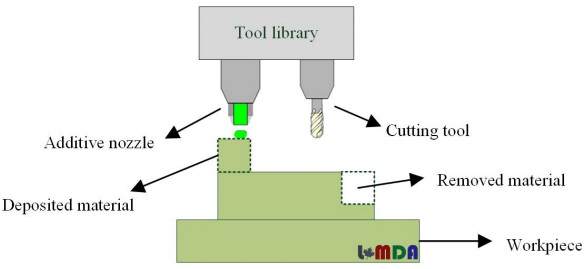
Citation: Yufan Zheng, Rafiq Ahmad. Feature extraction and process planning of integrated hybrid additive-subtractive system for remanufacturing[J]. Mathematical Biosciences and Engineering, 2020, 17(6): 7274-7301. doi: 10.3934/mbe.2020373
[1] | Lingling Li, Congbo Li, Li Li, Ying Tang, Qingshan Yang . An integrated approach for remanufacturing job shop scheduling with routing alternatives. Mathematical Biosciences and Engineering, 2019, 16(4): 2063-2085. doi: 10.3934/mbe.2019101 |
[2] | H. Y. Swathi, G. Shivakumar . Audio-visual multi-modality driven hybrid feature learning model for crowd analysis and classification. Mathematical Biosciences and Engineering, 2023, 20(7): 12529-12561. doi: 10.3934/mbe.2023558 |
[3] | Jian-xue Tian, Jue Zhang . Breast cancer diagnosis using feature extraction and boosted C5.0 decision tree algorithm with penalty factor. Mathematical Biosciences and Engineering, 2022, 19(3): 2193-2205. doi: 10.3934/mbe.2022102 |
[4] | Boyi Zeng, Jun Zhao, Shantian Wen . A textual and visual features-jointly driven hybrid intelligent system for digital physical education teaching quality evaluation. Mathematical Biosciences and Engineering, 2023, 20(8): 13581-13601. doi: 10.3934/mbe.2023606 |
[5] | Wenjuan Gu, Hongcheng Wang, Xiaobao Liu, Yanchao Yin, Biao Xu . Urban scene segmentation model based on multi-scale shuffle features. Mathematical Biosciences and Engineering, 2023, 20(7): 11763-11784. doi: 10.3934/mbe.2023523 |
[6] | Zhigao Zeng, Cheng Huang, Wenqiu Zhu, Zhiqiang Wen, Xinpan Yuan . Flower image classification based on an improved lightweight neural network with multi-scale feature fusion and attention mechanism. Mathematical Biosciences and Engineering, 2023, 20(8): 13900-13920. doi: 10.3934/mbe.2023619 |
[7] | Rongyan Shang, Changqing Peng, Pengfei Shao, Ruiming Fang . FFT-based equal-integral-bandwidth feature extraction of vibration signal of OLTC. Mathematical Biosciences and Engineering, 2021, 18(3): 1966-1980. doi: 10.3934/mbe.2021102 |
[8] | Li Wang, Changyuan Wang, Yu Zhang, Lina Gao . An integrated neural network model for eye-tracking during human-computer interaction. Mathematical Biosciences and Engineering, 2023, 20(8): 13974-13988. doi: 10.3934/mbe.2023622 |
[9] | Nan Mu, Jinjia Guo, Rong Wang . Automated polyp segmentation based on a multi-distance feature dissimilarity-guided fully convolutional network. Mathematical Biosciences and Engineering, 2023, 20(11): 20116-20134. doi: 10.3934/mbe.2023891 |
[10] | MingHao Zhong, Fenghuan Li, Weihong Chen . Automatic arrhythmia detection with multi-lead ECG signals based on heterogeneous graph attention networks. Mathematical Biosciences and Engineering, 2022, 19(12): 12448-12471. doi: 10.3934/mbe.2022581 |
Nowadays, the increasing developments and over-exploitation of resources outcome vast amount "end-of-life" products. Remanufacturing and repairing are identified as environmentally friendly approaches to deal with them since the energy consumption to produce a new part can be minimized by maintaining the intrinsic energy of the legacy part [1,2,3]. Repairing process is the correction of specified faults or restoring its original form of product. Whereas, remanufacturing, also called "reincarnation", is defined as being able to produce new products directly from the end-of-life product [4]. In different publications, the words "remanufacturing" and "repairing" have been used interchangeably with blurred definitions [4,5,6]. In this study, the authors differentiate them by defining "repairing" as restoring the part to its original form and "remanufacturing" as upgrades the part to have different functional features.
Recently, hybrid manufacturing (HM) system synergistically integrates additive manufacturing (AM) and subtractive manufacturing (SM) processes within a single workstation (Figure 1), has gained a lot of attention from academia and industry [7]. HM can capitalize on the strengths of independent techniques, whilst minimizing their disadvantages [8]. At the same time, it has the potentials to enable remanufacturing technologies to achieve further improvement because it can to remove and add features flexibly.
Most of the single platform workstations for HM processes are integrating subtractive CNC machining and additive directed energy deposition (DED) [9]. There are some commercial process planning software tools that have already been developed for industrial hybrid systems, such as Siemens NX used in DMG MoriSeiki, LaserTec 65 3D, and hyperMill used in Replicator and Cybaman [9]. However, the major limitation of these commercial tools is the poor support for automation. For example, AM and SM feature recognition is manually conducted by the user's knowledge. The consequence is that the process planning of complex remanufacturing problems is very tedious, and the quality of the derived process plan can hardly be evaluated. For this reason, an increasing level of interest in research on process planning for HM has been witnessed over recent years [9,10]. Joshi & Anand [11] developed a novel metric, called complexity score to quantify part's geometric complexities for decision-making among AM, SM, and HM. Chen et al. [12] discovered that complex geometry might have the tool accessibility issue for the SM process, in which the cutter cannot access the part's interior. They then developed an optimization algorithm for HM process planning, which derived the optimal HM process plan of an arbitrary geometry that was free of machinability issues. They also extended the concept to address the problem for quasi-rotational parts with columnar features in their other works [7]. A sequence planning method for five-axis additive-subtractive hybrid manufacturing is also presented by [13], and it greatly reduced the number of tool changes between different operations. Zhu et al. [14,15] and Newman et al. [16] presented a hybrid process planning framework, called iAtractive, which integrates CNC machining, fused filament fabrication, and inspection process for plastic components with internal structures. Behandish et al. [17] introduced an HM process planning method that formulated HM processes through logic representations, which has the capability to enumerate all the feasible sequences of operations to find the optimal plan. ElMaraghy & Moussa [18] developed a method of process planning for HM that included manual feature extraction, product platform design, and process determination. Basinger et al. [19] outlines a feature-based advanced HM process planning system which use feature, tolerance and material data as inputs to construct process plans. From these research results, the authors identify that SM is mostly playing a role as post-machining for AM in the HM process. Therefore, it is complicated to be applied directly in the remanufacturing, since SM is not only post-machining but also including geometric forming as AM process in remanufacturing.
Several studies have explored the potentials of AM for repairing or remanufacturing applications. Different aspects of AM techniques have been widely investigated, such as laser parameters [20], damaged volume reconstruction [21,22,23,24], energy and environmental impact analysis [6,25], and microstructure [26,27,28] and mechanical property analysis [29,30]. Recently, HM for repairing application is also actively investigated. Zheng et al. [22] introduced a 3D reconstruction and triangle intersection algorithm to identify additive repair and subtractive repair areas of a broken turbine surface. Hascoët et al. [31] proposed a method to automate the repairing process of metallic parts partially. In their practice, defects were initially machined into a surface cavity, and the cavity was refilled by laser metal deposition with the aid of an inspection system. An increasing level of development in research on HM-based repairing technology has been witnessed by those publications. However, in terms of remanufacturing, there are comparatively fewer studies. In comparison with repairing, remanufacturing requires more decision-making support [32] since the process planning result is not unique, and AM and SM feature extraction relies on algorithms for the automation process.
