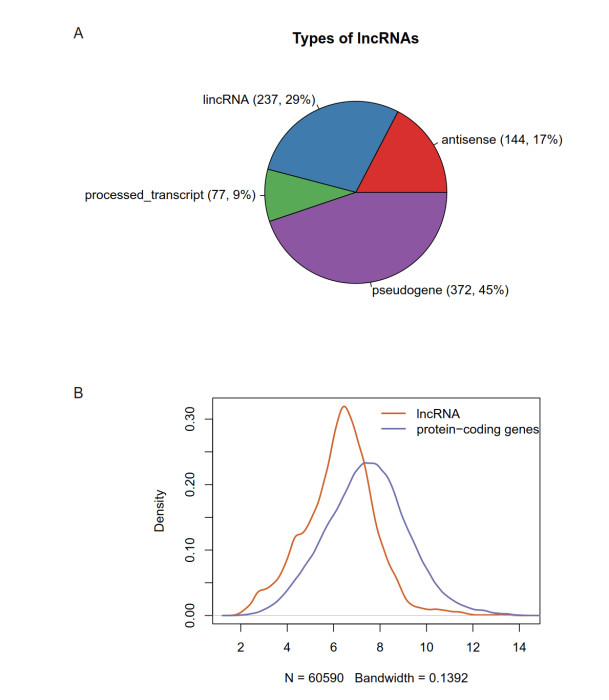
The utilization of intelligent computing in digital teaching quality evaluation has been a practical demand in smart cities. Currently, related research works can be categorized into two types: textual data-based approaches and visual data-based approaches. Due to the gap between their different formats and modalities, it remains very challenging to integrate them together when conducting digital teaching quality evaluation. In fact, the two types of information can both reflect distinguished knowledge from their own perspectives. To bridge this gap, this paper proposes a textual and visual features-jointly driven hybrid intelligent system for digital teaching quality evaluation. Visual features are extracted with the use of a multiscale convolution neural network by introducing receptive fields with different sizes. Textual features serve as the auxiliary contents for major visual features, and are extracted using a recurrent neural network. At last, we implement the proposed method through some simulation experiments to evaluate its practical running performance, and a real-world dataset collected from teaching activities is employed for this purpose. We obtain some groups of experimental results, which reveal that the hybrid intelligent system developed by this paper can bring more than 10% improvement of efficiency towards digital teaching quality evaluation.
Citation: Boyi Zeng, Jun Zhao, Shantian Wen. A textual and visual features-jointly driven hybrid intelligent system for digital physical education teaching quality evaluation[J]. Mathematical Biosciences and Engineering, 2023, 20(8): 13581-13601. doi: 10.3934/mbe.2023606
[1] | Lishui Shen, Xiaofeng Hu, Ting Chen, Guilin Shen, Dong Cheng . Integrated network analysis to explore the key mRNAs and lncRNAs in acute myocardial infarction. Mathematical Biosciences and Engineering, 2019, 16(6): 6426-6437. doi: 10.3934/mbe.2019321 |
[2] | Jie Chen, Jinggui Chen, Bo Sun, Jianghong Wu, Chunyan Du . Integrative analysis of immune microenvironment-related CeRNA regulatory axis in gastric cancer. Mathematical Biosciences and Engineering, 2020, 17(4): 3953-3971. doi: 10.3934/mbe.2020219 |
[3] | Chunxiang Fan, Zouqin Huang, Binbin Chen, Baojin Chen, Qi Wang, Weidong Liu, Donghai Yu . Comprehensive analysis of key lncRNAs in ischemic stroke. Mathematical Biosciences and Engineering, 2020, 17(2): 1318-1328. doi: 10.3934/mbe.2020066 |
[4] | Huili Yang, Wangren Qiu, Zi Liu . Anoikis-related mRNA-lncRNA and DNA methylation profiles for overall survival prediction in breast cancer patients. Mathematical Biosciences and Engineering, 2024, 21(1): 1590-1609. doi: 10.3934/mbe.2024069 |
[5] | Yonghua Xue, Yiqin Ge . Construction of lncRNA regulatory networks reveal the key lncRNAs associated with Pituitary adenomas progression. Mathematical Biosciences and Engineering, 2020, 17(3): 2138-2149. doi: 10.3934/mbe.2020113 |
[6] | Yun-xiang Li, Shi-ming Wang, Chen-quan Li . Four-lncRNA immune prognostic signature for triple-negative breast cancer. Mathematical Biosciences and Engineering, 2021, 18(4): 3939-3956. doi: 10.3934/mbe.2021197 |
[7] | Xudan Ma, Qijun Zhang, Haihong Zhu, Kefeng Huang, Weina Pang, Qin Zhang . Establishment and analysis of the lncRNA-miRNA-mRNA network based on competitive endogenous RNA identifies functional genes in heart failure. Mathematical Biosciences and Engineering, 2021, 18(4): 4011-4026. doi: 10.3934/mbe.2021201 |
[8] | Sisi Qi, Youyu Sheng, Ruiming Hu, Feng Xu, Ying Miao, Jun Zhao, Qinping Yang . Genome-wide expression profiling of long non-coding RNAs and competing endogenous RNA networks in alopecia areata. Mathematical Biosciences and Engineering, 2021, 18(1): 696-711. doi: 10.3934/mbe.2021037 |
[9] | Shuai Miao, Lijun Wang, Siyu Guan, Tianshu Gu, Hualing Wang, Wenfeng Shangguan, Weiding Wang, Yu Liu, Xue Liang . Integrated whole transcriptome analysis for the crucial regulators and functional pathways related to cardiac fibrosis in rats. Mathematical Biosciences and Engineering, 2023, 20(3): 5413-5429. doi: 10.3934/mbe.2023250 |
[10] | Beibei Zhu, Yue Mao, Mei Li . Identification of functional lncRNAs through constructing a lncRNA-associated ceRNA network in myocardial infarction. Mathematical Biosciences and Engineering, 2021, 18(4): 4293-4310. doi: 10.3934/mbe.2021215 |
The utilization of intelligent computing in digital teaching quality evaluation has been a practical demand in smart cities. Currently, related research works can be categorized into two types: textual data-based approaches and visual data-based approaches. Due to the gap between their different formats and modalities, it remains very challenging to integrate them together when conducting digital teaching quality evaluation. In fact, the two types of information can both reflect distinguished knowledge from their own perspectives. To bridge this gap, this paper proposes a textual and visual features-jointly driven hybrid intelligent system for digital teaching quality evaluation. Visual features are extracted with the use of a multiscale convolution neural network by introducing receptive fields with different sizes. Textual features serve as the auxiliary contents for major visual features, and are extracted using a recurrent neural network. At last, we implement the proposed method through some simulation experiments to evaluate its practical running performance, and a real-world dataset collected from teaching activities is employed for this purpose. We obtain some groups of experimental results, which reveal that the hybrid intelligent system developed by this paper can bring more than 10% improvement of efficiency towards digital teaching quality evaluation.
Osteoporosis (OP) is the most common bone disease characterized by a loss of bone mass and quality that results in fragility fractures. Osteoporosis is responsible for over 8.9 million fractures each year worldwide, with most cases occurring in postmenopausal women [1]. The most frequent osteoporotic fractures are fractures of the hip, wrist and spine, although most fractures in the elderly are probably at least partly related to osteoporosis. To prevent the osteoporosis, clinical risk factors [2,3,4,5,6,7], including age, female gender, rheumatoid arthritis, previous fragility fracture, genetic predisposition, smoking, > 14 units of alcohol per week, early-onset menopause (<45 years of age) and low BMI (<19 kg per m2), should be evaluated. To diagnose the osteoporosis, bone mineral density (BMD) measurement is widely used in clinical medicine as an indirect indicator of osteoporosis and fracture risk [8]. Specifically, the BMD measurement can category the subjects into four statuses, including normal, osteopenia, osteoporosis and severe osteoporosis.
Bone remodeling is accomplished by two specialized cells: bone-resorbing osteoclasts and bone-forming osteoblasts [9]. Osteoclasts can break down bone, while osteoblasts create new bone. Osteoclasts and osteoblasts can coordinate well for most of the human life [10]. In a pathological state of osteoporosis, this coordination can break down, and the osteoclasts begin to remove more bone than the osteoblasts can create [11]. To understand the molecular mechanism of osteoporosis, several studies introduced molecules, including SNP [12,13,14,15], DNA methylation [16], RNA [17] and microRNA [18], to investigate the genetic, epigenetic and transcriptomic regulators involved in osteoclastogenesis or osteoblastogenesis.
Osteoclastogenesis has been reported to cause osteoporosis by previous studies [19,20,21], however, the critical functional modules and signaling pathways still need to be explicitly characterized, which is useful for the discovery of the therapeutic targets in osteoclasts for the treatment of skeletal diseases. In this study, we collected gene expression datasets of peripheral blood monocytes (PBMs) from low or high BMD subjects. Notably, PBMs, which are osteoclast progenitor cells and produce cytokines for osteoclastogenesis and bone resorption, could be used for osteoporosis study. Furthermore, network based analysis and overrepresentation enrichment analysis highlighted critical functional modules and signaling pathways, which improved our understanding of the molecular mechanism, and provided the potential drug targets for osteoporosis.
Gene expression datasets were obtained from the NCBI Gene Expression Omnibus (GEO) (http://www.ncbi.nlm.nih.gov/geo) with accession numbers, GSE56814 [22]. Prior to downstream analysis, we firstly mapped the array probes to the GENCODE gene annotation v19. We calculated the average expression values of genes matching multiple probes.
The differential expression analysis was conducted in R programming language (https://www.r-project.org) with student-t test. The differentially expressed genes were identified at the threshold P-value < 0.05. The up-or down-regulation status was determined based on the fold change for each gene.
Overrepresentation enrichment analysis was also implemented at WEB-based Gene Set Analysis Toolkit (WebGestalt) [23]. The Gene Ontology biological processes and KEGG pathways were selected as the functional database.
The Search Tool for the Retrieval of Interacting Genes/ Proteins (STRING) [24] online software (https://string-db.org) was used to assess the interactions. The interactions of the proteins encoded by the differently expressed genes were searched using STRING online software, and the combined score of > 0.4 was used as the cut-off criterion. The PPI network was visualized using Cytoscape software (http://www.cytoscape.org). The Cytoscape MCODE plug-in (version 3.4.0) was applied to search for clustered sub-networks of highly connected nodes from the PPI network. The resulting network was subjected to module analyses with the Plugin MCODE with the following default parameters: Degree cut-off ≥3.
The Pearson correlation coefficient of DEG-lncRNA pairs was calculated according to their expression values. The co-expressed DEG-lncRNA pairs with an absolute value of the Pearson correlation coefficient of ≥0.8 were selected and the co-expression network was visualized by using Cytoscape software.
To identify the probes on the array corresponding to long non-coding RNA (lncRNA) sequences, we re-annotated all probes using GENCODE gene annotation with version 19. Finally, we identified a total of 830 long non-coding RNAs, with gene types such as processed transcript, antisense, long intergenic noncoding RNA (lincRNA) and pseudogene. We found that pseudogene accounted for the most of the identified lncRNAs (45%), followed by the long intergenic noncoding RNAs (29%, Figure 1A). In addition, the reannotation of the probes also mapped 17,051 probes to 17,743 protein-coding genes. We then compared the expression levels of lncRNAs with those of protein-coding genes, and found that expression levels were lower in lncRNAs than protein-coding genes (Figure 1B).
To uncover genes that may be responsible for osteoporosis, we compared the expression profiles of low BMD subjects with that of high BMD subjects. We identified a total of 496 differentially expressed genes (DE-genes), including 120 up-regulated and 376 down-regulated genes in low BMD subjects (t-test, P-value < 0.05, Supplementary Table 1). We observed that four zinc finger genes, ZNF528, ZNF79, ZNF223 and ZNF765, were included in the top-ten up-regulated genes, indicating that zinc finger genes may play key roles in osteoporosis. Notably, 24 lncRNAs were differentially expressed (DE) between samples with low and high BMD, which were presented in Supplementary Table 1. Visualization of the expression levels of DE-genes revealed that a fraction of normal controls (n = 15) exhibited similar expression patterns with the cases with low BMD (Figure 2). Furthermore, a higher proportion of postmenopausal normal controls were observed in the 15 samples than other normal controls (proportion test, P < 0.05, 66.7% vs. 22.2%), suggesting that postmenopausal normal controls had a higher risk of osteoporosis.
ID | gene_type | gene_symbol | status | p-value |
ENSG00000001630.11 | protein_coding | CYP51A1 | Up | 0.011965453 |
ENSG00000005075.11 | protein_coding | POLR2J | Down | 0.018005066 |
ENSG00000006282.15 | protein_coding | SPATA20 | Down | 0.030683456 |
ENSG00000007376.3 | protein_coding | RPUSD1 | Down | 0.046007757 |
ENSG00000007944.10 | protein_coding | MYLIP | Up | 0.012046727 |
ENSG00000008952.12 | protein_coding | SEC62 | Up | 0.04688829 |
ENSG00000014164.6 | protein_coding | ZC3H3 | Down | 0.035615575 |
ENSG00000020256.15 | protein_coding | ZFP64 | Up | 0.035853374 |
ENSG00000025770.14 | protein_coding | NCAPH2 | Down | 0.035381718 |
ENSG00000028277.16 | protein_coding | POU2F2 | Down | 0.025284331 |
ENSG00000029153.10 | protein_coding | ARNTL2 | Down | 0.010597798 |
ENSG00000032444.11 | protein_coding | PNPLA6 | Down | 0.015278704 |
ENSG00000034533.7 | protein_coding | ASTE1 | Up | 0.033013833 |
ENSG00000039123.11 | protein_coding | SKIV2L2 | Down | 0.048932372 |
ENSG00000047056.10 | protein_coding | WDR37 | Down | 0.034858116 |
ENSG00000050344.8 | protein_coding | NFE2L3 | Down | 0.033023849 |
ENSG00000051523.6 | protein_coding | CYBA | Down | 0.007486695 |
ENSG00000052723.7 | protein_coding | SIKE1 | Up | 0.045307581 |
ENSG00000052749.9 | protein_coding | RRP12 | Down | 0.026396768 |
ENSG00000054803.3 | protein_coding | CBLN4 | Down | 0.045582496 |
ENSG00000054967.8 | protein_coding | RELT | Down | 0.02019792 |
ENSG00000062598.13 | protein_coding | ELMO2 | Down | 0.025904437 |
ENSG00000064932.11 | protein_coding | SBNO2 | Down | 0.033420705 |
ENSG00000068024.12 | protein_coding | HDAC4 | Down | 0.048895859 |
ENSG00000068120.10 | protein_coding | COASY | Down | 0.009649617 |
ENSG00000069764.5 | protein_coding | PLA2G10 | Down | 0.003199821 |
ENSG00000070404.5 | protein_coding | FSTL3 | Down | 0.038524776 |
ENSG00000072778.15 | protein_coding | ACADVL | Down | 0.028472324 |
ENSG00000074842.3 | protein_coding | C19orf10 | Down | 0.00105098 |
ENSG00000077150.13 | protein_coding | NFKB2 | Down | 0.003922325 |
ENSG00000078804.8 | protein_coding | TP53INP2 | Down | 0.005193897 |
ENSG00000079393.16 | protein_coding | DUSP13 | Down | 0.024246462 |
ENSG00000079432.3 | protein_coding | CIC | Down | 0.019836428 |
ENSG00000081189.9 | protein_coding | MEF2C | Up | 0.047860138 |
ENSG00000083312.13 | protein_coding | TNPO1 | Up | 0.047418317 |
ENSG00000083844.6 | protein_coding | ZNF264 | Up | 0.010459153 |
ENSG00000087157.14 | protein_coding | PGS1 | Down | 0.042327569 |
ENSG00000087237.6 | protein_coding | CETP | Down | 0.032983061 |
ENSG00000087263.12 | protein_coding | OGFOD1 | Up | 0.043418236 |
ENSG00000087266.11 | protein_coding | SH3BP2 | Down | 0.030356676 |
ENSG00000087903.8 | protein_coding | RFX2 | Down | 0.020836355 |
ENSG00000088888.13 | protein_coding | MAVS | Down | 0.04764586 |
ENSG00000089123.11 | protein_coding | TASP1 | Up | 0.028910399 |
ENSG00000089248.6 | protein_coding | ERP29 | Down | 0.049446948 |
ENSG00000089351.10 | protein_coding | GRAMD1A | Down | 0.025055632 |
ENSG00000089639.6 | protein_coding | GMIP | Down | 0.014587545 |
ENSG00000090924.10 | protein_coding | PLEKHG2 | Down | 0.028301873 |
ENSG00000092929.7 | protein_coding | UNC13D | Down | 0.013293567 |
ENSG00000096088.12 | protein_coding | PGC | Down | 0.042062111 |
ENSG00000099139.9 | protein_coding | PCSK5 | Up | 0.027380288 |
ENSG00000099203.2 | protein_coding | TMED1 | Down | 0.028235039 |
ENSG00000099795.2 | protein_coding | NDUFB7 | Down | 0.040697141 |
ENSG00000099800.3 | protein_coding | TIMM13 | Down | 0.007324296 |
ENSG00000099821.9 | protein_coding | POLRMT | Down | 0.045124992 |
ENSG00000099958.10 | protein_coding | DERL3 | Down | 0.04060876 |
ENSG00000099991.12 | protein_coding | CABIN1 | Down | 0.034876772 |
ENSG00000100028.7 | protein_coding | SNRPD3 | Down | 0.044318218 |
ENSG00000100055.16 | protein_coding | CYTH4 | Down | 0.018939808 |
ENSG00000100092.16 | protein_coding | SH3BP1 | Down | 0.04634582 |
ENSG00000100242.11 | protein_coding | SUN2 | Down | 0.010030177 |
ENSG00000100290.2 | protein_coding | BIK | Down | 0.007141866 |
ENSG00000100403.10 | protein_coding | ZC3H7B | Down | 0.0410179 |
ENSG00000100429.13 | protein_coding | HDAC10 | Down | 0.033877634 |
ENSG00000100532.7 | protein_coding | CGRRF1 | Up | 0.013688977 |
ENSG00000100802.10 | protein_coding | C14orf93 | Down | 0.033127459 |
ENSG00000100906.6 | protein_coding | NFKBIA | Down | 0.005259074 |
ENSG00000101220.13 | protein_coding | C20orf27 | Down | 0.034454114 |
ENSG00000101473.12 | protein_coding | ACOT8 | Down | 0.016141884 |
ENSG00000101624.6 | protein_coding | CEP76 | Up | 0.013187998 |
ENSG00000101665.4 | protein_coding | SMAD7 | Down | 0.017676506 |
ENSG00000101997.8 | protein_coding | CCDC22 | Down | 0.039248809 |
ENSG00000102078.11 | protein_coding | SLC25A14 | Down | 0.039031376 |
ENSG00000102786.10 | protein_coding | INTS6 | Up | 0.044913283 |
ENSG00000102981.5 | protein_coding | PARD6A | Down | 0.041910914 |
ENSG00000103024.3 | protein_coding | NME3 | Down | 0.027773943 |
ENSG00000103047.3 | protein_coding | TANGO6 | Down | 0.049704948 |
ENSG00000103227.14 | protein_coding | LMF1 | Down | 0.022119939 |
ENSG00000103426.8 | protein_coding | CORO7-PAM16 | Down | 0.014976017 |
ENSG00000103449.7 | protein_coding | SALL1 | Down | 0.041904944 |
