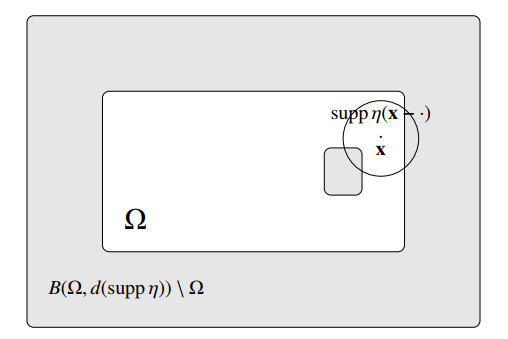
Citation: Raimund Bürger, Paola Goatin, Daniel Inzunza, Luis Miguel Villada. A non-local pedestrian flow model accounting for anisotropic interactions and domain boundaries[J]. Mathematical Biosciences and Engineering, 2020, 17(5): 5883-5906. doi: 10.3934/mbe.2020314
[1] | Paola Goatin, Matthias Mimault . A mixed system modeling two-directional pedestrian flows. Mathematical Biosciences and Engineering, 2015, 12(2): 375-392. doi: 10.3934/mbe.2015.12.375 |
[2] | Ziqiang Cheng, Jin Wang . Modeling epidemic flow with fluid dynamics. Mathematical Biosciences and Engineering, 2022, 19(8): 8334-8360. doi: 10.3934/mbe.2022388 |
[3] | Sebastien Motsch, Mehdi Moussaïd, Elsa G. Guillot, Mathieu Moreau, Julien Pettré, Guy Theraulaz, Cécile Appert-Rolland, Pierre Degond . Modeling crowd dynamics through coarse-grained data analysis. Mathematical Biosciences and Engineering, 2018, 15(6): 1271-1290. doi: 10.3934/mbe.2018059 |
[4] | Radu C. Cascaval, Ciro D'Apice, Maria Pia D'Arienzo, Rosanna Manzo . Flow optimization in vascular networks. Mathematical Biosciences and Engineering, 2017, 14(3): 607-624. doi: 10.3934/mbe.2017035 |
[5] | Jimin Yu, Jiajun Yin, Shangbo Zhou, Saiao Huang, Xianzhong Xie . An image super-resolution reconstruction model based on fractional-order anisotropic diffusion equation. Mathematical Biosciences and Engineering, 2021, 18(5): 6581-6607. doi: 10.3934/mbe.2021326 |
[6] | Azmy S. Ackleh, Rainey Lyons, Nicolas Saintier . Finite difference schemes for a structured population model in the space of measures. Mathematical Biosciences and Engineering, 2020, 17(1): 747-775. doi: 10.3934/mbe.2020039 |
[7] | Abdulhamed Alsisi, Raluca Eftimie, Dumitru Trucu . Non-local multiscale approach for the impact of go or grow hypothesis on tumour-viruses interactions. Mathematical Biosciences and Engineering, 2021, 18(5): 5252-5284. doi: 10.3934/mbe.2021267 |
[8] | Zhangli Peng, Andrew Resnick, Y.-N. Young . Primary cilium: a paradigm for integrating mathematical modeling with experiments and numerical simulations in mechanobiology. Mathematical Biosciences and Engineering, 2021, 18(2): 1215-1237. doi: 10.3934/mbe.2021066 |
[9] | O. E. Adebayo, S. Urcun, G. Rolin, S. P. A. Bordas, D. Trucu, R. Eftimie . Mathematical investigation of normal and abnormal wound healing dynamics: local and non-local models. Mathematical Biosciences and Engineering, 2023, 20(9): 17446-17498. doi: 10.3934/mbe.2023776 |
[10] | Boris Andreianov, Carlotta Donadello, Ulrich Razafison, Massimiliano D. Rosini . Riemann problems with non--local point constraints and capacity drop. Mathematical Biosciences and Engineering, 2015, 12(2): 259-278. doi: 10.3934/mbe.2015.12.259 |
This paper contributes to the macroscopic modelling of crowd movements. We consider the following initial-boundary value problem for a non-local scalar conservation law that describes the evolution of the local density ρ of pedestrians as a function of time t and position x=(x1,x2) on a crowd evolution domain Ω⊂R2:
{∂tρ+div (ρV(ρ)ν(x,I[ρ(t)](x)))=0,x∈Ω,t≥0,ρ(0,x)=ρ0(x),x∈Ω,ρ(t,x)=0,x∈∂Ω. | (1.1) |
Here ν=(ν1,ν2) is a vector field that (with slight abuse of notation) is defined as
ν(t,x):=ν(x,I[ρ(t)](x))=μ(x)+I[ρ(t)](x), | (1.2) |
where μ is the (normalized) fixed smooth vector field of preferred directions (e.g., given by the regularized solution of an eikonal equation), and I[ρ(t)] is a non-local correction term that depends on the current density distribution. This notation indicates a functional dependence, i.e., I depends on the function ρ(t):=ρ(t,⋅) as a whole. The function V:R+→R represents the average speed of pedestrians as a function of their density.
We assume that pedestrians move in a space surrounded by walls, and that the vector field ν points inward along the boundary ∂Ω of Ω, that is
ν(t,x)⋅n(x)≤0for all x∈∂Ω, t≥0, | (1.3) |
where n is the outward normal to Ω. (Of course, we may assume μ(x)⋅n(x)≤0 for all x∈∂Ω, then it is enough to ensure that also I[ρ(t)](x)⋅n(x)≤0 for all x∈∂Ω.) In this case, the condition ρ(t,x)=0 on ∂Ω corresponds to a zero-flux condition. If, for simulation reasons, we need to consider smaller domains and to add adsorbing conditions on the part of the boundary not corresponding to walls (and where the vector field points outwards), suitable modifications of the model are needed.
