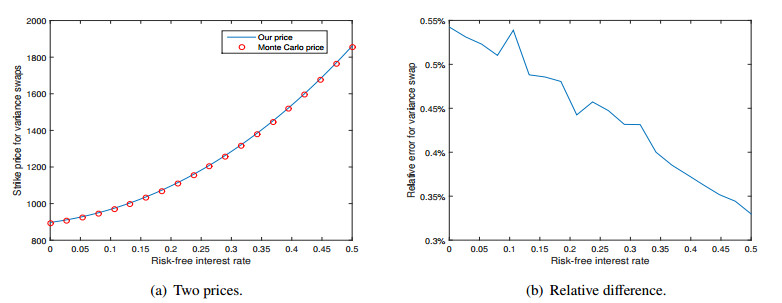
This work aims to present a reliable algorithm that can effectively generate accurate piecewise approximate analytical solutions for third- and fourth-order reaction-diffusion singular perturbation problems. These problems involve a discontinuous source term and exhibit both interior and boundary layers. The original problem was transformed into a system of coupled differential equations that are weakly interconnected. A zero-order asymptotic approximate solution was then provided, with known asymptotic analytical solutions for the boundary and interior layers, while the outer region solution was obtained analytically using an enhanced residual power series approach. This approach combined the standard residual power series method with the Padé approximation to yield a piecewise approximate analytical solution. It satisfies the continuity and smoothness conditions and offers higher accuracy than the standard residual power series method and other numerical methods like finite difference, finite element, hybrid difference scheme, and Schwarz method. The algorithm also provides error estimates, and numerical examples are included to demonstrate the high accuracy, low computational cost, and effectiveness of the method within a new asymptotic semi-analytical numerical framewor.
Citation: Essam R. El-Zahar, Ghaliah F. Al-Boqami, Haifa S. Al-Juaydi. Piecewise approximate analytical solutions of high-order reaction-diffusion singular perturbation problems with boundary and interior layers[J]. AIMS Mathematics, 2024, 9(6): 15671-15698. doi: 10.3934/math.2024756
[1] | Hui Sun, Zhongyang Sun, Ya Huang . Equilibrium investment and risk control for an insurer with non-Markovian regime-switching and no-shorting constraints. AIMS Mathematics, 2020, 5(6): 6996-7013. doi: 10.3934/math.2020449 |
[2] | Ahmed Ghezal, Mohamed balegh, Imane Zemmouri . Markov-switching threshold stochastic volatility models with regime changes. AIMS Mathematics, 2024, 9(2): 3895-3910. doi: 10.3934/math.2024192 |
[3] | Min-Ku Lee, Jeong-Hoon Kim . Closed-form approximate solutions for stop-loss and Russian options with multiscale stochastic volatility. AIMS Mathematics, 2023, 8(10): 25164-25194. doi: 10.3934/math.20231284 |
[4] | Min-Ku Lee, Jeong-Hoon Kim . Pricing vanilla, barrier, and lookback options under two-scale stochastic volatility driven by two approximate fractional Brownian motions. AIMS Mathematics, 2024, 9(9): 25545-25576. doi: 10.3934/math.20241248 |
[5] | Sun-Yong Choi, Donghyun Kim, Ji-Hun Yoon . An analytic pricing formula for timer options under constant elasticity of variance with stochastic volatility. AIMS Mathematics, 2024, 9(1): 2454-2472. doi: 10.3934/math.2024121 |
[6] | Mohammed Alhagyan, Mansour F. Yassen . Incorporating stochastic volatility and long memory into geometric Brownian motion model to forecast performance of Standard and Poor's 500 index. AIMS Mathematics, 2023, 8(8): 18581-18595. doi: 10.3934/math.2023945 |
[7] | Yuanfu Shao . Dynamics and optimal harvesting of a stochastic predator-prey system with regime switching, S-type distributed time delays and Lévy jumps. AIMS Mathematics, 2022, 7(3): 4068-4093. doi: 10.3934/math.2022225 |
[8] | Teh Raihana Nazirah Roslan, Sharmila Karim, Siti Zulaiha Ibrahim, Ali Fareed Jameel, Zainor Ridzuan Yahya . Stochastic pricing formulation for hybrid equity warrants. AIMS Mathematics, 2022, 7(1): 398-424. doi: 10.3934/math.2022027 |
[9] | Yue Li, Yunyan Wang . Strong consistency of the nonparametric kernel estimator of the transition density for the second-order diffusion process. AIMS Mathematics, 2024, 9(7): 19015-19030. doi: 10.3934/math.2024925 |
[10] | Shoude Huang, Xin-Jiang He . An analytical approximation formula for European option prices under a liquidity-adjusted non-affine stochastic volatility model. AIMS Mathematics, 2022, 7(6): 10364-10377. doi: 10.3934/math.2022577 |
This work aims to present a reliable algorithm that can effectively generate accurate piecewise approximate analytical solutions for third- and fourth-order reaction-diffusion singular perturbation problems. These problems involve a discontinuous source term and exhibit both interior and boundary layers. The original problem was transformed into a system of coupled differential equations that are weakly interconnected. A zero-order asymptotic approximate solution was then provided, with known asymptotic analytical solutions for the boundary and interior layers, while the outer region solution was obtained analytically using an enhanced residual power series approach. This approach combined the standard residual power series method with the Padé approximation to yield a piecewise approximate analytical solution. It satisfies the continuity and smoothness conditions and offers higher accuracy than the standard residual power series method and other numerical methods like finite difference, finite element, hybrid difference scheme, and Schwarz method. The algorithm also provides error estimates, and numerical examples are included to demonstrate the high accuracy, low computational cost, and effectiveness of the method within a new asymptotic semi-analytical numerical framewor.
