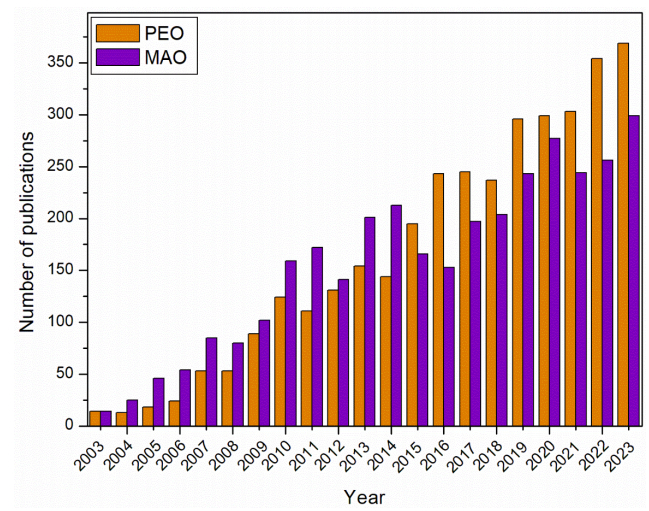
Identifying key proteins based on protein-protein interaction networks has emerged as a prominent area of research in bioinformatics. However, current methods exhibit certain limitations, such as the omission of subcellular localization information and the disregard for the impact of topological structure noise on the reliability of key protein identification. Moreover, the influence of proteins outside a complex but interacting with proteins inside the complex on complex participation tends to be overlooked. Addressing these shortcomings, this paper presents a novel method for key protein identification that integrates protein complex information with multiple biological features. This approach offers a comprehensive evaluation of protein importance by considering subcellular localization centrality, topological centrality weighted by gene ontology (GO) similarity and complex participation centrality. Experimental results, including traditional statistical metrics, jackknife methodology metric and key protein overlap or difference, demonstrate that the proposed method not only achieves higher accuracy in identifying key proteins compared to nine classical methods but also exhibits robustness across diverse protein-protein interaction networks.
Citation: Yongyin Han, Maolin Liu, Zhixiao Wang. Key protein identification by integrating protein complex information and multi-biological features[J]. Mathematical Biosciences and Engineering, 2023, 20(10): 18191-18206. doi: 10.3934/mbe.2023808
[1] | Jie Sun, Tzvetanka Boiadjieva-Scherzer, Hermann Kronberger . Incorporation of zirconium into PEO coating on Ti6Al4V alloy from acidic electrolyte. AIMS Materials Science, 2021, 8(6): 974-989. doi: 10.3934/matersci.2021059 |
[2] | Rafael Resende Lucas, Rita de Cássia Mendonça Sales-Contini, Luis Felipe Barbosa Marques, Jonas Frank Reis, Ana Beatriz Ramos Moreira Abrahão, Edson Cocchieri Botelho, Rogério Pinto Mota . Characterization of the hybrid joint between AA2024-T3 alloy and thermoplastic composite obtained by oxy-fuel welding (OFW). AIMS Materials Science, 2024, 11(3): 585-601. doi: 10.3934/matersci.2024029 |
[3] | Rucheng Zhu, Yota Mabuchi, Riteshkumar Vishwakarma, Balaram Paudel Jaisi, Haibin Li, Masami Naito, Masayoshi Umeno, Tetsuo Soga . Enhancing in-plane uniformity of graphene nanowalls using a rotating platform for solid-state lithium-ion battery. AIMS Materials Science, 2024, 11(4): 760-773. doi: 10.3934/matersci.2024037 |
[4] | Shaimaa Mazhar Mahdi, Majeed Ali Habeeb . Low-cost piezoelectric sensors and gamma ray attenuation fabricated from novel polymeric nanocomposites. AIMS Materials Science, 2023, 10(2): 288-300. doi: 10.3934/matersci.2023015 |
[5] | Bauyrzhan Rakhadilov, Lyaila Bayatanova, Sherzod Kurbanbekov, Ravil Sulyubayev, Nurdaulet Shektibayev, Nurbol Berdimuratov . Investigation on the effect of technological parameters of electrolyte-plasma cementation method on phase structure and mechanical properties of structural steel 20X. AIMS Materials Science, 2023, 10(5): 934-947. doi: 10.3934/matersci.2023050 |
[6] | Didar Yeskermessov, Bauyrzhan Rakhadilov, Laila Zhurerova, Akbota Apsezhanova, Zarina Aringozhina, Matthew Booth, Yerkezhan Tabiyeva . Surface modification of coatings based on Ni-Cr-Al by pulsed plasma treatment. AIMS Materials Science, 2023, 10(5): 755-766. doi: 10.3934/matersci.2023042 |
[7] | Min Lai, Xiaofang Yang, Qing Liu, Jinghua Li, Yanhua Hou, Xiuyong Chen, Kaiyong Cai . The surface nanostructures of titanium alloy regulate the proliferation of endothelial cells. AIMS Materials Science, 2014, 1(1): 45-58. doi: 10.3934/matersci.2014.1.45 |
[8] | Bauyrzhan Rakhadilov, Moldir Bayandinova, Rinat Kussainov, Almasbek Maulit . Electrolyte-plasma surface hardening of hollow steel applicator needles for point injection of liquid mineral fertilizers. AIMS Materials Science, 2024, 11(2): 295-308. doi: 10.3934/matersci.2024016 |
[9] | Yernat Kozhakhmetov, Mazhyn Skakov, Wojciech Wieleba, Kurbanbekov Sherzod, Nuriya Mukhamedova . Evolution of intermetallic compounds in Ti-Al-Nb system by the action of mechanoactivation and spark plasma sintering. AIMS Materials Science, 2020, 7(2): 182-191. doi: 10.3934/matersci.2020.2.182 |
[10] | Bauyrzhan Rakhadilov, Rinat Kussainov, Aisulu Kalitova, Zarina Satbayeva, Aibek Shynarbek . The impact of technological parameters of electrolytic-plasma treatment on the changes in the mechano-tribological properties of steel 45. AIMS Materials Science, 2024, 11(4): 666-683. doi: 10.3934/matersci.2024034 |
Identifying key proteins based on protein-protein interaction networks has emerged as a prominent area of research in bioinformatics. However, current methods exhibit certain limitations, such as the omission of subcellular localization information and the disregard for the impact of topological structure noise on the reliability of key protein identification. Moreover, the influence of proteins outside a complex but interacting with proteins inside the complex on complex participation tends to be overlooked. Addressing these shortcomings, this paper presents a novel method for key protein identification that integrates protein complex information with multiple biological features. This approach offers a comprehensive evaluation of protein importance by considering subcellular localization centrality, topological centrality weighted by gene ontology (GO) similarity and complex participation centrality. Experimental results, including traditional statistical metrics, jackknife methodology metric and key protein overlap or difference, demonstrate that the proposed method not only achieves higher accuracy in identifying key proteins compared to nine classical methods but also exhibits robustness across diverse protein-protein interaction networks.
Aluminum alloys are widely used in several industrial sectors, from basic products like pots and cutlery to components requiring extreme properties, such as in the case of aeronautical structures and substructures. To meet these requirements, aluminum is processed with the addition of alloy elements such as Cu, Zn, Fe, Ti, Mg, and Mn, giving rise to alloy families like 2XXX, 3XXX, and 4XXX [1]. However, alloyed aluminum is susceptible to localized corrosion, known as "pitting", due to the formation of micro-galvanic couples within the aluminum matrix. Additionally, it is characterized by relatively low abrasion resistance and can induce a stress-concentration region when subjected to loads. To mitigate this problem, surface treatments are developed to reduce corrosion and increase scratch resistance [1,2].
Aluminum and titanium alloys are also highly versatile and widely used, including in applications such as dental and orthopedic prostheses and aeronautical components (e.g., Ti-6Al-4V), among others. Although titanium is highly reactive, it exhibits good passivation by forming a thin oxide layer, mainly TiO2 (depending on its chemical composition). However, this passivation is highly dependent on factors such as alloy composition, surface finish, surface area, part geometry, and porosity, among others. This makes the alloy highly susceptible to attacks in environments rich in Cl− ions due to the passive coating's heterogeneous formation [3,4,5,6,7].
For this, electrochemical surface treatments have been developed, such as anodization and derived processes, such as plasma anodization, known as plasma electrolytic oxidation (PEO), and micro-arc oxidation (MAO), among others. These treatments aim to develop a thin oxide layer on lightweight alloys, adherent to the substrate, with higher hardness values than untreated material, in addition to improving physical and chemical characteristics [8,9,10,11]. Moreover, the PEO process is very similar to conventional electrochemical processes. It should be considered a completely distinct process mainly due to the presence of electrolysis in the aqueous medium by the application of a voltage by power supply and the occurrence of an electrical discharge on the surface's workpiece to be treated (anode). The anodization process and electrical discharge on the material's surface produce oxide coatings with optimized crystalline and morphological structures compared with those produced by conventional processes. The parameters used, such as immersion time, applied potential, current density, temperature, electrolyte composition, and anode chemistry, all strongly influence the coating's characteristics. Additionally, the plasma phenomenon allows the treatment to be carried out in a single step and shorter periods (in the order of minutes). Although the PEO process is widely used in aluminum, titanium, magnesium, and tantalum alloys, there are studies in the literature reporting that it has been applied to alloys that would be considered impossible to treat by conventional methods, such as AISI 1020 steel, copper, and brass [1,9,10,12,13,14,15]. They are usually carried out with much higher voltages than conventional processes (100–700 V) by using alkaline aqueous solutions based on silicates, aluminates, phosphates, etc.
Given this, the PEO process has been attracting a lot of attention from academia and technology companies, as it is an environmentally friendly process and provides advanced properties to treated materials, ensuring greater added value to new products. Over the last two decades, there has been a significant increase in publications on related topics, as shown in Figure 1.
