world networks, the network contains a high number of low-degree nodes and a small number of high-degree nodes. High-degree nodes (or high-connection nodes) are known to be related to the essentiality of the genes or proteins in the network and are not highly associated with the disease-related genes. Meanwhile, eigenvector centrality does not improve the prediction at all, but it reveals that disease-related genes are not involved with the high-connection nodes in the network. Therefore, closeness centrality is an effective measure to determine which genes are related to a disease and, when combined with ND, may be used to propose new disease-related genes in this work.
To propose new disease-related genes, five instances of ten-fold cross-validations were performed for each disease. Genes that commonly appeared in the Top 50 predicted genes for each run were reported as related to a disease with high confidence and some literature support. Some of these predicted genes are already in the DisGeNET database, while the rest are proposed as new disease-related genes. Notably, many of the new proposed disease-related genes found literature support, while the remaining ones comprised a great starting point for investigating disease treatment.
A new measure that can be used for the identification of disease-related genes based on ND and network centrality is proposed in this work. Through a constructed PPI network, ND and five network centralities consisting of betweenness, closeness, degree, eigenvector, and pagerank were calculated for each gene in the network and for each disease separately. Closeness centrality with ND was the best performer in many circumstances and was used to predict new potential disease-related genes. The results show that the top-ranking genes are highly related to the biological evidence, which is beneficial in developing the model for identification tasks and applying it to other diseases. All in all, our simple and effective method constitutes a great tool for pharmaceutical tasks and drug development.
This research was funded by Thailand Science Research and Innovation Fund, and King Mongkut's University of Technology North Bangkok with Contract no. KMUTNB-BasicR-64-33-3. Panisa Janyasupab was partially supported by Research Assistantship Fund, Faculty of Science, Chulalongkorn University. We would like to acknowledge National e-Science Infrastructure Consortium (http://www.e-science.in.th) for kindly supporting the high-performance computing resources.
The authors have no conflict of interest.
[1] |
A.-L. Barabási, N. Gulbahce, J. Loscalzo, Network medicine: A network-based approach to human disease, Nat. Rev. Genet., 12 (2011), 56-68. doi: 10.1038/nrg2918
![]() |
[2] |
M. Caldera, P. Buphamalai, F. Mueller, J. Menche, Interactome-based approaches to human disease, Curr. Opin. Syst. Biol., 3 (2017), 88-94. doi: 10.1016/j.coisb.2017.04.015
![]() |
[3] | E. K. Silverman, H. Schmidt, E. Anastasiadou, L. Altucci, M. Angelini, L. Badimon, et al., Molecular networks in network medicine: development and applications, Wiley Interdiscip. Rev. Syst. Biol. Med., 12 (2020), e1489. |
[4] |