If supp(ρ0)⊂Ω and ν(t,x)⋅n(x)≤0 for all x∈∂Ω and t>0, then problem (1.1) is equivalent to the Cauchy problem
{∂tρ+div (ρV(ρ)ν(x,I[ρ(t)](x)))=0,x∈R2,t≥0,ρ(0,x)=ρ0(x),x∈R2. | (1.4) |
Following Colombo et al. [1], we consider a non-local interaction term of the form
I[ρ(t)](x)=−ε∇(η∗wρ(t))(x)√1+‖∇(η∗wρ(t))(x)‖2,0<ε<1, | (1.5) |
where η is a smooth non-negative kernel with compact support such that ∬R2η(x)dx=1 and ε is a model parameter modulating the magnitude of the interaction term. The term (1.5) models how pedestrians account for other pedestrian distribution close to them to correct their path. To better account for the reaction of pedestrians to densities ahead of them, one may consider anisotropic kernels η, see e.g., [1] and [2,Appendix D]. To account for the presence of boundaries, and walls (or obstacles) into boundaries, unlike [3,4], we modify the usual convolution product as follows:
(η∗wρ(t))(x)=∬R2ρw(t,y)η(x−y)dy, | (1.6) |
where ρw:R2→R+ is defined as
ρw(t,x):={ρ(t,x)if x∈Ω, Rwif x∈B(Ω,d(suppη))∖Ω,0elsewhere, | (1.7) |
with Rw≥R big enough so that (1.3) holds. Here we denote by
d(A)=sup{|x−y|:x,y∈A} |
the diameter of a set A⊂R2 and by
B(Ω,ℓ)={x∈R2:infy∈Ω|x−y|≤ℓ} |
the "ball" of radius ℓ around Ω. Furthermore, we define
M:=∬R2χB(Ω,d(suppη))(y)dy, | (1.8) |
which is finite if Ω is bounded (χ denotes the characteristic function). See Figure 1.
The presence of high density values at the wall and obstacle locations included in (1.6) and (1.7) is intended to mimic their effect on the pedestrian dynamics. Indeed, in this way the non-local correction term (1.5) "sees" the presence of the wall and deviates the movement from the desired trajectory, thus acting as a discomfort term expressing the tendency of pedestrians to stay away from obstacles.
Remark 1. An explicit condition ensuring ∇(η∗wρ(t))(x)⋅n(x)≥0 for all x∈∂Ω (and thus ν(t,x)⋅n(x)≤0) is the following:
Rw∬Ωc∇η(x−y)⋅n(x)dy≥R∬Ω(∇η(x−y)⋅n(x))−dyfor all x∈∂Ω, |
where f(x)−:=max{−f(x),0}=−min{f(x),0} denotes the negative part of a function f and Ωc:=R2∖Ω is the complement of Ω. Indeed we have
∇(η∗wρ(t))(x)⋅n(x)=n(x)⋅∬R2ρ(t,y)∇η(x−y)dy=∬R2ρ(t,y)∇η(x−y)⋅n(x)dy=∬ΩcRw∇η(x−y)⋅n(x)dy+∬Ωρ(t,y)∇η(x−y)⋅n(x)dy≥Rw∬Ωc∇η(x−y)⋅n(x)dy−R∬Ω(∇η(x−y)⋅n(x))−dy. |
Macroscopic models of (vehicular and pedestrian) traffic flow are based on balance laws describing the spatio-temporal evolution of averaged quantities such as density and mean velocity. In analogy to vehicular traffic models, macroscopic crowd motion models were introduced starting from the beginning of this century based on scalar conservation laws [5,6], gas dynamics equations [7,8], gradient flow methods [9,10] and time evolving measures [11].
More recently, models consisting in non-local conservation laws in two-space dimension were proposed by different authors [1,3,4,12,13,14,15,16]. Conservation laws with non-local flux functions arise in a large variety of applications, such as traffic flow [17,18,19], sedimentation [20,21], and material flows on conveyor belts [22]. In particular, anisotropic kernels have been introduced in traffic models to account for downstream interactions, see e.g., [17,19]. Physically based derivations of vision-based interaction pedestrian dynamics can be found for example in [11,23,24,25]. We emphasize that none of the previously cited models (with the obvious exception of [1]) fits into the framework of the present approach, which is focused on the appropriate incorporation of boundary conditions and obstacles. That said, we are well aware of a vast body of literature on empirical and numerical studies of pedestrian flows in confined areas that call for the explicit incorporation of such boundaries, see for instance recent proceedings volumes of conferences on Pedestrian and Evacuation Dynamics [26,27,28]. (We refer in Section 4 to some specific contributions within these collections.)
The computation of numerical solutions for non-local models is challenging due to the high non-linearity of the system and the dependence of the flux function on the convolution product. In order to overcome the computational bottleneck, high order schemes have been developed for scalar equation [29] and systems [30,31] in one space dimension. For crowd dynamics models, first order finite volume approximations based on the Lax-Friedrichs scheme have been used in [1,3,4], aiming at demonstrating convergence properties for existence results. Here, we will consider the finite difference weighted essentially non-oscillatory (WENO) schemes developed in [32] to achieve high-resolution spatial accuracy. WENO schemes [33,34,35] are widely employed for the simulation of complex flow fields, their main advantage being their capability to achieve arbitrarily high order formal accuracy in smooth regions while maintaining stable, non-oscillatory and sharp discontinuity transitions.
The remainder of this paper is organized as follows. In Section 2, we deal with the well-posedness of problem (1.4), (1.5)–(1.7) under the hypothesis (1.3), so that (1.4), (1.5)–(1.7) is equivalent to (1.1), (1.5)–(1.7). Section 3 describes the WENO scheme used to compute approximate solutions and Section 4 collects three different numerical tests investigating the model characteristics. Conclusions and perspectives are elaborated in Section 5.
We summarize the assumptions concerning the domain Ω and the functions V, ν, μ and η:
(I1) The domain Ω⊆R2 is a non-empty bounded open set with smooth boundary ∂Ω, so that the outward normal n(x) is uniquely defined for all x∈∂Ω.