Newman et al. [16] and Zhu et al. [33] features a remanufacturing framework that consisted of fused filament fabrication, CNC machining, and inspection. The method enabled the remanufacturing of an existing part or even a recycled and legacy part into a new part with new functional features. Le et al. [34] proposed an HM process planning for remanufacturing based on feature extraction and knowledge interpretation. In a subsequent study [35], they have extended the process planning framework by discussing the environmental impact of the proposed remanufacturing strategy compared with traditional approaches (material recycling, casting, and machining). Liu et al. [3] developed a novel design-for-remanufacturing method under a level-set framework, which provides a solution for upgrading broken parts. A cost-driven process planning for PBF-CNC remanufacturing was proposed in the algorithmic framework laid down in our previous work [1]. In that work, an automated additive-subtractive feature extraction method is developed and process planning sequencing is formulated as a cost-minimization optimization problem. However, there are three significant limitations: 1). this work is focusing on primitives features and free-form features are not covered; 2). the inspection process for the used part or legacy part is not included; 3). commonly, there are defects or damaged area on the used part, the process for dealing with the defects should be investigated.
To sum up, the research gaps and objectives of this study is the development of a method that provides automated feature extraction and cost-driven process planning for an integrated HM machine. The main contributions are list as follows:
● Both primitive and free-form features are modelled in level set-based representations for the automated feature extraction which facilitate the process planning for HM remanufacturing;
● A collision-free DED-CNC process planning method is developed, resulting in the minimal cost in HM remanufacturing process;
● The defect and damaged area of the used part are considered to form a pre-machining feature in the process planning, which is an issue rarely addressed in the previous studies of process planning for remanufacturing.
The rest of the paper is structured as follows. In Section 2, the automated additive and subtractive feature extraction method is presented, which includes level set function representation for CAD and point clouds, extraction for pre-machining feature, intersection part extraction, intersection part modification for collision-free remanufacturing and individual feature extraction. Section 3 shows the cost-driven process planning methodology for DED-CNC remanufacturing, which includes cost estimation for DED-CNC remanufacturing, and sequence optimization. Section 4 demonstrates a case study to validate the efficacy of the proposed method. Finally, a conclusion is given in Section 5.
In geometric modelling, constructive solid geometry (CSG) and boundary representation (B-rep) are widely adopted. With CSG modelling, a physical object can be decomposed into multiple primitives and a sequence of Boolean operations. With the B-rep method, the solid is bounded by a set of closed and directional faces, which are bounded by edges and vertices. CSG modelling has the merits of supporting efficient Boolean operations and topology optimization [36]. Previous works on feature recognition/extraction in machining process planning have been developed for three decades [37,38]. However, these methods cannot be applied in remanufacturing or HM process. The reason is that most of the current machining feature extraction methods uses boundary representation (B-rep) format for feature modeling because it uniquely defines the faces and their topological patterns [38]. However, it has issues to deal with numerical calculations between two solid parts by adopting B-rep models for extracting features between the used part and final part. Therefore, in this work, a novel feature extraction method based on the level set function that implicitly represents models is developed for hybrid manufacturing in remanufacturing context.
The overall framework of the level set-based feature extraction method is presented in Figure 2. First the modeling history is extracted from the CAD model of the final part to be modelled in level set function representation (Section 2.1). The point cloud data is acquired from the used part and converted to be the level-set function representation (Section 2.2). Then, the pre-machining feature is extracted from the used part (Section 2.3). The intersection volume is then calculated by optimally overlapping the used part and the final part (Section 2.4). The intersection part is then modified with considering the collision problem and DED manufacturing constraints (Section 2.5). With The level set represented intersection part. The subtractive manufacturing volume (SMV) and additive manufacturing volume (AMV) can be identified and the individual AFs and SFs are extracted from the volumes (Section 2.6).
Level set function Φ(X)(Rn→R) describes the geometry in an implicit form, as shown in Eq (1).
{Φ(X)>0,X∈Ω/∂ΩΦ(X)=0,X∈∂ΩΦ(X)<0,X∈D/Ω | (1) |
where Ω/∂Ω is the material domain, D/Ω is the void, ∂Ω is the structural boundary.
In the level set function-based modelling approach, the 3D model is constructed by bounding the boundary surfaces, as:
Φ(X)=min{Φ1,Φ2,Φ3,…,Φn} | (2) |
As an example, the cube with (x0,y0,z0) as the center coordinates and (Hx,Hy,Hz) as the lengths on the x, y, z axis can be represented by bounding six planer surfaces of Φ1=Hx2−(x−x0)=0,Φ2=Hx2+(x−x0)=0,Φ3=Hy2−(y−y0)=0,Φ4=Hy2+(y−y0)=0,Φ5=Hz2−(z−z0)=0,Φ6=Hz2+(z−z0)=0, as shown in Figure 3.
In our previous work [1], the examples of primitive geometry (cube, sphere, cone and cylinder) are given. This work will focus on the level set function modelling for freeform geometries. From Eq (2), it can be manifested that finding the implicit forms for boundary surfaces is the most crucial work for the level set-based modelling approach. Algebraic techniques based on elimination theory enable the conversion of parametric expression to its implicit expression Φ(X)=0. Elimination theory investigates the conditions under that the sets of parametric expressions have common roots. The vanishing of the resultant is a necessary and sufficient condition for the parametric expressions to have a common non-trivial root. The implicitization of parametric geometry is based on the construction of these resultants.
A freeform 2.5D geometry can be constructed by extruding its freeform profile. As an example, in this study, the Bezier curve is implemented to represent the freeform profile. The parametric form of the Bezier curve is shown as:
F(u)=n∑i=0Bi,n(u)ˉPi | (3) |
where F(u)=[fx(u),fy(u)], ˉPi=[ˉpxi,ˉpyi], Bi,n(u)=C(n,i)ui(1−u)n−i, C(n,i) is the binomial coefficient: C(n,i)=n!i!(n−i)!, n is the degree of the curve, and i is the number of control points, ˉPix and ˉPiyare x and y coordinates of the control point.
By following elimination theory, the implicit form of the Bezier curve Φcurve(X) can be obtained by eliminating the parameter u between the parametric expressions in Eq (3) letting the resultant of them to be equal to zero.
As an example, a cubic Bezier curve is constructed by three control points: ˉP0=(0,0),ˉP1=(40,220),ˉP2=(200,40) and ˉP3=(0,0). By implementing the elimination theory, the implicit form for the Bezier curve can be obtained and the contour figure is shown in Figure 4a. Then, the 2.5D freeform shape can be modelled by Φ(X)=min(Φcurve,Φtop,Φbottom), where Φtop=157.5−z and Φbottom=z−122.5. (see Figure 4b.)
The parametric Bezier surface is extended from Bezier curve to u and v directions, as follows:
F(u,v)=n∑i=0m∑j=0Bi,n(u)Bj,m(v)ˉPi,j | (4) |
where F(u,v)=[fx(u,v),fy(u,v),fz(u,v)] and ˉPi,j=[ˉpxi,j,ˉpyi,j,ˉpzi,j].
Analogously, the elimination theory can help to find the implicit form for the parametric expression in Eq (4). A Bezier surface is modelled by 3 × 3 control points; the level set form of this surface is shown in Figure 5a. The Bezier shape is bounded with planar surfaces Φ1=x−200,Φ2=600−x,Φ3=y−200,Φ4=600−y, see Figure 5b.