Trading derivatives is of great popularity in today's financial markets, and thus, how to manage the risk caused by trading attracts much attention from researchers and market practitioners. Volatility is often used to measure asset price risk, and it is common knowledge that volatility values change randomly over time [1,2], which makes it even more difficult to figure out the nature of the volatility variations. As a result, volatility derivatives are developed to manage the relative risk associated with the asset.
In practice, variance and volatility swaps enjoy high trading volumes, which has resulted in the desire to evaluate the two contracts correctly. To be more specific, while the results in [3] are more general and model-independent, most other research results depend on different adopted models. For example, Grunbichler and Longstaff [4] valued the two contracts with the CIR stochastic volatility process, which is the same process used in the Heston model [5] to describe volatility evolution, while how to price the two contracts under stochastic volatility without giving a specific stochastic volatility dynamic is illustrated by Javaheri et al. [6]. However, these results rely on the realized variance as well as volatility used in final pay-off functions being continuous, which is certainly inappropriate since the realized variance and volatility in real markets are discretely sampled, and such a mis-specification could result in a pricing bias, making these results less valuable. Therefore, pricing discretely-sampled swaps is more meaningful since that is the definition used in reality.
The literature has already witnessed the explosive expansion of evaluating discrete swap contracts. For example, analytical solutions for these two contracts under the Heston model have already been presented in [7,8], respectively. Of course, the Heston model is unable to capture the significantly nonlinear mean revision property exhibited by asset volatility [9], which prompts research into developing more sophisticated models. In particular, He and Chen [10] introduced the concept of the stochastic mean-reversion level in the Heston (CIR) volatility process so that the nonlinear mean revision property can be partially addressed. Recently, the economic status has been shown to be changing [11], and such effects on derivative prices have been shown to be significant [12]. This has led to the emergence of regime switching models [13,14,15,16].
Motivated by the fact that the implied volatility as well as the variance swap curve cannot be appropriately fitted using the classical Heston model [17] and the significance of combining stochastic volatility models with regime switching [12], the He–Chen model is further modified by adding regime switching [10], so that the long-run mean is divided into two parts. One part is characterized by a stochastic process following a normal distribution, and another is a regime switching part. Based on the successful presentation of the forward characteristic function, analytical solutions used to price the two swap contracts are shown under the newly established model.
We organize the remainder of the article as follows: Section 2 briefly introduces the adopted model, and then the forward characteristic function is derived in closed form, leading to analytical solutions of the target contracts. In Section 3, we discuss the numerical results after implementing the solutions we obtain. The last section concludes.
The regime switching dynamics are embraced into the He–Chen model [10] so that the effects of economic cycles are taken into consideration. After that, we derive the two swap pricing formulae under this new model analytically.
As our model modifies the Heston model and the He–Chen model, we first introduce the Heston model. With the assumption that S is the stock price and v denotes asset volatility, the Heston model in a risk-neutral world is characterized through
dSS=rdt+√vdW1t,dv=k(ˉv−v)dt+σ1√vdW2t. | (2.1) |
Here, W1t and W2t are two Wiener processes, with dW1tdW2t=ρdt. The He–Chen model modifies it by proposing the concept of stochastic long-run mean, which formulates
dSS=rdt+√vdW1t,dv=k(ˉv+θ−v)dt+σ1√vdW2t,dθ=λdt+σ2dBt, | (2.2) |
where θ is the newly introduced stochastic source in the long-term mean. Bt is another Wiener process that is unrelated to the other two. Our model further revises the He–Chen model through the consideration of economic cycles on volatility
dSS=rdt+√vdW1t,dv=k(ˉvXt+θ−v)dt+σ1√vdW2t,dθ=λdt+σ2dBt. | (2.3) |
The two-state Markov chain Xt can take the value within the set {(1,0)T,(0,1)T} depending on the different states that the economy belongs to, so that ˉvXt=<ˉv,Xt>, with ˉv=(v1,v2)T. λij,i,j=1,2,i≠j denotes the transition rate of the Markov chain transferring from State i to j. Clearly, the presented model is actually a combination of the Heston stochastic volatility model with regime switching dynamics and a stochastic mean-reversion level. It will become the He–Chen model [10] if we remove regime switching mechanics by setting λ12=λ21=0. If we further make λ=σ2=0, it will go back to the Heston model.
To price the two swap contracts, one should be very clear about the cash flow between the long and short positions. If one longs a variance and volatility swap, he or she needs to pay a strike price specified in the contract at maturity while receiving a floating leg of realized variance and volatility written in an annualized form, whose computation depends on the actual asset price fluctuation during the lifetime of the swaps.