P. Paci, G. Fiscon, F. Conte, R.-S. Wang, L. Farina, J. Loscalzo, Gene co-expression in the interactome: moving from correlation toward causation via an integrated approach to disease module discovery, NPJ Syst. Biol. Appl., 7 (2021), 3. doi: 10.1038/s41540-020-00168-0
![]() |
[5] |
P. Paci, G. Fiscon, F. Conte, V. Licursi, J. Morrow, C. Hersh, et al., Integrated transcriptomic correlation network analysis identifies COPD molecular determinants, Sci. Rep., 10 (2020), 3361. doi: 10.1038/s41598-020-60228-7
![]() |
[6] |
G. Fiscon, F. Conte, V. Licursi, S. Nasi, P. Paci, Computational identification of specific genes for glioblastoma stem-like cells identity, Sci. Rep., 8 (2018), 7769. doi: 10.1038/s41598-018-26081-5
![]() |
[7] |
N. T. Doncheva, T. Kacprowski, M. Albrecht, Recent approaches to the prioritization of candidate disease genes, Wiley Interdiscip. Rev. Syst. Biol. Med., 4 (2012), 429-442. doi: 10.1002/wsbm.1177
![]() |
[8] |
A. Suratanee, K. Plaimas, Identification of inflammatory bowel disease-related proteins using a reverse k-nearest neighbor search, J. Bioinform. Comput. Biol., 12 (2014), 1450017. doi: 10.1142/S0219720014500176
![]() |
[9] | W. Guo, D.-M. Shang, J.-H. Cao, K. Feng, Y.-C. He, Y. Jiang, et al., Identifying and analyzing novel epilepsy-related genes using random walk with restart algorithm, Biomed. Res. Int., 2017 (2017), 1-14. |
[10] |
S. D. Ghiassian, J. Menche, A.-L. Barabási, A DIseAse MOdule Detection (DIAMOnD) algorithm derived from a systematic analysis of connectivity patterns of disease proteins in the human interactome, PLoS Comput. Biol., 11 (2015), e1004120. doi: 10.1371/journal.pcbi.1004120
![]() |
[11] |
P. Paci, T. Colombo, G. Fiscon, A. Gurtner, G. Pavesi, L. Farina, SWIM: A computational tool to unveiling crucial nodes in complex biological networks, Sci. Rep., 7 (2017), 44797. doi: 10.1038/srep44797
![]() |
[12] |
S. Picart-Armada, S. J. Barrett, D. R. Wille, A. Perera-Lluna, A. Gutteridge, B. H. Dessailly, Benchmarking network propagation methods for disease gene identification, PLoS Comput. Biol., 15 (2019), e1007276. doi: 10.1371/journal.pcbi.1007276
![]() |
[13] |
D. Lancour, A. Naj, R. Mayeux, J. L. Haines, M. A. Pericak-Vance, G. D. Schellenberg, et al., One for all and all for one: Improving replication of genetic studies through network diffusion, PLoS Genet., 14 (2018), e1007306. doi: 10.1371/journal.pgen.1007306
![]() |
[14] |
S. Picart-Armada, W. K. Thompson, A. Buil, A. Perera-Lluna, diffuStats: An R package to compute diffusion-based scores on biological networks, Bioinformatics, 34 (2018), 533-534. doi: 10.1093/bioinformatics/btx632
![]() |
[15] |
E. Mosca, M. Bersanelli, M. Gnocchi, M. Moscatelli, G. Castellani, L. Milanesi, et al., Network diffusion-based prioritization of autism risk genes identifies significantly connected gene modules, Front. Genet., 8 (2017), 129. doi: 10.3389/fgene.2017.00129
![]() |
[16] |
M. Bersanelli, E. Mosca, D. Remondini, G. Castellani, L. Milanesi, Network diffusion-based analysis of high-throughput data for the detection of differentially enriched modules, Sci. Rep., 6 (2016), 34841. doi: 10.1038/srep34841
![]() |
[17] |
A. Hill, S. Gleim, F. Kiefer, F. Sigoillot, J. Loureiro, J. Jenkins, et al., Benchmarking network algorithms for contextualizing genes of interest, PLoS Comput. Biol., 15 (2019), e1007403. doi: 10.1371/journal.pcbi.1007403
