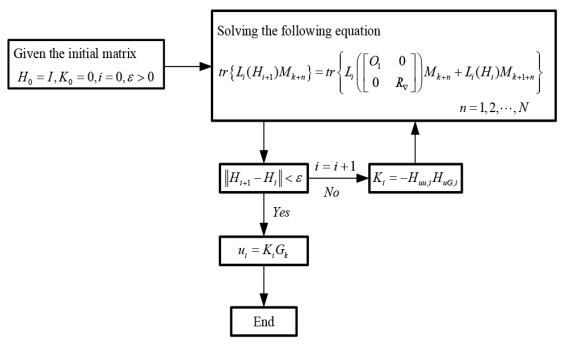
In this paper, we consider a delayed stage-structured predator-prey model incorporating prey refuge with Holling type Ⅱ functional response. It is assumed that prey can live in two different regions. One is the prey refuge and the other is the predatory region. Moreover, in real world application, we should consider the stage-structured model. It is assumed that the prey in the predatory region can divided by two stages: Mature predators and immature predators, and the immature predators have no ability to attack prey. Based on Mawhin's coincidence degree and novel estimation techniques for a priori bounds of unknown solutions to Lu = λNu, some sufficient conditions for the existence of periodic solution is obtained. Finally, an example demonstrate the validity of our main results.
Citation: Weijie Lu, Yonghui Xia, Yuzhen Bai. Periodic solution of a stage-structured predator-prey model incorporating prey refuge[J]. Mathematical Biosciences and Engineering, 2020, 17(4): 3160-3174. doi: 10.3934/mbe.2020179
[1] | Abdon Atangana, Seda İğret Araz . Piecewise differential equations: theory, methods and applications. AIMS Mathematics, 2023, 8(7): 15352-15382. doi: 10.3934/math.2023785 |
[2] | Woocheol Choi, Young-Pil Choi . A sharp error analysis for the DG method of optimal control problems. AIMS Mathematics, 2022, 7(5): 9117-9155. doi: 10.3934/math.2022506 |
[3] | Xumei Zhang, Junying Cao . A high order numerical method for solving Caputo nonlinear fractional ordinary differential equations. AIMS Mathematics, 2021, 6(12): 13187-13209. doi: 10.3934/math.2021762 |
[4] | Xin Liu, Yan Wang . Averaging principle on infinite intervals for stochastic ordinary differential equations with Lévy noise. AIMS Mathematics, 2021, 6(5): 5316-5350. doi: 10.3934/math.2021314 |
[5] | Shifan Luo, Dongshu Wang, Wenxiu Li . Dynamic analysis of a SIV Filippov system with media coverage and protective measures. AIMS Mathematics, 2022, 7(7): 13469-13492. doi: 10.3934/math.2022745 |
[6] | Essam R. El-Zahar, Ghaliah F. Al-Boqami, Haifa S. Al-Juaydi . Piecewise approximate analytical solutions of high-order reaction-diffusion singular perturbation problems with boundary and interior layers. AIMS Mathematics, 2024, 9(6): 15671-15698. doi: 10.3934/math.2024756 |
[7] | Saima Rashid, Fahd Jarad, Sobhy A. A. El-Marouf, Sayed K. Elagan . Global dynamics of deterministic-stochastic dengue infection model including multi specific receptors via crossover effects. AIMS Mathematics, 2023, 8(3): 6466-6503. doi: 10.3934/math.2023327 |
[8] | Wedad Albalawi, Muhammad Imran Liaqat, Kottakkaran Sooppy Nisar, Abdel-Haleem Abdel-Aty . Qualitative study of Caputo Erdélyi-Kober stochastic fractional delay differential equations. AIMS Mathematics, 2025, 10(4): 8277-8305. doi: 10.3934/math.2025381 |
[9] | Yanshou Dong, Junfang Zhao, Xu Miao, Ming Kang . Piecewise pseudo almost periodic solutions of interval general BAM neural networks with mixed time-varying delays and impulsive perturbations. AIMS Mathematics, 2023, 8(9): 21828-21855. doi: 10.3934/math.20231113 |
[10] | Dumitru Baleanu, Babak Shiri . Nonlinear higher order fractional terminal value problems. AIMS Mathematics, 2022, 7(5): 7489-7506. doi: 10.3934/math.2022420 |
In this paper, we consider a delayed stage-structured predator-prey model incorporating prey refuge with Holling type Ⅱ functional response. It is assumed that prey can live in two different regions. One is the prey refuge and the other is the predatory region. Moreover, in real world application, we should consider the stage-structured model. It is assumed that the prey in the predatory region can divided by two stages: Mature predators and immature predators, and the immature predators have no ability to attack prey. Based on Mawhin's coincidence degree and novel estimation techniques for a priori bounds of unknown solutions to Lu = λNu, some sufficient conditions for the existence of periodic solution is obtained. Finally, an example demonstrate the validity of our main results.
It is well known that the optimal tracking control (OTC) problem plays an important role in the field of optimal control and develops fast in applications[1,2,3,4]. The goal of OTC problem is to design a controller, which can make the output of the system track the reference trajectory by minimizing the cost function. Traditional OTC problem is realized by feedback linearization [5] and object inversion [6], but this usually requires complex mathematical analysis. As for the linear quadratic tracking (LQT) problem, the traditional method of LQT problem is to solve the algebraic Riccati equation (ARE) and the noncausal difference equation. However, these methods require accurate system model[7]. In practical situations, the system parameters are partially unknown or completely unknown, so it is impossible to be realized by traditional methods.
The key to the OTC problem is to solve Hamilton-Jacobi-Bellman (HJB) equation. However, HJB equation involves solving difference or differential equations, so it is difficult to solve it. Although dynamic programming has always been an effective method to solve the HJB equation, it is not feasible in the calculation of large dimensions because of "the curse of dimensionality". To solve the solution of the HJB equation, adaptive dynamic programming (ADP) algorithms have been widely used and developed. In [8], a policy iteration (PI) scheme was adopted to approximate the optimal control for the partly unknown continuous-time systems. In [9], B. Kiumarsi solves the LQT problem online only by measuring the input, output, and reference trajectory data of the system. In [10], a Q-learning method was proposed to calculate the optimal control, only relying on system parameters and command generators.