The complex geometry can be constructed through Boolean operations on the level-set functions [39]. However, this representation will cause non-differentiable problems in numerical calculation. R-functions can combine level functions of a complex structure into a new smooth level set function by operations of R-conjunction ⋀ and R-disjunction ⋁, which are equivalent to Boolean operations ∩ and ∪ [40]. The operations of R-functions are defined as:
Unite:Φ1∪Φ2=max(Φ1,Φ2)=Φ1+Φ2+√Φ21+Φ22Intersect:Φ1∩Φ2=min(Φ1,Φ2)=Φ1+Φ2−√Φ21+Φ22Subtract:Φ1∖Φ2=min(Φ1,−Φ2)=Φ1−Φ2−√Φ21+Φ22 | (5) |
For a given used part, data acquisition is the first step to digitalize the part to point clouds. Therefore, the other situation is that we have the point cloud as the geometry input. To converting the point cloud data to the level set function model, the surface fitting techniques can be applied to obtain the parameters of surfaces and these parameters are used to forming the level set function representations. In this study, random sample consensus (RANSAC) surface fitting [41] is employed for surface fitting. The pseudocode for the forming level set function representation of the point clouds is given in Table 1. Figure 6 provides two examples of the surface fitting for cylindrical surfaces and sphere from point clouds from our previous results [21]. The color scale bars indicate the distance of each point to the fitted surface. The parameters of fitted surfaces are utilized for forming the level set functions through the proposed algorithm.
Input: point clouds of the used part P |
Set the max distance and max angular variation for fitting |
i=1 Remaining points Pm,i←P For the plane fitting, cylinder fitting, cone fitting, sphere fitting, free-form surface fitting: While there are enough points for supporting surface fitting in Pm,i: Parametric surface parameters Si← plane fitting from Pm,i Converting the parametric plane to implicit function: Φi←Si i=i+1 Remove the points Si which fit from remaining points to form new remaining points Pm,i |
Forming the level set function representation from collected implicit functions by Boolean operations: Φu←{Φ1,…,Φi} |
End Output: level set function representation of the used part Φu |
In the repairing process, the defects on the damaged part need to be machined into a surface cavity. This cavity is to be refilled by the deposition of materials to recover the local geometry of the part. Equivalently, in terms of the remanufacturing process, it is necessary to carve out defects from the damaged part to eliminate the perturbation for the remanufacturing process planning caused by the defects. In this study, the authors define the machining feature to carve out defects as a pre-machining feature since it has a similar concept as the pre-machining in the traditional machining process to remove the imperfections of the stock. It is important to mention that this study is focusing on remanufacturing end-of-life part with small defects which do not cover the any entire features on the end-of-life part.
To construct the pre-machining feature, the first step is to segment surface defects from 3D scan data. Many methods for surface defect segmentation have been presented in the literature [21,42,43]. In this work, random walks for unorganized point cloud segmentation [42] is adopted, since it does not rely on strong assumptions made on the characteristics of the expected defect or the geometry of the surrounding area. The algorithm segments defect areas on a weighted, undirected k-nearest neighbour graph (k-NNG) defined by local changes in point cloud properties. Since it is not the contribution of the authors' work, the detailed algorithm is not demonstrated. The interested reader can refer to the original work [42]. An example of the defect segmentation from point clouds by the random walks algorithm is shown in Figure 7. In the figure, the blue points indicate the points without defects and red points refer the points on the defective area.
Different machining features can be applied to carve out the defect area. In this study, the authors only investigate two basic machining features for the sake of simplicity. It is trivial to extend the method for other machining features. The hole and rectangular pocket are shown in Figure 8, and the level set function to represent these features are presented in Eq (6).
Hole:Φ=min{[R2−(x−x0)2−(y−y0)2],H2−(z−z0),H2+(z−z0)}Rectangularpocket:Φ=min{Hx2−(x−x0),Hx2+(x−x0),Hy2−(y−y0),Hy2+(y−y0),Hz2−(z−z0),Hz2+(z−z0)} | (6) |
It is worth investigating the type and parameters of the machining feature, which leads to minimal materials being carved out. Meanwhile, the machining feature must remove all the defects. The problem can be mathematically formulated as a constrained optimization problem. For a given machining feature, the optimization problem is solving the optimal parameters. The objective function is minimizing the volume of the given machining feature by integrating dxdydz in the material domain, referred to Eq (7).
min.f(a)=∫H(Φ(X,a))dxdydz | (7) |
where H() is the Heaviside function, Φ() is the level set function for the given machining feature, X=(x,y,z), a=(a1,…,an) which indicates the parameters for the given machining feature.
To satisfy the condition that all defects are removed, the defective points need to be enclosed by the machining feature. Assuming there are defect points (p1,p2,…,pM), M series of constraints are formulated as in Eq (8) and the schematic plot is presented in Figure 9.
s.t.gi(a)=Φ(pi,a)≥0,i∈{1,…,M} | (8) |
Lagrange formulation of this problem is written as:
L(a1,…,an,λ1,…,λn,η1,…,ηn)=f(a1,…,an)−M∑i=1λi{gi(a1,…,an)−ηi2} | (9) |
where λi is the i-th Lagrange multiplier and ηi is the i-th slack variable.
The sensitivity ∂L∂a1 of the Lagrange formulation is derived through Eq (10); others can be calculated similarly.
∂f∂a1=∫∂H(Φ(X,a))∂Φ∂Φ∂a1dΩ=∫δ(Φ(X,a))∂Φ∂a1dΩ | (10) |
where δ is the Dirac delta function.
This problem can be solved with a gradient-based optimization solver by updating the variables a1,…,an,λ1,…,λn,η1,…,ηn with their corresponding sensitivity.
Examples of the optimal parameters of a hole and a rectangular pocket features are illustrated in Figure 10. From the two pre-machining features, the minimal volume of the feature can be determined. In this example, the hole feature has a volume of 3.657mm3 and rectangular pocket has the volume of 4.3665mm3. Therefore, the hole feature is optimal for pre-machining.
As the level set function of the used part and final part are formulated, the relative position needs to be identified between two solid models to prepare for feature extraction. The objective is to maximize the overlapping material volume because the cost of AM is strongly affected by the volume of the new material to deposit.
Φu and Φf are the level set functions of the used part and the final part, respectively, within the global coordinate system X=(x,y,z). ˜X is a local coordinate system attached to the used part. Through Eq (11), an optimization problem can be formulated to figure out the translation and rotation of the local coordinate system (˜X) needed to maximize the overlapping volume between Φu and Φf.
[˜X1]=[Rz(θx)Ry(θy)Rx(θz)T(tx,ty,tz)01][X1] | (11) |
where Rx, Ry,Rz are the rotation matrix along x, y, z direction with the variables of θx,θz,θy, T is the translation matrix with the variables of tx,ty,tz.
The used part Φi(θx,θz,θy,tx,ty,tz) has translation variables and rotation variables. The final part is fixed by Φf. The intersection part (Φi) is the intersection of the used part and the final part, given as:
Φi(θx,θz,θy,tx,ty,tz)=Φu(θx,θz,θy,tx,ty,tz)∩Φf=min(Φu(θx,θz,θy,tx,ty,tz),Φf) | (12) |
The maximization optimization problem can be formulated of maximizing the intersection part by optimizing thevariablesθx,θz,θy,tx,ty,tz, through in Eq (13):
min.f(θx,θz,θy,tx,ty,tz)=−∫H(Φi(X,θx,θz,θy,tx,ty,tz))dΩ | (13) |
The sensitivity ∂f∂tx of the objective function is derived through Eq (14); others can be calculated similarly.
∂f∂tx=−∫∂H(Φi(θx,θz,θy,tx,ty,tz))∂Φi∂Φi∂txdΩ=−∫δ(Φi(θx,θz,θy,tx,ty,tz))∂Φi∂txdΩ | (14) |
This problem can be solved with a gradient-based optimization solver. In the gradient-based optimization algorithm, the (θx,θz,θy,tx,ty,tz) = (0, 0, 0, 0, 0, 0) is set as the initial values. Generally, to prevent a local optimum issue, a multi-start strategy is suggested, i.e., to parallelly run the optimization program with a different initial guess of variables.