(I2) The speed function V∈C2(R;R) is non-increasing, V(0)=Vmax and V(R)=0 for some constants Vmax,R>0.
(I3) The vector field of preferred directions μ∈(C2∩W1,∞)(R2;R2) is defined such that divμ∈(W1,1∩W1,∞)(R2;R).
(I4) The vector field ν points inward along the boundary ∂Ω of Ω, i.e., ν(t,x)⋅n(x)≤0 for all x∈∂Ω, t≥0.
(I5) The kernel function η∈C3c(R2;R+) satisfies ∬R2η(x)dx=1.
Solutions of problem (1.4), (1.5)–(1.7), equivalently (1.1), (1.5)–(1.7), are intended in the following sense.
Definition 1. [1,Def. 2.1] For any T>0 and ρ0∈L1(R2,[0,R]) such that suppρ0⊂Ω, a function ρ∈C0([0,T],L1(R2;[0,R]) is said to be a weak entropy solution to (1.4), (1.5)–(1.7), if it is a Kružkov entropy solution to the Cauchy problem
{∂tρ+div (ρV(ρ)ν(t,x))=0in R2,ρ(0,x)=ρ0(x)in R2, | (2.1) |
i.e., for all κ∈R and all test functions ϕ∈C∞c(]−∞,T[×R2;R+) there holds
∫T0∫R2{|ρ−κ|∂tϕ+sgn(ρ−κ)(ρV(ρ)−κV(κ))ν(t,x)⋅∇ϕ}dxdt−∫T0∫R2κV(κ)div ν(t,x)sgn(ρ−κ)ϕdxdt+∫R2|ρ0(x)−κ|ϕ(0,x)dx≥0. | (2.2) |
Indeed, by mass conservation, we have that suppρ(t,⋅)⊂Ω for all t>0, and therefore ρ(t,x)=0 for a.e. x∈Ωc, see [1,Proposition 3.1]. Therefore, by abuse of notation, ρ∈L∞([0,T]×Ω;R) can be seen as a Kružkov semi-entropy solution in the sense of [37,Def. 1]: namely, from (2.2) we have
0≤∫T0∫Ω{(ρ−κ)±∂tϕ+sgn±(ρ−κ)(ρV(ρ)−κV(κ))ν(t,x)⋅∇ϕ}dxdt−∫T0∫ΩκV(κ)div ν(t,x)sgn±(ρ−κ)ϕdxdt+∫Ω(ρ0(x)−κ)±ϕ(0,x)dx≤∫T0∫Ω{(ρ−κ)±∂tϕ+sgn±(ρ−κ)(ρV(ρ)−κV(κ))ν(t,x)⋅∇ϕ}dxdt−∫T0∫ΩκV(κ)div ν(t,x)sgn±(ρ−κ)ϕdxdt+∫Ω(ρ0(x)−κ)±ϕ(0,x)dx+‖f′(ρ)ν‖L∞([0,T]×Ω×[0,R])∫T0∫∂Ω(−κ)±ϕ(t,x)dxdt, |
where f(ρ):=ρV(ρ), s+=max{s,0}, s−=max{−s,0}, sgn+(s)=sgn(s+) and sgn−(s)=−sgn(s−).
If ρ∈(L∞∩BV)([0,T]×Ω;R), then the classical definition introduced by Bardos, Le Roux and Nédélec [38] holds:
∫T0∫Ω{|ρ−κ|∂tϕ+sgn(ρ−κ)(ρV(ρ)−κV(κ))ν(t,x)⋅∇ϕ}dxdt−∫T0∫ΩκV(κ)div ν(t,x)sgn(ρ−κ)ϕdxdt+∫Ω|ρ0(x)−κ|ϕ(0,x)dx+∫T0∫∂Ωsgn(κ)(trρ(t,x)V(trρ(t,x))−κV(κ))ν(t,x)⋅n(x)ϕ(t,x)dxdt≥0, |
where trρ denotes the trace of ρ at the boundary ∂Ω.
We refer the reader to [36] for a discussion on the different notions of admissible solutions for scalar multi-dimensional initial-boundary value problems and their equivalence.
Under hypotheses (I1)–(I3), the non-local term I defined by (1.5)-(1.6)-(1.7) satisfies the following estimates for ε as in (1.5), Rw as in (1.7) and M as in (1.8):
‖I[ρ]‖L∞≤εRw‖∇η‖L1, | (2.3) |
‖I[ρ]‖L1≤εRwM‖∇η‖L1, | (2.4) |
‖div I[ρ]‖L∞≤εRw‖∇η‖W1,1(1+R2w‖∇η‖2L1), | (2.5) |
‖div I[ρ]‖L1≤εRwM‖∇η‖W1,1(1+R2w‖∇η‖2L1), | (2.6) |
‖∇div I[ρ]‖L1≤εRwM‖∇η‖W2,1(1+8R2w‖∇η‖L1‖∇η‖W1,1). | (2.7) |
Moreover, for any r1,r2∈L1(Ω;[0;R]) there hold
‖I[r1]−I[r2]‖L∞≤ε(1+R2w‖∇η‖2L1)‖∇η‖L∞‖r1−r2‖L1, | (2.8) |
‖I[r1]−I[r2]‖L1≤ε(1+R2w‖∇η‖2L1)‖∇η‖L1‖r1−r2‖L1, | (2.9) |
‖div(I[r1]−I[r2])‖L1≤ε‖r1−r2‖L1‖∇η‖W1,1(1+8R2w‖∇η‖2W1,1+3R4w‖∇η‖4W1,1), | (2.10) |
see [1,Proof of Lemma 3.1].
Recalling that f(ρ)=ρV(ρ) and following [1,Theorem 2.1], we have the following well-posedness result.
