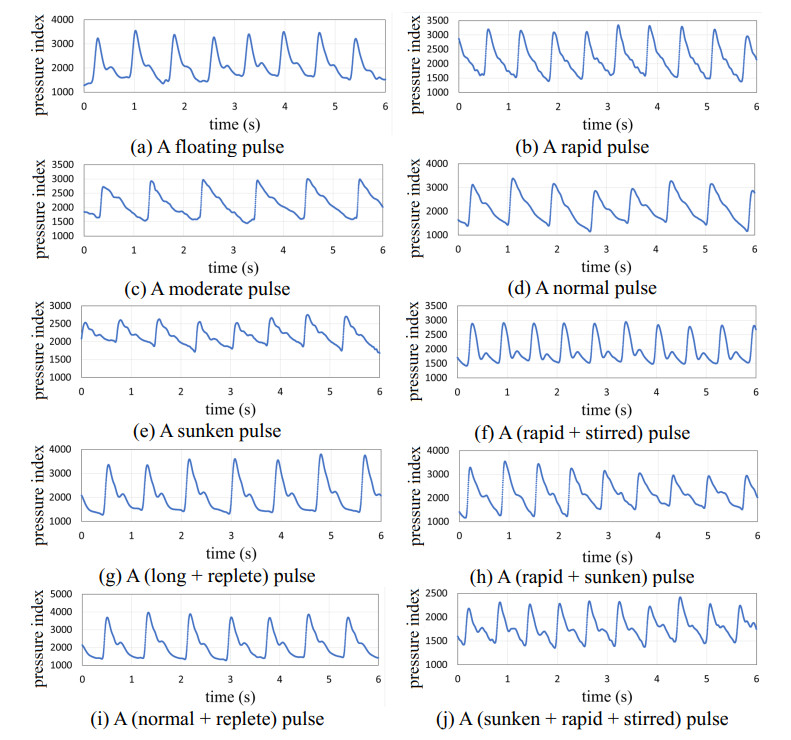
Citation: Ching-Han Huang, Yu-Min Wang, Shana Smith. Using high-dimensional features for high-accuracy pulse diagnosis[J]. Mathematical Biosciences and Engineering, 2020, 17(6): 6775-6790. doi: 10.3934/mbe.2020353
[1] | Wang Cai, Jianzhuang Wang, Longchao Cao, Gaoyang Mi, Leshi Shu, Qi Zhou, Ping Jiang . Predicting the weld width from high-speed successive images of the weld zone using different machine learning algorithms during laser welding. Mathematical Biosciences and Engineering, 2019, 16(5): 5595-5612. doi: 10.3934/mbe.2019278 |
[2] | Jian-xue Tian, Jue Zhang . Breast cancer diagnosis using feature extraction and boosted C5.0 decision tree algorithm with penalty factor. Mathematical Biosciences and Engineering, 2022, 19(3): 2193-2205. doi: 10.3934/mbe.2022102 |
[3] | Javad Hassannataj Joloudari, Faezeh Azizi, Issa Nodehi, Mohammad Ali Nematollahi, Fateme Kamrannejhad, Edris Hassannatajjeloudari, Roohallah Alizadehsani, Sheikh Mohammed Shariful Islam . Developing a Deep Neural Network model for COVID-19 diagnosis based on CT scan images. Mathematical Biosciences and Engineering, 2023, 20(9): 16236-16258. doi: 10.3934/mbe.2023725 |
[4] | Jingyao Liu, Qinghe Feng, Yu Miao, Wei He, Weili Shi, Zhengang Jiang . COVID-19 disease identification network based on weakly supervised feature selection. Mathematical Biosciences and Engineering, 2023, 20(5): 9327-9348. doi: 10.3934/mbe.2023409 |
[5] | Paweł Konieczka, Lech Raczyński, Wojciech Wiślicki, Oleksandr Fedoruk, Konrad Klimaszewski, Przemysław Kopka, Wojciech Krzemień, Roman Y. Shopa, Jakub Baran, Aurélien Coussat, Neha Chug, Catalina Curceanu, Eryk Czerwiński, Meysam Dadgar, Kamil Dulski, Aleksander Gajos, Beatrix C. Hiesmayr, Krzysztof Kacprzak, Łukasz Kapłon, Grzegorz Korcyl, Tomasz Kozik, Deepak Kumar, Szymon Niedźwiecki, Szymon Parzych, Elena Pérez del Río, Sushil Sharma, Shivani Shivani, Magdalena Skurzok, Ewa Łucja Stępień, Faranak Tayefi, Paweł Moskal . Transformation of PET raw data into images for event classification using convolutional neural networks. Mathematical Biosciences and Engineering, 2023, 20(8): 14938-14958. doi: 10.3934/mbe.2023669 |
[6] | Xiwen Qin, Shuang Zhang, Dongmei Yin, Dongxue Chen, Xiaogang Dong . Two-stage feature selection for classification of gene expression data based on an improved Salp Swarm Algorithm. Mathematical Biosciences and Engineering, 2022, 19(12): 13747-13781. doi: 10.3934/mbe.2022641 |
[7] | Chaofeng Ren, Xiaodong Zhi, Yuchi Pu, Fuqiang Zhang . A multi-scale UAV image matching method applied to large-scale landslide reconstruction. Mathematical Biosciences and Engineering, 2021, 18(3): 2274-2287. doi: 10.3934/mbe.2021115 |
[8] | Jinyi Tai, Chang Liu, Xing Wu, Jianwei Yang . Bearing fault diagnosis based on wavelet sparse convolutional network and acoustic emission compression signals. Mathematical Biosciences and Engineering, 2022, 19(8): 8057-8080. doi: 10.3934/mbe.2022377 |
[9] | Yuanyuan Ma, Jinwei Wang, Xiangyang Luo, Zhenyu Li, Chunfang Yang, Jun Chen . Image steganalysis feature selection based on the improved Fisher criterion. Mathematical Biosciences and Engineering, 2020, 17(2): 1355-1371. doi: 10.3934/mbe.2020068 |
[10] | Swati Shinde, Madhura Kalbhor, Pankaj Wajire . DeepCyto: a hybrid framework for cervical cancer classification by using deep feature fusion of cytology images. Mathematical Biosciences and Engineering, 2022, 19(7): 6415-6434. doi: 10.3934/mbe.2022301 |
Pulse taking has been used as one of the most important diagnostic methods in traditional Chinese medicine (TCM). TCM doctors use their index, middle, and ring fingers to take pulses at a patient’s wrist. Based on the tactile sensations on the fingertips, doctors can determine patients’ physiological conditions. However, accurate pulse diagnosis often requires rich clinical experience [1]. In addition, a doctor’s finger tactile sensation might be affected by many subjective and objective factors. Therefore, modern computer-aided pulse diagnostic methods have been developed to help doctors to rapidly and precisely determine a patient’s physiological conditions.
Most computer-aided pulse diagnostic methods first extract pulse features and then use certain classifiers to identify diseases or pulse types. The most commonly used classifiers are support vector machine (SVM) and artificial neural network (ANN). For example, Wang et al. [2] used multiscale sample entropy (Multi-SampEn) to extract 4 pulse features and then used SVM to classify healthy persons and diabetic patients. Guo et al. [3] used the wavelet packet transform (WPT) to extract 7 pulse features and then used SVM to hierarchically discriminate cholecystitis patients and nephrotic patients out of the rest of the population. Wang et al. [4] designed a multichannel sensor fusion device to create a 1572-dimension fusion feature vector and then used SVM to classify healthy persons and diabetic patients. Zhang et al. [5] applied the Jin’s pulse diagnosis method to extract 26 features and then used a cubic SVM algorithm to classify healthy individuals and lung cancer patients.
Other than using pulse features only, some studies integrated parameters from the pulse-taking devices to enhance classification performance. For example, Zhang et al. [6] used 5 Doppler ultrasonic diagnostic parameters and 16 pulse features extracted from WPT and then used SVM to discriminate the cholecystitis group from the healthy group. Chen et al. [7] used 4 Doppler ultrasonic diagnostic parameters and 2 pulse features extracted from an auto-regressive model and then used SVM to classify appendicitis patients and healthy persons. SVM was developed mainly for binary classification. If there were more than two groups, SVM usually compared each group alone against the whole set of other groups [3,8,9].
Artificial neural network (ANN) also has been used in the medical fields to help identify diseases. For example, Tang et al. [10] used a visual scale to extract 8 pulse features and then used an ANN to differentiate essential hypertension from normotension. Du and Stephanus [11] generated 4 features from photoplethysmography signals and then used an ANN to classify the degree of arteriovenous fistula stenosis in hemodialysis patients.
Contrary to the above hand-designed feature extractors, advanced machine learning techniques can extract features automatically [12]. Machine learning techniques can extract informative abstraction and features directly from the input pulse images. For example, Li et al. [13] used a convolution neural network (CNN) to classify 5 cardiovascular diseases and healthy persons. Although CNN has the advantage of excluding additional feature extraction tools, it fails to reveal the underlying physical meaning or implications hidden in pulse discrimination.