In recent years, stochastic system control theory has become the focus of optimal control theory because of its academic difficulty and wide application, especially the model-free SLQ optimal tracking problem has attracted more and more attention[11,12,13,14,15]. In [14], ADP algorithm based on neural networks is proposed to solve the model-free SLQ optimal tracking control problem. In addition, the Q-learning algorithm is used to solve the model-free SLQ optimal tracking control problem in [15]. For all we know, there seem to be many research results on the model-free SLQ optimal tracking problem based on ADP algorithm, but the SLQ optimal tracking problem with delays has received little attention. Time delay [16] is an important factor that cannot be ignored. It exists in many practical systems, such as industrial processes, power grids, chemical reactions, and so on [17,18,19,20]. However, in these methods[11,12,13,14,15], the influence of time delay on the system is neglected. If the time delay is ignored, it will affect the control effect and even make the system divergence. The method proposed in [16] takes into account the time delay but ignores the influence of stochastic disturbance disturbances on the system. As far as we know, there is no research on the optimal tracking problem of stochastic linear systems with delays. Therefore, how to use ADP algorithm to deal with the model-free SLQ optimal tracking control problem has important practical significance. This is the motivation we study in this paper.
The main contributions of this paper include:
(1) For stochastic linear system, this paper proposes Q-learning to model-free solve SLQ optimal tracking control problem with delays for the first time, which enhances the practicability of ADP algorithm in tracking problems.
(2) By introducing the delay factor, the influence of delays on the subsequent algorithm can be effectively eliminated.
(3) In this paper, the Q-learning algorithm is used to solve the model-free SLQ optimal tracking control problem with delays. Compared with other methods which need accurate system model to obtain the optimal control, this method makes full use of the online system state information to obtain the optimal control and avoids solving augmented stochastic algebraic equation (SAE).
The structure of this paper is organized as follows. In section 2, we give the problem formulation and conversion. In section 3, we derive the Q-learning algorithm and prove its convergence. In section 4, we give the implementation steps of Q-learning algorithm. In section 5, a simulation example is given to verify the effectiveness of the algorithm. In section 6, the conclusion is given.
Consider the following linear stochastic systems with delays
xk+1=Axk+Adxk−d+Buk+Bduk−d+(Cxk+Cdxk−d+Duk+Dduk−d)ωk,yk=Exk+Edxk−d | (2.1) |
where xk∈Rn is the system state vector, uk∈Rm is the control input vector, yk∈Rq is the system output, while xk−d,uk−d and yk−d are the delay variables with delay index d∈N. A∈Rn×n, B∈Rn×m, C∈Rn×n, D∈Rn×m, E∈Rq×n are given constant, Ad∈Rn×n, Bd∈Rn×m, Cd∈Rn×n, Dd∈Rn×m, Ed∈Rq×n are their corresponding delay dynamics matrices. One-dimensional stochastic disturbance sequence ωk is defined on the given probability space (Ω,F,P,Fk), and meets the following condition E(ωk∣Fk)=0, E(ω2k∣Fk)=1. The initial state x0 is irrelevant with ωk.
Assume the reference trajectory of SLQ optimal tracking control is generated by a command generator
rk+1=Frk | (2.2) |
where rk∈Rq represents the reference system trajectory, and F is the constant matrix.
The tracking error can be expressed as
ek=yk−rk | (2.3) |
where rk is the reference trajectory.
The goal of the SLQ optimal tracking problem with delays is to design an optimal controller, which can not only ensure that the output of the target system track the reference trajectory stably, but also minimize the cost function. The cost function is denoted as
J(xk,rk,uk)=E∞∑i=kUi(xi,xi−d,ui) | (2.4) |
where Ui(xi,xi−d,ui)=(yi−ri)TO(yi−ri)+uTiRui+uTi−dRdui−d is the utility function. O=OT∈Rq×q≥0, R=RT∈Rm×m≥0, Rd=RTd∈Rm×m≥0 are the constant matrices.
Only when F is Hurwitz can the cost function (2.4) be used, that is, the reference trajectory system is required to be asymptotically stable. If the reference trajectory does not tend to zero with time delay, then the cost function (2.4) will be unbounded. In practice, this condition is difficult to achieve. Therefore, a discount factor γ is introduced into the cost function (2.4) to relax this restriction. Based on (2.4), the cost function with discount factor is redefined as
J(xk,rk,uk)=E∞∑i=kγi−kUi(xi,xi−d,ui)=E∞∑i=kγi−k(yi−ri)TO(yi−ri)+uTiRui+uTi−dRdui−d | (2.5) |
where 0<γ≤1 is the discount factor.
Definition 1 ([21]). uk is called mean-square stabilizing at e0 if there exists a linear feedback form of uk for every initial state e0 satisfies limk→∞E(eTkek)=0. The system (2.3) with a mean-square stabilizing control uk is called mean-square stabilizable.
Definition 2 ([21]). uk is said to be admissible if uk satisfies the following: (1) uk is a Fk adapted and measurable stochastic process; (2) uk is mean-square stabilizing; (3) It enables the cost function to reach the minimum value.
The goal of this paper is to seek an admissible control, which not only minimizes the cost function (2.5) but also stabilizes the system (2.3) for each initial state e0. We denote the optimal cost function as follows
V(e0)=minuJ(e0,u). | (2.6) |
In order to achieve the above goal, this paper establishes an augmented system composed of system (2.1) and the reference trajectory system (2.2), and then transforms the optimal tracking problem into an optimal regulation problem.
