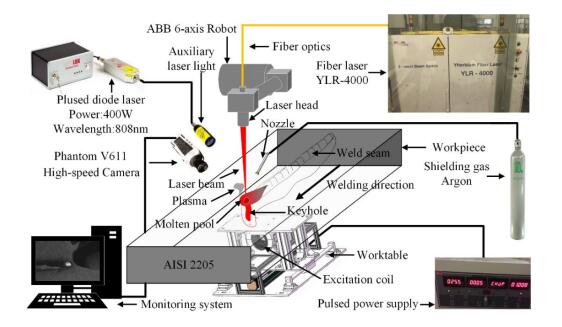
Citation: Wang Cai, Jianzhuang Wang, Longchao Cao, Gaoyang Mi, Leshi Shu, Qi Zhou, Ping Jiang. Predicting the weld width from high-speed successive images of the weld zone using different machine learning algorithms during laser welding[J]. Mathematical Biosciences and Engineering, 2019, 16(5): 5595-5612. doi: 10.3934/mbe.2019278
[1] | Xuewu Wang, Bin Tang, Xin Zhou, Xingsheng Gu . Double-robot obstacle avoidance path optimization for welding process. Mathematical Biosciences and Engineering, 2019, 16(5): 5697-5708. doi: 10.3934/mbe.2019284 |
[2] | Qiaokang Liang, Jianyong Long, Yang Nan, Gianmarc Coppola, Kunlin Zou, Dan Zhang, Wei Sun . Angle aided circle detection based on randomized Hough transform and its application in welding spots detection. Mathematical Biosciences and Engineering, 2019, 16(3): 1244-1257. doi: 10.3934/mbe.2019060 |
[3] | Yuquan Meng, Manjunath Rajagopal, Gowtham Kuntumalla, Ricardo Toro, Hanyang Zhao, Ho Chan Chang, Sreenath Sundar, Srinivasa Salapaka, Nenad Miljkovic, Placid Ferreira, Sanjiv Sinha, Chenhui Shao . Multi-objective optimization of peel and shear strengths in ultrasonic metal welding using machine learning-based response surface methodology. Mathematical Biosciences and Engineering, 2020, 17(6): 7411-7427. doi: 10.3934/mbe.2020379 |
[4] | Yifan Gu, Hua Xu, Jinfeng Yang, Rui Li . An improved memetic algorithm to solve the energy-efficient distributed flexible job shop scheduling problem with transportation and start-stop constraints. Mathematical Biosciences and Engineering, 2023, 20(12): 21467-21498. doi: 10.3934/mbe.2023950 |
[5] | Yufeng Qian . Exploration of machine algorithms based on deep learning model and feature extraction. Mathematical Biosciences and Engineering, 2021, 18(6): 7602-7618. doi: 10.3934/mbe.2021376 |
[6] | Naigong Yu, Hongzheng Li, Qiao Xu . A full-flow inspection method based on machine vision to detect wafer surface defects. Mathematical Biosciences and Engineering, 2023, 20(7): 11821-11846. doi: 10.3934/mbe.2023526 |
[7] | Jingchao Jiang, Chunling Yu, Xun Xu, Yongsheng Ma, Jikai Liu . Achieving better connections between deposited lines in additive manufacturing via machine learning. Mathematical Biosciences and Engineering, 2020, 17(4): 3382-3394. doi: 10.3934/mbe.2020191 |
[8] | Qian Shao, Rongrong Xuan, Yutao Wang, Jian Xu, Menglin Ouyang, Caoqian Yin, Wei Jin . Deep learning and radiomics analysis for prediction of placenta invasion based on T2WI. Mathematical Biosciences and Engineering, 2021, 18(5): 6198-6215. doi: 10.3934/mbe.2021310 |
[9] | Juan Du, Junying Wang, Xinghui Gai, Yan Sui, Kang Liu, Dewu Yang . Application of intelligent X-ray image analysis in risk assessment of osteoporotic fracture of femoral neck in the elderly. Mathematical Biosciences and Engineering, 2023, 20(1): 879-893. doi: 10.3934/mbe.2023040 |
[10] | Yudong Zhang, Juan Manuel Gorriz, Deepak Ranjan Nayak . Optimization Algorithms and Machine Learning Techniques in Medical Image Analysis. Mathematical Biosciences and Engineering, 2023, 20(3): 5917-5920. doi: 10.3934/mbe.2023255 |
Nomenclature and Abbreviations: BPNN: Back Propagation Neural Network; SVR: Support Vector Regression; RBFNN: Radial Based Function Neural Network; SVM: Support Vector Machine; PCA: Principal Component Analysis; PCs: Principal Components; ICI: Inline Coherent Imaging; MAE: Mean Absolute Error; RMSE: Root Mean Square Error; ED: Experiment Data; PD: Predicted Data; PE: Prediction Error; GPA: Global Prediction Accuracy; LPA: Local Prediction Accuracy
Laser welding obtains increasingly widespread application in the automotive industry, shipbuilding industry, aerospace, and microelectronics industry [1,2,3,4,5], due to its prominent merits such as high density of laser power, high productivity in industry, high penetration of weld seam, and the process parameters are easy to be controlled. External magnetic field assisted laser welding can ameliorate the weld quality to some extent by regulating the plasma, changing the mass transfer and heat transfer process of the weld zone [6,7]. The fluctuation of keyhole and molten pool still exist, this instability may cause the uneven penetration of weld seam and weld defects like pores and spatters [8,9]. To guarantee the quality of weld seam, it is an effective way to monitor keyhole [10] and molten pool [11] on-line with advanced sensor system to reflect weld quality by predicting the weld penetration, weld width or weld defects. This monitoring technique can be mainly divided into two important parts.
