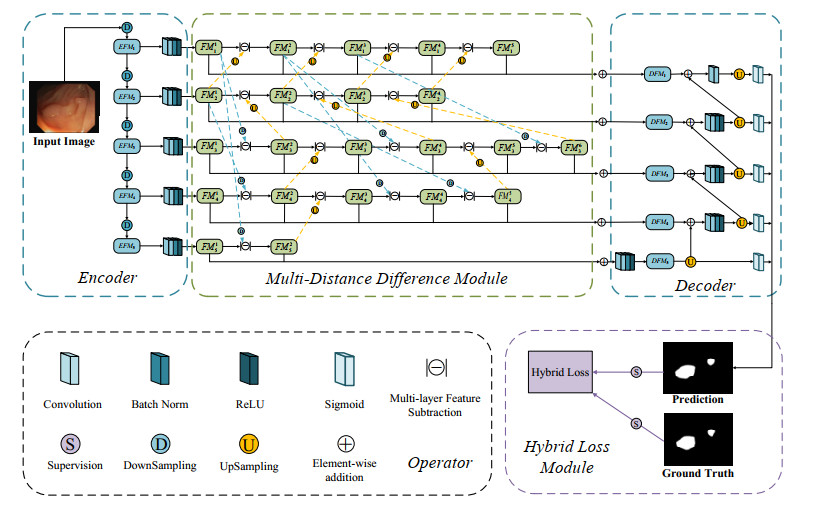
Colorectal malignancies often arise from adenomatous polyps, which typically begin as solitary, asymptomatic growths before progressing to malignancy. Colonoscopy is widely recognized as a highly efficacious clinical polyp detection method, offering valuable visual data that facilitates precise identification and subsequent removal of these tumors. Nevertheless, accurately segmenting individual polyps poses a considerable difficulty because polyps exhibit intricate and changeable characteristics, including shape, size, color, quantity and growth context during different stages. The presence of similar contextual structures around polyps significantly hampers the performance of commonly used convolutional neural network (CNN)-based automatic detection models to accurately capture valid polyp features, and these large receptive field CNN models often overlook the details of small polyps, which leads to the occurrence of false detections and missed detections. To tackle these challenges, we introduce a novel approach for automatic polyp segmentation, known as the multi-distance feature dissimilarity-guided fully convolutional network. This approach comprises three essential components, i.e., an encoder-decoder, a multi-distance difference (MDD) module and a hybrid loss (HL) module. Specifically, the MDD module primarily employs a multi-layer feature subtraction (MLFS) strategy to propagate features from the encoder to the decoder, which focuses on extracting information differences between neighboring layers' features at short distances, and both short and long-distance feature differences across layers. Drawing inspiration from pyramids, the MDD module effectively acquires discriminative features from neighboring layers or across layers in a continuous manner, which helps to strengthen feature complementary across different layers. The HL module is responsible for supervising the feature maps extracted at each layer of the network to improve prediction accuracy. Our experimental results on four challenge datasets demonstrate that the proposed approach exhibits superior automatic polyp performance in terms of the six evaluation criteria compared to five current state-of-the-art approaches.
Citation: Nan Mu, Jinjia Guo, Rong Wang. Automated polyp segmentation based on a multi-distance feature dissimilarity-guided fully convolutional network[J]. Mathematical Biosciences and Engineering, 2023, 20(11): 20116-20134. doi: 10.3934/mbe.2023891
[1] | Chenqian Li, Jun Liu, Jinshan Tang . Simultaneous segmentation and classification of colon cancer polyp images using a dual branch multi-task learning network. Mathematical Biosciences and Engineering, 2024, 21(2): 2024-2049. doi: 10.3934/mbe.2024090 |
[2] | Yun Jiang, Jie Chen, Wei Yan, Zequn Zhang, Hao Qiao, Meiqi Wang . MAG-Net : Multi-fusion network with grouped attention for retinal vessel segmentation. Mathematical Biosciences and Engineering, 2024, 21(2): 1938-1958. doi: 10.3934/mbe.2024086 |
[3] | Feiyu Chen, Haiping Ma, Weijia Zhang . SegT: Separated edge-guidance transformer network for polyp segmentation. Mathematical Biosciences and Engineering, 2023, 20(10): 17803-17821. doi: 10.3934/mbe.2023791 |
[4] | Zhigao Zeng, Cheng Huang, Wenqiu Zhu, Zhiqiang Wen, Xinpan Yuan . Flower image classification based on an improved lightweight neural network with multi-scale feature fusion and attention mechanism. Mathematical Biosciences and Engineering, 2023, 20(8): 13900-13920. doi: 10.3934/mbe.2023619 |
[5] | Rongrong Bi, Chunlei Ji, Zhipeng Yang, Meixia Qiao, Peiqing Lv, Haiying Wang . Residual based attention-Unet combing DAC and RMP modules for automatic liver tumor segmentation in CT. Mathematical Biosciences and Engineering, 2022, 19(5): 4703-4718. doi: 10.3934/mbe.2022219 |
[6] | Yalong Yang, Zhen Niu, Liangliang Su, Wenjing Xu, Yuanhang Wang . Multi-scale feature fusion for pavement crack detection based on Transformer. Mathematical Biosciences and Engineering, 2023, 20(8): 14920-14937. doi: 10.3934/mbe.2023668 |
[7] | Hong'an Li, Man Liu, Jiangwen Fan, Qingfang Liu . Biomedical image segmentation algorithm based on dense atrous convolution. Mathematical Biosciences and Engineering, 2024, 21(3): 4351-4369. doi: 10.3934/mbe.2024192 |
[8] | Zhenyin Fu, Jin Zhang, Ruyi Luo, Yutong Sun, Dongdong Deng, Ling Xia . TF-Unet:An automatic cardiac MRI image segmentation method. Mathematical Biosciences and Engineering, 2022, 19(5): 5207-5222. doi: 10.3934/mbe.2022244 |
[9] | Tong Shan, Jiayong Yan, Xiaoyao Cui, Lijian Xie . DSCA-Net: A depthwise separable convolutional neural network with attention mechanism for medical image segmentation. Mathematical Biosciences and Engineering, 2023, 20(1): 365-382. doi: 10.3934/mbe.2023017 |
[10] | Chaofan Li, Kai Ma . Entity recognition of Chinese medical text based on multi-head self-attention combined with BILSTM-CRF. Mathematical Biosciences and Engineering, 2022, 19(3): 2206-2218. doi: 10.3934/mbe.2022103 |
Colorectal malignancies often arise from adenomatous polyps, which typically begin as solitary, asymptomatic growths before progressing to malignancy. Colonoscopy is widely recognized as a highly efficacious clinical polyp detection method, offering valuable visual data that facilitates precise identification and subsequent removal of these tumors. Nevertheless, accurately segmenting individual polyps poses a considerable difficulty because polyps exhibit intricate and changeable characteristics, including shape, size, color, quantity and growth context during different stages. The presence of similar contextual structures around polyps significantly hampers the performance of commonly used convolutional neural network (CNN)-based automatic detection models to accurately capture valid polyp features, and these large receptive field CNN models often overlook the details of small polyps, which leads to the occurrence of false detections and missed detections. To tackle these challenges, we introduce a novel approach for automatic polyp segmentation, known as the multi-distance feature dissimilarity-guided fully convolutional network. This approach comprises three essential components, i.e., an encoder-decoder, a multi-distance difference (MDD) module and a hybrid loss (HL) module. Specifically, the MDD module primarily employs a multi-layer feature subtraction (MLFS) strategy to propagate features from the encoder to the decoder, which focuses on extracting information differences between neighboring layers' features at short distances, and both short and long-distance feature differences across layers. Drawing inspiration from pyramids, the MDD module effectively acquires discriminative features from neighboring layers or across layers in a continuous manner, which helps to strengthen feature complementary across different layers. The HL module is responsible for supervising the feature maps extracted at each layer of the network to improve prediction accuracy. Our experimental results on four challenge datasets demonstrate that the proposed approach exhibits superior automatic polyp performance in terms of the six evaluation criteria compared to five current state-of-the-art approaches.