ENSG00000103653.12 | protein_coding | CSK | Down | 0.010534323 |
ENSG00000104856.9 | protein_coding | RELB | Down | 0.015505238 |
ENSG00000104973.10 | protein_coding | MED25 | Down | 0.041089276 |
ENSG00000105063.14 | protein_coding | PPP6R1 | Down | 0.022586612 |
ENSG00000105135.11 | protein_coding | ILVBL | Down | 0.034386207 |
ENSG00000105497.3 | protein_coding | ZNF175 | Up | 0.013075356 |
ENSG00000105643.5 | protein_coding | ARRDC2 | Down | 0.039560218 |
ENSG00000105656.8 | protein_coding | ELL | Down | 0.035373441 |
ENSG00000106268.11 | protein_coding | NUDT1 | Down | 0.03304039 |
ENSG00000107362.9 | protein_coding | ABHD17B | Up | 0.02410498 |
ENSG00000107443.11 | protein_coding | CCNJ | Down | 0.029817026 |
ENSG00000107984.5 | protein_coding | DKK1 | Down | 0.049671011 |
ENSG00000108179.9 | protein_coding | PPIF | Down | 0.044960461 |
ENSG00000108518.7 | protein_coding | PFN1 | Down | 0.045797043 |
ENSG00000108840.11 | protein_coding | HDAC5 | Down | 0.01595402 |
ENSG00000108950.7 | protein_coding | FAM20A | Down | 0.012800605 |
ENSG00000109103.7 | protein_coding | UNC119 | Down | 0.03809287 |
ENSG00000109736.10 | protein_coding | MFSD10 | Down | 0.043758832 |
ENSG00000109917.6 | protein_coding | ZNF259 | Down | 0.042419417 |
ENSG00000109944.6 | protein_coding | C11orf63 | Down | 0.036421384 |
ENSG00000110063.4 | protein_coding | DCPS | Down | 0.044521345 |
ENSG00000110080.14 | protein_coding | ST3GAL4 | Down | 0.009630116 |
ENSG00000110090.8 | protein_coding | CPT1A | Down | 0.040715917 |
ENSG00000110619.12 | protein_coding | CARS | Down | 0.040080075 |
ENSG00000110931.14 | protein_coding | CAMKK2 | Down | 0.041840274 |
ENSG00000112149.5 | protein_coding | CD83 | Down | 0.021210986 |
ENSG00000112276.9 | protein_coding | BVES | Up | 0.013370759 |
ENSG00000112312.5 | protein_coding | GMNN | Down | 0.047237299 |
ENSG00000114650.14 | protein_coding | SCAP | Down | 0.032838904 |
ENSG00000115073.6 | protein_coding | ACTR1B | Down | 0.003540043 |
ENSG00000116017.6 | protein_coding | ARID3A | Down | 0.038762022 |
ENSG00000116670.10 | protein_coding | MAD2L2 | Down | 0.043537769 |
ENSG00000116852.10 | protein_coding | KIF21B | Down | 0.049989022 |
ENSG00000116871.11 | protein_coding | MAP7D1 | Down | 0.03998345 |
ENSG00000116985.6 | protein_coding | BMP8B | Down | 0.028382633 |
ENSG00000117600.8 | protein_coding | LPPR4 | Down | 0.046876942 |
ENSG00000118432.11 | protein_coding | CNR1 | Down | 0.017637126 |
ENSG00000118600.7 | protein_coding | TMEM5 | Down | 0.014944131 |
ENSG00000119013.4 | protein_coding | NDUFB3 | Down | 0.040614225 |
ENSG00000119121.17 | protein_coding | TRPM6 | Down | 0.048825853 |
ENSG00000119669.3 | protein_coding | IRF2BPL | Down | 0.005359191 |
ENSG00000120217.9 | protein_coding | CD274 | Down | 0.043686299 |
ENSG00000120699.8 | protein_coding | EXOSC8 | Up | 0.032500661 |
ENSG00000122203.10 | protein_coding | KIAA1191 | Up | 0.048031425 |
ENSG00000122490.14 | protein_coding | PQLC1 | Down | 0.015129367 |
ENSG00000122824.6 | protein_coding | NUDT10 | Down | 0.033200739 |
ENSG00000123094.11 | protein_coding | RASSF8 | Down | 0.029465179 |
ENSG00000123358.15 | protein_coding | NR4A1 | Down | 0.01086372 |
ENSG00000123576.5 | protein_coding | ESX1 | Down | 0.030485823 |
ENSG00000123689.5 | protein_coding | G0S2 | Down | 0.009066817 |
ENSG00000123989.9 | protein_coding | CHPF | Down | 0.040386914 |
ENSG00000125657.3 | protein_coding | TNFSF9 | Down | 0.049043875 |
ENSG00000125755.14 | protein_coding | SYMPK | Down | 0.049805392 |
ENSG00000125817.7 | protein_coding | CENPB | Down | 0.042137733 |
ENSG00000125945.10 | protein_coding | ZNF436 | Up | 0.047416168 |
ENSG00000125968.7 | protein_coding | ID1 | Down | 0.04512393 |
ENSG00000127054.14 | protein_coding | CPSF3L | Down | 0.04296422 |
ENSG00000127124.9 | protein_coding | HIVEP3 | Down | 0.000294073 |
ENSG00000127483.13 | protein_coding | HP1BP3 | Up | 0.047813073 |
ENSG00000127540.7 | protein_coding | UQCR11 | Down | 0.030739395 |
ENSG00000127588.4 | protein_coding | GNG13 | Down | 0.039828869 |
ENSG00000128059.4 | protein_coding | PPAT | Up | 0.006604019 |
ENSG00000128228.4 | protein_coding | SDF2L1 | Down | 0.003273018 |
ENSG00000128271.15 | protein_coding | ADORA2A | Down | 0.041530393 |
ENSG00000128309.12 | protein_coding | MPST | Down | 0.037538756 |
ENSG00000128694.7 | protein_coding | OSGEPL1 | Up | 0.036634744 |
ENSG00000129562.6 | protein_coding | DAD1 | Up | 0.039222956 |
ENSG00000129667.8 | protein_coding | RHBDF2 | Down | 0.030796176 |
ENSG00000129744.2 | protein_coding | ART1 | Down | 0.001540155 |
ENSG00000129968.11 | protein_coding | ABHD17A | Down | 0.043478487 |
ENSG00000129993.10 | protein_coding | CBFA2T3 | Down | 0.031277467 |
ENSG00000130522.4 | protein_coding | JUND | Down | 0.022542768 |
ENSG00000130766.4 | protein_coding | SESN2 | Down | 0.013640369 |
ENSG00000130935.5 | protein_coding | NOL11 | Up | 0.044393023 |
ENSG00000131142.9 | protein_coding | CCL25 | Down | 0.038185878 |
ENSG00000131171.8 | protein_coding | SH3BGRL | Up | 0.022819598 |
ENSG00000131653.8 | protein_coding | TRAF7 | Down | 0.036084131 |
ENSG00000131849.10 | protein_coding | ZNF132 | Down | 0.028132404 |
ENSG00000132275.6 | protein_coding | RRP8 | Down | 0.010570164 |
ENSG00000132507.13 | protein_coding | EIF5A | Down | 0.024112694 |
ENSG00000133606.6 | protein_coding | MKRN1 | Up | 0.041317442 |
ENSG00000133805.11 | protein_coding | AMPD3 | Down | 0.046431783 |
ENSG00000134107.4 | protein_coding | BHLHE40 | Down | 0.038744934 |
ENSG00000135047.10 | protein_coding | CTSL | Down | 0.0255056 |
ENSG00000135390.13 | protein_coding | ATP5G2 | Down | 0.02929579 |
ENSG00000136048.9 | protein_coding | DRAM1 | Down | 0.02091681 |
ENSG00000136122.11 | protein_coding | BORA | Up | 0.036657962 |
ENSG00000136738.10 | protein_coding | STAM | Up | 0.0389495 |
ENSG00000136840.14 | protein_coding | ST6GALNAC4 | Down | 0.016088565 |
ENSG00000136877.10 | protein_coding | FPGS | Down | 0.023271049 |
ENSG00000137124.6 | protein_coding | ALDH1B1 | Up | 0.03438067 |
ENSG00000137193.9 | protein_coding | PIM1 | Down | 0.009876567 |
ENSG00000137218.6 | protein_coding | FRS3 | Down | 0.042976435 |
ENSG00000137309.15 | protein_coding | HMGA1 | Down | 0.021493168 |
ENSG00000137312.10 | protein_coding | FLOT1 | Down | 0.039597455 |
ENSG00000137409.14 | protein_coding | MTCH1 | Down | 0.040766375 |
ENSG00000138382.9 | protein_coding | METTL5 | Up | 0.038252044 |
ENSG00000139053.2 | protein_coding | PDE6H | Down | 0.0207692 |
ENSG00000139610.1 | protein_coding | CELA1 | Up | 0.038317749 |
ENSG00000139668.7 | protein_coding | WDFY2 | Down | 0.019753557 |
ENSG00000140044.8 | protein_coding | JDP2 | Down | 0.036877537 |
ENSG00000140553.12 | protein_coding | UNC45A | Down | 0.042323486 |
ENSG00000140612.9 | protein_coding | SEC11A | Down | 0.017070625 |
ENSG00000140848.12 | protein_coding | CPNE2 | Down | 0.035194471 |
ENSG00000140941.8 | protein_coding | MAP1LC3B | Up | 0.010046471 |
ENSG00000141012.8 | protein_coding | GALNS | Down | 0.019933327 |
ENSG00000141034.5 | protein_coding | GID4 | Up | 0.036023887 |
ENSG00000141198.9 | protein_coding | TOM1L1 | Down | 0.043796522 |
ENSG00000141252.15 | protein_coding | VPS53 | Down | 0.020821593 |
ENSG00000141505.7 | protein_coding | ASGR1 | Down | 0.049034425 |
ENSG00000141646.9 | protein_coding | SMAD4 | Up | 0.041545876 |
ENSG00000141867.13 | protein_coding | BRD4 | Down | 0.040247622 |
ENSG00000142408.2 | protein_coding | CACNG8 | Down | 0.035337949 |
ENSG00000142538.1 | protein_coding | PTH2 | Down | 0.028959056 |
ENSG00000143549.15 | protein_coding | TPM3 | Down | 0.005503969 |
ENSG00000144120.8 | protein_coding | TMEM177 | Up | 0.022405325 |
ENSG00000144182.12 | protein_coding | LIPT1 | Up | 0.008805655 |
ENSG00000144381.12 | protein_coding | HSPD1 | Down | 0.048940793 |
ENSG00000144468.12 | protein_coding | RHBDD1 | Up | 0.048354944 |
ENSG00000144655.10 | protein_coding | CSRNP1 | Down | 0.03240296 |
ENSG00000144791.5 | protein_coding | LIMD1 | Down | 0.04142983 |
ENSG00000144802.7 | protein_coding | NFKBIZ | Down | 0.015266617 |
ENSG00000145901.10 | protein_coding | TNIP1 | Down | 0.031453499 |
ENSG00000146047.4 | protein_coding | HIST1H2BA | Down | 0.005162611 |
ENSG00000146232.10 | protein_coding | NFKBIE | Down | 0.020469032 |
ENSG00000146828.13 | protein_coding | SLC12A9 | Down | 0.042442305 |
ENSG00000146833.11 | protein_coding | TRIM4 | Up | 0.001031314 |
ENSG00000146856.10 | protein_coding | AGBL3 | Down | 0.044096515 |
ENSG00000147419.12 | protein_coding | CCDC25 | Up | 0.027233589 |
ENSG00000148187.13 | protein_coding | MRRF | Up | 0.01456252 |
ENSG00000148335.10 | protein_coding | NTMT1 | Down | 0.033476509 |
ENSG00000148602.5 | protein_coding | LRIT1 | Down | 0.038378276 |
ENSG00000148737.11 | protein_coding | TCF7L2 | Down | 0.03027628 |
ENSG00000148835.9 | protein_coding | TAF5 | Up | 0.017608599 |
ENSG00000149115.9 | protein_coding | TNKS1BP1 | Down | 0.031874191 |
ENSG00000149503.8 | protein_coding | INCENP | Down | 0.039618744 |
ENSG00000149792.4 | protein_coding | MRPL49 | Down | 0.014313227 |
ENSG00000149823.3 | protein_coding | VPS51 | Down | 0.034228234 |
ENSG00000149932.12 | protein_coding | TMEM219 | Down | 0.042059762 |
ENSG00000149968.7 | protein_coding | MMP3 | Down | 0.007039867 |
ENSG00000151093.3 | protein_coding | OXSM | Up | 0.032113374 |
ENSG00000151490.9 | protein_coding | PTPRO | Down | 0.0169847 |
ENSG00000151631.7 | pseudogene | AKR1C6P | Down | 0.004333285 |
ENSG00000151651.11 | protein_coding | ADAM8 | Down | 0.034809828 |
ENSG00000151726.9 | protein_coding | ACSL1 | Down | 0.040278414 |
ENSG00000152207.3 | protein_coding | CYSLTR2 | Up | 0.031075114 |
ENSG00000152382.5 | protein_coding | TADA1 | Down | 0.034551617 |
ENSG00000153140.4 | protein_coding | CETN3 | Up | 0.028301839 |
ENSG00000153207.10 | protein_coding | AHCTF1 | Down | 0.014567315 |
ENSG00000153266.8 | protein_coding | FEZF2 | Down | 0.036977261 |
ENSG00000153487.11 | protein_coding | ING1 | Down | 0.015188879 |
ENSG00000154370.9 | protein_coding | TRIM11 | Down | 0.018777551 |
ENSG00000154447.10 | protein_coding | SH3RF1 | Down | 0.009674391 |
ENSG00000154639.14 | protein_coding | CXADR | Down | 0.00685789 |
ENSG00000154781.11 | protein_coding | CCDC174 | Down | 0.049642675 |
ENSG00000154839.5 | protein_coding | SKA1 | Down | 0.046702852 |
ENSG00000154978.8 | protein_coding | VOPP1 | Down | 0.030307149 |
ENSG00000155438.7 | protein_coding | MKI67IP | Down | 0.03414805 |
ENSG00000155918.3 | protein_coding | RAET1L | Down | 0.047085308 |
ENSG00000156172.5 | protein_coding | C8orf37 | Up | 0.015241022 |
ENSG00000156508.13 | protein_coding | EEF1A1 | Down | 0.029958858 |
ENSG00000157227.8 | protein_coding | MMP14 | Down | 0.018623306 |
ENSG00000157326.14 | protein_coding | DHRS4 | Down | 0.047099568 |
ENSG00000157456.3 | protein_coding | CCNB2 | Down | 0.012184904 |
ENSG00000157637.8 | protein_coding | SLC38A10 | Down | 0.026642558 |
ENSG00000158014.10 | protein_coding | SLC30A2 | Down | 0.026315721 |
ENSG00000158483.11 | protein_coding | FAM86C1 | Down | 0.00975309 |
ENSG00000158497.2 | protein_coding | HMHB1 | Down | 0.040327646 |
ENSG00000158555.10 | protein_coding | GDPD5 | Down | 0.034837228 |
ENSG00000159069.9 | protein_coding | FBXW5 | Down | 0.01907369 |
ENSG00000159885.9 | protein_coding | ZNF222 | Up | 0.01199585 |
ENSG00000160111.8 | protein_coding | CPAMD8 | Down | 0.049299496 |
ENSG00000160179.14 | protein_coding | ABCG1 | Up | 0.022299138 |
ENSG00000160180.14 | protein_coding | TFF3 | Down | 0.042914253 |
ENSG00000160214.8 | protein_coding | RRP1 | Down | 0.049738569 |
ENSG00000160796.12 | protein_coding | NBEAL2 | Down | 0.035908182 |
ENSG00000160883.6 | protein_coding | HK3 | Down | 0.044846812 |
ENSG00000160888.6 | protein_coding | IER2 | Down | 0.027407148 |
ENSG00000161640.11 | protein_coding | SIGLEC11 | Down | 0.042121328 |
ENSG00000161791.9 | protein_coding | FMNL3 | Down | 0.011008036 |
ENSG00000162086.10 | protein_coding | ZNF75A | Up | 0.019226079 |
ENSG00000162302.8 | protein_coding | RPS6KA4 | Down | 0.048212217 |
ENSG00000162377.4 | protein_coding | SELRC1 | Up | 0.047979072 |
ENSG00000162413.12 | protein_coding | KLHL21 | Down | 0.048454407 |
ENSG00000162522.6 | protein_coding | KIAA1522 | Down | 0.016306649 |
ENSG00000162783.8 | protein_coding | IER5 | Down | 0.021919966 |
ENSG00000162913.9 | protein_coding | C1orf145 | Down | 0.018777551 |
ENSG00000162927.9 | protein_coding | PUS10 | Up | 0.04033551 |
ENSG00000162931.7 | protein_coding | TRIM17 | Down | 0.023650474 |
ENSG00000163162.4 | protein_coding | RNF149 | Down | 0.025666014 |
ENSG00000163239.8 | protein_coding | TDRD10 | Down | 0.043592404 |
ENSG00000163389.6 | protein_coding | POGLUT1 | Up | 0.022081661 |
ENSG00000163947.7 | protein_coding | ARHGEF3 | Up | 0.010264753 |
ENSG00000164105.3 | protein_coding | SAP30 | Up | 0.046694723 |
ENSG00000164342.8 | protein_coding | TLR3 | Up | 0.003277358 |
ENSG00000164402.9 | protein_coding | 8-Sep | Down | 0.024177765 |
ENSG00000164411.6 | protein_coding | GJB7 | Down | 0.026747052 |
ENSG00000165029.11 | protein_coding | ABCA1 | Up | 0.010492577 |
ENSG00000165188.9 | protein_coding | RNF183 | Down | 0.010089847 |
ENSG00000165406.11 | protein_coding | 8-Mar | Up | 0.047180108 |
ENSG00000165512.4 | protein_coding | ZNF22 | Up | 0.033208827 |
ENSG00000165782.6 | protein_coding | TMEM55B | Down | 0.022652362 |
ENSG00000165886.4 | protein_coding | UBTD1 | Down | 0.014531961 |
ENSG00000165915.9 | protein_coding | SLC39A13 | Down | 0.024410393 |
ENSG00000166265.7 | protein_coding | CYYR1 | Down | 0.037657919 |
ENSG00000166363.4 | protein_coding | OR10A5 | Up | 0.018183215 |
ENSG00000166446.10 | protein_coding | CDYL2 | Down | 0.011853205 |
ENSG00000166451.9 | protein_coding | CENPN | Up | 0.040214671 |
ENSG00000166452.7 | protein_coding | AKIP1 | Up | 0.048924644 |
ENSG00000166938.8 | protein_coding | DIS3L | Up | 0.036638539 |
ENSG00000166974.8 | protein_coding | MAPRE2 | Up | 0.048488455 |
ENSG00000167110.12 | protein_coding | GOLGA2 | Down | 0.023994018 |
ENSG00000167186.6 | protein_coding | COQ7 | Up | 0.000296612 |
ENSG00000167333.8 | protein_coding | TRIM68 | Up | 0.039246931 |
ENSG00000167394.8 | protein_coding | ZNF668 | Down | 0.015855291 |
ENSG00000167414.4 | protein_coding | GNG8 | Down | 0.0489265 |
ENSG00000167555.9 | protein_coding | ZNF528 | Up | 0.002098542 |
ENSG00000167604.9 | protein_coding | NFKBID | Down | 0.04908962 |
ENSG00000167657.7 | protein_coding | DAPK3 | Down | 0.045503987 |
ENSG00000168066.16 | protein_coding | SF1 | Down | 0.047265519 |
ENSG00000168310.6 | protein_coding | IRF2 | Up | 0.044253405 |
ENSG00000168594.11 | protein_coding | ADAM29 | Down | 0.003444856 |
ENSG00000168803.10 | protein_coding | ADAL | Up | 0.021101681 |
ENSG00000168818.5 | protein_coding | STX18 | Up | 0.022001045 |
ENSG00000168967.10 | pseudogene | PMCHL1 | Down | 0.008435479 |
ENSG00000169105.6 | protein_coding | CHST14 | Down | 0.024636798 |
ENSG00000169379.11 | protein_coding | ARL13B | Up | 0.010209654 |
ENSG00000169393.4 | protein_coding | ELSPBP1 | Down | 0.014495593 |
ENSG00000169429.6 | protein_coding | IL8 | Down | 0.028420528 |
ENSG00000170265.7 | protein_coding | ZNF282 | Down | 0.042671468 |
ENSG00000170369.3 | protein_coding | CST2 | Down | 0.041604924 |
ENSG00000170445.8 | protein_coding | HARS | Down | 0.029725289 |
ENSG00000170779.10 | protein_coding | CDCA4 | Down | 0.028547735 |
ENSG00000170837.2 | protein_coding | GPR27 | Down | 0.035077639 |
ENSG00000170891.6 | protein_coding | CYTL1 | Down | 0.032834474 |
ENSG00000171130.13 | protein_coding | ATP6V0E2 | Down | 0.039784296 |
ENSG00000171223.4 | protein_coding | JUNB | Down | 0.038177458 |
ENSG00000171357.5 | protein_coding | LURAP1 | Down | 0.033100787 |
ENSG00000171467.11 | protein_coding | ZNF318 | Down | 0.044327394 |
ENSG00000171530.9 | protein_coding | TBCA | Down | 0.009876223 |
ENSG00000171570.6 | protein_coding | RAB4B-EGLN2 | Down | 0.010162577 |
ENSG00000171657.5 | protein_coding | GPR82 | Up | 0.002688119 |
ENSG00000171766.11 | protein_coding | GATM | Up | 0.035375608 |
ENSG00000171827.6 | protein_coding | ZNF570 | Up | 0.016772935 |
ENSG00000171861.6 | protein_coding | RNMTL1 | Down | 0.041110394 |
ENSG00000171942.3 | protein_coding | OR10H2 | Down | 0.01466908 |
ENSG00000172208.3 | protein_coding | OR4X2 | Up | 0.038143037 |
ENSG00000172322.9 | protein_coding | CLEC12A | Up | 0.008850366 |
ENSG00000172375.8 | protein_coding | C2CD2L | Down | 0.012079432 |
ENSG00000172428.6 | protein_coding | MYEOV2 | Down | 0.025783713 |
ENSG00000173020.6 | protein_coding | ADRBK1 | Down | 0.043055525 |
ENSG00000173153.9 | protein_coding | ESRRA | Down | 0.008541886 |
ENSG00000173653.3 | protein_coding | RCE1 | Down | 0.030649495 |
ENSG00000173715.11 | protein_coding | C11orf80 | Down | 0.030649495 |
ENSG00000173812.6 | protein_coding | EIF1 | Down | 0.0170157 |
ENSG00000173846.8 | protein_coding | PLK3 | Down | 0.015509349 |
ENSG00000174600.9 | protein_coding | CMKLR1 | Up | 0.044214317 |
ENSG00000174886.8 | protein_coding | NDUFA11 | Down | 0.022192837 |
ENSG00000175130.6 | protein_coding | MARCKSL1 | Down | 0.038863502 |
ENSG00000175728.2 | protein_coding | C11orf44 | Up | 0.045296195 |
ENSG00000175874.5 | protein_coding | CREG2 | Down | 0.01537001 |
ENSG00000176485.6 | protein_coding | PLA2G16 | Down | 0.024862828 |
ENSG00000176563.5 | protein_coding | CNTD1 | Down | 0.016145384 |