Theorem 1. Let (I1)–(I4) hold and ρ0∈(L1∩BV)(R2;[0,R]) with suppρ0⊂Ω. Then there exists a unique weak entropy solution ρ∈C0(R+;L1(R2;[0,R])) to (1.4), (1.5)–(1.7) with suppρ(t,⋅)⊂Ω for t>0. Moreover, ρ satisfies the following estimates
‖ρ(t,⋅)‖L1=‖ρ0‖L1 for a.e. t>0,TV(ρ(t,⋅))≤TV(ρ0)eKt+πteKt‖f‖L∞([0,R])(‖∇divμ‖L1+CM(Rw)), | (2.11) |
where we define
K:=5‖f′‖L∞([0,R])(‖div μ‖L∞+εRw‖∇η‖W1,1(1+R2w‖∇η‖2L1)),CM(Rw):=εRwM‖∇η‖W2,1(1+8R2w‖∇η‖L1‖∇η‖W1,1). |
Stability with respect to ρ0, v and μ also holds from [1,Theorem 2.2].
PROOF OF THEOREM 1. Following [1], we have to check that we fit the required hypotheses. First of all, given any r∈C0([0,T];L1(Ω;[0,R])), we verify that the scalar conservation law
∂tρ+div (ρV(ρ)w(t,x))=0in R2, |
with w(t,x)=μ(x)+I[r(t)](x), satisfies the assumptions of [39,Theorem 5 and Sec. 5.4], and therefore admits a weak entropy solution ρ∈L∞(R+;L1(Ω;[0,R])) (see [1,Lemma 2.1]). Setting φ(t,x,ρ)=f(ρ)w(t,x), it is easy to check that φ,∂ρφ,∂2xi,ρφ,∂2xi,xjφ∈C0([0,T]×R2×[0,R]), ∂ρφ∈L∞([0,T]×R2×[0,R]) and div φ∈L∞([0,T]×R2×[0,R]), thanks to (I2), (I3) and (I4). Moreover, by (2.6), we have that
‖div w(t,⋅)‖L1≤‖divμ‖L1+εRwM‖∇η‖W1,1(1+R2w‖∇η‖2L1)<+∞, |
which guarantees that ρ∈C0(R+;L1(Ω;[0,R])). Moreover, by (2.5),
‖div ∂ρφ‖L∞≤‖f′‖L∞([0,R])(‖div μ‖L∞+εRw‖∇η‖W1,1(1+R2w‖∇η‖2L1)), |
and by (2.7)
‖∇div(μ+I[r(t)])‖L1≤‖∇div μ‖L1+CM(Rw). |
Therefore, by [40,Theorem 2.2], we get (2.11).
Estimates (2.8)–(2.10) ensure that the same fixed point argument used in [1,Proof of Theorem 2.1] can be applied to prove the existence and uniqueness of solutions to (1.4).
We take Ω=[xmin1,xmax1]×[xmin2,xmax2] and denote by ρ:[0,∞[×Ω→[0,R] and
f=f(t,x,ρ,(η∗wρ)):=ρV(ρ)ν(x,I[ρ(t)](x)) |
the solution and the flux function of problem (1.1)–(1.4). We use a uniform Cartesian grid with nodes (xi1,xj2), i=1,…,M1 and j=1,…,M2 such that xi1=(i−1/2)h, xj2=(j−1/2)h, h=(xmax1−xmin1)/M1=(xmax2−xmin2)/M2. This corresponds to M1×M2 grid points xi:=(xi1,xj2), where i=(i,j)∈M:={1,…,M1}×{1,…,M2}⊂N2, and as in [9] we utilize two-dimensional unit vectors e1:=(1,0) and e2:=(0,1) to address neighbouring grid points xi+e1=(xi+11,xj2) and xi+e2=(xi1,xj+12).
We define u:[0,∞)→RM1×M2 as a solution computed at an instant t in the grid points where
ui(t)=ρ(t,xi),fi=f(t,xi,ρ(t,xi),(η∗wρ(t))(xi))for i∈M. |
In Section 3.2 we will discuss the discretization of the convolution product. Using this notation, we may approximate the solution of (1.1)–(1.4) in semi-discrete form (that is, discrete in space but continuous in time) by a system of ODEs
dudt=C(u) | (3.1) |
where C(u) represents the spatial discretization of the convective term with entries given by C(u)=(C(u)i)i∈M with
C(u)i=−2∑l=11h(ˆfi+12el−ˆfi−12el), | (3.2) |
where ˆfi+12e1 and ˆfi+12e2 are the numerical fluxes, which in this paper will be a fifth-order version. To this end, we require the summands for l=1 and l=2 in (3.2) to be of the same order of approximation to ∂f/∂x1 and ∂f/∂x2, respectively, at x=xi. For upwinding and stability, a flux function f(ρ) is split as follows:
f(ρ)=f+(ρ)+f−(ρ),with df+(ρ)dρ≥0 and df−(ρ)dρ≤0. | (3.3) |
Then each component is approximated separately using its own "wind direction" with respect to el. The simple Lax-Friedrichs flux splitting
f±(ρ)=12(f(ρ)±αρ) |
with a suitable viscosity coefficient α>0 is used in this paper. We herein use
αk=maxρ|∂ρ(ρV(ρ))|supx∈Ω|ν(x)⋅ek|,k=1,2, |
in direction ek, k=1,2. The numerical fluxes are split accordingly, i.e.,
ˆfi+12ek=R+(f+i+(−r:r)ek)+R−(f−i+(−r+1:r+1)ek),k=1,2, | (3.4) |
where R±(fi+(−r:r)ek)=R±(fi−rek,…,fi+rek) denotes (2r−1)th-order WENO upwind-biased reconstructions for r=2,3,4, see [33,34,35].