Colorectal cancer (CRC), ranking as the third most common cancer in the world, posed a significant global health challenge in 2020, with over 1.9 million new cases and 930,000 deaths reported [1]. Clinically, identification of colon cancer plays an important role in cancer treatment. Nevertheless, the current methodologies for colon cancer detection remain limited in scope. One frequently employed strategy is the utilization of colonoscopy to assess the patient's gastrointestinal tract (i.e., bowel) with the objective of identifying and removing colonic polyps, which are often considered potential precursors to the development of colon cancer. Regrettably, accurately recognizing polyps from colonoscopy images can be challenging in three major aspects. First, it is essential to acknowledge that polyps exhibit diversity in terms of colors, shapes and appearances, which can evolve over time. Second, the context in which polyps manifest in various regions of the colon is complicated and varied. Lastly, the subtle color differentiations and low contrast between polyps and healthy tissue make it a daunting task to effectively distinguish their distinctive features. Consequently, this difficulty leads to diagnostic challenges, with physicians often struggling to exactly differentiate the boundaries of polyps from the surrounding healthy tissue. These limitations result in a substantial number of undetected cases, affecting approximately 10% of patients misdiagnosed with metastatic colon cancer, which leads to a delay in diagnosis and thus reduces the likelihood of patient survival [2]. Hence, it is crucial to develop automated computer-assisted techniques for highly accurate polyp segmentation is of paramount importance to enable effective prevention and treatment of colorectal cancer.
Recently, with the advancement of deep learning technology, the previous polyp segmentation methods that require manual extraction and construction of features are gradually being phased out. In its place, polyp segmentation methods based on convolutional neural networks (CNNs), a deep learning model commonly used in image analysis, are promising and efficient compared with traditional segmentation techniques [3,4].
Moreover, the employment of a fully convolutional network (FCN) [5,6] is common in the field of medical image segmentation and can be used to refine the feature representation and learning. There are three reasons for choosing to apply the FCN framework to automatic colon polyp segmentation: 1) Semantic segmentation capability: Since polyp segmentation is essentially a semantic segmentation task aimed at accurately depicting and distinguishing polyps from the background in endoscopic images, the architecture of FCN is well suited for this purpose as it can capture detailed spatial information to create pixel-level segmentation maps. 2) End-to-end learning: FCN implements end-to-end learning, i.e., it learns feature extraction and segmentation simultaneously, which is advantageous for polyp segmentation and facilitates capturing complex and changing polyp features. 3) Efficient: Known for its computational efficiency, FCN can process an entire image in a single forward pass. This is critical to enable fast and accurate polyp detection and segmentation in colonoscopy.
Notably, as a type of FCN, U-Net [7], an algorithm for semantic segmentation using fully convolutional networks, and its variants [8,9,10] are widely exploited for this purpose and have brought new advances in polyp segmentation. The advantages of U-Net for automated polyp segmentation are threefold: 1) Fully convolutional network architecture: U-Net utilizes the FCN framework, which can handle input images of arbitrary size and outputs pixel-level segmentation results. This flexibility is crucial in polyp segmentation, where the size and location of polyps can vary significantly. 2) Downsampling path and upsampling path: U-Net's structure consists of both a downsample path for capturing global features and an upsample path for preserving local details. This dual-path approach allows U-Net to simultaneously process a wide range of features and fine details, which is particularly beneficial for tasks like polyp segmentation. 3) Skip connections: U-Net introduces skip connections that connect features from the downsample path to the upsample path. These connections facilitate the propagation of information between layers, enabling better contextual understanding of the discriminative features. This is especially important when dealing with complex structures of polyps.
Nevertheless, the existing FCN approaches, including the U-Net methods, often fall short of the necessary accuracy for effectively segmenting tiny polyps. These polyps are characterized by their frequent occurrence, small volume, strong resemblance to the surrounding environment and significant background interference. Theoretically, the primary reason for this problem is that most FCN models are limited to mining more powerful features to capture information from small imperceptible polyps and exploring contextual knowledge to avoid interference from adjacent tissues or complex backgrounds.
To tackle these issues, we propose a novel automatic polyp segmentation approach based on multi-distance feature dissimilarity-guided FCN. In essence, the low-level features within a network have the ability to preserve intricate details, contours and background noise. In contrast, high-level features, while lacking the ability to discern sharp boundaries, offer consistent semantic properties and a wealth of contextual information. Recognizing the complementary nature of high-level and low-level features, we design a multi-distance difference (MDD) module, which aims to enhance the feature representation through the application of multi-layer feature subtraction (MLFS) operations, thus facilitating more comprehensive feature learning. More importantly, we specifically analyze the information variations between neighboring layers at short distances, cross-layers relationships at short distances and cross-layers connections over longer distances, thus effectively overcoming the challenges posed by the polyp's contextual environment. Furthermore, the ultimate polyp segmentation outcome is generated by merging the differential information from both adjacent and cross-layers. This approach incorporates the distinctive attributes of each layer into the decoder to perform convolution and upsampling. In addition, we leverage a hybrid loss (HL) module, which requires no training and is capable of supervising the feature maps of each decoded layer. Overall, this paper comprises the following four major contributions:
1) We present a novel network architecture for polyp segmentation that incorporates a multi-distance feature dissimilarity approach to capture feature information spanning from high-level layers to low-level layers. By employing feature subtraction operations, duplicate information between short-range and long-range feature layers is eliminated. This process ensures a comprehensive presentation of the differential information between adjacent layers and across layers, while emphasizing the complementary nature of the feature maps from the lower to the higher layers. Accordingly, we acquire more discriminative feature information for each pyramid layer, thus enhancing the recognition of polyps.
2) We are committed to expanding the perceptual range of the network by extracting features from various receptive fields. To achieve this, we develop a bi-directional feature complementation technique. In particular, this technique involves augmenting high-level features with low-level features that encompass considerable detail information in a bottom-up manner. Moreover, we adopt a top-down approach to complement low-level features with high-level features that contain meaningful semantic and contextual information. As a result, such a strategy is particularly suitable for segmenting polyps that are highly affected by background interference and are of smaller size.
3) We introduce a hybrid loss module designed for efficient feature supervision. This module utilizes learnable weights to dynamically tune the distribution of various losses, and incorporates a hierarchical difference loss to enhance the optimization of the details in segmented polyps by focusing on the finer features.
4) Through comprehensive experimental comparisons, our network model is validated on four benchmark polyp segmentation datasets. The results demonstrate its superior performance, particularly on the challenging Kvasir dataset, as evidenced by six evaluation metrics.
In the era of rapid computer technology advancements, especially in the field of machine learning, computer-aided cancer detection has become crucial in helping medical practitioners to identify and diagnose cancer. Colorectal cancer, ranking as the third most prevalent cancer worldwide, has seen continuous innovation in techniques for automated detection. In this section, we specifically delve into methods based on CNN and FCN.
Typically, traditional polyp segmentation methods involving manual extraction and design of features have proven to be inadequate in meeting current requirements due to their numerous limitations, e.g., a dependency on high-quality medical imaging, extended processing time and sensitivity to parameter selection. By contrast, with the innovation of deep learning techniques, polyp segmentation based on CNNs has emerged as a prospective method. For instance, Tajbakhsh et al. [11] presented a polyp detection approach based on a unique three-way image presentation and CNN, which dramatically reduced the false positives. Shin et al. [12] employed a region-based CNN method to automatically determine polyps from images and videos acquired during colonoscopy, which used the image enhancement strategy to solve the problem of a smaller amount of trained polyp images. Nisha et al. [13] introduced a dual-path CNN for detecting polyps in colonoscopy images. Although these methods have made valuable contributions to automated polyp segmentation, they exhibit limitations in terms of detection under homogeneous conditions. Detecting the precise structure and boundary details of polyps through convolutional operations alone remains challenging.
To improve the accuracy and efficiency of automatic segmentation, end-to-end FCNs have been increasingly employed to refine the representation and learning of polyp features in polyp segmentation tasks. Brandao et al. [5] converted three well-established networks into an FCN architecture to recognize and segment the polyps from colonoscopy images. Ji et al. [14] explored a progressive normalized self-attention network based on the FCN framework, which can effectively learn feature representations from polyp videos in real time. More advanced, Wen et al. [15] represented a simple effective polyp segmentation strategy that combines FCN-based segmentation and CNN-based classification tasks. Sanderson and Matuszewski [16] utilized a novel architecture that integrates FCN and transformer to improve polyp segmentation of colonoscopy images. Nevertheless, it is important to note that these FCN-based methods often ignore spatial coherence and pixel relationships, leading to blurred content and incomplete boundaries in the prediction outcomes.