ENSG00000176619.6 | protein_coding | LMNB2 | Down | 0.030268302 |
ENSG00000176624.9 | protein_coding | MEX3C | Up | 0.029845716 |
ENSG00000176714.9 | protein_coding | CCDC121 | Down | 0.022706601 |
ENSG00000176749.4 | protein_coding | CDK5R1 | Down | 0.04550598 |
ENSG00000176845.8 | protein_coding | METRNL | Down | 0.010677297 |
ENSG00000176894.5 | protein_coding | PXMP2 | Down | 0.013611729 |
ENSG00000176974.13 | protein_coding | SHMT1 | Up | 0.049830843 |
ENSG00000177051.5 | protein_coding | FBXO46 | Down | 0.047542187 |
ENSG00000177169.5 | protein_coding | ULK1 | Down | 0.016389135 |
ENSG00000177666.11 | protein_coding | PNPLA2 | Down | 0.043015592 |
ENSG00000178229.7 | protein_coding | ZNF543 | Up | 0.010459153 |
ENSG00000178951.4 | protein_coding | ZBTB7A | Down | 0.019056001 |
ENSG00000178980.10 | protein_coding | SEPW1 | Down | 0.016556027 |
ENSG00000179029.10 | protein_coding | TMEM107 | Down | 0.005945219 |
ENSG00000180008.8 | protein_coding | SOCS4 | Up | 0.039599073 |
ENSG00000180332.5 | protein_coding | KCTD4 | Down | 0.022125509 |
ENSG00000180346.2 | protein_coding | TIGD2 | Up | 0.037693259 |
ENSG00000180539.4 | protein_coding | C9orf139 | Down | 0.01622825 |
ENSG00000180725.4 | pseudogene | AC015871.1 | Down | 0.042511409 |
ENSG00000181378.9 | protein_coding | CCDC108 | Down | 0.038235948 |
ENSG00000181791.1 | pseudogene | AC009041.1 | Down | 0.0167024 |
ENSG00000182118.5 | protein_coding | FAM89A | Down | 0.002426708 |
ENSG00000182333.10 | protein_coding | LIPF | Down | 0.018324226 |
ENSG00000182368.4 | protein_coding | FAM27A | Down | 0.03035551 |
ENSG00000182541.13 | protein_coding | LIMK2 | Down | 0.041747303 |
ENSG00000182782.7 | protein_coding | HCAR2 | Down | 0.046508935 |
ENSG00000182841.8 | pseudogene | RRP7B | Down | 0.04819365 |
ENSG00000183019.3 | protein_coding | C19orf59 | Down | 0.031283921 |
ENSG00000183020.9 | protein_coding | AP2A2 | Down | 0.000692239 |
ENSG00000183087.10 | protein_coding | GAS6 | Down | 0.026705611 |
ENSG00000183648.5 | protein_coding | NDUFB1 | Down | 0.00863385 |
ENSG00000183696.9 | protein_coding | UPP1 | Down | 0.021571741 |
ENSG00000183709.7 | protein_coding | IFNL2 | Up | 0.001410201 |
ENSG00000183723.8 | protein_coding | CMTM4 | Down | 0.047938821 |
ENSG00000183779.5 | protein_coding | ZNF703 | Down | 0.033061055 |
ENSG00000184110.10 | protein_coding | EIF3C | Down | 0.008205594 |
ENSG00000184517.7 | protein_coding | ZFP1 | Up | 0.045781579 |
ENSG00000184619.3 | protein_coding | KRBA2 | Down | 0.025225822 |
ENSG00000184897.4 | protein_coding | H1FX | Down | 0.014748614 |
ENSG00000184922.9 | protein_coding | FMNL1 | Down | 0.01035533 |
ENSG00000184990.8 | protein_coding | SIVA1 | Down | 0.039061925 |
ENSG00000185641.5 | pseudogene | CTD-2287O16.1 | Down | 0.043078899 |
ENSG00000185787.10 | protein_coding | MORF4L1 | Down | 0.047667026 |
ENSG00000185972.4 | protein_coding | CCIN | Down | 0.044995407 |
ENSG00000186106.7 | protein_coding | ANKRD46 | Up | 0.042420836 |
ENSG00000186111.4 | protein_coding | PIP5K1C | Down | 0.042436011 |
ENSG00000186281.8 | protein_coding | GPAT2 | Down | 0.049178673 |
ENSG00000186594.8 | lincRNA | MIR22HG | Down | 0.017511035 |
ENSG00000186635.10 | protein_coding | ARAP1 | Down | 0.015438232 |
ENSG00000186795.1 | protein_coding | KCNK18 | Down | 0.020856507 |
ENSG00000187630.10 | protein_coding | DHRS4L2 | Down | 0.047099568 |
ENSG00000187688.10 | protein_coding | TRPV2 | Down | 0.003137583 |
ENSG00000188379.5 | protein_coding | IFNA2 | Down | 0.004976237 |
ENSG00000189042.9 | protein_coding | ZNF567 | Up | 0.015774858 |
ENSG00000189114.6 | protein_coding | BLOC1S3 | Down | 0.028793638 |
ENSG00000189332.4 | protein_coding | RP11-113D6.10 | Down | 0.029127001 |
ENSG00000196152.6 | protein_coding | ZNF79 | Up | 0.002879344 |
ENSG00000196331.5 | protein_coding | HIST1H2BO | Down | 0.025404112 |
ENSG00000196358.6 | protein_coding | NTNG2 | Down | 0.042750195 |
ENSG00000196371.2 | protein_coding | FUT4 | Down | 0.011093343 |
ENSG00000196415.5 | protein_coding | PRTN3 | Down | 0.035149627 |
ENSG00000196417.8 | protein_coding | ZNF765 | Up | 0.005727824 |
ENSG00000196652.7 | protein_coding | ZKSCAN5 | Up | 0.016188909 |
ENSG00000196668.3 | processed_transcript | LINC00173 | Down | 0.003213639 |
ENSG00000196693.10 | protein_coding | ZNF33B | Up | 0.010400288 |
ENSG00000196843.11 | protein_coding | ARID5A | Down | 0.023473729 |
ENSG00000196917.4 | protein_coding | HCAR1 | Down | 0.023288331 |
ENSG00000196981.2 | protein_coding | WDR5B | Up | 0.010645113 |
ENSG00000197016.7 | protein_coding | ZNF470 | Up | 0.021502777 |
ENSG00000197312.7 | protein_coding | DDI2 | Up | 0.049559262 |
ENSG00000197321.10 | protein_coding | SVIL | Down | 0.029323831 |
ENSG00000197919.3 | protein_coding | IFNA1 | Down | 0.011997429 |
ENSG00000198055.6 | protein_coding | GRK6 | Down | 0.018617852 |
ENSG00000198081.6 | protein_coding | ZBTB14 | Up | 0.03828373 |
ENSG00000198324.10 | protein_coding | FAM109A | Down | 0.015387399 |
ENSG00000198445.3 | protein_coding | CCT8L2 | Down | 0.008263414 |
ENSG00000198464.9 | protein_coding | ZNF480 | Up | 0.04695864 |
ENSG00000198471.1 | protein_coding | RTP2 | Up | 0.026862674 |
ENSG00000198551.5 | protein_coding | ZNF627 | Up | 0.007529781 |
ENSG00000198754.5 | protein_coding | OXCT2 | Down | 0.028382633 |
ENSG00000198830.6 | protein_coding | HMGN2 | Down | 0.034053765 |
ENSG00000198881.8 | protein_coding | ASB12 | Down | 0.042963309 |
ENSG00000198900.5 | protein_coding | TOP1 | Up | 0.036803174 |
ENSG00000198914.2 | protein_coding | POU3F3 | Down | 0.040875916 |
ENSG00000198945.3 | protein_coding | L3MBTL3 | Up | 0.040353636 |
ENSG00000198954.4 | protein_coding | KIAA1279 | Up | 0.014789739 |
ENSG00000198967.3 | protein_coding | OR10Z1 | Down | 0.02892736 |
ENSG00000203326.5 | protein_coding | ZNF525 | Up | 0.019737784 |
ENSG00000203684.5 | protein_coding | IBA57-AS1 | Down | 0.018777551 |
ENSG00000204807.1 | protein_coding | FAM27E2 | Down | 0.03035551 |
ENSG00000204983.8 | protein_coding | PRSS1 | Down | 0.022866585 |
ENSG00000205022.5 | protein_coding | PABPN1L | Down | 0.031277467 |
ENSG00000205409.3 | protein_coding | OR52E6 | Up | 0.039983429 |
ENSG00000212916.3 | protein_coding | MAP10 | Down | 0.04462334 |
ENSG00000213799.6 | protein_coding | ZNF845 | Up | 0.019737784 |
ENSG00000213828.1 | protein_coding | AC017028.1 | Down | 0.026268434 |
ENSG00000213888.2 | protein_coding | AC005003.1 | Down | 0.030588957 |
ENSG00000214253.4 | protein_coding | FIS1 | Down | 0.036530674 |
ENSG00000215612.5 | protein_coding | HMX1 | Down | 0.030423062 |
ENSG00000215695.1 | protein_coding | RSC1A1 | Up | 0.049559262 |
ENSG00000217930.3 | protein_coding | PAM16 | Down | 0.014976017 |
ENSG00000220848.4 | pseudogene | RPS18P9 | Down | 0.024449402 |
ENSG00000221837.4 | protein_coding | KRTAP10-9 | Down | 0.028181439 |
ENSG00000221840.2 | protein_coding | OR4A5 | Down | 0.013014151 |
ENSG00000221949.2 | protein_coding | C12orf61 | Down | 0.031578862 |
ENSG00000221954.2 | protein_coding | OR4C12 | Down | 0.010582213 |
ENSG00000224474.2 | protein_coding | AL355490.1 | Down | 0.026396768 |
ENSG00000228300.9 | protein_coding | C19orf24 | Down | 0.046090199 |
ENSG00000228336.1 | pseudogene | OR9H1P | Down | 0.031871552 |
ENSG00000230257.1 | antisense | NFE4 | Down | 0.021397338 |
ENSG00000232973.7 | antisense | CYP1B1-AS1 | Up | 0.031113526 |
ENSG00000233016.2 | antisense | SNHG7 | Down | 0.048765474 |
ENSG00000236773.1 | pseudogene | RP11-365O16.1 | Up | 0.015477893 |
ENSG00000237541.3 | protein_coding | HLA-DQA2 | Up | 0.020285026 |
ENSG00000238184.1 | processed_transcript | AC129929.5 | Down | 0.040824827 |
ENSG00000240720.3 | protein_coding | LRRD1 | Up | 0.006393288 |
ENSG00000240970.1 | pseudogene | RPL23AP64 | Down | 0.000986343 |
ENSG00000241360.1 | protein_coding | PDXP | Down | 0.04634582 |
ENSG00000244623.1 | protein_coding | OR2AE1 | Up | 0.001031314 |
ENSG00000245680.5 | protein_coding | ZNF585B | Up | 0.029339876 |
ENSG00000247077.2 | protein_coding | PGAM5 | Down | 0.03099048 |
ENSG00000251357.4 | protein_coding | AP000350.10 | Down | 0.044557673 |
ENSG00000254521.2 | protein_coding | SIGLEC12 | Up | 0.008757157 |
ENSG00000255769.3 | pseudogene | RP11-152F13.3 | Down | 0.031901455 |
ENSG00000255804.1 | protein_coding | OR6J1 | Up | 0.039222956 |
ENSG00000256235.1 | protein_coding | SMIM3 | Down | 0.036368455 |
ENSG00000256294.3 | protein_coding | ZNF225 | Up | 0.046416681 |
ENSG00000256453.1 | protein_coding | DND1 | Down | 0.023504581 |
ENSG00000256632.3 | protein_coding | RP13-672B3.2 | Down | 0.013611729 |
ENSG00000256683.2 | protein_coding | ZNF350 | Up | 0.021879236 |
ENSG00000257341.1 | protein_coding | CRIP1 | Down | 0.049720545 |
ENSG00000257702.3 | antisense | LBX2-AS1 | Down | 0.035045107 |
ENSG00000258405.5 | protein_coding | ZNF578 | Down | 0.021484975 |
ENSG00000258472.4 | protein_coding | RP11-192H23.4 | Down | 0.047286987 |
ENSG00000259571.1 | protein_coding | BLID | Down | 0.02508268 |
ENSG00000261713.2 | processed_transcript | SSTR5-AS1 | Down | 0.0167024 |
ENSG00000263002.3 | protein_coding | ZNF234 | Up | 0.035012293 |
ENSG00000263620.1 | protein_coding | RP11-599B13.6 | Down | 0.04868739 |
ENSG00000263809.1 | protein_coding | RP11-849F2.7 | Down | 0.025225822 |
ENSG00000264735.1 | lincRNA | RP11-498C9.17 | Down | 0.038254904 |
ENSG00000267022.1 | protein_coding | ZNF223 | Up | 0.005281393 |
ENSG00000267059.2 | protein_coding | UQCR11 | Down | 0.01521352 |
ENSG00000267360.2 | protein_coding | CTC-454I21.3 | Up | 0.036745114 |
ENSG00000267545.1 | protein_coding | AC005779.2 | Down | 0.028793638 |
ENSG00000267699.2 | protein_coding | RP11-729L2.2 | Up | 0.041545876 |
ENSG00000268614.1 | protein_coding | CTD-2207O23.10 | Down | 0.021055407 |
ENSG00000268797.1 | protein_coding | CTC-490E21.12 | Down | 0.003100426 |
ENSG00000269220.1 | lincRNA | LINC00528 | Down | 0.026695365 |
ENSG00000269636.1 | protein_coding | AC010441.1 | Down | 0.036368455 |
ENSG00000269858.1 | protein_coding | EGLN2 | Down | 0.003100426 |
ENSG00000271810.1 | protein_coding | RP11-426L16.10 | Down | 0.02868524 |
ENSG00000271959.1 | antisense | CTD-3064M3.7 | Down | 0.036335334 |
ENSG00000272906.1 | lincRNA | RP11-533E19.7 | Down | 0.011437491 |
ENSG00000273006.1 | lincRNA | RP11-314C9.2 | Up | 0.031113526 |
Next, the differentially expressed mRNAs were subjected to GO and KEGG analyses (Figure 2). GO analysis indicated that the upregulated genes were mainly involved in regulating nucleobase metabolic process, regulation of organelle assembly, regulation of plasma lipoprotein particle levels, response to interferon-gamma, snRNA metabolic process, ncRNA transcription and ncRNA processing (Figure 3A). Furthermore, the downregulated genes were mainly enriched in categories associated with regulation of sequence-specific DNA binding transcription factor activity, neutral lipid metabolic process, cytokinesis, nucleoside triphosphate metabolic process, regulation of cell-cell adhesion, nucleoside monophosphate metabolic process, and glycoprotein metabolic process (Figure 3B). The above pathways may therefore participate in regulating the bone miner density.
KEGG pathway analysis revealed that upregulated genes were primarily enriched in pathways associated with the glycine, serine and threonine metabolism, ABC transporters, arginine and proline metabolism, Jak-STAT signaling pathway, RNA degradation, and herpes simplex infection (Figure 3C). In accordance with the enriched GO terms, the up-regulated genes were significantly enriched in inflammation-related pathways. Downregulated genes were mainly associated with adipocytokine signaling pathway, metabolic pathways, oxidative phosphorylation, cytosolic DNA-sensing pathway, and glycerophospholipid metabolism (Figure 3D). The GO and KEGG enrichment analysis of the down-regulated genes suggested that metabolic pathways above may function by maintaining the bone miner density.
To investigate the interactions between the proteins encoded by the DEGs, we mapped the DEGs to protein-protein interaction network (Figure 4). The PPI network for the upregulated genes contained 38 nodes and 33 edges, and the hub nodes with the higher connectivity degree were SHMT1, MYLIP, MKRN1 and EXOSC8 (connectivity degree = 4, Figure 4A). The PPI network for the downregulated genes contained 198 nodes and 348 edges, and the hub nodes with the higher connectivity degree were IL8 (connectivity degree = 28), NFKBIA (connectivity degree = 13), HADC5 (connectivity degree = 12) and HDAC4 (connectivity degree = 12) (Figure 4B).
Furthermore, we also performed a module analysis of the network using MCODE plugin. With degree cut-off ≥3, four up-regulated proteins, including MEX3C, MKRN1, TRIM4 and MYLIP, were identified as key proteins/genes of a module in the up-regulated PPI network (Figure 5A). The high connectivity and components of a module suggested that MKRN1 and MYLIP may play important roles in regulating bone miner density. Similarly, the down-regulated proteins, including JDP2, HADC4, HDAC5, CDYL2, ACADVL, ACSL1 and BRD4, were identified as key proteins/genes in the down-regulated PPI network (Figure 5B). Moreover, IL8, CCL25, CNR1, HCAR2, HCAR1, GNG13 and GNG8 were also identified as a module in the down-regulated PPI network (Figure 5C). All these proteins except IL8 participated in G-alpha (ⅰ) signaling events, indicating that this pathway may be important for bone mineral homeostasis.
To predict the potential functional roles of the differentially expressed lncRNAs, the Pearson correlation coefficient for lncRNA-DE-mRNA pairs was first calculated according to their expression value. The co-expressed mRNA-lncRNA pairs with an absolute value of their Pearson correlation coefficient of ≥0.8 were selected. As presented in Figure 6A, the network included 7 differentially expressed lncRNAs and 70 DE-mRNAs.
To characterize the biological function of the 7 differentially expressed lncRNAs, we performed gene set enrichment analysis on the highly correlated DE-mRNAs. Based to the GO terms by enrichment analysis, we successfully annotated 6 of the 7 DE-lncRNAs (P < 0.05, Figure 6B). Specifically, AC009041.1 and SSTR5-AS1 were primarily enriched in DNA conformation change and DNA recombination. Particularly, RP11-498C9.17 was enriched in pathways involved in epigenetic regulation, such as macromolecule deacylation, epigenetic regulation of gene expression, chromatin remodeling, chromatin assembly or disassembly and protein-DNA complex subunit organization. Other lincRNAs may participate in pathways such as cell-cell signaling by Wnt, response to extracellular stimulus, autophagy, and cell cycle checkpoint.
Osteoclastogenesis and bone resorption play a fundamental role in osteoporosis pathogenesis. Better understanding the regulation of osteoclastogenesis is very important for the discovery of therapeutic targets for the treatment of osteoporosis.
In this study, we aimed to analyze the key mRNAs and lncRNAs in osteoporosis. To explore the molecular mechanism of regulating bone miner density, we collected gene expression datasets of peripheral blood monocytes (PBMs) from low or high BMD subjects. To identify the probes on the array corresponding to long non-coding RNA (lncRNA) sequences, we re-annotated all probes using GENCODE gene annotation, and identified a total of 830 long non-coding RNAs.
To investigate the biological differences between samples with low and high BMD, we performed differential expression analysis of the gene expression data from the discovery datasets, and identified a total of 496 differentially expressed genes (DEGs), including 120 up-regulated and 376 down-regulated in low BMD subjects, of which, 24 were long non-coding RNAs differentially expressed between samples with low and high BMD. Subsequently, the differentially expressed mRNAs were subjected to GO and KEGG analyses. The up-regulated mRNAs were significantly enriched in inflammation-related pathways, which was consistent with the conclusion that inflammatory condition could contribute to the differentiation from monocyte to osteoclast by previous study [25]. In contrast, the downregulated genes were mainly associated with adipocytokine signaling pathway, metabolic pathways, oxidative phosphorylation, cytosolic DNA-sensing pathway, and glycerophospholipid metabolism, suggesting that metabolic pathways may function by maintaining the bone miner density.
To investigate the interactions between the proteins encoded by the DEGs, we mapped the DEGs to protein-protein interaction network. We observed that the down-regulated proteins, including JDP2, HADC4, HDAC5, CDYL2, ACADVL, ACSL1 and BRD4, were key components in the down-regulated PPI network (Figure 5B). It is well established that the downregulation of histone deacetylases can promote osteoclastogenesis [26,27], indicating that the downregulation of histone deacetylases and cofactors, such as, HDAC4, HDAC5 and JDP2 may be critical regulators in osteoclastogenesis. Moreover, IL8, CCL25, CNR1, HCAR2, HCAR1, GNG13 and GNG8 were also identified as a module in the down-regulated PPI network (Figure 5C). All these proteins except IL8 participated in G-alpha (ⅰ) signaling events, which could inhibit cAMP dependent pathway [28]. Previous study [29] also reported that cAMP-PKA could regulate osteoclast differentiation, indicating that this pathway may be important for bone mineral homeostasis.