![]() |
[18] |
A. Al-Aamri, K. Taha, Y. Al-Hammadi, M. Maalouf, D. Homouz, Analyzing a co-occurrence gene-interaction network to identify disease-gene association, BMC Bioinform., 20 (2019), 70. doi: 10.1186/s12859-019-2634-7
![]() |
[19] |
X. Zhao, Z.-P. Liu, Analysis of topological parameters of complex disease genes reveals the importance of location in a biomolecular network, Genes, 10 (2019), 143. doi: 10.3390/genes10020143
![]() |
[20] | S. Izudheen, E. S. Sajan, I. George, J. John, C. S. Attipetty, Effect of community structures in protein--protein interaction network in cancer protein identification, Curr. Sci., 118 (2020), 62. |
[21] |
D. Szklarczyk, A. L. Gable, D. Lyon, A. Junge, S. Wyder, J. Huerta-Cepas, et al., STRING v11: Protein--protein association networks with increased coverage, supporting functional discovery in genome-wide experimental datasets, Nucleic Acids Res., 47 (2019), D607-D613. doi: 10.1093/nar/gky1131
![]() |
[22] | J. Pinero, N. Queralt-Rosinach, A. Bravo, J. Deu-Pons, A. Bauer-Mehren, M. Baron, et al., DisGeNET: A discovery platform for the dynamical exploration of human diseases and their genes. Database: the journal of biological databases and curation. 2015; 2015: bav028: Epub 2015/04/17. doi: 10.1093/database/bav028.PubMedPMID:25877637. |
[23] | A. J. Smola, R. Kondor, Kernels and regularization on graphs, Learn. theory kernel Mach., Springer, (2003), 144-158. |
[24] | P. Y. Chebotarev, E. Shamis, The matrix-forest theorem and measuring relations in small social Groups, arXiv, 58 (1997), 1505-1514. |
[25] |
A.-L. Barabási, R. Albert, Emergence of scaling in random networks, Science, 286 (1999), 509. doi: 10.1126/science.286.5439.509
![]() |
[26] | L. Page, S. Brin, R. Motwani, T. Winograd, The PageRank citation ranking: Bringing order to the web, 1999, 1-17. |
[27] | S. Ballouz, M. Weber, P. Pavlidis, J. Gillis, EGAD: Ultra-fast functional analysis of gene networks, Bioinformatics, 33 (2017), 612-614. |
[28] |
G. Valentini, G. Armano, M. Frasca, J. Lin, M. Mesiti, M. Re, RANKS: A flexible tool for node label ranking and classification in biological networks, Bioinformatics, 32(2016), 2872-2874. doi: 10.1093/bioinformatics/btw235
![]() |
[29] | S. Mostafavi, D. Ray, D. Warde-Farley, C. Grouios, Q. Morris, GeneMANIA: A real-time multiple association network integration algorithm for predicting gene function, Genome Biol., 9 (2008), S4. |
[30] |
S. Picart-Armada, F. Fernández-Albert, M. Vinaixa, M. A. Rodríguez, S. Aivio, T. H. Stracker, et al., Null diffusion-based enrichment for metabolomics data, PLoS One, 12 (2017), e0189012. doi: 10.1371/journal.pone.0177197
![]() |
[31] |
B. Jiang, K. Kloster, D. F. Gleich, M. Gribskov, AptRank: An adaptive PageRank model for protein function prediction on bi-relational graphs, Bioinformatics, 33 (2017), 1829-1836. doi: 10.1093/bioinformatics/btx029
![]() |
[32] |
Y. Zhang, R.-q. He, Y.-w. Dang, X.-l. Zhang, X. Wang, S.-n. Huang, et al., Comprehensive analysis of the long noncoding RNA HOXA11-AS gene interaction regulatory network in NSCLC cells, Cancer Cell Int., 16 (2016), 89. doi: 10.1186/s12935-016-0366-6
![]() |
[33] | K. Ge, J. Huang, W. Wang, M. Gu, X. Dai, Y. Xu, et al., Serine protease inhibitor kazal-type 6 inhibits tumorigenesis of human hepatocellular carcinoma cells via its extracellular action, Oncotarget, 8 (2016), 5965-5975. |