In TCM, pulses are classified into 28 single pulse types based on four main elements, pulse depth, pulse rate, pulse shape, and pulse strength [1,14]. Pulse depth describes the vertical position of a pulse. Pulse rate describes the number of pulses per unit time. Pulse shape describes the width and length of a pulse. Pulse strength describes the forcefulness of a pulse. If the normal pulse, i.e., the pulse of a healthy person, is included, totally, there are 29 single pulse types. A patient’s pulse might be a composition of several single pulse types, which is called a complex pulse [15]. In this study, a complex pulse is represented in the form of (single pulse A + single pulse B). Complex pulses might carry more physiological information than single pulses. For example, the (floating + rough) pulse might be related to a cold; the (slow + slippery) pulse might be related to asthma.
Some researchers have attempted to classify multiple TCM pulse types. For example, Wang and Cheng [16] extracted 13 features directly from the time-domain pulse waveforms and then used Bayesian networks to classify 5 TCM pulse types. Xu et al. [17] extracted 4 pulse features from the time-domain pulse waveforms and then used the Lempel-Ziv analysis to classify 7 TCM pulse types. Xu et al. [18] extracted 17 pulse features from the time-domain pulse waveforms and then used a fuzzy ANN to classify 16 TCM pulse types. Shu and Sun [19] used the gamma density function to obtain 7 pulse features to classify 13 TCM pulse types.
Most of the above-mentioned pulse classification methods used low-dimensional feature vectors to classify certain diseases or pule types. However, a feature generation method might only be effective for extracting certain features but not for others. For example, time domain features could not reveal the same pulse complexity as approximate entropy (ApEn) [20] and Multi-SampEn [21]. Some important pulse information is even too subtle to be extracted. To understand the underlying physical meaning or implications hidden in pulse discrimination, this study attempted to generate a high-dimensional feature vector from the time, spatial, and frequency domains to cover as much pulse information as possible to increase pulse classification accuracy.
In this study, pulse signals were measured using an ANSWatch wrist monitor (Taiwan Scientific Corp., Taipei, Taiwan). The measurement results given by ANSWatch include pulse types. Ten different wrist pulse types were collected, including floating pulse, rapid pulse, moderate pulse, normal pulse, sunken pulse, (rapid + stirred) pulse, (long + replete) pulse, (rapid + sunken) pulse, (normal + replete) pulse, (sunken + rapid + stirred) pulse. In TCM, floating pulse and sunken pulse are recognized by the depth of a pulse. Rapid pulse and moderate pulse are recognized by the rate of a pulse. Stirred pulse and long pulse are recognized by the shape of a pulse. Replete pulse is recognized by the strength of a pulse. The sampling rate was 500 Hz. Fifty 6-second data sets were collected for each pulse type. Since there were 10 pulse types, in all total, there were 500 data sets. The device used a pressure sensor to measure pulse signals, and they were converted to digital signals using a Micro Control Unit (MCU), ranging from 0 to 4095 digits. The digit is proportional to the pressure measured by the device sensor. However, because the actual pressure is unknown, “pressure index” is used as the unit of the vertical axis coordinate, as shown in Figure 1.
It is essential to record high quality pulse signals for precise computer-aided diagnosis. However, a subject’s movement or respiration might easily cause baseline drift in pulse signals. In this study, to achieve high classification accuracy, Zhang et al.’s iterative sliding window algorithm was used to remove baseline drift [5]. First, a cubic spline was created to fit the local minimums of the pulse signals, as shown in Figure 2(a). Then, the cubic spline was subtracted from the original pulse signals to remove the baseline drift, as shown in Figure 2(b). After the baseline drift was removed, the following feature extraction methods were applied to extract pulse features.
Seven features were extracted directly from the time series waveforms, including mean, standard deviation, variance, root mean square, average of the pulse intervals, standard deviation of the pulse intervals, and average of the peak amplitudes, and they are corresponding to “Mean”, “SDV”, “Variance”, “RMS”, “Avg. of the pulse interval”, “SDV of the pulse interval”, and “Avg. of the peak amp.” in Table 1, respectively.
Features | Original data set | Principal component | ||||||
1 | 2 | 500 | PC1 | PC2 | PC3 | |||
Waveform shape | Mean | 585.992 | 559.883 | … | 551.754 | -0.151 | 0.197 | -0.041 |
SDV | 550.465 | 545.270 | … | 538.055 | -0.109 | 0.017 | 0.098 | |
Variance | 303012 | 297320 | … | 289503 | -0.133 | 0.043 | 0.197 | |
RMS | 803.923 | 781.464 | … | 770.600 | -0.148 | 0.049 | 0.209 | |
Avg. of the pulse interval | 0.720 | 0.741 | … | 0.743 | -0.129 | -0.015 | 0.252 | |
SDV of the pulse interval | 0.029 | 0.072 | … | 0.053 | -0.143 | 0.204 | -0.068 | |
Avg. of the peak amp. | 1832.130 | 1824.517 | … | 1780.895 | -0.129 | 0.183 | -0.124 | |
Power spectral density | Main freq. | 1.465 | 1.465 | … | 1.465 | -0.135 | 0.199 | -0.113 |
Main freq. PSD | 260585 | 226554 | … | 238887 | -0.135 | 0.212 | -0.112 | |
BER1 (0-0.488HZ) | 28.609 | 23.850 | … | 22.926 | -0.134 | 0.215 | -0.113 | |
BER2 (0.488-1.465HZ) | 42.456 | 44.513 | … | 47.382 | -0.133 | 0.216 | -0.109 | |
BER3 (1.465-2.441HZ) | 9.975 | 12.375 | … | 11.247 | -0.133 | 0.212 | -0.111 | |
BER4 (2.441-3.418HZ) | 11.778 | 9.472 | … | 10.190 | -0.009 | -0.076 | 0.004 | |
BER5 (3.418-4.395HZ) | 5.184 | 7.071 | … | 5.596 | 0.178 | 0.043 | -0.050 | |
BER6 (4.395-5.371HZ) | 0.903 | 1.241 | … | 1.275 | -0.016 | 0.063 | -0.012 | |
BER7 (5.371-6.348HZ) | 0.634 | 0.708 | … | 0.853 | 0.141 | -0.193 | 0.122 | |
BER8 (6.348-7.324HZ) | 0.337 | 0.533 | … | 0.380 | -0.095 | 0.086 | -0.181 | |
BER9 (7.324-8.301HZ) | 0.087 | 0.179 | … | 0.109 | -0.123 | 0.139 | -0.051 | |
BER10 (8.301-9.277HZ) | 0.028 | 0.041 | … | 0.035 | -0.042 | 0.058 | -0.138 | |
BER11 (9.277-10.254HZ) | 0.009 | 0.018 | … | 0.007 | -0.102 | 0.174 | -0.021 | |
Mean freq. | 1.611 | 1.714 | … | 1.677 | -0.013 | 0.001 | 0.268 | |
Gamma density function | α | 1.813 | 1.210 | … | 1.786 | -0.045 | 0.132 | 0.057 |
β | 3.850 | 2.059 | … | 4.625 | -0.019 | 0.184 | 0.094 | |
A | 2563.632 | 2221.687 | … | 2549.752 | -0.044 | 0.184 | 0.048 | |
B | 865.356 | 991.690 | … | 711.243 | -0.024 | 0.153 | 0.206 | |
Δ | 7 | 10 | … | 8 | -0.118 | 0.148 | -0.057 | |
HHT | IMF1 (inst. amp.) | 83.463 | 49.466 | … | 5.519 | 0.200 | 0.049 | -0.054 |
IMF2 (inst. amp.) | 237.164 | 257.480 | … | 281.664 | 0.149 | 0.178 | 0.152 | |
IMF3 (inst. amp.) | 354.514 | 342.040 | … | 511.438 | 0.166 | 0.188 | 0.056 | |
IMF4 (inst. amp.) | 442.211 | 444.300 | … | 443.832 | 0.171 | 0.174 | 0.046 | |
IMF1 (inst. freq.) | 0.077 | 0.008 | … | 0.046 | 0.174 | 0.164 | 0.029 | |
IMF2 (inst. freq.) | 0.010 | 0.023 | … | 0.023 | 0.200 | 0.049 | -0.054 | |
IMF3 (inst. freq.) | 0.005 | 0.011 | … | 0.004 | 0.174 | 0.164 | 0.029 | |
IMF4 (inst. freq.) | 0.012 | 0.009 | … | 0.004 | 0.173 | 0.167 | 0.028 | |
IMF1 (power) | 1286.833 | 465.873 | … | 12.844 | 0.128 | 0.185 | 0.139 | |
IMF2 (power) | 4571.933 | 5682.005 | … | 5538.122 | 0.174 | 0.169 | 0.037 | |
IMF3 (power) | 11795.380 | 9420.495 | … | 16683.370 | 0.124 | 0.183 | 0.147 | |
IMF4 (power) | 11237.530 | 11440.120 | … | 8996.956 | -0.051 | 0.113 | 0.006 | |
ApEn | ApEn | 0.056 | 0.057 | … | 0.055 | 0.028 | 0.103 | 0.096 |
ApEn (IMF1) | 0.087 | 0.110 | … | 0.024 | 0.110 | 0.118 | 0.091 | |
ApEn (IMF2) | 0.102 | 0.110 | … | 0.103 | 0.109 | 0.155 | 0.168 | |