To predict the potential functional roles of the differentially expressed lncRNAs, the Pearson correlation coefficient for lncRNA-DE-mRNA pairs was calculated to construct the co-expression network for mRNAs and lncRNAs. Particularly, RP11-498C9.17 was highly correlated with epigenetic regulators, such as HDAC4, MORF4L1, HMGA1 and DND1. As we described above, the downregulation of histone deacetylases can promote osteoclastogenesis, indicating that the lncRNA RP11-498C9.17 may play a key role in bone mineral homeostasis via controlling osteoclastogenesis.
In conclusion, our integrative analysis revealed key mRNAs and lncRNAs, involved in bone mineral homeostasis and osteoclastogenesis. The results not only broaden our insights into lncRNAs in bone mineral homeostasis and osteoclastogenesis, but also improved our understanding of molecular mechanism.
This work were financially supported by the Doctoral Fund of Inner Mongolia Normal University under Grant No. 100900091719, the Scientific research projects of the Inner Mongolian higher educational system(NJZY19025)and Provincial Nature Science Research Project of Anhui Colleges (KJ2018A0331). The funders had no role in study design, data collection and analysis, decision to publish, or preparation of the manuscript.
The authors declare that they have no competing interests.
[1] |
Z. Guo, K. Yu, A. K. Bashir, D. Zhang, Y. D. Al-Otaibi, M. Guizani, Deep information fusion-driven POI scheduling for mobile social networks, IEEE Network, 36 (2022), 210–216, https://doi.org/10.1109/MNET.102.2100394 doi: 10.1109/MNET.102.2100394
![]() |
[2] |
Z. Shen, F. Ding, Y. Yao, A. Bhardwaj, Z. Guo, K. Yu, A privacy-preserving social computing framework for health management using federated learning, IEEE Trans. Comput. Soc. Syst., (2022), 1–13, https://doi.org/10.1109/TCSS.2022.3222682 doi: 10.1109/TCSS.2022.3222682
![]() |
[3] |
C. D. Wei, C. Liu, W. Shun, S. Wang, X. L. Wang, W. F. Wu, Research and application of multimedia digital platform in the teaching of college physical education course, J. Intell. Fuzzy Syst., 34 (2018), 893–901. https://doi.org/10.3233/JIFS-169383 doi: 10.3233/JIFS-169383
![]() |
[4] |
Z. Guo, K. Yu, A. Jolfaei, G. Li, F. Ding, A. Beheshti, Mixed graph neural network-based fake news detection for sustainable vehicular social networks, IEEE Trans. Intell. Trans. Syst., (2022), 1–13. https://doi.org/10.1109/TITS.2022.3185013 doi: 10.1109/TITS.2022.3185013
![]() |
[5] |
J. De-Kun, F. H. Memon, Design of mobile intelligent evaluation algorithm in physical education teaching, Mobile Networks Appl., 27 (2021), 527–534. https://doi.org/10.1007/s11036-021-01818-1 doi: 10.1007/s11036-021-01818-1
![]() |
[6] |
T. Kidokoro, Y. Kohmura, N. Fuku, Y. Someya, K. Suzuki, Secular trends in the grip strength and body mass index of sport university students between 1973 and 2016: J-fit+ study, J. Exercise Sci. Fitness, 18 (2020), 21–30. https://doi.org/10.1016/j.jesf.2019.08.002 doi: 10.1016/j.jesf.2019.08.002
![]() |
[7] |
D. Vinnikov, Z. Romanova, A. Dushpanova, K. Absatarova, Z. Utepbergenova, Prevalence of supplement use in recreationally active kazakhstan university students, J. Int. Soc. Sports Nutr., 15 (2018), 16. https://doi.org/10.1186/s12970-018-0220-4 doi: 10.1186/s12970-018-0220-4
![]() |
[8] |
D. Li, L. Deng, Z. Cai, K. Cai, Design of intelligent community security system based on visual tracking and large data natural language processing technology, J. Intell. Fuzzy Syst., 38 (2020), 7107–7117. https://doi.org/10.3233/JIFS-179789 doi: 10.3233/JIFS-179789
![]() |
[9] |
C. Themistocleous, K. Webster, A. Afthinos, K. Tsapkini, Part of speech production in patients with primary progressive aphasia: An analysis based on natural language processing, Am. J. Speech-Language Pathol., 30 (2021), 466–480. https://doi.org/10.1044/2020_AJSLP-19-00114 doi: 10.1044/2020_AJSLP-19-00114
![]() |
[10] |
T. Young, D. Hazarika, S. Poria, E. Cambria, Recent trends in deep learning based natural language processing, IEEE Comput. Intell. Mag., 13 (2018), 55–75. https://doi.org/10.1109/MCI.2018.2840738 doi: 10.1109/MCI.2018.2840738
![]() |
[11] |
Z. Taskin, U. Al, Natural language processing applications in library and information science, Online Inf. Rev., 43 (2019), 676–690. https://doi.org/10.1108/OIR-07-2018-0217 doi: 10.1108/OIR-07-2018-0217
![]() |
[12] |
H. Li, Deep learning for natural language processing: Advantages and challenges, Natl. Sci. Rev., 5 (2018), 24–26. https://doi.org/10.1093/nsr/nwx110 doi: 10.1093/nsr/nwx110
![]() |
[13] |
Y. Jararweh, M. Al-Ayyou, E. Benkhelifa, Advanced arabic natural language processing (ANLP) and its applications: Introduction to the special issue, Inf. Process. Manage., 55 (2019), 259–261. https://doi.org/10.1016/j.ipm.2018.09.003 doi: 10.1016/j.ipm.2018.09.003
![]() |
[14] |
H. Kim, S. Lee, A video captioning method based on multi-representation switching for sustainable computing, Sustainability, 13 (2021), 2250. https://doi.org/10.3390/su13042250 doi: 10.3390/su13042250
![]() |
[15] |
D. D. Clercq, Z. Wen, Q. Song, Innovation hotspots in food waste treatment, biogas, and anaerobic digestion technology: A natural language processing approach, Sci. Total Enviro., 673 (2019), 402–413. https://doi.org/10.1016/j.scitotenv.2019.04.051 doi: 10.1016/j.scitotenv.2019.04.051
![]() |
[16] |
S. R. Marder, Natural language processing: Its potential role in clinical care and clinical research, Schizophr. Bull., 48 (2022), 958–959. https://doi.org/10.1093/schbul/sbac092 doi: 10.1093/schbul/sbac092
![]() |
[17] |
N. Afzal, V. P. Mallipeddi, S. Sohn, H. Liu, R. Chaudhry, C. G. Scott, et al., Natural language processing of clinical notes for identification of critical limb ischemia, Int. J. Med. Inf., 111 (2018), 83–89. https://doi.org/10.1016/j.ijmedinf.2017.12.024 doi: 10.1016/j.ijmedinf.2017.12.024
![]() |
[18] |
V. Krishnamurthy, S. Gao, Syntactic enhancement to vsimm for roadmap based anomalous trajectory detection: A natural language processing approach, IEEE Trans. Signal Process., 66 (2018), 5212–5227. https://doi.org/10.1109/TSP.2018.2866386 doi: 10.1109/TSP.2018.2866386
![]() |
[19] |
A. Amaar, W. Aljedaani, F. Rustam, S. Ullah, V. Rupapara, S. Ludi, Detection of fake job postings by utilizing machine learning and natural language processing approaches, Neural Process. Lett., 54 (2022), 2219–2247. https://doi.org/10.1007/s11063-021-10727-z doi: 10.1007/s11063-021-10727-z
![]() |
[20] |
A. M. Hilal, F. N. Al-Wesabi, A. Abdelmaboud, M. A. Hamza, M. Mahzari, A. Q. A. Hassan, A hybrid intelligent text watermarking and natural language processing approach for transferring and receiving an authentic english text via internet, Comput. J., 65 (2021), 423–425. https://doi.org/10.1093/comjnl/bxab087 doi: 10.1093/comjnl/bxab087
![]() |
[21] |
S. Cheng, I. C. Prentice, Y. Huang, Y. Jin, Y. K. Guo, R. Arcucci, Data-driven surrogate model with latent data assimilation: Application to wildfire forecasting, J. Comput. Phys., 464 (2022), 111302. https://doi.org/10.1016/j.jcp.2022.111302 doi: 10.1016/j.jcp.2022.111302
![]() |
[22] |
C. Zhang, S. Cheng, M. Kasoar, R. Arcucci, Reduced order digital twin and latent data assimilation for global wildfire prediction, EGUsphere, (2022), 1–24. https://doi.org/10.5194/egusphere-2022-1167 doi: 10.5194/egusphere-2022-1167
![]() |
[23] |
C. Buizza, C. Q. Casas, P. Nadler, J. Mack, S. Marrone, Z. Titus, et al., Data learning: integrating data assimilation and machine learning, J. Comput. Sci., 58 (2022), 101525. https://doi.org/10.1016/j.jocs.2021.101525 doi: 10.1016/j.jocs.2021.101525
![]() |
[24] |
T. Soffer, A. Cohen, Students' engagement characteristics predict success and completion of online courses, J. Comput. Assist. Lear., 35 (2019), 378–389. https://doi.org/10.1111/jcal.12340 doi: 10.1111/jcal.12340
![]() |
[25] |
K. Oodaira, T. Miyazaki, Y. Sugaya, S. Omachi, Importance estimation for scene texts using visual features, Int. Inf. Sci., 28 (2022), 15–23. https://doi.org/10.4036/iis.2022.A.06 doi: 10.4036/iis.2022.A.06
![]() |
[26] |
Z. Guo, K. Yu, N. Kumar, W. Wei, S. Mumtaz, M. Guizani, Deep-distributed-learning-based poi recommendation under mobile-edge networks, IEEE Int. Things J., 10 (2022), 303–317. https://doi.org/10.1109/JIOT.2022.3202628 doi: 10.1109/JIOT.2022.3202628
![]() |
[27] |
Z. Guo, D. Meng, C. Chakraborty, X. Fan, A. Bhardwaj, K. Yu, Autonomous behavioral decision for vehicular agents based on cyber-physical social intelligence, IEEE Trans. Comput. Soc. Syst., (2022). https://doi.org/10.1109/TCSS.2022.3212864 doi: 10.1109/TCSS.2022.3212864
![]() |
[28] |
G. Hwang, S. Wang, Chiu-Lin Lai, Effects of a social regulation-based online learning framework on students' learning achievements and behaviors in mathematics, Comput. Educ., 160 (2021), 104031. https://doi.org/10.1016/j.compedu.2020.104031 doi: 10.1016/j.compedu.2020.104031
![]() |
[29] |
Q. Li, L. Liu, Z. Guo, P. Vijayakumar, F. Taghizadeh-Hesary, K. Yu, Smart assessment and forecasting framework for healthy development index in urban cities, Cities, 131 (2022), 103971. https://doi.org/10.1016/j.cities.2022.103971 doi: 10.1016/j.cities.2022.103971
![]() |
[30] |
Q. Zhang, Z. Guo, Y. Zhu, P. Vijayakumar, A. Castiglione, B. B. Gupta, A deep learning-based fast fake news detection model for cyber-physical social services, Pattern Recognition Letters, 168 (2023), 31–38. https://doi.org/10.1016/j.patrec.2023.02.026 doi: 10.1016/j.patrec.2023.02.026
![]() |
1. | Yang Yang, Wang Yujiao, Wang Fang, Yuan Linhui, Guo Ziqi, Wei Zhichen, Wang Zirui, Wang Shengwang, The roles of miRNA, lncRNA and circRNA in the development of osteoporosis, 2020, 53, 0717-6287, 10.1186/s40659-020-00309-z | |
2. | Zhaowei Teng, Yun Zhu, Xiguang Zhang, Yirong Teng, Sheng Lu, Osteoporosis Is Characterized by Altered Expression of Exosomal Long Non-coding RNAs, 2020, 11, 1664-8021, 10.3389/fgene.2020.566959 | |
3. | Kyoung-Tae Kim, Young-Seok Lee, Inbo Han, The Role of Epigenomics in Osteoporosis and Osteoporotic Vertebral Fracture, 2020, 21, 1422-0067, 9455, 10.3390/ijms21249455 | |
4. | Siriluck Ponsuksili, Henry Reyer, Frieder Hadlich, Frank Weber, Nares Trakooljul, Michael Oster, Puntita Siengdee, Eduard Muráni, Markus Rodehutscord, Amélia Camarinha-Silva, Jörn Bennewitz, Klaus Wimmers, Identification of the Key Molecular Drivers of Phosphorus Utilization Based on Host miRNA-mRNA and Gut Microbiome Interactions, 2020, 21, 1422-0067, 2818, 10.3390/ijms21082818 | |
5. | Li Duan, Yujie Liang, Xiao Xu, Jifeng Wang, Xingfu Li, Deshun Sun, Zhiqin Deng, Wencui Li, Daping Wang, Noncoding RNAs in subchondral bone osteoclast function and their therapeutic potential for osteoarthritis, 2020, 22, 1478-6362, 10.1186/s13075-020-02374-x | |
6. | Cinzia Aurilia, Simone Donati, Gaia Palmini, Francesca Miglietta, Teresa Iantomasi, Maria Luisa Brandi, The Involvement of Long Non-Coding RNAs in Bone, 2021, 22, 1422-0067, 3909, 10.3390/ijms22083909 | |
7. | Xiaowei Jiang, Pu Ying, Yingchao Shen, Yiming Miu, Wenbin Kong, Tong Lu, Qiang Wang, Identification of Critical Functional Modules and Signaling Pathways in Osteoporosis, 2021, 16, 15748936, 90, 10.2174/1574893615999200706002411 | |
8. | Shan Cong, Xiaohui Yao, Linhui Xie, Jingwen Yan, Li Shen, Genetic Influence Underlying Brain Connectivity Phenotype: A Study on Two Age-Specific Cohorts, 2022, 12, 1664-8021, 10.3389/fgene.2021.782953 | |
9. | Xiaochuan Wang, Chi Zhang, Guang Zhao, Keda Yang, Lin Tao, Obesity and lipid metabolism in the development of osteoporosis (Review), 2024, 54, 1107-3756, 10.3892/ijmm.2024.5385 | |
10. | Luoyi Wang, Zhaomin Mao, Fengmin Shao, Identification of toll‐like receptor 5 and acyl‐CoA synthetase long chain family member 1 as hub genes are correlated with the severe forms of COVID‐19 by Weighted gene co‐expression network analysis, 2023, 17, 1751-8849, 327, 10.1049/syb2.12079 | |
11. | Pan Gao, Xiaoguang Pan, Shang Wang, Sijia Guo, Zhanying Dong, Zhefeng Wang, Xue Liang, Yan Chen, Fang Fang, Ling Yang, Jinrong Huang, Chenxi Zhang, Conghui Li, Yonglun Luo, Songlin Peng, Fengping Xu, Identification of the transcriptome signatures and immune-inflammatory responses in postmenopausal osteoporosis, 2024, 10, 24058440, e23675, 10.1016/j.heliyon.2023.e23675 | |
12. | Wenjie Liu, Yunhui Zhang, Quanfeng Li, Xinglang Wang, Yanfeng Wu, Huiyong Shen, Peng Wang, Advances of long non-coding RNAs in osteoclast differentiation and osteoporosis, 2024, 260, 03440338, 155413, 10.1016/j.prp.2024.155413 | |
13. | Mojtaba Baniasadi, Sina Talebi, Khatere Mokhtari, Amir Hossein Zabolian, Elahe Mohandesi Khosroshahi, Maliheh Entezari, Farshid Dehkhoda, Noushin Nabavi, Mehrdad Hashemi, Role of non-coding RNAs in osteoporosis, 2024, 253, 03440338, 155036, 10.1016/j.prp.2023.155036 |
ID | gene_type | gene_symbol | status | p-value |
ENSG00000001630.11 | protein_coding | CYP51A1 | Up | 0.011965453 |
ENSG00000005075.11 | protein_coding | POLR2J | Down | 0.018005066 |
ENSG00000006282.15 | protein_coding | SPATA20 | Down | 0.030683456 |
ENSG00000007376.3 | protein_coding | RPUSD1 | Down | 0.046007757 |
ENSG00000007944.10 | protein_coding | MYLIP | Up | 0.012046727 |
ENSG00000008952.12 | protein_coding | SEC62 | Up | 0.04688829 |
ENSG00000014164.6 | protein_coding | ZC3H3 | Down | 0.035615575 |
ENSG00000020256.15 | protein_coding | ZFP64 | Up | 0.035853374 |
ENSG00000025770.14 | protein_coding | NCAPH2 | Down | 0.035381718 |
ENSG00000028277.16 | protein_coding | POU2F2 | Down | 0.025284331 |
ENSG00000029153.10 | protein_coding | ARNTL2 | Down | 0.010597798 |
ENSG00000032444.11 | protein_coding | PNPLA6 | Down | 0.015278704 |
ENSG00000034533.7 | protein_coding | ASTE1 | Up | 0.033013833 |
ENSG00000039123.11 | protein_coding | SKIV2L2 | Down | 0.048932372 |
ENSG00000047056.10 | protein_coding | WDR37 | Down | 0.034858116 |
ENSG00000050344.8 | protein_coding | NFE2L3 | Down | 0.033023849 |
ENSG00000051523.6 | protein_coding | CYBA | Down | 0.007486695 |
ENSG00000052723.7 | protein_coding | SIKE1 | Up | 0.045307581 |
ENSG00000052749.9 | protein_coding | RRP12 | Down | 0.026396768 |
ENSG00000054803.3 | protein_coding | CBLN4 | Down | 0.045582496 |
ENSG00000054967.8 | protein_coding | RELT | Down | 0.02019792 |
ENSG00000062598.13 | protein_coding | ELMO2 | Down | 0.025904437 |
ENSG00000064932.11 | protein_coding | SBNO2 | Down | 0.033420705 |
ENSG00000068024.12 | protein_coding | HDAC4 | Down | 0.048895859 |
ENSG00000068120.10 | protein_coding | COASY | Down | 0.009649617 |
ENSG00000069764.5 | protein_coding | PLA2G10 | Down | 0.003199821 |
ENSG00000070404.5 | protein_coding | FSTL3 | Down | 0.038524776 |
ENSG00000072778.15 | protein_coding | ACADVL | Down | 0.028472324 |
ENSG00000074842.3 | protein_coding | C19orf10 | Down | 0.00105098 |
ENSG00000077150.13 | protein_coding | NFKB2 | Down | 0.003922325 |
ENSG00000078804.8 | protein_coding | TP53INP2 | Down | 0.005193897 |
ENSG00000079393.16 | protein_coding | DUSP13 | Down | 0.024246462 |
ENSG00000079432.3 | protein_coding | CIC | Down | 0.019836428 |
ENSG00000081189.9 | protein_coding | MEF2C | Up | 0.047860138 |
ENSG00000083312.13 | protein_coding | TNPO1 | Up | 0.047418317 |
ENSG00000083844.6 | protein_coding | ZNF264 | Up | 0.010459153 |
ENSG00000087157.14 | protein_coding | PGS1 | Down | 0.042327569 |
ENSG00000087237.6 | protein_coding | CETP | Down | 0.032983061 |
ENSG00000087263.12 | protein_coding | OGFOD1 | Up | 0.043418236 |
ENSG00000087266.11 | protein_coding | SH3BP2 | Down | 0.030356676 |
ENSG00000087903.8 | protein_coding | RFX2 | Down | 0.020836355 |
ENSG00000088888.13 | protein_coding | MAVS | Down | 0.04764586 |
ENSG00000089123.11 | protein_coding | TASP1 | Up | 0.028910399 |
ENSG00000089248.6 | protein_coding | ERP29 | Down | 0.049446948 |