On the one hand, the intense laser-induced plasma [12] and metal vapor flames [13] cause the keyhole and molten pool are difficult to be monitored on-line to capture clear useful images. Consequently, A great quantity of exploration work has been done by many scientific researchers in the selection of visual monitoring equipment and the construction of the monitoring platform. Fan et al. [14] devised a three-optical-route visual sensor which can monitor the molten pool synchronously from three directions. Useful image sequences were recorded for extracting data. Fabbro et al. [15] observed the dynamic changes of keyhole by applying a coaxial camera and a paraxial photodiode. The top surface of keyhole was captured with ideal precision, but the inside morphology was hard to obtain. Kaierle et al. [16] researched coaxial monitoring system with charge-coupled device or complementary metal oxide semiconductor camera, and in [17], a near-infrared camera was added to this system to acquire the temperature distribution of welding zone. In recent years, the methods for obtaining internal geometry information of keyhole (such as the depth of keyhole) have been gradually increased [18,19]. Webster et al. [20,21] applied the inline coherent imaging (ICI) technique to obtain keyhole depth. The ICI metrical beam coincides with laser beam but does not interact with each other. ICI also owns the advantage like high speed and high dynamic range, but the expensive equipment may hinder its application in industry. In this paper, an on-line vision monitoring system based on high-speed camera is built to obtain keyhole and molten pool images. Results showed that the obtained data can be used to predict the weld quality after image processing.
On the other hand, it is essential to choose efficient algorithms to predict weld penetration, weld width or weld defects by analyzing the monitoring data. The application of machine learning algorithms in the establishment of weld quality forecasting model is more and more widespread [22,23,24]. Among them, the back propagation neural network (BPNN), radial basis function neural network (RBFNN) and support vector machine (SVM) are commonly used algorithms, and acquired reliable and credible results. Gao et al. [25] applied magneto optical imaging technique which based on the magnetic induction principle and Faraday rotation effect [26] to monitor welding process, and BPNN was used to diagnose weld defects. Results achieved a degree of accuracy with 93.5%. Wang et al. [27] used two machine learning algorithms (i.e. multiple linear regression and BPNN) to evaluate the weld quality by analyzing the monitoring data. Results showed that BPNN had a better performance. System error and measurement error are the common problem in monitoring and these errors also have the characteristics of non-linear and unknown distribution. Luo et al. [28] predicted the penetration of weld seam and inclination angle with RBFNN system in the transient state when process parameters changed abruptly. SVM is kernel based machine learning algorithm which is used in defects classification works and regression problems (i.e. support vector regression (SVR) model). The morphological geometry of metal vapor plume is related to the stability of welding process. Wang et al. [29,30] used improved SVM model to classify these dynamic features and the classification accuracy was higher than 90%. In [31], the established predicting model based on SVM and cross validation method also successfully classified weld defects (porosity) with high accuracy. The BPNN, RBFNN and SVM models all have their own advantages in laser welding monitoring. Choosing the suitable algorithm can reduce a lot of prophase work and increase prediction accuracy, above three basic machine learning algorithms were compared in this paper, aim to provides a guideline for the selection of the algorithm.
In this paper, an on-line vision system is established to continuously capture the keyhole and molten pool images. The welding zone is illuminated by the pulsed laser-assisted illumination equipment. After image processing and feature extraction, six keyhole features and one molten pool feature are extracted from the captured images. To improve the modeling efficiency, the principal component analysis (PCA) algorithm is applied to reduce the dimension of the extracted data. Then, different number of principal components (PCs) are chosen as the input data of these three models. Weld width is an important parameter for evaluating weld quality which will be used as the output data. Finally, the BPNN, RBFNN and SVR models are established and the prediction performances of these models are compared. With the help of these advanced algorithms, the weld quality can be monitored in real time. This work aims to find suitable methods to predict the weld width by comparing different PCs and machine learning algorithms. The results show the effectiveness of the applied algorithms and this work can provide a guideline for the selection of machine learning algorithm during laser welding on-line monitoring.