ApEn (IMF3) | 0.039 | 0.048 | … | 0.039 | 0.022 | 0.113 | 0.167 | |
ApEn (IMF4) | 0.033 | 0.032 | … | 0.027 | 0.075 | 0.110 | 0.191 | |
Multi-SampEn | τ=1 | 0.035 | 0.034 | … | 0.031 | 0.017 | 0.041 | 0.116 |
τ=5 | 0.122 | 0.122 | … | 0.109 | 0.008 | 0.024 | 0.160 | |
τ=10 | 0.159 | 0.1558 | … | 0.157 | 0.016 | 0.079 | 0.157 | |
τ=15 | 0.193 | 0.191 | … | 0.191 | 0.030 | 0.030 | 0.149 | |
τ=20 | 0.216 | 0.215 | … | 0.223 | 0.142 | 0.083 | -0.122 | |
τ=25 | 0.237 | 0.233 | … | 0.248 | 0.126 | 0.029 | -0.237 | |
τ=30 | 0.259 | 0.247 | … | 0.261 | 0.160 | -0.005 | -0.052 | |
WT | power (cA4) | 1.85E+09 | 1.77E+09 | … | 1.54E+09 | 0.093 | -0.091 | 0.142 |
power (cD1) | 1570.188 | 1549.465 | … | 1434.384 | -0.066 | 0.000 | 0.039 | |
power (cD2) | 6239.389 | 6607.213 | … | 5080.174 | -0.099 | -0.027 | 0.155 | |
power (cD3) | 85840 | 95480 | … | 74108 | -0.084 | 0.031 | 0.083 | |
power (cD4) | 1263707 | 1381416 | … | 1090375 | -0.080 | 0.011 | 0.117 | |
WPT | power C(4, 0) | 1.85E+09 | 1.77E+09 | … | 1.54E+09 | 0.107 | 0.099 | -0.115 |
power C(4, 1) | 1263707 | 1381416 | … | 1090375 | 0.069 | 0.031 | -0.236 | |
power C(4, 2) | 82527.030 | 92128.26 | … | 71890.45 | 0.140 | -0.017 | -0.013 | |
power C(4, 3) | 3813.841 | 3635.693 | … | 2255.475 | 0.050 | -0.104 | 0.186 | |
power C(4, 4) | 5628.319 | 5902.739 | … | 4668.485 | 0.199 | 0.047 | -0.088 | |
power C(4, 5) | 327.595 | 413.234 | … | 209.324 | 0.193 | 0.001 | -0.081 | |
power C(4, 6) | 265.937 | 137.527 | … | 94.082 | 0.192 | 0.012 | -0.050 | |
power C(4, 7) | 224.138 | 201.812 | … | 113.029 | 0.200 | 0.028 | -0.086 | |
power C(4, 8) | 754.763 | 502.210 | … | 377.318 | -0.009 | -0.076 | 0.004 | |
power C(4, 9) | 141.005 | 172.995 | … | 100.334 | -0.051 | 0.113 | 0.006 | |
power C(4, 10) | 146.798 | 167.296 | … | 148.572 | 0.192 | 0.019 | -0.080 | |
power C(4, 11) | 142.433 | 127.221 | … | 117.892 | 0.015 | 0.006 | 0.039 | |
power C(4, 12) | 151.214 | 153.350 | … | 117.711 | -0.011 | -0.001 | 0.025 | |
power C(4, 13) | 143.662 | 145.584 | … | 151.531 | -0.005 | -0.006 | -0.029 | |
power C(4, 14) | 139.631 | 188.992 | … | 168.549 | -0.013 | 0.000 | 0.054 | |
power C(4, 15) | 189.569 | 185.274 | … | 268.314 | 0.012 | -0.037 | -0.011 |
Fourteen features were extracted from PSD [22]. PSD is a measure of the distribution of the power contents of signals in the frequency domain. Band energy ratio (BER) is derived from the frequency spectrum to show the energy distribution in a specific range. Since the human pulse energy distribution is concentrated below 10 Hz, BER in a particular band with respect to the total energy in the range of 0 to 10 Hz is calculated as follows.
BER(n)=EnEt∗100 | (1) |
where En is the energy in the nth band, and Et is the total energy in the interval [0-10] Hz. In this study, signals in the interval [0-10] Hz were divided into 11 bands, as shown in Figure 3. Thus, 11 BERs were extracted, and they are corresponding to “BER1” to “BER11” in Table 1.
The main frequency is the frequency with the largest energy. After the baseline drift is removed, the main frequency is the frequency of the pulse signals. The main frequency of the pulse signals and its PSD were extracted as 2 features, and they are corresponding to “Main freq.” and “Main freq. PSD” in Table 1, respectively. For example, in Figure 3, the main frequency and its PSD are 1.4648 and 2.62 × 105, respectively.
Mean frequency, which is corresponding to “Mean freq.” in Table 1, is the sum of the product of the frequency and the corresponding PSD divided by the total PSD in the interval [0-10] Hz:
Mean frequency(MNF)=∑lj=1fjEj∑lj=1Ej | (2) |
where, Ej is the energy at frequency fj, and l is the data length in the interval [0-10] Hz.
Five pulse features were extracted from a signal using a gamma density function. Shu and Sun [19] decomposed a time series pulse waveform into a forward wave and a backward wave using a gamma density function:
F(t|α,β,Δ,A,B)=A∗f(t|α,β,0)+B∗f(t|α,β,Δ)=A∗tαe−βt10+B∗(t−Δ)αe−β(t−Δ)10 | (3) |
where A is the amplitude of the forward wave, B is the amplitude of the backward wave, α is the shape parameter of the pulse waveform, β is the rate parameter of the pulse waveform, and Δ is the phase shift or time delay between two waves. The best curve fitting was determined based on minimizing the difference between the recorded waveform and the gamma density function F(t|α,β,Δ,A,B).
For example, in Figure 4, the red dots are an actual floating pulse, and the solid line is the corresponding fitted gamma density function F(t|α,β,Δ,A,B) . In this study, particle swarm optimization (PSO) was used to acquire the optimized parameters. The average correlation of the fitted gamma density functions and the actual pulse waveforms was 0.99. After a fitted gamma density function F(t|α,β,Δ,A,B) was found, parameters α, β, Δ, А, and В were taken as 5 pulse features, as shown in Table 1.
Twelve features were extracted using HHT [23]. HHT is the result of the empirical mode decomposition (EMD) and Hilbert transform (HT) analysis. HHT was designed to analyze nonlinear and nonstationary data. HHT can provide a meaningful time-frequency-energy description of a time series. EMD decomposes signals into several intrinsic mode functions (IMFs) [24]. Figure 5 shows an example of using EMD to decompose a floating pulse into 7 IMFs and one residue. After obtaining all IMFs, average instantaneous amplitude −hn, average instantaneous frequency −ωn, and power Pn can be calculated as follows [8].
−hn=∑lt=1an(t)l | (4) |
−ωn=∑lt=1an(t)fn(t)∑lt=1an(t) | (5) |
Pn=∑lt=1|IMFn(t)|2√∑Nn=1∑lt=1|IMFn(t)|2 | (6) |
where l is the data length, N is the number of IMFs, an(t) is the instantaneous amplitude, and fn(t) is the instantaneous frequency. In this study, the first 4 IMFs were extracted. For each IMF, the average instantaneous amplitude, average instantaneous frequency, and power were calculated, and they are corresponding to “IMF1 (inst. amp.)” to “IM4 (inst. amp.)”, “IMF1 (inst. freq.)” to “IM4 (inst. freq.)”, and “IMF1 (power)” to “IM4 (power)” in Table 1, respectively. Therefore, 12 features were obtained using HHT.
Five features were derived using ApEn [20]. ApEn measures the complexity of a time series. Higher entropy values indicate a system exhibiting a greater degree of complex dynamics. Prior research found that the ApEn value of a normal health condition was higher than that of an abnormal health condition [25,26]. In this study, the ApEn of the original pulse signals and the first four IMFs were calculated to obtain 5 ApEn features, and they are corresponding to “ApEn” and “ApEn (IMF1)” to “ApEn (IMF4)” in Table 1, respectively.
Sample entropy (SampEn) is a modification of ApEn [27] and has the advantage of being less dependent on the time series length. However, a single-scale SampEn might not be sufficient to separate healthy and pathologic individuals. Costa et al. [21] calculated entropy using multiple time scales, called Multi-SampEn, to robustly separate the time series of healthy subjects and patients with severe heart disease. Compared with the traditional entropy method, Multi-SampEn can yield a higher complexity and more meaningful measure.
For the original signals with length l, a consecutive coarse-grained time series which represented the original time series on different time scales is constructed by
y(τ)(j)=1τjτ∑i=(j−1)τ+1x(i),1≤j≤lτ | (7) |
where τ is a scale factor. The original time series is divided into non-overlapping windows of length τ, and the data points inside each window are averaged. By acquiring the SampEn of the new coarse-grained time series, the Multi-SampEn of the signals at scale τ can be obtained. In this study, Multi-SampEn with 7 time scales (τ = 1, 5, 10, 15, 20, 25, and 30) were calculated for each data set. Therefore, 7 features were derived using Multi-SampEn, as shown in Table 1.