In fact, one can compute the values of the two contracts with a notional amount L at maturity through
Vvar=(RVvar−Kvar)L,Vvol=(RVvol−Kvol)L, |
where RVvar and Kvar are the annualized realized variance and variance swap strike price, respectively, with RVvol as well as Kvol representing the same meaning for a volatility swap. As swap contracts are worth zero values when they are initiated, we have the following to be fair to both long and short positions:
Kvar=E(RVvar),Kvol=E(RVvol). |
Clearly, the determination of Kvar (Kvol) requires that we compute the two expectations, implying that it is necessary for us to know how RVvar (RVvol) is defined before we proceed to figure out the expectation. Here, we adopt the expression widely used in the literature [13,18]
RVvar=1002TN∑i=1(Sti−Sti−1Sti−1)2,RVvol=100√π2NTN∑i=1|Sti−Sti−1Sti−1|. |
ti is ith observation time equally spaced within [0, T] with t0=0 and tN=T. Therefore, once we are able to calculate the expectation E[(Sti−Sti−1Sti−1)2] and E[|Sti−Sti−1Sti−1|] for every i=0,..,N, then we could obtain the delivery price straightforwardly by adding these expectations together.
To analytically work out these expectations, we shall first derive the forward characteristic function of the underlying price. In particular, if we write yT=ln(STSt), the forward characteristic function is formulated using
μ(ϕ;v0,θ0,X0,t,T)=E(ejϕyT|y0,v0,θ0,X0). | (2.4) |
The direct evaluation of this particular function is very difficult, and such difficulty arises from the stochastic nature of both the Winier processes and the Markov chain. To simplify the problem for the time being, we assume that the values of Xt,t∈[0,T] are given at the current time so that the target characteristic function becomes a conditional one
μ(ϕ;v0,θ0,t,T|Xt,t∈[0,T])=E(ejϕyT|y0,v0,θ0,XT). | (2.5) |
The solution to this particular function is presented below.
Proposition 2.1. If Eq (2.3) provides information on the model dynamics, the solution of μ(ϕ;v0,θ0,t,T|Xt,t∈[0,T]) satisfies
μ(ϕ;v0,θ0,t,T|Xt,t∈[0,T])=e˜C(ϕ;τ,t)+ˉD(ϕ;τ,t)v0+ˉE(ϕ;τ,t)θ0e∫T0<J(s),Xs>ds, | (2.6) |
where
ˉD(ϕ;τ,t)=2kσ2111−[1−2kσ21D(ϕ;τ)]ekt,ˉE(ϕ;τ,t)=E(ϕ;τ)+2kσ21{kt−ln(1−[1−2kσ21D(ϕ;τ)]ekt)+ln[2kσ21D(ϕ;τ)]},˜C(ϕ;τ,t)=∫τ012σ22E2(ϕ;s)+λE(ϕ;s)ds+∫t012σ22ˉE(ϕ;τ,s)+λˉE(ϕ;τ,s)ds+jrϕτ,J(s)=kˉvsD(ϕ;T−s)H(s)+kˉvsˉD(ϕ;τ,s)[1−H(s)],D(ϕ;τ)=d−(ρσ1jϕ−k)σ21(1−edτ1−gedτ),E(ϕ;τ)=kσ21{[d−(ρσ1jϕ−k)]τ−2ln(1−gedτ1−g)},d=√(ρσ1jϕ−k)2+σ21(jϕ+ϕ2),g=(ρσ1jϕ−k)−d(ρσ1jϕ−k)+d,H(s)={1,s>t,0,s≤t, |
with τ=T−t.
We prove the proposition in Appendix A.
Of course, the characteristic function presented in (2.6) is a conditional one, and one still has to work out its expectation since the forward characteristic function satisfies
μ(ϕ;v0,θ0,X0,t,T)=E(ejϕyT|y0,v0,θ0,X0),=E[E(ejϕyT|y0,v0,θ0,XT)|y0,v0,θ0,X0],=E[μ(ϕ;v0,θ0,t,T|Xt,t∈[0,T])|y0,v0,θ0,X0]. | (2.7) |
By noticing the fact that ˉv depends on the Markov chain Xt with t∈[0,T], substituting Eq (2.6) into (2.7) could yield
μ(ϕ;v0,θ0,X0,t,T)=e˜C(ϕ;τ,t)+ˉD(ϕ;τ,t)v0+ˉE(ϕ;τ,t)θ0E[e∫T0<J(s),Xs>ds|X0], | (2.8) |
which implies that E[e∫T0<J(s),Xs>ds|X0] has to be derived. Actually, this expectation can be figured out by simply following Elliott and Lian [13]. In this case, we could obtain
E[e∫T0<J(s),Xs>ds|X0]=<eMX0,I>, | (2.9) |
where X0∈{(1,0)′,(0,1)′}, and I=(1,1)′. With A being the matrix formulated by the transition rates associated with Xt, Matrix M is defined as
M=∫T0A′+diag[J(s)]ds, | (2.10) |
yielding
M=(ˉv1ˉE(ϕ;τ,t)−λ12Tλ21Tλ12Tˉv2ˉE(ϕ;τ,t)−λ21T). |
Therefore, we have finally obtained
μ(ϕ;v0,θ0,X0,t,T)=e˜C(ϕ;τ,t)+ˉD(ϕ;τ,t)v0+ˉE(ϕ;τ,t)θ0<eMX0,I>. | (2.11) |
With Eq (2.11), we are now able to present the formulae for pricing both swaps in the following proposition.