ENSG00000089351.10 | protein_coding | GRAMD1A | Down | 0.025055632 |
ENSG00000089639.6 | protein_coding | GMIP | Down | 0.014587545 |
ENSG00000090924.10 | protein_coding | PLEKHG2 | Down | 0.028301873 |
ENSG00000092929.7 | protein_coding | UNC13D | Down | 0.013293567 |
ENSG00000096088.12 | protein_coding | PGC | Down | 0.042062111 |
ENSG00000099139.9 | protein_coding | PCSK5 | Up | 0.027380288 |
ENSG00000099203.2 | protein_coding | TMED1 | Down | 0.028235039 |
ENSG00000099795.2 | protein_coding | NDUFB7 | Down | 0.040697141 |
ENSG00000099800.3 | protein_coding | TIMM13 | Down | 0.007324296 |
ENSG00000099821.9 | protein_coding | POLRMT | Down | 0.045124992 |
ENSG00000099958.10 | protein_coding | DERL3 | Down | 0.04060876 |
ENSG00000099991.12 | protein_coding | CABIN1 | Down | 0.034876772 |
ENSG00000100028.7 | protein_coding | SNRPD3 | Down | 0.044318218 |
ENSG00000100055.16 | protein_coding | CYTH4 | Down | 0.018939808 |
ENSG00000100092.16 | protein_coding | SH3BP1 | Down | 0.04634582 |
ENSG00000100242.11 | protein_coding | SUN2 | Down | 0.010030177 |
ENSG00000100290.2 | protein_coding | BIK | Down | 0.007141866 |
ENSG00000100403.10 | protein_coding | ZC3H7B | Down | 0.0410179 |
ENSG00000100429.13 | protein_coding | HDAC10 | Down | 0.033877634 |
ENSG00000100532.7 | protein_coding | CGRRF1 | Up | 0.013688977 |
ENSG00000100802.10 | protein_coding | C14orf93 | Down | 0.033127459 |
ENSG00000100906.6 | protein_coding | NFKBIA | Down | 0.005259074 |
ENSG00000101220.13 | protein_coding | C20orf27 | Down | 0.034454114 |
ENSG00000101473.12 | protein_coding | ACOT8 | Down | 0.016141884 |
ENSG00000101624.6 | protein_coding | CEP76 | Up | 0.013187998 |
ENSG00000101665.4 | protein_coding | SMAD7 | Down | 0.017676506 |
ENSG00000101997.8 | protein_coding | CCDC22 | Down | 0.039248809 |
ENSG00000102078.11 | protein_coding | SLC25A14 | Down | 0.039031376 |
ENSG00000102786.10 | protein_coding | INTS6 | Up | 0.044913283 |
ENSG00000102981.5 | protein_coding | PARD6A | Down | 0.041910914 |
ENSG00000103024.3 | protein_coding | NME3 | Down | 0.027773943 |
ENSG00000103047.3 | protein_coding | TANGO6 | Down | 0.049704948 |
ENSG00000103227.14 | protein_coding | LMF1 | Down | 0.022119939 |
ENSG00000103426.8 | protein_coding | CORO7-PAM16 | Down | 0.014976017 |
ENSG00000103449.7 | protein_coding | SALL1 | Down | 0.041904944 |
ENSG00000103653.12 | protein_coding | CSK | Down | 0.010534323 |
ENSG00000104856.9 | protein_coding | RELB | Down | 0.015505238 |
ENSG00000104973.10 | protein_coding | MED25 | Down | 0.041089276 |
ENSG00000105063.14 | protein_coding | PPP6R1 | Down | 0.022586612 |
ENSG00000105135.11 | protein_coding | ILVBL | Down | 0.034386207 |
ENSG00000105497.3 | protein_coding | ZNF175 | Up | 0.013075356 |
ENSG00000105643.5 | protein_coding | ARRDC2 | Down | 0.039560218 |
ENSG00000105656.8 | protein_coding | ELL | Down | 0.035373441 |
ENSG00000106268.11 | protein_coding | NUDT1 | Down | 0.03304039 |
ENSG00000107362.9 | protein_coding | ABHD17B | Up | 0.02410498 |
ENSG00000107443.11 | protein_coding | CCNJ | Down | 0.029817026 |
ENSG00000107984.5 | protein_coding | DKK1 | Down | 0.049671011 |
ENSG00000108179.9 | protein_coding | PPIF | Down | 0.044960461 |
ENSG00000108518.7 | protein_coding | PFN1 | Down | 0.045797043 |
ENSG00000108840.11 | protein_coding | HDAC5 | Down | 0.01595402 |
ENSG00000108950.7 | protein_coding | FAM20A | Down | 0.012800605 |
ENSG00000109103.7 | protein_coding | UNC119 | Down | 0.03809287 |
ENSG00000109736.10 | protein_coding | MFSD10 | Down | 0.043758832 |
ENSG00000109917.6 | protein_coding | ZNF259 | Down | 0.042419417 |
ENSG00000109944.6 | protein_coding | C11orf63 | Down | 0.036421384 |
ENSG00000110063.4 | protein_coding | DCPS | Down | 0.044521345 |
ENSG00000110080.14 | protein_coding | ST3GAL4 | Down | 0.009630116 |
ENSG00000110090.8 | protein_coding | CPT1A | Down | 0.040715917 |
ENSG00000110619.12 | protein_coding | CARS | Down | 0.040080075 |
ENSG00000110931.14 | protein_coding | CAMKK2 | Down | 0.041840274 |
ENSG00000112149.5 | protein_coding | CD83 | Down | 0.021210986 |
ENSG00000112276.9 | protein_coding | BVES | Up | 0.013370759 |
ENSG00000112312.5 | protein_coding | GMNN | Down | 0.047237299 |
ENSG00000114650.14 | protein_coding | SCAP | Down | 0.032838904 |
ENSG00000115073.6 | protein_coding | ACTR1B | Down | 0.003540043 |
ENSG00000116017.6 | protein_coding | ARID3A | Down | 0.038762022 |
ENSG00000116670.10 | protein_coding | MAD2L2 | Down | 0.043537769 |
ENSG00000116852.10 | protein_coding | KIF21B | Down | 0.049989022 |
ENSG00000116871.11 | protein_coding | MAP7D1 | Down | 0.03998345 |
ENSG00000116985.6 | protein_coding | BMP8B | Down | 0.028382633 |
ENSG00000117600.8 | protein_coding | LPPR4 | Down | 0.046876942 |
ENSG00000118432.11 | protein_coding | CNR1 | Down | 0.017637126 |
ENSG00000118600.7 | protein_coding | TMEM5 | Down | 0.014944131 |
ENSG00000119013.4 | protein_coding | NDUFB3 | Down | 0.040614225 |
ENSG00000119121.17 | protein_coding | TRPM6 | Down | 0.048825853 |
ENSG00000119669.3 | protein_coding | IRF2BPL | Down | 0.005359191 |
ENSG00000120217.9 | protein_coding | CD274 | Down | 0.043686299 |
ENSG00000120699.8 | protein_coding | EXOSC8 | Up | 0.032500661 |
ENSG00000122203.10 | protein_coding | KIAA1191 | Up | 0.048031425 |
ENSG00000122490.14 | protein_coding | PQLC1 | Down | 0.015129367 |
ENSG00000122824.6 | protein_coding | NUDT10 | Down | 0.033200739 |
ENSG00000123094.11 | protein_coding | RASSF8 | Down | 0.029465179 |
ENSG00000123358.15 | protein_coding | NR4A1 | Down | 0.01086372 |
ENSG00000123576.5 | protein_coding | ESX1 | Down | 0.030485823 |
ENSG00000123689.5 | protein_coding | G0S2 | Down | 0.009066817 |
ENSG00000123989.9 | protein_coding | CHPF | Down | 0.040386914 |
ENSG00000125657.3 | protein_coding | TNFSF9 | Down | 0.049043875 |
ENSG00000125755.14 | protein_coding | SYMPK | Down | 0.049805392 |
ENSG00000125817.7 | protein_coding | CENPB | Down | 0.042137733 |
ENSG00000125945.10 | protein_coding | ZNF436 | Up | 0.047416168 |
ENSG00000125968.7 | protein_coding | ID1 | Down | 0.04512393 |
ENSG00000127054.14 | protein_coding | CPSF3L | Down | 0.04296422 |
ENSG00000127124.9 | protein_coding | HIVEP3 | Down | 0.000294073 |
ENSG00000127483.13 | protein_coding | HP1BP3 | Up | 0.047813073 |
ENSG00000127540.7 | protein_coding | UQCR11 | Down | 0.030739395 |
ENSG00000127588.4 | protein_coding | GNG13 | Down | 0.039828869 |
ENSG00000128059.4 | protein_coding | PPAT | Up | 0.006604019 |
ENSG00000128228.4 | protein_coding | SDF2L1 | Down | 0.003273018 |
ENSG00000128271.15 | protein_coding | ADORA2A | Down | 0.041530393 |
ENSG00000128309.12 | protein_coding | MPST | Down | 0.037538756 |
ENSG00000128694.7 | protein_coding | OSGEPL1 | Up | 0.036634744 |
ENSG00000129562.6 | protein_coding | DAD1 | Up | 0.039222956 |
ENSG00000129667.8 | protein_coding | RHBDF2 | Down | 0.030796176 |
ENSG00000129744.2 | protein_coding | ART1 | Down | 0.001540155 |
ENSG00000129968.11 | protein_coding | ABHD17A | Down | 0.043478487 |
ENSG00000129993.10 | protein_coding | CBFA2T3 | Down | 0.031277467 |
ENSG00000130522.4 | protein_coding | JUND | Down | 0.022542768 |
ENSG00000130766.4 | protein_coding | SESN2 | Down | 0.013640369 |
ENSG00000130935.5 | protein_coding | NOL11 | Up | 0.044393023 |
ENSG00000131142.9 | protein_coding | CCL25 | Down | 0.038185878 |
ENSG00000131171.8 | protein_coding | SH3BGRL | Up | 0.022819598 |
ENSG00000131653.8 | protein_coding | TRAF7 | Down | 0.036084131 |
ENSG00000131849.10 | protein_coding | ZNF132 | Down | 0.028132404 |
ENSG00000132275.6 | protein_coding | RRP8 | Down | 0.010570164 |
ENSG00000132507.13 | protein_coding | EIF5A | Down | 0.024112694 |
ENSG00000133606.6 | protein_coding | MKRN1 | Up | 0.041317442 |
ENSG00000133805.11 | protein_coding | AMPD3 | Down | 0.046431783 |
ENSG00000134107.4 | protein_coding | BHLHE40 | Down | 0.038744934 |
ENSG00000135047.10 | protein_coding | CTSL | Down | 0.0255056 |
ENSG00000135390.13 | protein_coding | ATP5G2 | Down | 0.02929579 |
ENSG00000136048.9 | protein_coding | DRAM1 | Down | 0.02091681 |
ENSG00000136122.11 | protein_coding | BORA | Up | 0.036657962 |
ENSG00000136738.10 | protein_coding | STAM | Up | 0.0389495 |
ENSG00000136840.14 | protein_coding | ST6GALNAC4 | Down | 0.016088565 |
ENSG00000136877.10 | protein_coding | FPGS | Down | 0.023271049 |
ENSG00000137124.6 | protein_coding | ALDH1B1 | Up | 0.03438067 |
ENSG00000137193.9 | protein_coding | PIM1 | Down | 0.009876567 |
ENSG00000137218.6 | protein_coding | FRS3 | Down | 0.042976435 |
ENSG00000137309.15 | protein_coding | HMGA1 | Down | 0.021493168 |
ENSG00000137312.10 | protein_coding | FLOT1 | Down | 0.039597455 |
ENSG00000137409.14 | protein_coding | MTCH1 | Down | 0.040766375 |
ENSG00000138382.9 | protein_coding | METTL5 | Up | 0.038252044 |
ENSG00000139053.2 | protein_coding | PDE6H | Down | 0.0207692 |
ENSG00000139610.1 | protein_coding | CELA1 | Up | 0.038317749 |
ENSG00000139668.7 | protein_coding | WDFY2 | Down | 0.019753557 |
ENSG00000140044.8 | protein_coding | JDP2 | Down | 0.036877537 |
ENSG00000140553.12 | protein_coding | UNC45A | Down | 0.042323486 |
ENSG00000140612.9 | protein_coding | SEC11A | Down | 0.017070625 |
ENSG00000140848.12 | protein_coding | CPNE2 | Down | 0.035194471 |
ENSG00000140941.8 | protein_coding | MAP1LC3B | Up | 0.010046471 |
ENSG00000141012.8 | protein_coding | GALNS | Down | 0.019933327 |
ENSG00000141034.5 | protein_coding | GID4 | Up | 0.036023887 |
ENSG00000141198.9 | protein_coding | TOM1L1 | Down | 0.043796522 |
ENSG00000141252.15 | protein_coding | VPS53 | Down | 0.020821593 |
ENSG00000141505.7 | protein_coding | ASGR1 | Down | 0.049034425 |
ENSG00000141646.9 | protein_coding | SMAD4 | Up | 0.041545876 |
ENSG00000141867.13 | protein_coding | BRD4 | Down | 0.040247622 |
ENSG00000142408.2 | protein_coding | CACNG8 | Down | 0.035337949 |
ENSG00000142538.1 | protein_coding | PTH2 | Down | 0.028959056 |
ENSG00000143549.15 | protein_coding | TPM3 | Down | 0.005503969 |
ENSG00000144120.8 | protein_coding | TMEM177 | Up | 0.022405325 |
ENSG00000144182.12 | protein_coding | LIPT1 | Up | 0.008805655 |
ENSG00000144381.12 | protein_coding | HSPD1 | Down | 0.048940793 |
ENSG00000144468.12 | protein_coding | RHBDD1 | Up | 0.048354944 |
ENSG00000144655.10 | protein_coding | CSRNP1 | Down | 0.03240296 |
ENSG00000144791.5 | protein_coding | LIMD1 | Down | 0.04142983 |
ENSG00000144802.7 | protein_coding | NFKBIZ | Down | 0.015266617 |
ENSG00000145901.10 | protein_coding | TNIP1 | Down | 0.031453499 |
ENSG00000146047.4 | protein_coding | HIST1H2BA | Down | 0.005162611 |
ENSG00000146232.10 | protein_coding | NFKBIE | Down | 0.020469032 |
ENSG00000146828.13 | protein_coding | SLC12A9 | Down | 0.042442305 |
ENSG00000146833.11 | protein_coding | TRIM4 | Up | 0.001031314 |
ENSG00000146856.10 | protein_coding | AGBL3 | Down | 0.044096515 |
ENSG00000147419.12 | protein_coding | CCDC25 | Up | 0.027233589 |
ENSG00000148187.13 | protein_coding | MRRF | Up | 0.01456252 |
ENSG00000148335.10 | protein_coding | NTMT1 | Down | 0.033476509 |
ENSG00000148602.5 | protein_coding | LRIT1 | Down | 0.038378276 |
ENSG00000148737.11 | protein_coding | TCF7L2 | Down | 0.03027628 |
ENSG00000148835.9 | protein_coding | TAF5 | Up | 0.017608599 |
ENSG00000149115.9 | protein_coding | TNKS1BP1 | Down | 0.031874191 |
ENSG00000149503.8 | protein_coding | INCENP | Down | 0.039618744 |
ENSG00000149792.4 | protein_coding | MRPL49 | Down | 0.014313227 |
ENSG00000149823.3 | protein_coding | VPS51 | Down | 0.034228234 |
ENSG00000149932.12 | protein_coding | TMEM219 | Down | 0.042059762 |
ENSG00000149968.7 | protein_coding | MMP3 | Down | 0.007039867 |
ENSG00000151093.3 | protein_coding | OXSM | Up | 0.032113374 |
ENSG00000151490.9 | protein_coding | PTPRO | Down | 0.0169847 |
ENSG00000151631.7 | pseudogene | AKR1C6P | Down | 0.004333285 |
ENSG00000151651.11 | protein_coding | ADAM8 | Down | 0.034809828 |
ENSG00000151726.9 | protein_coding | ACSL1 | Down | 0.040278414 |
ENSG00000152207.3 | protein_coding | CYSLTR2 | Up | 0.031075114 |
ENSG00000152382.5 | protein_coding | TADA1 | Down | 0.034551617 |
ENSG00000153140.4 | protein_coding | CETN3 | Up | 0.028301839 |
ENSG00000153207.10 | protein_coding | AHCTF1 | Down | 0.014567315 |
ENSG00000153266.8 | protein_coding | FEZF2 | Down | 0.036977261 |
ENSG00000153487.11 | protein_coding | ING1 | Down | 0.015188879 |
ENSG00000154370.9 | protein_coding | TRIM11 | Down | 0.018777551 |
ENSG00000154447.10 | protein_coding | SH3RF1 | Down | 0.009674391 |
ENSG00000154639.14 | protein_coding | CXADR | Down | 0.00685789 |
ENSG00000154781.11 | protein_coding | CCDC174 | Down | 0.049642675 |
ENSG00000154839.5 | protein_coding | SKA1 | Down | 0.046702852 |
ENSG00000154978.8 | protein_coding | VOPP1 | Down | 0.030307149 |
ENSG00000155438.7 | protein_coding | MKI67IP | Down | 0.03414805 |
ENSG00000155918.3 | protein_coding | RAET1L | Down | 0.047085308 |
ENSG00000156172.5 | protein_coding | C8orf37 | Up | 0.015241022 |
ENSG00000156508.13 | protein_coding | EEF1A1 | Down | 0.029958858 |
ENSG00000157227.8 | protein_coding | MMP14 | Down | 0.018623306 |
ENSG00000157326.14 | protein_coding | DHRS4 | Down | 0.047099568 |
ENSG00000157456.3 | protein_coding | CCNB2 | Down | 0.012184904 |
ENSG00000157637.8 | protein_coding | SLC38A10 | Down | 0.026642558 |
ENSG00000158014.10 | protein_coding | SLC30A2 | Down | 0.026315721 |
ENSG00000158483.11 | protein_coding | FAM86C1 | Down | 0.00975309 |
ENSG00000158497.2 | protein_coding | HMHB1 | Down | 0.040327646 |
ENSG00000158555.10 | protein_coding | GDPD5 | Down | 0.034837228 |
ENSG00000159069.9 | protein_coding | FBXW5 | Down | 0.01907369 |
ENSG00000159885.9 | protein_coding | ZNF222 | Up | 0.01199585 |
ENSG00000160111.8 | protein_coding | CPAMD8 | Down | 0.049299496 |
ENSG00000160179.14 | protein_coding | ABCG1 | Up | 0.022299138 |
ENSG00000160180.14 | protein_coding | TFF3 | Down | 0.042914253 |
ENSG00000160214.8 | protein_coding | RRP1 | Down | 0.049738569 |
ENSG00000160796.12 | protein_coding | NBEAL2 | Down | 0.035908182 |
ENSG00000160883.6 | protein_coding | HK3 | Down | 0.044846812 |
ENSG00000160888.6 | protein_coding | IER2 | Down | 0.027407148 |
ENSG00000161640.11 | protein_coding | SIGLEC11 | Down | 0.042121328 |
ENSG00000161791.9 | protein_coding | FMNL3 | Down | 0.011008036 |
ENSG00000162086.10 | protein_coding | ZNF75A | Up | 0.019226079 |
ENSG00000162302.8 | protein_coding | RPS6KA4 | Down | 0.048212217 |
ENSG00000162377.4 | protein_coding | SELRC1 | Up | 0.047979072 |
ENSG00000162413.12 | protein_coding | KLHL21 | Down | 0.048454407 |
ENSG00000162522.6 | protein_coding | KIAA1522 | Down | 0.016306649 |
ENSG00000162783.8 | protein_coding | IER5 | Down | 0.021919966 |
ENSG00000162913.9 | protein_coding | C1orf145 | Down | 0.018777551 |
ENSG00000162927.9 | protein_coding | PUS10 | Up | 0.04033551 |
ENSG00000162931.7 | protein_coding | TRIM17 | Down | 0.023650474 |
ENSG00000163162.4 | protein_coding | RNF149 | Down | 0.025666014 |
ENSG00000163239.8 | protein_coding | TDRD10 | Down | 0.043592404 |
ENSG00000163389.6 | protein_coding | POGLUT1 | Up | 0.022081661 |
ENSG00000163947.7 | protein_coding | ARHGEF3 | Up | 0.010264753 |
ENSG00000164105.3 | protein_coding | SAP30 | Up | 0.046694723 |
ENSG00000164342.8 | protein_coding | TLR3 | Up | 0.003277358 |
ENSG00000164402.9 | protein_coding | 8-Sep | Down | 0.024177765 |
ENSG00000164411.6 | protein_coding | GJB7 | Down | 0.026747052 |
ENSG00000165029.11 | protein_coding | ABCA1 | Up | 0.010492577 |
ENSG00000165188.9 | protein_coding | RNF183 | Down | 0.010089847 |
ENSG00000165406.11 | protein_coding | 8-Mar | Up | 0.047180108 |
ENSG00000165512.4 | protein_coding | ZNF22 | Up | 0.033208827 |
ENSG00000165782.6 | protein_coding | TMEM55B | Down | 0.022652362 |