The purpose of this study is to conduct a brief case study presenting the application of conventional anodization treatment on aluminum and titanium parts, comparing them with parts anodized by the PEO process. The aim is to demonstrate to the scientific and industrial community the advantages of replacing conventional processes with plasma treatment.
When aluminum is immersed in an aqueous medium, the metal oxidizes, releasing Al3+ ions (Eq 1). Depending on the solution (neutral or slightly alkaline), the reduction of dissolved oxygen occurs (Eq 2).
Al(s)→Al3+(aq)+3e− | (1) |
O2(g)+2H2O(l)+4e−→4OH−(aq) | (2) |
With the first two equations, it is possible to derive the overall redox reaction of aluminum (Eq 3) and elucidate the formation of hydrated aluminum hydroxide (Eq 4) due to the reaction between Al3+ ions and OH−.
4Al(s)+3O2(g)+6H2O(l)→4Al3+(Aq)+12OH−(Aq) | (3) |
Al3+(Aq)+3OH−(Aq)→Al(OH)3(s) | (4) |
In solutions rich in Cl− ions, aluminum hydroxide can react to form soluble aluminum chloride (Eq 5).
Al(OH)3+3Cl−→AlCl3+3OH− | (5) |
Cl− ions accelerate pitting corrosion, as the local region becomes more acidic due to the hydrolysis of aluminum ions [16,17,18].
The redox process of titanium is similar to that of oxygen, except for photocatalysis. Initially, titanium oxidizes, releasing Ti4+ ions (Eq 6), while the reduction of oxygen occurs simultaneously, as shown in Eq 2.
Ti(s)→Ti4+(Aq)+4e− | (6) |
Due to its photocatalytic properties, titanium dioxide (TiO2) forms electron-hole pairs (Eq 7) [19,20].
TiO2+hv→TiO2(e−+h+) | (7) |
The excited electrons and generated holes can participate in oxidation and reduction reactions. The hole (h+) can oxidize water molecules, making the medium more acidic and producing hydroxyl radicals (•OH) (Eq 8). Meanwhile, the electrons (e−) reduce the dissolved oxygen (Eq 9).
H2O+h+→•OH+H+ | (8) |
O2+4e−+2H2O→4OH− | (9) |
With the formation of Ti4+ ions (Eq 6) and OH− groups (Eq 9), they react to form titanium hydroxide (Eq 10).
Ti4+(Aq)+4OH−(Aq)→Ti(OH)4 | (10) |
In studies involving the oxidation of titanium alloy with 99.997% purity and different processing temperatures, Vaquila et al. [21] reported that oxidation processes below 200 ℃ resulted in the presence of only the TiO2 phase on the surface. However, with increasing temperature, other phases were found, such as Ti2O3, and for thinner coatings, TiOx (where x < 2).
The Scopus database and Google Scholar were used to compile articles for the present investigation. The principal search terms encompassed plasma electrolytic oxidation, micro-arc oxidation, plasma anodization, conventional anodization, and hard anodization. Articles were chosen based on their discussion and results to facilitate a comparative evaluation with an alternative procedure. To illustrate, research focusing on the "morphological attributes of the 1XXX aluminum alloy subsequent to undergoing treatment by hard anodization" was juxtaposed with those concerning the identical alloy processed through the PEO method, and vice versa.
As a response to the challenges that metal structures face, including abrasion and corrosion, anodizing and PEO treatments aim to create an oxide layer on the workpiece within an electric field formed during treatment. Relative porosity and crystallinity can be controlled in the process by altering working parameters [22].
Among the treatment parameters in both conventional anodizing and PEO processes, immersion time, treatment temperature, voltage, and the electrolyte used can be considered (Table 1). However, when addressing the PEO process, many other parameters benefit the coating properties compared with conventional anodizing, such as duty cycle, frequency, and current density, among others (Table 2).
Parameters | Operational variations | Electrolyte | Substrate | Conclusions | Ref. |
Immersion time | 40, 80,120 min | C2H2O4 | Pure aluminum | The diameter of nanopores increased with the treatment time from 40 to 80 min. However, instability in the nanotubes' walls produced at 120 min resulted in their collapse. | [23] |
5, 10 h | NH4F (0.2 M) + H3PO4 (0.5 M) + H2O (distilled) + ethylene glycol | Titanium (99.7%) | With increasing anodization time, the pore diameter and length dimensions increased, reaching 28.7 and 284.6 nm after 5 h, and 30.0 and 376.5 nm after 10 h. | [24] | |
60, 90,120,150 min | C2H2O4 (0.4 M) | Pure aluminum | With increasing anodization time, an increase in the pores' diameter produced was observed, attributed to the rise in local temperature, leading to dissolution of the Al2O3 layer and consequently reducing corrosion resistance. | [25] | |
Temperature | 0, 10, 20, 30, 40, 50 ºC | C2H2O4 and NaOH | Sn | The increase in temperature from 0 to 30 ℃ results in larger and open pores, while temperatures of 40 and 50 ℃ lead to pore sealing. This temperature dependence also affects the coating thickness, as higher temperatures enhance the reaction kinetics of coating growth. | [26] |
20, 30, 40, 50 ºC | H3PO4 (0.4 M) | AA5052 | The temperature increase during the anodization process in the H3PO4 solution accelerated the barrier layer's formation, reducing the time from 80 s at 20 ℃ to 8 s at 50 ℃. | [27] | |
30, 40, 50 ºC | C2H2O4 | Pure aluminum | The temperature solution increase resulted in larger pore diameters and a decrease in their quantity. This also reduced the surface acidity due to fewer transported anions, despite the increase in specific surface area. | [28] | |
Voltage | 10, 20, 30 V (DC) | C2H2O4 | Pure aluminum | Increasing the applied voltage in the solution reduces the surface area, decreases pore density, and increases pore diameter. This leads to higher current per pore and electric field strength, allowing greater alumina deposition. | [28] |
16, 18, 20 V (DC) | H3PO4 | AA2024-T3 | Anodizing the AA2024-T3 alloy in H3PO4 solution revealed that increasing the voltage enhances the oxide layer's thickness. However, beyond 20 V, the thickness decreases due to elevated electrolyte temperature, resulting in the coating's dissolution. | [29] | |
5, 6, 7, 8, 12, 15 V (DC) | H2SO4 | Pure aluminum | At lower potentials (5–6 VDC), the oxide layer exhibits a thin membrane with smaller pore diameter and lower density. Increasing the voltage (12–15 VDC) enhances both the thickness and pores' density, resulting in a layer with higher void density. | [30] | |
Electrolyte | NaOH (1 M), KOH (1 M) |
NaOH (1 M), KOH (1 M) |
Ti (CP2) Ti6Al2Sn4Zr2Mo Ti6Al4V Ti Beta-C |
The NaOH solution yielded better corrosion resistance in Ti alloys due to an increase in impedance. | [3] |
H2SO4 (1 M), H3PO4 (1 M) |
H2SO4 (1 M), H3PO4 (1 M) |
Ti (CP2) Ti6Al2Sn4Zr2Mo Ti6Al4V Ti Beta-C |
H2SO4 showed a more homogeneous coating on Ti alloys with fine pores, whereas H3PO4 exhibited a coating with larger pores but fewer in quantity. | [4] | |
H2O + CH3OH (50%) + HF (1%), H2O + CH3OH (90%) + HF (1%) |
H2O + CH3OH (50%) + HF (1%), H2O + CH3OH (90%) + HF (1%) |
Pure titanium | With the increase in CH3OH concentration to 90%, the pore concentration decreases on the surface of Ti alloys, and the current density of the process becomes easily controllable. This makes the process of fabricating standardized nanomaterials better. | [31] |
Parameters | Operational variations | Electrolyte | Substrate | Conclusions | Ref. |
Immersion time | 120,210,300 s | Na2SiO3 (15 g/L) + Na3PO4 (1.5 g/L) |
AA2024-T3 | The increase in treatment time resulted in the growth of the oxide layer thickness up to 210 s; beyond this point, the coating began to dissolve, leading to a decrease in roughness. | [10] |
10, 20, 30, 40, 60 min | Na3PO4 | Magnesium alloy ML-10 | Treatment time influences coating thickness, resulting in 10 µm after 10 min and 40 µm after 60 min. Microhardness also increases, ranging from 165 to 360 HV. | [32] | |
60, 90 s | H2SO4 (1 M) + H3PO4 (1.5 M) | Ti-6Al-4V | The increase in anodization time caused the dissolution of the oxide coating, revealing higher levels of Ti on the surface, while there was a decrease in surface roughness. | [33] | |
Temperature | −5, 25 ºC | K3PO4 (1 M) + KOH (1.5 M) | Mg AZ31 | Room temperature accelerated the nucleation of the coating, while temperatures below 0 ℃ produced a denser coating with smaller pores, improving its corrosion resistance. | [34] |
5, 15, 25, 35 ºC | Na2SiO3 + (NaPO3)6NH4VO3 + KF + Na-Citrate + NaOH + EDTA (Ethylenediamine tetraacetic acid) | Mg AZ31B | The electrolyte temperature altered the coating's microstructure. As the temperature increased, the pore diameters decreased and their quantity increased, while the coating thickness gradually reduced. | [35] | |
10, 20, 30, 40, 50 ºC | NaAlO2 + NaOH + Na2SiO3 | Mg AZ91D | With the increase in electrolyte temperature from 10 to 50 ℃, the surface roughness (Ra) decreased from 0.7 to 0.15 μm, and the corrosion resistance increased from 3.5 to 9 in salt spray tests. The higher temperature also raised the magnesium content in the film from 25% to 63% by weight and reduced oxygen from 66% to 21% by weight, indicating dehydration of the film. | [36] | |
Voltage | +400/−0 V, +440/−0 V, +480/−0 V, +500/−0 V, +520/−0 V |
Na2SiO3 (14 g/L) + KOH (1 g/L) | Pure aluminum | The increase in voltage and the presence of high temperatures in plasma discharge channels lead to oxidation and melting of SiC reinforcement phases, evidenced by the appearance of CO and dissolved Si elements. Additionally, it enhances the decomposition and oxidation process of the electrode. | [37] |
300,340 V (DC) | NaH2PO2·H2O + Na2SiO3·9H2O | Pure niobium | At 300 V, the formed coating is compact, both on the top and transversally. Increasing the voltage to 340 V results in a uniformly distributed porous structure, with circular pores on the top, leading to an increase in porosity from 2.32% to 26.45%. | [38] | |
350,400,450 V (DC) | Na2SiO3 + NaOH + Na2H2P2O7 | AA2024 | The coatings obtained at 350 V exhibited micropores with diameters ranging from 0.1 to 1 µm. With increasing voltage, these pores reached diameters of up to 3 µm, accompanied by an increase in length from 0.5 to 5 µm for treatments at 350 and 450 V, respectively. | [39] | |
Electrolyte | Ta(OH)5: 10, 30, 40 g/L | KOH (2 g/L) + Ta(OH)5 (varying) | AISI 1020 | The addition of Ta(OH)5 to the electrolyte increases the deposition of species in the coating, resulting in the filling of pores and cavities and in the reduction of surface irregularity. | [13] |
Ⅰ: KOH (2 g/L) + Na2SiO3 (18 g/L) + Na3PO4 (2 g/L) Ⅱ: KOH (2 g/L) + Na2SiO3 (2 g/L) + Na3PO4 (18g/L) |
Ⅰ: 2K8Si2P Ⅱ: 2K2Si18P |
AA2024 | In the silicon-based electrolyte, the breakdown voltage was 280 V and the final voltage was 455 V, resulting in a rough surface of 2.6 ± 0.2 μm and porosity of 7.1 ± 1.0%. In the phosphorus-based electrolyte, the breakdown voltage was 260 V and the final voltage was 475 V, with a lower porosity of 4.4 ± 0.3% and smoother surface. | [40] | |
Ce(NO3)3: 0.25, 0.50, 1.0, 1.5 g/L | Na2SiO3 (10 g/L) + NaOH (2 g/L) + Ce(NO3)3 |
AA6061 | With the increase in Ce(NO3)3 concentration, the thickness of the ceramic layer gradually increased, micropores and fissures increased, and roughness decreased due to the sealing of some pores. | [41] |
Tables 1 and 2 provide a comprehensive overview of the properties of oxide coatings produced through the PEO process, showing the interaction of various parameters on surface characteristics, microstructure, and functional properties, such as biocompatibility and photocatalysis [10].