Ghost cells are needed to compute numerical fluxes near the boundary. To handle these cases we use the boundary condition in (1.1) and set ui=0 if i∉M and as the vector field ν points inward along ∂Ω, i.e., ν⋅n(x)≤0 for x∈∂Ω, we set
ˆfi+12el={R+(fi+(−r:r)el)if xi∈{xmin1}×[xmin2,xmax2]∪[xmin1,xmax2]×{xmin2},R−(fi+(−r:r)el)if xi∈{xmax1}×[xmax2,xmax2]∪[xmin1,xmax1]×{xmax2}. | (3.5) |
For evacuation problems, to avoid dealing with extended domains, we have to handle a vector field ν which points outward at the exit door D⊂∂Ω, i.e., ν⋅n(x)>0 for x∈D. In this case, we set
ˆfi+12el=R+(fi+(−r:r)el)for xi∈D. |
For more details about the implementation of high-order finite difference WENO schemes for crowd dynamics see [9,32].
In order to evaluate the non-local term in (1.5), we take into account that ∇(η∗wρ)=∇η∗wρ, where the convolution term ∗w is defined by (1.6). The corresponding convolutions (∂η/∂x1)∗wρ and (∂η/∂x2)∗wρ are calculated approximately on the discrete grid via a quadrature formula, in our cases a composite Simpson rule. Since supp(η)⊂[−n0h,n0h]×[−n0h,n0h] for n0∈N large enough, any convolution product is given by
(η∗ρ(t))(xi)≈n0∑p=−n0n0∑q=−n0h2cpcqρ(t,xi−p)η(xp), |
where cp and cq are the coefficients in the quadrature rule and p=(p,q). This formula for u=(ui)∈RM1×M2 and for the convolution product (1.6) can be written as
(η∗wu)(xi)=n0∑p=−n0n0∑q=−n0h2cpcquw,i−pη(xp), | (3.6) |
where uw,i is a discrete version of the function (1.7) defined by
uw,i={uiif i∈M, Rwif xi∈B(Ω,d(suppη))∖Ω, 0elsewhere. | (3.7) |
Clearly, the discrete convolution (3.6) causes a computational bottleneck. This is a classical problem in scientific computing that is effectively handled by fast convolution algorithms, mainly based on Fast Fourier Transforms [41] (see also [13,14]).
Finally, the semi-discrete scheme (3.1) is discretized by a third-order TVD Runge-Kutta time discretization method [34] that can be specified as follows. Assume that un is the vector of approximate solutions at t=tn. Then the approximate values un+1 associated with tn+1=tn+Δt are calculated by
u(1)=un+ΔtC(un),u(2)=34un+14u(1)+14ΔtC(u(1)),un+1=13un+23u(2)+23ΔtC(u(2)). | (3.8) |
The combined space and time discretizations define a fully discrete scheme. Observe that the time evolution is only of third order of accuracy, while the spatial discretization is fifth-order accurate. To have fifth-order accuracy also in time, a two-step SSP Runge-Kutta method should be used.
We aim at investigating the effects of the non-local operator (1.5)–(1.7) from the crowd dynamics modelling point of view. To this end, in the following numerical examples, we solve numerically (1.4)–(1.7) for t∈[0,T] and x∈Ω by using the high-resolution numerical scheme described in Section 3. In particular, we consider here a FD-WENO5 scheme with fifth-order of accuracy in space, see [32] for a discussion of high-order finite difference WENO schemes with different orders of accuracy for crowd dynamics. For each iteration, the time step Δt in (3.8) is determined by the formula
Δthmax{α1,α2}=12Ccfl. |
We choose Ccfl as the largest multiple of 0.05 that yields oscillation-free numerical solutions. In all numerical tests we have used Ccfl=0.2.
In contrast to (1.6)–(1.7), the model proposed in [3,4] uses the following definition of the convolution product in the non-local term (1.5)
(η∗Ωρ(t))(x)=1z(x)∬Ωρ(t,y)η(x−y)dy,wherez(x):=∬Ωη(x−y)dy. | (4.1) |
We remark that the model in [3,4] also displays non-locality in the speed, but this prevents a global maximum principle from holding. Therefore, here we keep the speed dependence on the density local.
We consider the evacuation problem proposed in [4,Section 2.1], where Ω=R∖(C1∪C2) is composed by a room R=[0,8]×[−2,2] with two symmetric columns C1=]4.5,7[×]0.8,1.5[ and C2=]4.5,7[×]−1.5,−0.8[ that guide the evacuation through the exit door set at D={8}×]−0.8,0.8[. The functions and parameters are chosen as
V(ρ)=2min{1,max{0,(1−ρ)}},ρ0(x)=0.9χ[0.5,3]×[−1.8,1.8](x),ε=0.6,Rw=1.5η(x)=315128πl18(l4−‖x‖4)4χ[0,l](x), | (4.2) |
where χ[0,l](x) denotes the characteristic function. The fixed vector field μ(x) is given by the unit vector tangent to the geodesic from x to the exit door, see Figure 2a. Since the non-local term defined by (4.1) does not guarantee that the resulting direction of motion points inside the domain (ν(t,x)⋅n(x)≤0 for x∈∂Ω, t≥0), we need to add to μ a (fixed) discomfort vector field with maximal intensity along the walls as in [3,4], resulting in the vector field showed in Figure 2b.
We display numerical approximations computed with FD-WENO5 scheme with h=1/80 at times T=1,3 and 6 for two kernel supports (l=0.45 in Figure 3 and l=0.9 in Figure 4). We observe that the non-local correction term (1.5) allows pedestrians to "see" the presence of the wall and obstacles, and to deviate the movement from the desired trajectory. For l=0.45, we can observe that pedestrians can pass between the obstacles and the wall, as in the Colombo-Rossi model, however this effect is less remarkable for larger kernel supports like l=0.9. Indeed, comparing Figures 3 and 4, we can see that a larger kernel support corresponds to a wider discomfort effect, impacting the velocity vector field on larger portions of the walkable domain. More generally, qualitative differences between the two models depend on the parameter choices of Rw and the discomfort vector field. Nevertheless, we remark that our definition of the convolution (1.6)–(1.7) qualitatively captures the discomfort due to the presence of walls and obstacles delimiting the walking domain.