ENSG00000165886.4 | protein_coding | UBTD1 | Down | 0.014531961 |
ENSG00000165915.9 | protein_coding | SLC39A13 | Down | 0.024410393 |
ENSG00000166265.7 | protein_coding | CYYR1 | Down | 0.037657919 |
ENSG00000166363.4 | protein_coding | OR10A5 | Up | 0.018183215 |
ENSG00000166446.10 | protein_coding | CDYL2 | Down | 0.011853205 |
ENSG00000166451.9 | protein_coding | CENPN | Up | 0.040214671 |
ENSG00000166452.7 | protein_coding | AKIP1 | Up | 0.048924644 |
ENSG00000166938.8 | protein_coding | DIS3L | Up | 0.036638539 |
ENSG00000166974.8 | protein_coding | MAPRE2 | Up | 0.048488455 |
ENSG00000167110.12 | protein_coding | GOLGA2 | Down | 0.023994018 |
ENSG00000167186.6 | protein_coding | COQ7 | Up | 0.000296612 |
ENSG00000167333.8 | protein_coding | TRIM68 | Up | 0.039246931 |
ENSG00000167394.8 | protein_coding | ZNF668 | Down | 0.015855291 |
ENSG00000167414.4 | protein_coding | GNG8 | Down | 0.0489265 |
ENSG00000167555.9 | protein_coding | ZNF528 | Up | 0.002098542 |
ENSG00000167604.9 | protein_coding | NFKBID | Down | 0.04908962 |
ENSG00000167657.7 | protein_coding | DAPK3 | Down | 0.045503987 |
ENSG00000168066.16 | protein_coding | SF1 | Down | 0.047265519 |
ENSG00000168310.6 | protein_coding | IRF2 | Up | 0.044253405 |
ENSG00000168594.11 | protein_coding | ADAM29 | Down | 0.003444856 |
ENSG00000168803.10 | protein_coding | ADAL | Up | 0.021101681 |
ENSG00000168818.5 | protein_coding | STX18 | Up | 0.022001045 |
ENSG00000168967.10 | pseudogene | PMCHL1 | Down | 0.008435479 |
ENSG00000169105.6 | protein_coding | CHST14 | Down | 0.024636798 |
ENSG00000169379.11 | protein_coding | ARL13B | Up | 0.010209654 |
ENSG00000169393.4 | protein_coding | ELSPBP1 | Down | 0.014495593 |
ENSG00000169429.6 | protein_coding | IL8 | Down | 0.028420528 |
ENSG00000170265.7 | protein_coding | ZNF282 | Down | 0.042671468 |
ENSG00000170369.3 | protein_coding | CST2 | Down | 0.041604924 |
ENSG00000170445.8 | protein_coding | HARS | Down | 0.029725289 |
ENSG00000170779.10 | protein_coding | CDCA4 | Down | 0.028547735 |
ENSG00000170837.2 | protein_coding | GPR27 | Down | 0.035077639 |
ENSG00000170891.6 | protein_coding | CYTL1 | Down | 0.032834474 |
ENSG00000171130.13 | protein_coding | ATP6V0E2 | Down | 0.039784296 |
ENSG00000171223.4 | protein_coding | JUNB | Down | 0.038177458 |
ENSG00000171357.5 | protein_coding | LURAP1 | Down | 0.033100787 |
ENSG00000171467.11 | protein_coding | ZNF318 | Down | 0.044327394 |
ENSG00000171530.9 | protein_coding | TBCA | Down | 0.009876223 |
ENSG00000171570.6 | protein_coding | RAB4B-EGLN2 | Down | 0.010162577 |
ENSG00000171657.5 | protein_coding | GPR82 | Up | 0.002688119 |
ENSG00000171766.11 | protein_coding | GATM | Up | 0.035375608 |
ENSG00000171827.6 | protein_coding | ZNF570 | Up | 0.016772935 |
ENSG00000171861.6 | protein_coding | RNMTL1 | Down | 0.041110394 |
ENSG00000171942.3 | protein_coding | OR10H2 | Down | 0.01466908 |
ENSG00000172208.3 | protein_coding | OR4X2 | Up | 0.038143037 |
ENSG00000172322.9 | protein_coding | CLEC12A | Up | 0.008850366 |
ENSG00000172375.8 | protein_coding | C2CD2L | Down | 0.012079432 |
ENSG00000172428.6 | protein_coding | MYEOV2 | Down | 0.025783713 |
ENSG00000173020.6 | protein_coding | ADRBK1 | Down | 0.043055525 |
ENSG00000173153.9 | protein_coding | ESRRA | Down | 0.008541886 |
ENSG00000173653.3 | protein_coding | RCE1 | Down | 0.030649495 |
ENSG00000173715.11 | protein_coding | C11orf80 | Down | 0.030649495 |
ENSG00000173812.6 | protein_coding | EIF1 | Down | 0.0170157 |
ENSG00000173846.8 | protein_coding | PLK3 | Down | 0.015509349 |
ENSG00000174600.9 | protein_coding | CMKLR1 | Up | 0.044214317 |
ENSG00000174886.8 | protein_coding | NDUFA11 | Down | 0.022192837 |
ENSG00000175130.6 | protein_coding | MARCKSL1 | Down | 0.038863502 |
ENSG00000175728.2 | protein_coding | C11orf44 | Up | 0.045296195 |
ENSG00000175874.5 | protein_coding | CREG2 | Down | 0.01537001 |
ENSG00000176485.6 | protein_coding | PLA2G16 | Down | 0.024862828 |
ENSG00000176563.5 | protein_coding | CNTD1 | Down | 0.016145384 |
ENSG00000176619.6 | protein_coding | LMNB2 | Down | 0.030268302 |
ENSG00000176624.9 | protein_coding | MEX3C | Up | 0.029845716 |
ENSG00000176714.9 | protein_coding | CCDC121 | Down | 0.022706601 |
ENSG00000176749.4 | protein_coding | CDK5R1 | Down | 0.04550598 |
ENSG00000176845.8 | protein_coding | METRNL | Down | 0.010677297 |
ENSG00000176894.5 | protein_coding | PXMP2 | Down | 0.013611729 |
ENSG00000176974.13 | protein_coding | SHMT1 | Up | 0.049830843 |
ENSG00000177051.5 | protein_coding | FBXO46 | Down | 0.047542187 |
ENSG00000177169.5 | protein_coding | ULK1 | Down | 0.016389135 |
ENSG00000177666.11 | protein_coding | PNPLA2 | Down | 0.043015592 |
ENSG00000178229.7 | protein_coding | ZNF543 | Up | 0.010459153 |
ENSG00000178951.4 | protein_coding | ZBTB7A | Down | 0.019056001 |
ENSG00000178980.10 | protein_coding | SEPW1 | Down | 0.016556027 |
ENSG00000179029.10 | protein_coding | TMEM107 | Down | 0.005945219 |
ENSG00000180008.8 | protein_coding | SOCS4 | Up | 0.039599073 |
ENSG00000180332.5 | protein_coding | KCTD4 | Down | 0.022125509 |
ENSG00000180346.2 | protein_coding | TIGD2 | Up | 0.037693259 |
ENSG00000180539.4 | protein_coding | C9orf139 | Down | 0.01622825 |
ENSG00000180725.4 | pseudogene | AC015871.1 | Down | 0.042511409 |
ENSG00000181378.9 | protein_coding | CCDC108 | Down | 0.038235948 |
ENSG00000181791.1 | pseudogene | AC009041.1 | Down | 0.0167024 |
ENSG00000182118.5 | protein_coding | FAM89A | Down | 0.002426708 |
ENSG00000182333.10 | protein_coding | LIPF | Down | 0.018324226 |
ENSG00000182368.4 | protein_coding | FAM27A | Down | 0.03035551 |
ENSG00000182541.13 | protein_coding | LIMK2 | Down | 0.041747303 |
ENSG00000182782.7 | protein_coding | HCAR2 | Down | 0.046508935 |
ENSG00000182841.8 | pseudogene | RRP7B | Down | 0.04819365 |
ENSG00000183019.3 | protein_coding | C19orf59 | Down | 0.031283921 |
ENSG00000183020.9 | protein_coding | AP2A2 | Down | 0.000692239 |
ENSG00000183087.10 | protein_coding | GAS6 | Down | 0.026705611 |
ENSG00000183648.5 | protein_coding | NDUFB1 | Down | 0.00863385 |
ENSG00000183696.9 | protein_coding | UPP1 | Down | 0.021571741 |
ENSG00000183709.7 | protein_coding | IFNL2 | Up | 0.001410201 |
ENSG00000183723.8 | protein_coding | CMTM4 | Down | 0.047938821 |
ENSG00000183779.5 | protein_coding | ZNF703 | Down | 0.033061055 |
ENSG00000184110.10 | protein_coding | EIF3C | Down | 0.008205594 |
ENSG00000184517.7 | protein_coding | ZFP1 | Up | 0.045781579 |
ENSG00000184619.3 | protein_coding | KRBA2 | Down | 0.025225822 |
ENSG00000184897.4 | protein_coding | H1FX | Down | 0.014748614 |
ENSG00000184922.9 | protein_coding | FMNL1 | Down | 0.01035533 |
ENSG00000184990.8 | protein_coding | SIVA1 | Down | 0.039061925 |
ENSG00000185641.5 | pseudogene | CTD-2287O16.1 | Down | 0.043078899 |
ENSG00000185787.10 | protein_coding | MORF4L1 | Down | 0.047667026 |
ENSG00000185972.4 | protein_coding | CCIN | Down | 0.044995407 |
ENSG00000186106.7 | protein_coding | ANKRD46 | Up | 0.042420836 |
ENSG00000186111.4 | protein_coding | PIP5K1C | Down | 0.042436011 |
ENSG00000186281.8 | protein_coding | GPAT2 | Down | 0.049178673 |
ENSG00000186594.8 | lincRNA | MIR22HG | Down | 0.017511035 |
ENSG00000186635.10 | protein_coding | ARAP1 | Down | 0.015438232 |
ENSG00000186795.1 | protein_coding | KCNK18 | Down | 0.020856507 |
ENSG00000187630.10 | protein_coding | DHRS4L2 | Down | 0.047099568 |
ENSG00000187688.10 | protein_coding | TRPV2 | Down | 0.003137583 |
ENSG00000188379.5 | protein_coding | IFNA2 | Down | 0.004976237 |
ENSG00000189042.9 | protein_coding | ZNF567 | Up | 0.015774858 |
ENSG00000189114.6 | protein_coding | BLOC1S3 | Down | 0.028793638 |
ENSG00000189332.4 | protein_coding | RP11-113D6.10 | Down | 0.029127001 |
ENSG00000196152.6 | protein_coding | ZNF79 | Up | 0.002879344 |
ENSG00000196331.5 | protein_coding | HIST1H2BO | Down | 0.025404112 |
ENSG00000196358.6 | protein_coding | NTNG2 | Down | 0.042750195 |
ENSG00000196371.2 | protein_coding | FUT4 | Down | 0.011093343 |
ENSG00000196415.5 | protein_coding | PRTN3 | Down | 0.035149627 |
ENSG00000196417.8 | protein_coding | ZNF765 | Up | 0.005727824 |
ENSG00000196652.7 | protein_coding | ZKSCAN5 | Up | 0.016188909 |
ENSG00000196668.3 | processed_transcript | LINC00173 | Down | 0.003213639 |
ENSG00000196693.10 | protein_coding | ZNF33B | Up | 0.010400288 |
ENSG00000196843.11 | protein_coding | ARID5A | Down | 0.023473729 |
ENSG00000196917.4 | protein_coding | HCAR1 | Down | 0.023288331 |
ENSG00000196981.2 | protein_coding | WDR5B | Up | 0.010645113 |
ENSG00000197016.7 | protein_coding | ZNF470 | Up | 0.021502777 |
ENSG00000197312.7 | protein_coding | DDI2 | Up | 0.049559262 |
ENSG00000197321.10 | protein_coding | SVIL | Down | 0.029323831 |
ENSG00000197919.3 | protein_coding | IFNA1 | Down | 0.011997429 |
ENSG00000198055.6 | protein_coding | GRK6 | Down | 0.018617852 |
ENSG00000198081.6 | protein_coding | ZBTB14 | Up | 0.03828373 |
ENSG00000198324.10 | protein_coding | FAM109A | Down | 0.015387399 |
ENSG00000198445.3 | protein_coding | CCT8L2 | Down | 0.008263414 |
ENSG00000198464.9 | protein_coding | ZNF480 | Up | 0.04695864 |
ENSG00000198471.1 | protein_coding | RTP2 | Up | 0.026862674 |
ENSG00000198551.5 | protein_coding | ZNF627 | Up | 0.007529781 |
ENSG00000198754.5 | protein_coding | OXCT2 | Down | 0.028382633 |
ENSG00000198830.6 | protein_coding | HMGN2 | Down | 0.034053765 |
ENSG00000198881.8 | protein_coding | ASB12 | Down | 0.042963309 |
ENSG00000198900.5 | protein_coding | TOP1 | Up | 0.036803174 |
ENSG00000198914.2 | protein_coding | POU3F3 | Down | 0.040875916 |
ENSG00000198945.3 | protein_coding | L3MBTL3 | Up | 0.040353636 |
ENSG00000198954.4 | protein_coding | KIAA1279 | Up | 0.014789739 |
ENSG00000198967.3 | protein_coding | OR10Z1 | Down | 0.02892736 |
ENSG00000203326.5 | protein_coding | ZNF525 | Up | 0.019737784 |
ENSG00000203684.5 | protein_coding | IBA57-AS1 | Down | 0.018777551 |
ENSG00000204807.1 | protein_coding | FAM27E2 | Down | 0.03035551 |
ENSG00000204983.8 | protein_coding | PRSS1 | Down | 0.022866585 |
ENSG00000205022.5 | protein_coding | PABPN1L | Down | 0.031277467 |
ENSG00000205409.3 | protein_coding | OR52E6 | Up | 0.039983429 |
ENSG00000212916.3 | protein_coding | MAP10 | Down | 0.04462334 |
ENSG00000213799.6 | protein_coding | ZNF845 | Up | 0.019737784 |
ENSG00000213828.1 | protein_coding | AC017028.1 | Down | 0.026268434 |
ENSG00000213888.2 | protein_coding | AC005003.1 | Down | 0.030588957 |
ENSG00000214253.4 | protein_coding | FIS1 | Down | 0.036530674 |
ENSG00000215612.5 | protein_coding | HMX1 | Down | 0.030423062 |
ENSG00000215695.1 | protein_coding | RSC1A1 | Up | 0.049559262 |
ENSG00000217930.3 | protein_coding | PAM16 | Down | 0.014976017 |
ENSG00000220848.4 | pseudogene | RPS18P9 | Down | 0.024449402 |
ENSG00000221837.4 | protein_coding | KRTAP10-9 | Down | 0.028181439 |
ENSG00000221840.2 | protein_coding | OR4A5 | Down | 0.013014151 |
ENSG00000221949.2 | protein_coding | C12orf61 | Down | 0.031578862 |
ENSG00000221954.2 | protein_coding | OR4C12 | Down | 0.010582213 |
ENSG00000224474.2 | protein_coding | AL355490.1 | Down | 0.026396768 |
ENSG00000228300.9 | protein_coding | C19orf24 | Down | 0.046090199 |
ENSG00000228336.1 | pseudogene | OR9H1P | Down | 0.031871552 |
ENSG00000230257.1 | antisense | NFE4 | Down | 0.021397338 |
ENSG00000232973.7 | antisense | CYP1B1-AS1 | Up | 0.031113526 |
ENSG00000233016.2 | antisense | SNHG7 | Down | 0.048765474 |
ENSG00000236773.1 | pseudogene | RP11-365O16.1 | Up | 0.015477893 |
ENSG00000237541.3 | protein_coding | HLA-DQA2 | Up | 0.020285026 |
ENSG00000238184.1 | processed_transcript | AC129929.5 | Down | 0.040824827 |
ENSG00000240720.3 | protein_coding | LRRD1 | Up | 0.006393288 |
ENSG00000240970.1 | pseudogene | RPL23AP64 | Down | 0.000986343 |
ENSG00000241360.1 | protein_coding | PDXP | Down | 0.04634582 |
ENSG00000244623.1 | protein_coding | OR2AE1 | Up | 0.001031314 |
ENSG00000245680.5 | protein_coding | ZNF585B | Up | 0.029339876 |
ENSG00000247077.2 | protein_coding | PGAM5 | Down | 0.03099048 |
ENSG00000251357.4 | protein_coding | AP000350.10 | Down | 0.044557673 |
ENSG00000254521.2 | protein_coding | SIGLEC12 | Up | 0.008757157 |
ENSG00000255769.3 | pseudogene | RP11-152F13.3 | Down | 0.031901455 |
ENSG00000255804.1 | protein_coding | OR6J1 | Up | 0.039222956 |
ENSG00000256235.1 | protein_coding | SMIM3 | Down | 0.036368455 |
ENSG00000256294.3 | protein_coding | ZNF225 | Up | 0.046416681 |
ENSG00000256453.1 | protein_coding | DND1 | Down | 0.023504581 |
ENSG00000256632.3 | protein_coding | RP13-672B3.2 | Down | 0.013611729 |
ENSG00000256683.2 | protein_coding | ZNF350 | Up | 0.021879236 |
ENSG00000257341.1 | protein_coding | CRIP1 | Down | 0.049720545 |
ENSG00000257702.3 | antisense | LBX2-AS1 | Down | 0.035045107 |
ENSG00000258405.5 | protein_coding | ZNF578 | Down | 0.021484975 |
ENSG00000258472.4 | protein_coding | RP11-192H23.4 | Down | 0.047286987 |
ENSG00000259571.1 | protein_coding | BLID | Down | 0.02508268 |
ENSG00000261713.2 | processed_transcript | SSTR5-AS1 | Down | 0.0167024 |
ENSG00000263002.3 | protein_coding | ZNF234 | Up | 0.035012293 |
ENSG00000263620.1 | protein_coding | RP11-599B13.6 | Down | 0.04868739 |
ENSG00000263809.1 | protein_coding | RP11-849F2.7 | Down | 0.025225822 |
ENSG00000264735.1 | lincRNA | RP11-498C9.17 | Down | 0.038254904 |
ENSG00000267022.1 | protein_coding | ZNF223 | Up | 0.005281393 |
ENSG00000267059.2 | protein_coding | UQCR11 | Down | 0.01521352 |
ENSG00000267360.2 | protein_coding | CTC-454I21.3 | Up | 0.036745114 |
ENSG00000267545.1 | protein_coding | AC005779.2 | Down | 0.028793638 |
ENSG00000267699.2 | protein_coding | RP11-729L2.2 | Up | 0.041545876 |
ENSG00000268614.1 | protein_coding | CTD-2207O23.10 | Down | 0.021055407 |
ENSG00000268797.1 | protein_coding | CTC-490E21.12 | Down | 0.003100426 |
ENSG00000269220.1 | lincRNA | LINC00528 | Down | 0.026695365 |
ENSG00000269636.1 | protein_coding | AC010441.1 | Down | 0.036368455 |
ENSG00000269858.1 | protein_coding | EGLN2 | Down | 0.003100426 |
ENSG00000271810.1 | protein_coding | RP11-426L16.10 | Down | 0.02868524 |
ENSG00000271959.1 | antisense | CTD-3064M3.7 | Down | 0.036335334 |
ENSG00000272906.1 | lincRNA | RP11-533E19.7 | Down | 0.011437491 |
ENSG00000273006.1 | lincRNA | RP11-314C9.2 | Up | 0.031113526 |
ID | gene_type | gene_symbol | status | p-value |
ENSG00000001630.11 | protein_coding | CYP51A1 | Up | 0.011965453 |
ENSG00000005075.11 | protein_coding | POLR2J | Down | 0.018005066 |
ENSG00000006282.15 | protein_coding | SPATA20 | Down | 0.030683456 |
ENSG00000007376.3 | protein_coding | RPUSD1 | Down | 0.046007757 |
ENSG00000007944.10 | protein_coding | MYLIP | Up | 0.012046727 |
ENSG00000008952.12 | protein_coding | SEC62 | Up | 0.04688829 |
ENSG00000014164.6 | protein_coding | ZC3H3 | Down | 0.035615575 |
ENSG00000020256.15 | protein_coding | ZFP64 | Up | 0.035853374 |
ENSG00000025770.14 | protein_coding | NCAPH2 | Down | 0.035381718 |
ENSG00000028277.16 | protein_coding | POU2F2 | Down | 0.025284331 |
ENSG00000029153.10 | protein_coding | ARNTL2 | Down | 0.010597798 |
ENSG00000032444.11 | protein_coding | PNPLA6 | Down | 0.015278704 |
ENSG00000034533.7 | protein_coding | ASTE1 | Up | 0.033013833 |
ENSG00000039123.11 | protein_coding | SKIV2L2 | Down | 0.048932372 |
ENSG00000047056.10 | protein_coding | WDR37 | Down | 0.034858116 |
ENSG00000050344.8 | protein_coding | NFE2L3 | Down | 0.033023849 |
ENSG00000051523.6 | protein_coding | CYBA | Down | 0.007486695 |
ENSG00000052723.7 | protein_coding | SIKE1 | Up | 0.045307581 |
ENSG00000052749.9 | protein_coding | RRP12 | Down | 0.026396768 |
ENSG00000054803.3 | protein_coding | CBLN4 | Down | 0.045582496 |
ENSG00000054967.8 | protein_coding | RELT | Down | 0.02019792 |
