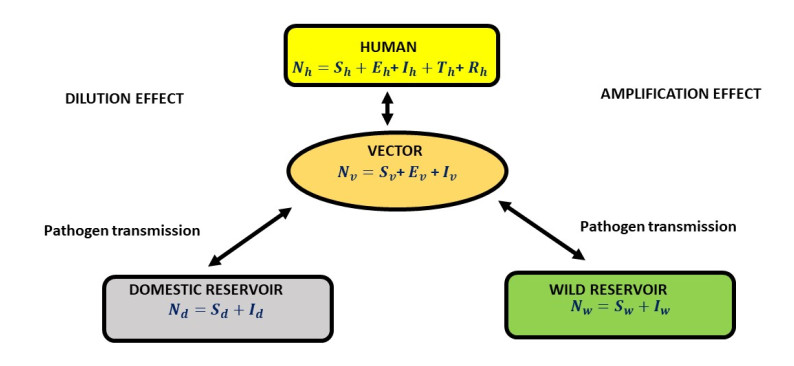
Citation: Huqing Wang, Feng Xiang, Wenbing Zhao, Zhixin Sun. ONS resolution prediction based on Rasch model[J]. Mathematical Biosciences and Engineering, 2019, 16(6): 6683-6695. doi: 10.3934/mbe.2019333
[1] | Simone Göttlich, Stephan Knapp . Modeling random traffic accidents by conservation laws. Mathematical Biosciences and Engineering, 2020, 17(2): 1677-1701. doi: 10.3934/mbe.2020088 |
[2] | Stephan Gerster, Michael Herty, Elisa Iacomini . Stability analysis of a hyperbolic stochastic Galerkin formulation for the Aw-Rascle-Zhang model with relaxation. Mathematical Biosciences and Engineering, 2021, 18(4): 4372-4389. doi: 10.3934/mbe.2021220 |
[3] | Daniele Bernardo Panaro, Andrea Trucchia, Vincenzo Luongo, Maria Rosaria Mattei, Luigi Frunzo . Global sensitivity analysis and uncertainty quantification for a mathematical model of dry anaerobic digestion in plug-flow reactors. Mathematical Biosciences and Engineering, 2024, 21(9): 7139-7164. doi: 10.3934/mbe.2024316 |
[4] | Francesca Marcellini . The Riemann problem for a Two-Phase model for road traffic with fixed or moving constraints. Mathematical Biosciences and Engineering, 2020, 17(2): 1218-1232. doi: 10.3934/mbe.2020062 |
[5] | Alex Capaldi, Samuel Behrend, Benjamin Berman, Jason Smith, Justin Wright, Alun L. Lloyd . Parameter estimation and uncertainty quantification for an epidemic model. Mathematical Biosciences and Engineering, 2012, 9(3): 553-576. doi: 10.3934/mbe.2012.9.553 |
[6] | Sebastien Motsch, Mehdi Moussaïd, Elsa G. Guillot, Mathieu Moreau, Julien Pettré, Guy Theraulaz, Cécile Appert-Rolland, Pierre Degond . Modeling crowd dynamics through coarse-grained data analysis. Mathematical Biosciences and Engineering, 2018, 15(6): 1271-1290. doi: 10.3934/mbe.2018059 |
[7] | Raimund Bürger, Paola Goatin, Daniel Inzunza, Luis Miguel Villada . A non-local pedestrian flow model accounting for anisotropic interactions and domain boundaries. Mathematical Biosciences and Engineering, 2020, 17(5): 5883-5906. doi: 10.3934/mbe.2020314 |
[8] | Piotr Klejment . Application of supervised machine learning as a method for identifying DEM contact law parameters. Mathematical Biosciences and Engineering, 2021, 18(6): 7490-7505. doi: 10.3934/mbe.2021370 |
[9] | H. T. Banks, Robert Baraldi, Karissa Cross, Kevin Flores, Christina McChesney, Laura Poag, Emma Thorpe . Uncertainty quantification in modeling HIV viral mechanics. Mathematical Biosciences and Engineering, 2015, 12(5): 937-964. doi: 10.3934/mbe.2015.12.937 |
[10] | Dandan Fan, Dawei Li, Fangzheng Cheng, Guanghua Fu . Effects of congestion charging and subsidy policy on vehicle flow and revenue with user heterogeneity. Mathematical Biosciences and Engineering, 2023, 20(7): 12820-12842. doi: 10.3934/mbe.2023572 |
Vector-borne diseases that demonstrate specific feeding preferences and a predilection for competent hosts are a relevant public health issue due to the complexity of these two combined effects, particularly in bio-diverse environments. Existing literature that seeks to understand the combined effects of host competency and vector-feeding preference is limited, and further research is needed. Existing studies have modeled vector-host transmission using either vector-feeding preference or host competence, albeit separately [1,2,3,4]. However, no research has been conducted to analyze the combination of both transmission effects in multiple hosts for cutaneous leishmaniasis and Chagas disease. In this novel study, we build upon the work of [1,2,3,4,5,6,7] and analyze how different host competencies and vector-feeding preferences are combined in transmission when the vector's successful biting is fractioned. The vector bites are diverted by the fraction of successful biting from the vector, which potentially amplifies the disease to (humans/domestic host/wild host), and by the fraction of successful biting from vector, which potentially dilutes the disease to (humans/domestic host/ wild host). Although this complex dynamic is exemplified using cutaneous leishmaniasis and Chagas disease, this mathematical model can be applied to other vector-borne diseases. The mathematical model presented in the next section can be used to analyze the combined effects of different host competencies and vector-feeding preferences in leishmaniasis, Chagas disease, dengue fever, west Nile virus, malaria, and other diseases lined to humans and domestic and wild animal hosts [2,8]. Wild animals commonly serve as a major vector for the transmission of zoonotic agents to hosts such as domestic animals and humans [9]. Managing animal hosts often plays a crucial role in effective disease control [10,11,12,13]. Identifying which species are the most common reservoir hosts serving to infect those vectors could help to suggest new host-control strategies.
Host competence is an important component to incorporate in vector-host transmission models and is defined as the ability of a host to effectively transmit infection to another susceptible host or vector [14]. Host competence is determined by several factors, including susceptibility to infection with the pathogen, whether it can sustain infection with the pathogen, and whether it can infect vectors with the pathogen [3]. Host competence is a crucial determinant of epidemiological dynamics, because it considers what happens inside a host to what happens among its hosts communities [3]. A competent host for the agent of a vector-borne disease readily acquires infection from the vector, permits proliferation of the agent, and readily infects another vector [15]. Furthermore, a competent host must be able to sustain the infection agent for an extended period of time [9,16]. Conversely, the presence of a less-competent host could decrease the risk of disease occurring at the community level [17]. Different host competencies could dilute, maintain, or amplify the presence of a parasite in the environment and facilitate epidemics [3].