Five features were extracted using WT [28]. WT has been widely used in various fields such as signal processing and data compression. Using WT, a signal is split into two coefficient groups, a low-frequency approximation group (cAx) and a high-frequency detail group (cDx), where x is the level number. The decomposition process is iterated, with the successive approximation groups being decomposed in turn until a predefined level is reached.
In this study, a Daubechies wavelet of order 2 (db2) was used as the filter, and the decomposition process was carried out until level 4 [6,29]. The result included 4 detail coefficients (cD1-cD4) and 1 approximation coefficient (cA4). After calculating the wavelet coefficients, the wavelet powers were calculated as follows:
EcDi=LcDi∑k=1cD2i(k),i=1,…,4 | (8) |
EcA4=LcA4∑k=1cA24(k) | (9) |
where LcDi is the data length of cDi, LcA4 is the data length of cA4, k is the sample data, and i is the level number. The wavelet powers of the approximation coefficient (cA4) and 4 detail coefficients (cD1-cD4) are corresponding to “power (cA4)” and “power (cD1)” to “power (cD4)” in Table 1, respectively.
Sixteen features were extracted using WPT [6,30]. Contrary to WT, WPT splits both the detail coefficients and the approximation coefficients. Thus, WPT provids a more sophisticated analysis of the signals. In this study, a Daubechies wavelet of order 2 (db2) was used as the filter, and the decomposition process was carried out until level 4. Therefore, the signals were decomposed into 16 sub-bands. The wavelet packet power of each sub-band was calculated as follows.
Ei=LC(4,i)∑k=1C2(4,i)(k)i=1,…16 | (10) |
where Lc(4, i) is the length of the i-th sub-band at level 4, and k is the sample data. The wavelet packet power of the 16 sub-bands are corresponding to “power C(4, 0)” to “power C(4, 15)” in Table 1, respectively.
In this study, 500 pulse data sets were collected. For each original data set, a 71-dimensional feature vector was created using the above-mentioned feature extraction methods. Table 1 shows the final pulse features and the corresponding values of the original 500 dataset and the loadings of each feature element in the first 3 Principal Components (PCs).
Although a high dimensional feature vector contains more pulse information, it might also contain unwanted noises or redundant information, which might affect classification accuracy and increase computational complexity. To improve classification performance and understand the underlying physical meaning or implications hidden in pulse classification, PCA was applied to extract the most representative information.
Figure 6 shows that the first 3PCs covers about 52% of the total accumulative variances (PC1 gives 32.7478%, PC2 gives 12.9812%, and PC3 gives 6.3234%). That means that the first 3 PCs explain about 52% of the variability in the original 71 variables. If the complexity of the data is reduced by using the first 3 PCs, 48% of data information will be lost. The last three columns in Table 1 show the coefficients (or loadings) of each feature element in the first 3 PCs. The larger the absolute value of the loading, the more important the corresponding feature is in calculating the component. The loadings in the first 3 PCs show that the first component (PC1) has large associations with HHT features and WPT features, which indicates that PC1 measures the energy and local instantaneous characteristics of the pulses. The second component (PC2) has large associations with PSD features around the main frequency, which indicates that PC2 measures the characteristics of the main frequency. The third component (PC3) has large associations with mean frequency, waveform features and entropy features, which indicates that PC3 measures the complexity of the waveforms.
After extracting all features, a basic ANN was used to classify the 10 pulse types. Although the first 3 PCs covered more than 50% of the total variances, because some pulse information was subtle, five ANN models using the first 8, 13, 21, 27, and 71 PCs, which accounted for 70%, 80%, 90%, 95%, and 100% of the total variances, were trained, respectively, using the scaled conjugate gradient back-propagation algorithm to update the weights and bias values of the ANN.
In this study, the number of nodes in the ANN input layer was based on the feature dimension, and the numbers of nodes in the hidden layer and the output layer were 10 each. The maximum number of epochs was 1000, the learning rate was 0.01, and the minimum gradient was e-6. The sigmoid activation function was used for the hidden layer, and the softmax function was used for the output layer. The training was terminated when the average error was less than 10-4. This study randomly selected 70% of the data for training, 15% for validation, and 15% for test.
Table 2 shows that Model 4, using the first 27 PCs, has the highest overall average classification accuracy of 98.2%. Figure 7 shows the distances of the clusters of the 10 pulse types in the 27-dimensional PC space, based on the Ward’s hierarchical clustering method. It reveals that pulses 7 and 9, and pulses 3 and 4 have the shortest inter-cluster distances. It might affect their discriminability. However, since ANN is a nonlinear regression method, the distance between two pulses or two clusters might not be the only factor in determining pulse discriminability.
ANN model | Model 1 | Model 2 | Model 3 | Model 4 | Model 5 |
No. of PCs | 8 | 13 | 21 | 27 | 71 |
Accumulative variances (%) | 70 | 80 | 90 | 95 | 100 |
Classification accuracy (%) | 92.8 | 94.6 | 97.4 | 98.2 | 96.6 |
Table 3 shows the confusion matrix of Model 4. Each row corresponds to the predicted pulse (Output class) and each column corresponds to the true pulse (Target class). The diagonal cells correspond to the results that are correctly classified. The far right column of the table is the precision rates, which represent the percentages of all the predicted pulses in a row that are correctly classified. The row at the bottom of the table is the recall rates, which represent the percentages of all the true pulses in a column that are correctly predicted. The cell in the bottom right of the table is the overall accuracy. The result shows that the precision and recall rates differ for each pulse type.
Pulses | Target class | |||||||||||
1 | 2 | 3 | 4 | 5 | 6 | 7 | 8 | 9 | 10 | Precision | ||
![]() |
1 | 48 | 0 | 0 | 0 | 0 | 0 | 0 | 0 | 0 | 0 | 100% |
2 | 0 | 50 | 0 | 0 | 1 | 0 | 0 | 0 | 0 | 0 | 98% | |
3 | 0 | 0 | 49 | 0 | 0 | 0 | 0 | 0 | 0 | 0 | 100% | |
4 | 0 | 0 | 1 | 50 | 0 | 0 | 0 | 0 | 0 | 0 | 98% | |
5 | 0 | 0 | 0 | 0 | 49 | 0 | 0 | 0 | 0 | 0 | 100% | |
6 | 0 | 0 | 0 | 0 | 0 | 50 | 0 | 0 | 0 | 0 | 100% | |
7 | 1 | 0 | 0 | 0 | 0 | 0 | 47 | 0 | 1 | 0 | 95.9% | |
8 | 0 | 0 | 0 | 0 | 0 | 0 | 0 | 50 | 0 | 1 | 98% | |
9 | 0 | 0 | 0 | 0 | 0 | 0 | 3 | 0 | 49 | 0 | 94.2% | |
10 | 1 | 0 | 0 | 0 | 0 | 0 | 0 | 0 | 0 | 49 | 98% | |
Recall | 96% | 100% | 98% | 100% | 98% | 100% | 94% | 100% | 98% | 98% | 98.2% |
The F-measure ( = 2∙precision∙recallprecision+recall), a balance between precision and recall, was used to represent the classification rate for each pulse type. Table 4 shows that pulses 7 ((long+ replete) pulse) and 9 ((normal + replete) pulse) have the lowest classification rates in average. Most models confuse pulses 7 and 9. It might be because pulses 7 and 9 both contain replete pulse features and have the shortest average inter-cluster distance in the 27-dimensional PC space. It indicates that replete pulse dominates long pulse and normal pulse. It also indicates that the strength features of a pulse dominates the shape features of a pulse.
Pulse Type | 1 | 2 | 3 | 4 | 5 | 6 | 7 | 8 | 9 | 10 | |
![]() |
Model 1 | 98 | 90.7 | 91.9 | 91.1 | 93.1 | 98.1 | 88.9 | 97.2 | 83.7 | 95.1 |
Model 2 | 97.2 | 92.9 | 98 | 97.2 | 96 | 97.1 | 85.2 | 99 | 84.4 | 100 | |
Model 3 | 100 | 97.1 | 100 | 98 | 99 | 100 | 92.5 | 99 | 89.8 | 99 | |
Model 4 | 98 | 99 | 99 | 99 | 99 | 100 | 95 | 99 | 96.1 | 98 | |
Model 5 | 92.4 | 93.9 | 99 | 97 | 98.1 | 97 | 99 | 99 | 96 | 96 | |
Avg. | 97.1 | 94.7 | 97.6 | 96.5 | 97.0 | 98.4 | 92.1 | 98.6 | 90.0 | 97.6 |
However, on the other hand, pulses 2 (rapid pulse), 6 ((rapid + stirred) pulse), 8 ((rapid + sunken) pulse), and 10 (sunken + rapid + stirred) contain rapid pulse and are neighboring each other in the 27-dimensional PC space, their discrimination rates are very high, especially for pulse 6. It indicates that stirred pulse and sunken pulse are very distinct, and they dominate rapid pulse. It also indicate that the shape features and the depth features of a pulse are very distinct, and they dominate the rate features.
Table 5 shows a comparison between the proposed method and the prior TCM pulse classification methods. Most prior pulse classification methods used low-dimensional feature vectors. Therefore, some important but subtle pulse information might be ignored. The proposed high-dimensional feature classification method could retain more pulse information and thus achieved a higher classification accuracy.