Proposition 2.2. If Eq (2.3) provides information on the model dynamics, the prices of variance and volatility swaps can be formulated as
Kvar=1002TN∑i=1[μ(−2j;ti−1,ti,v0,θ0,X0)−2μ(−j;ti−1,ti,v0,θ0,X0)+1],Kvol=100√2πNT∫+∞0N∑i=1RE[μ(ϕ−j;v0,θ0,X0,ti−1,ti)−μ(ϕ;v0,θ0,X0,ti−1,ti)jϕ]dϕ. | (2.12) |
We prove the proposition in Appendix B.
By now, we have successfully worked out the two swap prices analytically. Once a formula for derivative prices has been derived under a new model, one may be very interested in the influence that adopting the new model would bring about, which makes it rather important to make a comparison between our model and the existing related models to find the different numerical behavior. The related discussions are provided below.
This section will mainly analyze how the results provided by the He–Chen model [10] can be affected by adding regime switching. In particular, we will first check whether our model can represent the He–Chen model if transition rates are zero. Then, we will compare our results with those under the He–Chen model without regime switching to identify the difference caused by introducing regime switching mechanics. The parameter values for the analysis are defaulted as follows, whose magnitude is consistent with a number of different pieces of literature [13,19,20]. Both parameters in the process describing the stochastic part of the long-term mean, i.e., λ and σ2, are set to be 0.01, and the initial value of this particular stochastic process, θ0, is 0.03. The expiration date T is set to be 1 year. We also assume that ˉv in the He–Chen model takes the value of ˉv1 for comparison. Other model parameters include r=0.05,k=10,σ1=0.1,v0=0.03 and ρ=−0.5.
Before we numerically study the swap pricing formulae, their accuracy should first be checked using the semi-Monte Carlo benchmark, which is much more efficient than the standard Monte Carlo approach. The detailed procedure in a single simulation will be illustrated in the following: First, by simulating the jump moments using the exponential distribution and the transition rates, a Markov chain within the period [0,T] is generated so that λXt and ηXt become time-dependent parameters. Then, we are able to evaluate the two swaps through the conditional characteristic function (2.6). Finally, by repeating 500,000 times the above steps, we could obtain one variance and one volatility swap price. In the following, we will only present the verification results of the variance swap pricing formula, which is shown in Figure 1, since the two formulae are quite similar to each other and there is no need to check the volatility swap pricing formula once variance swap pricing formula is proved. Specifically, what could be detected first in Figure 1(a) is that our variance swap prices are point-wise close to those from the Monte Carlo approach, and the relative difference displayed in Figure 1(b) is below 0.6%, demonstrating that our formulae are clearly accurate.
The He–Chen model [10] being a special case of ours, can be easily observed from the model dynamics if the regime switching mechanics are removed. In this case, whether our formulae can degenerate to those under the He–Chen model is of interest, and this is also a sign of the correctness of the formulae. A scaling parameter z∈[0,1] is employed with λ12=10∗z,λ21=20∗z, and the corresponding swap prices are shown in Figure 2. Specifically, Figure 2 shows the variance and volatility swap price with and without regime switching, and as expected, the two prices are exactly the same when the two transition rates become zero. It can also be easily observed that with the selected set of parameters, our price is no larger than that without regime switching, and it decreases with the scaling parameter z.
What can be seen in Figure 3 are different swap prices when the constant long-term mean for State 1 is greater than State 2. As shown in Figure 3(a), variance swap prices keep linearly increasing as the time to expiry increases. Moreover, the introduction of regime switching under the current settings will lower swap prices. A very similar decreasing pattern is also shown in Figure 3(b) when we consider volatility swap prices, except that the curve is much more flattening, which is mainly due to the much lower magnitude of volatility swap prices.
Naturally, one may draw the conclusion that the variance and volatility swap prices with regime switching will always be lower than those without regime switching. In order to prove the incorrectness of this viewpoint, we reverse the values of ˉv1 and ˉv2, and the results in this situation are presented in Figure 4. Clearly, if the constant mean-reversion level for current state is lower compared with the other one, our variance and volatility swap price are larger than those obtained under the He–Chen model without regime switching, which is shown in Figure 4. Also, the variance and volatility swap prices in both models decrease when we lower the constant long-term mean for the current state.
One may also be interested in the difference between our model and the well-known Heston model. We show the corresponding comparison results in Figure 5. One can clearly see that variance (volatility) swap prices under our model exceed those under the Heston model when λ is positive, while our prices would be lower than the Heston prices with a negative λ. This can be understood from the fact that λ controls the trend of long-run volatility, with positive (or negative) λ indicating increasing (or decreasing) long-run volatility, leading to higher (or smaller) risks. This also reflects the greater flexibility of our model compared to the Heston model.
