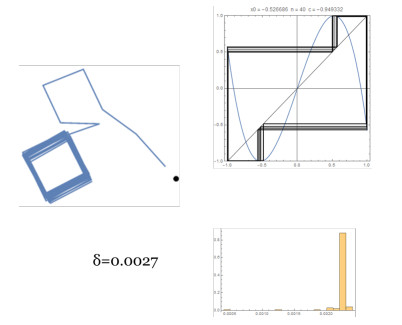
Citation: Carlos Ramos. Animal movement: symbolic dynamics and topological classification[J]. Mathematical Biosciences and Engineering, 2019, 16(5): 5464-5489. doi: 10.3934/mbe.2019272
[1] | Jorge Duarte, Cristina Januário, Nuno Martins . A chaotic bursting-spiking transition in a pancreatic beta-cells system: observation of an interior glucose-induced crisis. Mathematical Biosciences and Engineering, 2017, 14(4): 821-842. doi: 10.3934/mbe.2017045 |
[2] | J. Leonel Rocha, Sandra M. Aleixo . An extension of Gompertzian growth dynamics: Weibull and Fréchet models. Mathematical Biosciences and Engineering, 2013, 10(2): 379-398. doi: 10.3934/mbe.2013.10.379 |
[3] | Alex John Quijano, Michele L. Joyner, Edith Seier, Nathaniel Hancock, Michael Largent, Thomas C. Jones . An aggregate stochastic model incorporating individual dynamics for predation movements of anelosimus studiosus. Mathematical Biosciences and Engineering, 2015, 12(3): 585-607. doi: 10.3934/mbe.2015.12.585 |
[4] | Erik M. Bollt, Joseph D. Skufca, Stephen J . McGregor . Control entropy: A complexity measure for nonstationary signals. Mathematical Biosciences and Engineering, 2009, 6(1): 1-25. doi: 10.3934/mbe.2009.6.1 |
[5] | Xiujun Zhang, Umair Saleem, Muhammad Waheed, Muhammad Kamran Jamil, Muhammad Zeeshan . Comparative study of five topological invariants of supramolecular chain of different complexes of N-salicylidene-L-valine. Mathematical Biosciences and Engineering, 2023, 20(7): 11528-11544. doi: 10.3934/mbe.2023511 |
[6] | Thomas Hillen, Kevin J. Painter, Amanda C. Swan, Albert D. Murtha . Moments of von mises and fisher distributions and applications. Mathematical Biosciences and Engineering, 2017, 14(3): 673-694. doi: 10.3934/mbe.2017038 |
[7] | Xuan Zhang, Huiqin Jin, Zhuoqin Yang, Jinzhi Lei . Effects of elongation delay in transcription dynamics. Mathematical Biosciences and Engineering, 2014, 11(6): 1431-1448. doi: 10.3934/mbe.2014.11.1431 |
[8] | Baojian Hong . Bifurcation analysis and exact solutions for a class of generalized time-space fractional nonlinear Schrödinger equations. Mathematical Biosciences and Engineering, 2023, 20(8): 14377-14394. doi: 10.3934/mbe.2023643 |
[9] | Qiang Hou, Haiyan Qin . Global dynamics of a multi-stage brucellosis model with distributed delays and indirect transmission. Mathematical Biosciences and Engineering, 2019, 16(4): 3111-3129. doi: 10.3934/mbe.2019154 |
[10] | Yongbing Nie, Xiangdong Sun, Hongping Hu, Qiang Hou . Bifurcation analysis of a sheep brucellosis model with testing and saturated culling rate. Mathematical Biosciences and Engineering, 2023, 20(1): 1519-1537. doi: 10.3934/mbe.2023069 |
Animal dispersal is a key factor that underlies a variety of phenomena in biology, ecology and biogeography. Nowadays, relevant improvements on the subject depends naturally on appropriate mathematical methods and have deep impact on the study of population dynamics, spreading of diseases, animal locomotion, plagues in agriculture, and many other areas. The study of complex individual movement, either animal or other kind, has attracted much attention since Mandelbrot observed that certain types of trajectories exhibit scale invariance and fractal properties. The properties, of statistical nature, are usually expressed by the distribution of the movement lengths, for example, inverse power law-like tail, among other possibilities. Many approaches in the literature follow this perspective and have been applied to different animal movements, bird flight, insect movement and also human movement, see for example the survey [1], where several models are described. An important reference in the subject is [2] where a conceptual framework for movement in ecology is proposed, with a mechanistic perspective. Their approach is very general and includes certain components which determine the movement path. Three of these components regard the individual and are: internal state, motion capacity and navigation capacity. A fourth component, corresponding to the external factors, represents all aspects of the abiotic and biotic environment which influence the movement.