Vector-feeding preference is relevant to include in models because vectors with strong host selection contribute to the spread of vector-borne zoonotic diseases. Vector-feeding preference can be defined as the specific trait of vectors' preferentially selecting specific hosts above others [2]. As a rule of thumb, blood is the most important nutritional resource of vectors [18]. Host-selection preference by vectors can be understood as an adaptive factor that leads to optimal reproductive fitness of the vector [2]. Kilpatrick et al. affirmed that Cx. Pipiens feeding preference on robins contributes a fitness benefit to the vector that cannot be compared with any other bird [19]. A vector's preference for a specific host is affected by internal and external factors [20]. Internal factors include the genetic and physiological makeup of the host as well as the presence of internal parasites [20]. External factors include odors, color, body heat, body mass, temperature, gender, and parasites [20]. In leishmaniasis, Lutzomyia longipalpis reveals specific preference for dogs, chickens, and horses. These animals attract Lutzomyias to breeding sites in peri-domestic areas [21]. In Chagas disease, experiments show that the Triatoma infestans bugs prefer to feed on dogs rather than chickens and cats [22]. In dengue, laboratory studies have reported a very strong preference of Ae.aegypti for human odors [20]. In malaria, Anopheles gambie shows utmost preference for human blood [23]. Host preference also depends on the time of feeding and the availability of the vector to feed indoors or outdoors. Both human and animal host species are critical for both feeding vectors and serving as reservoirs of zoonotic vector-born pathogens [10]. According to [14], a reservoir is defined as "one or more epidemiologically connected populations or environments in which the pathogen could be permanently maintained and from which infection is transmitted to the defined target host population." Pathogens that can infect more than one host species are commonly found in host populations [11,17,24,25]. As stated in [26], diversity amplification occurs when the vector prefers to feed on the reservoir host with the highest transmission capability, while dilution effect is presented when the vector prefers to bite the host with the least transmission capability or when it does not show any preference. It should be noted that in this work, we derived a general model for the transmission dynamic between vectors and three different hosts. Despite the generality of the mathematical model, we are interested in its application to cutaneous leishmaniasis and Chagas disease, considering the relationship of the most relevant different combinations of vector-feeding preferences with their respective host competencies.
The model in Figure 1 describes the dynamic of vectors and humans with two different types of reservoirs. We hypothesized the following:
1. The vector population transmit the pathogen through the amplification and dilution effects to the human population, the domestic and wild reservoir population.
2. Human population (Nh) (indexed by h) is divided in five stages: susceptible (Sh), asymptomatic (Eh), Infectious, clinically ill (Ih), Treated (Th), Recovered and non-immune (Rh). Domestic reservoir (Nd) (indexed by d) and wild reservoir (Nw) (indexed by w) populations are divided into two stages: susceptible (Sd and Sw), infectious, ill (Id and Iw), the vector population (indexed by v) is divided into three stages: susceptible (Sv), infected but not infectious (Ev) and infectious (Iv). The biting feeding of the vector population is fractioned of successful biting from vectors that amplify the disease to (human/ domestic reservoir/ wild reservoir) populations and by the fraction of successful biting from vectors that dilute the disease to (human / domestic reservoir/ wild reservoir) populations (See Table 1 for parameter description).
Parameter | Definition |
αavh | feeding preference by vector that amplifies the disease on humans |
αdvh | feeding preference by vector that dilutes the disease on humans |
αavdr | feeding preference by vector that amplifies the disease on domestic reservoir |
αdvdr | feeding preference by vector that dilutes the disease on domestic reservoir |
αavwr | feeding preference by vector that amplifies the disease on wild reservoir |
αdvwr | feeding preference by vector that dilutes the disease on wild reservoir |
βavh | pathogen transmission probability from vector that amplifies the disease to humans |
βdvh | pathogen transmission probability from vector that dilutes the disease to humans |
βavdr | pathogen transmission probability from vector that amplifies the disease to domestic reservoir |
βdvdr | pathogen transmission probability from vector that dilutes the disease to domestic reservoir |
βavwr | pathogen transmission probability from vector that amplifies the disease to wild reservoir |
βdvwr | pathogen transmission probability from vector that dilutes the disease to wild reservoir |
bh | biting rate from vector to humans |
bd | biting rate from vector to domestic reservoir |
bw | biting rate from vector to wild reservoir |
fh | fraction of successful biting from vector that amplifies the disease to humans |
1−fh | fraction of successful biting from vector that dilutes the disease to humans |
fd | fraction of successful biting from vector that amplifies the disease to domestic reservoir |
1−fd | fraction of successful biting from vector that dilutes the disease to domestic reservoir |
fw | fraction of successful biting from vector that amplifies the disease to domestic reservoir |
1−fw | fraction of successful biting from vector that dilutes the disease to wild reservoir |
f | fraction of recovery rate from infectious human |
η | fraction of recovery rate by treatment |
a | fraction of infected humans transmitting the pathogen |
ωh | loss immunity rate from humans |
ωd | loss immunity rate from domestic reservoir |
ωw | loss immunity rate from wild reservoir |
μh | human mortality rate |
μd | domestic reservoir mortality rate |
μw | wild reservoir mortality rate |
μv | vector mortality rate |
σh | intrinsic incubation period in humans |
σv | extrinsic incubation period in vectors |
δh | Disease induced death rate in humans |
δd | Disease induced death rate in domestic reservoirs |
δw | Disease induced death rate in wild reservoirs |
rh | recovery rate for infectious humans receiving treatment |
θh | treatment rate |
ch | human ability to transmit the pathogen to vector |
cd | domestic reservoir ability to transmit the pathogen to vector |
cw | wild reservoir ability to transmit the pathogen to vector |
3. The presence of wild and domestic reservoirs for vectors might alter vector's feeding preference away from humans thus reducing or increasing the pathogen transmission [27].
4. The following mathematical model is an extension of the model presented in [1,2,3,4], due to the fact that we are comparing both, vector's feeding preferences and host's competencies when bites are fractioned.
We tackle these hypotheses explaining why these effects arise using a compartmental model in Figure 1 that combine the eco-epidemiological interactions between vector, human, domestic and wild reservoir populations. We translate the compartmental model plus the hypotheses formulated above into the following set of differential equations.
Dynamic of human population:
S′h=μhNh−λhSh+ωhIh−μhShE′h=λhSh−Q1EhI′h=fσhEh−Q2IhT′h=ηrhIh−Q3ThR′h=rh(1−η)Ih+(1−f)σhEh+θhTh−(ωh+μh)Rh | (2.1) |
Dynamic of domestic reservoir population:
S′d=μdNd−λdSd+ωdId−μdSdI′d=λdSd−Q4Id | (2.2) |
Dynamic of wild reservoir population:
S′w=μwNw−λwSw+ωwIw−μwSwI′w=λwSw−Q5Iw | (2.3) |
Dynamic of vector population:
S′v=μvNv−λvSv−μvSvE′v=λvSv−Q6EvI′v=σvEv−μvIv | (2.4) |
and the forces of infection of the model are:
λh=bhαavhβavhfhIv+αdvhbhβdvh(1−fh)Iv(αavh+αdvh)Nh+(αadr+αdvdr)Nd+(αavwr+αdvwr)Nw |
λd=bdαavdrβavdrfdIv+αdvdrbdβdvdr(1−fd)Iv(αavh+αdvh)Nh+(αavdr+αdvdr)Nd+(αavwr+αdvwr)Nw |
λw=bwαavwrβavwrfwIv+αdvwrbwβdvwr(1−fw)Iv(αavh+αdvh)Nh+(αavdr+αdvdr)Nd+(αavwr+αdvwr)Nw |
λv=aa(Ih+aEh)+bbId+ccIw(αavh+αdvh)Nh+(αavdr+αdvdr)Nd+(αavwr+αdvwr)Nw |
where,
Q1=(σh+μh), Q2=(ωh+μh+δh),Q3=(θh+μh), Q4=(ωd+μd+δd), Q5=(ωw+μw+δw), Q6=(σv+μv), |
aa=bhch(αavhβavhfh+αdvhβdvh(1−fh)) |
bb=bdcd(αavdrβavdrfd+αdvdrβdvdr(1−fd)) |
cc=bwcw(αavwrβavwrfw+αdvwrβdvwr(1−fw)) |
According to Anderson and May in [28] the Disease Free equilibrium (DFE) state describes the situation in which there is no disease in the population. The DFE for the mathematical model described before is:
E0=(N0h,0,0,0,0,N0d,0,N0w,0,N0v,0,0) |
The basic reproduction number indicated by R0, is the expected number of secondary cases produced in a completely susceptible population, by a typical infectious individual. If R0<1, then each infectious individual produces on average, less than one new infectious individual during its infectious period, and the infection cannot grow. Contrariwise, if R0>1, then each infectious individual produces on average, more than one new infection, and the disease can invade the population [29,30]. In order to calculate the R0 of our model, we used the technique presented by Van den Driessche and Watmough in [29]. Essentially, the R0 analysis is a particular case of stability analysis of the disease free equilibrium state condition. We present the equation of R0 and its corresponding biological significance as follows:
R0=√σvRRWWμvQ5Q6+σvMMTTμvQ4Q6+σvAAμvQ6(DDfσhQ2Q1+SSQ1) |
where
σvRRWWμvQ5Q6 |
captures the effective transmission of the pathogen from vectors to wild reservoirs plus the dilution and amplification effects. Also, this fraction captures the wild reservoir ability to transmit the pathogen to vectors, plus the dilution and amplification effects.