Both in the conventional anodizing process and in the PEO/MAO process, the system configuration is an electrolytic cell, where two electrodes are present: the material to be treated on the positive pole (anode) and the cathode, which can be an inert material. In most of the literature, stainless steel and platinum are commonly found as cathode materials [1,42,43].
Alloys susceptible to passivation, such as aluminum and titanium, develop a thin oxide layer on their surface, in the order of nanometers, when exposed to oxygen-rich environments. As mentioned earlier, treated metal ions penetrate this barrier layer to form a new oxide layer. The electric field formed during the process surpasses the activation energy of this barrier layer, thus forming a new oxide layer [22,44,45]. Usually, for titanium alloys, the required potential is above 100 V (depending on the setup), while for aluminum alloys, Frank et al. [24] employed 25V/7A to anodize the AA2024-T3 alloy in a 10% phosphoric acid (H3PO4) solution. More recent studies have applied statistical methods to optimize working parameters in both conventional processes and plasma processes [46,47,48,49].
The parameter "electrolyte" significantly affects the composition of the generated coating. In conventional anodizing, the electrolytic composition affects the formation and dissolution of oxide films, crucial for achieving the desired surface finish [40,50]. In both conventional anodizing and the PEO process, the anion electrolytic incorporation into the anodic film is crucial, with studies showing that it may be necessary for the ignition process in materials such as aluminum, magnesium, and titanium [1,50]. Similarly, studies involving adding additives such as ceramic particles, graphene oxide, and organic particles are investigated for various purposes to functionalize the surface [51].
The immersion time significantly affects the properties of oxide coatings, both in conventional anodizing processes and plasma processes. In conventional anodizing, longer anodizing times, such as 45 min, result in greater corrosion resistance, improved adhesion, and increased oxide layer thickness, enhancing the overall coating performance [47,52]. In the PEO process, immersion time is extremely important as it leads to an increase in pore diameter and a decrease in their quantity, along with an increase in coating thickness [42,53].
The processing temperature influences the properties of coatings in both anodizing and the PEO process. In studies applying different temperatures, Guo et al. [54] anodized the AA6061 alloy with a sulfuric acid (H2SO4) solution. They found that as the temperature increases, the surface changes from a porous structure to a coral-like structure with greater thickness [34], conducting a study on plasma anodizing in a Mg alloy, comparing temperatures below zero (268 K) with room temperature (298 K). They found that coatings produced at lower temperatures had fewer cracks, thus improving the samples' corrosion resistance. However, this also resulted in a decrease in coating thickness.
The applied voltage plays a fundamental role in PEO treatment, strongly influencing gas evolution around the anode, the crystalline composition, and corrosion resistance. In studies characterizing the electrolytic plasma, Liao et al. [37] found that increasing the voltage in the treatment led to higher concentrations of H2, O2, and traces of CO. The concentration of H2 and O2 was higher in the silicate solution due to strong sparks in the anode. The applied potential can significantly influence the photocatalytic titanium alloys' properties. For example, increasing the potential causes an increase in the coating's growth rate, as well as an increase in the pore's diameter and the spacing between pores [55].
In general, electrochemical treatments significantly modify the surface of metal parts, potentially increasing or decreasing wettability, functionalizing surfaces for several applications, and enhancing wear resistance, among other benefits [22,56,57]. In Table 3, brief comparisons of the properties of coatings obtained by anodizing and the PEO process are reported.
Properties | Anodizing | PEO |
Morphology | Porous coating or nanotubes | Highly porous coating |
Thickness | To 50 µm | To 300 µm |
Crystallinity | Amorphous | Crystalline or amorphous |
Hardness | 100 to 400 HV | 300 to 1500 HV |
Adhesion | Low | High |
Corrosion resistance | Low | High |
Wettability | Hydrophilic | Hydrophilic or hydrophobic |
Operational cost | Low | High |
In a study developed by Lunder, Olsen, and Nisancioglu [61] involving the AA6060 aluminum alloy, various surface treatments were conducted to facilitate adhesion using epoxy-based adhesive bonding. The surface treatment, using conventional anodization (CA) in sulfuric acid, allowed obtaining a coating with a thickness of 0.2 µm showing better results and supporting loads between 30 to 35 MPa.
Using the same aluminum alloy AA6060-T6, Shore et al. [62] utilized the PEO process with an alkaline solution of sodium silicate (Na2SiO3) + sodium pyrophosphate (N4P2O7·10H2O) and potassium hydroxide (KOH). The parameters used were an anodic voltage of 530 V and a cathodic voltage of 190 V, with a frequency of 2.4 kHz and a duty cycle of 40%. The processes lasted for 3 to 5 min after reaching the specified voltages. After treatment, the samples were bonded with a thermo-polymerizable adhesive "Dow-Betamate 4600F" with a bonded area of 10 × 25 mm and an adhesive thickness of 0.2 mm. Heating was performed in several stages, totaling 60 min of curing, with a final temperature of 190 ℃. The coating had a thickness of 15.0 µm, with globular morphologies with an average diameter of 5 to 20 µm. There were sporadic pores in the coating (relative porosity of 10%), but it allowed good adhesion with the thermo-polymerizable adhesive, with a strength of 34.32 MPa.
It is common for surfaces coated with PEO to exhibit various distinct morphologies including pores, spherical protrusions, and coral-like morphologies. These characteristics vary depending on the electrolyte composition, and when enriched with silicon, it can result in needle-like microstructures known as mullite. This phenomenon leads to a coating's high densification and an increase in surface hardness, making it highly suitable for several technological applications [9,11,12,42,63,64].
It is important to emphasize that, despite the similar values obtained in the resistance through the single lap shear test, the PEO process can readily replace conventional anodization methods. This is due to the greater environmental efficiency, resulting from the use of a single alkaline electrolyte, as well as the unified nature of the PEO process, which substantially enhances the physical and chemical coating properties [1,12,42,62]. Some works on adhesion between aluminum and composite or aluminum with polymeric adhesives are shown in Table 4, with respective shear values.
Materials | MPa | Ref. |
Welding with oxy-gas LPG/PEI glass fiber + AA2024 (PEO) | 5.2 | [65] |
Welding with oxyacetylene/PEI glass fiber + AA2024 (PEO) | 10.7 | [48] |
Friction injection joining (F-IJ)/AA6082-T6 + PEI glass fiber | 1.1 | [66] |
Friction stir spot welding (FSSW)/AA5052 (PEO) + polypropylene | 1.36 | [67] |
Welding by YB-laser in AA2024-T3 (anodized) + PEI glass fiber | 16.0 | [68] |
Adhesive in AA6060-T6 + AA6060-T6 | 34.3 | [62] |
Gu, Zhang, and Yu [69] treated the AA5052 aluminum alloy by anodization with a mixed acid electrolyte (citric acid + sulfuric acid) to increase the corrosion resistance in simulated acid rain tests. The authors confirmed that with the correct addition of citric acid to the solution, it acts as a corrosion inhibitor, in addition to increasing the thickness of the oxide coating produced.
In a study involving PEO, Lucas, Gonçalves, and Santos [1] treated the AA5052-H34 aluminum alloy with a solution containing Na2SiO3 and several concentrations of Na3PO4 as an additive. It was observed that the crystalline phases (ɣ-Al2O3) and mullite tend to increase with the additive's increase, which was corroborated by scanning electron microscopy (SEM) analysis, as shown in Figure 2, highlighting the presence of sealed pores and improving the corrosion resistance. However, there is a limit to the proportion of additives, as an excessive increase results in the presence of amorphous phases, affecting both the morphology and corrosion behavior.