The system (2.1) can be rewritten as the following equivalent form:
xk+1=[AAd][xkxk−d]+[BBd][ukuk−d]+([CCd][xkxk−d]+[DDd][ukuk−d])ωk,yk=[EEd][xkxk−d]. | (2.7) |
According to [16,22,23], we define the delay operator ∇d satisfies ∇dxk=xk−d and (∇dxk)T=xTk−d. Then, the system (2.7) can be expressed as
xk+1=A∇xk+B∇uk+(C∇xk+D∇uk)ωk,yk=E∇xk | (2.8) |
where A∇=A+Ad∇d, B∇=B+Bd∇d, C∇=C+Cd∇d, D∇=D+Dd∇d, E∇=E+Ed∇d.
Based on the system (2.1) and the reference trajectory system (2.2), the augmented system can be defined as
Gk+1=[xk+1rk+1]=[A∇+C∇ωk00F][xkrk]+[B∇+D∇ωk0]uk=TGk+B0uk | (2.9) |
where Gk=[xkrk]∈Rn+q, T∈R(n+q)×(n+q), B0∈R(n+q)×m.
Based on the augmented system (2.9), the cost function (2.5) can be expressed as
J(Gk,uk)=E∞∑i=kγi−k[GTiO1Gi+uTiR∇ui] | (2.10) |
where O1=[E−I]TO[E−I]∈R(n+q)×(n+q), R∇=R+Rd∇d.
The state feedback linear controller is defined as
uk=KGk,K∈Rm×(n+q) | (2.11) |
where K represents the control gain matrix of the system.
Substituting (2.11) into (2.10), the cost function (2.10) can be transformed into
J(Gk,K)=E∞∑i=kγi−kGTi[O1+KTR∇K]Gi. | (2.12) |
Therefore, the target of SQL optimal tracking problem with delays can be further expressed as
V(G0,K)=minKJ(G0,K). | (2.13) |
Definition 3. The SLQ optimal control problem is well posed if
−∞<V(G0,K)<+∞. |
Before solving the SLQ control problem, we need to know whether it is well-posed. Therefore, we give the following lemma first.
Lemma 1. If there exists an admissible control uk=KGk, then the SLQ optimal tracking control is well-posed, and the cost function can be expressed as
J(Gk,K)=E(GTkPGk) | (2.14) |
where the matrix P∈R(n+q)×(n+q) satisfies the following augmented SAE
P=γ(A1+B1K)TP(A1+B1K)+γ(C1+D1K)TP(C1+D1K)+O1+KTR∇K | (2.15) |
where A1=[A∇00F]∈R(n+q)×(n+q), B1=[B∇0]∈R(n+q)×m, C1=[C∇000]∈R(n+q)×(n+q), D1=[D∇0]∈R(n+q)×m.
Proof. Assuming that the control uk is admissible and the matrix P satisfies (2.15), then
E∞∑i=k[γGi+1TPGi+1−GiTPGi]=E∞∑i=k{γ[(A1+B1K)Gi+(C1ωi+D1Kωi)Gi]TP[(A1+B1K)Gi+(C1ωi+D1Kωi)Gi]−GiTPGi}=E∞∑i=k{GiT[γ(A1+B1K)TP(A1+B1K)+γ(C1+D1K)TP(C1+D1K)−P]Gi}. |
Based on (2.12) and (2.15), we have
J(Gk,K)=E∞∑i=kγi−kGTi[O1+KTR∇K]Gi]=E∞∑i=kγi−kGTi[P−γ(A1+B1K)TP(A1+B1K)−γ(C1+D1K)TP(C1+D1K)]Gi=−E∞∑i=kγi−k[γGTi+1PGi+1−GTiPGi]=E(GTkPGk)−limi→∞γi−k+1E(GTiPGi)=E(GTkPGk). |
Since the feedback control uk is admissible, we can obtain J(Gk,K)=E(GkTPGk), which satisfies the well-posedness of SLQ optimal tracking control problem.
To make sure the mean-square stable control, we make the following assumption.
Assumption 1. The system (2.9) is mean-square stabilizable.
At present, ADP algorithm has achieved great success in the optimal tracking control of deterministic systems [24,25,26], which inspires us to transform stochastic problems into deterministic problems through system transformation.
Let Mk=E(GkGTk), then the system (2.9) can be converted to
Mk+1=E(Gk+1GTk+1)=E((TGk+B0uk)(TGk+B0uk)T)=(A1+B1K)Mk(A1+B1K)T+(C1+D1K)Mk(C1+D1K)T | (2.16) |
where Mk∈R(n+q)×(n+q) is the state of a deterministic system and M0 is the initial state.
Therefore, the cost function (2.10) can be rewritten as
J(Mk,K)=tr{∞∑i=kγi−k[(O1+KTR∇K)Mk]}. | (2.17) |
Remark 1. After system transformation, the stochastic system is transformed into deterministic system. The system (2.17) completely gets rid of stochastic disturbance ωk and will only be dependent on the initial state M0 and control gain matrix K, which makes preparation for the derivation and application of Q-learning algorithm.
In this paper, Q-learning method is used to solve the SLQ optimal tracking problem, which avoids the need for accurate system model. Thus we first give the formula of the optimal control and the corresponding augmented SAE.