The remainder of this paper is organized as follows. In Section 2, the experimental details of the external magnetic field assisted laser welding on the AISI 2205 duplex stainless steel plates are given. The description of the image process and feature extraction of the keyhole and molten pool is presented in Section 3. In Section 4, three different machine learning algorithms are compared under different number of PCs. In section 5, the results and discussion about BPNN, SVR and RBFNN models are given. Finally, the concluding remarks are given in Section 6.
Figure 1 demonstrates the equipments of laser welding on-line monitoring. An ABB 6-axis robot (IRB 4400 M 2004), and a ytterbium-doped fiber laser device (IPG YLR-4000, 4 kW), a worktable that can supply different magnetic field by changing the current, and an argon shielding gas equipment (flow rate: 25 L/min) are assembled as the welding system. The AISI 2205 duplex stainless steel plates are machined to the appropriate size (i.e. 150 × 100 × 4 mm3) for welding. Table 1 shows the chemical composition in weight percent of the base metal. The diameter of the light spot on workpiece is about 300 μm by utilizing a focusing lens which is installed in the laser head and the focus length is 250 mm. A phantom V611 high-speed camera (Fps: 5000 frame/s, 500 pixels × 256 pixels) and an auxiliary pulsed diode laser light illuminant system (wavelength: 808nm, 400W) are equipped at the side of the worktable to photograph the keyhole and molten pool. A narrow-band filter is installed in front of the high-speed camera to filter the radiations from plasma, spatters radiation and laser beam etc..
Elements | C | Ni | Mo | Cr | Mn | Si | Fe |
Composition | 0.025 | 5.53 | 3.03 | 22.73 | 1.89 | 0.34 | Balance |
Two experiments results are compared in this paper. The welding speed is 1m/min. The laser power is 3 kW and magnetizing current are 10 A and 20 A. The high-speed camera takes images at the speed of 5000 f/s. 5500 consecutive images in two experiments are selected at the same section on two weld seams. The length of the weld seam is divided into the same amount as the captured images. The information of each image corresponds to each weld width. Two weld seams are scanned to high-resolution (4898pixels × 803pixels) images for obtaining the weld width. The upper edge and the lower edge of the weld seam are extracted by applying image processing method. The weld width could be obtained by calculating the distance between the upper and the lower edges of the weld seam. Figure 2 shows the detailed implementation procedures in MATLAB 2016a. The definition features were extracted and analyzed with PCA algorithm, and the corresponding weld width data were also obtained. Finally, the obtained data were applied to train and test the BPNN, RBFNN and SVR models.
In this section, the method for obtaining features of the keyhole and molten pool is introduced in detail. Seven features are defined and the schematic of these features are shown in Figure 3. The width of keyhole (Wk), the length of keyhole (Lk), the area of keyhole (Ak), the perimeter of keyhole (pk), the center coordinates of keyhole (Xk, Yk) and the width of molten pool (Wm) are defined according to the appearance of the keyhole and molten pool.
Figure 4 shows the detailed information of the extraction process of the keyhole features. The first step is to take out the keyhole part from the original image to remove a large amount of useless information and reduce the influence of the surrounding spatters and other noises, so the information of the keyhole will be more accurate. Then, a linear grayscale transformation algorithm is applied to the intercepted image to linearly stretch treat each pixel of the image, aim to enhance the contrast between the keyhole and the surroundings. Next, the median filtering technology is used to eliminate noise interferences such as outliers and line segments. Threshold segmentation operating converts the filtered image into the binary image so that the keyhole will be composed of white pixels and the surroundings will be filled with black pixels. Finally, the keyhole morphological data can be obtained by counting the number of white pixels.
As shown in Figure 3, the molten pool is symmetric about the center coordinates (Xk, Yk) of the keyhole. Consequently, the molten pool can be divided into two equal parts. Half width of molten pool can be obtained by image processing of clearly illuminated part by auxiliary light, so the whole weld width will be acquired. The molten pool image processing steps are the same with keyhole image processing. The detailed information is shown in Figure 5.
Figure 6 and Figure 7 exhibit the curves of seven features extracted from 5500 images. It can be seen that the features extracted from different experiments (10 A and 20 A) have the same tendency. The keyhole features are stable with few deviated points except the Xk. The value of Xk become smaller during laser welding, indicating that the weld seam deviates from the default straight line. The curve about the width of molten pool fluctuates sharply which means the molten pool is very unstable during laser welding.
Reducing the dimension of data helps to increase modeling efficiency. In this paper, the PCA algorithm [33] is used to reduce the dimensions of the input data. In this paper, the feature vector Ni consists 7 features and every feature combined with 5500 samples. In these samples, Nmax and Nmin are the maximal and minimal values. The feature vector N was normalized between –1 and 1 by the formula below,
ˆNi=2(Ni−Nmin)Nmax−Nmin−1,i=1,2,⋯5500 | (1) |
Then the PCA algorithm will transform ˆNi into a new vector Mi with the Eq 2,
Mi=LTˆNi | (2) |
where L is the 7 × 7 orthogonal matrix. The tth eigenvector of the I is the tth column xt. By using the Eq 4, the eigen value problem can be solved.