Figure 11 demonstrates an example of the intersection part extraction. The used part and the final part are represented by its level set function Φu and Φf respectively. Eq (13) helps to find the optimal transformation to transform Φu to ˜Φu with the optimized rotation angle (θx=−90o,θy=0o,θz=0o) and translation (tx=−20.1,ty=−110.7,tz=15). The intersection part Φi can be obtained by ˜Φu∩Φf.
The geometry of intersection part geometry is not generally acceptable for AM processes. Figure 12 provides an illustration of collision problems in the DED process, and the material deposition nozzles may have collisions with the intersection part. Therefore, it is crucial to modify the intersection part by analyzing the tool accessibility constraints of the DED process.
Initially, the intersection part and DED nozzle are formulated as Φi and Φn by the level-set representation, respectively. In addition, the deposited material volume by AM is represented as Φd=Φf/Φi, as shown in Figure 13. The voxel representations for Φi, Φn and Φd are computing by applying Heaviside functions Vi=H(Φi), Vn=H(Φi), and Vd=H(Φd), separately.
As far as the collision detection is concerned, the collision-free rigid motion of the DED nozzle in rigid motion needs to be calculated. In this study, dilation as a morphology operation is adopted to analyze the spatial planning of the DED nozzle. As one of the basic operations in mathematical morphology, dilation operation ⊕ is defined as:
A⊕B=⋃b∈BAb | (15) |
where Ab represents the solid A transformed by a rigid transformation b, B is a structuring element, which is termed filters. For level set function represented models, the dilation operation is expressed as:
ΦA⊕ΦB=⋃ΦB(b)≥0ΦA(b) | (16) |
For the DED process, the materials are deposited on the deposition volume layer by layer, which indicates that the tip of the deposition nozzle requires going through each point of the deposition volume (Figure 14a). It is crucial to mention that in a practical case, the vertical distance between the tip of the DED nozzle and the deposition area is not zero. The vertical distance is assumed to be zero for the sake of simplifying in explaining the proposed method. The technical implantation of the approach will be discussed with considering the vertical distance later in this section. It is meaningful to explore all motions of the DED nozzle to deposit the materials in the deposition volume. In the proposed method, the motions of the DED nozzle during deposition is calculated by dilating the deposition volume by the DED nozzle: Φm=Φn⊕Φd, and the authors define Φm as motion space of the DED nozzle, as presented in Figure 14b.
Theorem:
The modified intersection part ˜Φi can be derived from: ˜Φi=Φi∖Φm=Φi∖(Φn⊕Φd), which leads no collision with the DED nozzle in operation, as shown in Figure 14d.
Proof:
In the condition of no collision occurring during deposition, ˜Φi∩˜Φm=∅ is required to be satisfied, where ˜Φm is the new motion space derived from the modified intersection part ˜Φm=Φn⊕˜Φd. ˜Φm can be expanded into:
˜Φm=Φn⊕(Φf∖˜Φi)=Φn⊕(Φf∩(−˜Φi))=Φn⊕(Φf∩(−(Φi∩(−Φm)))) | (17) |
where Φf indicates the level set function of the final part. According to the associativity, commutativity of Boolean operation and distribution of dilation, Eq (17) can be rearranged as:
˜Φm=(Φn⊕Φd)∩(Φn⊕Φm) | (18) |
Therefore, no collusion condition is derived as:
˜Φi∩˜Φm=Φi∩(−Φm)∩(Φn⊕Φd)∩(Φn⊕Φm) | (19) |
Since Φn⊕Φd=Φm, (−Φm)∩(Φn⊕Φd)=∅. So, ˜Φi∩˜Φm=Φi∩∅∩(Φn⊕Φm). Due to the annihilator law for ∩, ˜Φi∩˜Φm=∅.
The authors develop an algorithm to implement the proposed method for discrete level set function representations, also considering the vertical distance (d) between the nozzle tip and deposition area. The pseudocode for the proposed algorithm is presented in Table 2. An example of the implantation of the proposed method is shown in Figure 15. Figure 15a presents the original intersection part and Figure 15b shows deposition volume derived from the original intersection part by Φf∖Φi. The motion space calculated from Eq (16) is shown in Figure 15c, and Figure 15d gives the modified intersection part ˜Φi.
Input: discrete level set function Φn, Φd and Φi; vertical distance d |
Φm←0 with size of Φd |
[x, y, z] = index of Φd>0 in x, y, z directions The position of the tip is obtained from Φn as x_tip, y_tip, z_tip |
For i = 1 to size of x direction: Move on x direction Mx = x (i) - x_tip Move on y direction My = y (i) - y_tip |
Move on z direction Mz = z (i) - z_tip + d |
New position of DED nozzle Φ˜n = translating the Φd over Mx,My,Mz Φm=Φm∪Φ˜n=max(Φm,Φ˜n) End Output: discrete level set function of modified intersection part ˜Φi=Φi∖Φm=min(Φi,−Φm) |
As the modified intersection part has been calculated, a subtractive manufacturing volume (SMV) and an additive manufacturing volume (AMV) can be calculated in their level set function representations as:
ΦSFV=Φu∖˜ΦiΦAFV=Φf∖˜Φi | (20) |
Individual features are required to be recognized and extracted from SMV and AMV. The research of CSG-based feature recognition has been developed from the 1990s, but this technique did not go far primarily due to the non-uniqueness of CSG trees [38]. Recently, there are some research efforts have been devoted to address non-uniqueness problems in CSG and show the strength in recognize sophisticated machining features by 3D convolution neuron network [44,45]. Therefore, developing a new machining feature recognition method is not a contribution to this study. The level set function representation of SFV is converted into 3D voxel grid information VSFG by Heaviside functionH, and then subtractive features (SFs) can be extracted by any CSG-based feature recognition techniques; see Eq (21).
VSFV=H(ΦSFV)→{SF1,SF2,SF3,...SFn} | (21) |
AFV comprises both additive features (AFs) and SFs. In the AM process, leaving a sufficient over-thickness to have a finishing operation is vital for meeting the tolerance and surface roughness requirements. The over-thickness value is estimated by the required specifications of the final feature, the surface roughness generated by the AM processes and the machining conditions [34]. With considering over-thickness, the modified additive feature volume (¯AFV) is modified. The level set function representation of ¯AFV can be derived via Eq (22), where t represents the over-thickness value. The residual subtractive feature volume is obtained via Eq (23). Similarly, the individual SFs could be recognized by a CSG-based feature recognition method by Eq (24).
Φ¯AFV=(ΦAFV+t)∖˜Φi | (22) |
Φ¯SFV=Φ¯AFV∖ΦAFV | (23) |
Φ¯SFV→{SFn+1,SFn+2,SFn+3,...SFn+m} | (24) |
A large number of AFs and SFs are obtained from the proposed feature extraction method. Each feature represents an operation in the AM or SM process. Under considering the topological relationship of different features, the sequences of some operations are forced. Therefore, precedence constraints between operations are required to formulated to respect the hybrid additive-subtractive manufacturing rules. The precedence constraints are comprehensively summarized, and interested readers can refer to [1,34].
Although precedence constraints are formulated, some residual process sequences are still undermined. In order to develop the process planning problem into a process sequence optimization, the cost for each operation/feature is required to be estimated. With precedence constraints between features and cost model for each feature, an integer programming model is formulated to calculate the optimal process plan that minimizes the overall remanufacturing cost.
The motivation of the cost estimation for this study is approximating the cost models for the integer programming model to determine the optimal process plan, rather than exploring the precise cost for each operation. Although there are numbers of publications are relevant to cost estimation for the subtractive and additive manufacturing process [46,47,48], few researchers focus on the constructing cost model for hybrid additive-subtractive manufacturing system, explicitly considering the change cost between two different operations. Therefore, in this section, rough and fast estimations are given for SFs and AFs, and the change costs between various features are also introduced.