To address these issues, the U-Net structure [7] has been employed to supplement feature information transfer from the encoder to the decoder through long-skip connections, which is capable of accurately maintain the complex details of the target tissues and has gained great popularity in the field of biomedical image segmentation. Based on U-Net, the variant UNet++ [17] captures fine-grained details of foreground objects more efficiently through nested and dense skip connections. Similarly, Jha et al. [18] utilized the ResUNet++ structure to segment polyps, which takes full advantage of residual blocks, attention blocks, atrous spatial pyramid pooling, etc. Recent studies have concentrated on calibrating the misalignment between the polyp regions and boundaries to detect some ambiguous boundaries. For example, Fan et al. [19] established associations between region and boundary cues by exploiting the reverse attention module, and Zhao et al. [20] generated differential features at neighboring layers with degradation units. More recently, Wu et al. [21] introduced a multi-scale transformer attention mechanism for high-precision polyp segmentation, which embeds transformer blocks into a U-shaped encoder-decoder framework to efficiently realize multi-scale attention for adaptive feature integration. Lewis et al. [22] proposed a dual encoder-decoder based network for polyp segmentation which combines a transformer encoder and an enhanced dilated transformer decoder for improving the overall polyp segmentation capability. However, despite the notable advancements achieved by these U-Net-based methods in polyp segmentation, they are unsatisfactory for accurately segmenting tiny polyps with high similarity in appearance to the surrounding, cluttered background and severe interference.
In contrast to conventional FCN models, our research introduces a multi-distance difference module to enhance feature representativeness at each pyramid layer and seamlessly integrates the high-level and low-level features. This integration allows our model to capture fine-grained polyp information essential for accurately identifying small-volume polyps. Our innovation stands out in three key aspects: 1) Elimination of background interference: We address the issue of misdetection that arises from traditional FCN approaches, which struggle to capture polyp context information and are susceptible to background interference. To overcome this, we eliminate redundant background information and emphasize the complementary polyp features. This is achieved through feature subtraction, which leverages the difference information between adjacent or cross-layer features. This enhancement significantly bolsters the network's ability to distinguish polyps. 2) Extending perceptual range: We mitigate a common limitation of standard U-Net models, which may struggle to recognize small targets due to the increasing relative receptive fields during downsampling. Our solution involves the development of a bi-directional feature complementation technique. This technique extracts features from various receptive fields, extending the network's perceptual range and enhancing the synergy between high-level and low-level features. This synergy is crucial for identifying small polyps effectively. 3) Optimizing fine polyp details: To address the issue of insufficient details in traditional CNN segmentation results, our supervised process incorporates a hierarchical difference loss. This specialized loss function prioritizes the refinement of finer polyp features, optimizing the quality of segmented polyps by capturing the necessary intricate details.
In summary, our research introduces the multi-distance difference module and hybrid loss module to enhance polyp segmentation by effectively addressing background interference, extending the network's ability to identify small polyps and optimizing the details of segmented polyps. These innovations collectively contribute to the accurate identification and segmentation of polyps in medical imaging.
The proposed multi-distance feature dissimilarity-guided FCN model is illustrated in Figure 1, which mostly consists of three key components: An encoder-decoder, a multi-distance difference (MDD) module and a hybrid loss (HL) module. 1) Encoder-decoder architecture: The foundation of our model is an encoder-decoder framework. The encoder primarily consists of a five-layer pyramid structure, which serves to extract essential features from the input image. Through a series of hierarchical convolution operations, the raw image undergoes transformation, yielding feature maps of varying dimensions. In the decoder stage, the received features are further processed through convolution and upsampling operations. The final touch is the application of the Sigmoid function, which yields the prediction results. 2) MDD module: The MDD module is core innovation of our approach. It plays a pivotal role in enhancing the model's performance by skillfully aggregating and disaggregating features from adjacent layers or across layers, thus highlighting their complementarities and differences. To achieve this, we have developed a bi-directional feature complementation strategy, effectively extending the network's perceptual range. This strategy facilitates the harmonious integration of high-level and low-level features, a critical aspect of polyp recognition, particularly when dealing with intricate polyp characteristics. Notably, each layer's features within the MDD module are fused and propagated to the corresponding pyramid layer within the decoder. 3) HL module: Another essential aspect of our innovation is the HL Module, which fully accounts for losses from hierarchical differences. This dynamic learning process enhances the model's ability to generate precise segmentation results. It takes a central role in supervising the training process. During training, it closely monitors the prediction outcomes and ground truths. The network's parameters are updated by efficiently propagating the prediction errors through the backpropagation method.
While our underlying framework adheres to the standard FCN structure involving encoding and decoding, our contributions are far from conventional. Notably, we have introduced two pivotal components, the multi-distance difference module and a hybrid loss module, which play a decisive role in our quest for precision in polyp segmentation. These innovations are particularly essential when dealing with the accurate identification of polyps, including the challenging task of detecting small polyps amidst intricate background interference.
The encoder consists of five encoding layers, the feature maps generated by each layer are defined as EFMi,i∈{1,2,3,4,5}, which are extracted using Res2Net [23] as the backbone network. For the original image, it is progressively passed to the next layer through downsampling operations, and the feature maps of each pyramid layer are propagated to the layers of the MDD module through a Conv−BN−ReLU combination operation. Let FMji, i∈{1,2,3,4,5} denote the five layers of features within the MDD module (as illustrated in Figure 1), it encompasses the following features:
FMji={FMj1,j∈{1,2,3,4,5}FMj2,j∈{1,2,3,4}FMj3,j∈{1,2,3,4,5,6}FMj4,j∈{1,2,3,4,5}FMj5,j∈{1,2}, | (1) |
where i indicates the layer number and j denotes the depth of the features extracted by each layer, i.e., the number of features. The FM1i of all five feature layers in the MDD module are extracted from each of the five layers in the encoder, which can be represented as:
FM1i=ReLU(BN(Conv(EFMi,K))), | (2) |
where ReLU, BN and Conv denote the activation, batch normalization and convolution operations, respectively, and K denotes the different convolution kernels.
For feature maps in the MDD module, we calculate the dissimilarity between features of adjacent layer features and cross-layer features by applying the multi-layer feature subtraction procedure (i.e., MLFS, see Figure 2 for details), which can be defined as:
MLFS=∑3i=1|Convi(Fmap1)−Convi(Fmap2)|, | (3) |
where Fmap1 and Fmap2 belong to FMji, which are two different feature maps, |⋅| denotes the computation of absolute value, Convi(⋅),i∈{1,2,3} represents the 1×1, 3×3 and 5×5 convolution operations, respectively.
In particular, to adequately capture the differences between the features of each layer, we perform MLFS on three categories of features, i.e., short-range adjacent layer features, short-range cross-layer features and long-range cross-layer features, respectively. The specific set of features for implementing MLFS is summarized in Table 1. This configuration allows the proposed MDD module not only to consider the perceptual differences between features with different sizes of receptive fields, but also to store the information differences between multi-scale features, enabling the network to explore the details of the overall polyp structure. Moreover, the complementary of the high- and low-level features can produce a more comprehensive knowledge representation, and a large amount of redundant information is mitigated. This, in turn, enhances the precision of polyp localization and the clarity of boundaries for polyps of varying sizes.
Fmap1 | Fmap2 | Parameter range | |
Short-distance adjacent layer features | FMj1 | FMj+11 | j∈{1,2,3,4} |
FMj2 | FMj+12 | j∈{1,2,3} | |
FMj3 | FMj+13 | j∈{1,2,3,4,5} | |
FMj4 | FMj+14 | j∈{1,2,3,4} | |
FMj5 | FMj+15 | j∈{1} | |
Short-distance cross-layer features | FMj2 | FMj+11 | j∈{1,2,3,4} |
FMj3 | FMj2+12 | j∈{2,4,6} | |
FMj4 | FMj+13 | j∈{2} | |
FMj4 | FMj3 | j∈{5} | |
FMj5 | FMj+14 | j∈{2} | |
Long-distance cross-layer features | FMj1 | FM2j3 | j∈{1,2,3} |
FMj2 | FMj2+14 | j∈{1,2} | |
FMj1 | FM2j4 | j∈{2} | |
FMj1 | FM2j5 | j∈{1} |
Finally, after subtracting and combining the features of each pyramid layer along the vertical direction in the MDD module, the generated feature maps of each layer are propagated to the decoder of the same layer through horizontal aggregation. The operation is expressed as follows:
DFMi=Conv(∑nj=1FMji), | (4) |
where DFMi,i∈{1,2,3,4,5} indicate the five layers of feature maps generated in the decoder (see Figure 1 for details), and n denotes the number of features in each layer of the MDD module, see Eq (1). All layer features within the decoder are activated by the Sigmoid function and are fused to generate the ultimate prediction. In particular, we leverage element-wise addition or concatenation in the decoder to progressively fuse the features at all levels. This approach results in fewer parameters in the subsequent backpropagation compared to traditional methods, rendering the proposed network relatively straightforward to train.