In a brief analysis, it can be observed that plasma anodization tends to offer superior corrosion resistance compared to oxide coatings produced by conventional processes. Morphologically, PEO coatings exhibit more closed pores and denser layers. This difference can be compared through data obtained by linear polarization, as demonstrated in Table 5.
Sample | Ecorr (V) | Jcorr (A/cm2) |
AA5052 (anodized) | −0.484 | 1.6 × 10−6 |
AA5052 (plasma-anodized) | −0.120 | 2.20 × 10−8 |
It is observed that the PEO coating exhibited higher nobility (more positive Ecorr) compared to the coating produced by the conventional process, representing an increase of approximately 75.2%. Considering the corrosion rate in mm/year, according to ASTM G102 standard [70], and using Eq 11, the corrosion rate equation becomes:
CR=K×jcorr×EWD | (11) |
where, CR: corrosion rate (mm/year); icorr: corrosion current density (µA/cm²); K: 3.27 × 10−3 mm·g/µA·cm/year; EW: equivalent weight (9.05 for AA5052); D: material density (g/cm³).
Considering Eq 11, the corrosion rate of AA5052 aluminum alloy anodized by the conventional process would be approximately 1.8 × 10−14 mm/year, while the alloy treated by the plasma anodization process would be 2.4 × 10−16 mm/year, a considerable decrease in the corrosion rate, highlighting the effectiveness of the PEO treatment. These results highlight the significant improvement provided by the plasma treatment, approximately 87%.
It is observed that, due to the morphological characteristics of oxide coating produced by the PEO treatment, it can offer much superior corrosion resistance compared to coatings produced by conventional anodization. This reiterates that plasma processes have the potential to replace conventional methods of surface treatment by conversion, providing more promising results by processing products with higher added value.
The process PEO facilitates the anodization of aluminum alloys (Al-Si), a task known for its difficulty when approached through traditional techniques. Pezzato et al. [71] and Valentini et al. [72] carried out PEO procedures on the AlSi10Mg alloy, incorporating a range of additives such as molybdenum, manganese, cerium, and graphene. These investigations illustrated notable enhancements in the resistance to corrosion with the inclusion of these additives in the fundamental solution. To clarify the mechanisms by which the additives improved the corrosion resistance of the oxide layer on the AlSi10Mg substrate, the authors provided a schematic representation of the infiltration of the additives into the pre-existing pores post-treatment, depicted in Figure 3.
In studies of anodization using NaOH and KOH solution, Tiburcio et al. [3] treated different titanium alloys (Ti CP2, Ti-6Al-2Sn-4Zr-4V, and Ti Beta-C), aiming to characterize the electrochemical effect of samples, especially when exposed to environments rich in NaCl and H2SO4. The morphological analysis is presented in Figure 4.
Tiburcio et al. [3] also observed that in the NaOH solution, the TiO2 oxide coating formation occurred entirely heterogeneously, displaying preferential deposition regions as well as microcracks. They reported that the Ti Beta-C alloy was the only one to show a low rate of oxide coating generation for the medium used. Regarding the coatings' thickness, it was observed that the Ti CP2 alloy presented a thickness ranging from 376 to 400 nm, the Ti-6Al-2Sn-4Zr-2Mo alloy from 1.92 to 2.63 µm, the Ti-6Al-4V alloy in the range of 23 to 25 nm, and finally, the Ti Beta-C alloy exhibited coatings with a thickness ranging from 660 to 756 nm.
In studies involving the titanium alloys VT1-0 and VT5 (Table 6), Ramazanova, Zamalitdinova, and Kovalenko [7] treated the alloys by PEO with a pulsed system (anodic/cathodic current: 250 µs/5 ms; frequency: 50 Hz; voltage: 360 to 365 V), with the process lasting 10 min, using three types of electrolytes, as described in Table 7.
Elements | VT1-0 | VT5 |
Ti | 99.0% (Min) | Balance |
Al | - | 6% |
V | - | 4% |
O | < 0.12% | < 0.20% |
N | < 0.04% | < 0.05% |
C | < 0.05% | < 0.10% |
Fe | < 0.08% | < 0.30% |
H | < 0.0015% | < 0.015% |
Electrolyte | Description | Concentration (g/L) |
A | Sodium silicate (metasilicate) 9 aqueous | 100 |
NaOH | 8.0 | |
Aluminum oxide (1.1–1.5 µm) | 20.0 | |
B | Sodium phosphoric acid 3–substituted, 12 aqueous | 70.0 |
Aluminum hydroxide (0.6 µm) | 20.0 | |
C | Sodium phosphoric acid, two-substituted, 12 aqueous | 40.0 |
Sodium tetraborate 10 aqueous | 30.0 | |
Boric acid | 22.0 | |
Ammonium fluoride (NH4F) | 10.0 | |
Aluminum oxide | 20.0 |
As a result, the authors reported a porous oxide coating, with varied thicknesses for each type of electrolyte and alloy used, as follows: VT1-0 with thicknesses of 5.0, 15.0, and 21.0 µm for electrolytes A, B, and C, respectively; VT5 with thicknesses of 7.5, 9.5, and 19.5 µm for the same electrolytes. The coatings on the alloys exhibited statistically similar thicknesses and relative porosities, with alloy VT1-0 having pores with a mean diameter of 0.4 µm and porosity of approximately 8.7%, whereas alloy VT5 exhibited pores with a mean diameter of 0.4 µm and porosity of approximately 9.2%, as shown in Figure 5.
A key observation is that PEO treatments can yield oxide coatings that are thicker and denser than with conventional processes. This is attributed to the plasma properties, which enhance the coating's physical and chemical properties. Additionally, the PEO process offers the advantage of being carried out in fewer steps, resulting in reduced processing time. Furthermore, it is environmentally friendly due to the use of "clean" electrolytes, as reported by several authors [61,62,73,74].
To investigate the wear behavior of oxide coatings produced on the Ti-6Al-4V alloy by PEO, Santos et al. [75] treated samples using the PEO process with a duty cycle of 38.5%, a frequency of 300 Hz, and potentials ranging from +250 to −24 V. In SEM analysis, the coatings exhibited characteristic PEO treatment morphologies, such as porous volcanic morphology and cracks along the coating, resulting from the rapid cooling of the surface in contact with the aqueous solution. Additionally, they displayed a brownish color, attributed to the high phosphate concentration in the electrolyte, as shown in Figure 6.
These findings are corroborated by other studies in the literature. For example, Grigoriev et al. [76] observed similar morphological characteristics in oxide coatings produced by PEO on the Ti-6Al-4V alloy, where the porosity and cracks were attributed to the same rapid cooling mechanisms. Additionally, they found that the addition of GO decreases the coefficient of friction due to the formation of a lubricating layer.
The coatings exhibited an average thickness ranging from 2.05 to 23.83 µm, with an inner layer varying from 0.41 to 1.16 µm, which is responsible for the increased wear resistance, as reported by other authors [9,14,15,62].
Santos et al. [75] emphasized that the produced coatings exhibit a significant increase in hardness, ranging between 400 and 600 HV, compared to the 332 HV (uncoated substrate). This increase correlates with the improved treated material wear, which showed a loss rate of 2.19 × 10−6 mm3/s compared to the untreated substrate with 9.79 × 10−5 mm3/s (an improvement of 99.79%).
In the literature, explanations for the formation of micropores attribute it to the development of sparks (micro-arcs) that occur randomly across the sample surface due to the strong electric field. This phenomenon tends to generate very high, localized temperatures (10000–25000 K), causing alumina liquefaction and gas production. These pores result from the release of these gases, according to the illustration in Figure 7 [9,11,48,77].
Initially, due to the passivation characteristic of the substrate, a thin dense barrier layer forms on the substrate within the electrolyte solution (Figure 7a). As the applied potential increases, the barrier layer ruptures due to strong electric fields, resulting in the presence of random arcs on the surface, generally around 100 to 120 V, depending on the system configuration (Figure 7b). As the treatment time extends, the density of the electric arcs increases, raising the temperature of the solution and favoring the oxidation reaction. Literature shows that the oxide coating grows not only above the substrate but also penetrates it, allowing for better anchoring of the coating to the substrate (Figure 7c) [73,74,77].
In essence, the breakdown mechanism of the passive oxide layer involves three sequential stages: (Ⅰ) first, the rupture of the passive coating occurs due to a sudden rise in temperature from the Joule effect; (Ⅱ) second, the localized aluminum melts from the intense electric discharges' high temperatures (ranging from 10000 to 25000 K), leading to its oxidation; and (Ⅲ) finally, following the cessation of discharges, the aluminum oxide cools upon contact with the liquid, subsequently being deposited on the channels' walls (pores), thereby augmenting the coating's thickness. This process is commonly referred to as "breakdown-melt-ejection-deposition" [71,72,78].
In Figure 8, the progression of voltage with respect to time in a direct current system is illustrated. This illustration may be supplemented by Figure 7, wherein Figure 7a is associated with phase I. During this phase, the PEO procedure resembles traditional anodization, characterized by a swift upsurge in voltage and the emission of gas at the anode. Phase 2 is marked by the initiation of sparking (occurring between 120 and 140 V, depending on the specific parameters), along with the formation of pores and the growth of an oxide coating, demonstrated in Figure 7b. In phase 3, the voltage escalation is less abrupt, yet it still facilitates the uninterrupted advancement of the process, as delineated in Figure 7c.
Hussein, Nie and Northwood [79] delineated two distinct growth mechanisms within the framework of the PEO process utilized for the treatment of magnesium alloys; the first is external growth, which entails the expulsion and oxidation of magnesium metallic ions, and the second is internal growth, propelled by the diffusion of oxygen and the depletion of metal within the substrate, as shown in the diagram in Figure 7. These mechanisms give rise to a proportional augmentation in coating thickness in compliance with Faraday's principles of electrolysis. Sudararajan and Krishna [80], Matykina et al. [81], and Rogov et al. [82] also deliberated upon and observed these mechanisms, underscoring the significance of comprehending both external and internal processes involved in the development of PEO coatings on magnesium alloys. This comprehension serves as a pivotal element in the optimization of coating characteristics and the reinforcement of corrosion resistance in applications involving magnesium alloys.
