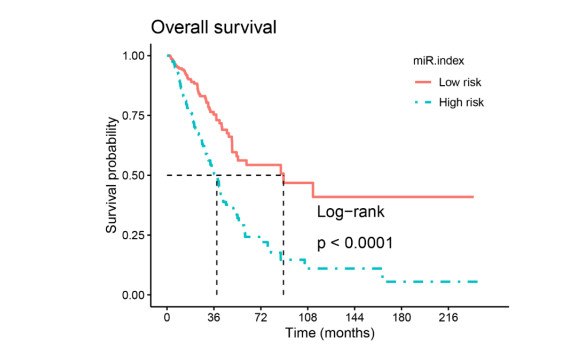
Citation: Guohong Xin, Xiaoci Cao, Wujie Zhao, Pintian Lv, Gang Qiu, Yaxing Li, Bin Wang, Baoshuan Fang, Yitao Jia. MicroRNA expression profile and TNM staging system predict survival in patients with lung adenocarcinoma[J]. Mathematical Biosciences and Engineering, 2020, 17(6): 8074-8083. doi: 10.3934/mbe.2020409
[1] | Gaozhong Sun, Tongwei Zhao . Lung adenocarcinoma pathology stages related gene identification. Mathematical Biosciences and Engineering, 2020, 17(1): 737-746. doi: 10.3934/mbe.2020038 |
[2] | Pingping Song, Jing Chen, Xu Zhang, Xiaofeng Yin . Construction of competitive endogenous RNA network related to circular RNA and prognostic nomogram model in lung adenocarcinoma. Mathematical Biosciences and Engineering, 2021, 18(6): 9806-9821. doi: 10.3934/mbe.2021481 |
[3] | Yong Ding, Jian-Hong Liu . The signature lncRNAs associated with the lung adenocarcinoma patients prognosis. Mathematical Biosciences and Engineering, 2020, 17(2): 1593-1603. doi: 10.3934/mbe.2020083 |
[4] | Siqi Hu, Fang Wang, Junjun Yang, Xingxiang Xu . Elevated ADAR expression is significantly linked to shorter overall survival and immune infiltration in patients with lung adenocarcinoma. Mathematical Biosciences and Engineering, 2023, 20(10): 18063-18082. doi: 10.3934/mbe.2023802 |
[5] | Kunpeng Li, Zepeng Wang, Yu Zhou, Sihai Li . Lung adenocarcinoma identification based on hybrid feature selections and attentional convolutional neural networks. Mathematical Biosciences and Engineering, 2024, 21(2): 2991-3015. doi: 10.3934/mbe.2024133 |
[6] | Dongchen Lu, Wei Han, Kai Lu . Identification of key microRNAs involved in tumorigenesis and prognostic microRNAs in breast cancer. Mathematical Biosciences and Engineering, 2020, 17(4): 2923-2935. doi: 10.3934/mbe.2020164 |
[7] | Yanping Xie, Zhaohui Dong, Junhua Du, Xiaoliang Zang, Huihui Guo, Min Liu, Shengwen Shao . The relationship between mouse lung adenocarcinoma at different stages and the expression level of exosomes in serum. Mathematical Biosciences and Engineering, 2020, 17(2): 1548-1557. doi: 10.3934/mbe.2020080 |
[8] | Shuyi Cen, Kaiyou Fu, Yue Shi, Hanliang Jiang, Jiawei Shou, Liangkun You, Weidong Han, Hongming Pan, Zhen Liu . A microRNA disease signature associated with lymph node metastasis of lung adenocarcinoma. Mathematical Biosciences and Engineering, 2020, 17(3): 2557-2568. doi: 10.3934/mbe.2020140 |
[9] | Bijiong Wang, Yaodong Tang, Biyun Yu, Di Gui, Hui Xu . Expression of autophagy-related factor p62 for lung cancer diagnosis and prognosis: A systematic review and meta-analysis. Mathematical Biosciences and Engineering, 2019, 16(6): 6805-6821. doi: 10.3934/mbe.2019340 |
[10] | Jian Huang, Zheng-Fu Xie . Identification of SSBP1 as a prognostic marker in human lung adenocarcinoma using bioinformatics approaches. Mathematical Biosciences and Engineering, 2022, 19(3): 3022-3035. doi: 10.3934/mbe.2022139 |
Lung cancer are the most common causes of cancer-related deaths worldwide, which accounts for over 25% of all cancer-related deaths [1,2]. Lung cancer is classified into four main histological categories: lung adenocarcinoma (LUAD), lung squamous cell carcinoma (LUSC), large cell lung carcinoma (LCLC) and small cell lung cancer (SCLC). Recent development in molecular targeted therapies have markedly improved the survival of individuals with LUAD.