ENSG00000062598.13 | protein_coding | ELMO2 | Down | 0.025904437 |
ENSG00000064932.11 | protein_coding | SBNO2 | Down | 0.033420705 |
ENSG00000068024.12 | protein_coding | HDAC4 | Down | 0.048895859 |
ENSG00000068120.10 | protein_coding | COASY | Down | 0.009649617 |
ENSG00000069764.5 | protein_coding | PLA2G10 | Down | 0.003199821 |
ENSG00000070404.5 | protein_coding | FSTL3 | Down | 0.038524776 |
ENSG00000072778.15 | protein_coding | ACADVL | Down | 0.028472324 |
ENSG00000074842.3 | protein_coding | C19orf10 | Down | 0.00105098 |
ENSG00000077150.13 | protein_coding | NFKB2 | Down | 0.003922325 |
ENSG00000078804.8 | protein_coding | TP53INP2 | Down | 0.005193897 |
ENSG00000079393.16 | protein_coding | DUSP13 | Down | 0.024246462 |
ENSG00000079432.3 | protein_coding | CIC | Down | 0.019836428 |
ENSG00000081189.9 | protein_coding | MEF2C | Up | 0.047860138 |
ENSG00000083312.13 | protein_coding | TNPO1 | Up | 0.047418317 |
ENSG00000083844.6 | protein_coding | ZNF264 | Up | 0.010459153 |
ENSG00000087157.14 | protein_coding | PGS1 | Down | 0.042327569 |
ENSG00000087237.6 | protein_coding | CETP | Down | 0.032983061 |
ENSG00000087263.12 | protein_coding | OGFOD1 | Up | 0.043418236 |
ENSG00000087266.11 | protein_coding | SH3BP2 | Down | 0.030356676 |
ENSG00000087903.8 | protein_coding | RFX2 | Down | 0.020836355 |
ENSG00000088888.13 | protein_coding | MAVS | Down | 0.04764586 |
ENSG00000089123.11 | protein_coding | TASP1 | Up | 0.028910399 |
ENSG00000089248.6 | protein_coding | ERP29 | Down | 0.049446948 |
ENSG00000089351.10 | protein_coding | GRAMD1A | Down | 0.025055632 |
ENSG00000089639.6 | protein_coding | GMIP | Down | 0.014587545 |
ENSG00000090924.10 | protein_coding | PLEKHG2 | Down | 0.028301873 |
ENSG00000092929.7 | protein_coding | UNC13D | Down | 0.013293567 |
ENSG00000096088.12 | protein_coding | PGC | Down | 0.042062111 |
ENSG00000099139.9 | protein_coding | PCSK5 | Up | 0.027380288 |
ENSG00000099203.2 | protein_coding | TMED1 | Down | 0.028235039 |
ENSG00000099795.2 | protein_coding | NDUFB7 | Down | 0.040697141 |
ENSG00000099800.3 | protein_coding | TIMM13 | Down | 0.007324296 |
ENSG00000099821.9 | protein_coding | POLRMT | Down | 0.045124992 |
ENSG00000099958.10 | protein_coding | DERL3 | Down | 0.04060876 |
ENSG00000099991.12 | protein_coding | CABIN1 | Down | 0.034876772 |
ENSG00000100028.7 | protein_coding | SNRPD3 | Down | 0.044318218 |
ENSG00000100055.16 | protein_coding | CYTH4 | Down | 0.018939808 |
ENSG00000100092.16 | protein_coding | SH3BP1 | Down | 0.04634582 |
ENSG00000100242.11 | protein_coding | SUN2 | Down | 0.010030177 |
ENSG00000100290.2 | protein_coding | BIK | Down | 0.007141866 |
ENSG00000100403.10 | protein_coding | ZC3H7B | Down | 0.0410179 |
ENSG00000100429.13 | protein_coding | HDAC10 | Down | 0.033877634 |
ENSG00000100532.7 | protein_coding | CGRRF1 | Up | 0.013688977 |
ENSG00000100802.10 | protein_coding | C14orf93 | Down | 0.033127459 |
ENSG00000100906.6 | protein_coding | NFKBIA | Down | 0.005259074 |
ENSG00000101220.13 | protein_coding | C20orf27 | Down | 0.034454114 |
ENSG00000101473.12 | protein_coding | ACOT8 | Down | 0.016141884 |
ENSG00000101624.6 | protein_coding | CEP76 | Up | 0.013187998 |
ENSG00000101665.4 | protein_coding | SMAD7 | Down | 0.017676506 |
ENSG00000101997.8 | protein_coding | CCDC22 | Down | 0.039248809 |
ENSG00000102078.11 | protein_coding | SLC25A14 | Down | 0.039031376 |
ENSG00000102786.10 | protein_coding | INTS6 | Up | 0.044913283 |
ENSG00000102981.5 | protein_coding | PARD6A | Down | 0.041910914 |
ENSG00000103024.3 | protein_coding | NME3 | Down | 0.027773943 |
ENSG00000103047.3 | protein_coding | TANGO6 | Down | 0.049704948 |
ENSG00000103227.14 | protein_coding | LMF1 | Down | 0.022119939 |
ENSG00000103426.8 | protein_coding | CORO7-PAM16 | Down | 0.014976017 |
ENSG00000103449.7 | protein_coding | SALL1 | Down | 0.041904944 |
ENSG00000103653.12 | protein_coding | CSK | Down | 0.010534323 |
ENSG00000104856.9 | protein_coding | RELB | Down | 0.015505238 |
ENSG00000104973.10 | protein_coding | MED25 | Down | 0.041089276 |
ENSG00000105063.14 | protein_coding | PPP6R1 | Down | 0.022586612 |
ENSG00000105135.11 | protein_coding | ILVBL | Down | 0.034386207 |
ENSG00000105497.3 | protein_coding | ZNF175 | Up | 0.013075356 |
ENSG00000105643.5 | protein_coding | ARRDC2 | Down | 0.039560218 |
ENSG00000105656.8 | protein_coding | ELL | Down | 0.035373441 |
ENSG00000106268.11 | protein_coding | NUDT1 | Down | 0.03304039 |
ENSG00000107362.9 | protein_coding | ABHD17B | Up | 0.02410498 |
ENSG00000107443.11 | protein_coding | CCNJ | Down | 0.029817026 |
ENSG00000107984.5 | protein_coding | DKK1 | Down | 0.049671011 |
ENSG00000108179.9 | protein_coding | PPIF | Down | 0.044960461 |
ENSG00000108518.7 | protein_coding | PFN1 | Down | 0.045797043 |
ENSG00000108840.11 | protein_coding | HDAC5 | Down | 0.01595402 |
ENSG00000108950.7 | protein_coding | FAM20A | Down | 0.012800605 |
ENSG00000109103.7 | protein_coding | UNC119 | Down | 0.03809287 |
ENSG00000109736.10 | protein_coding | MFSD10 | Down | 0.043758832 |
ENSG00000109917.6 | protein_coding | ZNF259 | Down | 0.042419417 |
ENSG00000109944.6 | protein_coding | C11orf63 | Down | 0.036421384 |
ENSG00000110063.4 | protein_coding | DCPS | Down | 0.044521345 |
ENSG00000110080.14 | protein_coding | ST3GAL4 | Down | 0.009630116 |
ENSG00000110090.8 | protein_coding | CPT1A | Down | 0.040715917 |
ENSG00000110619.12 | protein_coding | CARS | Down | 0.040080075 |
ENSG00000110931.14 | protein_coding | CAMKK2 | Down | 0.041840274 |
ENSG00000112149.5 | protein_coding | CD83 | Down | 0.021210986 |
ENSG00000112276.9 | protein_coding | BVES | Up | 0.013370759 |
ENSG00000112312.5 | protein_coding | GMNN | Down | 0.047237299 |
ENSG00000114650.14 | protein_coding | SCAP | Down | 0.032838904 |
ENSG00000115073.6 | protein_coding | ACTR1B | Down | 0.003540043 |
ENSG00000116017.6 | protein_coding | ARID3A | Down | 0.038762022 |
ENSG00000116670.10 | protein_coding | MAD2L2 | Down | 0.043537769 |
ENSG00000116852.10 | protein_coding | KIF21B | Down | 0.049989022 |
ENSG00000116871.11 | protein_coding | MAP7D1 | Down | 0.03998345 |
ENSG00000116985.6 | protein_coding | BMP8B | Down | 0.028382633 |
ENSG00000117600.8 | protein_coding | LPPR4 | Down | 0.046876942 |
ENSG00000118432.11 | protein_coding | CNR1 | Down | 0.017637126 |
ENSG00000118600.7 | protein_coding | TMEM5 | Down | 0.014944131 |
ENSG00000119013.4 | protein_coding | NDUFB3 | Down | 0.040614225 |
ENSG00000119121.17 | protein_coding | TRPM6 | Down | 0.048825853 |
ENSG00000119669.3 | protein_coding | IRF2BPL | Down | 0.005359191 |
ENSG00000120217.9 | protein_coding | CD274 | Down | 0.043686299 |
ENSG00000120699.8 | protein_coding | EXOSC8 | Up | 0.032500661 |
ENSG00000122203.10 | protein_coding | KIAA1191 | Up | 0.048031425 |
ENSG00000122490.14 | protein_coding | PQLC1 | Down | 0.015129367 |
ENSG00000122824.6 | protein_coding | NUDT10 | Down | 0.033200739 |
ENSG00000123094.11 | protein_coding | RASSF8 | Down | 0.029465179 |
ENSG00000123358.15 | protein_coding | NR4A1 | Down | 0.01086372 |
ENSG00000123576.5 | protein_coding | ESX1 | Down | 0.030485823 |
ENSG00000123689.5 | protein_coding | G0S2 | Down | 0.009066817 |
ENSG00000123989.9 | protein_coding | CHPF | Down | 0.040386914 |
ENSG00000125657.3 | protein_coding | TNFSF9 | Down | 0.049043875 |
ENSG00000125755.14 | protein_coding | SYMPK | Down | 0.049805392 |
ENSG00000125817.7 | protein_coding | CENPB | Down | 0.042137733 |
ENSG00000125945.10 | protein_coding | ZNF436 | Up | 0.047416168 |
ENSG00000125968.7 | protein_coding | ID1 | Down | 0.04512393 |
ENSG00000127054.14 | protein_coding | CPSF3L | Down | 0.04296422 |
ENSG00000127124.9 | protein_coding | HIVEP3 | Down | 0.000294073 |
ENSG00000127483.13 | protein_coding | HP1BP3 | Up | 0.047813073 |
ENSG00000127540.7 | protein_coding | UQCR11 | Down | 0.030739395 |
ENSG00000127588.4 | protein_coding | GNG13 | Down | 0.039828869 |
ENSG00000128059.4 | protein_coding | PPAT | Up | 0.006604019 |
ENSG00000128228.4 | protein_coding | SDF2L1 | Down | 0.003273018 |
ENSG00000128271.15 | protein_coding | ADORA2A | Down | 0.041530393 |
ENSG00000128309.12 | protein_coding | MPST | Down | 0.037538756 |
ENSG00000128694.7 | protein_coding | OSGEPL1 | Up | 0.036634744 |
ENSG00000129562.6 | protein_coding | DAD1 | Up | 0.039222956 |
ENSG00000129667.8 | protein_coding | RHBDF2 | Down | 0.030796176 |
ENSG00000129744.2 | protein_coding | ART1 | Down | 0.001540155 |
ENSG00000129968.11 | protein_coding | ABHD17A | Down | 0.043478487 |
ENSG00000129993.10 | protein_coding | CBFA2T3 | Down | 0.031277467 |
ENSG00000130522.4 | protein_coding | JUND | Down | 0.022542768 |
ENSG00000130766.4 | protein_coding | SESN2 | Down | 0.013640369 |
ENSG00000130935.5 | protein_coding | NOL11 | Up | 0.044393023 |
ENSG00000131142.9 | protein_coding | CCL25 | Down | 0.038185878 |
ENSG00000131171.8 | protein_coding | SH3BGRL | Up | 0.022819598 |
ENSG00000131653.8 | protein_coding | TRAF7 | Down | 0.036084131 |
ENSG00000131849.10 | protein_coding | ZNF132 | Down | 0.028132404 |
ENSG00000132275.6 | protein_coding | RRP8 | Down | 0.010570164 |
ENSG00000132507.13 | protein_coding | EIF5A | Down | 0.024112694 |
ENSG00000133606.6 | protein_coding | MKRN1 | Up | 0.041317442 |
ENSG00000133805.11 | protein_coding | AMPD3 | Down | 0.046431783 |
ENSG00000134107.4 | protein_coding | BHLHE40 | Down | 0.038744934 |
ENSG00000135047.10 | protein_coding | CTSL | Down | 0.0255056 |
ENSG00000135390.13 | protein_coding | ATP5G2 | Down | 0.02929579 |
ENSG00000136048.9 | protein_coding | DRAM1 | Down | 0.02091681 |
ENSG00000136122.11 | protein_coding | BORA | Up | 0.036657962 |
ENSG00000136738.10 | protein_coding | STAM | Up | 0.0389495 |
ENSG00000136840.14 | protein_coding | ST6GALNAC4 | Down | 0.016088565 |
ENSG00000136877.10 | protein_coding | FPGS | Down | 0.023271049 |
ENSG00000137124.6 | protein_coding | ALDH1B1 | Up | 0.03438067 |
ENSG00000137193.9 | protein_coding | PIM1 | Down | 0.009876567 |
ENSG00000137218.6 | protein_coding | FRS3 | Down | 0.042976435 |
ENSG00000137309.15 | protein_coding | HMGA1 | Down | 0.021493168 |
ENSG00000137312.10 | protein_coding | FLOT1 | Down | 0.039597455 |
ENSG00000137409.14 | protein_coding | MTCH1 | Down | 0.040766375 |
ENSG00000138382.9 | protein_coding | METTL5 | Up | 0.038252044 |
ENSG00000139053.2 | protein_coding | PDE6H | Down | 0.0207692 |
ENSG00000139610.1 | protein_coding | CELA1 | Up | 0.038317749 |
ENSG00000139668.7 | protein_coding | WDFY2 | Down | 0.019753557 |
ENSG00000140044.8 | protein_coding | JDP2 | Down | 0.036877537 |
ENSG00000140553.12 | protein_coding | UNC45A | Down | 0.042323486 |
ENSG00000140612.9 | protein_coding | SEC11A | Down | 0.017070625 |
ENSG00000140848.12 | protein_coding | CPNE2 | Down | 0.035194471 |
ENSG00000140941.8 | protein_coding | MAP1LC3B | Up | 0.010046471 |
ENSG00000141012.8 | protein_coding | GALNS | Down | 0.019933327 |
ENSG00000141034.5 | protein_coding | GID4 | Up | 0.036023887 |
ENSG00000141198.9 | protein_coding | TOM1L1 | Down | 0.043796522 |
ENSG00000141252.15 | protein_coding | VPS53 | Down | 0.020821593 |
ENSG00000141505.7 | protein_coding | ASGR1 | Down | 0.049034425 |
ENSG00000141646.9 | protein_coding | SMAD4 | Up | 0.041545876 |
ENSG00000141867.13 | protein_coding | BRD4 | Down | 0.040247622 |
ENSG00000142408.2 | protein_coding | CACNG8 | Down | 0.035337949 |
ENSG00000142538.1 | protein_coding | PTH2 | Down | 0.028959056 |
ENSG00000143549.15 | protein_coding | TPM3 | Down | 0.005503969 |
ENSG00000144120.8 | protein_coding | TMEM177 | Up | 0.022405325 |
ENSG00000144182.12 | protein_coding | LIPT1 | Up | 0.008805655 |
ENSG00000144381.12 | protein_coding | HSPD1 | Down | 0.048940793 |
ENSG00000144468.12 | protein_coding | RHBDD1 | Up | 0.048354944 |
ENSG00000144655.10 | protein_coding | CSRNP1 | Down | 0.03240296 |
ENSG00000144791.5 | protein_coding | LIMD1 | Down | 0.04142983 |
ENSG00000144802.7 | protein_coding | NFKBIZ | Down | 0.015266617 |
ENSG00000145901.10 | protein_coding | TNIP1 | Down | 0.031453499 |
ENSG00000146047.4 | protein_coding | HIST1H2BA | Down | 0.005162611 |
ENSG00000146232.10 | protein_coding | NFKBIE | Down | 0.020469032 |
ENSG00000146828.13 | protein_coding | SLC12A9 | Down | 0.042442305 |
ENSG00000146833.11 | protein_coding | TRIM4 | Up | 0.001031314 |
ENSG00000146856.10 | protein_coding | AGBL3 | Down | 0.044096515 |
ENSG00000147419.12 | protein_coding | CCDC25 | Up | 0.027233589 |
ENSG00000148187.13 | protein_coding | MRRF | Up | 0.01456252 |
ENSG00000148335.10 | protein_coding | NTMT1 | Down | 0.033476509 |
ENSG00000148602.5 | protein_coding | LRIT1 | Down | 0.038378276 |
ENSG00000148737.11 | protein_coding | TCF7L2 | Down | 0.03027628 |
ENSG00000148835.9 | protein_coding | TAF5 | Up | 0.017608599 |
ENSG00000149115.9 | protein_coding | TNKS1BP1 | Down | 0.031874191 |
ENSG00000149503.8 | protein_coding | INCENP | Down | 0.039618744 |
ENSG00000149792.4 | protein_coding | MRPL49 | Down | 0.014313227 |
ENSG00000149823.3 | protein_coding | VPS51 | Down | 0.034228234 |
ENSG00000149932.12 | protein_coding | TMEM219 | Down | 0.042059762 |
ENSG00000149968.7 | protein_coding | MMP3 | Down | 0.007039867 |
ENSG00000151093.3 | protein_coding | OXSM | Up | 0.032113374 |
ENSG00000151490.9 | protein_coding | PTPRO | Down | 0.0169847 |
ENSG00000151631.7 | pseudogene | AKR1C6P | Down | 0.004333285 |
ENSG00000151651.11 | protein_coding | ADAM8 | Down | 0.034809828 |
ENSG00000151726.9 | protein_coding | ACSL1 | Down | 0.040278414 |
ENSG00000152207.3 | protein_coding | CYSLTR2 | Up | 0.031075114 |
ENSG00000152382.5 | protein_coding | TADA1 | Down | 0.034551617 |
ENSG00000153140.4 | protein_coding | CETN3 | Up | 0.028301839 |
ENSG00000153207.10 | protein_coding | AHCTF1 | Down | 0.014567315 |
ENSG00000153266.8 | protein_coding | FEZF2 | Down | 0.036977261 |
ENSG00000153487.11 | protein_coding | ING1 | Down | 0.015188879 |
ENSG00000154370.9 | protein_coding | TRIM11 | Down | 0.018777551 |
ENSG00000154447.10 | protein_coding | SH3RF1 | Down | 0.009674391 |
ENSG00000154639.14 | protein_coding | CXADR | Down | 0.00685789 |
ENSG00000154781.11 | protein_coding | CCDC174 | Down | 0.049642675 |
ENSG00000154839.5 | protein_coding | SKA1 | Down | 0.046702852 |
ENSG00000154978.8 | protein_coding | VOPP1 | Down | 0.030307149 |
ENSG00000155438.7 | protein_coding | MKI67IP | Down | 0.03414805 |
ENSG00000155918.3 | protein_coding | RAET1L | Down | 0.047085308 |
ENSG00000156172.5 | protein_coding | C8orf37 | Up | 0.015241022 |
ENSG00000156508.13 | protein_coding | EEF1A1 | Down | 0.029958858 |
ENSG00000157227.8 | protein_coding | MMP14 | Down | 0.018623306 |
ENSG00000157326.14 | protein_coding | DHRS4 | Down | 0.047099568 |
ENSG00000157456.3 | protein_coding | CCNB2 | Down | 0.012184904 |
ENSG00000157637.8 | protein_coding | SLC38A10 | Down | 0.026642558 |
ENSG00000158014.10 | protein_coding | SLC30A2 | Down | 0.026315721 |
ENSG00000158483.11 | protein_coding | FAM86C1 | Down | 0.00975309 |
ENSG00000158497.2 | protein_coding | HMHB1 | Down | 0.040327646 |
ENSG00000158555.10 | protein_coding | GDPD5 | Down | 0.034837228 |
ENSG00000159069.9 | protein_coding | FBXW5 | Down | 0.01907369 |
ENSG00000159885.9 | protein_coding | ZNF222 | Up | 0.01199585 |
ENSG00000160111.8 | protein_coding | CPAMD8 | Down | 0.049299496 |
ENSG00000160179.14 | protein_coding | ABCG1 | Up | 0.022299138 |
ENSG00000160180.14 | protein_coding | TFF3 | Down | 0.042914253 |
ENSG00000160214.8 | protein_coding | RRP1 | Down | 0.049738569 |
ENSG00000160796.12 | protein_coding | NBEAL2 | Down | 0.035908182 |
ENSG00000160883.6 | protein_coding | HK3 | Down | 0.044846812 |
ENSG00000160888.6 | protein_coding | IER2 | Down | 0.027407148 |
ENSG00000161640.11 | protein_coding | SIGLEC11 | Down | 0.042121328 |
ENSG00000161791.9 | protein_coding | FMNL3 | Down | 0.011008036 |