Research | Data | Feature Dimension | Classes | Classifier | Accuracy |
Wang and Cheng [16] | 407 | 13 | 5 pulse types | Bayesian networks | 84.20% |
Xu et al. [31] | 900 | n/a | 6 pulse types | wavelet network | 83% |
Xu et al. [17] | n/a | 4 | 7 pulse types | Lempel-Ziv complexity analysis | 97.10% |
Shu and Sun [19] | n/a | 4 | 13 pulse types | n/a | n/a |
Xu et al. [18] | 320 | 17 | 16 pulse types | FNN | 90.25% |
The proposed method | 500 | 71 (w/o PCA) | 10 pulse types | ANN | 96.60% |
500 | 27 (w. PCA) | 10 pulse types | ANN | 98.20% |
There are some limitations in this study. First, TCM doctors generally use their index, middle, and ring fingers to take patients’ pulses at three different locations (Cun, Guan, and Chi) on the wrists, using three different pressures (light touch, moderate touch, and heavy touch). However, in this study, pulses at the Guan location on the left wrist were taken only. In the future, pulses on both wrists with different pressing forces will be taken to include more pulse types in pulse classification.
Most prior pulse classification research used low-dimensional feature vectors to classify few known diseases or pule types. However, a feature generation method might only be effective for extracting certain features but not for others. In order to increase pulse classification accuracy, this research proposed a novel high-dimensional feature extraction method to extract as much important and subtle pulse information as possible from the time, spatial, and frequency domains. Eight different feature generation methods were applied to construct a 71-dimensional feature vector. Extracting high-dimensional features can also help to understand the underlying physical meaning or implications hidden in pulse discrimination.
ANN results show that PCA accounted for 95% of the total variances achieved the highest accuracy of 98.2% in pulse classification. The results also showed that pulse energy, local instantaneous characteristics, main frequency, and waveform complexity are the major factors determining pulse discriminability. Some pulse features also outperform or dominate other pulse features. For example, the strength features dominate the shape features, and the shape features and the depth features dominate the rate features.
The authors would like to thank the Ministry of Science and Technology of Taiwan for financially supporting this research under contract MOST 108-2221-E-002-161-MY2.
The authors declare no conflict of interest.
[1] | A. C. Y. Tang, Review of traditional Chinese medicine pulse diagnosis quantification, Complementary Ther. Contemp. Healthcare, 2012 (2012), 61-80. |
[2] | P. Wang, W. Zuo, D. Zhang, A compound pressure signal acquisition system for multichannel wrist pulse signal analysis, IEEE Trans. Instrum. Meas., 63 (2014), 1556-1565. |
[3] | Q. L. Guo, K. Q. Wang, D. Y. Zhang, N. M. Li, A wavelet packet based pulse waveform analysis for cholecystitis and nephrotic syndrome diagnosis, 2008 International Conference on Wavelet Analysis and Pattern Recognition, 2008. Available from: https://ieeexplore.ieee.org/abstract/document/4635834. |
[4] |
D. Wang, D. Zhang, G. Lu, An optimal pulse system design by multichannel sensors fusion, IEEE J. Biomed. Health Infor., 20 (2016), 450-459. doi: 10.1109/JBHI.2015.2392132
![]() |
[5] |
Z. Zhang, Y. Zhang, L. Yao, H. Song, A. Kos, A sensor-based wrist pulse signal processing and lung cancer recognition, J. Biomed. Infor., 79 (2018), 107-116. doi: 10.1016/j.jbi.2018.01.009
![]() |
[6] | D. Zhang, L. Zhang, D. Zhang, Y. Zheng, Wavelet based analysis of doppler ultrasonic wrist-pulse signals, International Conference on BioMedical Engineering and Informatics, 2008. Available from: https://ieeexplore.ieee.org/abstract/document/4549232/. |
[7] |
Y. Chen, L. Zhang, D. Zhang, D. Zhang, Computerized wrist pulse signal diagnosis using modified auto-regressive models, J. Med. Syst., 35 (2011), 321-328. doi: 10.1007/s10916-009-9368-4
![]() |
[8] |
D. Y. Zhang, W. M. Zuo, D. Zhang, H. Z. Zhang, N. M. Li, Wrist blood flow signal-based computerized pulse diagnosis using spatial and spectrum features, J. Biomed. Sci. Eng., 3 (2010), 361-366. doi: 10.4236/jbise.2010.34050
![]() |
[9] | L. Liu, N. Li, W. Zuo, D. Zhang, H. Zhang, Multiscale sample entropy analysis of wrist pulse blood flow signal for disease diagnosis, Intelligent Science and Intelligent Data Engineering, 2012. Available from: https://link.springer.com/chapter/10.1007/978-3-642-36669-7_58. |
[10] | C. Y. Tang, W. Y. Chung, K. S. Wong, Validation of a novel traditional Chinese medicine pulse diagnostic model using an artificial neural network, J. Evidence Based Complementary Altern. Med., 2012 (2012), 1-7. |
[11] |
Y. C. Du, A. Stephanus, Levenberg-marquardt neural network algorithm for degree of arteriovenous fistula stenosis classification using a dual optical photoplethysmography sensor, Sensors, 18 (2018), 2322. doi: 10.3390/s18072322
![]() |
[12] | Y. LeCun, Y. Bengio, G. Hinton, Deep learning, Nature, 521 (2015), 436-444. |
[13] |
G. Li, K. Watanabe, H. Anzai, X. Song, A. Qiao, M. Ohta, Pulse-wave-pattern classification with a convolutional neural network, Sci. Rep., 9 (2019), 14930. doi: 10.1038/s41598-019-51334-2
![]() |
[14] | J. Y. Lee, M. Jang, S. H. Shin, Study on the depth, rate, shape, and strength of pulse with cardiovascular simulator, J. Evidence Based Complementary Altern. Med., 2017 (2017), 1-11. |
[15] | L. Xu, M. Q. Meng, K. Q. Wang, Pulse image recognition using fuzzy neural network, 2007 29th Annual International Conference of the IEEE Engineering in Medicine and Biology Society, 2007. Available from: https://www.sciencedirect.com/science/article/abs/pii/S0957417408001437. |
[16] | H. Wang, Y. Cheng, A quantitative system for pulse diagnosis in traditional Chinese medicine, 2005 IEEE Engineering in Medicine and Biology 27th Annual Conference, 2005. Available from: https://ieeexplore.ieee.org/abstract/document/1615774/. |
[17] | L. Xu, D. Zhang, K. Wang, L. Wang, Arrhythmic pulses detection using lempel-ziv complexity analysis, EURASIP J. Appl. Signal Proc., 2006 (2006), 1-12. |
[18] |