I=15500ˆNiTˆNi | (3) |
λtx=Ixt,t=1,2,⋯7 | (4) |
In Eq 4, λt is one of the eigen value of I and xt is the corresponding eigenvector. The component Mi can be calculated as the orthogonal transformations of ˆNi, i.e.,
Mi=xtTˆNi,t=1,2,⋯7 | (5) |
This method can convert a set of potentially related variables into a set of independent linear variables which were called the PCs. Different number of PCs will be selected as the inputs of three models which will be introduced in Section 4. The process of dimensional reduction with PCA algorithm is shown in the Figure 8. There are eight important steps during the PCA process.
Seven PCs are obtained and variance of each PC are shown in Figure 9. In order to get the appropriate dimension after PCA processing as the input data. The different number of PCs are chosen train the models for comparing their performance, including 2 PCs, 3 PCs, 4 PCs, 5 PCs. The prediction accuracy of models could be improved as much as possible with accurately input PCs.
The network structure of BPNN is a forward multilayer networks [34]. The learning process consists of two steps: namely positive propagation of signals and backward propagation of errors. The input samples are processed by the hidden layer (one or more), and the results will be delivered to the output layer. If the actual output of the output layer does not match the expected outcome, then the reverse propagation phase of the error would be reversed.
During the initialized phase, the number of neurons in input layer, hidden layer, and output layer are set as n, m and l. The threshold of hidden layer and output layer are set as α and β. The f is the active functions of hidden layer.
The output Hj of neuron j in hidden layer can be calculated by the fellow formula,
Hj=f(n∑i=1wijxi+αj),i=1,2,3,⋯,n;j=1,2,3,⋯,l | (6) |
where wij indicates the connection weigh between the input layer neuron i and the hidden layer neuronj, xi is the input variable and αj denotes the threshold of neuron k in the output layer.
Then the result Qk of the output layer would be obtained by Eq 7,
Qk=l∑j=1Hjwjk−βk,k=1,2,3,⋯,m;j=1,2,3,⋯,l | (7) |
where Yk is the desired outcome, so the predicted error Ek can be calculated by Eq 8,
Ek=Yk−Qk,k=1,2,3,⋯,m | (8) |
The smaller the predicted error Ek, the better predicted performance. The adjusted weight will change the output. The weight wij connects the input layer and the hidden layer will be updated by Eq 9. The weight wjk connects the hidden layer and output layer will be replaced by Eq 10. Thresholds of hidden layer and output layer are renovated by Eq 11 and Eq 12, respectively.
wij=wij+ξHj(1−Hj)x(i)m∑k=1wijek,i=1,2,3,⋯,n;k=1,2,3,⋯,m;j=1,2,3,⋯,l | (9) |
wjk=wjk+ξHjek,k=1,2,3,⋯,m;j=1,2,3,⋯,l | (10) |
αj=αj+ξHj(1−Hj)x(i)m∑k=1wjkek,k=1,2,3,⋯,m;j=1,2,3,⋯,l | (11) |
βk=βk+Ek,k=1,2,3,⋯,m | (12) |
For each sample, if the error is smaller than the threshold or reaching the maximum iterations, the training process will be finished. Otherwise the training process will go back to continue the above procedures again.
The RBFNN [35] model uses discrete multivariate data to fit unknown functions. The core of this model is to use the function
F(x)=m∑i=1αiψ(ri,a), αi=[α1α2⋮αm], ψ(ri,a)=[ψ(r1,a)ψ(r2,a)⋮ψ(rm,a)] | (13) |
Then the simulation function can be expressed as,
⌢y(x)=f(x)=m∑i=1αiψ(ri,a)(‖x−xi‖) | (14) |
where the distance ri(x)=‖x−xi‖ is the ith sampling point x in the design space xi. ψ(r) is the basis function.
When using the above equation as a predicted model, the following interpolation conditions must be satisfied,
f(xi)=F(xi),i=1,2,⋯,m | (15) |
Then the equations are got as below,
Ψα=F,Ψ=[ψ(‖x1−x1‖)ψ(‖x1−x2‖)⋯ψ(‖x1−xm‖)ψ(‖x2−x1‖)ψ(‖x2−x2‖)⋯ψ(‖x2−xm‖)⋮⋮⋮ψ(‖xm−x1‖)ψ(‖xm−x2‖)⋯ψ(‖xm−xm‖)],F=[f(x1)f(x2)⋮f(xm)] | (16) |
When the sample points do not coincide, there is a unique solution when ψ(r) is the positive definite function, give the result as Eq 17.
α=Ψ−1F | (17) |
The typical RBFNN structure consisted of an input layer, a hidden layer, and an output layer. It reflects the functional relationship f:x→⌢y between multiple inputs x∈Rn and multiple outputs y∈Rn of the system. The input node passes the signal to the hidden layer, and the output node is usually a simple linear function.