ENSG00000162086.10 | protein_coding | ZNF75A | Up | 0.019226079 |
ENSG00000162302.8 | protein_coding | RPS6KA4 | Down | 0.048212217 |
ENSG00000162377.4 | protein_coding | SELRC1 | Up | 0.047979072 |
ENSG00000162413.12 | protein_coding | KLHL21 | Down | 0.048454407 |
ENSG00000162522.6 | protein_coding | KIAA1522 | Down | 0.016306649 |
ENSG00000162783.8 | protein_coding | IER5 | Down | 0.021919966 |
ENSG00000162913.9 | protein_coding | C1orf145 | Down | 0.018777551 |
ENSG00000162927.9 | protein_coding | PUS10 | Up | 0.04033551 |
ENSG00000162931.7 | protein_coding | TRIM17 | Down | 0.023650474 |
ENSG00000163162.4 | protein_coding | RNF149 | Down | 0.025666014 |
ENSG00000163239.8 | protein_coding | TDRD10 | Down | 0.043592404 |
ENSG00000163389.6 | protein_coding | POGLUT1 | Up | 0.022081661 |
ENSG00000163947.7 | protein_coding | ARHGEF3 | Up | 0.010264753 |
ENSG00000164105.3 | protein_coding | SAP30 | Up | 0.046694723 |
ENSG00000164342.8 | protein_coding | TLR3 | Up | 0.003277358 |
ENSG00000164402.9 | protein_coding | 8-Sep | Down | 0.024177765 |
ENSG00000164411.6 | protein_coding | GJB7 | Down | 0.026747052 |
ENSG00000165029.11 | protein_coding | ABCA1 | Up | 0.010492577 |
ENSG00000165188.9 | protein_coding | RNF183 | Down | 0.010089847 |
ENSG00000165406.11 | protein_coding | 8-Mar | Up | 0.047180108 |
ENSG00000165512.4 | protein_coding | ZNF22 | Up | 0.033208827 |
ENSG00000165782.6 | protein_coding | TMEM55B | Down | 0.022652362 |
ENSG00000165886.4 | protein_coding | UBTD1 | Down | 0.014531961 |
ENSG00000165915.9 | protein_coding | SLC39A13 | Down | 0.024410393 |
ENSG00000166265.7 | protein_coding | CYYR1 | Down | 0.037657919 |
ENSG00000166363.4 | protein_coding | OR10A5 | Up | 0.018183215 |
ENSG00000166446.10 | protein_coding | CDYL2 | Down | 0.011853205 |
ENSG00000166451.9 | protein_coding | CENPN | Up | 0.040214671 |
ENSG00000166452.7 | protein_coding | AKIP1 | Up | 0.048924644 |
ENSG00000166938.8 | protein_coding | DIS3L | Up | 0.036638539 |
ENSG00000166974.8 | protein_coding | MAPRE2 | Up | 0.048488455 |
ENSG00000167110.12 | protein_coding | GOLGA2 | Down | 0.023994018 |
ENSG00000167186.6 | protein_coding | COQ7 | Up | 0.000296612 |
ENSG00000167333.8 | protein_coding | TRIM68 | Up | 0.039246931 |
ENSG00000167394.8 | protein_coding | ZNF668 | Down | 0.015855291 |
ENSG00000167414.4 | protein_coding | GNG8 | Down | 0.0489265 |
ENSG00000167555.9 | protein_coding | ZNF528 | Up | 0.002098542 |
ENSG00000167604.9 | protein_coding | NFKBID | Down | 0.04908962 |
ENSG00000167657.7 | protein_coding | DAPK3 | Down | 0.045503987 |
ENSG00000168066.16 | protein_coding | SF1 | Down | 0.047265519 |
ENSG00000168310.6 | protein_coding | IRF2 | Up | 0.044253405 |
ENSG00000168594.11 | protein_coding | ADAM29 | Down | 0.003444856 |
ENSG00000168803.10 | protein_coding | ADAL | Up | 0.021101681 |
ENSG00000168818.5 | protein_coding | STX18 | Up | 0.022001045 |
ENSG00000168967.10 | pseudogene | PMCHL1 | Down | 0.008435479 |
ENSG00000169105.6 | protein_coding | CHST14 | Down | 0.024636798 |
ENSG00000169379.11 | protein_coding | ARL13B | Up | 0.010209654 |
ENSG00000169393.4 | protein_coding | ELSPBP1 | Down | 0.014495593 |
ENSG00000169429.6 | protein_coding | IL8 | Down | 0.028420528 |
ENSG00000170265.7 | protein_coding | ZNF282 | Down | 0.042671468 |
ENSG00000170369.3 | protein_coding | CST2 | Down | 0.041604924 |
ENSG00000170445.8 | protein_coding | HARS | Down | 0.029725289 |
ENSG00000170779.10 | protein_coding | CDCA4 | Down | 0.028547735 |
ENSG00000170837.2 | protein_coding | GPR27 | Down | 0.035077639 |
ENSG00000170891.6 | protein_coding | CYTL1 | Down | 0.032834474 |
ENSG00000171130.13 | protein_coding | ATP6V0E2 | Down | 0.039784296 |
ENSG00000171223.4 | protein_coding | JUNB | Down | 0.038177458 |
ENSG00000171357.5 | protein_coding | LURAP1 | Down | 0.033100787 |
ENSG00000171467.11 | protein_coding | ZNF318 | Down | 0.044327394 |
ENSG00000171530.9 | protein_coding | TBCA | Down | 0.009876223 |
ENSG00000171570.6 | protein_coding | RAB4B-EGLN2 | Down | 0.010162577 |
ENSG00000171657.5 | protein_coding | GPR82 | Up | 0.002688119 |
ENSG00000171766.11 | protein_coding | GATM | Up | 0.035375608 |
ENSG00000171827.6 | protein_coding | ZNF570 | Up | 0.016772935 |
ENSG00000171861.6 | protein_coding | RNMTL1 | Down | 0.041110394 |
ENSG00000171942.3 | protein_coding | OR10H2 | Down | 0.01466908 |
ENSG00000172208.3 | protein_coding | OR4X2 | Up | 0.038143037 |
ENSG00000172322.9 | protein_coding | CLEC12A | Up | 0.008850366 |
ENSG00000172375.8 | protein_coding | C2CD2L | Down | 0.012079432 |
ENSG00000172428.6 | protein_coding | MYEOV2 | Down | 0.025783713 |
ENSG00000173020.6 | protein_coding | ADRBK1 | Down | 0.043055525 |
ENSG00000173153.9 | protein_coding | ESRRA | Down | 0.008541886 |
ENSG00000173653.3 | protein_coding | RCE1 | Down | 0.030649495 |
ENSG00000173715.11 | protein_coding | C11orf80 | Down | 0.030649495 |
ENSG00000173812.6 | protein_coding | EIF1 | Down | 0.0170157 |
ENSG00000173846.8 | protein_coding | PLK3 | Down | 0.015509349 |
ENSG00000174600.9 | protein_coding | CMKLR1 | Up | 0.044214317 |
ENSG00000174886.8 | protein_coding | NDUFA11 | Down | 0.022192837 |
ENSG00000175130.6 | protein_coding | MARCKSL1 | Down | 0.038863502 |
ENSG00000175728.2 | protein_coding | C11orf44 | Up | 0.045296195 |
ENSG00000175874.5 | protein_coding | CREG2 | Down | 0.01537001 |
ENSG00000176485.6 | protein_coding | PLA2G16 | Down | 0.024862828 |
ENSG00000176563.5 | protein_coding | CNTD1 | Down | 0.016145384 |
ENSG00000176619.6 | protein_coding | LMNB2 | Down | 0.030268302 |
ENSG00000176624.9 | protein_coding | MEX3C | Up | 0.029845716 |
ENSG00000176714.9 | protein_coding | CCDC121 | Down | 0.022706601 |
ENSG00000176749.4 | protein_coding | CDK5R1 | Down | 0.04550598 |
ENSG00000176845.8 | protein_coding | METRNL | Down | 0.010677297 |
ENSG00000176894.5 | protein_coding | PXMP2 | Down | 0.013611729 |
ENSG00000176974.13 | protein_coding | SHMT1 | Up | 0.049830843 |
ENSG00000177051.5 | protein_coding | FBXO46 | Down | 0.047542187 |
ENSG00000177169.5 | protein_coding | ULK1 | Down | 0.016389135 |
ENSG00000177666.11 | protein_coding | PNPLA2 | Down | 0.043015592 |
ENSG00000178229.7 | protein_coding | ZNF543 | Up | 0.010459153 |
ENSG00000178951.4 | protein_coding | ZBTB7A | Down | 0.019056001 |
ENSG00000178980.10 | protein_coding | SEPW1 | Down | 0.016556027 |
ENSG00000179029.10 | protein_coding | TMEM107 | Down | 0.005945219 |
ENSG00000180008.8 | protein_coding | SOCS4 | Up | 0.039599073 |
ENSG00000180332.5 | protein_coding | KCTD4 | Down | 0.022125509 |
ENSG00000180346.2 | protein_coding | TIGD2 | Up | 0.037693259 |
ENSG00000180539.4 | protein_coding | C9orf139 | Down | 0.01622825 |
ENSG00000180725.4 | pseudogene | AC015871.1 | Down | 0.042511409 |
ENSG00000181378.9 | protein_coding | CCDC108 | Down | 0.038235948 |
ENSG00000181791.1 | pseudogene | AC009041.1 | Down | 0.0167024 |
ENSG00000182118.5 | protein_coding | FAM89A | Down | 0.002426708 |
ENSG00000182333.10 | protein_coding | LIPF | Down | 0.018324226 |
ENSG00000182368.4 | protein_coding | FAM27A | Down | 0.03035551 |
ENSG00000182541.13 | protein_coding | LIMK2 | Down | 0.041747303 |
ENSG00000182782.7 | protein_coding | HCAR2 | Down | 0.046508935 |
ENSG00000182841.8 | pseudogene | RRP7B | Down | 0.04819365 |
ENSG00000183019.3 | protein_coding | C19orf59 | Down | 0.031283921 |
ENSG00000183020.9 | protein_coding | AP2A2 | Down | 0.000692239 |
ENSG00000183087.10 | protein_coding | GAS6 | Down | 0.026705611 |
ENSG00000183648.5 | protein_coding | NDUFB1 | Down | 0.00863385 |
ENSG00000183696.9 | protein_coding | UPP1 | Down | 0.021571741 |
ENSG00000183709.7 | protein_coding | IFNL2 | Up | 0.001410201 |
ENSG00000183723.8 | protein_coding | CMTM4 | Down | 0.047938821 |
ENSG00000183779.5 | protein_coding | ZNF703 | Down | 0.033061055 |
ENSG00000184110.10 | protein_coding | EIF3C | Down | 0.008205594 |
ENSG00000184517.7 | protein_coding | ZFP1 | Up | 0.045781579 |
ENSG00000184619.3 | protein_coding | KRBA2 | Down | 0.025225822 |
ENSG00000184897.4 | protein_coding | H1FX | Down | 0.014748614 |
ENSG00000184922.9 | protein_coding | FMNL1 | Down | 0.01035533 |
ENSG00000184990.8 | protein_coding | SIVA1 | Down | 0.039061925 |
ENSG00000185641.5 | pseudogene | CTD-2287O16.1 | Down | 0.043078899 |
ENSG00000185787.10 | protein_coding | MORF4L1 | Down | 0.047667026 |
ENSG00000185972.4 | protein_coding | CCIN | Down | 0.044995407 |
ENSG00000186106.7 | protein_coding | ANKRD46 | Up | 0.042420836 |
ENSG00000186111.4 | protein_coding | PIP5K1C | Down | 0.042436011 |
ENSG00000186281.8 | protein_coding | GPAT2 | Down | 0.049178673 |
ENSG00000186594.8 | lincRNA | MIR22HG | Down | 0.017511035 |
ENSG00000186635.10 | protein_coding | ARAP1 | Down | 0.015438232 |
ENSG00000186795.1 | protein_coding | KCNK18 | Down | 0.020856507 |
ENSG00000187630.10 | protein_coding | DHRS4L2 | Down | 0.047099568 |
ENSG00000187688.10 | protein_coding | TRPV2 | Down | 0.003137583 |
ENSG00000188379.5 | protein_coding | IFNA2 | Down | 0.004976237 |
ENSG00000189042.9 | protein_coding | ZNF567 | Up | 0.015774858 |
ENSG00000189114.6 | protein_coding | BLOC1S3 | Down | 0.028793638 |
ENSG00000189332.4 | protein_coding | RP11-113D6.10 | Down | 0.029127001 |
ENSG00000196152.6 | protein_coding | ZNF79 | Up | 0.002879344 |
ENSG00000196331.5 | protein_coding | HIST1H2BO | Down | 0.025404112 |
ENSG00000196358.6 | protein_coding | NTNG2 | Down | 0.042750195 |
ENSG00000196371.2 | protein_coding | FUT4 | Down | 0.011093343 |
ENSG00000196415.5 | protein_coding | PRTN3 | Down | 0.035149627 |
ENSG00000196417.8 | protein_coding | ZNF765 | Up | 0.005727824 |
ENSG00000196652.7 | protein_coding | ZKSCAN5 | Up | 0.016188909 |
ENSG00000196668.3 | processed_transcript | LINC00173 | Down | 0.003213639 |
ENSG00000196693.10 | protein_coding | ZNF33B | Up | 0.010400288 |
ENSG00000196843.11 | protein_coding | ARID5A | Down | 0.023473729 |
ENSG00000196917.4 | protein_coding | HCAR1 | Down | 0.023288331 |
ENSG00000196981.2 | protein_coding | WDR5B | Up | 0.010645113 |
ENSG00000197016.7 | protein_coding | ZNF470 | Up | 0.021502777 |
ENSG00000197312.7 | protein_coding | DDI2 | Up | 0.049559262 |
ENSG00000197321.10 | protein_coding | SVIL | Down | 0.029323831 |
ENSG00000197919.3 | protein_coding | IFNA1 | Down | 0.011997429 |
ENSG00000198055.6 | protein_coding | GRK6 | Down | 0.018617852 |
ENSG00000198081.6 | protein_coding | ZBTB14 | Up | 0.03828373 |
ENSG00000198324.10 | protein_coding | FAM109A | Down | 0.015387399 |
ENSG00000198445.3 | protein_coding | CCT8L2 | Down | 0.008263414 |
ENSG00000198464.9 | protein_coding | ZNF480 | Up | 0.04695864 |
ENSG00000198471.1 | protein_coding | RTP2 | Up | 0.026862674 |
ENSG00000198551.5 | protein_coding | ZNF627 | Up | 0.007529781 |
ENSG00000198754.5 | protein_coding | OXCT2 | Down | 0.028382633 |
ENSG00000198830.6 | protein_coding | HMGN2 | Down | 0.034053765 |
ENSG00000198881.8 | protein_coding | ASB12 | Down | 0.042963309 |
ENSG00000198900.5 | protein_coding | TOP1 | Up | 0.036803174 |
ENSG00000198914.2 | protein_coding | POU3F3 | Down | 0.040875916 |
ENSG00000198945.3 | protein_coding | L3MBTL3 | Up | 0.040353636 |
ENSG00000198954.4 | protein_coding | KIAA1279 | Up | 0.014789739 |
ENSG00000198967.3 | protein_coding | OR10Z1 | Down | 0.02892736 |
ENSG00000203326.5 | protein_coding | ZNF525 | Up | 0.019737784 |
ENSG00000203684.5 | protein_coding | IBA57-AS1 | Down | 0.018777551 |
ENSG00000204807.1 | protein_coding | FAM27E2 | Down | 0.03035551 |
ENSG00000204983.8 | protein_coding | PRSS1 | Down | 0.022866585 |
ENSG00000205022.5 | protein_coding | PABPN1L | Down | 0.031277467 |
ENSG00000205409.3 | protein_coding | OR52E6 | Up | 0.039983429 |
ENSG00000212916.3 | protein_coding | MAP10 | Down | 0.04462334 |
ENSG00000213799.6 | protein_coding | ZNF845 | Up | 0.019737784 |
ENSG00000213828.1 | protein_coding | AC017028.1 | Down | 0.026268434 |
ENSG00000213888.2 | protein_coding | AC005003.1 | Down | 0.030588957 |
ENSG00000214253.4 | protein_coding | FIS1 | Down | 0.036530674 |
ENSG00000215612.5 | protein_coding | HMX1 | Down | 0.030423062 |
ENSG00000215695.1 | protein_coding | RSC1A1 | Up | 0.049559262 |
ENSG00000217930.3 | protein_coding | PAM16 | Down | 0.014976017 |
ENSG00000220848.4 | pseudogene | RPS18P9 | Down | 0.024449402 |
ENSG00000221837.4 | protein_coding | KRTAP10-9 | Down | 0.028181439 |
ENSG00000221840.2 | protein_coding | OR4A5 | Down | 0.013014151 |
ENSG00000221949.2 | protein_coding | C12orf61 | Down | 0.031578862 |
ENSG00000221954.2 | protein_coding | OR4C12 | Down | 0.010582213 |
ENSG00000224474.2 | protein_coding | AL355490.1 | Down | 0.026396768 |
ENSG00000228300.9 | protein_coding | C19orf24 | Down | 0.046090199 |
ENSG00000228336.1 | pseudogene | OR9H1P | Down | 0.031871552 |
ENSG00000230257.1 | antisense | NFE4 | Down | 0.021397338 |
ENSG00000232973.7 | antisense | CYP1B1-AS1 | Up | 0.031113526 |
ENSG00000233016.2 | antisense | SNHG7 | Down | 0.048765474 |
ENSG00000236773.1 | pseudogene | RP11-365O16.1 | Up | 0.015477893 |
ENSG00000237541.3 | protein_coding | HLA-DQA2 | Up | 0.020285026 |
ENSG00000238184.1 | processed_transcript | AC129929.5 | Down | 0.040824827 |
ENSG00000240720.3 | protein_coding | LRRD1 | Up | 0.006393288 |
ENSG00000240970.1 | pseudogene | RPL23AP64 | Down | 0.000986343 |
ENSG00000241360.1 | protein_coding | PDXP | Down | 0.04634582 |
ENSG00000244623.1 | protein_coding | OR2AE1 | Up | 0.001031314 |
ENSG00000245680.5 | protein_coding | ZNF585B | Up | 0.029339876 |
ENSG00000247077.2 | protein_coding | PGAM5 | Down | 0.03099048 |
ENSG00000251357.4 | protein_coding | AP000350.10 | Down | 0.044557673 |
ENSG00000254521.2 | protein_coding | SIGLEC12 | Up | 0.008757157 |
ENSG00000255769.3 | pseudogene | RP11-152F13.3 | Down | 0.031901455 |
ENSG00000255804.1 | protein_coding | OR6J1 | Up | 0.039222956 |
ENSG00000256235.1 | protein_coding | SMIM3 | Down | 0.036368455 |
ENSG00000256294.3 | protein_coding | ZNF225 | Up | 0.046416681 |
ENSG00000256453.1 | protein_coding | DND1 | Down | 0.023504581 |
ENSG00000256632.3 | protein_coding | RP13-672B3.2 | Down | 0.013611729 |
ENSG00000256683.2 | protein_coding | ZNF350 | Up | 0.021879236 |
ENSG00000257341.1 | protein_coding | CRIP1 | Down | 0.049720545 |
ENSG00000257702.3 | antisense | LBX2-AS1 | Down | 0.035045107 |
ENSG00000258405.5 | protein_coding | ZNF578 | Down | 0.021484975 |
ENSG00000258472.4 | protein_coding | RP11-192H23.4 | Down | 0.047286987 |
ENSG00000259571.1 | protein_coding | BLID | Down | 0.02508268 |
ENSG00000261713.2 | processed_transcript | SSTR5-AS1 | Down | 0.0167024 |
ENSG00000263002.3 | protein_coding | ZNF234 | Up | 0.035012293 |
ENSG00000263620.1 | protein_coding | RP11-599B13.6 | Down | 0.04868739 |
ENSG00000263809.1 | protein_coding | RP11-849F2.7 | Down | 0.025225822 |
ENSG00000264735.1 | lincRNA | RP11-498C9.17 | Down | 0.038254904 |
ENSG00000267022.1 | protein_coding | ZNF223 | Up | 0.005281393 |
ENSG00000267059.2 | protein_coding | UQCR11 | Down | 0.01521352 |
ENSG00000267360.2 | protein_coding | CTC-454I21.3 | Up | 0.036745114 |
ENSG00000267545.1 | protein_coding | AC005779.2 | Down | 0.028793638 |
ENSG00000267699.2 | protein_coding | RP11-729L2.2 | Up | 0.041545876 |
ENSG00000268614.1 | protein_coding | CTD-2207O23.10 | Down | 0.021055407 |
ENSG00000268797.1 | protein_coding | CTC-490E21.12 | Down | 0.003100426 |
ENSG00000269220.1 | lincRNA | LINC00528 | Down | 0.026695365 |
ENSG00000269636.1 | protein_coding | AC010441.1 | Down | 0.036368455 |
ENSG00000269858.1 | protein_coding | EGLN2 | Down | 0.003100426 |
ENSG00000271810.1 | protein_coding | RP11-426L16.10 | Down | 0.02868524 |
ENSG00000271959.1 | antisense | CTD-3064M3.7 | Down | 0.036335334 |
ENSG00000272906.1 | lincRNA | RP11-533E19.7 | Down | 0.011437491 |
ENSG00000273006.1 | lincRNA | RP11-314C9.2 | Up | 0.031113526 |