The PEO process and traditional anodizing techniques are widely used to improve the surface characteristics of various light alloys. Table 8 describes a juxtaposition of the main technological attributes and expenses associated with each methodology.
Characteristics | Anodizing | PEO |
Formation mechanism | Controlled oxidation in acidic solution with electric current, forming a uniform oxide layer | Electric discharges in an electrolyte solution, resulting in high local temperature and the formation of thick oxide |
Compatibility with aluminum alloys | Primarily purer aluminum alloys | Can treat alloys difficult to anodize, such as Al-Si |
Process complexity | Simpler, well-established | More complex and requires precise control of discharge parameters |
Equipment cost | Moderate, more common, and accessible equipment | High due to the need for high-voltage sources and precise control |
Operational cost | Relatively low, less energy consumption | High due to energy consumption and specialized maintenance |
Environmental impact | Can use more environmentally friendly electrolytes, but most are acidic | Can be optimized with eco-friendly electrolytes |
Typical applications | Architecture, appliances, and decorative applications | Aerospace, automotive, and biomedical industries |
The initial investment required for the installation of a PEO system is significantly elevated owing to the necessity of high-voltage sources and sophisticated control mechanisms, leading to escalated operational expenses from increased electricity consumption. Moreover, the maintenance of equipment is costlier due to the intricate nature of the system. Nevertheless, notwithstanding the considerable expenses incurred, PEO may prove to be a more cost-effective solution in the long run for scenarios where longevity and resistance to corrosion are of paramount importance, thus diminishing the frequency of replacements and repairs [83,84].
This study contributes to the existing literature with new articles published in the field of surface treatments by conventional anodization and the PEO process. It provides a detailed comparison of the physical and chemical characteristics of the generated coatings, emphasizing the improvements brought by the PEO process in terms of both product performance and environmental impact. Additionally, the PEO process offers significant environmental advantages, including reduced use of toxic chemicals and lower pollutant emissions. This comprehensive analysis reinforces the relevance of PEO as an advanced and sustainable technique for metal surface treatment. The PEO treatment has proven to be of great value both in the academic and industrial sectors for producing oxide coatings on surfaces of lightweight alloys (Al, Ti, Mg, etc.) with optimized properties.
PEO coatings demonstrate comparable or superior results to those obtained by anodizing or hard anodizing (H/A) in basic studies. Examples include research on the corrosion of AA5052 alloys, highlighting a significant improvement of approximately 87% provided by plasma treatment. This is due to the morphology of the PEO coating, which tends to develop a thicker layer and, depending on the system configuration, a denser coating, preventing the material from coming into contact with aggressive environments.
In research focused on joining materials by bonding, the PEO coating can ensure better mechanical anchoring between the joined parts due to the formation of pores, allowing the adhesive to penetrate and solidify, thus ensuring adhesion. Compared to conventional anodizing processes, the PEO process provides the same resistance when subjected to lap shear tests. These results reinforce the superiority of the coatings' characteristics produced by the PEO process, such as the greater thickness, which is attributed to the breakdown of the alumina dielectric layer, promoting more efficient growth.
Many recent works have applied statistical studies to optimize the properties of oxide coatings produced by the PEO process, reducing electricity consumption, which is still one of its weak points, making it not so attractive for low-scale productions. However, when it comes to high-value-added products, PEO applications include treating the surface of turbomolecular pump rotors due to increased abrasion resistance and structures for heat dissipation in various industries such as defense, electronics, automotive, general tools, and nuclear industries. Therefore, future research involving optimizations in energy consumption and high reproducibility will be highly necessary, both in the industrial sector, aiming to reduce costs and, consequently, the price of products, and in the academic sector.
Future research should be directed toward the development and experimental validation of optimized parameters for both the PEO and traditional anodization methods. The advancement of the PEO process, in conjunction with meticulous experimental methodologies, will play a pivotal role in enhancing coating properties, catering to industrial needs, and minimizing energy consumption. Regarding conventional anodization techniques, investigations into enhanced and eco-friendly electrolytes will emerge as notable contributions in compliance with recent regulations and environmental campaigns.
The authors declare they have not used Artificial Intelligence (AI) tools in the creation of this article.
The authors would like to thank the Coordination for the Improvement of Higher Education Personnel (CAPES) for the financial support provided under grant numbers 88887.827403/2023-00 and 88881.933644/2024-1 (PhD Scholarship–Rafael Resende Lucas).
Writing—original draft: R.R.L., R.C.M.S.C., F.J.G.S., E.C.B. and R.P.M.; writing—review and editing: R.R.L., R.C.M.S.C., F.J.G.S., E.C.B. and R.P.M.
Rita De Cássia M. Sales-Contini and Francisco J. G. Silva are on a special issue editorial board for AIMS Materials Science and were not involved in the editorial review or the decision to publish this article. All authors declare that there are no competing interests.
[1] |
L. yan Wang, Z. Zhang, Y. Li, Y. Wan, B. Xing, Integrated bioinformatic analysis of rna binding proteins in hepatocellular carcinoma, Aging (Albany NY), 13 (2020), 2480–2505. https://doi.org/10.18632/aging.202281 doi: 10.18632/aging.202281
![]() |
[2] |
X. Wang, J. Zhao, Targeted cancer therapy based on acetylation and deacetylation of key proteins involved in double-strand break repair, Cancer Manag. Res., (2022), 259–271. https://doi.org/10.2147/CMAR.S346052 doi: 10.2147/CMAR.S346052
![]() |
[3] |
Y. Yue, C. Ye, P.-Y. Peng, H.-X. Zhai, I. Ahmad, C. Xia, et al., A deep learning framework for identifying essential proteins based on multiple biological information, BMC Bioinform., 23 (2022), 318. https://doi.org/10.1186/s12859-022-04868-8 doi: 10.1186/s12859-022-04868-8
![]() |
[4] |
Y. Liu, W. Chen, Z. He, Essential protein recognition via community significance, IEEE/ACM Transactions on Computational Biology and Bioinformatics, 18 (2021), 2788–2794. https://doi.org/10.1109/TCBB.2021.3102018 doi: 10.1109/TCBB.2021.3102018
![]() |
[5] |
L. Shen, J. Zhang, F. Wang, K. Liu, Predicting essential proteins based on integration of local fuzzy fractal dimension and subcellular location information, Genes, 13 (2022), 173. https://doi.org/10.3390/genes13020173 doi: 10.3390/genes13020173
![]() |
[6] |
X.-J. Lei, Y. Gao, L. Guo, Mining protein complexes based on topology potential weight in dynamic protein-protein interaction networks, Acta Electon. Sin., 46 (2018), 145. https://doi.org/10.3969/j.issn.0372-2112.2018.01.020 doi: 10.3969/j.issn.0372-2112.2018.01.020
![]() |
[7] |
T. Tang, X. Zhang, Y. Liu, H. Peng, B. Zheng, et al., Machine learning on protein–protein interaction prediction: models, challenges and trends, Brief. Bioinform., 24 (2023), bbad076. https://doi.org/10.1093/bib/bbad076 doi: 10.1093/bib/bbad076
![]() |
[8] |
M. Li, H. Zhang, J.-x. Wang, Y. Pan, A new essential protein discovery method based on the integration of protein-protein interaction and gene expression data, BMC Syst. Biol., 6 (2012), 1–9. https://doi.org/10.1186/1752-0509-6-15 doi: 10.1186/1752-0509-6-15
![]() |
[9] |
W. Peng, J. Wang, Y. Cheng, Y. Lu, F. Wu, Y. Pan, Udonc: An algorithm for identifying essential proteins based on protein domains and protein-protein interaction networks, IEEE/ACM Transact. Comput. Biol. Bioinform., 12 (2014), 276–288. https://doi.org/10.1109/TCBB.2014.2338317 doi: 10.1109/TCBB.2014.2338317
![]() |
[10] |
X. Shang, Y. Wang, B. Chen, Identifying essential proteins based on dynamic protein-protein interaction networks and rna-seq datasets, Sci. China Inform. Sci., 59 (2016), 1–11. https://doi.org/10.1007/s11432-016-5583-z doi: 10.1007/s11432-016-5583-z