Risk stratification and survival estimation are very important to guide personalized treatment decision in patients with cancer. The principal clinical determinant in the therapeutics option and prognosis prediction for most solid tumors is tumor status, characterized by TNM staging system. However, there are heterogeneous clinical outcomes in patients sharing similar clinical features, indicating that TNM staging system is insufficient in risk stratification and the management of tumors could be improved by combining bio molecular signatures with traditional TNM staging system.
miRNAs are short (approximately 18–24 nucleotides) noncoding RNAs and are common mechanisms of the posttranscriptional gene expression [3,4,5]. miRNAs play important roles in various process of tumor genesis and development [6,7]. They are highly conserved and their dysregulation are observed in all the types of tumors [6,7,8]. As miRNAs are very stable due to their resistance to RNase activity and to inferior circumstances [3], they can serve as cancer markers. Furthermore, the miRNA signature associated with survival outcome will be helpful to elucidate the mechanisms of miRNAs in lung cancer as well as develop novo therapeutics. In fact, a number of miRNAs have been characterized as promising molecule biomarkers for diagnosis, prediction of treatment efficacy, recurrence, metastasis and prognosis in patients with head and neck cancer [9,10], lung cancer [11,12,13,14,15,16,17], breast cancer [18,19,20,21], gastric cancer [22], pancreatic cancer [23], colorectal cancer [24,25], prostate cancer [26], cervical cancer [27,28] and bladder cancer [29].
In our present study, a prognosis predicting model was constructed by a combination of miRNA expression profile and TNM classification parameters, and the prognostic model was visualized with a nomogram.
The miRNA expression data (Data S1) and corresponding clinical information (Data S2) were downloaded from The Cancer Genome Atlas (TCGA) database (https://cancergenome.nih.gov/) at March 2019. There were 1881 miRNAs from 567 samples, including 521 LUAD tissues and 46 adjacent normal lung tissues, which were collected from patients with LUAD. Individuals with missing records in overall survival, endpoint event, sex, age at diagnosis, smoking history, TNM stages, cancer status, postoperative treatment, radiotherapy and treatment outcome, were excluded. Individuals whose survival time shorter than 2 months were also excluded, because they are likely to die from causes other than cancer. 460 individuals with LUAD were included in Cox regression analysis and randomly assigned into two distinct subetaoups, 306‐patient training set and 154‐patient validating set, at a ratio of 2:1, when visualizing the prognostic model with a nomogram.
The expression data for miRNAs was normalized by log 2 transformation. Differential expression analysis between two groups (cancer vs control) was performed through Bayesian test with package ‘limma’ [30] for R (v3.6.3) [31] on the criteria of |log 2 FC (fold change)| ≥ 1 and P value < 0.05 [32,33,34].
The differentially expressed miRNA data and clinical data were combined through function ‘merge’ in R. Then the possible prognostic value of differentially expressed miRNAs and clinicopathological parameters (194 differentially expressed miRNAs, T stage, N stage and Metastasis status) were assessed by univariate Cox regression analysis with R package ‘survival’ [35]. Subsequently, the candidate miRNAs and clinicopathological parameters with P value < 0.1 (41 miRNAs, T stage, N stage and Metastasis status) were further evaluated by multivariate Cox regression analysis with R package ‘survival’ [35].
miRNA index was calculated by a linear combination of miRNA expression levels weighted by regression coefficient (β) from multivariate Cox regression analysis [10,11,12,19,21,25,28]. The formula was as follows: miR.index = βgene1 × exprgene1 + βgene2 × exprgene2 + … + βgenen × exprgenen, where “expr” indicated the expression levels of miRNA. With the median miR.index as the cutoff point, patients were stratified into two groups: low risk group (<median miR.index) and high risk group (>miR.index). Kaplan-Meier survival analysis was performed for the low and high risk groups with R package ‘survival’ [35] and ‘survminer’ [36].
A nomogram was generated to visualize the effect of the miR.index and clinicopathological parameters on the survival of patients with LUAD in the training set, using R package ‘rms’ [37]. Subsequently, the prognostic model was tested by calculating the C-index and drawing the calibration curve in the training set and validating set, respectively.
The aforementioned methods used in this study were illustrated in a flow diagram (Figure S1).
The target genes of the identified prognostic miRNAs were predicted using miRTarBase (Release 7.0, http://miRTarBase.mbc.nctu.edu.tw/) bioinformatics analysis tools, which contains a great number of experimentally validated miRNA target genes. These target genes were then incorporated into Gene Ontology (GO) functional enrichment analysis and Kyoto Encyclopedia of Genes and Genomes (KEGG) pathway enrichment analysis via the online bioinformatics tool, the Database for Annotation, Visualization, and Integrated Discovery (Release 6.8, https://david.ncifcrf.gov/).
All statistical analyses were performed with R. Log-rank test was conducted to compare the difference for survival. Chi-square test was employed to test the clinicopathological parameters between the low risk and high risk groups. All statistical tests were two-tailed and P values < 0.05, except for P value < 0.1 in the univariate Cox regression analysis, were regarded as significant.
Totally, 460 patients with LUAD were enrolled in the present study. The detailed clinicopathological characteristics of enrolled patients were shown in Data S3.