σvMMTTμvQ4Q6 |
captures the effective transmission of the pathogen from vectors to domestic reservoirs plus the dilution and amplification effects. Also, this fraction captures the domestic reservoir ability to transmit the pathogen to vectors, plus the dilution and amplification effects.
σvAAμvQ6(DDfσhQ2Q1+SSQ1) |
captures the effective transmission of the pathogen from vectors to humans plus the dilution and amplification effects. Also, this fraction captures the humans ability to transmit the pathogen to vectors, plus the dilution and amplification effects.
AA=(bhαdvhβdvh(1−fh)+αavhβavhbhfh)N0h(αavh+αdvh)N0h+(αavdr+αdvdr)N0d+(αavwr+αdvwr)N0w |
is a fraction that captures the successful transmission of the pathogen from vectors to humans plus the dilution and amplification effects.
MM=(bdαdvdrβdvdr(1−fd)+αavdrβavdrbdfd)N0d(αavh+αdvh)N0h+(αavdr+αdvdr)N0d+(αavwr+αdvwr)N0w |
is a fraction that captures the successful transmission of the pathogen from vectors to domestic reservoirs plus the dilution and amplification effects.
RR=(bwαdvwrβdvwr(1−fw)+αavwrβavwrbwfw)N0w(αavh+αdvh)N0h+(αavdr+αdvdr)N0d+(αavwr+αdvwr)N0w |
is a fraction that captures the successful transmission of the pathogen from vectors to wild reservoirs plus the dilution and amplification effects.
SS=(ach(bhαdvhβdvh(1−fh)+αavhβavhbhfh))N0v(αavh+αdvh)N0h+(αavdr+αdvdr)N0d+(αavwr+αdvwr)N0w |
is a fraction that captures a portion of humans' ability to transmit the pathogen to vectors plus the dilution and amplification effects.
DD=(ch(bhαdvhβdvh(1−fh)+αavhβavhbhfh))N0v(αavh+αdvh)N0h+(αavdr+αdvdr)N0d+(αavwr+αdvwr)N0w |
is a fraction that captures the humans' ability to transmit the pathogen to vectors plus the dilution and amplification effects.
TT=(cd(bdαdvdrβdvdr(1−fd)+αavdrβavdrbdfd))N0v(αavh+αdvh)N0h+(αavdr+αdvdr)N0d+(αavwr+αdvwr)N0w |
is a fraction that captures the domestic reservoirs' ability to transmit the pathogen to vectors plus the dilution and amplification effects.
WW=(cw(bwαdvwrβdvwr(1−fw)+αavwrβavwrbwfw))N0w(αavh+αdvh)N0h+(αavdr+αdvdr)N0d+(αavwr+αdvwr)N0w |
is a fraction that captures the wild reservoirs' ability to transmit the pathogen to vectors plus the dilution and amplification effects.
Cutaneous leishmaniasis is a skin infection that is generated by Leishmania protozoa, which is transmitted by the bite of a female sandfly [31,32,33,34,35,36,37,38] to humans and domestic and wild reservoirs. Investigations in [32,39,40,41], have determined that sandflies have nocturnal feeding behavior, and their indoor and outdoor populations are an essential part of the transmission of Leishmania. This behavior of sandflies has been reported in approximately 90 countries [39]. The knowledge and the discovery of Leishmania species and their natural vectors and reservoirs behavior are helping to determine the nature and amplitude of this disease. Cutaneous leishmaniasis is endemic in most of the places located between latitudes 5S and 13S of the Neotropical ecozone [2,31].
Chagas disease is caused by Trypanozoma cruzi parasite which is transmitted by triatomine bugs to humans and domestic and wild animals [42]. This disease is located only in the Americas, particularly in rural areas of Latin America. The evidence in [22] shows that the domestic reservoirs of Chagas disease, such as dogs, cats, rodents, and guinea pigs, are able to maintain T. cruzi in absence of any other reservoirs. According to [43], Chagas disease and its reservoirs and vectors are characterized by enzooty, which means that the disease could be maintained by wild animals and vectors, with reported cases from the southern United States of America to South American countries.
The diseases caused by protozoa of the order Kinetoplastida, collectively known as the Trypanosomiases and leishmaniasis, torture millions of the world's poorest population [44]. Reviews in [24] and [45] described around 87 reservoirs for Leishmania and 150 for T.cruzi in the Americas. Cutaneous leishmaniasis and Chagas disease are considered to be zoonotic due to the fact that both are naturally transmitted from animals to humans.
In this research we are interested in finding the relationship of cutaneous leishmaniasis and Chagas disease, because they have a domestic and wild route of parasite transmission by vectors to different reservoir hosts, including humans. There are some investigations of mathematical modeling of cutaneous leishmaniasis and Chagas disease in previous literature [1,2,6,22,39,46,47,48], but no other has inserted both characteristics of vector-feeding preference and host competencies.
We use the corresponding parameters of cutaneous leishmaniasis (Table 2, Figure 2, Figure 3) and Chagas disease (Table 3, Figure 4, Figure 5) for illustrating simulations of the relationship between the different feeding preferences by vectors and the host competencies for the mathematical model described in the methods section.