To optimize the prediction of the network, we employ a hybrid loss module for supervised learning between the prediction results and the ground truth (GT). The hybrid loss function (denoted as LHybrid) is expressed as follows:
LHybrid=mean|a×lIOU+b×lBCE|+lHD, | (5) |
where mean|⋅| represents the mean calculation, a and b denote the corresponding learnable weights for adaptive loss distribution, and lIOU and lBCE represent the weighted intersection over union (IOU) loss and binary cross entropy (BCE) loss, respectively, lHD denotes the loss derived from hierarchical differencing, which is applied to correct the loss function. The lHD can be calculated as follows:
lHD=∑5i=1liHD, | (6) |
where liHD, i∈{1,2,3,4,5} denotes the Euclidean distance between the predicted results (denoted as Fmapipred) after multi-scale feature extraction from the five decoder layers and the corresponding GTs (denoted as FmapiGT). The liHD can be defined as:
liHD=√(Fmapipred2−FmapiGT2). | (7) |
The lowest layer of the lHD contains a large amount of boundary information, while the higher layers contain rich location knowledge. Thus, the inclusion of lHD enhances the generalization ability of the model to detect various types of polyps.
To verify the effectiveness of the proposed model, we evaluated it on four medical polyp segmentation benchmark datasets, including CVC-ColonDB [24], CVC-ClinicDB [25], CVC-T [26] and Kvasir [27]. We assume that the four datasets used for the experiments represents actual clinical cases and contains a diversity of polyp characteristics. A brief description of the functionality and application areas of each dataset is provided below: 1) CVC-ColonDB is a dedicated dataset for colonic polyps, comprising images of polyps with diverse types, sizes and shapes. It is mainly used in the field of medical image processing and computer-aided diagnosis, particularly in the detection and segmentation of colonic polyps during colonoscopy. 2) CVC-ClinicDB is characterized by its diversity, including a wide array of endoscopic images depicting various diseases and clinical scenarios. It encompasses widespread application in medical image analysis, covering tasks such as colonic polyp segmentation and the analysis of diverse endoscopic images. 3) Similar to CVC-ClinicDB, the CVC-T dataset presents a variety of endoscopic images, each depicting different diseases, making it a valuable resource for algorithm testing and evaluation. It is instrumental in the testing and validation of medical image processing algorithms, including colonic polyp segmentation. 4) The Kvasir dataset contains images from different endoscopy devices and clinical scenarios, serving as a resource for research and analysis in multiple medical image tasks. It is widely adopted in medical image processing research, covering a spectrum of tasks including colonic polyp segmentation, lesion detection and the analysis of other endoscopic images. In summary, these four datasets have extensive applications and representativeness in the field of colonic polyp segmentation. They encompass endoscopic images from various clinical scenarios, different devices and diverse patient cases, thus providing a wealth of diverse data for our research.
In the training stage, we used the same training set similar to the polyp segmentation model MSNet [20], that is, about 38% of the images are from CVC-ClinicDB dataset and 62% from Kvasir dataset, totaling 1450 images. We also supplemented the training set with selected data from other datasets. For a comprehensive evaluation of the model's performance and to obtain more convincing results, we utilized six common evaluation metrics in the field of object detection and image segmentation, as listed below:
● The mean Intersection over Union (meanIoU), which is mainly used to calculate the similarity between the predicted segmentation and actual one via:
meanIoU(P,T)=|P⋂T||P⋃T|, | (8) |
where P and T denote the number of elements for the predicted and actual values, respectively.
● The mean Dice coefficient (meanDice), which is usually to measure the consistency between the segmented regions of interest and manually segmented one via:
meanDice(P,T)=2|P⋂T||P|+|T|. | (9) |
● The mean absolute error (MAE), which calculates the absolute error between predicted value and the corresponding actual value, and takes the average of all errors as follows:
MAE=∑mj|Vj−V'j|m, | (10) |
where Vj and V'j represent the predicted and corresponding actual values, respectively.
● The weighted Dice metrics (Fωβ) [28], which is a variant of the Dice coefficient, allocates weights to multiple categories based on demand and computes a weighted average coefficient for each category, is defined as:
Fωβ=(1+β2)×Pω×Rωβ2×Pω×Rω, | (11) |
where P and R denote the precision and recall, respectively, and β and ω indicate the corresponding weight coefficients.
● The S-measure (Sα) [29], which simultaneously measures the similarity of object structures, object regions and object boundaries to assess the consistency of predicted segmentation and ground truth, is computed as:
Sα=α∗So+(1−α)∗Sr, | (12) |
where So and Sr represent the object-oriented and region-oriented structural similarity measures, respectively, and α denotes the corresponding weight coefficient, which is set to 0.5 in our experiment.
● The E-measure (Ex,y) [30], which encrypts the entropy of an element-by-element match by comparing the correlation between the segmented result and the real mask to determine the evaluation performance, is defined as:
Ex,y=∑Wx=1∑Hy=1M(x,y)W×H, | (13) |
where M refers to the augmentation matrix of the response correlations, and W and H denote the width and height of the matrix, respectively.
In the preprocessing phase, all input images are scaled to 352 × 352 and a traditional multi-view training method is applied. The proposed method is implemented using PyTorch and executed on dual NVIDIA Ge-Force RTX 3090 GPUs. In the training phase, the parameters of the stochastic gradient descent (SGD) optimizer are set to a learning rate of 0.05, a batch size of 16, a momentum of 0.9, a weight decay of 0.0005 and epochs of 50. For data optimization, we adopt a stochastic cropping and flipping approach to prevent overfitting.
To fully demonstrate the superiority of the proposed method, we perform quantitative and qualitative experiments by comparing it with three medical image segmentation models (i.e., PraNet [19], MSNet [20] and Inf-Net [31]) and two state-of-the-art salient object detection models (i.e., DCFM [32] and UMNet [33]). We hypothesize that the network architecture mentioned above is based on prior research in the field that is applicable to the polyp segmentation task.
Tables 2–5 list a comparison of the quantitative evaluation results of the proposed model with those of the other five models. Based on the statistics provided in Tables 2 and 5, it is clear that our model ranks first on all six evaluation metrics on both the CVC-ColonDB and Kvasir datasets. Moreover, from Table 3, it can be observed that on the CVC-ClinicDB dataset, our model achieves optimal results in all metrics except for the Ex,y metric, which ranks second, and the Ex,y metric is only 0.0038 less than that of the first-ranked PraNet model. Similarly, as can be seen in Table 4, except for the meanIoU, which is slightly lower than that of the top-ranked PraNet model, the proposed model shows the best performance in all the other metrics with respect to the five compared models. Undoubtedly, our model stands out as state-of-the-art performance, underscoring its capability to comprehensively and accurately segment polyps of varying sizes, which is attributed to the novel multi-distance feature dissimilarity approach it employs.