A total of 194 differentially expressed (99 up-regulated and 95 down-regulated) miRNAs were identified between LUAD tissues and matched normal tissues, based on the cut-off criteria of (|log 2 FC| > 1.0 and P < 0.05). The differentially expressed miRNAs were presented in Data S4.
A set of 41 miRNAs, T stage, N stage and Metastasis status, which had potential relationship to patient survival, were screened out by univariate Cox proportional hazards regression analysis (Data S5). These candidate miRNAs and TNM classification parameters were further verified by multivariate Cox proportional hazards regression analysis. Nine miRNAs (hsa-let-7i, hsa-mir-1976, hsa-mir-199a-1, hsa-mir-31, hsa-mir-3940, hsa-mir-450a-2, hsa-mir-4677, hsa-mir-548v and hsa‐mir‐6803) and N stage were identified as independent prognostic indicators for patients with LUAD (Data S6). Patients in low risk group had a superior overall survival compared to those in high risk group (89.4 months vs 38.2 months, P = 1e-08) (Figure 1). The demographic and clinicopathological characteristics between patients of the two groups were presented in Table 1. Among these nine miRNAs, hsa-mir-1976, hsa-mir-199a-1, hsa-mir-4677, hsa-mir-548v and hsa‐mir‐6803 were positively correlated with the overall survival, hsa-let-7i, hsa-mir-31, hsa-mir-3940 and hsa-mir-450a-2 were negatively correlated with the overall survival.
Overall | Low Risk | High Risk | p | ||
460 | 230 | 230 | |||
Sex (%) | Female | 243 (52.8) | 127 (55.2) | 116 (50.4) | 0.35 |
Male | 217 (47.2) | 103 (44.8) | 114 (49.6) | ||
age (%) | ≤ 66(Median) | 229 (49.8) | 116 (50.4) | 113 (49.1) | 0.852 |
>66(Median) | 158 (47.6) | 84 (49.4) | 74 (45.7) | ||
smoking_status (%) | Never Smokers | 62 (13.9) | 40 (17.8) | 22 (10.0) | 0.055 |
Former Smokers | 273 (61.2) | 130 (57.8) | 143 (64.7) | ||
Current Smokers | 111 (24.9) | 55 (24.4) | 56 (25.3) | ||
T (%) | T1 | 158 (34.3) | 83 (36.1) | 75 (32.6) | 0.52 |
T2 | 243 (52.8) | 122 (53.0) | 121 (52.6) | ||
T3 | 42 (9.1) | 19 (8.3) | 23 (10.0) | ||
T4 | 17 (3.7) | 6 (2.6) | 11 (4.8) | ||
N (%) | N0 | 303 (65.9) | 158 (68.7) | 145 (63.0) | 0.231 |
N1 | 90 (19.6) | 39 (17.0) | 51 (22.2) | ||
N2 | 65 (14.1) | 31 (13.5) | 34 (14.8) | ||
N3 | 2 (0.4) | 2 (0.9) | 0 (0.0) | ||
M (%) | M0 | 442 (96.1) | 225 (97.8) | 217 (94.3) | 0.092 |
M1 | 18 (3.9) | 5 (2.2) | 13 (5.7) | ||
Stage (%) | Stage Ⅰ | 248 (54.5) | 132 (58.1) | 116 (50.9) | 0.168 |
Stage Ⅱ | 112 (24.6) | 54 (23.8) | 58 (25.4) | ||
Stage Ⅲ | 77 (16.9) | 36 (15.9) | 41 (18.0) | ||
Stage Ⅳ | 18 (4.0) | 5 (2.2) | 13 (5.7) | ||
stage_event.system_version (%) | 3rd | 3 (0.7) | 0 (0.0) | 3 (1.3) | 0.225 |
4th | 5 (1.1) | 3 (1.3) | 2 (0.9) | ||
5th | 29 (6.3) | 13 (5.7) | 16 (7.0) | ||
6th | 161 (35.0) | 74 (32.2) | 87 (37.8) | ||
7th | 247 (53.7) | 134 (58.3) | 113 (49.1) | ||
Not Available | 15 (3.3) | 6 (2.6) | 9 (3.9) | ||
Cancer_Status (%) | Tumor Free | 304 (74.5) | 172 (81.9) | 132 (66.7) | 0.001 |
With Tumor | 104 (25.5) | 38 (18.1) | 66 (33.3) | ||
outcome (%) | CR | 273 (72.8) | 162 (81.8) | 111 (62.7) | < 0.001 |
PR | 4 (1.1) | 0 (0.0) | 4 (2.3) | ||
SD | 29 (7.7) | 15 (7.6) | 14 (7.9) | ||
PD | 69 (18.4) | 21 (10.6) | 48 (27.1) | ||
POT (%) | No | 140 (34.5) | 72 (34.3) | 68 (34.7) | 1 |
Yes | 266 (65.5) | 138 (65.7) | 128 (65.3) | ||
RT (%) | No | 56 (13.8) | 23 (11.0) | 33 (16.8) | 0.12 |
Yes | 351 (86.2) | 187 (89.0) | 164 (83.2) | ||
T, tumor; N, node; M, metastasis; CR, complete response; PR, partial response; SD, stable disease; PD, progressive disease; POT, postoperative treatment; RT, radiation therapy. |
A total of 1757 target genes of the nine miRNAs were collected from the miRTarBase database (Data S7). The GO biological processes were mainly enriched in regulation of transcription, apoptotic process and response to heat or toxic substance (Data S8). The GO molecular function were linked to protein binding, transcription factor binding, nucleic acid binding and protein tyrosine kinase activity (Data S9). In addition, the KEGG pathways were significantly enriched in mRNA surveillance pathway, transcriptional misregulation in cancer, pathways in cancer, viral carcinogenesis and adherens junction (Data S10).