Figure 5 illustrates the effect of the parameter value Rw. Specifically, the scenario of the left column of Figure 5 is the same as in the left column of Figure 3, with l=0.45 and Rw=1.5 replaced by Rw=2. On the right column of Figure 5, we change also l to l=0.9 and compare it to Figure 4, left. Larger values of Rw increase the "discomfort" effect exerted by walls and obstacles, as it becomes apparent if one compares, for instance, the widths of the streams of pedestrians to either side and between the obstacles: they are thinner for Rw=2 than for Rw=1.5.
Finally, Figure 6 shows the impact of the parameters Rw and l on the evacuation time for both models. We can observe that the model is quite sensitive to changes in the convolution support since, as previously mentioned, this affects heavily the resulting velocity field near obstacles and walls. Furthermore, the evacuation process for a given value of l is slower for Rw=2 than for Rw=1.5. We mention that the parameters and obstacles have been chosen to illustrate the model and the difference to [3,4], but do not correspond to a specific real-world scenario. There are however, abundant experimental studies providing data on pedestrian flows with obstacles that could form the basis for future comparison with simulations, see e.g., [42,43,44,45].
In this section, we consider that pedestrians have a limited vision field oriented in a given direction. We study a simple example of evacuation of a rectangular room Ω=[0,8]×[−3,3], where the vector field μ(x)=(1,0) is fixed constantly oriented towards the right of the domain. We investigate the influence of a conic convolution kernel on the evacuation dynamics and the pattern formation. We take the kernel function η(x) given in (4.2) and cut a conic section η(x)χS(x,l,α,γ)(x) of angle 2α oriented to direction γ(x) which is described by the region
S(x,l,α,γ)={y∈R2:‖y−x‖≤l,(y−x)⋅γ(x)‖y−x‖‖γ(x)‖≥cosα} |
(see Figure 7). The section ηχS(x,l,α,γ) is smoothed by convolution with a Gaussian kernel g(x)=exp(−(‖x‖2/2σ)) with σ=5×10−4, then normalized and finally shifted by the distance 0.04 to ensure that the maximum of the normalized smoothed kernel is centered in (0,0). Other parameters are taken as
V(ρ)=6min{1,max{0,(1−ρ)}},ε=0.6,l=0.9,ρ0(x)=0.9χ[0.5,4]×[−1,1](x). |
We compare the dynamics given by different kernel orientations and different angle amplitudes. Besides the circular symmetric kernel η (i.e., α=π), we consider
● γ(x)=(−1,0), corresponding to forward interaction (by central symmetry of the convolution product), and α=π/2,π/4;
● γ(x)=(1,0), corresponding to backward interaction, and α=π/4;
● γ(x)=(0,−1), modeling pedestrian reacting to the presence of other individuals (or obstacles) on their left, and α=π/4;
● γ(x)=(−1,−1), for forward-left interactions, and α=π/4.
The corresponding convolution kernels are depicted in Figure 7. In Figures 8–10, we display numerical approximations and the corresponding vector fields of preferred directions computed with FD-WENO5 scheme with h=1/80 at times T=0.1, T=0.2 and T=0.4. We observe that symmetric and half-circular downstream interaction kernels lead to similar patterns consisting of horizontal lanes (see Figure 8), while narrower angles α lead to vertical patterns, both for forward and backward interactions (displayed in Figure 9). Finally, lateral interactions mostly lead to diagonal waves (Figure 10). Again, the scenario is hypothetical, but could be compared with experimental results from the literature [26,27,28,46].
In this section, we consider the problem of evacuating people in a room through a door. In particular, we are interested in studying the impact of the presence of obstacles in front of the door on the evacuation time. This problem has already been discussed by several authors, see for example [4,47,48] and references therein. In these works, the authors infer that, in some cases, the location and size of the obstacles may speed up the population to the exit. The interest in this phenomenon, sometimes called "faster-is-slower effect" [49,50], goes back to the well-known paper by Helbing et al. [51]. Delays during evacuation due to other effects have been investigated, for instance, in [52,53]. For this example, we consider the walking domain available to pedestrians is Ω=R∖Ci, i=1,2,3, where the room R=[0,8]×[−3,3] contains obstacles Ci. The door D, the functions v and η, and the parameter ε and Rw are the same as in (4.2). The initial condition is a linear combination of characteristic functions with values 0.9 in [0.5,2]×[−2.2,0], 0.6 in [0.5,2.2]×[0,2.2], 0.5 in [2,4]×[−2.2,0] and 0.8 in [2.2,4]×[0,2.2] (see Figure 11). The obstacles and parameter l for the three different scenarios considered are
C1=∅,C2=]7,7.8[×(]−1.8,1.3[∪]1.3,1.8[),C3=]5,6[×]−0.25,0.25[,l=1. | (4.3) |
The numerical solutions for the three scenarios at three different times is displayed in Figure 12, where we have used the FD-WENO5 scheme to computed the approximate solutions.
Figure 13 shows the time evolution of the mass inside the room. We observe that in case C1 the evacuation time is higher in comparison to cases C2 and C3, where the evacuation time is lower, especially for case C3. This is confirmed by Figure 12, which shows the evacuation of the populations at times T=6,20 and 32, for the cases C1, C2 and C3 respectively.
In this work, we have proposed and studied a non-local macroscopic pedestrian flow model accounting for the presence of walls and obstacles limiting the walking domain. In particular, the proposed model is able to capture pedestrians' discomfort near obstacles and walls. Under suitable regularity assumptions, the model turns out to be well-posed. Moreover, we analyzed the impact of different anisotropic kernels on the formation of patterns in the solutions. High-resolution numerical schemes of WENO type allow to perform accurate simulations, bypassing the computational bottleneck given by the dependence of the flux function on integral terms. Future research should focus on multi-population models accounting for groups with different characteristics and/or destinations, and on the theoretical analysis of the observed pattern formation.