Lemma 2. Given the admissible control uk, we can get the following optimal control
u∗k=K∗Gk=−(R∇+γBT1PB1)−1γ(BT1PA1+DT1PD1)Gk | (3.1) |
and the optimal cost function
V(Gk)=E(GTkPGk)=tr(PMk) | (3.2) |
where the matrix P satisfies the following augmented SAE
{P=O1+γ(AT1PA1+CT1PC1)−γ(AT1PB1+CT1PD1)×(R∇+γBT1PB1+γDT1PD1)−1γ(BT1PA1+DT1PC1)R∇+γBT1PB1+DT1PD1>0. | (3.3) |
Proof. Suppose uk is an admissible control. According to Lemma 1 and (2.17), the cost function can be written as
J(Mk,K)=tr{∞∑i=kγi−k[(O1+KTR∇K)Mi]}=tr{(O1+KTR∇K)Mi}+tr{∞∑i=k+1γi−k[(O1+KTR∇K)Mi]}=tr{(O1+KTR∇K)Mi}+J(Mk+1,K). | (3.4) |
According to Bellman optimality principle, the optimal cost function satisfies
V(Mk)=minK{tr{(O1+KTR∇K)Mk}+V(Mk+1)}. | (3.5) |
The optimal control gain matrix can be obtained as follow
K∗(Mk)=argminK{tr{(O1+KTR∇K)Mk}+V(Mk+1)}. | (3.6) |
Considering the first-order necessary condition
∂[tr{(O1+KTR∇K)Mk}+V(Mk+1)]∂K=0, | (3.7) |
we can obtain
(R∇+γBT1PB1+γDT1PDT)KGk+γ(BT1PA1+DT1PC1)Gk=0 | (3.8) |
where the matrix P satisfies augmented SAE (2.15).
Supposing R∇+γBT1PB1+γDT1PDT>0, we have
K∗=−(R∇+γBT1PB1)−1γ(BT1PA1+DT1PD1). | (3.9) |
When taking (3.9) into the (2.15), we can obtain
P=O1+γ(AT1PA1+CT1PC1)−γ(AT1PB1+CT1PD1)×(R∇+γBT1PB1+γDT1PDT)−1γ(BT1PA1+DT1PC1). | (3.10) |
From Lemma 2, the SQL optimal tracking problem can be dealt with by the solution of augmented SAE (3.3). However, solving augmented SAE (3.3) requires accurate system model, so this method is not feasible when the dynamics are unknown.
To solve model-free SQL optimal tracking problem with delays, we give the definition of the Q function and the corresponding matrix H.
Based on (2.10) and Bellman optimality principle, we know that the optimal cost function satisfies Hamilton Jacobi Bellman (HJB) equation
V(Gk)=minuk{E[GTkO1Gk+uTkR∇uk]+γV(Gk+1)}. | (3.11) |
The Q-function is defined as
Q(Gk,uk)=E[GkTO1Gk+uTkR∇uk]+γV(Gk+1). | (3.12) |
According to Lemma 1, V(Gk+1) can be written as
V(Gk+1)=E(GTk+1PGk+1)=E{(TGk+B0uk)TP(TGk+B0uk)}=E{[(A1Gk+C1ωkGk)+(B1uk+D1ωkuk)]TP[(A1Gk+C1ωkGk)+(B1uk+D1ωkuk)]}. | (3.13) |
Substitute (3.13) into (3.12), we can get
Q(Gk,uk)=E{[Gkuk]T[HGGHGuHuGHuu][Gkuk]}=E{[Gkuk]TH[Gkuk]} | (3.14) |
where H=HT∈R(n+q+m)×(n+q+m),
H=[HGGHGuHuGHuu]=[O1+γAT1PA1+γCT1PC1γAT1PB1+γCT1PD1γBT1PA1+γDT1PC1γBT1PB1+γDT1PD1+R∇]. | (3.15) |
Let ∂Q(Gk,uk)∂uk=0, then the optimal control can be obtained as follow
u∗k=−H−1uuHuGGk. | (3.16) |
From Lemma 1 and (3.15), we can know the relationship between matrix P and matrix H.
P=[IKT]H[IKT]T. | (3.17) |
As can be seen from (3.16), the optimal control only depends on the matrix H, which is completely get rid of the constraints of the system parameters. Next, we will present the Q-learning iterative algorithm for estimating the matrix H.
In this section, we propose Q-learning iterative algorithm based on the Ⅵ. This method starts with the initial value Q0(Gk,uk)=0 and the initial admissible control u0(Gk), Q1(Gk,uk) will be updated by the initial value and the initial control as follows
Q1(Gk,uk)=E[GkTO1Gk+uT0(Gk)R∇u0(Gk)]+γQ0(Gk+1,u0(Gk+1)). | (3.18) |
The control is updated as follows
u1(Gk)=argminu(Gk)Q1(Gk,uk) | (3.19) |
for i≥1, Q-learning algorithm iterates between
Qi+1(Gk,uk)=E[GkTO1Gk+uTi(Gk)R∇ui(Gk)]+γQi(Gk+1,ui(Gk+1)) | (3.20) |
and
ui+1(Gk)=argminuk{E[GTkO1Gk+uTkR∇uk]+minuk+1Qi(Gk+1,uk+1)} | (3.21) |
where i is the iteration index and k is time index.
According to (3.14), the Q function can be rewritten as
Qi+1(Gk,uk)=[GkTuTi(Gk)]Hi+1[GkTuTi(Gk)]T=E{[GkTuTi(Gk)][O100R∇][GkTuTi(Gk)]T+γ[Gk+1TuTi(Gk+1)]Hi[Gk+1TuTi(Gk+1)]T} | (3.22) |
and we can obtain the optimal controller
ui(Gk)=−H−1uu,iHuG,iGk. | (3.23) |
According to (3.17), we can get
Pi=[IKTi]Hi[IKTi]T. | (3.24) |
Before proving the convergence of Q-learning algorithm, we first give the following two lemmas.