![]() |
[11] |
M. LI, X.-t. WANG, H.-m. LUO, X.-m. MENG, J.-x. WANG, Progress on random walk and its application in network biology, Acta Electon. Sin., 46 (2018), 2035. https://doi.org/10.3969/j.issn.0372-2112.2018.08.033 doi: 10.3969/j.issn.0372-2112.2018.08.033
![]() |
[12] |
M. Li, Y. Lu, Z. Niu, F.-X. Wu, United complex centrality for identification of essential proteins from ppi networks, IEEE/ACM Transact. Comput. Biol. Bioinform., 14 (2015), 370–380. https://doi.org/10.1109/TCBB.2015.2394487 doi: 10.1109/TCBB.2015.2394487
![]() |
[13] |
J. Zhong, C. Tang, W. Peng, M. Xie, Y. Sun, Q. Tang, et al., A novel essential protein identification method based on ppi networks and gene expression data, BMC Bioinform., 22 (2021), 1–21. https://doi.org/10.1186/s12859-021-04175-8 doi: 10.1186/s12859-021-04175-8
![]() |
[14] |
C. Qin, Y. Sun, Y. Dong, A new method for identifying essential proteins based on network topology properties and protein complexes, PloS One, 11 (2016), e0161042. https://doi.org/10.1371/journal.pone.0161042 doi: 10.1371/journal.pone.0161042
![]() |
[15] |
G. Yu, G. Fu, J. Wang and H. Zhu, Predicting protein function via semantic integration of multiple networks, IEEE/ACM Transact. Comput. Biol. Bioinform., 13 (2015), 220–232. https://doi.org/10.1109/TCBB.2015.2459713 doi: 10.1109/TCBB.2015.2459713
![]() |
[16] |
J. Luo, Y. Qi, Identification of essential proteins based on a new combination of local interaction density and protein complexes, PloS One, 10 (2015), e0131418. https://doi.org/10.1371/journal.pone.0131418 doi: 10.1371/journal.pone.0131418
![]() |
[17] |
X. Yang, Z. Niu, Y. Liu, B. Song, W. Lu, L. Zeng, et al., Modality-dta: Multimodality fusion strategy for drug–target affinity prediction, IEEE/ACM Transact. Comput. Biol. Bioinform., 20 (2022), 1200–1210. https://doi.org/10.1109/TCBB.2022.3205282 doi: 10.1109/TCBB.2022.3205282
![]() |
[18] |
W. Zhang, J. Xu, Y. Li, X. Zou, Detecting essential proteins based on network topology, gene expression data, and gene ontology information, IEEE/ACM Transact. Comput. Biol. Bioinform., 15 (2016), 109–116. https://doi.org/10.1109/TCBB.2016.2615931 doi: 10.1109/TCBB.2016.2615931
![]() |
[19] |
B. Chen, W. Fan, J. Liu, F.-X. Wu, Identifying protein complexes and functional modules—from static ppi networks to dynamic ppi networks, Brief. Bioinform., 15 (2014), 177–194. https://doi.org/10.1093/bib/bbt039 doi: 10.1093/bib/bbt039
![]() |
[20] |
R. R. Vallabhajosyula, D. Chakravarti, S. Lutfeali, A. Ray, A. Raval, Identifying hubs in protein interaction networks, PloS One, 4 (2009), e5344. https://doi.org/10.1371/journal.pone.0005344 doi: 10.1371/journal.pone.0005344
![]() |
[21] |
M. P. Joy, A. Brock, D. E. Ingber, S. Huang, High-betweenness proteins in the yeast protein interaction network, J. Biomed. Biotechnol., 2005 (2005), 96. https://doi.org/10.1155/JBB.2005.96 doi: 10.1155/JBB.2005.96
![]() |
[22] |
E. Estrada, J. A. Rodriguez-Velazquez, Subgraph centrality in complex networks, Phys. Rev. E, 71 (2005), 056103. https://doi.org/10.1103/PhysRevE.71.056103 doi: 10.1103/PhysRevE.71.056103
![]() |
[23] |
J. Wang, M. Li, H. Wang, Y. Pan, Identification of essential proteins based on edge clustering coefficient, IEEE/ACM Transact. Comput. Biol. Bioinform., 9 (2011), 1070–1080. https://doi.org/10.1109/TCBB.2011.147 doi: 10.1109/TCBB.2011.147
![]() |
[24] |
P. Lu, J. Yu, A mixed clustering coefficient centrality for identifying essential proteins, Int. J. Modern Phys. B, 34 (2020), 2050090. https://doi.org/10.1142/S0217979220500897 doi: 10.1142/S0217979220500897
![]() |
[25] |
I. Xenarios, L. Salwinski, X. J. Duan, P. Higney, S.-M. Kim, D. Eisenberg, Dip, the database of interacting proteins: a research tool for studying cellular networks of protein interactions, Nucleic Acids Res., 30 (2002), 303–305. https://doi.org/10.1093/nar/28.1.289 doi: 10.1093/nar/28.1.289
![]() |
[26] |
N. J. Krogan, G. Cagney, H. Yu, G. Zhong, X. Guo, A. Ignatchenko, et al., Global landscape of protein complexes in the yeast saccharomyces cerevisiae, Nature, 440 (2006), 637–643. https://doi.org/10.1038/nature04670 doi: 10.1038/nature04670
![]() |
[27] |
U. Güldener, M. Münsterkötter, M. Oesterheld, P. Pagel, A. Ruepp, H.-W. Mewes, et al., Mpact: The mips protein interaction resource on yeast, Nucleic Acids Res., 34 (2006), D436–D441. https://doi.org/10.1093/nar/gkj003 doi: 10.1093/nar/gkj003
![]() |
[28] |
G. O. Consortium, Gene ontology annotations and resources, Nucleic Acids Res., 41 (2012), D530–D535. https://doi.org/10.1093/nar/gks1050 doi: 10.1093/nar/gks1050
![]() |
[29] |
J. X. Binder, S. Pletscher-Frankild, K. Tsafou, C. Stolte, S. I. O'Donoghue, R. Schneider, et al., Compartments: Unification and visualization of protein subcellular localization evidence, Database, 2014. https://doi.org/10.1093/database/bau012 doi: 10.1093/database/bau012
![]() |
[30] |
R. Zhang, Y. Lin, Deg 5.0, a database of essential genes in both prokaryotes and eukaryotes, Nucleic Acids Res., 37 (2009), D455–D458. https://doi.org/10.1093/nar/gkn858 doi: 10.1093/nar/gkn858
![]() |
[31] |
H.-W. Mewes, C. Amid, R. Arnold, D. Frishman, U. Güldener, G. Mannhaupt, et al., Mips: Analysis and annotation of proteins from whole genomes, Nucleic Acids Res., 32 (2004), D41–D44. https://doi.org/10.1093/nar/gkh092 doi: 10.1093/nar/gkh092
![]() |
[32] |
J. M. Cherry, C. Adler, C. Ball, S. A. Chervitz, S. S. Dwight, E. T. Hester, et al., Sgd: Saccharomyces genome database, Nucleic Acids Res., 26 (1998), 73–79. https://doi.org/10.1093/nar/26.1.73 doi: 10.1093/nar/26.1.73
![]() |
[33] |
E. A. Winzeler, D. D. Shoemaker, A. Astromoff, H. Liang, K. Anderson, B. Andre, et al., Functional characterization of the s. cerevisiae genome by gene deletion and parallel analysis, Science, 285 (1999), 901–906. https://doi.org/10.1126/science.285.5429.90 doi: 10.1126/science.285.5429.90
![]() |
1. | Salvacion B. Orgen, Eden May B. Dela Pena, Microstructure and Corrosion Behavior of PEO-Coated AA7075 Under Pulsed Unipolar Potential Control Mode, 2024, 14, 2079-6412, 1498, 10.3390/coatings14121498 |
Parameters | Operational variations | Electrolyte | Substrate | Conclusions | Ref. |
Immersion time | 40, 80,120 min | C2H2O4 | Pure aluminum | The diameter of nanopores increased with the treatment time from 40 to 80 min. However, instability in the nanotubes' walls produced at 120 min resulted in their collapse. | [23] |
5, 10 h | NH4F (0.2 M) + H3PO4 (0.5 M) + H2O (distilled) + ethylene glycol | Titanium (99.7%) | With increasing anodization time, the pore diameter and length dimensions increased, reaching 28.7 and 284.6 nm after 5 h, and 30.0 and 376.5 nm after 10 h. | [24] | |
60, 90,120,150 min | C2H2O4 (0.4 M) | Pure aluminum | With increasing anodization time, an increase in the pores' diameter produced was observed, attributed to the rise in local temperature, leading to dissolution of the Al2O3 layer and consequently reducing corrosion resistance. | [25] | |
Temperature | 0, 10, 20, 30, 40, 50 ºC | C2H2O4 and NaOH | Sn | The increase in temperature from 0 to 30 ℃ results in larger and open pores, while temperatures of 40 and 50 ℃ lead to pore sealing. This temperature dependence also affects the coating thickness, as higher temperatures enhance the reaction kinetics of coating growth. | [26] |
20, 30, 40, 50 ºC | H3PO4 (0.4 M) | AA5052 | The temperature increase during the anodization process in the H3PO4 solution accelerated the barrier layer's formation, reducing the time from 80 s at 20 ℃ to 8 s at 50 ℃. | [27] | |
30, 40, 50 ºC | C2H2O4 | Pure aluminum | The temperature solution increase resulted in larger pore diameters and a decrease in their quantity. This also reduced the surface acidity due to fewer transported anions, despite the increase in specific surface area. | [28] | |
Voltage | 10, 20, 30 V (DC) | C2H2O4 | Pure aluminum | Increasing the applied voltage in the solution reduces the surface area, decreases pore density, and increases pore diameter. This leads to higher current per pore and electric field strength, allowing greater alumina deposition. | [28] |
16, 18, 20 V (DC) | H3PO4 | AA2024-T3 | Anodizing the AA2024-T3 alloy in H3PO4 solution revealed that increasing the voltage enhances the oxide layer's thickness. However, beyond 20 V, the thickness decreases due to elevated electrolyte temperature, resulting in the coating's dissolution. | [29] | |
5, 6, 7, 8, 12, 15 V (DC) | H2SO4 | Pure aluminum | At lower potentials (5–6 VDC), the oxide layer exhibits a thin membrane with smaller pore diameter and lower density. Increasing the voltage (12–15 VDC) enhances both the thickness and pores' density, resulting in a layer with higher void density. | [30] | |
Electrolyte | NaOH (1 M), KOH (1 M) |
NaOH (1 M), KOH (1 M) |