[34] | J. Li, X. Wang, J. Yang, S. Zhao, T. Liu, L. Wang, Identification of hub genes in Hepatocellular Carcinoma related to progression and prognosis by weighted gene co-expression network analysis, Med. Sci. Monit, 26 (2020), e920854. |
[35] |
Y. J. Sung, T.W. Winkler, L. de Las Fuentes, A. R. Bentley, M. R. Brown, A. T. Kraja, et al., A large-scale multi-ancestry genome-wide study accounting for smoking behavior identifies multiple significant loci for blood pressure, Am. J. Hum. Genet., 102 (2018), 375-400. doi: 10.1016/j.ajhg.2018.01.015
![]() |
[36] |
S. Pasquin, M. Sharma, J.-F. Gauchat, Ciliary neurotrophic factor (CNTF): New facets of an old molecule for treating neurodegenerative and metabolic syndrome pathologies, Cytokine Growth Factor Rev., 26 (2015), 507-515. doi: 10.1016/j.cytogfr.2015.07.007
![]() |
[37] |
C. Conejero-Goldberg, T. M. Hyde, S. Chen, U. Dreses-Werringloer, M. M. Herman, J. E. Kleinman, et al., Molecular signatures in post-mortem brain tissue of younger individuals at high risk for Alzheimer's disease as based on APOE genotype, Mol. Psychiatry, 16 (2011), 836-847. doi: 10.1038/mp.2010.57
![]() |
[38] |
Y. Hashimoto, M. Kurita, M. Matsuoka, Identification of soluble WSX-1 not as a dominant-negative but as an alternative functional subunit of a receptor for an anti-Alzheimer's disease rescue factor Humanin, Biochem. Biophys. Res. Commun., 389 (2009), 95-99. doi: 10.1016/j.bbrc.2009.08.095
![]() |
[39] |
Y. Hashimoto, M. Kurita, S. Aiso, I. Nishimoto, M. Matsuoka, Humanin inhibits neuronal cell death by interacting with a cytokine receptor complex or complexes Involving CNTF Receptor/WSX-1/gp130, Mol. Biol. Cell, 20 (2009), 2864-2873. doi: 10.1091/mbc.e09-02-0168
![]() |
![]() |
![]() |
1. | Pakorn Sagulkoo, Apichat Suratanee, Kitiporn Plaimas, Immune-Related Protein Interaction Network in Severe COVID-19 Patients toward the Identification of Key Proteins and Drug Repurposing, 2022, 12, 2218-273X, 690, 10.3390/biom12050690 | |
2. | Piyanut Tangmanussukum, Thitipong Kawichai, Apichat Suratanee, Kitiporn Plaimas, 2021, Heterogeneous Network Propagation with Optimal Similarity Measure for Drug-Target Associations, 978-1-6654-1197-4, 155, 10.1109/ICSEC53205.2021.9684655 | |
3. | Pakorn Sagulkoo, Apichat Suratanee, Kitiporn Plaimas, 2022, Network-based methods with heterogeneous data to identify severe COVID immune-related genes, 978-1-6654-9198-3, 334, 10.1109/ICSEC56337.2022.10049313 | |
4. | Satanat Kitsiranuwat, Apichat Suratanee, Kitiporn Plaimas, Integration of various protein similarities using random forest technique to infer augmented drug-protein matrix for enhancing drug-disease association prediction, 2022, 105, 0036-8504, 003685042211092, 10.1177/00368504221109215 | |
5. | Pakorn Sagulkoo, Hathaichanok Chuntakaruk, Thanyada Rungrotmongkol, Apichat Suratanee, Kitiporn Plaimas, Multi-Level Biological Network Analysis and Drug Repurposing Based on Leukocyte Transcriptomics in Severe COVID-19: In Silico Systems Biology to Precision Medicine, 2022, 12, 2075-4426, 1030, 10.3390/jpm12071030 | |