Parameter | Value | Range | Reference |
αavh | 3.0 | [0–40.0] | hypothetical |
αdvh | 2.0 | [0–40.0] | hypothetical |
αavdr | 4.0 | [0–40.0] | hypothetical |
αdvdr | 2.0 | [0–40.0] | hypothetical |
αavwr | 5.0 | [0–40.0] | hypothetical |
αdvwr | 2.0 | [0–40.0] | hypothetical |
βavh | 0.7198 | [0.01–0.9] | [39] [40] |
βdvh | 0.0521 | [0.01–0.9] | hypothetical |
βavdr | 0.850 | [0.01–0.9] | hypothetical |
βdvdr | 0.097 | [0.01–0.9] | hypothetical |
βavwr | 0.7 | [0.01–0.9] | hypothetical |
βdvwr | 0.07 | [0.01–0.9] | hypothetical |
bh | 0.6878 | [0.025–2.0] | [39], [40] |
bd | 0.3 | [0.025–2.0] | [40] |
bw | 0.08 | [0.025–2.0] | [40] |
fh | 0.6 | [0.0001–1.0] | hypothetical |
fd | 0.8 | [0.0001–1.0] | hypothetical |
fw | 0.4 | [0.0001–1.0] | hypothetical |
f | 0.005 | [0.001–0.9] | hypothetical |
η | 0.65 | [0.07–0.8] | hypothetical |
a | 0.5 | [0.007–0.8] | hypothetical |
ωh | 0.0013 | [0.000004–0.04] | [41], [49] |
ωd | 0.003 | [0.00003–0.03] | [50] |
ωw | 0.0007 | [0.00003–0.002] | [46] |
μh | 0.000000413 | [0.0000003, 0.0003] | [39] |
μd | 0.0000821 | [0.00001, 0.009] | [46] |
μw | 0.021 | [0.0002–0.09] | [46] |
μv | 0.0135 | [0.002–0.9] | [41] |
σh | 0.0158 | [0.00013–0.5] | [41], [49] |
σv | 0.035 | [0.003–0.7] | [41] |
δh | 0.0000008 | [0.0000001–0.009] | [39] |
δd | 0.0008 | [0.00005–0.05] | hypothetical |
δw | 0.00008 | [0.00005–0.03] | hypothetical |
rh | 0.091 | [0.00001–0.9] | hypothetical |
θh | 0.035 | [0.0002–0.7] | [51] |
ch | 0.4 | [0.00001–1.0] | hypothetical |
cd | 0.3 | [0.00001–1.0] | hypothetical |
cw | 0.1 | [0.00001–1.0] | hypothetical |
N0h | 100,050 | [100.0–1000000.0] | hypothetical |
N0d | 100,050 | [100.0–200000.0] | hypothetical |
N0w | 50,050 | [100.0–100000.0] | hypothetical |
N0v | 5'000,500 | [1000.0–10000000.0] | hypothetical |
Parameter | Value | Range | Reference |
αavh | 6.0 | [0–40.0] | hypothetical |
αdvh | 4.0 | [0–40.0] | hypothetical |
αavdr | 7.0 | [0–40.0] | hypothetical |
αdvdr | 3.0 | [0–40.0] | hypothetical |
αavwr | 5.0 | [0–40.0] | hypothetical |
αdvwr | 2.0 | [0–40.0] | hypothetical |
βavh | 0.49 | [0.001–0.9] | [14], [52] |
βdvh | 0.02 | [0.001–0.9] | [7] |
βavdr | 0.5 | [0.001–0.9] | hypothetical |
βdvdr | 0.09 | [0.001–0.9] | hypothetical |
βavwr | 0.4 | [0.001–0.9] | [47] |
βdvwr | 0.05 | [0.001–0.9] | [48] |
bh | 0.9 | [0.0025–3.0] | [6] |
bd | 0.3 | [0.0025–3.0] | hypothetical |
bw | 0.1 | [0.0025–3.0] | hypothetical |
fh | 0.6 | [0.0001–1.0] | hypothetical |
fd | 0.8 | [0.0001–1.0] | hypothetical |
fw | 0.4 | [0.0001–1.0] | hypothetical |
f | 0.7 | [0.001–0.9] | hypothetical |
η | 0.02 | [0.007–0.8] | hypothetical |
a | 0.5 | [0.007–0.8] | hypothetical |
ωh | 0.0013 | [0.000004–0.04] | hypothetical |
ωd | 0.003 | [0.00003–0.03] | hypothetical |
ωw | 0.0007 | [0.00003–0.002] | hypothetical |
μh | 0.000000413 | [0.0000003, 0.0003] | [39] |
μd | 0.001 | [0.00001, 0.09] | [52] |
μw | 0.00021 | [0.0001–0.09] | [46] |
μv | 0.00327 | [0.001–0.9] | [53] |
σh | 0.1 | [0.00013–0.9] | [54] |
σv | 0.035 | [0.003–0.9] | [41] |
δh | 0.000057 | [0.0000001–0.0009] | [6] |
δd | 0.0005 | [0.00005–0.05] | hypothetical |
δw | 0.0003 | [0.00005–0.03] | hypothetical |
rh | 0.03 | [0.00001–0.9] | hypothetical |
θh | 0.016 | [0.0002–0.7] | [44] |
ch | 0.9 | [0.00001–1.0] | hypothetical |
cd | 0.3 | [0.00001–1.0] | hypothetical |
cw | 0.7 | [0.00001–1.0] | hypothetical |
N0h | 500,050 | [100.0–1000000.0] | hypothetical |
N0d | 100,050 | [100.0–200000.0] | hypothetical |
N0w | 50,050 | [100.0–100000.0] | hypothetical |
N0v | 5'000,500 | [1000.0–10000000.0] | hypothetical |
Results from Figure 2(a) and Figure 4(a) contrast the differences in cutaneous leishmaniasis and Chagas disease, respectively, between the relationship of the domestic reservoir competence and the feeding preference by vector that amplifies the disease on domestic reservoir (αavdr). Figure 2(b) and Figure 4(b) contrast the differences in cutaneous leishmaniasis and Chagas disease, respectively, between the relationship of the domestic reservoir competence (cd) and the feeding preference by vector that dilutes the disease on domestic reservoir (αdvdr).
Figure 2(c) and Figure 4(c) contrast the differences in cutaneous leishmaniasis and Chagas disease, respectively, between the relationship of the human competence (ch) and the feeding preference by vector that amplifies the disease to humans (αavh). However, Figure 2(d) and Figure 4(d) illustrate a similar pattern for cutaneous leishmaniasis and Chagas disease, respectively, between the wild reservoir competence cw and the feeding preference by vector that dilutes the disease to wild reservoirs (αdvwr).
On the other hand, Figure 3(a) illustrates the proportion of infectious vectors (sandflies) and the domestic reservoir competence (cd) regarding cutaneous leishmaniasis. The proportion of infectious vectors increases, while the domestic reservoir competence increases. The proportion of infectious vectors varies over time between the values 0.1 to 0.26. Figure 5(a) illustrates the proportion of infectious vectors (triatomines) and the domestic reservoir competence (cd) regarding Chagas disease. The proportion of infectious vectors increases, while the domestic reservoir competence increases. The proportion of infectious vectors varies over time between the values 0.1 to 0.37. Figure 3(b) illustrates the proportion of infectious vectors (sandflies) and the human competence (ch) regarding cutaneous leishmaniasis. Proportion of infectious vectors increases, while (ch) increases. The proportion of infectious vectors varies over time between the values 0.1 to 0.22 regarding to cutaneous leishmaniasis.
Figure 5(b) illustrates the proportion of infectious vectors (triatomines) and human competence(ch) regarding Chagas disease. Proportion of infectious vectors increases while the human competence increases. The proportion of infectious vectors varies between the values 0.1 to 0.37. Figure 3(c) illustrates the proportion of infectious vectors (sandflies) and the feeding preference by vector that dilutes the disease to humans (αdvh) regarding cutaneous leishmaniasis. Proportion of infectious vectors decreases, while (αdvh) increases. The proportion of infectious vectors varies over time between the values 0.1 to 0.22.
Figure 5(c) illustrates the proportion of infectious vectors (triatomines) and the feeding preference by vector that dilutes the disease to humans (αdvh) regarding Chagas disease. Proportion of infectious vectors decreases while (αdvh) increases. The proportion of infectious vectors varies over time between the values 0.1 to 0.36. Figure 3(d) illustrates the proportion of infectious vectors (sandflies) and the feeding preference by vector that amplifies the disease to humans (αavh) regarding cutaneous leishmaniasis. Proportion of infectious vectors increases, while (αavh) increases. The proportion of infectious vectors varies over time between the values 0.1 to 0.23. Figure 5(d) illustrates the proportion of infectious vectors (triatomines) and the feeding preference by vector that amplifies the disease to humans (αavh) regarding Chagas disease. Proportion of infectious vectors increases, while (αavh) increases. The proportion of infectious vectors varies over time between the values 0.1 to 0.46.