Several existing reviews, in the literature, focus on different aspects of animal movement modeling. In [3], the authors review literature on the agent based modeling approach which is able to incorporate in simulations many features of animal behavior and the interaction with different environments. Alternative methods are discussed in the papers, [4,5,6] were the authors consider state space models appropriate to deal with biological and statistical features of satellite tracking data, and take into account the different methodologies of collecting data, using statistically robust methods. The state-space framework considers the internal state of the animal as a possible state variable which influence the displacement, therefore allowing the transition between behavior states or modes, within the model. This is determinant for the observed type of trajectory, since the animal can be migrating, searching for water, foraging, exploratory, and this types of behavior produces distinct types of trajectories.
In [7], the authors outline alternative methods for modeling animal trajectories with hidden Markov models, where the modeling is based on existing non-observable states which are governed by some probability distributions, e.g., Markov chains. The authors discuss these methods comparing it with the state-space model approach. The computational tractability and mathematical simplicity are stressed as main advantages of the referred methods, and the authors manage to apply the models to changing behavior of the animals and to multiple animal movement description.
Regarding the appropriate length probability distribution, in the description of animal movements, and the stochastic nature of animal movement, still recently, remains subject to debate and certain controversy, namely regarding the importance or not of the Levy walk type. See for example [8,9].
We may refer some reasons why animals move: search for food or water, escape from danger or some gradient such as temperature, search for mates, or other. However, here we do not discuss the causes, the motivation for the movement, neither the influential external factors. For the moment, we consider a descriptive perspective - the kinematics of animal motion - or kinematics of complex motion, since our method apply to whatever moving object: the path of a fly in the air, a particle in a liquid suspension, the movement of individual macromolecules, seed dispersal by the wind, or a person walking in a street.
The main objective of the present paper is to produce and classify different types of trajectories using a very simple iterated map of the interval - a symmetric cubic map. The model is theoretical as the discussion here developed. Nevertheless we expect that the produced trajectory may be identified as a typical path of a certain animal, which at some extent is isolated or with stable behavior. The characteristics, patterns or irregularities of the trajectory are codified in the symbolic description of the orbits of the iterated map, through the alphabet , corresponding to the partition of monotonicity of the interval map. Therefore, the iterated map is seen as a descriptive classifying tool, a kind of dictionary for trajectories. The model depends on a real parameter, more precisely, a number in the interval . Nevertheless, it is sufficiently rich to describe and characterize an enormous diversity of trajectories. For different values of the parameter we obtain regular, periodic motion, almost constant direction motion and irregular motions with different kinds of statistical and geometrical properties. Some of these trajectories are approximately random walks, other approximate Levy walks, to refer some of the distributions more frequently considered in the literature, among many other types of length distributions.