The SVR model [36] is based on the application of SVM model to regression problems [37]. SVR has excellent non-linear processing capability, especially when dealing with high-dimensional data. Each sample point would be mapped to a high-dimensional feature space by using a nonlinear function. Then performing linear regression in the high-dimensional feature space to achieve the effect of linear regression in the original space. The functionfcan be expressed as blew,
f(xi)=wTφ(x)+a | (18) |
where w is the weight vector and the a is constant. To reduce the risk caused by w, the structural risk functions in Eq 19 is introduced,
F(w)=12wTw+m∑i=1ε[g(xi)] | (19) |
According to the structural risk criterion ε in the statistics as the loss function, the minimization objective function in Eq 20 is used to obtain w and a.
F(w,ξi,ξ∗i)=min(12wTw+Cm∑i=1(ξi,ξ∗i))s.t.{yi−wφ(x)−a⩽δ+ξiwφ(x)+a−yi⩽δ+ξ∗iξi⩾0,ξ∗i⩾0 | (20) |
where C is the penalty parameter. ξi and ξ∗i denote the introduced slack variables. δ is the pre-set value and yi, yi∈{−1,1} is the mappings of sample space. Therefore, the minimum solution of the objective function F(w,ξi,ξ∗i) is obtained. The output of the SVR model is shown in Eq 21.
f(x,αi,α∗i)=m∑i=1αiK(x,xi)+a | (21) |
where K(x,xi) is the kernel function. The f is the output result and x is the multidimensional space vector.
When the magnetizing current is 10 A, the weld width is shown in Figure 10. From Section 3.3, seven features are extracted from 5, 500 continuously images, the samples of weld width numbered from 1, 001 to 6, 500 are corresponding to those analyzed 5500 images of the keyhole and molten pool. Consequently, 5500 samples of 2 PCs, 3 PCs, 4 PCs and 5 PCs are chosen as the input data of these three models and the corresponding weld width are selected as the output data. The former 5000 samples are chosen as the training set and the last 500 samples are used as testing set to establish the BPNN, RBFNN and SVR models. The ratio of training samples to testing samples is 10:1. To make these three models rapidly converge, all the samples in the training set and testing set are normalized to the interval of [0, 1] in MATLAB 2016a.
To judge which PCs is the best combination of PC to establish three models, and which model owns the best performance. The mean absolute error (MAE) and the root mean square error (RMSE) [38,39,40] are used as the accuracy metrics. The MAE is the average of the absolute error which can reflect the actual situation of the prediction error (PE). The local prediction accuracy (LPA) of model will be better if the MAE is smaller. As for RMSE, it can be used to ensure the accuracy index consistent with the unit of output performance. RMSE is more commonly used in the global accuracy test of model. The smaller the RMSE is, the higher the global prediction accuracy (GPA) of the model is.
PE=(ˆyi(xi)−yi(xi))/(ˆyi(xi)−yi(xi))yi(xi)yi(xi);xi∈Xt | (22) |
MAE=1tt∑i=1|yi(xi)−ˆyi(xi)|;xi∈Xt | (23) |
RMSE=√1tt∑i=1(yi(xi)−ˆyi(xi))2;xi∈Xt | (24) |
where yi(xi) represents experimental data (ED) and ˆyi(xi) is the corresponding predicted data (PD). The Xt is the set of verification points, t is the number of verification points and xi is the verification point.
The results of MAE and RMSE of three models with different PCs are shown in Figure 11.
It can be concluded from Figure 11 that it is better to choose 2 PCs as input data, due to the MAE and RMSE are obvious smaller than other PCs. When 2 PCs are chosen as input data, the PE curves of three models are calculated with 500 ED samples from the testing set and their corresponding PD. The PE curves, MAE and RMSE values of models are shown in Figure 12.
The PE curve of BPNN model is smoothest, and BPNN model has the smallest MAE. The RMSE of BPNN model is bigger than SVR model, but the PE curve of the SVR model fluctuates sharply from No. 5300 to No. 5500. Synthetically speaking, BPNN model performs better than SVR model. As for RBFNN model, the PE curve fluctuates acutely and has the biggest MAE and RSME among three models, so the performance of the RBFNN model is worst. All in all, BPNN model performs better than SVR model and SVR model performs worst when the magnetizing current is 10A.
When the magnetizing current changed to 20 A and other conditions are the same with the 10 A experiment. By applying the image processing method, the weld width is extracted and shown in Figure 13.
In MATLAB 2016a, the same methods are taken to deal with the normalized 5000 training data and 500 testing data. The results of MAE and RMSE of three models with different PCs are shown in Figure 14.
It can be concluded from Figure 14 that the BPNN model and SVR model have almost similar performance no matter which PCs is chosen. When choose 4 PCs and 5 PCs as input data, RBFNN model performs badly due to big MAE. The 3 PCs owns better RMSE than 2 PCs and almost identical MAE. Comprehensive speaking, it is better to choose 3 PCs as the input data. The PE curves, MAE and RMSE values of models are shown in Figure 15.