mean IoU↑ | mean Dice↑ | MAE↓ | Fωβ↑ | Sα↑ | Ex,y↑ | |
PraNet | 0.6660 | 0.7319 | 0.0288 | 0.7144 | 0.8369 | 0.8636 |
MSNet | 0.6905 | 0.7653 | 0.0236 | 0.7410 | 0.8539 | 0.8876 |
Inf-Net | 0.0347 | 0.0637 | 0.0988 | 0.0533 | 0.4721 | 0.5959 |
DCFM | 0.0545 | 0.0870 | 0.4269 | 0.0569 | 0.3247 | 0.3379 |
UMNet | 0.1073 | 0.1674 | 0.1208 | 0.1345 | 0.5061 | 0.5847 |
Proposed | 0.6989 | 0.7757 | 0.0236 | 0.7506 | 0.8579 | 0.8912 |
mean IoU↑ | mean Dice↑ | MAE↓ | Fωβ↑ | Sα↑ | Ex,y↑ | |
PraNet | 0.8905 | 0.9319 | 0.0071 | 0.9292 | 0.9490 | 0.9789 |
MSNet | 0.8711 | 0.9138 | 0.0084 | 0.8984 | 0.9411 | 0.9545 |
Inf-Net | 0.1415 | 0.1906 | 0.1234 | 0.1633 | 0.5160 | 0.6871 |
DCFM | 0.1281 | 0.2045 | 0.5100 | 0.1319 | 0.3327 | 0.3271 |
UMNet | 0.1808 | 0.2726 | 0.3766 | 0.1857 | 0.4362 | 0.4474 |
Proposed | 0.9108 | 0.9392 | 0.0067 | 0.9319 | 0.9566 | 0.9751 |
mean IoU↑ | mean Dice↑ | MAE↓ | Fωβ↑ | Sα↑ | Ex,y↑ | |
PraNet | 0.8839 | 0.8727 | 0.0099 | 0.8433 | 0.9243 | 0.9381 |
MSNet | 0.7723 | 0.8433 | 0.0122 | 0.8059 | 0.9077 | 0.9093 |
Inf-Net | 0.0678 | 0.0925 | 0.0482 | 0.0840 | 0.5151 | 0.6684 |
DCFM | 0.0242 | 0.0383 | 0.1078 | 0.0293 | 0.4483 | 0.4690 |
UMNet | 0.0612 | 0.1148 | 0.0609 | 0.1021 | 0.5056 | 0.6661 |
Proposed | 0.8135 | 0.8741 | 0.0087 | 0.8471 | 0.9258 | 0.9437 |
mean IoU↑ | mean Dice↑ | MAE↓ | Fωβ↑ | Sα↑ | Ex,y↑ | |
PraNet | 0.8704 | 0.9180 | 0.0189 | 0.9064 | 0.9313 | 0.9615 |
MSNet | 0.8519 | 0.9014 | 0.0301 | 0.8837 | 0.9171 | 0.9423 |
Inf-Net | 0.1873 | 0.2796 | 0.1692 | 0.2392 | 0.5397 | 0.7023 |
DCFM | 0.2024 | 0.3080 | 0.5008 | 0.2084 | 0.3667 | 0.3482 |
UMNet | 0.4196 | 0.5193 | 0.1035 | 0.4580 | 0.6788 | 0.7791 |
Proposed | 0.8799 | 0.9224 | 0.0165 | 0.9121 | 0.9364 | 0.9669 |
To assess the statistical differences between our model and the comparison models across the four datasets, we computed p-values by comparing the Dice values. The specific outcomes are detailed in Table 6. P-values less than 0.05 signify a statistically significant distinction between the two subgroups. Referring to Table 6, it is evident that the Dice results of Inf-Net, DCFM and UMNet exhibit statistically significant differences compared to our model across all four datasets. This implies that the performance of these alternative models is notably inferior to our approach. Furthermore, PraNet and MSNet show no significant statistical differences with our model when evaluated on CVC-ClinicDB and Kvasir datasets, but they do display statistical distinctions on the CVC-ColonDB and CVC-T datasets, respectively. As a result, when considering the cumulative analysis across all datasets, our model emerges as the more favorable choice for polyp detection.
CVC-ColonDB | CVC-ClinicDB | CVC-T | Kvasir | |
PraNet vs. Proposed | 0.0000 | 0.8618 | 0.7090 | 0.8905 |
MSNet vs. Proposed | 0.7987 | 0.9617 | 0.0259 | 0.1305 |
Inf-Net vs. Proposed | 0.0000 | 0.0000 | 0.0000 | 0.0000 |
DCFM vs. Proposed | 0.0000 | 0.0000 | 0.0000 | 0.0000 |
UMNet vs. Proposed | 0.0000 | 0.0000 | 0.0000 | 0.0000 |
Figure 3 visually shows a qualitative comparison of the segmentation results from various models. It can be observed that the proposed model can achieve accurate detection results regardless of whether the polyp to be detected is in a shaded and narrow region (see 1st row) or in a bright region (see 2nd row). In addition, for images with cluttered background interference (see row 3) or images with tissue interference similar to the appearance of polyps (see row 4), our approch can accurately localize the correct polyp region. Notably, for tiny polyps, our method can also detect them completely (see row 1). Comparatively, the Inf-Net, DCFM and UMNet models have difficulty in determining polyp regions, and the PraNet model struggles to recognize small polyp regions and is susceptible to background interference. Similarly, the performance of MSNet is also highly affected by the background noise.
To validate the performance of the proposed MDD module and HL module, we conducted ablation experiments on two datasets, CVC-ColonDB and Kvasir, and performed elaborate quantitative comparisons of different configurations of baselines in terms of five evaluation metrics, as demonstrated in Tables 7 and 8, which include: 1) Baseline 1, which consists of the backbone network of encoder-decoder and the features ∑5i=1FM1i, FM23, FM24, denoted as BN+F1; 2) Baseline 2, which adds features FM21, FM22, FM25, FM33, FM34, FM43, FM44, FM54 to Baseline 1, denoted as BN+F1+F2; 3) Baseline 3, which adds FM31, FM32, FM53, FM63 to Baseline 2, denoted as BN+F1+F2+F3; 4) Baseline 4, which adds FM41, FM42 to Baseline 3, denoted as BN+F1+F2+F3+F4; 5) Baseline 5, which adds FM51 to Baseline 4 to form the complete MDD module, denoted as BN+MDD; 6) Baseline 6, i.e., the proposed model, containing the HL module, denoted as BN+MDD+HL.
mean IoU↑ | mean Dice↑ | Fωβ↑ | Sα↑ | Ex,y↑ | |
BN+F1 | 0.1335 | 0.2221 | 0.2006 | 0.5585 | 0.7351 |
BN+F1+F2 | 0.6666 | 0.7405 | 0.7112 | 0.8286 | 0.8518 |
BN+F1+F2+F3 | 0.6292 | 0.7043 | 0.6644 | 0.8016 | 0.8039 |
BN+F1+F2+F3+F4 | 0.6547 | 0.7313 | 0.7007 | 0.8190 | 0.8424 |
BN+MDD | 0.6767 | 0.7515 | 0.7214 | 0.8350 | 0.8527 |
BN+MDD+HL | 0.6989 | 0.7757 | 0.7506 | 0.8579 | 0.8912 |
mean IoU↑ | mean Dice↑ | Fωβ↑ | Sα↑ | Ex,y↑ | |
BN+F1 | 0.2587 | 0.3740 | 0.3599 | 0.6110 | 0.8099 |
BN+F1+F2 | 0.8622 | 0.9091 | 0.8942 | 0.9265 | 0.9434 |
BN+F1+F2+F3 | 0.8350 | 0.8862 | 0.8623 | 0.9045 | 0.9315 |
BN+F1+F2+F3+F4 | 0.8294 | 0.8833 | 0.8647 | 0.9052 | 0.9319 |
BN+MDD | 0.8445 | 0.8944 | 0.8732 | 0.9095 | 0.9342 |
BN+MDD+HL | 0.8799 | 0.9224 | 0.9121 | 0.9364 | 0.9669 |
As illustrated in Table 7, the metrics of the segmentation results obtained from the BN+F1 combination are notably low on the CVC-ColonDB dataset. Comparatively, the performance of BN+F1+F2 improves dramatically after adding additional features and performing MLFS operations between neighboring layers. In addition, the performance of all metrics continues to improve with increasing MLFS operations in baselines BN+F1+F2+F3, BN+F1+F2+F3+F4 and BN+MDD. Moreover, our baseline BN+MDD+HL achieves an improvement of 0.0222, 0.0242, 0.0292, 0.0229 and 0.0385 for the metrics meanIoU, meanDice, Fωβ, Sα and Ex,y, respectively, compared to the BN+MDD after the implementation of the HL module. Similar trends are observed in the performance of the test results on the Kvasir dataset, as presented in Table 8. Once again, the performance of BN+MDD+HL configuration stands out as the optimal choice with respect to the other baselines, and the performance gradually improves from BN+F1 to BN+MDD+HL. This further underscores the efficacy of our proposed modules.
Figure 4 provides the visualized segmentation results for different baselines. Upon close examination, it becomes evident that the polyp region identified by BN+F1 appears quite blurry, making it challenging to pinpoint the precise location of the polyp (refer to Figure 4(c)). Furthermore, the polyp detected by BN+F1+F2 with the adoption of MLFS outlines a rough boundary, but still leaves certain regions unsegmented due to the interference of relatively high exposure intensity (observe Figure 4(d)). Subsequently, the baselines BN+F1+F2+F3 and BN+F1+F2+F3+F4 overcome the interference of high exposure to some extent, but their results are still interfered by background noise, as evident in (e) and (f) of Figure 4. In comparison, BN+MDD successfully overcomes the impact of noise, although the polyp region appears incomplete in its structure (as shown in Figure 4(g)). Interestingly, our final prediction achieves strikingly similar results to the ground truth results by employing the HL module (see Figure 4(h) compared to Figure 4(b)).