Lemma 3. Q-learning algorithm (3.22) and (3.23) is equivalent to
Pi+1=O1+γ(AT1PiA1+CT1PiC1)−γ(AT1PiB1+CT1PiD1)×(R+γBT1PiB1+γDT1PiD1)−1γ(BT1PiA1+DT1PiC1). | (3.25) |
Proof. According to (2.11), the last term of (3.22) can be written as
E{[Gk+1TuTi(Gk+1)]Hi[Gk+1TuTi(Gk+1)]T}=E{Gk+1T[IKTi]Hi[IKTi]TGk+1}=E{[(A1Gk+C1ωkGk)+(B1ui(Gk)+D1ωkui(Gk))]T[IKTi]Hi[IKTi]T(A1Gk+C1ωkGk)+(B1ui(Gk)+D1ωkui(Gk))]}=E{[GkTuiT(Gk)][A1B1]T[IKiT]Hi[IKiT]T[A1B1][GkTuiT(Gk)]T+[GkTuiT(Gk)][C1D1]T[IKiT]Hi[IKiT]T[C1D1][GkTuiT(Gk)]T}. | (3.26) |
Substitute (3.26) into (3.22), according to (3.24), we can get
Hi+1=[O100R∇]+[γAT1PiA1γAT1PiB1γBT1PiA1γBT1PiB1]+[γCT1PiC1γCT1PiD1γDT1PiC1γDT1PiD1]. | (3.27) |
Based on (3.24), we have
Pi+1=[IKTi+1]Hi+1[IKTi+1]T. | (3.28) |
Substitute (3.27) into (3.28), we can get
Pi+1=O1+γ(AT1PiA1+CT1PiC1)−γ(AT1PiB1+CT1PiD1)×(R+γBT1PiB1+γDT1PiD1)−1γ(BT1PiA1+DT1PiC1) | (3.29) |
where R∇+γBT1PB1+DT1PD1>0.
Lemma 4 ([27]). The value iteration algorithm iterates between
Vi+1(Gk)=E(GTk(O1+KiTR∇Ki)Gk)+γVi(Gk+1) | (3.30) |
and
Ki+1=argminK{E(GTk(O1+KiTR∇Ki)Gk)+γVi(Gk+1)} | (3.31) |
is the convergence, then
limi→∞Vi(Gk)=V(Gk)=E(GkTPGk)=tr{PMk}, |
limi→∞Ki=K∗=−(R∇+γBT1PB1+γDT1PD1)−1γ(BT1PA1+DT1PC1) |
where the matrix P satisfies the augmented SAE (3.3).
Theorem 3.1. Assuming that system (2.9) is mean-square stabilizable, the matrix sequence {Hi} calculated by Q-learning algorithm (3.22) converges to matrix H and the matrix sequence {Pi} calculated by (3.24) converges to the solution P of augmented SAE (3.3).
Proof. According to Lemma 4, (3.30) can be rewritten as
Vi+1(Gk)=E(GkTPi+1Gk)=E[GkT(O1+KiR∇Ki)Gk]+E(GTk+1PiGk+1)=E{GkT(O1+KiR∇Ki)Gk+[(A1+B1K)Gi+(C1ωi+D1Kωi)Gi]TP[(A1+B1K)Gi+(C1ωi+D1Kωi)Gi]}=E(GiT[(A1+B1K)TP(A1+B1K)+(C1+D1K)TP(C1+D1K)+O1+KiTR∇Ki]Gi). | (3.32) |
We can update the control gain matrix by (3.31) as follows
Ki=−(R∇+γBT1PiB1+γDT1PiD1)−1γ(BT1PiA1+DT1PiC1). | (3.33) |
Substituting (3.33) into (3.32), we can get
Pi+1=O1+γ(AT1PiA1+CT1PiC1)−γ(AT1PiB1+CT1PiD1)×(R+γBT1PiB1+γDT1PiD1)−1γ(BT1PiA1+DT1PiC1). | (3.34) |
According to Lemmas 3 and 4, we can conclude limi→∞Pi=P. when i→∞, the matrix P satisfies
P=O1+γ(AT1PA1+CT1PC1)−γ(AT1PB1+CT1PD1)×(R+γBT1PB1+γDT1PD1)−1γ(BT1PA1+DT1PC1). | (3.35) |
Based on (3.27), we can know H satisfies limi→∞Hi=H, where
H=[γAT1PA1+γCT1PC1+Q1γAT1PB1+γCT1PD1BT1PA1+γDT1PC1γBT1PB1+γDT1PD1+R∇]. | (3.36) |
So the Q-learning algorithm converges.
Due to the existence of stochastic disturbance, the output trajectory of the system is uncertain, and the cost function has expectations, the online algorithm cannot achieve the function. Therefore, it is necessary to transform the stochastic Q-learning algorithm into a deterministic Q-learning algorithm. In this section, we will give the implementation steps of deterministic Q-learning algorithm. The flow chart of Q learning algorithm is shown in Figure 1.
According to Eq (2.11), the left side of (3.22) can be simplified to
E{[GkTuTi(Gk)]Hi+1[GkTuTi(Gk)]}=E{GkT[IKTi]Hi+1[IKTi]TGk}=tr{[IKTi]Hi+1[IKTi]TMk}. | (4.1) |
The right side of (3.22) can be simplified as
E{GkT[IKTi][O100R∇][IKTi]TGk+Gk+1T[IKTi]Hi[IKTi]TGk+1}=tr{[IKTi][O100R∇][IKTi]TMk+[IKTi]Hi[IKTi]TMk+1}. | (4.2) |
For simplicity, let
Li(Hi)=[IKTi]Hi[IKTi]T,i=1,2,3,⋯. | (4.3) |
Then (3.22) can be simplified as
tr{Li(Hi+1)Mk}=tr{Li([O100R∇])Mk+Li(Hi)Mk+1}. | (4.4) |
The Q-learning iterative algorithm consisting of (4.4) and (3.23) only relies on determining the state Mk of the system (2.16) and iteratively controlling the gain matrix Ki, avoiding the constraints of system parameters and stochastic disturbance.
Remark 2. The Q-learning algorithm based on Ⅵ is performed online and solves (4.4) using least squares (LS) without knowing augmented system. In fact, (4.4) is a scalar equation and H is a symmetric (n+q+m)×(n+q+m) matrix with (n+q+m)×(n+q+m+1)/2 independent elements. Therefore, at least (n+q+m+1)×(n+q+m+1)/2 data tuples are required before (4.4) can be solved using LS.