The complexity description and the characterization of the movements are based on the topological classification and the combinatorial structure of the considered discrete dynamical system. The methods of symbolic dynamics and iterated maps of the interval were extensively studied by Sousa Ramos and his collaborators, see [10,11,12], regarding symbolic dynamics for bimodal maps. The main classifying tool is the kneading invariant and the topological entropy is a complete invariant for the bimodal family, in particular for the cubic family we are here considering. Therefore, topological entropy can be used to produce a classifying measure for animal trajectories. The occurring symbolic sequences as itineraries of some initial condition, called admissible sequences, are determined by a combinatorial criteria, with an ordering in the set of sequences.
Instead of giving a fixed distribution of the lengths, or of the direction changes, which we expect describe the trajectories of a certain animal, we define a family of symmetric cubic maps , , of the interval, which produce the referred lengths in each Cartesian coordinate, through iteration. The cubic family is characterized by a special pair of symbolic sequences, , usually called the kneading invariant. These sequences correspond to the symbolic itineraries of the critical points which are atractors for the dynamics. Therefore, these pair of orbits determine the behavior of every orbit in the system. Roughly speaking, we associate to a particular patch, or piece of trajectory, a symbolic sequence or a class of symbolic sequences. Thus, the study of the possible trajectories and its properties can be made enumerating symbolic sequences satisfying certain combinatorial constrains. On the other hand, using ergodic theory for symbolic dynamics and for iterated maps of the interval we may derive the probability distributions which are, in this case, a consequence of the model.
The usual approaches in the literature, as referred above, uses stochastic methods and statistical models and needs at some point a given probability distribution, or a set of distributions. Then is necessary to fit the observations estimating the parameters of the models. Usually, except in [6,7], different models must be used to consider different modes of behavior, that is, directed paths, exploratory paths, foraging paths, among other possibilities.
Our model is one of the most simple models found in the literature, which classify and produce complex trajectories. There is one parameter , and we have the possibility of modeling the change of behavior with a dependence of the parameter on the position. It is scale independent, since it can be applied directly from the microscopic scale to the scale of the largest animal in earth. This is obtained through the choice of a scale parameter , which represents the largest linear step possible for the particular animal.
We do not have a fixed probability distribution. We have a whole family of probability distributions, depending only on the chosen parameter , or on the kneading invariant. Nevertheless, we do not expect to reproduce exactly a particular observed animal trajectory through our model. The main point here is that from the pattern of an observed trajectory, we expect to identify the number of consecutive steps where the direction is approximately maintained, identify the changes in direction, and the consecutive steps of changing direction, then we can produce a sequence in the referred alphabet . Next, we may compute the minimal kneading sequence which turns the observed sequence into admissible with respect to the iterated map. In this case, we can compute the topological entropy which characterizes the system and the value of parameter which realizes the kneading sequence. In this situation, we have the topological entropy characterizing the global trajectories of the animal, and the parameter , which allows simulations of different trajectories of an animal in an equivalent state of the observed one.
Typically, low topological entropy values give direct motion, with very few changes in direction, or regular changes in direction. On the other hand, high topological entropy gives large variation on direction and many different possible patterns of changing direction.
In our model, extreme data arising from an apparently non-typical observed trajectory may not be removed, since we have no fixed probability distribution. In fact, within the same fixed parameter , the choice of the initial condition can lead to apparent strange transient behavior, which usually in the statistical approach must be considered an outlier. In our model the transient behavior is classified and incorporated.
In a first stage will be necessary to conduct experiments, with real animals in controlled environments, to produce the referred catalogue, associating animal/behavior to the class of appropriate kneading sequences, topological entropy, and parameter . Next, with the catalogue, we will be able to interpret observed trajectories from nature directly and to infer on the behavior of the observed animal crossing that information with biological knowledge. If experimental observations shows that the animal trajectories are not geometrically very close to the cubic-trajectories from the model, at least the average behavior observed can be fitted in large classes of behavior types and associated to classes of kneading sequences (and intervals of values of topological entropy). This means that the relevant average quantities such average displacement, regions of recurrence, average of direction changes, patterns of direction change, average area covered, among others quantities, are close to same quantities calculated on the produced trajectories in the model. On the other hand, using the catalogue, choosing the corresponding values of , for fixed animal/behavior, we obtain a pseudo random number generator suitable to animal motion simulations.