This article proposes a particular regime switching stochastic volatility model, dividing the long-run mean into two parts, with one being characterized by a normal distribution and the other incorporating regime switching mechanics. After successfully working out the analytical pricing formulae, we have also shown through the numerical experiments that adding regime switching in the modeling framework has a large impact, which thus implies that this model can be used as an alternative to the existing models in practice.
Xin-Jiang He: investigation, methodology, software, writing-original draft; Sha Lin: conceptualization, software, validation, writing-review & editing. All authors have read and approved the final version of the manuscript for publication.
The authors declare they have not used Artificial Intelligence (AI) tools in the creation of this article.
This work was supported by the National Natural Science Foundation of China (No. 12101554, No. 12301614), and the Fundamental Research Funds for Zhejiang Provincial Universities (No. GB202103001).
The authors declare that they have no conflicts of interest.
We now prove Proposition 2.1. We can express
μ(ϕ;v0,θ0,t,T|Xt,t∈[0,T])=E(ejϕyT|y0,v0,θ0,XT)=E[E(ejϕyT|yt,vt,θt,XT)|y0,v0,θ0,XT]. | (A-1) |
As a result, we will firstly deal with the inner expectation
h(ϕ;vs,θs,Xs,s,T)=E(ejϕyT|ys,vs,θs,XT),s∈[t,T], |
which should satisfy the following PDE
{∂h∂s+12v∂2h∂y2+12σ21v∂2h∂v2+12σ22∂2h∂θ2+ρσ1v∂2h∂v∂y+(r−12v)∂h∂y+k(ˉv+θ−v)∂h∂v+λ∂h∂θ=0,h(ϕ;vs,θs,Xs,s,T)|s=T=ejϕyT. | (A-2) |
Following a number of different literature [21,22,23], if we write
h(ϕ;vs,θs,Xs,s,T)=eC(ϕ,τ)+D(ϕ,τ)vs+E(ϕ,τ)θs+jϕys, | (A-3) |
with τ defined as T−s, then substituting it into PDE (A-2) could yield the three ODEs (ordinary differential equations)
∂D∂τi=12σ21D2+(ρσϕj−k)D−12(jϕ+ϕ2),∂E∂τi=kD,∂C∂τi=12σ22E2+λE+kˉvD+r(jϕ−1). |
From this, we could obtain the solutions to the first two ODEs presented in Proposition 2.1 with s=t. Then, directly integrating the ODE for C derives
C(ϕ,τ)=∫τ012σ22E2(ϕ;s)+λE(ϕ;s)ds+jrϕτ+∫TtkˉvtD(T−s)ds. | (A-4) |
Since we have ys=0 when s=t, we can write
h(ϕ;vt,θt,Xt,t,T)=eC(ϕ,τ)+D(ϕ,τ)vt+E(ϕ,τ)θt. | (A-5) |
We now reformulate Eq (A-1) so that
μ(ϕ;v0,θ0,t,T|Xt,t∈[0,T])=eC(ϕ,τ)E[eD(ϕ,τ)vt+E(ϕ,τ)θt|y0,v0,θ0,XT]. | (A-6) |
If we define
f(ϕ;v0,θ0,t,T|Xt,t∈[0,T])=E[eD(ϕ,τ)vt+E(ϕ,τ)θt|y0,v0,θ0,XT], |
and considering the fact that f is independent of y, f(ϕ;vs,θs,s,t,T|Xa,a∈[0,T]) is a solution to
{∂f∂s+12σ21v∂2f∂v2+12σ22∂2f∂θ2+k(ˉv+θ−v)∂f∂v+λ∂f∂θ=0,f(ϕ;vs,θs,s,t,T|Xa,a∈[0,T])|s=t=eD(ϕ,τ)vt+E(ϕ,τ)θt. | (A-7) |
Similarly, by guessing that the solution to PDE (A-7) be
f(ϕ;vs,θs,s,t,T|Xa,a∈[0,T])=eˉC(ϕ;τ,t−s)+ˉD(ϕ;τ,t−s)vs+ˉE(ϕ;τ,t−s)θs, | (A-8) |
and substituting it into PDE (A-7), we could obtain
∂ˉD∂τs+12σ21ˉD2−kˉD=0,∂ˉE∂τs+kˉD=0,∂ˉC∂τs+12σ22ˉE2+kˉvˉD+λˉE=0, |
with τs=t−s, and the initial condition
ˉD(ϕ;τ,0)=D(ϕ,τ),ˉE(ϕ;τ,0)=E(ϕ,τ),ˉC(ϕ;τ,0)=0. |
Obviously, the first two ODEs can be directly solved, which could contribute to solving the ODE for ˉC(ϕ;τ,τs), so that
ˉC(ϕ;τ,τs)=∫τs012σ22ˉE2(ϕ;τ,p)+λˉE(ϕ;τ,p)dp+∫τs0kˉvtˉD(ϕ;τ,p)dp. | (A-9) |
As a result, by letting s=0, we could finally reach our target. This has completed the proof.