Remark 3. Q-learning algorithm based on Ⅵ requires a persistent excitation (PE) condition [28] to ensure the sufficient exploration of the state space.
In this section, a simulation example is given to illustrate the effectiveness of Q-learning algorithm. Consider the following stochastic linear system with delays
xk+1=Axk+Adxk−d+Buk+Bduk−d+(Cxk+Cdxk−d+Duk+Dduk−d)ωk,yk=Exk+Edxk−d |
in which A=(0.2−0.80.5−0.7), Ad=(0.2−0.20.10.15), B=(0.03−0.5), Bd=(0.3−0.2), C=(−0.040.4−0.30.13), Cd=(0.2−0.10.20.11), D=(0.05−0.3), Dd=(0.10.1), E=(33), Ed=(0.10.12).
Suppose the reference trajectory is as follows
rk+1=−rk |
where r0=1.
The cost function is considered as (2.5) with R=1, Rd=1, O=10 and delay index d=1. The initial state for augmented system (2.9) is chosen as G0=[10−101]T. The initial control gain matrix is selected as K=[000]. In each iteration of the algorithm, 21 samples are collected to update the control gain matrix K.
In order to verify the effectiveness of the iterative Q-learning algorithm, we compared K with optimal solution K∗ solved by SAE (3.1). Figure 2 shows the control gain matrix K converges to the optimal control gain matrix K∗ as the number of iterations increases. Figure 3 shows the convergence process of H to its optimal values H∗, which can be calculated by (3.15). The goal of the optimal tracking problem is to trace the reference signal trajectory. In Figure 4, the expectation of system output E(y) can track the reference trajectory rk. This further proves the effectiveness of the proposed Q-learning algorithm.
For the model-free SLQ optimal tracking problem with delays, Q-learning algorithm based on Ⅵ is proposed in this paper. This method makes full use of the system information to approximate the optimal control online, and never needs the system parameter information. In the iterative process of the algorithm, the H matrix sequence and the control gain matrix K sequence are guaranteed to approximate the optimal value. Finally, the simulation results show that the system output can track the reference trajectory effectively.
The authors declare that they have no conflicts of interest.
[1] | A. Lotka, Elements of Physical Biology, USA: Williams Wilkins Co., Balitmore, 1925. |
[2] | V. Volterra, Variazioni e fluttuazioni del numero dindividui in specie animali conviventi, Mem. Acad Lincei Roma, 2 (1926), 31-113. |
[3] |
G. F. Gause, N. P. Smaragdova, A. A. Witt, Further studies of interaction between predators and prey, J. Anim. Ecol., 5 (1936), 1-18. doi: 10.2307/1087
![]() |
[4] | G. F. Gause, The Struggle for Existence, USA: Williams Wilkins Co., Balitmore, 1934. |
[5] |
S. Magalhães, P. C. J. V. Rijn, M. Montserrat, A. Pallini, M. W. Sabelis, Population dynamics of thrips prey and their mite predators in a refuge, Oecologia, 150 (2007), 557-568. doi: 10.1007/s00442-006-0548-3
![]() |
[6] |
J. Ghosh, B. Sahoo, S. Poria, Prey-predator dynamics with prey refuge providing additional food to predator, Chaos Soliton. Fract., 96 (2017), 110-119. doi: 10.1016/j.chaos.2017.01.010
![]() |
[7] |
B. Sahoo, S. Poria, Effects of additional food in a delayed predator-prey model, Math. Biosci., 261 (2015), 62-73. doi: 10.1016/j.mbs.2014.12.002
![]() |
[8] | B. Sahoo, S. Poria, Dynamics of predator-prey system with fading memory, Appl. Math. Comput., 347 (2019), 319-333. |
[9] |
U. Ufuktepe, B. Kulahcioglu, O. Akman, Stability analysis of a prey refuge predator-prey model with Allee effects, J. Biosciences, 44 (2019), 85. doi: 10.1007/s12038-019-9911-5
![]() |
[10] |
Y. Xie, J. Lu, Z. Wang, Stability analysis of a fractional-order diffused prey-predator model with prey refuges, Physica A., 526 (2019), 120773. doi: 10.1016/j.physa.2019.04.009
![]() |
[11] | C. S. Holling, The functional response of predators to prey density and its role in mimicry and population regulation, Mem. Entomol. Soc. Canada, 45 (1965), 1-60. |
[12] | Q. Y. Bie, Q. R. Wang, Z. A. Yao, Cross-diffusion induced instability and pattern formation for a Holling type-Ⅱ predator-prey model, Appl. Math. Comput., 247 (2014), 1-12. |
[13] |
L. Chen, F. Chen, L. Chen, Qualitative analysis of a predator-prey model with Holling type Ⅱ functional response incorporating a constant prey refuge, Nonlinear. Anal-Real., 11 (2010), 246-252. doi: 10.1016/j.nonrwa.2008.10.056
![]() |
[14] | Z. J. Du, X. Chen, Z. S. Feng, Multiple positive periodic solutions to a predator-prey model with Leslie-Gower Holling-type Ⅱ functional response and harvesting terms, Discrete. Contin. Dyn. Syst., 7 (2014), 1203-1214. |
[15] |
J. J. Jiao, L. S. Chen, S. H. Cai, A delayed stage-structured Holling Ⅱ predator-prey model with mutual interference and impulsive perturbations on predator, Chaos Soliton. Fract., 40 (2009), 1946-1955. doi: 10.1016/j.chaos.2007.09.074
![]() |
[16] |