We expect that using our method to describe the motion of an animal, through a dynamical catalogue or dictionary of types of trajectories, we may obtain the possibility to develop a more complete model to include interactions with the environment and with other animals.
The paper is organized as follows: In Section 2 we describe general assumptions which support certain aspects of the model. Namely, the justification of trying to analyze and describe the trajectories, at a first stage without explicit causes or motivations for the motion.
In Section 3 we review the symbolic dynamics techniques used for bimodal maps, namely its classification, and the definition of the topological Markov chain in the periodic and pre-periodic case.
In Section 4 we introduce the model and discuss its interpretation and direct consequences. We describe the values of the parameter for which we have simple movement, associated with or low topological entropy, and some properties in the case of periodic critical orbits
In Section 5 we give explicit results regarding certain special typical situations: the full shift, where the statistical and geometrical characteristics are close to a random walk and the cases in which we have intermittent behavior. This last situation produces trajectories which are close to the Levy walk. A result on specific type of parameters is presented, with the definition of the Markov partition, the transition matrix and the characterization of the topological entropy. We give also the estimation of the step intervals associated of the Markov symbols, in an example.
In Section 6 we show how the model can be applied directly to 3D motion, with exactly the same topological invariants.
Our approach starts with an analogy with physics. Kinematics study the motion of an isolated particle or object. There are intrinsic characteristics of the particle which can be considered such as mass or the electrical charge. If there is no force we obtain uniform movement with constant velocity and zero acceleration. With a constant force, e.g., the gravitic force, we may have, depending on the initial conditions, a straight line or a parabola. With a central potential - gravitational or Couloub force - we may have a line, ellipse, parabola or hyperbola. With a time dependent force we jump from kinematics for dynamics, in a certain sense we have to introduce an interaction between the objects and have to consider Newton laws of motion.
By similar reasoning we may consider the kinematics of the isolated animal, or simply animal kinematics. In this case, the concept of isolated animal is similar to the notion of isolated object/particle in physics. An isolated system does not exist in real world, however, is an useful abstraction to progressive design an experimental or observational apparatus, reducing the known or identified interactions, in order to a particular phenomena or inter-relation of concepts. In the context of the animal movement the interaction with the surroundings is set to the minimum.
We can imagine an animal put in a plane (e.g. insect), to test different rugosities, different materials, to conclude that some of these materials are irrelevant to the typical behavior, and so on. Once this detailed experimental procedure is concluded we may collect data to determine what are the types of trajectories used by animals when the surroundings are producing the minimal stimulus possible.
Next we can observe if the animal stays still. In this case we have to consider a rest position. We observe repeated experiments to determine, if the same animal can exhibit different behaviors according to its internal state. It is reasonable to think that a certain animal or a certain specie have a finite set of type of behavior. And that these behaviors follows some pattern, which may correspond to some strategy.
Next we can introduce different kinds of interactions or environments to analyze the change of typical behaviors in the quasi-isolated regime through the introduced interactions or stimulae. We then may ask:
What is a typical motion on this condition of isolation?
With a change in the terrain, surface under the animal the type of movement changes?
It would be interesting to see how far we can go, either theoretically or experimentally, with the simplifying assumptions given below.
First general assumptions:
(1) The trajectory is composed of discrete linear steps.
(2) There is a maximal displacement, in one step, which is a consequence of the finite size of the animal.
(3) Any step depends on the previous step taken: Markov hypothesis.
These three assumptions allows the use of an iterated map of an interval to produce the steps at discrete time instants, , which can be scaled so that .
Next, the following assumptions determine the structure of the model and the specific families of iterated maps we may consider to produce the trajectory.
(4) There is a coordinate system , so that
for a certain piecewise monotone interval map .