6. | Panisa Janyasupab, Apichat Suratanee, Kitiporn Plaimas, 2022, Heterogeneous data analysis of hypertrophic cardiomyopathy to prioritize important genes, 978-1-6654-9198-3, 325, 10.1109/ICSEC56337.2022.10049332 | |
7. | Piyanut Tangmanussukum, Thitipong Kawichai, Apichat Suratanee, Kitiporn Plaimas, Heterogeneous network propagation with forward similarity integration to enhance drug–target association prediction, 2022, 8, 2376-5992, e1124, 10.7717/peerj-cs.1124 | |
8. | Thasni M A, Susan George, 2022, Structural analysis of Air Transport Network using Network indicators, 978-1-6654-9175-4, 1, 10.1109/IPRECON55716.2022.10059483 | |
9. | Paola Stolfi, Andrea Mastropietro, Giuseppe Pasculli, Paolo Tieri, Davide Vergni, Pier Luigi Martelli, NIAPU: network-informed adaptive positive-unlabeled learning for disease gene identification, 2023, 39, 1367-4811, 10.1093/bioinformatics/btac848 | |
10. | Apichat Suratanee, Kitiporn Plaimas, Gene Association Classification for Autism Spectrum Disorder: Leveraging Gene Embedding and Differential Gene Expression Profiles to Identify Disease-Related Genes, 2023, 13, 2076-3417, 8980, 10.3390/app13158980 | |
11. | Nirmala Parisutham, The Impact of Centrality Measures in Protein–Protein Interaction Networks: Tools, Databases, Challenges and Future Directions, 2024, 23, 2737-4165, 815, 10.1142/S2737416524400076 | |
12. | Ernesto Estrada, Communicability cosine distance: similarity and symmetry in graphs/networks, 2024, 43, 2238-3603, 10.1007/s40314-023-02571-9 | |
13. | Kenan Menguc, Alper Yilmaz, Improving model performance of shortest‐path‐based centrality measures in network models through scale space, 2024, 36, 1532-0626, 10.1002/cpe.8082 | |
14. | Panisa Janyasupab, Kodchanan Singhanat, Malee Warnnissorn, Peti Thuwajit, Apichat Suratanee, Kitiporn Plaimas, Chanitra Thuwajit, Identification of Tumor Budding-Associated Genes in Breast Cancer through Transcriptomic Profiling and Network Diffusion Analysis, 2024, 14, 2218-273X, 896, 10.3390/biom14080896 | |
15. | David B Blumenthal, Marta Lucchetta, Linda Kleist, Sándor P Fekete, Markus List, Martin H Schaefer, Emergence of power law distributions in protein-protein interaction networks through study bias, 2024, 13, 2050-084X, 10.7554/eLife.99951 |
Gold Standard | |||
Positive | Negative | ||
Predicted | Positive | True Positive (TP) | False Positive (FP) |
Negative | False Negative (FN) | True Negative (TN) |
AUROC | pAUROC | AUPRC | Top 100 | |
Betweenness centrality | 0.6532 ± 0.0150 | 0.1027 ± 0.0065 | 0.2754 ± 0.0633 | 57.6000 ± 9.7212 |
Closeness centrality | 0.6645 ± 0.0335 | 0.0940 ± 0.0173 | 0.2840 ± 0.0773 | 61.8000 ± 13.0977 |
Degree | 0.6048 ± 0.0164 | 0.0380 ± 0.0130 | 0.1970 ± 0.0591 | 22.2250 ± 6.8444 |
Eigenvector centrality | 0.5862 ± 0.0265 | 0.0125 ± 0.0033 | 0.1750 ± 0.0584 | 9.5750 ± 5.8172 |
PageRank centrality | 0.6128 ± 0.0137 | 0.0784 ± 0.0122 | 0.2366 ± 0.0613 | 50.0500 ± 9.9123 |
AUROC | pAUROC | AUPRC | Top 100 | |
Network diffusion (ND) | 0.7279 ± 0.0175 | 0.1411 ± 0.0164 | 0.3458 ± 0.0680 | 49.7917 ± 9.9291 |
ND + Betweenness centrality | 0.7322 ± 0.0181 | 0.1600 ± 0.0163 | 0.3641 ± 0.0668 | 57.0167 ± 9.4824 |
ND + Closeness centrality | 0.7316 ± 0.0189 | 0.1822 ± 0.0192 | 0.3797 ± 0.0622 | 60.8500 ± 8.7981 |
ND + Degree | 0.7170 ± 0.0164 | 0.1446 ± 0.0156 | 0.3405 ± 0.0670 | 57.6500 ± 9.0467 |