Figures 3 and 5 represent the proportion of infectious vectors (y-axes) as a function of parameters (x-axes) and time (box plots) regarding cutaneous leishmaniasis and Chagas disease. The box plots represent the time series data for the proportion of infectious vectors at a given value of parameter on the x-axis: the lower end of the box plot corresponds to time = 0, beginning from a fixed initial condition for solving the ODE. The upper end is at time = ‘typical life-span of vectors’ (30 days for sand flies in leishmaniasis and 305 days for triatomine bugs in Chagas disease). This is the reason why all box plots indicate the same minimum value while the maximum value and other quantiles (25th, median, 75th percentiles) vary depending on amplification or dilution effects in the system. For amplification the quantiles and maximum value increase monotonically while they decrease monotonically in dilution effect. Therefore, the box plots show a time-series data obtained by solving the ODE system, as opposed to a sample data drawn from any distribution, as is traditionally the case.
In the following Tables 2 and 3, we use some parameters from the literature and we hypothesized some parameter for cutaneous leishmaniasis and Chagas disease in the tropical and subtropical zones.
The precision of the results in mathematical and computational models in eco-epidemiological systems depend on the certainty of the parameters. There are situations where some parameters are subjected to uncertainty due to the absence of a complete information about their source and also due to the lack of laboratory and field experiments. Although, the forty-two parameters in Figure 6 and Figure 7 have biological interpretations for cutaneous leishmaniasis and Chagas disease, they either known imprecisely or vary significantly from region to region. Thus, parameter space sampling is necessary for global sensitivity analysis [2,39,55]. Then, it is essential to identify how the parameters of the model may vary over realistic ranges. Therefore, to achieve the global sensitivity analysis for R0, the Partial Rank Correlation Coefficient (PRCC) is performed, which is a sensitivity analysis method that calculates the partial rank correlation coefficient for the model inputs and outputs. PRCC is performed computationally by sampling parameters from a uniformly distributed range using Latin Hypercube Sampling (LHS), an statistical sampling method that evaluates sensitivity of an outcome variable to all its input variables. PRCC is a robust sensitivity measure for nonlinear but monotonic relationships between the output and input parameters. The calculated PRCC values are between −1 and 1 and they are comparable among different model inputs. Uncertainty and global sensitivity analysis techniques help to determine and control these uncertainties. We use Latin Hypercube Sampling (LHS) and Partial Rank Correlation Coefficient (PRCC) to rank the influence of the 42 input parameters to R0 in cutaneous leishmaniasis which is linked to the model in Figure 1. The forty-two parameters were computed by sampling them for 100000 times.
The most significant PRCC-ranked parameters greater than 0.4 for cutaneous leishmaniasis disease (Figure 6) are the natural mortality rate of sandflies (μv), biting rate from vector to humans, and the sandfly population (N0v). Then we present the intermediate significant parameters between 0.2 and 0.4, which are the human population (Nh), the extrinsic incubation in sandflies (σv), the pathogen transmission probability from sand flies that amplifies the disease to humans (βavh), the biting rate from sand flies to domestic reservoir (bd), the pathogen transmission probability from sandflies that dilute the disease to humans (βdvh), and the fraction of recovery rate from infectious human (f).
Therefore, this study in cutaneous leishmaniasis suggests the effective disease control based on minimizing the contact that domestic reservoir and human populations have with sandfly vectors by decreasing the total sandfly population (N0v), such as spreading insecticide to homes and trees close to homes. Fumigation and spraying repellent are suggested to avoid the sand flies feeding. We use Table 2 for the global sensitivity analysis of R0. The PRCC results for cutaneous leishmaniasis are shown in Figure 6.
The most significant PRCC-ranked parameters greater than 0.4 for Chagas disease (Figure 7) are the natural mortality rate of triatomine bugs (μv), the biting rate from triatomine bugs to humans, and the triatomine bug population (N0v). Then we present the intermediate significant parameters between 0.2 and 0.4, which are the extrinsic incubation in triatomine bugs (σv), the pathogen transmission probability from triatomine bugs that amplifies the disease to humans(βavh), the human population (Nh), the fraction of recovery rate from infectious human (f), the biting rate from triatomine bugs to domestic reservoir (bd), the loss immunity rate from humans, and the pathogen transmission probability from triatomine bugs that dilute the disease to humans (βdvh).
Therefore, this study suggests the effective disease control based on minimizing the contact that domestic reservoir and human populations have with triatomine bugs vectors by decreasing the total triatomine bug population (N0v) such as spreading insecticide to homes and trees close to homes. Fumigation and spraying repellent are suggested to avoid the bugs biting. Moreover, it will be advisable for veterinarian health to consider control strategies in domestic reservoirs to avoid the higher ability to transmit the pathogen from reservoirs to vectors. We use Table 3 for the global sensitivity analysis of R0. The PRCC results for Chagas disease are shown in Figure 7.
In this work, we build a general mathematical model to help determine the combined effects of host competence and vector feeding preference. We applied this model to cutaneous leishmaniasis and Chagas disease. Based on this general model, we conclude for cutaneous leishmaniasis that when the domestic host competence (cd) decreases, the feeding preference by the vector that amplifies the disease on the domestic host (αavdr) and the reproduction number R0 increase. Additionally, the feeding preference by the vector that dilutes the disease on the domestic host (αdvdr) increases while R0 and the domestic host competency decreases (cd). R0 decreases while the feeding preference by vector that amplifies the disease on human (αavh) and the human competency (ch) increases. The feeding preference by vector that dilutes the disease on wild host (αdvwr) and wild host competency (cw) increases while R0 decreases. Moreover, the proportion of infectious vector increases when the domestic host competency (cd) increases (Figure 3a). According to Figure 3b, the proportion of infectious vector increases when the human competence (ch) increases. The proportion of infectious vector population decreases when the feeding preference by vectors that dilutes the disease on humans (αdvh) increases (Figure 3c). The proportion of infectious vectors decreases when the feeding preference by vectors that amplify the disease to humans (αavh) increases.
We come up to Chagas disease in Figure 4 and Figure 5 emphasizing that R0 decreases while the feeding preference by vector that amplifies the disease on domestic reservoir (αavdr) and the domestic host competency increases (cd). The feeding preference by vector that dilutes the disease on domestic reservoir (αdvdr) and the domestic host competency increases (cd) while R0 decreases. The feeding preference by vector that amplifies on human (αavh) and R0 increases while the human competency (ch) decreases. R0 decreases while the wild host competency increases (cw) as well as the feeding preference by vector that dilutes the disease on wild reservoir (αdvwr). In addition to these, the proportion of infectious vector increases as well as the domestic host competency increases (cd). The proportion of infectious vector increases as well as the feeding preference by vector that amplifies the disease to humans (αavh). The proportion of infectious vectors increases as well as (ch). The proportion of infectious vector decreases while the feeding preference by vector that dilutes the disease on human (αdvh) increases.
Due to the lack of data on vector-feeding preferences and host competencies, researchers should focus on laboratory and field experiments to quantify the precise vector-feeding preference value and the host competence value to obtain more specific predictions. Obtaining more specific predictions on multi-host vector systems would contribute to more informed decisions regarding public health policy and intervention. It is important to note that the dynamics of infection can differ between host species. Subsequently, these differing dynamics can affect overall disease prevalence and pose a high risk of infection to hosts with vector-borne diseases. Due to the scarcity of experimental data, we use the example of cutaneous leishmaniasis and Chagas disease to hypothesize the values for vector feeding preference, human competence, domestic host competence, and wild host competency [16,56,57]. Collectively, the establishment of the mathematical model (Section 2), the calculation of R0 (Section 3) and the numerical simulations (Section 4) help us to understand the relationship between the vector’s feeding preference and host competencies in different settings. The global sensitivity analyses show that the best solution and the most effective way to reduce R0 and avoid an epidemic is to reduce sandfly and triatomine bug populations, and control the biting rates. According to [58], mathematical models are useful tools in understanding eco-epidemiological phenomena and informing strategies to prevent, control or eliminate disease. Understanding the factors that affect the vector-feeding preference for specific host and host competencies are relevant for estimating transmission risks and predicting the effects of control tactics targeting multiple hosts. Key questions related to the identification of infective reservoirs, host competencies, host feeding preference by vectors and ecological mechanisms that affects human health in a domestic and wild environment have been described here in order to bridge knowledge gaps in public health, veterinary, and ecological health.