Ti (CP2) Ti6Al2Sn4Zr2Mo Ti6Al4V Ti Beta-C |
The NaOH solution yielded better corrosion resistance in Ti alloys due to an increase in impedance. | [3] |
H2SO4 (1 M), H3PO4 (1 M) |
H2SO4 (1 M), H3PO4 (1 M) |
Ti (CP2) Ti6Al2Sn4Zr2Mo Ti6Al4V Ti Beta-C |
H2SO4 showed a more homogeneous coating on Ti alloys with fine pores, whereas H3PO4 exhibited a coating with larger pores but fewer in quantity. | [4] | |
H2O + CH3OH (50%) + HF (1%), H2O + CH3OH (90%) + HF (1%) |
H2O + CH3OH (50%) + HF (1%), H2O + CH3OH (90%) + HF (1%) |
Pure titanium | With the increase in CH3OH concentration to 90%, the pore concentration decreases on the surface of Ti alloys, and the current density of the process becomes easily controllable. This makes the process of fabricating standardized nanomaterials better. | [31] |
Parameters | Operational variations | Electrolyte | Substrate | Conclusions | Ref. |
Immersion time | 120,210,300 s | Na2SiO3 (15 g/L) + Na3PO4 (1.5 g/L) |
AA2024-T3 | The increase in treatment time resulted in the growth of the oxide layer thickness up to 210 s; beyond this point, the coating began to dissolve, leading to a decrease in roughness. | [10] |
10, 20, 30, 40, 60 min | Na3PO4 | Magnesium alloy ML-10 | Treatment time influences coating thickness, resulting in 10 µm after 10 min and 40 µm after 60 min. Microhardness also increases, ranging from 165 to 360 HV. | [32] | |
60, 90 s | H2SO4 (1 M) + H3PO4 (1.5 M) | Ti-6Al-4V | The increase in anodization time caused the dissolution of the oxide coating, revealing higher levels of Ti on the surface, while there was a decrease in surface roughness. | [33] | |
Temperature | −5, 25 ºC | K3PO4 (1 M) + KOH (1.5 M) | Mg AZ31 | Room temperature accelerated the nucleation of the coating, while temperatures below 0 ℃ produced a denser coating with smaller pores, improving its corrosion resistance. | [34] |
5, 15, 25, 35 ºC | Na2SiO3 + (NaPO3)6NH4VO3 + KF + Na-Citrate + NaOH + EDTA (Ethylenediamine tetraacetic acid) | Mg AZ31B | The electrolyte temperature altered the coating's microstructure. As the temperature increased, the pore diameters decreased and their quantity increased, while the coating thickness gradually reduced. | [35] | |
10, 20, 30, 40, 50 ºC | NaAlO2 + NaOH + Na2SiO3 | Mg AZ91D | With the increase in electrolyte temperature from 10 to 50 ℃, the surface roughness (Ra) decreased from 0.7 to 0.15 μm, and the corrosion resistance increased from 3.5 to 9 in salt spray tests. The higher temperature also raised the magnesium content in the film from 25% to 63% by weight and reduced oxygen from 66% to 21% by weight, indicating dehydration of the film. | [36] | |
Voltage | +400/−0 V, +440/−0 V, +480/−0 V, +500/−0 V, +520/−0 V |
Na2SiO3 (14 g/L) + KOH (1 g/L) | Pure aluminum | The increase in voltage and the presence of high temperatures in plasma discharge channels lead to oxidation and melting of SiC reinforcement phases, evidenced by the appearance of CO and dissolved Si elements. Additionally, it enhances the decomposition and oxidation process of the electrode. | [37] |
300,340 V (DC) | NaH2PO2·H2O + Na2SiO3·9H2O | Pure niobium | At 300 V, the formed coating is compact, both on the top and transversally. Increasing the voltage to 340 V results in a uniformly distributed porous structure, with circular pores on the top, leading to an increase in porosity from 2.32% to 26.45%. | [38] | |
350,400,450 V (DC) | Na2SiO3 + NaOH + Na2H2P2O7 | AA2024 | The coatings obtained at 350 V exhibited micropores with diameters ranging from 0.1 to 1 µm. With increasing voltage, these pores reached diameters of up to 3 µm, accompanied by an increase in length from 0.5 to 5 µm for treatments at 350 and 450 V, respectively. | [39] | |
Electrolyte | Ta(OH)5: 10, 30, 40 g/L | KOH (2 g/L) + Ta(OH)5 (varying) | AISI 1020 | The addition of Ta(OH)5 to the electrolyte increases the deposition of species in the coating, resulting in the filling of pores and cavities and in the reduction of surface irregularity. | [13] |
Ⅰ: KOH (2 g/L) + Na2SiO3 (18 g/L) + Na3PO4 (2 g/L) Ⅱ: KOH (2 g/L) + Na2SiO3 (2 g/L) + Na3PO4 (18g/L) |
Ⅰ: 2K8Si2P Ⅱ: 2K2Si18P |
AA2024 | In the silicon-based electrolyte, the breakdown voltage was 280 V and the final voltage was 455 V, resulting in a rough surface of 2.6 ± 0.2 μm and porosity of 7.1 ± 1.0%. In the phosphorus-based electrolyte, the breakdown voltage was 260 V and the final voltage was 475 V, with a lower porosity of 4.4 ± 0.3% and smoother surface. | [40] | |
Ce(NO3)3: 0.25, 0.50, 1.0, 1.5 g/L | Na2SiO3 (10 g/L) + NaOH (2 g/L) + Ce(NO3)3 |
AA6061 | With the increase in Ce(NO3)3 concentration, the thickness of the ceramic layer gradually increased, micropores and fissures increased, and roughness decreased due to the sealing of some pores. | [41] |
Properties | Anodizing | PEO |
Morphology | Porous coating or nanotubes | Highly porous coating |
Thickness | To 50 µm | To 300 µm |
Crystallinity | Amorphous | Crystalline or amorphous |
Hardness | 100 to 400 HV | 300 to 1500 HV |
Adhesion | Low | High |
Corrosion resistance | Low | High |
Wettability | Hydrophilic | Hydrophilic or hydrophobic |
Operational cost | Low | High |
Materials | MPa | Ref. |
Welding with oxy-gas LPG/PEI glass fiber + AA2024 (PEO) | 5.2 | [65] |
Welding with oxyacetylene/PEI glass fiber + AA2024 (PEO) | 10.7 | [48] |
Friction injection joining (F-IJ)/AA6082-T6 + PEI glass fiber | 1.1 | [66] |
Friction stir spot welding (FSSW)/AA5052 (PEO) + polypropylene | 1.36 | [67] |
Welding by YB-laser in AA2024-T3 (anodized) + PEI glass fiber | 16.0 | [68] |
Adhesive in AA6060-T6 + AA6060-T6 | 34.3 | [62] |
Sample | Ecorr (V) | Jcorr (A/cm2) |
AA5052 (anodized) | −0.484 | 1.6 × 10−6 |
AA5052 (plasma-anodized) | −0.120 | 2.20 × 10−8 |
Elements | VT1-0 | VT5 |
Ti | 99.0% (Min) | Balance |
Al | - | 6% |
V | - | 4% |
O | < 0.12% | < 0.20% |
N | < 0.04% | < 0.05% |
C | < 0.05% | < 0.10% |
Fe | < 0.08% | < 0.30% |
H | < 0.0015% | < 0.015% |
Electrolyte | Description | Concentration (g/L) |
A | Sodium silicate (metasilicate) 9 aqueous | 100 |
NaOH | 8.0 | |
Aluminum oxide (1.1–1.5 µm) | 20.0 | |
B | Sodium phosphoric acid 3–substituted, 12 aqueous | 70.0 |
Aluminum hydroxide (0.6 µm) | 20.0 | |
C | Sodium phosphoric acid, two-substituted, 12 aqueous | 40.0 |
Sodium tetraborate 10 aqueous | 30.0 | |
Boric acid | 22.0 | |
Ammonium fluoride (NH4F) | 10.0 | |
Aluminum oxide | 20.0 |
Characteristics | Anodizing | PEO |
Formation mechanism | Controlled oxidation in acidic solution with electric current, forming a uniform oxide layer | Electric discharges in an electrolyte solution, resulting in high local temperature and the formation of thick oxide |
Compatibility with aluminum alloys | Primarily purer aluminum alloys | Can treat alloys difficult to anodize, such as Al-Si |
Process complexity | Simpler, well-established | More complex and requires precise control of discharge parameters |
Equipment cost | Moderate, more common, and accessible equipment | High due to the need for high-voltage sources and precise control |
Operational cost | Relatively low, less energy consumption | High due to energy consumption and specialized maintenance |
Environmental impact | Can use more environmentally friendly electrolytes, but most are acidic | Can be optimized with eco-friendly electrolytes |
Typical applications | Architecture, appliances, and decorative applications | Aerospace, automotive, and biomedical industries |
Parameters | Operational variations | Electrolyte | Substrate | Conclusions | Ref. |
Immersion time | 40, 80,120 min | C2H2O4 | Pure aluminum | The diameter of nanopores increased with the treatment time from 40 to 80 min. However, instability in the nanotubes' walls produced at 120 min resulted in their collapse. | [23] |
5, 10 h | NH4F (0.2 M) + H3PO4 (0.5 M) + H2O (distilled) + ethylene glycol | Titanium (99.7%) | With increasing anodization time, the pore diameter and length dimensions increased, reaching 28.7 and 284.6 nm after 5 h, and 30.0 and 376.5 nm after 10 h. | [24] | |
60, 90,120,150 min | C2H2O4 (0.4 M) | Pure aluminum | With increasing anodization time, an increase in the pores' diameter produced was observed, attributed to the rise in local temperature, leading to dissolution of the Al2O3 layer and consequently reducing corrosion resistance. | [25] | |
Temperature | 0, 10, 20, 30, 40, 50 ºC | C2H2O4 and NaOH | Sn | The increase in temperature from 0 to 30 ℃ results in larger and open pores, while temperatures of 40 and 50 ℃ lead to pore sealing. This temperature dependence also affects the coating thickness, as higher temperatures enhance the reaction kinetics of coating growth. | [26] |
20, 30, 40, 50 ºC | H3PO4 (0.4 M) | AA5052 | The temperature increase during the anodization process in the H3PO4 solution accelerated the barrier layer's formation, reducing the time from 80 s at 20 ℃ to 8 s at 50 ℃. | [27] | |