In this paper, we propose a novel network architecture for automatic polyp segmentation, utilizing a multi-distance feature dissimilarity-guided FCN, which is composed of three core modules, i.e., the encoder-decoder, MMD and HL. The MDD module effectively mitigates the challenges posed by cluttered backgrounds as well as the influence of the normal tissue areas that are very similar to the appearance of the polyps. It accomplishes this by capturing the dissimilarity information between the different network layers of the encoder, thus delivering more discriminative features to the decoder. Additionally, the MDD module further enhances the feature expression capability of micro-polyp segmentation by supplementing semantic and contextual information for the low-level features, while also incorporating detailed information for the high-level features to improve the discriminative ability of tiny polyps. Based on the differential features at various scales and receptive fields aggregated by the MDD module, the HL module further optimizes the completeness of polyp segmentation and the clarity of polyp details by supervising features at multiple scales. Through a series of experiments conducted on four challenging datasets, our model exhibits state-of-the-art performance across six evaluation metrics, which confirms that the proposed method can facilitate the identification of colon cancer at an early stage.
The authors declare that they have not used Artificial Intelligence (AI) tools in the creation of this article.
This work was supported by the National Natural Science Foundation of China (62006165) and the Sichuan Science and Technology Program (2023NSFSC1397).
The authors declare that there are no conflicts of interest.
[1] |
E. Morgan, M. Arnold, A. Gini1, V. Lorenzoni, C. J. Cabasag, M. Laversanne, et al., Global burden of colorectal cancer in 2020 and 2040: incidence and mortality estimates from GLOBOCAN, Gut, 72 (2023), 338–344. https://doi.org/10.1136/gutjnl-2022-327736 doi: 10.1136/gutjnl-2022-327736
![]() |
[2] |
L. Rabeneck, H. El-Serag, J. Davila, R. Sandler, Outcomes of colorectal cancer in the United States: no change in survival (1986–1997), Am. J. Gastroenterol., 98 (2003), 471–477. https://doi.org/10.1111/j.1572-0241.2003.07260.x doi: 10.1111/j.1572-0241.2003.07260.x
![]() |
[3] | J. Tang, S. Millington, S. T. Acton, J. Crandall, S. Hurwitz, Ankle cartilage surface segmentation using directional gradient vector flow snakes, in International Conference on Image Processing, (2004), 2745–2748. https://doi.org/10.1109/ICIP.2004.1421672 |
[4] |
J. Tang, S. Guo, Q. Sun, Y. Deng, D. Zhou, Speckle reducing bilateral filter for cattle follicle segmentation, BMC Genomics, 11 (2010), 1–9. https://doi.org/10.1186/1471-2164-11-1 doi: 10.1186/1471-2164-11-1
![]() |
[5] | P. Brandao, E. Mazomenos, G. Ciuti, R. Caliò, F. Bianchi, A. Menciassi, et al., Fully convolutional neural networks for polyp segmentation in colonoscopy, in Medical Imaging 2017: Computer-Aided Diagnosis, 10134 (2017), 101–107. https://doi.org/10.1117/12.2254361 |
[6] |
N. Mu, H. Wang, Y. Zhang, J. Jiang, J. Tang, Progressive global perception and local polishing network for lung infection segmentation of COVID-19 CT images, Pattern Recognit., 121 (2021), 1–12. https://doi.org/10.1016/j.patcog.2021.108168 doi: 10.1016/j.patcog.2021.108168
![]() |
[7] | O. Ronneberger, P. Fischer, T. Brox, U-net: Convolutional networks for biomedical image segmentation, in Medical Image Computing and Computer-Assisted Intervention, (2015), 234–241. https://doi.org/10.1007/978-3-319-24574-4_28 |
[8] |
N. Mu, Z. Lyu, M. Rezaeitaleshmahalleh, J. Tang, J. Jiang, An attention residual u-net with differential preprocessing and geometric postprocessing: Learning how to segment vasculature including intracranial aneurysms, Med. Image Anal., 84 (2023), 1–12. https://doi.org/10.1016/j.media.2022.102697 doi: 10.1016/j.media.2022.102697
![]() |
[9] |
J. He, Q. Zhu, K. Zhang, P. Yu, J. Tang, An evolvable adversarial network with gradient penalty for COVID-19 infection segmentation, Appl. Soft Comput., 113 (2021), 1–10. https://doi.org/10.1016/j.asoc.2021.107947 doi: 10.1016/j.asoc.2021.107947
![]() |
[10] |
N. Mu, Z. Lyu, M. Rezaeitaleshmahalleh, X. Zhang, T. Rasmussen, R. McBane, et al., Automatic segmentation of abdominal aortic aneurysms from CT angiography using a context-aware cascaded U-Net, Comput. Biol. Med., 158 (2023), 1–11. https://doi.org/10.1016/j.compbiomed.2023.106569 doi: 10.1016/j.compbiomed.2023.106569
![]() |
[11] | N. Tajbakhsh, S. Gurudu, J. Liang, Automatic polyp detection in colonoscopy videos using an ensemble of convolutional neural networks, in IEEE 12th International Symposium on Biomedical Imaging, (2015), 79–83. https://doi.org/10.1109/ISBI.2015.7163821 |
[12] |
Y. Shin, H. A. Qadir, L. Aabakken, J. Bergsland, I. Balasingham, Automatic colon polyp detection using region based deep CNN and post learning approaches, IEEE Access, 6 (2018), 40950–40962. https://doi.org/10.1109/ACCESS.2018.2856402 doi: 10.1109/ACCESS.2018.2856402
![]() |
[13] |
J. S. Nisha, V. P. Gopi, P. Palanisamy, Automated colorectal polyp detection based on image enhancement and dual-path CNN architecture, Biomed. Signal Process. Control, 73 (2022), 103465. https://doi.org/10.1016/j.bspc.2021.103465 doi: 10.1016/j.bspc.2021.103465
![]() |
[14] | G. Ji, Y. Chou, D. Fan, G. Chen, H. Fu, Progressively normalized self-attention network for video polyp segmentation, in International Conference on Medical Image Computing and Computer-Assisted Intervention, (2021), 142–152. https://doi.org/10.1007/978-3-030-87193-2_14 |
[15] |
Y. Wen, L. Zhang, X. Meng, X. Ye, Rethinking the transfer learning for FCN based polyp segmentation in colonoscopy, IEEE Access, 11 (2023), 16183–16193. https://doi.org/10.1109/ACCESS.2023.3245519 doi: 10.1109/ACCESS.2023.3245519