A nomogram was generated to illustrate the relationship between prognostic indicators and the predicted survival outcomes of patients with LUAD. As shown in Figure 2, miR.index, T stage, N stage and Metastasis status were associated with survival. The clinicopathological parameters between the training set and validating set were well balanced (Table S1). The C-indexes were 0.68 and 0.72 in the training and validating set, respectively. The both C-indexes were close to or greater than 0.7, which confirmed the reliability of the model. Predictive accuracy of the model was further tested by calibration curves comparing actual 3 or 5-year overall survival (proportion) with predicted 3 or 5-year overall survival probability, both in the training and validating set (Figures S2–S5).
LUAD is one of the most fatal malignancies worldwide [1,2]. The prognosis of patients with LUAD would be improved to some extent if tumor behavior could be predicted before initial treatment. Accurate prediction is definitely based on the clinicopathological characteristics, the identification of novel biomarkers and the elucidation of the precise molecular mechanisms underlying LUAD occurrence and development. The TNM staging system, based on the clinicopathological characteristics of cancer, is insufficient in risk stratification and prognosis prediction, and the prediction power could be enhanced when combined with molecular biomarkers.
miRNAs are small non-coding RNAs that regulate gene expression [3,4,5]. They are involved in carcinogenesis and are presented as potential diagnostic or prognostic biomarkers in cancers [6,7]. Several miRNA markers have been identified for prediction of treatment outcome, recurrence, metastasis and prognosis in patients with cancers [9,10,11,14,15,16,17,18,19,20,21,22,23,24,25,26,27,28,29], including LUAD [14,15,16]. Li et al determined that miR-101-1, miR-220a, miR-450a-2 and miR-4661 were related to survival outcome in patients with LUAD [14]. Lin et al proposed a four miRNAs signature (miR-148a-5p, miR-31-5p, miR-548v and miR-550a-5p) associated with prognosis in patients with LUAD [15]. Interestingly, the miRNA signatures obtained by various studies do not overlap, or just overlap partly, perhaps due to the variability in the methods used to identify differentially expressed miRNAs and screen out risky miRNAs. In most of the aforementioned studies, risk score or prognosis index were constructed with linear combination of miRNA expression levels multiplied by Cox regression coefficient (β). Bias is likely to occur when explain the contribution of risk score or prognosis index to survival, because there was significant difference among the expression levels of each individual miRNA. To avoid this possible bias, we normalized the expression levels of miRNAs by their quantile distribution. Lin et al removed miRNAs with expression levels of zero in ≥ 50% of the patients, because they thought the feasibility of implementation could be enhanced [15]. But to our opinion, some miRNAs downregulated in cancer could be missed when this part of the data was excluded in analysis.
In our research, we identified nine miRNAs (hsa-let-7i, hsa-mir-1976, hsa-mir-199a-1, hsa-mir-31, hsa-mir-3940, hsa-mir-450a-2, hsa-mir-4677, hsa-mir-548v and hsa‐mir‐6803) associated with overall survival in patients with LUAD. Among them, miR-31, miR-450a-2 and miR-548v have been reported previously to be related to clinical outcome in patients with LUAD [14,15].
To gain a deep understanding of the nine miRNAs, we obtained the target genes of these nine miRNAs, and predicted the biological functions and pathways associated with their targets using bioinformatics analysis. The target genes of the nine miRNAs were mainly enriched in key cancer-related biological processes and pathways, such as cell proliferation, cell differentiation, transcription regulation, cell transformation, cell cycle, response to drug and apoptosis. The results suggested that these miRNAs played an important role in the occurrence, development and prognosis of LUAD.
Despite the potential ability of our model to predict prognosis in patients with LUAD, it had some limitations. The utility of different TNM staging system (3rd to 7th edition) and the incomplete records about treatment information (surgery, chemotherapy, radiotherapy and molecular targeted therapy) might cause biases in our analysis. Therefore, the prognostic value of the nine-miRNA signature is warranted for further validations.
The prognostic model constructed with nine miRNA expression profile and TNM classification parameters can predict the survival in patients with LUAD, and the predictive power of the model are warranted for further validations.
The authors want to thank professor Zhongxin Li for his kind help in revising the manuscript.
The authors declare that there are no conflicts of interest.