L. Xu, M. Q. Meng, K. Wang, W. Lu, N. Li, Pulse images recognition using fuzzy neural network, Expert Syst. Appl., 36 (2009), 3805-3811. doi: 10.1016/j.eswa.2008.02.028
![]() |
[19] |
J. J. Shu, Y. Sun, Developing classification indices for Chinese pulse diagnosis, Complementary Ther. Med, 15 (2007), 190-198. doi: 10.1016/j.ctim.2006.06.004
![]() |
[20] |
S. M. Pincus, Approximate entropy as a measure of system complexity, Proc. Natl. Acad. Sci., 88 (1991), 2297-2301. doi: 10.1073/pnas.88.6.2297
![]() |
[21] |
M. Costa, A. L. Goldberger, C. K. Peng, Multiscale entropy analysis of complex physiologic time series, Phys. Rev. Let., 89 (2002), 068102. doi: 10.1103/PhysRevLett.89.068102
![]() |
[22] | K. Goyal, R. Agarwal, Pulse based sensor design for wrist pulse signal analysis and health diagnosis, Biomed. Res., 28 (2017), 5187-5195. |
[23] | N. E. Huang, Z. Shen, S. R. Long, M. C. Wu, H. H. Shih, Q. Zheng, et al., The empirical mode decomposition and the Hilbert spectrum for nonlinear and non-stationary time series analysis, Proc. R. Soc. London, Ser. A, 454 (1998), 903-995. |
[24] | G. Wang, X. Chen, F. L. Qiao, Z. Wu, N. Huang, On intrinsic mode function, Adv. Adapt. Data Anal., 2 (2010), 277-293. |
[25] | N. Arunkumar, M. K. M. Sirajudeen, Approximate entropy based ayurvedic pulse diagnosis for diabetics-a case study, The 3rd International Conference on Trendz in Information Sciences & Computing (TISC2011), 2011. Available from: https://ieeexplore.ieee.org/abstract/document/6169099/. |
[26] | J. Nie, M. Ji, Y. Chu, X. Meng, Y. Wang, J. Zhong, et al., Human pulses reveal health conditions by a piezoelectret sensor via the approximate entropy analysis, Nano Energy, 58 (2019), 528-535. |
[27] | J. S. Richman, J. R. Moorman, Physiological time-series analysis using approximate entropy and sample entropy, Am. J. Phys. Heart Circ. Phys., 278 (2000), H2039-H2049. |
[28] | I. Daubechies, The wavelet transform, time-frequency localization and signal analysis, IEEE Trans. Infor. Theory, 36 (1990), 961-1005. |
[29] | D. Cvetkovic, E. D. Ü beyli, I. Cosic, Wavelet transform feature extraction from human PPG, ECG, and EEG signal responses to ELF PEMF exposures: A pilot study, Digital Signal Proc., 18 (2008), 861-874. |
[30] | I. Daubechies, Ten Lectures on Wavelets, Society for industrial and applied mathematics, 1992. |
[31] | L. Xu, K. Q. Wang, L. Wang, Pulse waveforms classification based on wavelet network, IEEE Engineering in Medicine and Biology 27th Annual Conference, 2005. Available from: https://ieeexplore.ieee.org/abstract/document/1615493/. |
1. | Tzu-Chieh Hsieh, Chien-Min Wu, Cheng-Chung Tsai, Wen-Chien Lo, Yu-Min Wang, Shana Smith, Portable Interactive Pulse Tactile Recorder and Player System, 2021, 21, 1424-8220, 4339, 10.3390/s21134339 | |
2. | Jianjun Yan, Xianglei Cai, Songye Chen, Rui Guo, Haixia Yan, Yiqin Wang, Ensemble Learning-Based Pulse Signal Recognition: Classification Model Development Study, 2021, 9, 2291-9694, e28039, 10.2196/28039 | |
3. | Sachin Kumar, Karan Veer, Sanjeev Kumar, Development of a novel wrist pulse system for early diagnosis of pathogenic bacterial infections using optimized feature selection with machine learning approaches, 2024, 87, 17468094, 105503, 10.1016/j.bspc.2023.105503 | |
4. | Jayani Umasha, Janaka Wijayakulasooriya, Ruwan Ranaweera, High-Dimensional Feature Space for Diabetes Diagnosis and Identification of Diabetic-Sensitive Features in Ayurvedic Nadi Signals, 2023, 1, 2591-7757, 1, 10.25082/CRTM.2023.01.001 | |
5. | Sachin Kumar, Karan Veer, Evaluation of Current Trends in Biomedical Applications Using Soft Computing, 2023, 18, 15748936, 693, 10.2174/1574893618666230706112826 | |
6. | Danping Pan, Yilei Guo, Yongfu Fan, Haitong Wan, Development and Application of Traditional Chinese Medicine Using AI Machine Learning and Deep Learning Strategies, 2024, 52, 0192-415X, 605, 10.1142/S0192415X24500265 |
Features | Original data set | Principal component | ||||||
1 | 2 | 500 | PC1 | PC2 | PC3 | |||
Waveform shape | Mean | 585.992 | 559.883 | … | 551.754 | -0.151 | 0.197 | -0.041 |
SDV | 550.465 | 545.270 | … | 538.055 | -0.109 | 0.017 | 0.098 | |
Variance | 303012 | 297320 | … | 289503 | -0.133 | 0.043 | 0.197 | |
RMS | 803.923 | 781.464 | … | 770.600 | -0.148 | 0.049 | 0.209 | |
Avg. of the pulse interval | 0.720 | 0.741 | … | 0.743 | -0.129 | -0.015 | 0.252 | |
SDV of the pulse interval | 0.029 | 0.072 | … | 0.053 | -0.143 | 0.204 | -0.068 | |
Avg. of the peak amp. | 1832.130 | 1824.517 | … | 1780.895 | -0.129 | 0.183 | -0.124 | |
Power spectral density | Main freq. | 1.465 | 1.465 | … | 1.465 | -0.135 | 0.199 | -0.113 |
Main freq. PSD | 260585 | 226554 | … | 238887 | -0.135 | 0.212 | -0.112 | |
BER1 (0-0.488HZ) | 28.609 | 23.850 | … | 22.926 | -0.134 | 0.215 | -0.113 | |
BER2 (0.488-1.465HZ) | 42.456 | 44.513 | … | 47.382 | -0.133 | 0.216 | -0.109 | |
BER3 (1.465-2.441HZ) | 9.975 | 12.375 | … | 11.247 | -0.133 | 0.212 | -0.111 | |
BER4 (2.441-3.418HZ) | 11.778 | 9.472 | … | 10.190 | -0.009 | -0.076 | 0.004 | |
BER5 (3.418-4.395HZ) | 5.184 | 7.071 | … | 5.596 | 0.178 | 0.043 | -0.050 | |
BER6 (4.395-5.371HZ) | 0.903 | 1.241 | … | 1.275 | -0.016 | 0.063 | -0.012 | |
BER7 (5.371-6.348HZ) | 0.634 | 0.708 | … | 0.853 | 0.141 | -0.193 | 0.122 | |
BER8 (6.348-7.324HZ) | 0.337 | 0.533 | … | 0.380 | -0.095 | 0.086 | -0.181 | |
BER9 (7.324-8.301HZ) | 0.087 | 0.179 | … | 0.109 | -0.123 | 0.139 | -0.051 | |
BER10 (8.301-9.277HZ) | 0.028 | 0.041 | … | 0.035 | -0.042 | 0.058 | -0.138 | |
BER11 (9.277-10.254HZ) | 0.009 | 0.018 | … | 0.007 | -0.102 | 0.174 | -0.021 | |
Mean freq. | 1.611 | 1.714 | … | 1.677 | -0.013 | 0.001 | 0.268 | |
Gamma density function | α | 1.813 | 1.210 | … | 1.786 | -0.045 | 0.132 | 0.057 |
β | 3.850 | 2.059 | … | 4.625 | -0.019 | 0.184 | 0.094 | |
A | 2563.632 | 2221.687 | … | 2549.752 | -0.044 | 0.184 | 0.048 | |
B | 865.356 | 991.690 | … | 711.243 | -0.024 | 0.153 | 0.206 | |
Δ | 7 | 10 | … | 8 | -0.118 | 0.148 | -0.057 | |
HHT | IMF1 (inst. amp.) | 83.463 | 49.466 | … | 5.519 | 0.200 | 0.049 | -0.054 |
IMF2 (inst. amp.) | 237.164 | 257.480 | … | 281.664 | 0.149 | 0.178 | 0.152 | |
IMF3 (inst. amp.) | 354.514 | 342.040 | … | 511.438 | 0.166 | 0.188 | 0.056 | |
IMF4 (inst. amp.) | 442.211 | 444.300 | … | 443.832 | 0.171 | 0.174 | 0.046 | |