![]() |
[16] | E. Sanderson, B. J. Matuszewski, FCN-transformer feature fusion for polyp segmentation, in Annual Conference on Medical Image Understanding and Analysis, (2022), 892–907. https://doi.org/10.1007/978-3-031-12053-4_65 |
[17] | Z. Zhou, M. Siddiquee, N. Tajbakhsh, J. Liang, UNet++: A nested U-Net architecture for medical image segmentation, in Deep Learning in Medical Image Analysis and Multimodal Learning for Clinical Decision Support: 4th International Workshop, (2018), 3–11. https://doi.org/10.1007/978-3-030-00889-5_1 |
[18] | D. Jha, P. Smedsrud, M. Riegler, D. Johansen, T. De Lange, Resunet++: An advanced architecture for medical image segmentation, in IEEE International Symposium on Multimedia, (2019), 225–230. https://doi.org/10.1109/ISM46123.2019.00049 |
[19] | D. P. Fan, G. Ji, T. Zhou, G. Chen, H. Fu, Pranet: Parallel reverse attention network for polyp segmentation, in Medical Image Computing and Computer Assisted Intervention, (2020), 263–273. https://doi.org/10.1007/978-3-030-59725-2_26 |
[20] | X. Zhao, L. Zang, H. Lu, Automatic polyp segmentation via multi-scale subtraction network, in Medical Image Computing and Computer Assisted Intervention, (2021), 120–130. https://doi.org/10.1007/978-3-030-87193-2_12 |
[21] |
H. Wu, Z. Zhao, Z. Wang, META-Unet: Multi-scale efficient transformer attention Unet for fast and high-accuracy polyp segmentation, IEEE Trans. Autom. Sci. Eng., 2023 (2023), 1–12. https://doi.org/10.1109/TASE.2023.3292373 doi: 10.1109/TASE.2023.3292373
![]() |
[22] |
J. Lewis, Y. J. Cha, J. Kim, Dual encoder–decoder-based deep polyp segmentation network for colonoscopy images, Sci. Rep., 13 (2023), 1–12. https://doi.org/10.1038/s41598-022-26890-9 doi: 10.1038/s41598-022-26890-9
![]() |
[23] |
S. Gao, M. Cheng, K. Zhao, X. Zhang, M. Yang, Res2net: A new multi-scale backbone architecture, IEEE Trans. Pattern Anal. Mach. Intell., 43 (2019), 652–662. https://doi.org/10.1109/TPAMI.2019.2938758 doi: 10.1109/TPAMI.2019.2938758
![]() |
[24] |
N. Tajbakhsh, S. R. Gurudu, J. Liang, Automated polyp detection in colonoscopy videos using shape and context information, IEEE Trans. Med. Imaging, 35 (2015), 630–644. https://doi.org/10.1109/TMI.2015.2487997 doi: 10.1109/TMI.2015.2487997
![]() |
[25] |
J. Bernal, F. Sánchez, G. Fernández-Esparrach, D. Gil, C. Rodríguez, WM-DOVA maps for accurate polyp highlighting in colonoscopy: Validation vs. saliency maps from physicians, Comput. Med. Imaging Graphics, 43 (2015), 99–111. https://doi.org/10.1016/j.compmedimag.2015.02.007 doi: 10.1016/j.compmedimag.2015.02.007
![]() |
[26] |
D. Vázquez, J. Bernal, F. J. Sánchez, G. Fernández-Esparrach, A. M. López, A. Romero, et al., A benchmark for endoluminal scene segmentation of colonoscopy images, J. Healthcare Eng., 2017 (2017), 1–10. https://doi.org/10.1155/2017/4037190 doi: 10.1155/2017/4037190
![]() |
[27] | D. Jha, P. H. Smedsrud, M. A. Riegler, P. Halvorsen, T. D. Lange, D. Johansen, et al., Kvasir-seg: A segmented polyp dataset, in MMM: 26th International Conference, (2020), 451–462. https://doi.org/10.1007/978-3-030-37734-2_37 |
[28] | R. Margolin, L. Zelnik-Manor, A. Tal, How to evaluate foreground maps? in IEEE Conference on Computer Vision and Pattern Recognition, (2014), 248–255. https://doi.org/10.1109/CVPR.2014.39 |
[29] | D. P. Fan, M. Cheng, Y. Liu, T. Li, A. Borji, Structure-measure: A new way to evaluate foreground maps, in Proceedings of the IEEE International Conference on Computer Vision, (2017), 4548–4557. |
[30] | D. P. Fan, C. Gong, Y. Cao, B. Ren, M. M. Cheng, A. Borji, Enhanced-alignment measure for binary foreground map evaluation, in International Joint Conference on Artificial Intelligence, (2018), 1–7. |
[31] |
D. P. Fan, T. Zhou, G. Ji, Y. Zhou, G. Chen, Inf-net: Automatic COVID-19 lung infection segmentation from CT images, IEEE Trans. Med. Imaging, 39 (2020), 2626–2637. https://doi.org/10.1109/TMI.2020.2996645 doi: 10.1109/TMI.2020.2996645
![]() |
[32] | S. Yu, J. Xiao, B. Zhang, E. Lim, Democracy does matter: Comprehensive feature mining for co-salient object detection, in IEEE/CVF Conference on Computer Vision and Pattern Recognition, (2022), 979–988. |
[33] | Y. Wang, W. Zang, L. Wang, T. Liu, H. Lu, Multi-source uncertainty mining for deep unsupervised saliency detection, in IEEE/CVF Conference on Computer Vision and Pattern Recognition, (2022), 11727–11736. https://doi.org/10.1109/CVPR52688.2022.01143 |
1. | Anil Kumar, Nikhil Aravind, Tayiba Gillani, Deepak Kumar, Artificial intelligence breakthrough in diagnosis, treatment, and prevention of colorectal cancer – A comprehensive review, 2025, 101, 17468094, 107205, 10.1016/j.bspc.2024.107205 |
Fmap1 | Fmap2 | Parameter range | |
Short-distance adjacent layer features | FMj1 | FMj+11 | j∈{1,2,3,4} |
FMj2 | FMj+12 | j∈{1,2,3} | |
FMj3 | FMj+13 | j∈{1,2,3,4,5} | |
FMj4 | FMj+14 | j∈{1,2,3,4} | |
FMj5 | FMj+15 | j∈{1} | |
Short-distance cross-layer features | FMj2 | FMj+11 | j∈{1,2,3,4} |
FMj3 | FMj2+12 | j∈{2,4,6} | |
FMj4 | FMj+13 | j∈{2} | |
FMj4 | FMj3 | j∈{5} | |
FMj5 | FMj+14 | j∈{2} | |
Long-distance cross-layer features | FMj1 | FM2j3 | j∈{1,2,3} |
FMj2 | FMj2+14 | j∈{1,2} | |
FMj1 | FM2j4 | j∈{2} | |
FMj1 | FM2j5 | j∈{1} |
mean IoU↑ | mean Dice↑ | MAE↓ | Fωβ↑ | Sα↑ | Ex,y↑ | |
PraNet | 0.6660 | 0.7319 | 0.0288 | 0.7144 | 0.8369 | 0.8636 |
MSNet | 0.6905 | 0.7653 | 0.0236 | 0.7410 | 0.8539 | 0.8876 |
Inf-Net | 0.0347 | 0.0637 | 0.0988 | 0.0533 | 0.4721 | 0.5959 |
DCFM | 0.0545 | 0.0870 | 0.4269 | 0.0569 | 0.3247 | 0.3379 |
UMNet | 0.1073 | 0.1674 | 0.1208 | 0.1345 | 0.5061 | 0.5847 |
Proposed | 0.6989 | 0.7757 | 0.0236 | 0.7506 | 0.8579 | 0.8912 |