[1] |
F. Bray, J. Ferlay, I. Soerjomataram, R. L. Siegel, L. A. Torre, A. Jemal, Global cancer statistics 2018: GLOBOCAN estimates of incidence and mortality worldwide for 36 cancers in 185 countries, Ca-Cancer J. Clin., 68 (2018), 394-424. doi: 10.3322/caac.21492
![]() |
[2] |
C. Allemani, T. Matsuda, V. Di Carlo, R. Harewood, M. Matz, M. Nikšić, et al., Global surveillance of trends in cancer survival 2000-14 (CONCORD-3): Analysis of individual records for 37 513 025 patients diagnosed with one of 18 cancers from 322 population-based registries in 71 countries, Lancet, 391 (2018), 1023-1075. doi: 10.1016/S0140-6736(17)33326-3
![]() |
[3] | E. Zlotorynski, Insights into the kinetics of microRNA biogenesis and turnover, Nat. Rev. Mol. Cell. Biol., 20 (2019), 511. |
[4] | N. Treiber, T. Treiber, G. Meister, Regulation of microRNA biogenesis and its crosstalk with other cellular pathways, Nat. Rev. Mol. Cell Biol., 20 (2019), 5-20. |
[5] |
D. P. Bartel, MicroRNAs: Genomics, biogenesis, mechanism, and function, Cell, 116 (2004), 281-297. doi: 10.1016/S0092-8674(04)00045-5
![]() |
[6] |
J. Winter, S. Jung, S. Keller, R. I. Gregory, S. Diederichs, Many roads to maturity: MicroRNA biogenesis pathways and their regulation, Nat. Cell Biol., 11 (2009), 228-234. doi: 10.1038/ncb0309-228
![]() |
[7] |
R. Rupaimoole, F. J. Slack, MicroRNA therapeutics: Towards a new era for the management of cancer and other diseases, Nat. Rev. Drug Discov., 16 (2017), 203-222. doi: 10.1038/nrd.2016.246
![]() |
[8] |
G. A. Calin, C. M. Croce, MicroRNA signatures in human cancers, Nat. Rev. Cancer, 6 (2006), 857-866. doi: 10.1038/nrc1997
![]() |
[9] |
D. A. Clump, C. R. Pickering, H. D. Skinner, Predicting outcome in head and neck cancer: MiRNAs with potentially big effects, Clin. Cancer Res., 25 (2019), 1441-1442. doi: 10.1158/1078-0432.CCR-18-3078
![]() |
[10] |
J. Hess, K. Unger, C. Maihoefer, L. Schuttrumpf, L. Wintergerst, T. Heider, et al., A Five-MicroRNA signature predicts survival and disease control of patients with head and neck cancer negative for HPV infection, Clin. Cancer Res., 25 (2019), 1505-1516. doi: 10.1158/1078-0432.CCR-18-0776
![]() |
[11] |
P. Ulivi, E. Petracci, G. Marisi, S. Baglivo, R. Chiari, M. Billi, et al., Prognostic role of circulating miRNAs in early-stage non-small cell lung cancer, J. Clin. Med., 8 (2019), 131. doi: 10.3390/jcm8020131
![]() |
[12] |
Y. Zhang, J. A. Roth, H. Yu, Y. Ye, K. Xie, H. Zhao, et al., A 5-microRNA signature identified from serum microRNA profiling predicts survival in patients with advanced stage non-small cell lung cancer, Carcinog., 40 (2019), 643-650. doi: 10.1093/carcin/bgy132
![]() |
[13] | H. Yan, S. Xin, J. Ma, H. Wang, H. Zhang, J. Liu, A three microRNA-based prognostic signature for small cell lung cancer overall survival, J. Cell. Biochem., 120 (2018), 8723-8730. |
[14] |
X. Li, Z. An, P. Li, H. Liu, A prognostic model for lung adenocarcinoma patient survival with a focus on four miRNAs, Oncol. Lett., 14 (2017), 2991-2995. doi: 10.3892/ol.2017.6481
![]() |
[15] | Y. Lin, Y. Lv, R. Liang, C. Yuan, J. Zhang, D. He, et al., Four-miRNA signature as a prognostic tool for lung adenocarcinoma, Onco. Targets Ther., 11 (2018), 29-36. |
[16] |
S. S. Yerukala, S. Y. Ho, Identifying the miRNA signature associated with survival time in patients with lung adenocarcinoma using miRNA expression profiles, Sci. Rep., 7 (2017), 7507. doi: 10.1038/s41598-017-07739-y