ND + Eigenvector centrality | 0.7185 ± 0.0169 | 0.0935 ± 0.0129 | 0.3045 ± 0.0686 | 18.2083 ± 7.9535 |
ND + PageRank centrality | 0.7241 ± 0.0176 | 0.1569 ± 0.0164 | 0.3561 ± 0.0648 | 61.0333 ± 9.1980 |
Network diffusion (ND) | ND + Betweenness centrality | ND + Closeness centrality | ||
AUROC | ≥ 0.75 | 4 | 6 | 5 |
≥ 0.70 | 35 | 37 | 36 | |
≥ 0.65 | 40 | 40 | 40 | |
pAUROC | ≥ 0.20 | 0 | 1 | 6 |
≥ 0.15 | 8 | 30 | 39 | |
≥ 0.10 | 40 | 40 | 40 | |
AUPRC | ≥ 0.50 | 2 | 2 | 3 |
≥ 0.40 | 7 | 8 | 11 | |
≥ 0.30 | 29 | 35 | 39 | |
Top 100 | ≥ 70 | 2 | 4 | 7 |
≥ 60 | 7 | 12 | 18 | |
≥ 50 | 16 | 31 | 37 |
Disease | Proposed disease-related genes that are not found in DisGeNET | |
1 | Adenocarcinoma | SPINK6*, CYP2R1*, GSTM5*, SCGB1D2*, CLDN12*, JAML, FJX1*, PTGDS*, GLO1**, CLDN23*, METAP1* |
2 | Glioblastoma | PCSK2, NANP, JAML, CD6, PRDM14, PMAIP1*, IL17RE, ZBTB33, XBP1**, PFKFB2*, MITF*, BID*, IL20RA |
3 | Schizophrenia | GABRA2**, DLGAP4*, GABRA3*, NLGN3*, HOMER3 |
4 | Alzheimer's Disease | TBXAS1, CNTFR*, AKTIP*, FTS**, FAM160A2, FCN1, MAG*, ZBTB33* |
5 | Rheumatoid Arthritis | IL20RB*, SPN*, TNFRSF4**, JAML, SELPLG*, IL20RA*, TNFSF8* |
6 | Malignant neoplasm of breast | IL17RA*, PRSS57, HSD17B3* |
7 | Malignant tumor of colon | JAML, PRDM14*, S100PBP, POR*, UGT1A10**, PMAIP1*, TBXAS1*, RAG2* |
8 | Diabetes Mellitus | IL17RA**, DUOXA2, NKX6-1**, SCG5**, SGNE1*, CIDEA**, POGZ, TRPM8*, PPRC1, FABP6*, TFR2**, TBXAS1*, BTLA* |
9 | Melanoma | NCR3**, DIABLO*, JAML, STAT5A*, POGZ, TP53BP2*, TIGAR* |
10 | Neoplasm Metastasis | MFAP2*, MFAP5, CLDN12* |
11 | Obesity | TNFSF4, TNLG2B, EPHX1**, HSD17B2* |
12 | Prostatic Neoplasms | HSD17B2**, HSD17B6*, CYP11B1*, AKR1C2**, CYP2R1*, AKR1C4*, S100PBP, AKR1D1, CD6, HGFAC, WT1*, ZBTB33*, HSD11B1*, PTGES**, GSTM2* |
13 | Malignant neoplasm of lung | CHRNB2, GSTM5*, JAML, DIABLO*, MAP3K4*, FJX1*, GSR*, HEL-75 |
14 | Malignant neoplasm of prostate | AKR1C1**, CYP11B1*, AKR1D1, TXN2, PTGIS*, HSD11B1* |
15 | Prostate carcinoma | CYP11B2*, CYP11B1*, AKR1C1*, AKR1D1*, DNAJC1, ASCC3 |
16 | Breast Carcinoma | HSD3B2*, HSD17B3*, UGT1A4** |
17 | Liver carcinoma | HSD17B2*, HSD17B7*, HSD3B1* |
18 | Squamous cell carcinoma | PKP2*, CYP2B6, DSC1*, PKP3, JAML, TBXAS1, CYP3A4*, CYP2C9**, CD6* |
19 | Colorectal Carcinoma | UGT1A4*, RTCB, UGT1A8*, UGT1A3*, C2orf49, ZNRF3*, TBXAS1* |
20 | Leukemia | DUOXA2, HOXA6*, HOXB5*, HOXB6*, FBXO45, TCF12* |
21 | Malignant neoplasm of stomach | POGZ, PORCN*, TBXAS1, PAX9*, NAXE, FJX1, HGFAC* |
22 | Neuroblastoma | DCT, POGZ*, PANX1*, METAP1, PMEL, MITF*, RAG2, CNTFR*, NEUROG3*, BID*, MSRB2, DIABLO*, AIFM1*, BCL2L1*, PAX9* |
23 | Pancreatic carcinoma | IL17RA, IL25, S100P**, RAG1, DHPS*, NKX6-1, C1GALT1, BID, BTLA |
24 | Malignant neoplasm of pancreas | IL17RA, TRPA1*, ASCC2, DHPS, BTLA*, C1GALT1* |
25 | Carcinogenesis | IL17RE, IL17B, UGT1A10*, TXN2*, TBXAS1*, UGT1A9*, TCF3*, GSTM5* |
26 | Carcinoma of lung | SLC24A5, JAML, STK3*, TRPV1*, ZBTB33*, NEIL1*, QTRT2, CD6, CYP2C8* |
27 | Colon Carcinoma | UGT1A4*, UGT1A10*, UGT1A3*, HCST, PRDM14, JAML, UGT1A8*, DIABLO*, S100PBP, CD6, APPL1, HAVCR2* |
28 | Stomach Carcinoma | CLDN12*, POGZ, LHPP, WNT4, PTGIS, PORCN, WNT8A |
29 | Central neuroblastoma | POGZ, METAP1, PMEL, MITF, PRDX1, ABCD4, BCL2L1*, BID, IL17RE |