This research was supported by the INRIA Associated Team "Efficient numerical schemes for non-local transport phenomena" (NOLOCO; 2018–2020). RB is supported by Fondecyt project 1170473 and CRHIAM, project ANID/FONDAP/15130015. LMV is supported by Fondecyt project 1181511. RB, DI and LMV are also supported by CONICYT/PIA/Concurso Apoyo a Centros Científicos y Tecnológicos de Excelencia con Financiamiento Basal AFB170001.
The authors declare there is no conflicts of interest in this paper.
[1] | R. M. Colombo, M. Garavello, M. Lécureux-Mercier, A class of nonlocal models for pedestrian traffic, Math. Models Methods Appl. Sci., 22 (2012), 1150023. |
[2] | M. Mimault, Lois de conservation pour la modélisation des mouvements de foule, PhD thesis, 2015, University of Nice. Available from: http://www.theses.fr/2015NICE4102/document. |
[3] | R. M. Colombo, E. Rossi, Nonlocal conservation laws in bounded domains, SIAM J. Math. Anal., 50 (2018), 4041-4065. |
[4] | R. M. Colombo, E. Rossi, Modelling crowd movements in domains with boundaries, IMA J. Appl. Math., 84 (2019), 833-853. |
[5] | R. Colombo, M. Rosini, Pedestrian flows and nonclassical shocks, Math. Methods Appl. Sci., 28 (2008), 1553-1567. |
[6] | R. L. Hughes, A continuum theory for the flow of pedestrians, Transpn. Res.-B, 36 (2002), 507-535. |
[7] | N. Bellomo, C. Dogbé, On the modelling crowd dynamics from scaling to hyperbolic macroscopic models, Math. Models Methods Appl. Sci., 18 (2008), 1317-1345. |
[8] | Y. Jiang, P. Zhang, S. Wong, R. Liu, A higher-order macroscopic model for pedestrian flows, Physica A, 389 (2010), 4623-4635. |
[9] | R. Bürger, D. Inzunza, P. Mulet, L. M. Villada, Implicit-explicit methods for a class of nonlinear nonlocal gradient flow equations modelling collective behaviour, Appl. Numer. Math., 144 (2019), 234-252. |
[10] | B. Maury, A. Roudneff-Chupin, F. Santambrogio, A macroscopic crowd motion model of gradient flow type, Math. Models Methods Appl. Sci., 20 (2009), 1787-1821. |
[11] | B. Piccoli, A. Tosin, Pedestrian flows in bounded domains with obstacles, Contin. Mech. Thermodyn., 21 (2009), 85-107. |
[12] | L. Bruno, A. Tosin, P. Tricerri, F. Venuti, Non-local first-order modelling of crowd dynamics: a multidimensional framework with applications, Appl. Math. Model., 35 (2011), 426-445. |
[13] | R. Bürger, G. Chowell, E. Gavilán, P. Mulet, L. M. Villada, Numerical solution of a spatio-temporal gender-structured model for hantavirus infection in rodents, Math. Biosci. Eng., 15 (2018), 95-123. |
[14] | R. Bürger, G. Chowell, E. Gavilán, P. Mulet, L. M. Villada, Numerical solution of a spatio-temporal predator-prey model with infected prey, Math. Biosci. Eng., 16 (2019), 438-473. |
[15] | R. M. Colombo, M. Lécureux-Mercier, Nonlocal crowd dynamics models for several populations, Acta Math. Sci. Ser. B (Engl. Ed.), 32 (2012), 177-196. |
[16] | P. Kachroo, S. J. Al-Nasur, S. A. Wadoo, A. Shende, Pedestrian Dynamics, Springer-Verlag, 2008. |
[17] | S. Blandin, P. Goatin, Well-posedness of a conservation law with non-local flux arising in traffic flow modeling, Numer. Math., 132 (2016), 217-241. |
[18] | A. Kurganov, A. Polizzi, Non-oscillatory central schemes for traffic flow models with arrhenius look-ahead dynamics, Netw. Heterog. Media, 4 (2009), 431-451. |
[19] | A. Sopasakis, M. Katsoulakis, Stochastic modelling and simulation of traffic flow: asymmetric single exclusion process with arrhenius look-ahead dynamics, SIAM J. Appl. Math., 66 (2006), 921-944. |
[20] | F. Betancourt, R. Bürger, K. H. Karlsen, E. M. Tory, On nonlocal conservation laws modelling sedimentation, Nonlinearity, 24 (2011), 855-885. |
[21] | K. Zumbrun, On a nonlocal dispersive equation modeling particle suspensions, Quart. J. Appl. Math., 57 (1999), 573-600. |
[22] | S. Göttlich, S. Hoher, P. Schindler, V. Schleper, A. Verl, Modeling, simulation and validation of material flow on conveyor belts, Appl. Math. Model., 38 (2014), 3295-3313. |
[23] | C. Appert-Rolland, J. Cividini, H. J. Hilhorst, P. Degond, Pedestrian flows: from individuals to crowds, Transp. Res. Procedia, 2 (2014), 468-476. |
[24] | P. Degond, C. Appert-Rolland, J. Pettré, G. Theraulaz, Vision-based macroscopic pedestrian models, Kinet. Relat. Models, 6 (2013), 809-839. |
[25] | R. Etikyala, S. Göttlich, A. Klar, S. Tiwari, Particle methods for pedestrian flow models: from microscopic to nonlocal continuum models, Math. Models Methods Appl. Sci., 24 (2014), 2503-2523. |
[26] | W. Daamen, D. C. Duives, S. P. Hoogendoorn (eds.), Proceedings of the Conference on Pedestrian and Evacuation Dynamics 2014 (PED 2014), 22-24 October 2014, Delft, The Netherlands, vol. 2 of Transportation Research Procedia, 2014. |
[27] | A. Dederichs, G. Köster, A. Schadschneider (eds.), Proceedings of Pedestrian and Evacuation Dynamics 2018 (PED 2018), vol. A26 of Collective Dynamics, 2020. |