![]() |
[17] |
M. Raponi, L. Dossey, T. Jatkoe, X. Wu, G. Chen, H. Fan, et al., MicroRNA classifiers for predicting prognosis of squamous cell lung cancer, Cancer Res., 69 (2009), 5776-5783. doi: 10.1158/0008-5472.CAN-09-0587
![]() |
[18] | R. Bajaj, D. Doval, R. Tripathi, T. Sridhar, A. Korlimarla, K. D. Choudhury, et al., Prognostic role of microRNA 182 and microRNA 18a in locally advanced triple negative breast cancer, Ann. Oncol., 30 (2019), 19. |
[19] |
H. Li, J. Liu, J. Chen, H. Wang, L. Yang, F. Chen, et al., A serum microRNA signature predicts trastuzumab benefit in HER2-positive metastatic breast cancer patients, Nat. Commun., 9 (2018), 1614. doi: 10.1038/s41467-018-03537-w
![]() |
[20] |
S. Di Cosimo, V. Appierto, S. Pizzamiglio, P. Tiberio, M. V. Iorio, F. Hilbers, et al., Plasma miRNA levels for predicting therapeutic response to neoadjuvant treatment in HER2-positive breast cancer: Results from the NeoALTTO trial, Clin. Cancer Res., 25 (2019), 3887-3895. doi: 10.1158/1078-0432.CCR-18-2507
![]() |
[21] |
S. Shiino, J. Matsuzaki, A. Shimomura, J. Kawauchi, S. Takizawa, H. Sakamoto, et al., Serum miRNA-based prediction of axillary lymph node metastasis in breast cancer, Clin. Cancer Res., 25 (2019), 1817-1827. doi: 10.1158/1078-0432.CCR-18-1414
![]() |
[22] |
L. Yu, D. Wu, H. Gao, J. J. Balic, A. Tsykin, T. S. Han, et al., Clinical utility of a STAT3-Regulated miRNA-200 family signature with prognostic potential in early gastric cancer, Clin. Cancer Res., 24 (2018), 1459-1472. doi: 10.1158/1078-0432.CCR-17-2485
![]() |
[23] |
P. Wang, W. Li, B. Zhai, X. Jiang, H. Jiang, C. Zhang, et al., Integrating high-throughput microRNA and mRNA expression data to identify risk mRNA signature for pancreatic cancer prognosis, J. Cell. Biochem., 121 (2020), 3090-3098. doi: 10.1002/jcb.29576
![]() |
[24] |
X. Zhang, H. Zhang, B. Shen, X. F. Sun, Novel MicroRNA biomarkers for colorectal cancer early diagnosis and 5-Fluorouracil chemotherapy resistance but not prognosis: A study from databases to AI-Assisted verifications, Cancers (Basel), 12 (2020), 341. doi: 10.3390/cancers12020341
![]() |
[25] |
R. Kandimalla, F. Gao, T. Matsuyama, T. Ishikawa, H. Uetake, N. Takahashi, et al., Genome-wide discovery and identification of a novel miRNA signature for recurrence prediction in stage II and III colorectal cancer, Clin. Cancer Res., 24 (2018), 3867-3877. doi: 10.1158/1078-0432.CCR-17-3236
![]() |
[26] |
L. Schmidt, J. Fredsoe, H. Kristensen, S. H. Strand, A. Rasmussen, S. Hoyer, et al., Training and validation of a novel 4-miRNA ratio model (MiCaP) for prediction of postoperative outcome in prostate cancer patients, Ann. Oncol., 29 (2018), 2003-2009. doi: 10.1093/annonc/mdy243
![]() |
[27] |
J. S. Nahand, S. Taghizadeh-Boroujeni, M. Karimzadeh, S. Borran, M. H. Pourhanifeh, M. Moghoofei, et al., MicroRNAs: New prognostic, diagnostic, and therapeutic biomarkers in cervical cancer, J. Cell. Physiol., 234 (2019), 17064-17099. doi: 10.1002/jcp.28457
![]() |
[28] |
B. Liang, Y. Li, T. Wang, A three miRNAs signature predicts survival in cervical cancer using bioinformatics analysis, Sci. Rep., 7 (2017), 5624. doi: 10.1038/s41598-017-18338-2
![]() |
[29] |
X. H. Yin, Y. H. Jin, Y. Cao, Y. Wong, H. Weng, C. Sun, et al., Development of a 21-miRNA signature associated with the prognosis of patients with bladder cancer, Front. Oncol., 9 (2019), 729. doi: 10.3389/fonc.2019.00729
![]() |