(5) Differentiability: close steps give origin to close steps. There is no special reason for this assumption. The alternative would need a cause or an explanation for the existing discontinuity, or point without derivative.
Finally, the last class of assumptions which are in fact characteristics which may be present or not. These are not fundamental and depend on the animal specie, or on empirical evidence.
(6) Preferential direction for the motion: Isotropy or symmetry breaking. Since we are considering isolated animal if there is symmetry breaking this means that the animal has in its internal process a preference to some direction. For example, the possibility of detecting magnetic field, light, heat or other phenomena. This point is very interesting for certain classes of animals, however in this case we don't have isolation. In the present work, we will consider the isotropy assumption.
(7) The rest position is a possible solution: Depends on the internal state. It may be a solution or not. This means that we may have an external parameter codifying this situation.
(7a) stable: rest situation, exhaustion or animal strategy.
(7b) unstable: a small perturbation leads to a progressive path, spiral or direct.
Remark 1. The generalization for 3 dimensions is obtained changing (4): There is a coordinate system , so that
for a certain piecewise monotone interval map .
Remark 2. We could assume that there are discontinuity points (in a finite number) for some reason. Eventually empirical data may require this adjustment. In future work we plan to deal with this issue.
Remark 3. Different types of coordinates can be considered. We have tested already polar coordinates. However in the present paper we focus on Cartesian coordinates.
General bimodal maps, which are continuous maps with two critical points, are characterized by two real parameters, up to topological conjugacy. In an equivalent way, are characterized by two symbolic sequences called kneading sequences, corresponding to the symbolic itineraries of the critical points, see [10], for details, and below for the definition. Assuming the conditions referred above: isotropic motion, rest position as a possible solution and the simplest situation in which we have one atractor or two symmetrical atractors, we arrive to a symmetric bimodal family of interval maps. This family is equivalent to a one-parameter family of the interval . The simplest case possible, in terms of analytical expression, is a symmetric cubic map.
If we do not force the existence of the rest solution and considering possible non-isotropy lead us directly to a general bimodal map with two parameters, maintaining the case treated in this paper, as special cases.
Let us consider the family of symmetric surjective bimodal maps on the interval ,
The discrete dynamical system, referred in the introduction, is the pair and the orbit of a point is the set
obtained under iteration of .
The topological entropy measures the chaotic behavior of a discrete dynamical system and can be computed as the logarithm of the growth rate of periodic orbits for fixed size . It was proven in [13] that the topological entropy grows monotonically with the parameter , and is a topological invariant for the family .
The critical points of are denoted by
and their orbits are very important to understand the global behavior of the iterated map , for a fixed parameter . Let denote the intervals where is contractive. They are intervals centered in the critical points . Every point starting in will be attracted to the orbit of the critical point . Almost every point in the interval , under iteration, will reach one of , in finite time, and therefore almost every orbit is strongly conditioned by the orbits of the critical points. If the critical point is periodic then almost every point in the interval, is eventually attracted to the periodic orbit. If the critical orbit is aperiodic then almost every point in the interval is eventually attracted to it and we have chaotic motion. However, if the topological entropy is positive, in the chaotic regime, even the case of periodic critical point has appreciable complex behavior. On one hand, the transient behavior changes significantly, changing the parameter . On the other hand, there is an infinite number of periodic orbits, unstable, of any period, which coexist with the given attractive periodic orbit.
To classify every situation, and to know exactly what type of orbits coexist, given , is very useful the combinatorial method called symbolic dynamics in which numerical orbits are represented by symbolic sequences. In what follows we give the notions necessary to use symbolic dynamics. For more details, on symbolic dynamics of bimodal maps, we refer the works [10,11,12].
To simplify exposition assume that . We may consider orbits in the case , however in this case the absolute value of the critical points are larger or equal to , the dynamics is very simple and the symbolic description is trivial.