From Figure 15, the MAE and RMSE of BPNN is smaller than SVR model and the PE curve is also smoother. Consequently, BPNN model performs better than SVR model. RBFNN model has the biggest MAE and RMSE, and the PE curve fluctuates sharply. Overall, the performance of BPNN model is better than SVR model, and RBFNN model is worst when compared with BPNN model and SVR model.
It can be concluded from the above two different experiments that BPNN model performs better than SVR model, and RBFNN model performs worst.
In this research, an on-line monitoring system is established to observe the dynamic behavior of the keyhole and molten pool and the prediction performance of three machine learning algorithms are compared with different PCs as inputs in two different experiments. A high-speed camera is used to capture the images of the keyhole and molten pool, which are closely associated with the welding quality. With image processing and feature extraction, seven features are obtained. Then the PCA algorithm is applied to reduce the redundancy of data to accelerate the training process. Finally, the prediction accuracy of three machine learning algorithms under different chosen PCs are compared in detail. Following conclusions can be drawn:
(1) An advanced high-speed visual image capturing system is devised to capture the morphology information of the keyhole and molten pool. Results show that this visible welding tracking system is effective and practical.
(2) The PCA algorithm is used to reduce the data redundancy to enhance modeling efficiency. The performance of different PCs is distinct. Results show that reduce data dimension is helpful to improve prediction accuracy.
(3) The BPNN, RBFNN, and SVR models are established to model the relation between the weld width and the chosen PCs which contains the information of keyhole and molten pool. The prediction accuracy are compared by comparing the MAE, RMSE and PE results. Results indicate that the BPNN model has best performance, and SVR model is better than RBFNN model.
Overall, this work shows that machine learning algorithms can accurately predict welding quality in real time. Among them, BPNN works best, and effective machine learning algorithm is the key to realize intelligent welding. In recent years, deep learning is increasingly exploited in welding process monitoring and process control. Future research should focus on the combination of multi-sensor and deep learning, through powerful big data processing capabilities of deep learning to process the information collected by multiple sensor fusion technology, the efficiency and reliability of welding process monitoring will increase significantly.
This research has been supported by National Natural Science Foundation of China (NSFC) under Grant No. 51775203, No. 51721092 and No. 51861165202, the Fundamental Research Funds for the Central Universities, HUST: Grant No. 2016YXMS272.
All authors declare no conflicts of interest in this paper.
[1] | E. Assunção, L. Quintino and R. Miranda, Comparative study of laser welding in tailor blanks for the automotive industry, Int. J. Adv. Manuf. Tech., 49 (2010), 123–131. |
[2] | X. Cao, M. Jahazi, J. Immarigeon, et al., A review of laser welding techniques for magnesium alloys,J. Mater. Process. Tech., 171 (2006), 188–204. |
[3] | R. T. Yang and Z. W. Chen, A study on fiber laser lap welding of thin stainless steel, Int. J. Precis. Eng. Man., 14 (2013), 207–214. |
[4] | J. Stavridis, A. Papacharalampopoulos and P. Stavropoulos, Quality assessment in laser welding: a critical review, Int. J. Adv. Manuf. Tech., 94 (2017), 1825–1847. |
[5] | M. Jager and F. A. Hamprecht, Principal component imagery for the quality monitoring of dynamic laser welding processes, IEEE T. Ind. Electron., 56 (2008), 1307–1313. |
[6] | L. E. Afanas'eva, I. A. Barabonova, P. O. Zorenko, et al., Laser welding in external electrical and magnetic fields, Weld. Int., 27 (2013), 545–547. |
[7] | A. Schneider, V. Avilov, A. Gumenyuk, et al., Laser beam welding of aluminum alloys under the influence of an electromagnetic field, Phys. Procedia, 41 (2013), 4–11. |
[8] | J. Volpp, Keyhole stability during laser welding-Part II: process pores and spatters, Prod. Eng. Res. Devel., 11 (2016), 9–18. |
[9] | J. Stavridis, A. Papacharalampopoulos and P. Stavropoulos, A cognitive approach for quality assessment in laser welding, Procedia CIRP, 72 (2018), 1542–1547. |