The authors would like to thank the Bill and Melinda Gates Foundation, the Neglected Tropical Disease consortium, and anonymous reviewers.
The authors declare no conflicts of interest.
[1] | L. Atzori, A. Iera, G. Morabito, The internet of things: A survey, Comput. Netw., 54(2010), 2787–2805. |
[2] | C. Chang and C. Li, Algebraic secret sharing using privacy homomorphisms for IoT-based healthcare systems, Math. Biosci. Eng., 16(2019), 3367–3381. |
[3] | C. Stergiou, K. E. Psannis, A. P. Plageras, et al., Algorithms for efficient digital media transmission over IoT and cloud networking, J. Multi. Inf. Syst., 5(2018), 27–34. |
[4] | F. X. Yu, I-Japan strategy 2015, Zhong guo Xinxihua, 13(2014), 13–23. |
[5] | F. Gao, Z. Y. Zhao, K. X. Zhao, et al., Interpretation of the medium-term and long-term strategy of Korean ICT R&D (2013–2017), Sci. Tech. Mgt. Res., 6(2015), 30–34. |
[6] | W. Ejaz, M. Naeem, A. Shahid, et al., Efficient energy management for the internet of thins in smart cities, IEEE Commun. Mag., 55(2017), 84–91. |
[7] | C. M. Medaglia and A. Serbanati, An overview of privacy and security issues in the Internet of Things, Proceedings of the 20th Tyrrhenian Workshop on Digital Communications (Italy), Springer, (2010), 389–394. |
[8] | Y. L. Liu, P. Hua and J. Wang, Verifiable diversity ranking search over encrypted outsourced data, CMC-Comput. Mater. Con., 55(2018), 037–057. |
[9] | J. Wang, C. W. Ju, Y. Gao, et al., A PSO based energy efficient coverage control algorithm for wireless sensor networks, CMC-Comput. Mater. Con., 56(2018), 433–446. |
[10] | Z. W. Li and Y. Xie, A security query protocol of ONS in EPC system, 2012 International Conference on Anti-Counterfeiting, Security and Identification (Taipei), IEEE, (2012), Article number: 6325293. |
[11] | L. J. Zhang and Z. Q. Wu, A controllable trusted and anonymous query mechanism of Internet of Things, Comput. Technol. Develop., 23(2013), 122–125. |
[12] | C. Fachkha, H. B. Elias and M. Debbabi, Fingerprinting internet DNS amplification DDoS Activities, 2014 6th International Conference on New Technologies, Mobility and Security ( Dubai), OALib J., (2014), article number: 6814019. |
[13] | A. Friedlander, A. Mankin, W. D. Maughan, et al., DNSSEC: A protocol toward securing the Internet infrastructure, Commu. ACM, 50(2007), 44–50. |
[14] | M. Dempsky, DNSCurve: Link-Level Security for the Domain Name System, Int. Draft, 2004. |
[15] | Z. Wu, Y. Zhou and J. Ma, A security transmission model for Internet of Things, Chinese J. Comput., 34(2011), 1351–1364. |
[16] | W. Ren, L. Ma and Y. Ren, APP: An Ultralightweight scheme to authenticate ONS and protect epc privacy without cryptography in EPCglobal networks, Int J. Distrib. Sens. N., (2013), Article ID: 784618. |
[17] | P. Danielis, V. Altmann, J. Skodzik, et al., P-DONAS: A P2P-based domain name system in access networks, ACM T. Int. Techn., 15(2015), 1–11. |
[18] | E. Zhang, X. T. Duan, S. M. Xiu, et al., Server-aided multi-secret sharing scheme for weak computational devices, CMC-Comput. Mater. Con., 56(2018), 401–414. |
[19] | R. Georg, Probalilistic models for some intelligence and attainment tests, Copenhagen: Institute of Education Research, 1960. |
1. | A. Habchi, B. Hartiti, H. Labrim, S. Fadili, A. Benyoussef, N. Belouaggadia, A. Faddouli, M. Benaissa, H. EZ-Zahraouy, Performance study of a new hybrid parabolic trough collector system integrated with hybrid tubular thermoelectric generator, 2021, 13594311, 116656, 10.1016/j.applthermaleng.2021.116656 | |
2. | Mokhtar Mohammed, M. Taha Janan, Exergetic improvement potential analysis of a new design of concentration based solar distiller, 2022, 43, 0143-0750, 8936, 10.1080/01430750.2022.2120072 | |
3. | Saad Eddin Lachhab, A. Bliya, H. Diyagh, H. En-nkhili, H. Shindou, S. Ouhssain, H. Slimane, E. Al Ibrahmi, L. Dlimi, Comparative evaluation of SnO2/CdS/CuBi2O4 structure performance based on SnO2 window layer numerical and experimental analysis, 2022, 99, 00194522, 100699, 10.1016/j.jics.2022.100699 | |
4. | Mokhtar Mohammed, Taha Janan Mourad, Development of Solar Desalination Units Using Solar Concentrators or/and Internal Reflectors, 2021, 12, 2226-809X, 45, 10.46604/ijeti.2021.8304 | |
5. | Irina Meghea, Comparison of Statistical Production Models for a Solar and a Wind Power Plant, 2023, 11, 2227-7390, 1115, 10.3390/math11051115 | |
6. | Sanjay N Havaldar, Harsh V Malapur, Kaustubh G Kulkarni, Gary A. Anderson, Numerical Investigation of Concentrated Solar Central Billboard with Hexagonal Tubes., 2022, 1084, 1755-1307, 012021, 10.1088/1755-1315/1084/1/012021 | |
7. | A. Bliya, Saad Eddin Lachhab, E. Al Ibrahmi, Improving the semiconductor performance of SnO2 / CdS / CuBi2O4 by optimizing the optical properties of the CuBi2O4 absorbent layer, 2023, 183, 27730123, 207667, 10.1016/j.micrna.2023.207667 | |
8. | Rowida Meligy, Alaric Montenon, Hadeer A. Hassan, Comparative analysis of machine learning models of linear Fresnel solar collector, 2024, 230, 09601481, 120865, 10.1016/j.renene.2024.120865 | |
9. | Ádám Nagy, István Bodnár, Endre Kovács, Simulation of the Thermal Behavior of a Photovoltaic Solar Panel Using Recent Explicit Numerical Methods, 2024, 7, 2513-0390, 10.1002/adts.202400089 |
Parameter | Definition |
αavh | feeding preference by vector that amplifies the disease on humans |
αdvh | feeding preference by vector that dilutes the disease on humans |
αavdr | feeding preference by vector that amplifies the disease on domestic reservoir |
αdvdr | feeding preference by vector that dilutes the disease on domestic reservoir |
αavwr | feeding preference by vector that amplifies the disease on wild reservoir |
αdvwr | feeding preference by vector that dilutes the disease on wild reservoir |
βavh | pathogen transmission probability from vector that amplifies the disease to humans |
βdvh | pathogen transmission probability from vector that dilutes the disease to humans |