W. Ko, K. Ryu, Qualitative analysis of a predator-prey model with Holling type Ⅱ functional response incorporating a prey refuge, J. Differ. Equations, 231 (2006), 534-550. doi: 10.1016/j.jde.2006.08.001
![]() |
[17] |
V. Krivan, J. Eisner, The effect of the Holling type Ⅱ functional response on apparent competition, Theor. Popul. Biol., 70 (2006), 421-430. doi: 10.1016/j.tpb.2006.07.004
![]() |
[18] |
V. Krivan, On the Gause predator prey model with a refuge: A fresh look at the history, J. Theor. Biol., 274 (2011), 67-73. doi: 10.1016/j.jtbi.2011.01.016
![]() |
[19] |
Q. Liu, D. Q.Jiang, H. Tasawar, A. Ahmed, Dynamics of a stochastic predator-prey model with stage structure for predator and holling type Ⅱ functional response, J. Nonlinear Sci., 28 (2018), 1151-1187. doi: 10.1007/s00332-018-9444-3
![]() |
[20] | S. P. Li, W. N. Zhang, Bifurcations of a discrete prey-predator model with Holling type Ⅱ functional response, Discrete Cont. Dyn-B., 14 (2010), 159-176. |
[21] |
H. Molla, S. R. Md, S. Sahabuddin, Dynamics of a predator-prey model with holling type Ⅱ functional response incorporating a prey refuge depending on both the species, Int. J. Nonlin. Sci. Num., 20 (2019), 1-16. doi: 10.1515/ijnsns-2017-0166
![]() |
[22] |
J. Song, Y. Xia, Y. Bai, Y. Cai, D. O'Regan, A non-autonomous Leslie-Gower model with Holling type Ⅳ functional response and harvesting complexity, Adv. Differ. Equ-Ny., 2019 (2019), 1-12. doi: 10.1186/s13662-018-1939-6
![]() |
[23] | D. Ye, M. Fan, W. P. Zhang, Periodic solutions of density dependent predator-prey systems with Holling Type 2 functional response and infinite delays, J. Appl. Math. Mec., 85 (2005), 213-221. |
[24] |
S. W. Zhang, L. S. Chen, A Holling Ⅱ functional response food chain model with impulsive perturbations, Chaos Soliton. Fract., 24 (2005), 1269-1278. doi: 10.1016/j.chaos.2004.09.051
![]() |
[25] |
J. Zhou, C. L. Mu, Coexistence states of a Holling type-Ⅱ predator-prey system, J. Math. Anal. Appl., 369 (2010), 555-563. doi: 10.1016/j.jmaa.2010.04.001
![]() |
[26] |
S. Jana, M. Chakraborty, K. Chakraborty, T. K. Kar, Global stability and bifurcation of time delayed prey-predator system incorporating prey refuge. Math. Comput. Simulat., 85 (2012), 57-77. doi: 10.1016/j.matcom.2012.10.003
![]() |
[27] | W. G. Aiello, H. I. Freedman, J. Wu, Analysis of a model representing stage-structured population growth with state-dependent timedelay, SIAM J. Appl. Math., 52 (1992), 885-889. |
[28] |
F. Brauer, Z. Ma, Stability of stage-structured population models, J. Math. Anal. Appl., 126 (1987), 301-315. doi: 10.1016/0022-247X(87)90041-2
![]() |
[29] |
H. I. Freedman, J. Wu, Persistence and global asymptotic stability of single species dispersal models with stage-structure, Q. Appl. Math., 49 (1991), 351-371. doi: 10.1090/qam/1106397
![]() |
[30] | W. Wang, L. Chen, A predator-prey system with stage-structure for predator, Comput. Math. Appl., 33 (1997), 83-91. |
[31] |
W. Wang, G. Mulone, F. Salemi, V. Salone, Permanence and stability of a stage-structured predator prey model, J. Math. Anal. Appl., 262 (2001), 499-528. doi: 10.1006/jmaa.2001.7543
![]() |
[32] |
Y. Chen, Multiple periodic solution of delayed predator-prey systems with type Ⅳ functional responses, Nonlinear. Anal-Hybri., 5 (2004), 45-53. doi: 10.1016/S1468-1218(03)00014-2
![]() |
[33] | M. Fan, Q. Wang, X. F. Zou, Dynamics of a nonautonomous ratio-dependent predator-prey system, P. Roy. Soc. Lond. A. Math., 133 (2003), 97-118. |
[34] |
M. Fan, P. J. Y. Wong, R. P. Agarwal, Periodicity and stability in periodic n-species Lotka-Volterra competition system with feedback controls and deviating arguments, Acta. Math. Sin., 19 (2003), 801-822. doi: 10.1007/s10114-003-0311-1
![]() |
[35] | R. Gaines, J. Mawhin, Coincidence Degree and Nonlinear Differential Equations, Lecture Notes in Mathematics, Springer, Berlin, 1977. |
[36] |
H. Zheng, L. Guo, Y. Z. Bai, Y. H. Xia, Periodic solutions of a non-autonomous predator-prey system with migrating prey and disease infection: Via Mawhin's coincidence degree theory, J. Fix. Point Theory A., 21 (2019), 21-37. doi: 10.1007/s11784-019-0660-8
![]() |
[37] | Y. H. Xia, Y. Shen, An nonautonomous predator-prey model with refuge effect, J. Xuzhou Inst. Tech., 34 (2019), 1-7. |
[38] | F. Chen, On a periodic multi-species ecological model, Appl. Math. Comput., 171 (2005), 492-510. |
[39] | F. Chen, Positive periodic solutions of neutral Lotka-Volterra system with feedback control, Appl. Math. Comput., 162 (2005), 1279-1302. |
[40] | F. Chen, F. Lin, X. Chen, Sufficient conditions for the existence positive periodic solutions of a class of neutral delay models with feedback control, Appl. Math. Comput., 158 (2004), 45-68. |
[41] | L. Chen. Mathematical Models and Methods in Ecology, Science Press, Beijing Chinese, 1998. |