[30] |
M. E. Ritchie, B. Phipson, Di Wu, Y. Hu, C. W. Law, W. Shi, et al., {Limma} powers differential expression analyses for {RNA}-sequencing and microarray studies, Nucleic. Acids. Res., 43 (2015), 47. doi: 10.1093/nar/gkv007
![]() |
[31] | R Core Team, R: A Language and Environment for Statistical Computing, Vienna, Austria, 2020. |
[32] |
G. Yang, Y. Zhang, J. Yang, A Five-microRNA signature as prognostic biomarker in colorectal cancer by bioinformatics analysis, Front. Oncol., 9 (2019), 1207. doi: 10.3389/fonc.2019.01207
![]() |
[33] |
Y. Luo, C. Zhang, F. Tang, J. Zhao, C. Shen, C. Wang, et al., Bioinformatics identification of potentially involved microRNAs in Tibetan with gastric cancer based on microRNA profiling, Cancer Cell Int., 15 (2015), 115. doi: 10.1186/s12935-015-0266-1
![]() |
[34] | Y. Lv, J. Duanmu, X. Fu, T. Li, Q. Jiang, Identifying a new microRNA signature as a prognostic biomarker in colon cancer, PLoS. One, 15 (2020), 228575. |
[35] | Therneau, M. Terry, A Package for Survival Analysis in R, 2020. |
[36] | A. Kassambara, M. Kosinski, P. Biecek, Survminer: Drawing Survival Curves using 'ggplot2', 2019. |
[37] | F. E. H. Jr, Rms: Regression Modeling Strategies, 2019. |
![]() |
![]() |
1. | Zhiqiang Wang, Fan Hu, Ruijie Chang, Xiaoyue Yu, Chen Xu, Yujie Liu, Rongxi Wang, Hui Chen, Shangbin Liu, Danni Xia, Yingjie Chen, Xin Ge, Tian Zhou, Shuixiu Zhang, Haoyue Pang, Xueni Fang, Yushuang Zhang, Jin Li, Kaiwen Hu, Yong Cai, Development and Validation of a Prognostic Model to Predict Overall Survival for Lung Adenocarcinoma: A Population-Based Study From the SEER Database and the Chinese Multicenter Lung Cancer Database, 2022, 21, 1533-0346, 153303382211332, 10.1177/15330338221133222 | |
2. | Zhichao Lin, Wenhai Huang, Zehua Xie, Yongsheng Yi, Zumei Li, Expression, Clinical Significance, Immune Infiltration, and Regulation Network of miR-3940-5p in Lung Adenocarcinoma Based on Bioinformatic Analysis and Experimental Validation, 2022, Volume 15, 1178-7074, 6451, 10.2147/IJGM.S375761 | |
3. | Zhenghua Liu, Shize Yang, Siyu Zhou, Shiyao Dong, Jiang Du, Prognostic Value of lncRNA DRAIC and miR-3940-3p in Lung Adenocarcinoma and Their Effect on Lung Adenocarcinoma Cell Progression, 2021, Volume 13, 1179-1322, 8367, 10.2147/CMAR.S320616 | |
4. | Beata Smolarz, Adam Durczyński, Hanna Romanowicz, Krzysztof Szyłło, Piotr Hogendorf, miRNAs in Cancer (Review of Literature), 2022, 23, 1422-0067, 2805, 10.3390/ijms23052805 |
Overall | Low Risk | High Risk | p | ||
460 | 230 | 230 | |||
Sex (%) | Female | 243 (52.8) | 127 (55.2) | 116 (50.4) | 0.35 |
Male | 217 (47.2) | 103 (44.8) | 114 (49.6) | ||
age (%) | ≤ 66(Median) | 229 (49.8) | 116 (50.4) | 113 (49.1) | 0.852 |
>66(Median) | 158 (47.6) | 84 (49.4) | 74 (45.7) | ||
smoking_status (%) | Never Smokers | 62 (13.9) | 40 (17.8) | 22 (10.0) | 0.055 |
Former Smokers | 273 (61.2) | 130 (57.8) | 143 (64.7) | ||
Current Smokers | 111 (24.9) | 55 (24.4) | 56 (25.3) | ||
T (%) | T1 | 158 (34.3) | 83 (36.1) | 75 (32.6) | 0.52 |
T2 | 243 (52.8) | 122 (53.0) | 121 (52.6) | ||
T3 | 42 (9.1) | 19 (8.3) | 23 (10.0) | ||
T4 | 17 (3.7) | 6 (2.6) | 11 (4.8) | ||
N (%) | N0 | 303 (65.9) | 158 (68.7) | 145 (63.0) | 0.231 |
N1 | 90 (19.6) | 39 (17.0) | 51 (22.2) | ||
N2 | 65 (14.1) | 31 (13.5) | 34 (14.8) | ||
N3 | 2 (0.4) | 2 (0.9) | 0 (0.0) | ||
M (%) | M0 | 442 (96.1) | 225 (97.8) | 217 (94.3) | 0.092 |
M1 | 18 (3.9) | 5 (2.2) | 13 (5.7) | ||