[10] | C. H. Kim and D. C. Ahn, Coaxial monitoring of keyhole during Yb:YAG laser welding, Opt. Laser Technol., 44 (2012), 1874–1880. |
[11] | A. Matsunawa, J. D. Kim, N. Seto, et al., Dynamics of keyhole and molten pool in laser welding, J. Laser Appl., 10 (1998), 247–254. |
[12] | G. Chen, M. Zhang, Z. Zhao, et al., Measurements of laser-induced plasma temperature field in deep penetration laser welding, Opt. Laser Technol., 45 (2013), 551–557. |
[13] | J. Wang, C. Wang, X. Meng, et al., Study on the periodic oscillation of plasma/vapour induced during high power fibre laser penetration welding, Opt. Laser Technol., 44 (2012), 67–70. |
[14] | C. Fan, F. Lv and S. Chen, Visual sensing and penetration control in aluminum alloy pulsed GTA welding, Int. J. Adv. Manuf. Tech., 42 (2009), 126–137. |
[15] | R. Fabbro, S. Slimani, F. Coste, et al., Study of keyhole behaviour for full penetration Nd–Yag CW laser welding, J. Phys. D. Appl. Phys., 38 (2005), 1881. |
[16] | K. Stefan, Process monitoring and control of laser beam welding: Measuring quantifiable data for improved processing results, Laser Technik J., 5 (2008), 41–43. |
[17] | K. Stefan, Understanding the Laser Process new approaches for Process monitoring in laser materials Processing, Laser Technik J., 7, (2010), 49–52. |
[18] | Z. Al-Sarraf and M. Lucas, A study of weld quality in ultrasonic spot welding of similar and dissimilar metals, J. Phys. Conference Series, 382 (2012), 012013. |
[19] | L. Yang and I. C. Ume, Inspection of simulated weld penetration depth using laser-generated Lamb waves and wavelet signal processing, AIP Conference Proceedings. AIP, 1650 (2015), 1386–1391. |
[20] | M. A. Maher, P. J. L. Webster, J. C. Chiao, et al., Coaxial real-time metrology and gas assisted laser micromachining: process development, stochastic behavior, and feedback control, Micromachining and Microfabrication Process Technology XV. International Society for Optics and Photonics, 7590 (2010), 759003. |
[21] | P. J. L. Webster, G. L. Wright, Y. Ji, et al., Automatic laser welding and milling with in situ inline coherent imaging, Opt. Lett., 39 (2014), 6217–6220. |
[22] | A. Khan, B. Baharudin, L. H. Lee, et al., A review of machine learning algorithms for text-documents classification, J. Adv. Inform. Tech., 1 (2010), 4–20. |
[23] | P. Aivaliotis, A. Zampetis, G. Michalos, et al., A machine learning approach for visual recognition of complex parts in robotic manipulation, Procedia Manuf., 11 (2017), 423–430. |
[24] | K. Kokkalis, G. Michalos, P. Aivaliotis, et al., An approach for implementing power and force limiting in sensorless industrial robots, Procedia CIRP, 76 (2018), 138–143. |
[25] | X. Gao, C. Lan, D. You, et al., Weldment Nondestructive Testing Using Magneto-optical Imaging Induced by Alternating Magnetic Field,J. Nondestruct. Eval., 36 (2017), 55. |
[26] | T. K. Xia, P. M. Hui and D. Stroud, Theory of faraday rotation in granular magnetic materials, J. Appl. Phys., 67 (1990), 2736–2741. |
[27] | X. Wan, Y. Wang, D. Zhao, et al., Weld quality monitoring research in small scale resistance spot welding by dynamic resistance and neural network, Measurement, 99 (2017), 120–127. |
[28] | M. Luo and Y. C. Shin, Estimation of keyhole geometry and prediction of welding defects during laser welding based on a vision system and a radial basis function neural network, Int. J. Adv. Manuf. Tech., 81 (2015), 263–276. |
[29] | T. Wang, J. Chen, X. Gao, et al., Quality Monitoring for Laser Welding Based on High-Speed Photography and Support Vector Machine, Appl. Sci., 7 (2017), 299. |
[30] | T. Wang, J. Chen, X. Gao, et al., Real-time monitoring for disk laser welding based on feature selection and SVM, Appl. Sci., 7, (2017), 884. |
[31] | Z. Zhang, E. Kannatey-Asibu, S. Chen, et al., Online defect detection of Al alloy in arc welding based on feature extraction of arc spectroscopy signal, Int. J. Adv. Manuf. Tech., 79 (2015), 2067–2077. |
[32] | J. Michalska and M. Sozańska, Qualitative and quantitative analysis of σ and χ phases in 2205 duplex stainless steel, Mater. Charact., 56 (2006), 355–362. |
[33] | S. M. Holland, Principal components analysis (PCA), Department of Geology, University of Georgia, Athens,GA (2008), 30602–2501. |
[34] | M. Buscema, Back propagation neural networks, Int. J. Addictions, 33 (1998), 233–270. |