[42] |
X. Chen, Z. J. Du, Existence of positive periodic solutions for a neutral delay predator-prey model with Hassell-Varley type functional response and impulse, Qual. Theor. Dyn. Syst., 17 (2018), 67-80. doi: 10.1007/s12346-017-0223-6
![]() |
[43] |
Z. J. Du, Z. S. Feng, Periodic solutions of a neutral impulsive predator-prey model with Beddington-DeAngelis functional response with delays, J. Comput. Appl. Math., 258 (2014), 87-98. doi: 10.1016/j.cam.2013.09.008
![]() |
[44] | S. Gao, L. Chen, Z. Teng, Hopf bifurcation and global stability for a delayed predator-prey system with stage structure for predator, Appl. Math. Comput., 202 (2008), 721-729. |
[45] |
S. Kant, V. Kumar, Stability analysis of predator-prey system with migrating prey and disease infection in both species. Appl. Math. Model., 42 (2017), 509-539. doi: 10.1016/j.apm.2016.10.003
![]() |
[46] | Y. Kuang, Delay Differential Equations: With Applications in Population Dynamics, Academic Press, San Diego, 1993. |
[47] |
S. Liu, L. Chen, Z. Liu, Extinction and permanence in nonautonomous competitive system with stage structure, J. Math. Anal. Appl., 274 (2002), 667-684. doi: 10.1016/S0022-247X(02)00329-3
![]() |
[48] | S. Lu, W. Ge, Existence of positive periodic solutions for neutral population model with multiple delays, Appl. Math. Comput., 153 (2004), 885-902. |
[49] |
X. Z. Meng, S. N. Zhao, T. Feng, T. H. Zhang, Dynamics of a novel nonlinear stochastic SIS epidemic model with double epidemic hypothesis, J. Math. Anal. Appl., 433 (2016), 227-242. doi: 10.1016/j.jmaa.2015.07.056
![]() |
[50] | J. Song, M. Hu, Y. Z. Bai, Y. Xia, Dynamic analysis of a non-autonomous ratio-dependent predator-prey model with additional food. J. Comput. Anal. Appl., 8 (2018), 1893-1909. |
[51] |
Y. L. Song, H. P. Jiang, Q. X. Liu, Y. Yuan, Spatiotemporal dynamics of the diffusive Mussel-Algae model near Turing-Hopf bifurcation, SIAM J. Appl. Dyn. Syst., 16 (2017), 2030-2062. doi: 10.1137/16M1097560
![]() |
[52] |
Y. L. Song, X. S. Tang, Stability, steady-state bifurcations and turing patterns in a predator-prey model with herd behavior and prey-taxis, Stud. Appl. Math., 139 (2017), 371-404. doi: 10.1111/sapm.12165
![]() |
[53] |
Y. L. Song, S. H. Wu, H. Wang, Spatiotemporal dynamics in the single population modelwith memory-based diffusion and nonlocal effect, J. Differ. Equations. 267 (2019), 6316-6351. doi: 10.1016/j.jde.2019.06.025
![]() |
[54] |
J. J. Wei, M. Y. Li, Hopf bifurcation analysis in a delayed nicholson blowflies equation, Nonlinear Anal-Theor., 60 (2005), 1351-1367. doi: 10.1016/j.na.2003.04.002
![]() |
[55] | Z. Wei, Y. H. Xia, T. Zhang, Stability and bifurcation analysis of a amensalism model with weak Allee effect, Qual. Theor. Dyn. Syst., 2020. |
[56] |
R. Xu, Z. Ma, Stability and Hopf bifurcation in a ratio-dependent predator prey system with stage structure, Chaos Soliton. Fract., 38 (2008), 669-684. doi: 10.1016/j.chaos.2007.01.019
![]() |
[57] | J. Y. Xu, T. H. Zhang, K. Y. Song, A stochastic model of bacterial infection associated with neutrophils, Appl. Math. Comput., 373 (2020), 125025. |
[58] | F. Xu, C. Ross, K. Vlastimil, Evolution of mobility in predator-prey systems, Discrete Cont. DynB., 19 (2014), 3397-3432. |
[59] |
F. Xu, M. Connell, An investigation of the combined effect of an annual mass gathering event and seasonal infectiousness on disease outbreak, Math. Biosci., 312 (2019), 50-58. doi: 10.1016/j.mbs.2019.03.006
![]() |
[60] |
J. Y. Yang, Z. Jin, F. Xu, Threshold dynamics of an age-space structured SIR model on heterogeneous environment, Appl. Math. Lett., 96 (2019), 69-74. doi: 10.1016/j.aml.2019.03.009
![]() |
[61] |
F. Q. Yi, J. J. Wei, J. P. Shi, Bifurcation and spatiotemporal patterns in a homogeneous diffusive predater-prey system, J. Differ. Equations, 246 (2009), 1944-1977. doi: 10.1016/j.jde.2008.10.024
![]() |
[62] |
F. Q. Yi, J. J. Wei, J. P. Shi, Diffusion-driven instability and bifurcation in the Lengyel-Epstein system, Nonlinear Anal-Real., 9 (2008), 1038-1051. doi: 10.1016/j.nonrwa.2007.02.005
![]() |
[63] |
T. H. Zhang, T. Q. Zhang, X. Z. Meng, Stability analysis of a chemostat model with maintenance energy, Appl. Math. Lett., 68 (2017), 1-7. doi: 10.1016/j.aml.2016.12.007
![]() |
[64] |
T. H. Zhang, Z. W. Geem, Review of harmony search with respect to algorithm structure, Swarm Evol. Comput., 48 (2019), 31-43. doi: 10.1016/j.swevo.2019.03.012
![]() |
[65] |
X. G. Zhang, C. H. Shan, Z. Jin, H. P. Zhu, Complex dynamics of epidemic models on adaptive networks, J. Differ. Equations, 266 (2019), 803-832. doi: 10.1016/j.jde.2018.07.054
![]() |
1. | Heng Zhang, Na Li, Data‐driven policy iteration algorithm for continuous‐time stochastic linear‐quadratic optimal control problems, 2024, 26, 1561-8625, 481, 10.1002/asjc.3223 |