Stage (%) | Stage Ⅰ | 248 (54.5) | 132 (58.1) | 116 (50.9) | 0.168 |
Stage Ⅱ | 112 (24.6) | 54 (23.8) | 58 (25.4) | ||
Stage Ⅲ | 77 (16.9) | 36 (15.9) | 41 (18.0) | ||
Stage Ⅳ | 18 (4.0) | 5 (2.2) | 13 (5.7) | ||
stage_event.system_version (%) | 3rd | 3 (0.7) | 0 (0.0) | 3 (1.3) | 0.225 |
4th | 5 (1.1) | 3 (1.3) | 2 (0.9) | ||
5th | 29 (6.3) | 13 (5.7) | 16 (7.0) | ||
6th | 161 (35.0) | 74 (32.2) | 87 (37.8) | ||
7th | 247 (53.7) | 134 (58.3) | 113 (49.1) | ||
Not Available | 15 (3.3) | 6 (2.6) | 9 (3.9) | ||
Cancer_Status (%) | Tumor Free | 304 (74.5) | 172 (81.9) | 132 (66.7) | 0.001 |
With Tumor | 104 (25.5) | 38 (18.1) | 66 (33.3) | ||
outcome (%) | CR | 273 (72.8) | 162 (81.8) | 111 (62.7) | < 0.001 |
PR | 4 (1.1) | 0 (0.0) | 4 (2.3) | ||
SD | 29 (7.7) | 15 (7.6) | 14 (7.9) | ||
PD | 69 (18.4) | 21 (10.6) | 48 (27.1) | ||
POT (%) | No | 140 (34.5) | 72 (34.3) | 68 (34.7) | 1 |
Yes | 266 (65.5) | 138 (65.7) | 128 (65.3) | ||
RT (%) | No | 56 (13.8) | 23 (11.0) | 33 (16.8) | 0.12 |
Yes | 351 (86.2) | 187 (89.0) | 164 (83.2) | ||
T, tumor; N, node; M, metastasis; CR, complete response; PR, partial response; SD, stable disease; PD, progressive disease; POT, postoperative treatment; RT, radiation therapy. |
Overall | Low Risk | High Risk | p | ||
460 | 230 | 230 | |||
Sex (%) | Female | 243 (52.8) | 127 (55.2) | 116 (50.4) | 0.35 |
Male | 217 (47.2) | 103 (44.8) | 114 (49.6) | ||
age (%) | ≤ 66(Median) | 229 (49.8) | 116 (50.4) | 113 (49.1) | 0.852 |
>66(Median) | 158 (47.6) | 84 (49.4) | 74 (45.7) | ||
smoking_status (%) | Never Smokers | 62 (13.9) | 40 (17.8) | 22 (10.0) | 0.055 |
Former Smokers | 273 (61.2) | 130 (57.8) | 143 (64.7) | ||
Current Smokers | 111 (24.9) | 55 (24.4) | 56 (25.3) | ||
T (%) | T1 | 158 (34.3) | 83 (36.1) | 75 (32.6) | 0.52 |
T2 | 243 (52.8) | 122 (53.0) | 121 (52.6) | ||
T3 | 42 (9.1) | 19 (8.3) | 23 (10.0) | ||
T4 | 17 (3.7) | 6 (2.6) | 11 (4.8) | ||
N (%) | N0 | 303 (65.9) | 158 (68.7) | 145 (63.0) | 0.231 |
N1 | 90 (19.6) | 39 (17.0) | 51 (22.2) | ||
N2 | 65 (14.1) | 31 (13.5) | 34 (14.8) | ||
N3 | 2 (0.4) | 2 (0.9) | 0 (0.0) | ||
M (%) | M0 | 442 (96.1) | 225 (97.8) | 217 (94.3) | 0.092 |
M1 | 18 (3.9) | 5 (2.2) | 13 (5.7) | ||
Stage (%) | Stage Ⅰ | 248 (54.5) | 132 (58.1) | 116 (50.9) | 0.168 |
Stage Ⅱ | 112 (24.6) | 54 (23.8) | 58 (25.4) | ||
Stage Ⅲ | 77 (16.9) | 36 (15.9) | 41 (18.0) | ||
Stage Ⅳ | 18 (4.0) | 5 (2.2) | 13 (5.7) | ||
stage_event.system_version (%) | 3rd | 3 (0.7) | 0 (0.0) | 3 (1.3) | 0.225 |
4th | 5 (1.1) | 3 (1.3) | 2 (0.9) | ||
5th | 29 (6.3) | 13 (5.7) | 16 (7.0) | ||
6th | 161 (35.0) | 74 (32.2) | 87 (37.8) | ||
7th | 247 (53.7) | 134 (58.3) | 113 (49.1) | ||
Not Available | 15 (3.3) | 6 (2.6) | 9 (3.9) | ||
Cancer_Status (%) | Tumor Free | 304 (74.5) | 172 (81.9) | 132 (66.7) | 0.001 |
With Tumor | 104 (25.5) | 38 (18.1) | 66 (33.3) | ||
outcome (%) | CR | 273 (72.8) | 162 (81.8) | 111 (62.7) | < 0.001 |
PR | 4 (1.1) | 0 (0.0) | 4 (2.3) | ||
SD | 29 (7.7) | 15 (7.6) | 14 (7.9) | ||
PD | 69 (18.4) | 21 (10.6) | 48 (27.1) | ||
POT (%) | No | 140 (34.5) | 72 (34.3) | 68 (34.7) | 1 |
Yes | 266 (65.5) | 138 (65.7) | 128 (65.3) | ||
RT (%) | No | 56 (13.8) | 23 (11.0) | 33 (16.8) | 0.12 |
Yes | 351 (86.2) | 187 (89.0) | 164 (83.2) | ||
T, tumor; N, node; M, metastasis; CR, complete response; PR, partial response; SD, stable disease; PD, progressive disease; POT, postoperative treatment; RT, radiation therapy. |