βavdr | pathogen transmission probability from vector that amplifies the disease to domestic reservoir |
βdvdr | pathogen transmission probability from vector that dilutes the disease to domestic reservoir |
βavwr | pathogen transmission probability from vector that amplifies the disease to wild reservoir |
βdvwr | pathogen transmission probability from vector that dilutes the disease to wild reservoir |
bh | biting rate from vector to humans |
bd | biting rate from vector to domestic reservoir |
bw | biting rate from vector to wild reservoir |
fh | fraction of successful biting from vector that amplifies the disease to humans |
1−fh | fraction of successful biting from vector that dilutes the disease to humans |
fd | fraction of successful biting from vector that amplifies the disease to domestic reservoir |
1−fd | fraction of successful biting from vector that dilutes the disease to domestic reservoir |
fw | fraction of successful biting from vector that amplifies the disease to domestic reservoir |
1−fw | fraction of successful biting from vector that dilutes the disease to wild reservoir |
f | fraction of recovery rate from infectious human |
η | fraction of recovery rate by treatment |
a | fraction of infected humans transmitting the pathogen |
ωh | loss immunity rate from humans |
ωd | loss immunity rate from domestic reservoir |
ωw | loss immunity rate from wild reservoir |
μh | human mortality rate |
μd | domestic reservoir mortality rate |
μw | wild reservoir mortality rate |
μv | vector mortality rate |
σh | intrinsic incubation period in humans |
σv | extrinsic incubation period in vectors |
δh | Disease induced death rate in humans |
δd | Disease induced death rate in domestic reservoirs |
δw | Disease induced death rate in wild reservoirs |
rh | recovery rate for infectious humans receiving treatment |
θh | treatment rate |
ch | human ability to transmit the pathogen to vector |
cd | domestic reservoir ability to transmit the pathogen to vector |
cw | wild reservoir ability to transmit the pathogen to vector |
Parameter | Value | Range | Reference |
αavh | 3.0 | [0–40.0] | hypothetical |
αdvh | 2.0 | [0–40.0] | hypothetical |
αavdr | 4.0 | [0–40.0] | hypothetical |
αdvdr | 2.0 | [0–40.0] | hypothetical |
αavwr | 5.0 | [0–40.0] | hypothetical |
αdvwr | 2.0 | [0–40.0] | hypothetical |
βavh | 0.7198 | [0.01–0.9] | [39] [40] |
βdvh | 0.0521 | [0.01–0.9] | hypothetical |
βavdr | 0.850 | [0.01–0.9] | hypothetical |
βdvdr | 0.097 | [0.01–0.9] | hypothetical |
βavwr | 0.7 | [0.01–0.9] | hypothetical |
βdvwr | 0.07 | [0.01–0.9] | hypothetical |
bh | 0.6878 | [0.025–2.0] | [39], [40] |
bd | 0.3 | [0.025–2.0] | [40] |
bw | 0.08 | [0.025–2.0] | [40] |
fh | 0.6 | [0.0001–1.0] | hypothetical |
fd | 0.8 | [0.0001–1.0] | hypothetical |
fw | 0.4 | [0.0001–1.0] | hypothetical |
f | 0.005 | [0.001–0.9] | hypothetical |
η | 0.65 | [0.07–0.8] | hypothetical |
a | 0.5 | [0.007–0.8] | hypothetical |
ωh | 0.0013 | [0.000004–0.04] | [41], [49] |
ωd | 0.003 | [0.00003–0.03] | [50] |
ωw | 0.0007 | [0.00003–0.002] | [46] |
μh | 0.000000413 | [0.0000003, 0.0003] | [39] |
μd | 0.0000821 | [0.00001, 0.009] | [46] |
μw | 0.021 | [0.0002–0.09] | [46] |
μv | 0.0135 | [0.002–0.9] | [41] |
σh | 0.0158 | [0.00013–0.5] | [41], [49] |
σv | 0.035 | [0.003–0.7] | [41] |
δh | 0.0000008 | [0.0000001–0.009] | [39] |
δd | 0.0008 | [0.00005–0.05] | hypothetical |
δw | 0.00008 | [0.00005–0.03] | hypothetical |
rh | 0.091 | [0.00001–0.9] | hypothetical |
θh | 0.035 | [0.0002–0.7] | [51] |
ch | 0.4 | [0.00001–1.0] | hypothetical |
cd | 0.3 | [0.00001–1.0] | hypothetical |
cw | 0.1 | [0.00001–1.0] | hypothetical |
N0h | 100,050 | [100.0–1000000.0] | hypothetical |
N0d | 100,050 | [100.0–200000.0] | hypothetical |
N0w | 50,050 | [100.0–100000.0] | hypothetical |
N0v | 5'000,500 | [1000.0–10000000.0] | hypothetical |
Parameter | Value | Range | Reference |
αavh | 6.0 | [0–40.0] | hypothetical |
αdvh | 4.0 | [0–40.0] | hypothetical |
αavdr | 7.0 | [0–40.0] | hypothetical |
αdvdr | 3.0 | [0–40.0] | hypothetical |
αavwr | 5.0 | [0–40.0] | hypothetical |
αdvwr | 2.0 | [0–40.0] | hypothetical |
βavh | 0.49 | [0.001–0.9] | [14], [52] |
βdvh | 0.02 | [0.001–0.9] | [7] |
βavdr | 0.5 | [0.001–0.9] | hypothetical |
βdvdr | 0.09 | [0.001–0.9] | hypothetical |
βavwr | 0.4 | [0.001–0.9] | [47] |
βdvwr | 0.05 | [0.001–0.9] | [48] |
bh | 0.9 | [0.0025–3.0] | [6] |
bd | 0.3 | [0.0025–3.0] | hypothetical |
bw | 0.1 | [0.0025–3.0] | hypothetical |
fh | 0.6 | [0.0001–1.0] | hypothetical |
fd | 0.8 | [0.0001–1.0] | hypothetical |
fw | 0.4 | [0.0001–1.0] | hypothetical |
f | 0.7 | [0.001–0.9] | hypothetical |
η | 0.02 | [0.007–0.8] | hypothetical |
a | 0.5 | [0.007–0.8] | hypothetical |
ωh | 0.0013 | [0.000004–0.04] | hypothetical |
ωd | 0.003 | [0.00003–0.03] | hypothetical |
ωw | 0.0007 | [0.00003–0.002] | hypothetical |
μh | 0.000000413 | [0.0000003, 0.0003] | [39] |
μd | 0.001 | [0.00001, 0.09] | [52] |
μw | 0.00021 | [0.0001–0.09] | [46] |
μv | 0.00327 | [0.001–0.9] | [53] |
σh | 0.1 | [0.00013–0.9] | [54] |
σv | 0.035 | [0.003–0.9] | [41] |
δh | 0.000057 | [0.0000001–0.0009] | [6] |
δd | 0.0005 | [0.00005–0.05] | hypothetical |
δw | 0.0003 | [0.00005–0.03] | hypothetical |
rh | 0.03 | [0.00001–0.9] | hypothetical |
θh | 0.016 | [0.0002–0.7] | [44] |
ch | 0.9 | [0.00001–1.0] | hypothetical |
cd | 0.3 | [0.00001–1.0] | hypothetical |
cw | 0.7 | [0.00001–1.0] | hypothetical |
N0h | 500,050 | [100.0–1000000.0] | hypothetical |
N0d | 100,050 | [100.0–200000.0] | hypothetical |
N0w | 50,050 | [100.0–100000.0] | hypothetical |
N0v | 5'000,500 | [1000.0–10000000.0] | hypothetical |
Parameter | Definition |
αavh | feeding preference by vector that amplifies the disease on humans |
αdvh | feeding preference by vector that dilutes the disease on humans |
αavdr | feeding preference by vector that amplifies the disease on domestic reservoir |
αdvdr | feeding preference by vector that dilutes the disease on domestic reservoir |