IMF1 (inst. freq.) | 0.077 | 0.008 | … | 0.046 | 0.174 | 0.164 | 0.029 | |
IMF2 (inst. freq.) | 0.010 | 0.023 | … | 0.023 | 0.200 | 0.049 | -0.054 | |
IMF3 (inst. freq.) | 0.005 | 0.011 | … | 0.004 | 0.174 | 0.164 | 0.029 | |
IMF4 (inst. freq.) | 0.012 | 0.009 | … | 0.004 | 0.173 | 0.167 | 0.028 | |
IMF1 (power) | 1286.833 | 465.873 | … | 12.844 | 0.128 | 0.185 | 0.139 | |
IMF2 (power) | 4571.933 | 5682.005 | … | 5538.122 | 0.174 | 0.169 | 0.037 | |
IMF3 (power) | 11795.380 | 9420.495 | … | 16683.370 | 0.124 | 0.183 | 0.147 | |
IMF4 (power) | 11237.530 | 11440.120 | … | 8996.956 | -0.051 | 0.113 | 0.006 | |
ApEn | ApEn | 0.056 | 0.057 | … | 0.055 | 0.028 | 0.103 | 0.096 |
ApEn (IMF1) | 0.087 | 0.110 | … | 0.024 | 0.110 | 0.118 | 0.091 | |
ApEn (IMF2) | 0.102 | 0.110 | … | 0.103 | 0.109 | 0.155 | 0.168 | |
ApEn (IMF3) | 0.039 | 0.048 | … | 0.039 | 0.022 | 0.113 | 0.167 | |
ApEn (IMF4) | 0.033 | 0.032 | … | 0.027 | 0.075 | 0.110 | 0.191 | |
Multi-SampEn | τ=1 | 0.035 | 0.034 | … | 0.031 | 0.017 | 0.041 | 0.116 |
τ=5 | 0.122 | 0.122 | … | 0.109 | 0.008 | 0.024 | 0.160 | |
τ=10 | 0.159 | 0.1558 | … | 0.157 | 0.016 | 0.079 | 0.157 | |
τ=15 | 0.193 | 0.191 | … | 0.191 | 0.030 | 0.030 | 0.149 | |
τ=20 | 0.216 | 0.215 | … | 0.223 | 0.142 | 0.083 | -0.122 | |
τ=25 | 0.237 | 0.233 | … | 0.248 | 0.126 | 0.029 | -0.237 | |
τ=30 | 0.259 | 0.247 | … | 0.261 | 0.160 | -0.005 | -0.052 | |
WT | power (cA4) | 1.85E+09 | 1.77E+09 | … | 1.54E+09 | 0.093 | -0.091 | 0.142 |
power (cD1) | 1570.188 | 1549.465 | … | 1434.384 | -0.066 | 0.000 | 0.039 | |
power (cD2) | 6239.389 | 6607.213 | … | 5080.174 | -0.099 | -0.027 | 0.155 | |
power (cD3) | 85840 | 95480 | … | 74108 | -0.084 | 0.031 | 0.083 | |
power (cD4) | 1263707 | 1381416 | … | 1090375 | -0.080 | 0.011 | 0.117 | |
WPT | power C(4, 0) | 1.85E+09 | 1.77E+09 | … | 1.54E+09 | 0.107 | 0.099 | -0.115 |
power C(4, 1) | 1263707 | 1381416 | … | 1090375 | 0.069 | 0.031 | -0.236 | |
power C(4, 2) | 82527.030 | 92128.26 | … | 71890.45 | 0.140 | -0.017 | -0.013 | |
power C(4, 3) | 3813.841 | 3635.693 | … | 2255.475 | 0.050 | -0.104 | 0.186 | |
power C(4, 4) | 5628.319 | 5902.739 | … | 4668.485 | 0.199 | 0.047 | -0.088 | |
power C(4, 5) | 327.595 | 413.234 | … | 209.324 | 0.193 | 0.001 | -0.081 | |
power C(4, 6) | 265.937 | 137.527 | … | 94.082 | 0.192 | 0.012 | -0.050 | |
power C(4, 7) | 224.138 | 201.812 | … | 113.029 | 0.200 | 0.028 | -0.086 | |
power C(4, 8) | 754.763 | 502.210 | … | 377.318 | -0.009 | -0.076 | 0.004 | |
power C(4, 9) | 141.005 | 172.995 | … | 100.334 | -0.051 | 0.113 | 0.006 | |
power C(4, 10) | 146.798 | 167.296 | … | 148.572 | 0.192 | 0.019 | -0.080 | |
power C(4, 11) | 142.433 | 127.221 | … | 117.892 | 0.015 | 0.006 | 0.039 | |
power C(4, 12) | 151.214 | 153.350 | … | 117.711 | -0.011 | -0.001 | 0.025 | |
power C(4, 13) | 143.662 | 145.584 | … | 151.531 | -0.005 | -0.006 | -0.029 | |
power C(4, 14) | 139.631 | 188.992 | … | 168.549 | -0.013 | 0.000 | 0.054 | |
power C(4, 15) | 189.569 | 185.274 | … | 268.314 | 0.012 | -0.037 | -0.011 |
ANN model | Model 1 | Model 2 | Model 3 | Model 4 | Model 5 |
No. of PCs | 8 | 13 | 21 | 27 | 71 |
Accumulative variances (%) | 70 | 80 | 90 | 95 | 100 |
Classification accuracy (%) | 92.8 | 94.6 | 97.4 | 98.2 | 96.6 |
Pulses | Target class | |||||||||||
1 | 2 | 3 | 4 | 5 | 6 | 7 | 8 | 9 | 10 | Precision | ||
![]() |
1 | 48 | 0 | 0 | 0 | 0 | 0 | 0 | 0 | 0 | 0 | 100% |
2 | 0 | 50 | 0 | 0 | 1 | 0 | 0 | 0 | 0 | 0 | 98% | |
3 | 0 | 0 | 49 | 0 | 0 | 0 | 0 | 0 | 0 | 0 | 100% | |
4 | 0 | 0 | 1 | 50 | 0 | 0 | 0 | 0 | 0 | 0 | 98% | |
5 | 0 | 0 | 0 | 0 | 49 | 0 | 0 | 0 | 0 | 0 | 100% | |
6 | 0 | 0 | 0 | 0 | 0 | 50 | 0 | 0 | 0 | 0 | 100% | |
7 | 1 | 0 | 0 | 0 | 0 | 0 | 47 | 0 | 1 | 0 | 95.9% | |
8 | 0 | 0 | 0 | 0 | 0 | 0 | 0 | 50 | 0 | 1 | 98% | |
9 | 0 | 0 | 0 | 0 | 0 | 0 | 3 | 0 | 49 | 0 | 94.2% | |
10 | 1 | 0 | 0 | 0 | 0 | 0 | 0 | 0 | 0 | 49 | 98% | |
Recall | 96% | 100% | 98% | 100% | 98% | 100% | 94% | 100% | 98% | 98% | 98.2% |
Pulse Type | 1 | 2 | 3 | 4 | 5 | 6 | 7 | 8 | 9 | 10 | |
![]() |
Model 1 | 98 | 90.7 | 91.9 | 91.1 | 93.1 | 98.1 | 88.9 | 97.2 | 83.7 | 95.1 |
Model 2 | 97.2 | 92.9 | 98 | 97.2 | 96 | 97.1 | 85.2 | 99 | 84.4 | 100 | |
Model 3 | 100 | 97.1 | 100 | 98 | 99 | 100 | 92.5 | 99 | 89.8 | 99 | |
Model 4 | 98 | 99 | 99 | 99 | 99 | 100 | 95 | 99 | 96.1 | 98 | |
Model 5 | 92.4 | 93.9 | 99 | 97 | 98.1 | 97 | 99 | 99 | 96 | 96 | |
Avg. | 97.1 | 94.7 | 97.6 | 96.5 | 97.0 | 98.4 | 92.1 | 98.6 | 90.0 | 97.6 |
Research | Data | Feature Dimension | Classes | Classifier | Accuracy |
Wang and Cheng [16] | 407 | 13 | 5 pulse types | Bayesian networks | 84.20% |
Xu et al. [31] | 900 | n/a | 6 pulse types | wavelet network | 83% |
Xu et al. [17] | n/a | 4 | 7 pulse types | Lempel-Ziv complexity analysis | 97.10% |
Shu and Sun [19] | n/a | 4 | 13 pulse types | n/a | n/a |
Xu et al. [18] | 320 | 17 | 16 pulse types | FNN | 90.25% |
The proposed method | 500 | 71 (w/o PCA) | 10 pulse types | ANN | 96.60% |
500 | 27 (w. PCA) | 10 pulse types | ANN | 98.20% |
Features | Original data set | Principal component | ||||||
1 | 2 | 500 | PC1 | PC2 | PC3 | |||
Waveform shape | Mean | 585.992 | 559.883 | … | 551.754 | -0.151 | 0.197 | -0.041 |
SDV | 550.465 | 545.270 | … | 538.055 | -0.109 | 0.017 | 0.098 | |
Variance | 303012 | 297320 | … | 289503 | -0.133 | 0.043 | 0.197 | |
RMS | 803.923 | 781.464 | … | 770.600 | -0.148 | 0.049 | 0.209 | |
Avg. of the pulse interval | 0.720 | 0.741 | … | 0.743 | -0.129 | -0.015 | 0.252 | |
SDV of the pulse interval | 0.029 | 0.072 | … | 0.053 | -0.143 | 0.204 | -0.068 | |
Avg. of the peak amp. | 1832.130 | 1824.517 | … | 1780.895 | -0.129 | 0.183 | -0.124 | |
Power spectral density | Main freq. | 1.465 | 1.465 | … | 1.465 | -0.135 | 0.199 | -0.113 |
Main freq. PSD | 260585 | 226554 | … | 238887 | -0.135 | 0.212 | -0.112 | |
BER1 (0-0.488HZ) | 28.609 | 23.850 | … | 22.926 | -0.134 | 0.215 | -0.113 | |
BER2 (0.488-1.465HZ) | 42.456 | 44.513 | … | 47.382 | -0.133 | 0.216 | -0.109 | |
BER3 (1.465-2.441HZ) | 9.975 | 12.375 | … | 11.247 | -0.133 | 0.212 | -0.111 | |
BER4 (2.441-3.418HZ) | 11.778 | 9.472 | … | 10.190 | -0.009 | -0.076 | 0.004 | |
BER5 (3.418-4.395HZ) | 5.184 | 7.071 | … | 5.596 | 0.178 | 0.043 | -0.050 | |