We now prove Proposition 2.2. The variance swap price is equal to
Kvar=1002TN∑i=1E[(Sti−Sti−1Sti−1)2]=1002TN∑i=1E[(eyti−1)2]=1002TN∑i=1E(e2yti−2eyti+1)=1002TN∑i=1[μ(−2j;ti−1,ti,v0,θ0,X0)−2μ(−j;ti−1,ti,v0,θ0,X0)+1], | (B-1) |
according to the specific formulation of the forward characteristic function.
We now start to deal with volatility swaps. We can compute
E[|Sti−Sti−1Sti−1|]=∫+∞0(eyti−1)p(yti)dyti+∫0−∞(1−eyti)p(yti)dyti,=−∫+∞0p(yti)dyti+∫0−∞p(yti)dyti+∫+∞0eytip(yti)dyti−∫0−∞eytip(yti)dyti, | (B-2) |
with p(yti) defined as the forward density function of yti. Clearly, all we need to do is to work out the four integrations. In fact, considering the fact that p(yti) is a density function, we could easily obtain
∫+∞0p(yti)dyti=12+1π∫+∞0RE[μ(ϕ;v0,θ0,X0,ti−1,ti)jϕ]dϕ, | (B-3) |
which is a famous theory relating the characteristic function and the density. Moreover, it should also be pointed out that
∫+∞−∞eytip(yti)dyti=μ(−j;v0,θ0,X0,ti−1,ti), | (B-4) |
which would lead to the conclusion that
ˉμ(ϕ;v0,θ0,X0,ti−1,ti)=μ(ϕ−j;v0,θ0,X0,ti−1,ti)μ(−j;v0,θ0,X0,ti−1,ti) | (B-5) |
denotes a characteristic function corresponding to a density eytip(yti)μ(−j;v0,θ0,X0,ti−1,ti). Therefore, one could obtain
∫+∞0eytip(yti)μ(−j;v0,θ0,X0,ti−1,ti)dyti=12+1π∫+∞0RE[μ(ϕ−j;v0,θ0,X0,ti−1,ti)jϕμ(−j;v0,θ0,X0,ti−1,ti)]dϕ. | (B-6) |
Combining Eqs (B-3) and (B-6) will lead us to the following equality
E[|Sti−Sti−1Sti−1|]=2π∫+∞0RE[μ(ϕ−j;v0,θ0,X0,ti−1,ti)−μ(ϕ;v0,θ0,X0,ti−1,ti)jϕ]dϕ, | (B-7) |
from which we could reach
Kvol=100√π2NTN∑i=1E[|Sti−Sti−1Sti−1|]=100√2πNT∫+∞0N∑i=1RE[μ(ϕ−j;v0,θ0,X0,ti−1,ti)−μ(ϕ;v0,θ0,X0,ti−1,ti)jϕ]dϕ. | (B-8) |
[1] |
P. V. Kokotović, Applications of singular perturbation techniques to control problems, SIAM Rev., 26 (1984), 501–550. https://doi.org/10.1137/1026104 doi: 10.1137/1026104
![]() |
[2] |
A. J. Chamkha, A. M. Rashad, E. R. El-Zahar, H. A. EL-Mky, Analytical and numerical investigation of Fe3O4-water nanofluid flow over a moveable plane in a parallel stream with high suction, Energies, 12 (2019), 198. https://doi.org/10.3390/en12010198 doi: 10.3390/en12010198
![]() |
[3] |
P. W. Hsieh, Y. Shih, S. Y. Yang, A tailored finite point method for solving steady MHD duct flow problems with boundary layers, Commun. Comput. Phys., 10 (2011), 161–182. https://doi.org/10.4208/cicp.070110.020710a doi: 10.4208/cicp.070110.020710a
![]() |
[4] |
M. Amir, Q. Ali, A. Raza, M. Y. Almusawa, W. Hamali, A. H. Ali, Computational results of convective heat transfer for fractionalized Brinkman type tri-hybrid nanofluid with ramped temperature and non-local kernel, Ain Shams Eng. J., 15 (2024), 102576. https://doi.org/10.1016/j.asej.2023.102576 doi: 10.1016/j.asej.2023.102576
![]() |
[5] |
O. Nave, M. Sharma, Singular perturbed vector field (SPVF) applied to complex ode system with hidden hierarchy application to turbocharger engine model, Int. J. Nonlinear Sci. Numer. Simul., 21 (2019), 99–113. https://doi.org/10.1515/ijnsns-2019-0024 doi: 10.1515/ijnsns-2019-0024
![]() |
[6] | G. P. Thomas, Towards an improved turbulence model for wave-current interactions, 2nd Annual Report to EU MAST-III Project The Kinematics and Dynamics of Wave-Current Interactions, 1988. |
[7] | R. O'Malley, Introduction to singular perturbations, Academic Press, 1974. |
[8] | J. Kevorkian, J. D. Cole, Perturbation methods in applied mathematics, Springer Science & Business Media, 1981. https://doi.org/10.1007/978-1-4757-4213-8 |
[9] | J. J. Miller, E. O'Riordan, G. Shishkin, Fitted numerical methods for singular perturbation problems, World Scientific, 2012. |
[10] |
C. S. Liu, E. R. El-Zahar, C. W. Chang, Higher-order asymptotic numerical solutions for singularly perturbed problems with variable coefficients, Mathematics, 10 (2022), 2791. https://doi.org/10.3390/math10152791 doi: 10.3390/math10152791
![]() |
[11] |
E. R. El-Zahar, S. M. El-Kabeir, A new method for solving singularly perturbed boundary value problems, Appl. Math. Inf. Sci., 7 (2013), 927. https://doi.org/10.12785/amis/070329 doi: 10.12785/amis/070329
![]() |
[12] |