The total cost comprises operation cost and tool cost as:
CSF=Chh∗tSF+Ctool∗ntool | (25) |
where CSF is the total cost of an SF, Chh is the hourly operation cost for HM machine operation, tSF is the machining time for the SF, Ctool is the cost of each cutting tool, and ntool is the number of tool changes. Most commercial CAM software systems can estimate the machining time tSF by dividing the tool path in the milling process by the programmed feed rate.
The cost of AF is determined by the machine cost and material consumption cost, see Eq (26).
CAF=Chh∗tAF+Cm−AF | (26) |
where CAF is the total cost of an AF, Chh is the hourly operation cost for HM machine operation, tAF is the building time of the AF, and Cm−AF is the cost of material consumption.
The cost of material consumption in DED process is approximately calculated from the volume of the feature and its support structure, as:
Cm−AF=(VAFρ+VAF−s¯ρ)μCunitmaterial | (27) |
where, VAF and VAF−s refer to the volume of the building part and support structure, ρ is the material density, ¯ρ is the material density of the support structure, and Cunitmaterial indicates the price per unit of material. In DED process, complex gas flow leads a diffusion of powder distribution, which results the low powder efficiency because some powder cannot reach to the meltpool. Powder efficiency rate μ varies between different machines, and in this research the value is referred to [49,50], as 70%.
For the DED process, the building time tAF estimation adopted an analytical build time model which is proposed in [51], and the general equation is:
tAF=DepositionTime+RapidMovementsTime | (28) |
This model uses G-code of the part as input and an algorithm extracts the kinematic characteristics of the nozzle to estimate very accurate build time results, since the acceleration and deceleration of the machine head is considered.
For the hybrid additive-subtractive system, the AM/SM operations are switching by changing different tools. It is crucial to discuss the cost of change between two consecutive operations since it is costly due to frequent tool changing. Besides the tool change cost, the re-orientation of the workpiece also results in costs. In this study, CR and CT represent indexes of the re-orientation cost, and the tool change cost, respectively. The details of the calculation are given below.
1) Re-orientation cost:
While the orientation of the workpiece is switched in the HM machine, a re-orientation change occurs that requires workpiece fixing and laser calibration.
CR=Chh∗tre | (29) |
For the index of feature i,j∈F={1,2,...,F}, the index of cost for orientation CR is formed by grouping the cost of orientation between any two features.
2) Tool change cost:
For HM machine the tool change cost is formulated as:
CT=Chh∗ttc | (30) |
Similarly, the index of tool change cost CT is constructed by grouping the cost of tool change cost between any two features.
As the cost model for each feature, change cost, and precedence constraints are obtained. The process sequence optimization problem is formulated as an integer programming model and solved by branch and fathoming algorithm [52]. In a directed graph visualization of the model, a node represents a feature/operation and the cost model for them is calculated from Section 4.1.1 and 4.1.2, and the directed line indicates the precedence relation between two features/operations and the change costs are derived from Section 4.1.3. As an example, AFs (AF1 and AF2) and SFs (SF1, SF2, SF3) are extracted from the proposed feature recognition method. The cost for each node is calculated and the change cost between any two nodes are formulated. The precedence constraints are applied to these nodes initially as dash lines (Figure 16a). With the optimization of the integer programming model, the output is a sequence of operations that results in minimal cost (Figure 16b).
In this section, the proposed method is verified by the correctness and efficiency of a virtual case study.
Figure 17a shows the point cloud scanned from the used part, which is required to be remanufactured. Figure 17b represents the CAD model of the final part, which has different functionalities compared to the used part. Especially, the final part has primitive features and 2.5D freeform feature.
The level set function for the final part is built from the CAD modelling on a design domain of size 150 × 150 × 150 with grid size ∆x (0.5 mm), as Figure 18 shown. In the figure, the modelling history is also given, and the mathematical formulation of each level set function is provided in the Appendix.
In terms of the used part, the RANSAC surface fitting technique is adapted and the max distance and angular distance variants are set as 0.002 m and 5 degrees, respectively. The surface fitting results are shown in Figure 19a. By implementing the k-NNG-based defect identification method, the defect points are differentiated from the point clouds Figure 19b. The level set function for the used part is constructed in Figure 20a with modelling history: Φu=min(Φcube1,−Φcube2,−Φcube3,−Φcube4)and formulations for each level set functions are given in the Appendix. The optimal pre-machining feature can be obtained from the defect points, and the used part is updated with the pre-machining feature, as shown in Figure 20b.
The proposed intersection part extraction algorithm is applied to find the relative position between the used part and the final part. The optimization results of the translation and rotation variables are listed in Table 3 and the output of the intersection part is shown in Figure 21.
Translation (mm) | Rotation (°) | |||||
tx | ty | tz | θx | θy | θz | |
Optimal variables: | 4.07 | 4.21 | −1.33 | 0 | 0 | 0 |
For the next step, the intersection part is modified by considering the collision problem in the DED process. The DED nozzle is modelled in level set function as shown in Figure 22a and the vertical distance d is set as 10 mm. Figure 22b shows the deposition volume, which is obtained by subtracting the final part by the original intersection part. The motion space that represents all possible motions of the DED nozzle during deposition is derived from the proposed algorithm (Table 2); see Figure 22c. The result of the modified intersection part through subtracting the original intersection part by motion space is presented in Figure 22d.
By subtracting the final part and the used part by the modified intersection part, SFV and AFV can be collected, respectively. Then, each SF and AF are extracted, as presented in Figure 23. With respecting hybrid manufacturing rules, precedence constraints are applied to all features.
The parameters and machine resources that are used in the cost estimation are listed in Table 4. In this study, Ti-6Al-4V is used as the material for the DED process. The sequence optimization problem is solved by a branch-and-bound solver and the optimized process plans are presented in Table 5, and the optimal remanufacturing plan costs $ 1835.24.
Manufacturing parameters | Manufacturing resources | ||||
Parameter | Notation | Value | Tool ID | Tool type | Diameter (mm) |
Machine cost /hour | Chh | 100 $/h | T1 | End mill | 20 |
Each tool cost | Ctool | 5 $ | T2 | End mill | 10 |
Density | ρ | 4.43kg/dm3 | T3 | End mill | 5 |
Support structure ratio | ¯ρ/ρ | 0.4 | T4 | Drill | 5 |
Unit price of the metal powder | Cunitmaterial | 450 $/kg | T5 | Drill | 10 |
Re-orientation time | tre | 0.35 h | T6 | DED | - |
Tool change time | ttc | 0.17 h |
Sequence | 1 | 2 | 3 | 4 | 5 | 7 | 8 | 9 | 10 | 11 | 12 |
Features | SF1 | SF2 | AF1 | SF9 | AF2 | SF5 | SF8 | SF3 | SF6 | SF7 | SF4 |
Machine | M1 | M1 | M2 | M1 | M2 | M1 | M1 | M1 | M1 | M1 | M1 |
TAD | + z | + z | + z | + z | + z | + y | + x | + x | + y | + y | + y |
Tool | T1 | T1 | T6 | T2 | T6 | T4 | T4 | T4 | T2 | T3 | T3 |
Nowadays, the combination of AM and SM in a single workstation is emerging to provide a more flexible and productive and capable manufacturing approach comparing with traditional manufacturing strategies. Because it utilizes the merits of AM and SM to add and remove features flexibly, HM has the potentials to raise remanufacturing technology to a higher level. In this study, taking to account the benefits of the HM process, hybrid DED-CNC manufacturing technology in a single workstation is investigated to remanufacture an end of life part (used part) to a new part (final part) with new functionalities, avoiding the material recycling process.
This paper has demonstrated a novel feature extraction algorithm and a cost-driven process planning method for hybrid DED-CNC manufacturing in a remanufacturing context. Specifically, starting from point clouds for the used part and a solid CAD model for the final part, geometry modeling is performed to transform the input to level set representations. Also, the defects on the used part are investigated and an optimal pre-machining feature is derived from the proposed method and applied to remove the perturbation caused by the defects. The feature extraction method, developed under the level set framework, is proposed as an automated process to extract the AM and SM features for remanufacturing process planning, which addresses the numerical calculation between two solid models. Moreover, the collision problems of DED nozzle during deposition are considered and this method provides collision-free motions. With the carefully developed hybrid DED-CNC cost model, the process planning work is converted to an -integer programming model as an optimization problem. Finally, the optimal process plan can be determined by solving the optimization problem.