[35] | S. Chen, C. F. Cowan and P. M. Grant, Orthogonal least squares learning algorithm for radial basis function networks, IEEE T. Neural Networ., 2 (1991), 302–309. |
[36] | H. Drucker, C. J. Burges, L. Kaufman, et al., Support vector regression machines, Adv. Neural Inform. Process. Sys., (1997), 155–161. |
[37] | S. Altarazi, L. Hijazi and E. Kaiser, Process parameters optimization for multiple-inputs- multiple-outputs pulsed green laser welding via response surface methodology, 2016 IEEE International Conference on Industrial Engineering and Engineering Management (IEEM). IEEE, (2016), 1041–1045. |
[38] | C. J. Willmott, Some comments on the evaluation of model performance, B. Am. Meteorol. Soc., 63 (1982), 1309–1313. |
[39] | J. Qian, J. Yi, Y. Cheng, et al., A sequential constraints updating approach for Kriging surrogate model-assisted engineering optimization design problem, Eng. Comput-Germany., (2019), 1–17. |
[40] | Z. H. Han, C. Z. Xu, Z. Liang, et al., Efficient aerodynamic shape optimization using variable-fidelity surrogate models and multilevel computational grids, Chinese J. Aeronaut., (2019). |
1. | Rishikesh Mahadevan R, Avinaash Jagan, Lakshmi Pavithran, Ashutosh Shrivastava, Senthil Kumaran Selvaraj, Intelligent welding by using machine learning techniques, 2021, 22147853, 10.1016/j.matpr.2020.12.1149 | |
2. | Huaping Li, Hang Ren, Zhenhui Liu, Fule Huang, Guangjie Xia, Yu Long, In-situ monitoring system for weld geometry of laser welding based on multi-task convolutional neural network model, 2022, 204, 02632241, 112138, 10.1016/j.measurement.2022.112138 | |
3. | Aditya Raj, Utkarsh Chadha, Arisha Chadha, R. Rishikesh Mahadevan, Buddhi Rohan Sai, Devanshi Chaudhary, Senthil Kumaran Selvaraj, R. Lokeshkumar, Sreethul Das, B. Karthikeyan, R. Nagalakshmi, Vishjit Chandramohan, Haitham Hadidi, Weld quality monitoring via machine learning-enabled approaches, 2023, 1955-2513, 10.1007/s12008-022-01165-9 | |
4. | Weijun Xie, Shuo Wei, Zhaohui Zheng, Zhaoli Chang, Deyong Yang, Developing a stacked ensemble model for predicting the mass of fresh carrot, 2022, 186, 09255214, 111848, 10.1016/j.postharvbio.2022.111848 | |
5. | Xi’an Fan, Xiangdong Gao, Guiqian Liu, Nvjie Ma, Yanxi Zhang, Research and prospect of welding monitoring technology based on machine vision, 2021, 115, 0268-3768, 3365, 10.1007/s00170-021-07398-4 | |
6. | Leilei Wang, Yanqiu Zhao, Yue Li, Xiaohong Zhan, Droplet Transfer Induced Keyhole Fluctuation and Its Influence Regulation on Porosity Rate during Hybrid Laser Arc Welding of Aluminum Alloys, 2021, 11, 2075-4701, 1510, 10.3390/met11101510 | |
7. | Ralph Rudi Schmidt, Jorg Hildebrand, Ivan Kraljevski, Frank Duckhorn, Constanze Tschope, 2022, A Study for Laser Additive Manufacturing Quality and Material Classification Using Machine Learning, 978-1-6654-8464-0, 1, 10.1109/SENSORS52175.2022.9967311 | |
8. | Pornsak Srisungsitthisunti, Boonrit Kaewprachum, Zhigang Yang, Guhui Gao, Real-Time Quality Monitoring of Laser Cladding Process on Rail Steel by an Infrared Camera, 2022, 12, 2075-4701, 825, 10.3390/met12050825 | |
9. | Juan G Vidal, Germán O Barrionuevo, Jorge A Ramos-Grez, Julio Vergara, José L Mullo, Vibration-assisted laser welding: Frequency and amplitude vibration effect on the microhardness of A36 steel, 2022, 236, 1464-4207, 2139, 10.1177/14644207221102659 | |
10. | Xi'an Fan, Perry P. Gao, Xiangdong Gao, Yuhui Huang, Prediction of Weld Widths for Laser-MIG Hybrid Welding Using Informer Model, 2024, 71, 0278-0046, 6221, 10.1109/TIE.2023.3294634 | |
11. | Dong Wang, Yongjia Zheng, Wei Dai, Ding Tang, Yinghong Peng, Deep Network-Assisted Quality Inspection of Laser Welding on Power Battery, 2023, 23, 1424-8220, 8894, 10.3390/s23218894 | |
12. | Yue Qiu, Jiang Ping, Leshi Shu, Minjie Song, Deyuan Ma, Xiuhui Yan, Shixuan Li, Defect monitoring of high-power laser-arc hybrid welding process based on an improved channel attention convolutional neural network, 2024, 0956-5515, 10.1007/s10845-024-02354-x | |
13. | R. Naga Srinu, Ramkumar Madhavan, 2025, 3252, 0094-243X, 020009, 10.1063/5.0259535 | |
14. | Suleiman Elhamali, Hmeda Musbah, Lubna Zawi, Asmaa Shuwehdi, Hajer Faris, Aisha Mahdawe, Artificial Intelligence Meets Laser Technology: A Review of Recent Advances, 2025, 26668459, 100484, 10.1016/j.rsurfi.2025.100484 |
Elements | C | Ni | Mo | Cr | Mn | Si | Fe |
Composition | 0.025 | 5.53 | 3.03 | 22.73 | 1.89 | 0.34 | Balance |