30 | Primary malignant neoplasm of lung | GSTM5, TNFRSF8, TXN2, SFTPC*, PCSK2*, CD6, UGT1A3, UGT1A10, NEIL1* |
31 | Mammary Neoplasms | EPHX1*, TBXAS1*, HSD3B2*, SRD5A1*, TNFRSF18, RERE*, HPGDS*, JAML, CYP4A11*, CYP11B2*, HSD17B3*, CD6* |
32 | Colorectal Cancer | UGT1A3*, RTCB, ZBTB8OS, UGT1A8*, C2orf49, RSPO1*, ZNRF3*, TBXAS1*, UGT1A4* |
33 | Non-Small Cell Lung Carcinoma | SPINK6, PUM2*, PTGDS*, DHPS, DIABLO*, PRDM14*, UGT1A3*, TSNAX, UGT1A10*, DOHH, BBC3* |
34 | Diabetes Mellitus, Non-Insulin-Dependent | NPPC, SCG5*, SGNE1*, ATP2A1*, HSD17B6, SELPLG*, CYP24A1* |
35 | Glioma | PCSK2, MLANA*, IL17RE, LINGO1*, POGZ, SPINT1*, ZBTB33*, BID, BCL2L11* |
36 | Leukemia, Myelocytic, Acute | HOXA6*, HOXC6*, HOXC5*, JAML*, TNFRSF8*, TNFRSF4*, LGALS9*, SECTM1*, DRG1, BID |
37 | Ovarian Carcinoma | DNAJC1, MFAP2, JAML, POGZ, ZBTB33, FASLG, TNFSF6 |
38 | Tumor Progression | HCST, JAML, TRPV1*, NCR3*, DHPS*, MYOCD, PANX1*, IL22RA1*, FBLN2, METAP1* |
39 | Malignant neoplasm of ovary | DNAJC1, MFAP2, CYP2R1*, MST1*, DHPS, PSTPIP1, TNFSF9*, TNLG5A |
40 | Intellectual Disability | ALG1, PIGP*, PIGM*, KCNJ8* |
Gold Standard | |||
Positive | Negative | ||
Predicted | Positive | True Positive (TP) | False Positive (FP) |
Negative | False Negative (FN) | True Negative (TN) |
AUROC | pAUROC | AUPRC | Top 100 | |
Betweenness centrality | 0.6532 ± 0.0150 | 0.1027 ± 0.0065 | 0.2754 ± 0.0633 | 57.6000 ± 9.7212 |
Closeness centrality | 0.6645 ± 0.0335 | 0.0940 ± 0.0173 | 0.2840 ± 0.0773 | 61.8000 ± 13.0977 |
Degree | 0.6048 ± 0.0164 | 0.0380 ± 0.0130 | 0.1970 ± 0.0591 | 22.2250 ± 6.8444 |
Eigenvector centrality | 0.5862 ± 0.0265 | 0.0125 ± 0.0033 | 0.1750 ± 0.0584 | 9.5750 ± 5.8172 |
PageRank centrality | 0.6128 ± 0.0137 | 0.0784 ± 0.0122 | 0.2366 ± 0.0613 | 50.0500 ± 9.9123 |
AUROC | pAUROC | AUPRC | Top 100 | |
Network diffusion (ND) | 0.7279 ± 0.0175 | 0.1411 ± 0.0164 | 0.3458 ± 0.0680 | 49.7917 ± 9.9291 |
ND + Betweenness centrality | 0.7322 ± 0.0181 | 0.1600 ± 0.0163 | 0.3641 ± 0.0668 | 57.0167 ± 9.4824 |
ND + Closeness centrality | 0.7316 ± 0.0189 | 0.1822 ± 0.0192 | 0.3797 ± 0.0622 | 60.8500 ± 8.7981 |
ND + Degree | 0.7170 ± 0.0164 | 0.1446 ± 0.0156 | 0.3405 ± 0.0670 | 57.6500 ± 9.0467 |
ND + Eigenvector centrality | 0.7185 ± 0.0169 | 0.0935 ± 0.0129 | 0.3045 ± 0.0686 | 18.2083 ± 7.9535 |
ND + PageRank centrality | 0.7241 ± 0.0176 | 0.1569 ± 0.0164 | 0.3561 ± 0.0648 | 61.0333 ± 9.1980 |
Network diffusion (ND) | ND + Betweenness centrality | ND + Closeness centrality | ||
AUROC | ≥ 0.75 | 4 | 6 | 5 |
≥ 0.70 | 35 | 37 | 36 | |
≥ 0.65 | 40 | 40 | 40 | |
pAUROC | ≥ 0.20 | 0 | 1 | 6 |
≥ 0.15 | 8 | 30 | 39 | |
≥ 0.10 | 40 | 40 | 40 | |
AUPRC | ≥ 0.50 | 2 | 2 | 3 |
≥ 0.40 | 7 | 8 | 11 | |
≥ 0.30 | 29 | 35 | 39 | |
Top 100 | ≥ 70 | 2 | 4 | 7 |
≥ 60 | 7 | 12 | 18 | |
≥ 50 | 16 | 31 | 37 |
Disease | Proposed disease-related genes that are not found in DisGeNET | |
1 | Adenocarcinoma | SPINK6*, CYP2R1*, GSTM5*, SCGB1D2*, CLDN12*, JAML, FJX1*, PTGDS*, GLO1**, CLDN23*, METAP1* |
2 | Glioblastoma | PCSK2, NANP, JAML, CD6, PRDM14, PMAIP1*, IL17RE, ZBTB33, XBP1**, PFKFB2*, MITF*, BID*, IL20RA |
3 | Schizophrenia | GABRA2**, DLGAP4*, GABRA3*, NLGN3*, HOMER3 |
4 | Alzheimer's Disease | TBXAS1, CNTFR*, AKTIP*, FTS**, FAM160A2, FCN1, MAG*, ZBTB33* |