[28] | W. Song, J. Ma, L. Fu (eds.), Proceedings of Pedestrian and Evacuation Dynamics 2016 (PED 2016), 17-21 October 2016, Hefei, China, University of Science and Technology Press, Hefei, China, 2016. |
[29] | C. Chalons, P. Goatin, L. M. Villada, High-order numerical schemes for one-dimensional nonlocal conservation laws, SIAM J. Sci. Comput., 40 (2018), A288-A305. |
[30] | F. A. Chiarello, P. Goatin, L. M. Villada, High-order Finite Volume WENO schemes for non-local multi-class traffic flow models, in Proceedings of the XVⅡ International Conference (HYP2018) on Hyperbolic Problems, 25-29 June 2018, Pennsylvania State University, USA (eds. A. Bressan, M. Lewicka, D. Wang and Y. Zheng), American Institute of Mathematical Sciences, Springfield MO, USA, 2020, 353-360. |
[31] | F. A. Chiarello, P. Goatin, L. M. Villada, Lagrangian-antidiffusive remap schemes for non-local multi-class traffic flow models, Comput. Appl. Math., 39 (2020), https://doi.org/10.1007/s40314-020-1097-9. |
[32] | D. Inzunza, Métodos Implicitos-Explicitos para Problemas de Convección-Difusión-Reacción no Lineales y no Locales, PhD thesis, 2019, https://www.ci2ma.udec.cl/publicaciones/tesisposgrado/graduado.php?id=70, Universidad de Concepcion. |
[33] | G. S. Jiang, C. W. Shu, Efficient implementation of weighted ENO schemes, J. Comput. Phys., 126 (1996), 202-228. |
[34] | X. D. Liu, S. Osher, T. Chan, Weighted essentially non-oscillatory schemes, J. Comput. Phys., 115 (1994), 200-212. |
[35] | C.-W. Shu, High order weighted essentially nonoscillatory schemes for convection dominated problems, SIAM Rev., 51 (2009), 82-126. |
[36] | E. Rossi, Definitions of solutions to the IBVP for multi-dimensional scalar balance laws, J. Hyperbolic Differ. Equ., 15 (2018), 349-374. |
[37] | J. Vovelle, Convergence of finite volume monotone schemes for scalar conservation laws on bounded domains, Numer. Math., 90 (2002), 563-596. |
[38] | C. Bardos, A. Y. Le Roux, J.-C. Nédélec, First order quasilinear equations with boundary conditions, Comm. Partial Differential Equations, 4 (1979), 1017-1034. |
[39] | S. N. Kružkov, First order quasilinear equations with several independent variables, Mat. Sb. (N.S.), 81 (1970), 228-255. |
[40] | M. Lécureux-Mercier, Improved stability estimates on general scalar balance laws, 2010. |
[41] | J. Von Zur Gathen, J. Gerhard, Modern Computer Algebra, Cambridge University Press, 2013. |
[42] | A. Alhawsawi, M. Sarvi, M. Haghani, A. Rajabifard, Investigating pedestrians' obstacle avoidance behaviour, Collective Dynamics, A26 (2020), 413-422. |
[43] | C. Dias, O. Ejtemai, M. Sarvi, M. Burd, Exploring pedestrian walking through angled corridors, Transp. Res. Procedia, 2 (2014), 19-25. |
[44] | K. Fujii, T. Sano, Experimental study on crowd flow passing through ticket gates in railway stations, Transp. Res. Procedia, 2 (2014), 630-635. |
[45] | X. Liu, W. Song, L. Fu, H. Zhang, Pedestrian inflow process under normal and special situations, in Proceedings of Pedestrian and Evacuation Dynamics 2016 (PED 2016), 17-21 October 2016, Hefei, China (eds. W. Song, J. Ma and L. Fu), University of Science and Technology Press, Hefei, China, 2016, 136-143. |
[46] | X. Mai, X. Zhu, W. Song, J. Ma, Qualitative analysis on two-dimensional pedestrian flows - unidirectional and bidirectional, in Proceedings of Pedestrian and Evacuation Dynamics 2016 (PED 2016), 17-21 October 2016, Hefei, China (eds. W. Song, J. Ma and L. Fu), University of Science and Technology Press, Hefei, China, 2016, 151-156. |
[47] | M. Twarogowska, P. Goatin, R. Duvigneau, Comparative study of macroscopic pedestrian models, Transp. Res. Procedia, 2 (2014), 477-485. |
[48] | M. Twarogowska, P. Goatin, R. Duvigneau, Macroscopic modeling and simulations of room evacuation, Appl. Math. Model., 38 (2014), 5781-5795. |
[49] | J. Chen, Q. Zeng, W. Zhang, Y. Wu, Q. Liu, P. Lin, Restudy the faster-is-slower effect by using mice under high competition, in Proceedings of Pedestrian and Evacuation Dynamics 2016 (PED 2016), 17-21 October 2016, Hefei, China (eds. W. Song, J. Ma and L. Fu), University of Science and Technology Press, Hefei, China, 2016, 159-162. |
[50] | T. Mashiko, T. Suzuki, Speed-up of evacuation by additional walls near the exit, in Proceedings of Pedestrian and Evacuation Dynamics 2016 (PED 2016), 17-21 October 2016, Hefei, China (eds. W. Song, J. Ma and L. Fu), University of Science and Technology Press, Hefei, China, 2016, 334-339. |
[51] | D. Helbing, I. Farkas, T. Vicsek, Simulating dynamical features of escape panic, Nature, 407 (2000), 487-490. |
[52] | C. Feliciano, K. Nishinari, Investigation of pedestrian evacuation scenarios through congestion level and crowd danger, Collective Dynamics, A26 (2020), 150-157. |
[53] | Z. Shahhoseini, M. Sravi, M. Saberi, The impact of merging maneuvers on delay during evacuation, in Proceedings of Pedestrian and Evacuation Dynamics 2016 (PED 2016), 17-21 October 2016, Hefei, China (eds. W. Song, J. Ma and L. Fu), University of Science and Technology Press, Hefei, China, 2016, 100-104. |