αavwr | feeding preference by vector that amplifies the disease on wild reservoir |
αdvwr | feeding preference by vector that dilutes the disease on wild reservoir |
βavh | pathogen transmission probability from vector that amplifies the disease to humans |
βdvh | pathogen transmission probability from vector that dilutes the disease to humans |
βavdr | pathogen transmission probability from vector that amplifies the disease to domestic reservoir |
βdvdr | pathogen transmission probability from vector that dilutes the disease to domestic reservoir |
βavwr | pathogen transmission probability from vector that amplifies the disease to wild reservoir |
βdvwr | pathogen transmission probability from vector that dilutes the disease to wild reservoir |
bh | biting rate from vector to humans |
bd | biting rate from vector to domestic reservoir |
bw | biting rate from vector to wild reservoir |
fh | fraction of successful biting from vector that amplifies the disease to humans |
1−fh | fraction of successful biting from vector that dilutes the disease to humans |
fd | fraction of successful biting from vector that amplifies the disease to domestic reservoir |
1−fd | fraction of successful biting from vector that dilutes the disease to domestic reservoir |
fw | fraction of successful biting from vector that amplifies the disease to domestic reservoir |
1−fw | fraction of successful biting from vector that dilutes the disease to wild reservoir |
f | fraction of recovery rate from infectious human |
η | fraction of recovery rate by treatment |
a | fraction of infected humans transmitting the pathogen |
ωh | loss immunity rate from humans |
ωd | loss immunity rate from domestic reservoir |
ωw | loss immunity rate from wild reservoir |
μh | human mortality rate |
μd | domestic reservoir mortality rate |
μw | wild reservoir mortality rate |
μv | vector mortality rate |
σh | intrinsic incubation period in humans |
σv | extrinsic incubation period in vectors |
δh | Disease induced death rate in humans |
δd | Disease induced death rate in domestic reservoirs |
δw | Disease induced death rate in wild reservoirs |
rh | recovery rate for infectious humans receiving treatment |
θh | treatment rate |
ch | human ability to transmit the pathogen to vector |
cd | domestic reservoir ability to transmit the pathogen to vector |
cw | wild reservoir ability to transmit the pathogen to vector |
Parameter | Value | Range | Reference |
αavh | 3.0 | [0–40.0] | hypothetical |
αdvh | 2.0 | [0–40.0] | hypothetical |
αavdr | 4.0 | [0–40.0] | hypothetical |
αdvdr | 2.0 | [0–40.0] | hypothetical |
αavwr | 5.0 | [0–40.0] | hypothetical |
αdvwr | 2.0 | [0–40.0] | hypothetical |
βavh | 0.7198 | [0.01–0.9] | [39] [40] |
βdvh | 0.0521 | [0.01–0.9] | hypothetical |
βavdr | 0.850 | [0.01–0.9] | hypothetical |
βdvdr | 0.097 | [0.01–0.9] | hypothetical |
βavwr | 0.7 | [0.01–0.9] | hypothetical |
βdvwr | 0.07 | [0.01–0.9] | hypothetical |
bh | 0.6878 | [0.025–2.0] | [39], [40] |
bd | 0.3 | [0.025–2.0] | [40] |
bw | 0.08 | [0.025–2.0] | [40] |
fh | 0.6 | [0.0001–1.0] | hypothetical |
fd | 0.8 | [0.0001–1.0] | hypothetical |
fw | 0.4 | [0.0001–1.0] | hypothetical |
f | 0.005 | [0.001–0.9] | hypothetical |
η | 0.65 | [0.07–0.8] | hypothetical |
a | 0.5 | [0.007–0.8] | hypothetical |
ωh | 0.0013 | [0.000004–0.04] | [41], [49] |
ωd | 0.003 | [0.00003–0.03] | [50] |
ωw | 0.0007 | [0.00003–0.002] | [46] |
μh | 0.000000413 | [0.0000003, 0.0003] | [39] |
μd | 0.0000821 | [0.00001, 0.009] | [46] |
μw | 0.021 | [0.0002–0.09] | [46] |
μv | 0.0135 | [0.002–0.9] | [41] |
σh | 0.0158 | [0.00013–0.5] | [41], [49] |
σv | 0.035 | [0.003–0.7] | [41] |
δh | 0.0000008 | [0.0000001–0.009] | [39] |
δd | 0.0008 | [0.00005–0.05] | hypothetical |
δw | 0.00008 | [0.00005–0.03] | hypothetical |
rh | 0.091 | [0.00001–0.9] | hypothetical |
θh | 0.035 | [0.0002–0.7] | [51] |
ch | 0.4 | [0.00001–1.0] | hypothetical |
cd | 0.3 | [0.00001–1.0] | hypothetical |
cw | 0.1 | [0.00001–1.0] | hypothetical |
N0h | 100,050 | [100.0–1000000.0] | hypothetical |
N0d | 100,050 | [100.0–200000.0] | hypothetical |
N0w | 50,050 | [100.0–100000.0] | hypothetical |
N0v | 5'000,500 | [1000.0–10000000.0] | hypothetical |
Parameter | Value | Range | Reference |
αavh | 6.0 | [0–40.0] | hypothetical |
αdvh | 4.0 | [0–40.0] | hypothetical |
αavdr | 7.0 | [0–40.0] | hypothetical |
αdvdr | 3.0 | [0–40.0] | hypothetical |
αavwr | 5.0 | [0–40.0] | hypothetical |
αdvwr | 2.0 | [0–40.0] | hypothetical |
βavh | 0.49 | [0.001–0.9] | [14], [52] |
βdvh | 0.02 | [0.001–0.9] | [7] |
βavdr | 0.5 | [0.001–0.9] | hypothetical |
βdvdr | 0.09 | [0.001–0.9] | hypothetical |
βavwr | 0.4 | [0.001–0.9] | [47] |
βdvwr | 0.05 | [0.001–0.9] | [48] |
bh | 0.9 | [0.0025–3.0] | [6] |
bd | 0.3 | [0.0025–3.0] | hypothetical |
bw | 0.1 | [0.0025–3.0] | hypothetical |
fh | 0.6 | [0.0001–1.0] | hypothetical |
fd | 0.8 | [0.0001–1.0] | hypothetical |
fw | 0.4 | [0.0001–1.0] | hypothetical |
f | 0.7 | [0.001–0.9] | hypothetical |
η | 0.02 | [0.007–0.8] | hypothetical |
a | 0.5 | [0.007–0.8] | hypothetical |
ωh | 0.0013 | [0.000004–0.04] | hypothetical |
ωd | 0.003 | [0.00003–0.03] | hypothetical |
ωw | 0.0007 | [0.00003–0.002] | hypothetical |
μh | 0.000000413 | [0.0000003, 0.0003] | [39] |
μd | 0.001 | [0.00001, 0.09] | [52] |
μw | 0.00021 | [0.0001–0.09] | [46] |
μv | 0.00327 | [0.001–0.9] | [53] |
σh | 0.1 | [0.00013–0.9] | [54] |
σv | 0.035 | [0.003–0.9] | [41] |
δh | 0.000057 | [0.0000001–0.0009] | [6] |
δd | 0.0005 | [0.00005–0.05] | hypothetical |
δw | 0.0003 | [0.00005–0.03] | hypothetical |
rh | 0.03 | [0.00001–0.9] | hypothetical |
θh | 0.016 | [0.0002–0.7] | [44] |
ch | 0.9 | [0.00001–1.0] | hypothetical |
cd | 0.3 | [0.00001–1.0] | hypothetical |
cw | 0.7 | [0.00001–1.0] | hypothetical |
N0h | 500,050 | [100.0–1000000.0] | hypothetical |
N0d | 100,050 | [100.0–200000.0] | hypothetical |
N0w | 50,050 | [100.0–100000.0] | hypothetical |
N0v | 5'000,500 | [1000.0–10000000.0] | hypothetical |