mean IoU↑ | mean Dice↑ | MAE↓ | Fωβ↑ | Sα↑ | Ex,y↑ | |
PraNet | 0.8905 | 0.9319 | 0.0071 | 0.9292 | 0.9490 | 0.9789 |
MSNet | 0.8711 | 0.9138 | 0.0084 | 0.8984 | 0.9411 | 0.9545 |
Inf-Net | 0.1415 | 0.1906 | 0.1234 | 0.1633 | 0.5160 | 0.6871 |
DCFM | 0.1281 | 0.2045 | 0.5100 | 0.1319 | 0.3327 | 0.3271 |
UMNet | 0.1808 | 0.2726 | 0.3766 | 0.1857 | 0.4362 | 0.4474 |
Proposed | 0.9108 | 0.9392 | 0.0067 | 0.9319 | 0.9566 | 0.9751 |
mean IoU↑ | mean Dice↑ | MAE↓ | Fωβ↑ | Sα↑ | Ex,y↑ | |
PraNet | 0.8839 | 0.8727 | 0.0099 | 0.8433 | 0.9243 | 0.9381 |
MSNet | 0.7723 | 0.8433 | 0.0122 | 0.8059 | 0.9077 | 0.9093 |
Inf-Net | 0.0678 | 0.0925 | 0.0482 | 0.0840 | 0.5151 | 0.6684 |
DCFM | 0.0242 | 0.0383 | 0.1078 | 0.0293 | 0.4483 | 0.4690 |
UMNet | 0.0612 | 0.1148 | 0.0609 | 0.1021 | 0.5056 | 0.6661 |
Proposed | 0.8135 | 0.8741 | 0.0087 | 0.8471 | 0.9258 | 0.9437 |
mean IoU↑ | mean Dice↑ | MAE↓ | Fωβ↑ | Sα↑ | Ex,y↑ | |
PraNet | 0.8704 | 0.9180 | 0.0189 | 0.9064 | 0.9313 | 0.9615 |
MSNet | 0.8519 | 0.9014 | 0.0301 | 0.8837 | 0.9171 | 0.9423 |
Inf-Net | 0.1873 | 0.2796 | 0.1692 | 0.2392 | 0.5397 | 0.7023 |
DCFM | 0.2024 | 0.3080 | 0.5008 | 0.2084 | 0.3667 | 0.3482 |
UMNet | 0.4196 | 0.5193 | 0.1035 | 0.4580 | 0.6788 | 0.7791 |
Proposed | 0.8799 | 0.9224 | 0.0165 | 0.9121 | 0.9364 | 0.9669 |
CVC-ColonDB | CVC-ClinicDB | CVC-T | Kvasir | |
PraNet vs. Proposed | 0.0000 | 0.8618 | 0.7090 | 0.8905 |
MSNet vs. Proposed | 0.7987 | 0.9617 | 0.0259 | 0.1305 |
Inf-Net vs. Proposed | 0.0000 | 0.0000 | 0.0000 | 0.0000 |
DCFM vs. Proposed | 0.0000 | 0.0000 | 0.0000 | 0.0000 |
UMNet vs. Proposed | 0.0000 | 0.0000 | 0.0000 | 0.0000 |
mean IoU↑ | mean Dice↑ | Fωβ↑ | Sα↑ | Ex,y↑ | |
BN+F1 | 0.1335 | 0.2221 | 0.2006 | 0.5585 | 0.7351 |
BN+F1+F2 | 0.6666 | 0.7405 | 0.7112 | 0.8286 | 0.8518 |
BN+F1+F2+F3 | 0.6292 | 0.7043 | 0.6644 | 0.8016 | 0.8039 |
BN+F1+F2+F3+F4 | 0.6547 | 0.7313 | 0.7007 | 0.8190 | 0.8424 |
BN+MDD | 0.6767 | 0.7515 | 0.7214 | 0.8350 | 0.8527 |
BN+MDD+HL | 0.6989 | 0.7757 | 0.7506 | 0.8579 | 0.8912 |
mean IoU↑ | mean Dice↑ | Fωβ↑ | Sα↑ | Ex,y↑ | |
BN+F1 | 0.2587 | 0.3740 | 0.3599 | 0.6110 | 0.8099 |
BN+F1+F2 | 0.8622 | 0.9091 | 0.8942 | 0.9265 | 0.9434 |
BN+F1+F2+F3 | 0.8350 | 0.8862 | 0.8623 | 0.9045 | 0.9315 |
BN+F1+F2+F3+F4 | 0.8294 | 0.8833 | 0.8647 | 0.9052 | 0.9319 |
BN+MDD | 0.8445 | 0.8944 | 0.8732 | 0.9095 | 0.9342 |
BN+MDD+HL | 0.8799 | 0.9224 | 0.9121 | 0.9364 | 0.9669 |
Fmap1 | Fmap2 | Parameter range | |
Short-distance adjacent layer features | FMj1 | FMj+11 | j∈{1,2,3,4} |
FMj2 | FMj+12 | j∈{1,2,3} | |
FMj3 | FMj+13 | j∈{1,2,3,4,5} | |
FMj4 | FMj+14 | j∈{1,2,3,4} | |
FMj5 | FMj+15 | j∈{1} | |
Short-distance cross-layer features | FMj2 | FMj+11 | j∈{1,2,3,4} |
FMj3 | FMj2+12 | j∈{2,4,6} | |
FMj4 | FMj+13 | j∈{2} | |
FMj4 | FMj3 | j∈{5} | |
FMj5 | FMj+14 | j∈{2} | |
Long-distance cross-layer features | FMj1 | FM2j3 | j∈{1,2,3} |
FMj2 | FMj2+14 | j∈{1,2} | |
FMj1 | FM2j4 | j∈{2} | |
FMj1 | FM2j5 | j∈{1} |
mean IoU↑ | mean Dice↑ | MAE↓ | Fωβ↑ | Sα↑ | Ex,y↑ | |
PraNet | 0.6660 | 0.7319 | 0.0288 | 0.7144 | 0.8369 | 0.8636 |
MSNet | 0.6905 | 0.7653 | 0.0236 | 0.7410 | 0.8539 | 0.8876 |
Inf-Net | 0.0347 | 0.0637 | 0.0988 | 0.0533 | 0.4721 | 0.5959 |
DCFM | 0.0545 | 0.0870 | 0.4269 | 0.0569 | 0.3247 | 0.3379 |
UMNet | 0.1073 | 0.1674 | 0.1208 | 0.1345 | 0.5061 | 0.5847 |
Proposed | 0.6989 | 0.7757 | 0.0236 | 0.7506 | 0.8579 | 0.8912 |
mean IoU↑ | mean Dice↑ | MAE↓ | Fωβ↑ | Sα↑ | Ex,y↑ | |
PraNet | 0.8905 | 0.9319 | 0.0071 | 0.9292 | 0.9490 | 0.9789 |
MSNet | 0.8711 | 0.9138 | 0.0084 | 0.8984 | 0.9411 | 0.9545 |
Inf-Net | 0.1415 | 0.1906 | 0.1234 | 0.1633 | 0.5160 | 0.6871 |
DCFM | 0.1281 | 0.2045 | 0.5100 | 0.1319 | 0.3327 | 0.3271 |
UMNet | 0.1808 | 0.2726 | 0.3766 | 0.1857 | 0.4362 | 0.4474 |
Proposed | 0.9108 | 0.9392 | 0.0067 | 0.9319 | 0.9566 | 0.9751 |
mean IoU↑ | mean Dice↑ | MAE↓ | Fωβ↑ | Sα↑ | Ex,y↑ | |
PraNet | 0.8839 | 0.8727 | 0.0099 | 0.8433 | 0.9243 | 0.9381 |
MSNet | 0.7723 | 0.8433 | 0.0122 | 0.8059 | 0.9077 | 0.9093 |
Inf-Net | 0.0678 | 0.0925 | 0.0482 | 0.0840 | 0.5151 | 0.6684 |
DCFM | 0.0242 | 0.0383 | 0.1078 | 0.0293 | 0.4483 | 0.4690 |
UMNet | 0.0612 | 0.1148 | 0.0609 | 0.1021 | 0.5056 | 0.6661 |
Proposed | 0.8135 | 0.8741 | 0.0087 | 0.8471 | 0.9258 | 0.9437 |
mean IoU↑ | mean Dice↑ | MAE↓ | Fωβ↑ | Sα↑ | Ex,y↑ | |
PraNet | 0.8704 | 0.9180 | 0.0189 | 0.9064 | 0.9313 | 0.9615 |
MSNet | 0.8519 | 0.9014 | 0.0301 | 0.8837 | 0.9171 | 0.9423 |
Inf-Net | 0.1873 | 0.2796 | 0.1692 | 0.2392 | 0.5397 | 0.7023 |
DCFM | 0.2024 | 0.3080 | 0.5008 | 0.2084 | 0.3667 | 0.3482 |
UMNet | 0.4196 | 0.5193 | 0.1035 | 0.4580 | 0.6788 | 0.7791 |
Proposed | 0.8799 | 0.9224 | 0.0165 | 0.9121 | 0.9364 | 0.9669 |
CVC-ColonDB | CVC-ClinicDB | CVC-T | Kvasir | |
PraNet vs. Proposed | 0.0000 | 0.8618 | 0.7090 | 0.8905 |
MSNet vs. Proposed | 0.7987 | 0.9617 | 0.0259 | 0.1305 |
Inf-Net vs. Proposed | 0.0000 | 0.0000 | 0.0000 | 0.0000 |
DCFM vs. Proposed | 0.0000 | 0.0000 | 0.0000 | 0.0000 |
UMNet vs. Proposed | 0.0000 | 0.0000 | 0.0000 | 0.0000 |
mean IoU↑ | mean Dice↑ | Fωβ↑ | Sα↑ | Ex,y↑ | |
BN+F1 | 0.1335 | 0.2221 | 0.2006 | 0.5585 | 0.7351 |
BN+F1+F2 | 0.6666 | 0.7405 | 0.7112 | 0.8286 | 0.8518 |
BN+F1+F2+F3 | 0.6292 | 0.7043 | 0.6644 | 0.8016 | 0.8039 |
BN+F1+F2+F3+F4 | 0.6547 | 0.7313 | 0.7007 | 0.8190 | 0.8424 |
BN+MDD | 0.6767 | 0.7515 | 0.7214 | 0.8350 | 0.8527 |
BN+MDD+HL | 0.6989 | 0.7757 | 0.7506 | 0.8579 | 0.8912 |
mean IoU↑ | mean Dice↑ | Fωβ↑ | Sα↑ | Ex,y↑ | |
BN+F1 | 0.2587 | 0.3740 | 0.3599 | 0.6110 | 0.8099 |
BN+F1+F2 | 0.8622 | 0.9091 | 0.8942 | 0.9265 | 0.9434 |
BN+F1+F2+F3 | 0.8350 | 0.8862 | 0.8623 | 0.9045 | 0.9315 |
BN+F1+F2+F3+F4 | 0.8294 | 0.8833 | 0.8647 | 0.9052 | 0.9319 |
BN+MDD | 0.8445 | 0.8944 | 0.8732 | 0.9095 | 0.9342 |
BN+MDD+HL | 0.8799 | 0.9224 | 0.9121 | 0.9364 | 0.9669 |