30, 40, 50 ºC | C2H2O4 | Pure aluminum | The temperature solution increase resulted in larger pore diameters and a decrease in their quantity. This also reduced the surface acidity due to fewer transported anions, despite the increase in specific surface area. | [28] | |
Voltage | 10, 20, 30 V (DC) | C2H2O4 | Pure aluminum | Increasing the applied voltage in the solution reduces the surface area, decreases pore density, and increases pore diameter. This leads to higher current per pore and electric field strength, allowing greater alumina deposition. | [28] |
16, 18, 20 V (DC) | H3PO4 | AA2024-T3 | Anodizing the AA2024-T3 alloy in H3PO4 solution revealed that increasing the voltage enhances the oxide layer's thickness. However, beyond 20 V, the thickness decreases due to elevated electrolyte temperature, resulting in the coating's dissolution. | [29] | |
5, 6, 7, 8, 12, 15 V (DC) | H2SO4 | Pure aluminum | At lower potentials (5–6 VDC), the oxide layer exhibits a thin membrane with smaller pore diameter and lower density. Increasing the voltage (12–15 VDC) enhances both the thickness and pores' density, resulting in a layer with higher void density. | [30] | |
Electrolyte | NaOH (1 M), KOH (1 M) |
NaOH (1 M), KOH (1 M) |
Ti (CP2) Ti6Al2Sn4Zr2Mo Ti6Al4V Ti Beta-C |
The NaOH solution yielded better corrosion resistance in Ti alloys due to an increase in impedance. | [3] |
H2SO4 (1 M), H3PO4 (1 M) |
H2SO4 (1 M), H3PO4 (1 M) |
Ti (CP2) Ti6Al2Sn4Zr2Mo Ti6Al4V Ti Beta-C |
H2SO4 showed a more homogeneous coating on Ti alloys with fine pores, whereas H3PO4 exhibited a coating with larger pores but fewer in quantity. | [4] | |
H2O + CH3OH (50%) + HF (1%), H2O + CH3OH (90%) + HF (1%) |
H2O + CH3OH (50%) + HF (1%), H2O + CH3OH (90%) + HF (1%) |
Pure titanium | With the increase in CH3OH concentration to 90%, the pore concentration decreases on the surface of Ti alloys, and the current density of the process becomes easily controllable. This makes the process of fabricating standardized nanomaterials better. | [31] |
Parameters | Operational variations | Electrolyte | Substrate | Conclusions | Ref. |
Immersion time | 120,210,300 s | Na2SiO3 (15 g/L) + Na3PO4 (1.5 g/L) |
AA2024-T3 | The increase in treatment time resulted in the growth of the oxide layer thickness up to 210 s; beyond this point, the coating began to dissolve, leading to a decrease in roughness. | [10] |
10, 20, 30, 40, 60 min | Na3PO4 | Magnesium alloy ML-10 | Treatment time influences coating thickness, resulting in 10 µm after 10 min and 40 µm after 60 min. Microhardness also increases, ranging from 165 to 360 HV. | [32] | |
60, 90 s | H2SO4 (1 M) + H3PO4 (1.5 M) | Ti-6Al-4V | The increase in anodization time caused the dissolution of the oxide coating, revealing higher levels of Ti on the surface, while there was a decrease in surface roughness. | [33] | |
Temperature | −5, 25 ºC | K3PO4 (1 M) + KOH (1.5 M) | Mg AZ31 | Room temperature accelerated the nucleation of the coating, while temperatures below 0 ℃ produced a denser coating with smaller pores, improving its corrosion resistance. | [34] |
5, 15, 25, 35 ºC | Na2SiO3 + (NaPO3)6NH4VO3 + KF + Na-Citrate + NaOH + EDTA (Ethylenediamine tetraacetic acid) | Mg AZ31B | The electrolyte temperature altered the coating's microstructure. As the temperature increased, the pore diameters decreased and their quantity increased, while the coating thickness gradually reduced. | [35] | |
10, 20, 30, 40, 50 ºC | NaAlO2 + NaOH + Na2SiO3 | Mg AZ91D | With the increase in electrolyte temperature from 10 to 50 ℃, the surface roughness (Ra) decreased from 0.7 to 0.15 μm, and the corrosion resistance increased from 3.5 to 9 in salt spray tests. The higher temperature also raised the magnesium content in the film from 25% to 63% by weight and reduced oxygen from 66% to 21% by weight, indicating dehydration of the film. | [36] | |
Voltage | +400/−0 V, +440/−0 V, +480/−0 V, +500/−0 V, +520/−0 V |
Na2SiO3 (14 g/L) + KOH (1 g/L) | Pure aluminum | The increase in voltage and the presence of high temperatures in plasma discharge channels lead to oxidation and melting of SiC reinforcement phases, evidenced by the appearance of CO and dissolved Si elements. Additionally, it enhances the decomposition and oxidation process of the electrode. | [37] |
300,340 V (DC) | NaH2PO2·H2O + Na2SiO3·9H2O | Pure niobium | At 300 V, the formed coating is compact, both on the top and transversally. Increasing the voltage to 340 V results in a uniformly distributed porous structure, with circular pores on the top, leading to an increase in porosity from 2.32% to 26.45%. | [38] | |
350,400,450 V (DC) | Na2SiO3 + NaOH + Na2H2P2O7 | AA2024 | The coatings obtained at 350 V exhibited micropores with diameters ranging from 0.1 to 1 µm. With increasing voltage, these pores reached diameters of up to 3 µm, accompanied by an increase in length from 0.5 to 5 µm for treatments at 350 and 450 V, respectively. | [39] | |
Electrolyte | Ta(OH)5: 10, 30, 40 g/L | KOH (2 g/L) + Ta(OH)5 (varying) | AISI 1020 | The addition of Ta(OH)5 to the electrolyte increases the deposition of species in the coating, resulting in the filling of pores and cavities and in the reduction of surface irregularity. | [13] |
Ⅰ: KOH (2 g/L) + Na2SiO3 (18 g/L) + Na3PO4 (2 g/L) Ⅱ: KOH (2 g/L) + Na2SiO3 (2 g/L) + Na3PO4 (18g/L) |
Ⅰ: 2K8Si2P Ⅱ: 2K2Si18P |
AA2024 | In the silicon-based electrolyte, the breakdown voltage was 280 V and the final voltage was 455 V, resulting in a rough surface of 2.6 ± 0.2 μm and porosity of 7.1 ± 1.0%. In the phosphorus-based electrolyte, the breakdown voltage was 260 V and the final voltage was 475 V, with a lower porosity of 4.4 ± 0.3% and smoother surface. | [40] | |
Ce(NO3)3: 0.25, 0.50, 1.0, 1.5 g/L | Na2SiO3 (10 g/L) + NaOH (2 g/L) + Ce(NO3)3 |
AA6061 | With the increase in Ce(NO3)3 concentration, the thickness of the ceramic layer gradually increased, micropores and fissures increased, and roughness decreased due to the sealing of some pores. | [41] |
Properties | Anodizing | PEO |
Morphology | Porous coating or nanotubes | Highly porous coating |
Thickness | To 50 µm | To 300 µm |
Crystallinity | Amorphous | Crystalline or amorphous |
Hardness | 100 to 400 HV | 300 to 1500 HV |
Adhesion | Low | High |
Corrosion resistance | Low | High |
Wettability | Hydrophilic | Hydrophilic or hydrophobic |
Operational cost | Low | High |
Materials | MPa | Ref. |
Welding with oxy-gas LPG/PEI glass fiber + AA2024 (PEO) | 5.2 | [65] |
Welding with oxyacetylene/PEI glass fiber + AA2024 (PEO) | 10.7 | [48] |
Friction injection joining (F-IJ)/AA6082-T6 + PEI glass fiber | 1.1 | [66] |
Friction stir spot welding (FSSW)/AA5052 (PEO) + polypropylene | 1.36 | [67] |
Welding by YB-laser in AA2024-T3 (anodized) + PEI glass fiber | 16.0 | [68] |
Adhesive in AA6060-T6 + AA6060-T6 | 34.3 | [62] |
Sample | Ecorr (V) | Jcorr (A/cm2) |
AA5052 (anodized) | −0.484 | 1.6 × 10−6 |
AA5052 (plasma-anodized) | −0.120 | 2.20 × 10−8 |
Elements | VT1-0 | VT5 |
Ti | 99.0% (Min) | Balance |
Al | - | 6% |
V | - | 4% |
O | < 0.12% | < 0.20% |
N | < 0.04% | < 0.05% |
C | < 0.05% | < 0.10% |
Fe | < 0.08% | < 0.30% |
H | < 0.0015% | < 0.015% |
Electrolyte | Description | Concentration (g/L) |
A | Sodium silicate (metasilicate) 9 aqueous | 100 |
NaOH | 8.0 | |
Aluminum oxide (1.1–1.5 µm) | 20.0 | |
B | Sodium phosphoric acid 3–substituted, 12 aqueous | 70.0 |
Aluminum hydroxide (0.6 µm) | 20.0 | |
C | Sodium phosphoric acid, two-substituted, 12 aqueous | 40.0 |
Sodium tetraborate 10 aqueous | 30.0 | |
Boric acid | 22.0 | |
Ammonium fluoride (NH4F) | 10.0 | |
Aluminum oxide | 20.0 |
Characteristics | Anodizing | PEO |
Formation mechanism | Controlled oxidation in acidic solution with electric current, forming a uniform oxide layer | Electric discharges in an electrolyte solution, resulting in high local temperature and the formation of thick oxide |
Compatibility with aluminum alloys | Primarily purer aluminum alloys | Can treat alloys difficult to anodize, such as Al-Si |
Process complexity | Simpler, well-established | More complex and requires precise control of discharge parameters |
Equipment cost | Moderate, more common, and accessible equipment | High due to the need for high-voltage sources and precise control |
Operational cost | Relatively low, less energy consumption | High due to energy consumption and specialized maintenance |
Environmental impact | Can use more environmentally friendly electrolytes, but most are acidic | Can be optimized with eco-friendly electrolytes |
Typical applications | Architecture, appliances, and decorative applications | Aerospace, automotive, and biomedical industries |