BER6 (4.395-5.371HZ) | 0.903 | 1.241 | … | 1.275 | -0.016 | 0.063 | -0.012 | |
BER7 (5.371-6.348HZ) | 0.634 | 0.708 | … | 0.853 | 0.141 | -0.193 | 0.122 | |
BER8 (6.348-7.324HZ) | 0.337 | 0.533 | … | 0.380 | -0.095 | 0.086 | -0.181 | |
BER9 (7.324-8.301HZ) | 0.087 | 0.179 | … | 0.109 | -0.123 | 0.139 | -0.051 | |
BER10 (8.301-9.277HZ) | 0.028 | 0.041 | … | 0.035 | -0.042 | 0.058 | -0.138 | |
BER11 (9.277-10.254HZ) | 0.009 | 0.018 | … | 0.007 | -0.102 | 0.174 | -0.021 | |
Mean freq. | 1.611 | 1.714 | … | 1.677 | -0.013 | 0.001 | 0.268 | |
Gamma density function | α | 1.813 | 1.210 | … | 1.786 | -0.045 | 0.132 | 0.057 |
β | 3.850 | 2.059 | … | 4.625 | -0.019 | 0.184 | 0.094 | |
A | 2563.632 | 2221.687 | … | 2549.752 | -0.044 | 0.184 | 0.048 | |
B | 865.356 | 991.690 | … | 711.243 | -0.024 | 0.153 | 0.206 | |
Δ | 7 | 10 | … | 8 | -0.118 | 0.148 | -0.057 | |
HHT | IMF1 (inst. amp.) | 83.463 | 49.466 | … | 5.519 | 0.200 | 0.049 | -0.054 |
IMF2 (inst. amp.) | 237.164 | 257.480 | … | 281.664 | 0.149 | 0.178 | 0.152 | |
IMF3 (inst. amp.) | 354.514 | 342.040 | … | 511.438 | 0.166 | 0.188 | 0.056 | |
IMF4 (inst. amp.) | 442.211 | 444.300 | … | 443.832 | 0.171 | 0.174 | 0.046 | |
IMF1 (inst. freq.) | 0.077 | 0.008 | … | 0.046 | 0.174 | 0.164 | 0.029 | |
IMF2 (inst. freq.) | 0.010 | 0.023 | … | 0.023 | 0.200 | 0.049 | -0.054 | |
IMF3 (inst. freq.) | 0.005 | 0.011 | … | 0.004 | 0.174 | 0.164 | 0.029 | |
IMF4 (inst. freq.) | 0.012 | 0.009 | … | 0.004 | 0.173 | 0.167 | 0.028 | |
IMF1 (power) | 1286.833 | 465.873 | … | 12.844 | 0.128 | 0.185 | 0.139 | |
IMF2 (power) | 4571.933 | 5682.005 | … | 5538.122 | 0.174 | 0.169 | 0.037 | |
IMF3 (power) | 11795.380 | 9420.495 | … | 16683.370 | 0.124 | 0.183 | 0.147 | |
IMF4 (power) | 11237.530 | 11440.120 | … | 8996.956 | -0.051 | 0.113 | 0.006 | |
ApEn | ApEn | 0.056 | 0.057 | … | 0.055 | 0.028 | 0.103 | 0.096 |
ApEn (IMF1) | 0.087 | 0.110 | … | 0.024 | 0.110 | 0.118 | 0.091 | |
ApEn (IMF2) | 0.102 | 0.110 | … | 0.103 | 0.109 | 0.155 | 0.168 | |
ApEn (IMF3) | 0.039 | 0.048 | … | 0.039 | 0.022 | 0.113 | 0.167 | |
ApEn (IMF4) | 0.033 | 0.032 | … | 0.027 | 0.075 | 0.110 | 0.191 | |
Multi-SampEn | τ=1 | 0.035 | 0.034 | … | 0.031 | 0.017 | 0.041 | 0.116 |
τ=5 | 0.122 | 0.122 | … | 0.109 | 0.008 | 0.024 | 0.160 | |
τ=10 | 0.159 | 0.1558 | … | 0.157 | 0.016 | 0.079 | 0.157 | |
τ=15 | 0.193 | 0.191 | … | 0.191 | 0.030 | 0.030 | 0.149 | |
τ=20 | 0.216 | 0.215 | … | 0.223 | 0.142 | 0.083 | -0.122 | |
τ=25 | 0.237 | 0.233 | … | 0.248 | 0.126 | 0.029 | -0.237 | |
τ=30 | 0.259 | 0.247 | … | 0.261 | 0.160 | -0.005 | -0.052 | |
WT | power (cA4) | 1.85E+09 | 1.77E+09 | … | 1.54E+09 | 0.093 | -0.091 | 0.142 |
power (cD1) | 1570.188 | 1549.465 | … | 1434.384 | -0.066 | 0.000 | 0.039 | |
power (cD2) | 6239.389 | 6607.213 | … | 5080.174 | -0.099 | -0.027 | 0.155 | |
power (cD3) | 85840 | 95480 | … | 74108 | -0.084 | 0.031 | 0.083 | |
power (cD4) | 1263707 | 1381416 | … | 1090375 | -0.080 | 0.011 | 0.117 | |
WPT | power C(4, 0) | 1.85E+09 | 1.77E+09 | … | 1.54E+09 | 0.107 | 0.099 | -0.115 |
power C(4, 1) | 1263707 | 1381416 | … | 1090375 | 0.069 | 0.031 | -0.236 | |
power C(4, 2) | 82527.030 | 92128.26 | … | 71890.45 | 0.140 | -0.017 | -0.013 | |
power C(4, 3) | 3813.841 | 3635.693 | … | 2255.475 | 0.050 | -0.104 | 0.186 | |
power C(4, 4) | 5628.319 | 5902.739 | … | 4668.485 | 0.199 | 0.047 | -0.088 | |
power C(4, 5) | 327.595 | 413.234 | … | 209.324 | 0.193 | 0.001 | -0.081 | |
power C(4, 6) | 265.937 | 137.527 | … | 94.082 | 0.192 | 0.012 | -0.050 | |
power C(4, 7) | 224.138 | 201.812 | … | 113.029 | 0.200 | 0.028 | -0.086 | |
power C(4, 8) | 754.763 | 502.210 | … | 377.318 | -0.009 | -0.076 | 0.004 | |
power C(4, 9) | 141.005 | 172.995 | … | 100.334 | -0.051 | 0.113 | 0.006 | |
power C(4, 10) | 146.798 | 167.296 | … | 148.572 | 0.192 | 0.019 | -0.080 | |
power C(4, 11) | 142.433 | 127.221 | … | 117.892 | 0.015 | 0.006 | 0.039 | |
power C(4, 12) | 151.214 | 153.350 | … | 117.711 | -0.011 | -0.001 | 0.025 | |
power C(4, 13) | 143.662 | 145.584 | … | 151.531 | -0.005 | -0.006 | -0.029 | |
power C(4, 14) | 139.631 | 188.992 | … | 168.549 | -0.013 | 0.000 | 0.054 | |
power C(4, 15) | 189.569 | 185.274 | … | 268.314 | 0.012 | -0.037 | -0.011 |
ANN model | Model 1 | Model 2 | Model 3 | Model 4 | Model 5 |
No. of PCs | 8 | 13 | 21 | 27 | 71 |
Accumulative variances (%) | 70 | 80 | 90 | 95 | 100 |
Classification accuracy (%) | 92.8 | 94.6 | 97.4 | 98.2 | 96.6 |
Pulses | Target class | |||||||||||
1 | 2 | 3 | 4 | 5 | 6 | 7 | 8 | 9 | 10 | Precision | ||
![]() |
1 | 48 | 0 | 0 | 0 | 0 | 0 | 0 | 0 | 0 | 0 | 100% |
2 | 0 | 50 | 0 | 0 | 1 | 0 | 0 | 0 | 0 | 0 | 98% | |
3 | 0 | 0 | 49 | 0 | 0 | 0 | 0 | 0 | 0 | 0 | 100% | |
4 | 0 | 0 | 1 | 50 | 0 | 0 | 0 | 0 | 0 | 0 | 98% | |
5 | 0 | 0 | 0 | 0 | 49 | 0 | 0 | 0 | 0 | 0 | 100% | |
6 | 0 | 0 | 0 | 0 | 0 | 50 | 0 | 0 | 0 | 0 | 100% | |
7 | 1 | 0 | 0 | 0 | 0 | 0 | 47 | 0 | 1 | 0 | 95.9% | |
8 | 0 | 0 | 0 | 0 | 0 | 0 | 0 | 50 | 0 | 1 | 98% | |
9 | 0 | 0 | 0 | 0 | 0 | 0 | 3 | 0 | 49 | 0 | 94.2% | |
10 | 1 | 0 | 0 | 0 | 0 | 0 | 0 | 0 | 0 | 49 | 98% | |
Recall | 96% | 100% | 98% | 100% | 98% | 100% | 94% | 100% | 98% | 98% | 98.2% |
Pulse Type | 1 | 2 | 3 | 4 | 5 | 6 | 7 | 8 | 9 | 10 | |
![]() |
Model 1 | 98 | 90.7 | 91.9 | 91.1 | 93.1 | 98.1 | 88.9 | 97.2 | 83.7 | 95.1 |
Model 2 | 97.2 | 92.9 | 98 | 97.2 | 96 | 97.1 | 85.2 | 99 | 84.4 | 100 | |
Model 3 | 100 | 97.1 | 100 | 98 | 99 | 100 | 92.5 | 99 | 89.8 | 99 | |
Model 4 | 98 | 99 | 99 | 99 | 99 | 100 | 95 | 99 | 96.1 | 98 | |
Model 5 | 92.4 | 93.9 | 99 | 97 | 98.1 | 97 | 99 | 99 | 96 | 96 | |
Avg. | 97.1 | 94.7 | 97.6 | 96.5 | 97.0 | 98.4 | 92.1 | 98.6 | 90.0 | 97.6 |
Research | Data | Feature Dimension | Classes | Classifier | Accuracy |
Wang and Cheng [16] | 407 | 13 | 5 pulse types | Bayesian networks | 84.20% |
Xu et al. [31] | 900 | n/a | 6 pulse types | wavelet network | 83% |
Xu et al. [17] | n/a | 4 | 7 pulse types | Lempel-Ziv complexity analysis | 97.10% |
Shu and Sun [19] | n/a | 4 | 13 pulse types | n/a | n/a |
Xu et al. [18] | 320 | 17 | 16 pulse types | FNN | 90.25% |
The proposed method | 500 | 71 (w/o PCA) | 10 pulse types | ANN | 96.60% |
500 | 27 (w. PCA) | 10 pulse types | ANN | 98.20% |