E. R. El-Zahar, Approximate analytical solutions of singularly perturbed fourth order boundary value problems using differential transform method, J. King Saud Univ., 25 (2013), 257–265. https://doi.org/10.1016/j.jksus.2013.01.004 doi: 10.1016/j.jksus.2013.01.004
![]() |
[13] |
E. R. El-Zahar, Piecewise approximate analytical solutions of high-order singular perturbation problems with a discontinuous source term, Int. J. Differ. Equations, 2016 (2016), 1015634. https://doi.org/10.1155/2016/1015634 doi: 10.1155/2016/1015634
![]() |
[14] |
T. Valanarasu, N. Ramanujam, Asymptotic initial-value method for a system of singularly perturbed second-order ordinary differential equations of convection-diffusion type, Int. J. Comput. Math., 81 (2004), 1381–1393. https://doi.org/10.1080/0020716042000293187 doi: 10.1080/0020716042000293187
![]() |
[15] |
W. G. Melesse, A. A. Tiruneh, G. A. Derese, Solving systems of singularly perturbed convection diffusion problems via initial value method, J. Appl. Math., 2020 (2020), 1062025. https://doi.org/10.1155/2020/1062025 doi: 10.1155/2020/1062025
![]() |
[16] |
S. Valarmathi, N. Ramanujam, An asymptotic numerical method for singularly perturbed third-order ordinary differential equations of convection-diffusion type, Comput. Math. Appl., 44 (2002), 693–710. https://doi.org/10.1016/S0898-1221(02)00183-9 doi: 10.1016/S0898-1221(02)00183-9
![]() |
[17] |
J. C. Roja, A. Tamilselvan, Numerical method for singularly perturbed third order ordinary differential equations of convection-diffusion type, Numer. Math., 7 (2014), 265–287. https://doi.org/10.1017/S1004897900000118 doi: 10.1017/S1004897900000118
![]() |
[18] |
M. Cui, F. Geng, A computational method for solving third order singularly perturbed boundary-value problems, Appl. Math. Comput., 198 (2008), 896–903. https://doi.org/10.1016/j.amc.2007.09.023 doi: 10.1016/j.amc.2007.09.023
![]() |
[19] |
V. Shanthi, N. Ramanujam, A boundary value technique for boundary value problems for singularly perturbed fourth-order ordinary differential equations, Comput. Math. Appl., 47 (2004), 1673–1688. https://doi.org/10.1016/j.camwa.2004.06.015 doi: 10.1016/j.camwa.2004.06.015
![]() |
[20] |
M. I. Syam, B. S. Attili, Numerical solution of singularly perturbed fifth order two-point boundary value problem, Appl. Math. Comput., 170 (2005), 1085–1094. https://doi.org/10.1016/j.amc.2005.01.003 doi: 10.1016/j.amc.2005.01.003
![]() |
[21] |
V. Shanthi, N. Ramanujam, An asymptotic numerical method for fourth order singular perturbation problems with a discontinuous source term, Int. J. Comput. Math., 85 (2008), 1147–1159. https://doi.org/10.1080/00207160701478862 doi: 10.1080/00207160701478862
![]() |
[22] | T. Valanarasu, N. Ramanujam, Asymptotic numerical method for singularly perturbed third order ordinary differential equations with a discontinuous source term, Novi Sad J. Math., 37 (2007), 41–57. |
[23] |
A. R. Babu, N. Ramanujam, An asymptotic finite element method for singularly perturbed third and fourth order ordinary differential equations with discontinuous source term, Appl. Math. Comput., 191 (2007), 372–380. https://doi.org/10.1016/j.amc.2007.02.093 doi: 10.1016/j.amc.2007.02.093
![]() |
[24] | A. R. Babu, N. Ramanujam, An asymptotic finite element method for singularly perturbed higher order ordinary differential equations of convection-diffusion type with discontinuous source term, J. Appl. Math. Inf., 26 (2008), 1057–1069. |
[25] |
V. Shanthi, N. Ramanujam, An asymptotic hybrid difference scheme for singularly perturbed third and fourth order ordinary differential equations with discontinuous source term, Neural Parallel Sci. Comput., 16 (2008), 327–336. https://doi.org/10.5555/1561709.1561712 doi: 10.5555/1561709.1561712
![]() |
[26] |
M. Chandr, V. Shanthi, A Schwarz method for fourth-order singularly perturbed reaction-diffusion problem with discontinuous source term, J. Appl. Math. Inf., 34 (2016), 495–508. http://doi.org/10.14317/jami.2016.495 doi: 10.14317/jami.2016.495
![]() |
[27] |
P. C. Podila, V. Sundrani, H. Ramos, Numerical solution of a fourth-order singularly perturbed boundary value problem with discontinuities via Haar wavelets, Math. Methods Appl. Sci., 45 (2022), 10904–10916. https://doi.org/10.1002/mma.8424 doi: 10.1002/mma.8424