For future work, there are more research works need to be done to expand the current methodology. A practical case study is required to validate the work presented in this paper. In the next stage, the relevant use case can be remanufacturing for high-value component, such as mold, die, and turbine blade. Moreover, in the present study, the AM and SM processes are considered as a 3-axis type. However, the feature extraction and process planning problems for multi-axis capability need to be addressed. In addition, the fixture design, AM support structures [53] in the real cases need to be investigated in the future work. In the metal additive manufacturing, the residual deformation is a significant problem and it also may affect the feature extraction and process planning, so it can also be explored in the further studies.
We express our appreciation to the other team members in the Laboratory of Intelligent Manufacturing, Design and Automation (LIMDA) group for sharing their wisdom during the research. The authors acknowledge the NSERC (Grant No. RGPIN-2017-04516 and CRDPJ 537378-18) and Minister of Economic Development, Trade and Tourism (through Major Innovation Project) for funding this project.
All authors declare no conflicts of interests in this paper.
[1] |
Y. Zheng, J. Liu, R. Ahmad, A cost-driven process planning method for hybrid additive-subtractive remanufacturing, J. Manuf. Syst., 55 (2020), 248-263. doi: 10.1016/j.jmsy.2020.03.006
![]() |
[2] |
G. D. Hatcher, W. L. Ijomah, J. F. C. Windmill, Design for remanufacture: a literature review and future research needs, J. Cleaner Prod., 19 (2011), 2004-2014. doi: 10.1016/j.jclepro.2011.06.019
![]() |
[3] |
J. Liu, Y. Zheng, Y. Ma, A. Qureshi, R. Ahmad, A Topology Optimization Method for Hybrid Subtractive-Additive Remanufacturing, Int. J. Precis. Eng. Manuf., 7 (2020), 939-953. doi: 10.1007/s40684-019-00075-8
![]() |
[4] | A. M. King, S. C. Burgess, W. Ijomah, C. A. McMahon, Reducing waste: repair, recondition, remanufacture or recycle?, J. Sustain. Dev., 14 (2006), 257-267. |
[5] |
X. Zhang, W. Li, W. Cui, F. Liou, Modeling of worn surface geometry for engine blade repair using Laser-aided Direct Metal Deposition process, Manuf. Lett., 15 (2018), 1-4. doi: 10.1016/j.mfglet.2017.11.001
![]() |
[6] | Z. Liu, Q. Jiang, T. Li, S. Dong, S. Yan, H. Zhang, et al., Environmental benefits of remanufacturing: A case study of cylinder heads remanufactured through laser cladding, J. Cleaner Prod., 133 (2016), 1027-1033. |
[7] | L. Chen, T. Y. Lau, K. Tang, Manufacturability analysis and process planning for additive and subtractive hybrid manufacturing of Quasi-rotational parts with columnar features, Comput. Aided Des., 118 (2020), 102759. |
[8] | P. Stavropoulos, P. Foteinopoulos, A. Papacharalampopoulos, H. Bikas, Addressing the challenges for the industrial application of additive manufacturing: Towards a hybrid solution, Int. J. Lightweight Mater. Manuf., 1 (2018), 157-168. |
[9] |
J. M. Flynn, A. Shokrani, S. T. Newman, V. Dhokia, Hybrid additive and subtractive machine tools - Research and industrial developments, Int. J. Mach. Tools Manuf., 101 (2016), 79-101. doi: 10.1016/j.ijmachtools.2015.11.007
![]() |
[10] | M. Cortina, J. I. Arrizubieta, J. E. Ruiz, E. Ukar, A. Lamikiz, Latest Developments in Industrial Hybrid Machine Tools that Combine Additive and Subtractive Operations, Materials, 11 (2018), 2583. |
[11] |
A. Joshi, S. Anand, Geometric Complexity Based Process Selection for Hybrid Manufacturing, Procedia Manuf., 10 (2017), 578-589. doi: 10.1016/j.promfg.2017.07.056
![]() |
[12] |
L. Chen, K. Xu, K. Tang, Optimized sequence planning for multi-axis hybrid machining of complex geometries, Comput. Graph., 70 (2018), 176-187. doi: 10.1016/j.cag.2017.07.018
![]() |
[13] |
C. Liu, Y. Li, S. Jiang, Z. Li, K. Xu, A sequence planning method for five-axis hybrid manufacturing of complex structural parts, J. Eng. Manuf., 234 (2020), 421-430. doi: 10.1177/0954405419883052
![]() |
[14] |
Z. Zhu, V. G. Dhokia, A. Nassehi, S. T. Newman, A review of hybrid manufacturing processes - state of the art and future perspectives, Int. J. Comput. Integr. Manuf., 26 (2013), 596-615. doi: 10.1080/0951192X.2012.749530
![]() |
[15] |
Z. Zhu, V. Dhokia, S. T. Newman, A. Nassehi, Application of a hybrid process for high precision manufacture of difficult to machine prismatic parts, Int. J. Adv. Manuf. Technol., 74 (2014), 1115-1132. doi: 10.1007/s00170-014-6053-7
![]() |
[16] |
S. T. Newman, Z. Zhu, V. Dhokia, A. Shokrani, Process planning for additive and subtractive manufacturing technologies, CIRP Ann., 64 (2015), 467-470. doi: 10.1016/j.cirp.2015.04.109
![]() |
[17] | M. Behandish, S. Nelaturi, J. de Kleer, Automated process planning for hybrid manufacturing, Comput. Aided Des., 102 (2018), 115-127. |
[18] |
H. ElMaraghy, M. Moussa, Optimal platform design and process plan for managing variety using hybrid manufacturing, CIRP Ann., 68 (2019), 443-446. doi: 10.1016/j.cirp.2019.03.025
![]() |
[19] | K. L. Basinger, C. B. Keough, C. E. Webster, R. A. Wysk, T. M. Martin, O. L. Harrysson, Development of a modular computer-aided process planning (CAPP) system for additive-subtractive hybrid manufacturing of pockets, holes, and flat surfaces, Int. J. Adv. Manuf. Technol., 96 (2018), 2407-2420. |
[20] | R. Cottam, M. Brandt, Laser Cladding of Ti-6Al-4V Powder on Ti-6Al-4V Substrate: Effect of Laser Cladding Parameters on Microstructure, Phys. Procedia, 12 (2011), 323-329. |
[21] |
Y. Zheng, J. Liu, Z. Liu, T. Wang, R. Ahmad, A primitive-based 3D reconstruction method for remanufacturing, Int. J. Adv. Manuf. Technol., 103 (2019), 3667-3681. doi: 10.1007/s00170-019-03824-w
![]() |
[22] |
Y. Zheng, A. J. Qureshi, R. Ahmad, Algorithm for remanufacturing of damaged parts with hybrid 3D printing and machining process, Manuf. Lett., 15 (2018), 38-41. doi: 10.1016/j.mfglet.2018.02.010
![]() |
[23] |
X. Zhang, W. Li, K. M. Adkison, F. Liou, Damage reconstruction from tri-dexel data for laser-aided repairing of metallic components, Int. J. Adv. Manuf. Technol., 96 (2018), 3377-3390. doi: 10.1007/s00170-018-1830-3
![]() |
[24] | M. Goyal, S. Murugappan, C. Piya, W. Benjamin, Y. Fang, M. Liu, et al., Towards locally and globally shape-aware reverse 3D modeling, Comput. Aided Des., 44 (2012), 537-553. |
[25] |