5 | Rheumatoid Arthritis | IL20RB*, SPN*, TNFRSF4**, JAML, SELPLG*, IL20RA*, TNFSF8* |
6 | Malignant neoplasm of breast | IL17RA*, PRSS57, HSD17B3* |
7 | Malignant tumor of colon | JAML, PRDM14*, S100PBP, POR*, UGT1A10**, PMAIP1*, TBXAS1*, RAG2* |
8 | Diabetes Mellitus | IL17RA**, DUOXA2, NKX6-1**, SCG5**, SGNE1*, CIDEA**, POGZ, TRPM8*, PPRC1, FABP6*, TFR2**, TBXAS1*, BTLA* |
9 | Melanoma | NCR3**, DIABLO*, JAML, STAT5A*, POGZ, TP53BP2*, TIGAR* |
10 | Neoplasm Metastasis | MFAP2*, MFAP5, CLDN12* |
11 | Obesity | TNFSF4, TNLG2B, EPHX1**, HSD17B2* |
12 | Prostatic Neoplasms | HSD17B2**, HSD17B6*, CYP11B1*, AKR1C2**, CYP2R1*, AKR1C4*, S100PBP, AKR1D1, CD6, HGFAC, WT1*, ZBTB33*, HSD11B1*, PTGES**, GSTM2* |
13 | Malignant neoplasm of lung | CHRNB2, GSTM5*, JAML, DIABLO*, MAP3K4*, FJX1*, GSR*, HEL-75 |
14 | Malignant neoplasm of prostate | AKR1C1**, CYP11B1*, AKR1D1, TXN2, PTGIS*, HSD11B1* |
15 | Prostate carcinoma | CYP11B2*, CYP11B1*, AKR1C1*, AKR1D1*, DNAJC1, ASCC3 |
16 | Breast Carcinoma | HSD3B2*, HSD17B3*, UGT1A4** |
17 | Liver carcinoma | HSD17B2*, HSD17B7*, HSD3B1* |
18 | Squamous cell carcinoma | PKP2*, CYP2B6, DSC1*, PKP3, JAML, TBXAS1, CYP3A4*, CYP2C9**, CD6* |
19 | Colorectal Carcinoma | UGT1A4*, RTCB, UGT1A8*, UGT1A3*, C2orf49, ZNRF3*, TBXAS1* |
20 | Leukemia | DUOXA2, HOXA6*, HOXB5*, HOXB6*, FBXO45, TCF12* |
21 | Malignant neoplasm of stomach | POGZ, PORCN*, TBXAS1, PAX9*, NAXE, FJX1, HGFAC* |
22 | Neuroblastoma | DCT, POGZ*, PANX1*, METAP1, PMEL, MITF*, RAG2, CNTFR*, NEUROG3*, BID*, MSRB2, DIABLO*, AIFM1*, BCL2L1*, PAX9* |
23 | Pancreatic carcinoma | IL17RA, IL25, S100P**, RAG1, DHPS*, NKX6-1, C1GALT1, BID, BTLA |
24 | Malignant neoplasm of pancreas | IL17RA, TRPA1*, ASCC2, DHPS, BTLA*, C1GALT1* |
25 | Carcinogenesis | IL17RE, IL17B, UGT1A10*, TXN2*, TBXAS1*, UGT1A9*, TCF3*, GSTM5* |
26 | Carcinoma of lung | SLC24A5, JAML, STK3*, TRPV1*, ZBTB33*, NEIL1*, QTRT2, CD6, CYP2C8* |
27 | Colon Carcinoma | UGT1A4*, UGT1A10*, UGT1A3*, HCST, PRDM14, JAML, UGT1A8*, DIABLO*, S100PBP, CD6, APPL1, HAVCR2* |
28 | Stomach Carcinoma | CLDN12*, POGZ, LHPP, WNT4, PTGIS, PORCN, WNT8A |
29 | Central neuroblastoma | POGZ, METAP1, PMEL, MITF, PRDX1, ABCD4, BCL2L1*, BID, IL17RE |
30 | Primary malignant neoplasm of lung | GSTM5, TNFRSF8, TXN2, SFTPC*, PCSK2*, CD6, UGT1A3, UGT1A10, NEIL1* |
31 | Mammary Neoplasms | EPHX1*, TBXAS1*, HSD3B2*, SRD5A1*, TNFRSF18, RERE*, HPGDS*, JAML, CYP4A11*, CYP11B2*, HSD17B3*, CD6* |
32 | Colorectal Cancer | UGT1A3*, RTCB, ZBTB8OS, UGT1A8*, C2orf49, RSPO1*, ZNRF3*, TBXAS1*, UGT1A4* |
33 | Non-Small Cell Lung Carcinoma | SPINK6, PUM2*, PTGDS*, DHPS, DIABLO*, PRDM14*, UGT1A3*, TSNAX, UGT1A10*, DOHH, BBC3* |
34 | Diabetes Mellitus, Non-Insulin-Dependent | NPPC, SCG5*, SGNE1*, ATP2A1*, HSD17B6, SELPLG*, CYP24A1* |
35 | Glioma | PCSK2, MLANA*, IL17RE, LINGO1*, POGZ, SPINT1*, ZBTB33*, BID, BCL2L11* |
36 | Leukemia, Myelocytic, Acute | HOXA6*, HOXC6*, HOXC5*, JAML*, TNFRSF8*, TNFRSF4*, LGALS9*, SECTM1*, DRG1, BID |
37 | Ovarian Carcinoma | DNAJC1, MFAP2, JAML, POGZ, ZBTB33, FASLG, TNFSF6 |
38 | Tumor Progression | HCST, JAML, TRPV1*, NCR3*, DHPS*, MYOCD, PANX1*, IL22RA1*, FBLN2, METAP1* |
39 | Malignant neoplasm of ovary | DNAJC1, MFAP2, CYP2R1*, MST1*, DHPS, PSTPIP1, TNFSF9*, TNLG5A |
40 | Intellectual Disability | ALG1, PIGP*, PIGM*, KCNJ8* |