![]() |
[28] |
M. S. Alam, N. Sharif, M. H. U. Molla, Combination of modified Lindstedt-Poincare and homotopy perturbation methods, J. Low Freq. Noise Vibration Active Control, 42 (2022), 642–653. https://doi.org/10.1177/14613484221148049 doi: 10.1177/14613484221148049
![]() |
[29] |
N. H. Aljahdaly, A. M. Alweldi, On the modified Laplace homotopy perturbation method for solving damped modified Kawahara equation and its application in a fluid, Symmetry, 15 (2023), 394. https://doi.org/10.3390/sym15020394 doi: 10.3390/sym15020394
![]() |
[30] |
S. R. M. Noori, N. Taghizadeh, Modified differential transform method for solving linear and nonlinear pantograph type of differential and Volterra integro-differential equations with proportional delays, Adv. Differ. Equations, 2020 (2020), 649. https://doi.org/10.1186/s13662-020-03107-9 doi: 10.1186/s13662-020-03107-9
![]() |
[31] |
B. Benhammouda, H. Vazquez-Leal, L. Hernandez-Martinez, Modified differential transform method for solving the model of pollution for a system of lakes, Discrete Dyn. Nat. Soc., 2014 (2014), 645726. https://doi.org/10.1155/2014/645726 doi: 10.1155/2014/645726
![]() |
[32] |
A. E. Ebaid, A reliable aftertreatment for improving the differential transformation method and its application to nonlinear oscillators with fractional nonlinearities, Commun. Nonlinear Sci. Numer. Simul., 16 (2011), 528–536. https://doi.org/10.1016/j.cnsns.2010.03.012 doi: 10.1016/j.cnsns.2010.03.012
![]() |
[33] |
S. Momani, O. A. Arqub, M. A. Hammad, Z. A. Hammour, A residual power series technique for solving systems of initial value problems, Appl. Math. Inf. Sci., 10 (2016), 765–775. https://doi.org/10.18576/AMIS/100237 doi: 10.18576/AMIS/100237
![]() |
[34] |
E. R. El-Zahar, G. F. Al-Boqami, H. S. Al-Juaydi, Approximate analytical solutions for strongly coupled systems of singularly perturbed convection-diffusion problems, Mathematics, 12 (2024), 277. https://doi.org/10.3390/math12020277 doi: 10.3390/math12020277
![]() |
[35] |
A. Dawar, H. Khan, S. Islam, W. Khan, The improved residual power series method for a system of differential equations: a new semi-numerical method, Int. J. Model. Simul., 43 (2023), 1–14. https://doi.org/10.1080/02286203.2023.2270884 doi: 10.1080/02286203.2023.2270884
![]() |
[36] |
F. Chen, Q. Q. Liu, Adomian decomposition method combined with Padé approximation and Laplace transform for solving a model of HIV infection of CD4+T cells, Discrete Dyn. Nat. Soc., 2015 (2015), 584787. https://doi.org/10.1155/2015/584787 doi: 10.1155/2015/584787
![]() |
[37] |
W. B. Jones, W. J. Thron, On convergence of Padé approximants, SIAM J. Math. Anal., 6 (1975), 9–16. https://doi.org/10.1137/0506002 doi: 10.1137/0506002
![]() |
[38] | J. Anderson, Fundamentals of aerodynamics, McGraw-Hill Education. https://doi.org/10.2514/152157 |
[39] | K. Stephan, Heat transfer in condensation and boiling, Springer-Verlag, 1992. https://doi.org/10.1007/978-3-642-52457-8 |
[40] | J. Jackson, Classical electrodynamics, Wiley, 1998. |
[41] | R. Burden, J. D. Faires, A. M. Burden, Numerical analysis, Cengage Learning, 2021. |
1. | Xin-Jiang He, Puneet Pasricha, Sha Lin, Analytically pricing European options in dynamic markets: Incorporating liquidity variations and economic cycles, 2024, 139, 02649993, 106839, 10.1016/j.econmod.2024.106839 | |
2. | Xin-Jiang He, Puneet Pasricha, Tuantuan Lu, Sha Lin, Vulnerable options with regime switching and stochastic liquidity, 2024, 98, 10629769, 101930, 10.1016/j.qref.2024.101930 | |
3. | Xin-Jiang He, Shou-De Huang, Sha Lin, A closed-form solution for pricing European-style options under the Heston model with credit and liquidity risks, 2025, 143, 10075704, 108595, 10.1016/j.cnsns.2025.108595 | |
4. | Xin‐Jiang He, Hang Chen, Sha Lin, A Closed‐Form Formula for Pricing European Options With Stochastic Volatility, Regime Switching, and Stochastic Market Liquidity, 2025, 0270-7314, 10.1002/fut.22573 | |
5. | Beng Feng, Congyin Fan, American call option pricing under the KoBoL model with Poisson jumps, 2025, 20, 1556-1801, 143, 10.3934/nhm.2025009 |