J. M. Wilson, C. Piya, Y. C. Shin, F. Zhao, K. Ramani, Remanufacturing of turbine blades by laser direct deposition with its energy and environmental impact analysis, J. Cleaner Prod., 80 (2014), 170-178. doi: 10.1016/j.jclepro.2014.05.084
![]() |
[26] | Q. Liu, Y. Wang, H. Zheng, K. Tang, H. Li, S. Gong, TC17 titanium alloy laser melting deposition repair process and properties, Opt. Laser Technol., 82 (2016), 1-9. |
[27] |
X. Zhang, W. Li, X. Chen, W. Cui, F. Liou, Evaluation of component repair using direct metal deposition from scanned data, Int. J. Adv. Manuf. Technol., 95 (2018), 3335-3348. doi: 10.1007/s00170-017-1455-y
![]() |
[28] |
Z. Zhao, J. Chen, Q. Zhang, H. Tan, X. Lin, W. Huang, Microstructure and mechanical properties of laser additive repaired Ti17 titanium alloy, Trans. Nonferr. Metals Soc., 27 (2017), 2613-2621. doi: 10.1016/S1003-6326(17)60289-9
![]() |
[29] |
D. Baca, R. Ahmad, The impact on the mechanical properties of multi-material polymers fabricated with a single mixing nozzle and multi-nozzle systems via fused deposition modeling, Int. J. Adv. Manuf. Technol., 106 (2020), 4509-4520. doi: 10.1007/s00170-020-04937-3
![]() |
[30] | D. M. B. Lopez, R. Ahmad, Tensile Mechanical Behaviour of Multi-Polymer Sandwich Structures via Fused Deposition Modelling, Polymers, 12 (2020), 651. |
[31] |
J. Y. Hascoë t, S. Touzé, M. Rauch, Automated identification of defect geometry for metallic part repair by an additive manufacturing process, Weld. World, 62 (2018), 229-241. doi: 10.1007/s40194-017-0523-0
![]() |
[32] |
R. Ahmad, S. Tichadou, J. Y. Hascoet, A knowledge-based intelligent decision system for production planning, Int. J. Adv. Manuf. Technol., 89 (2017), 1717-1729. doi: 10.1007/s00170-016-9214-z
![]() |
[33] |
Z. Zhu, V. Dhokia, S. T. Newman, A novel decision-making logic for hybrid manufacture of prismatic components based on existing parts, J. Intell. Manuf., 28 (2017), 131-148. doi: 10.1007/s10845-014-0966-8
![]() |
[34] |
V. T. Le, H. Paris, G. Mandil, Process planning for combined additive and subtractive manufacturing technologies in a remanufacturing context, J. Manuf. Syst., 44 (2017), 243-254. doi: 10.1016/j.jmsy.2017.06.003
![]() |
[35] |
V. T. Le, H. Paris, G. Mandil, Environmental impact assessment of an innovative strategy based on an additive and subtractive manufacturing combination, J. Cleaner Prod., 164 (2017), 508-523. doi: 10.1016/j.jclepro.2017.06.204
![]() |
[36] |
J. Liu, Q. Chen, Y. Zheng, R. Ahmad, J. Tang, Y. Ma, Level set-based heterogeneous object modeling and optimization, Comput. Aided Des., 110 (2019), 50-68. doi: 10.1016/j.cad.2019.01.002
![]() |
[37] |
B. Babic, N. Nesic, Z. Miljkovic, A review of automated feature recognition with rule-based pattern recognition, Comput. Ind., 59 (2008), 321-337. doi: 10.1016/j.compind.2007.09.001
![]() |
[38] |
A. K. Verma, S. Rajotia, A review of machining feature recognition methodologies, Int. J. Comput. Integr. Manuf., 23 (2010), 353-368. doi: 10.1080/09511921003642121
![]() |
[39] | J. Liu, A. C. To, Computer-Aided Design-Based Topology Optimization System With Dynamic Feature Shape and Modeling History Evolution, J. Mech. Des., 142 (2020), 071704. |
[40] |
S. Cai, W. Zhang, J. Zhu, T. Gao, Stress constrained shape and topology optimization with fixed mesh: A B-spline finite cell method combined with level set function, Comput. Methods Appl. Mech. Eng., 278 (2014), 361-387. doi: 10.1016/j.cma.2014.06.007
![]() |
[41] |
R. Schnabel, R. Wahl, R. Klein, Efficient RANSAC for point-cloud shape detection, Comput. Graph. Forum, 26 (2007), 214-226. doi: 10.1111/j.1467-8659.2007.01016.x
![]() |
[42] |
T. Hitchcox, Y. F. Zhao, Random walks for unorganized point cloud segmentation with application to aerospace repair, Procedia Manuf., 26 (2018), 1483-1491. doi: 10.1016/j.promfg.2018.07.093
![]() |
[43] |
L. Li, C. Li, Y. Tang, Y. Du, An integrated approach of reverse engineering aided remanufacturing process for worn components, Robot. Comput. Integr. Manuf., 48 (2017), 39-50. doi: 10.1016/j.rcim.2017.02.004
![]() |
[44] |
Z. Zhang, P. Jaiswal, R. Rai, FeatureNet: Machining feature recognition based on 3D Convolution Neural Network, Comput. Aided Des., 101 (2018), 12-22. doi: 10.1016/j.cad.2018.03.006
![]() |
[45] |
P. Shi, Q. Qi, Y. Qin, P. Scott, X. Jiang, A novel learning-based feature recognition method using multiple sectional view representation, J. Intell. Manuf., 31 (2020), 1291-1309. doi: 10.1007/s10845-020-01533-w
![]() |
[46] |
J. Y. Jung, Manufacturing cost estimation for machined parts based on manufacturing features, J. Intell. Manuf., 13 (2002), 227-238. doi: 10.1023/A:1016092808320
![]() |
[47] | M. Barclift, S. Joshi, T. Simpson, C. Dickman, Cost Modeling and Depreciation for Reused Powder Feedstocks in Powder Bed Fusion Additive Manufacturing, Proceedings of the 26th Annual International Solid Freeform Fabrication Symposium - An Additive Manufacturing Conference. (2016), 2007-2028. |
[48] |
Z. Bouaziz, J. Ben Younes, A. Zghal, Cost estimation system of dies manufacturing based on the complex machining features, Int. J. Adv. Manuf. Technol., 28 (2006), 262-271. doi: 10.1007/s00170-004-2179-3
![]() |
[49] |
S. Takemura, R. Koike, Y. Kakinuma, Y. Sato, Y. Oda, Design of powder nozzle for high resource efficiency in directed energy deposition based on computational fluid dynamics simulation, Int. J. Adv. Manuf. Technol., 105 (2019), 4107-4121. doi: 10.1007/s00170-019-03552-1
![]() |
[50] |
R. Koike, S. Takemura, Y. Kakinuma, M. Kondo, Enhancement of powder supply efficiency in directed energy deposition based on gas-solid multiphase-flow simulation, Procedia CIRP, 78 (2018), 133-137. doi: 10.1016/j.procir.2018.09.061
![]() |
[51] |
G. Komineas, P. Foteinopoulos, A. Papacharalampopoulos, P. Stavropoulos, Build Time Estimation Models in Thermal Extrusion Additve Manufacturing Process, Procedia Manuf., 21 (2018), 647-654. doi: 10.1016/j.promfg.2018.02.167
![]() |
[52] |
D.-H. Lee, D. Kiritsis, P. Xirouchakis, Branch and fathoming algorithms for operation sequencing in process planning, Int. J. Prod. Res, 39 (2001), 1649-1669. doi: 10.1080/00207540010028100
![]() |
[53] | H. Yu, J. Liu, Self-Support Topology Optimization With Horizontal Overhangs for Additive Manufacturing, J. Manuf. Sci. Eng., 142 (2020), 091003. |
![]() |
![]() |
1. | Habiba Zahir Imam, Hamdan Al-Musaibeli, Yufan Zheng, Pablo Martinez, Rafiq Ahmad, Vision-based spatial damage localization method for autonomous robotic laser cladding repair processes, 2023, 80, 07365845, 102452, 10.1016/j.rcim.2022.102452 | |
2. | Wenkang Zhang, Yufan Zheng, Rafiq Ahmad, The integrated process planning and scheduling of flexible job-shop-type remanufacturing systems using improved artificial bee colony algorithm, 2022, 0956-5515, 10.1007/s10845-022-01969-2 | |
3. | Kumar Kanishka, Bappa Acherjee, A systematic review of additive manufacturing-based remanufacturing techniques for component repair and restoration, 2023, 89, 15266125, 220, 10.1016/j.jmapro.2023.01.034 | |
4. | Owen Cooke, Hamdan Al-Musaibeli, Rafiq Ahmad, 2023, A Feature Extraction Algorithm for Hybrid Manufacturing and Its Application in Robot-Based Additive and Subtractive Processes, 978-1-6654-6472-7, 1, 10.1109/ICRAI57502.2023.10089593 | |
5. | Jikai Liu, Jiaqi Huang, Yufan Zheng, Shuai Hou, Shuzhi Xu, Yongsheng Ma, Chuanzhen Huang, Bin Zou, Lei Li, Challenges in topology optimization for hybrid additive-subtractive manufacturing: A review, 2023, 00104485, 103531, 10.1016/j.cad.2023.103531 | |
6. | Wenkang Zhang, Yufan Zheng, Rafiq Ahmad, An energy-efficient multi-objective integrated process planning and scheduling for a flexible job-shop-type remanufacturing system, 2023, 56, 14740346, 102010, 10.1016/j.aei.2023.102010 | |
7. | Neil Wilson, Manhar Gupta, Maciej Mazur, Milan J. Patel, Vu Nguyen, Stefan Gulizia, Ivan S. Cole, Analysis of self-supporting conformal cooling channels additively manufactured by hybrid directed energy deposition for IM tooling, 2024, 132, 0268-3768, 421, 10.1007/s00170-024-13291-7 | |
8. | Sridhar Meka, Sreeramulu Dowluru, Lingaraju Dumpala, Automatic Feature Recognition Techniques for the Integration of CAD and CAM: A Review, 2024, 8, 2520-6478, 83, 10.1520/SSMS20230016 |
Input: point clouds of the used part P |
Set the max distance and max angular variation for fitting |
i=1 Remaining points Pm,i←P For the plane fitting, cylinder fitting, cone fitting, sphere fitting, free-form surface fitting: While there are enough points for supporting surface fitting in Pm,i: Parametric surface parameters Si← plane fitting from Pm,i Converting the parametric plane to implicit function: Φi←Si i=i+1 Remove the points Si which fit from remaining points to form new remaining points Pm,i |
Forming the level set function representation from collected implicit functions by Boolean operations: Φu←{Φ1,…,Φi} |
End Output: level set function representation of the used part Φu |
Input: discrete level set function Φn, Φd and Φi; vertical distance d |
Φm←0 with size of Φd |
[x, y, z] = index of Φd>0 in x, y, z directions The position of the tip is obtained from Φn as x_tip, y_tip, z_tip |
For i = 1 to size of x direction: Move on x direction Mx = x (i) - x_tip Move on y direction My = y (i) - y_tip |
Move on z direction Mz = z (i) - z_tip + d |
New position of DED nozzle Φ˜n = translating the Φd over Mx,My,Mz Φm=Φm∪Φ˜n=max(Φm,Φ˜n) End Output: discrete level set function of modified intersection part ˜Φi=Φi∖Φm=min(Φi,−Φm) |
Translation (mm) | Rotation (°) | |||||
tx | ty | tz | θx | θy | θz | |
Optimal variables: | 4.07 | 4.21 | −1.33 | 0 | 0 | 0 |
Manufacturing parameters | Manufacturing resources | ||||
Parameter | Notation | Value | Tool ID | Tool type | Diameter (mm) |
Machine cost /hour | Chh | 100 $/h | T1 | End mill | 20 |
Each tool cost | Ctool | 5 $ | T2 | End mill | 10 |
Density | ρ | 4.43kg/dm3 | T3 | End mill | 5 |
Support structure ratio | ¯ρ/ρ | 0.4 | T4 | Drill | 5 |
Unit price of the metal powder | Cunitmaterial | 450 $/kg | T5 | Drill | 10 |
Re-orientation time | tre | 0.35 h | T6 | DED | - |
Tool change time | ttc | 0.17 h |
Sequence | 1 | 2 | 3 | 4 | 5 | 7 | 8 | 9 | 10 | 11 | 12 |
Features | SF1 | SF2 | AF1 | SF9 | AF2 | SF5 | SF8 | SF3 | SF6 | SF7 | SF4 |
Machine | M1 | M1 | M2 | M1 | M2 | M1 | M1 | M1 | M1 | M1 | M1 |
TAD | + z | + z | + z | + z | + z | + y | + x | + x | + y | + y | + y |
Tool | T1 | T1 | T6 | T2 | T6 | T4 | T4 | T4 | T2 | T3 | T3 |
Input: point clouds of the used part P |
Set the max distance and max angular variation for fitting |
i=1 Remaining points Pm,i←P For the plane fitting, cylinder fitting, cone fitting, sphere fitting, free-form surface fitting: While there are enough points for supporting surface fitting in Pm,i: Parametric surface parameters Si← plane fitting from Pm,i Converting the parametric plane to implicit function: Φi←Si i=i+1 Remove the points Si which fit from remaining points to form new remaining points Pm,i |
Forming the level set function representation from collected implicit functions by Boolean operations: Φu←{Φ1,…,Φi} |
End Output: level set function representation of the used part Φu |
Input: discrete level set function Φn, Φd and Φi; vertical distance d |
Φm←0 with size of Φd |
[x, y, z] = index of Φd>0 in x, y, z directions The position of the tip is obtained from Φn as x_tip, y_tip, z_tip |
For i = 1 to size of x direction: Move on x direction Mx = x (i) - x_tip Move on y direction My = y (i) - y_tip |
Move on z direction Mz = z (i) - z_tip + d |
New position of DED nozzle Φ˜n = translating the Φd over Mx,My,Mz Φm=Φm∪Φ˜n=max(Φm,Φ˜n) End Output: discrete level set function of modified intersection part ˜Φi=Φi∖Φm=min(Φi,−Φm) |
Translation (mm) | Rotation (°) | |||||
tx | ty | tz | θx | θy | θz | |
Optimal variables: | 4.07 | 4.21 | −1.33 | 0 | 0 | 0 |
Manufacturing parameters | Manufacturing resources | ||||
Parameter | Notation | Value | Tool ID | Tool type | Diameter (mm) |
Machine cost /hour | Chh | 100 $/h | T1 | End mill | 20 |
Each tool cost | Ctool | 5 $ | T2 | End mill | 10 |
Density | ρ | 4.43kg/dm3 | T3 | End mill | 5 |
Support structure ratio | ¯ρ/ρ | 0.4 | T4 | Drill | 5 |
Unit price of the metal powder | Cunitmaterial | 450 $/kg | T5 | Drill | 10 |
Re-orientation time | tre | 0.35 h | T6 | DED | - |
Tool change time | ttc | 0.17 h |
Sequence | 1 | 2 | 3 | 4 | 5 | 7 | 8 | 9 | 10 | 11 | 12 |
Features | SF1 | SF2 | AF1 | SF9 | AF2 | SF5 | SF8 | SF3 | SF6 | SF7 | SF4 |
Machine | M1 | M1 | M2 | M1 | M2 | M1 | M1 | M1 | M1 | M1 | M1 |
TAD | + z | + z | + z | + z | + z | + y | + x | + x | + y | + y | + y |
Tool | T1 | T1 | T6 | T2 | T6 | T4 | T4 | T4 | T2 | T3 | T3 |