The address of is defined by
The itinerary of a point , which collects the addresses of the points in the orbit of , is defined by
which allows the correspondence of orbits, under iteration of , with symbolic one-sided sequences in the alphabet or , if we consider the orbits of the critical points. The space of one-sided sequences is denoted by or .
We denote the kneading invariant of by
where and , are the itineraries of the critical points and called kneading sequences.
Since is symmetric is useful the usual notation , to express the symmetry of the admissible sequences. In particular for any we have .
In general, we have two distinct critical orbits , in this case necessarily, (two symmetrical atractors). Nevertheless, we may have one critical orbit, that is, the two critical points belong to the same orbit (one atractor), and in this case the kneading sequences must be periodic and , where is the period.
The iteration of corresponds to the shift map on the symbolic space sequences, that is, we have a semi-conjugation
where
For different values of different types of sequences occur. When considering the alphabet some careful is needed since the symbols are special. They represent points and the symbols represent intervals. To the symbol only follows the sequence , to the symbol only follows the sequence , and no other possibility. On the contrary to the symbols , , , and the combinatorial diversity of sequences can be enormous, depending on .
To determine exactly which sequences occur as itineraries in the set of all possible sequences, , it is necessary to introduce an order relation in the set of sequences. First, we need a parity or sign function: the bimodal sign function is defined by
with
for , and
Consider the set ordered by
Let us define an induced order relation in the set of one-sided sequences . Consider two sequences and of . There is a number such that for . Then we set
if and only if
or
We can easily see that the symbolic order structure is compatible with the order in the real line. In fact, for , if and only if .
Let us characterize the orbits and symbolic sequences which are not critical, that is, does not have the symbol . A sequence is called admissible, with respect to , or , if, by definition, there is so that .
The combinatorial characterization for the admissible sequences is the following: A sequence is admissible if and only if and or and .
Let us now characterize the orbits and symbolic sequences which are kneading sequences: Periodic if , pre-periodic if or aperiodic . In every case . In the following sentences is in one of the previous three types.
A sequence is a maximal (resp. minimal) kneading sequence, or if and only if (resp. ). for every .
Let us consider the case of periodic and pre-periodic kneading sequences, that is, in the form
or
The values of the parameter for which the critical orbits are pre-periodic are called Misiurewicz points. In this two situations we have a Markov partition of the interval , with respect to iteration of . The Markov partition is obtained directly from the orbits of the critical points . From the periodicity of the tail of the kneading sequences there is a least natural number so that
for any . In this case, the set constitute the set of boundary points of the Markov partition for . Each interval of the partition is associated to a finite word in the alphabet and to a Markov state.
Let be an admissible periodic sequence. Which means that . Let denote the point in the interval satisfying . If there is no ambiguity we write , to simplify notation.
In the case are periodic, of period , we have a Markov partition which can be explicitly obtained from symbolic dynamics, and also the transition matrix induced by . The Markov partition is given precisely by the successive shifts of the kneading sequences and ordered by . Let
There is a unique permutation on the set which re-orders the previous list of sequences with respect to :
Therefore, the partition is given by
a total of intervals, and note that, since is surjective,
Due to the specificities of our approach, we introduce a different enumeration, for the Markov alphabet, which is not usual in general. Since we have a symmetrical bimodal map, and there are two symmetrical kneading sequences, the total number of symbols in periodic sequences is even, , using the above notation. Therefore, the number of intervals is odd, . Moreover, since is always a fixed point we have always a Markov state associated to an interval containing , and a symmetry for the boundary points of the partition. Therefore, is natural to introduce the notation, for the Markov states enumeration:
with . Therefore, the Markov intervals, with this enumeration, are
Since the boundary points belong to the orbit and therefore is a Markov partition of for . The image of any interval in the partition, under , is a union of intervals in the partition. This fact allows the definition of the transition matrix, , given as usual
The topological entropy is given by the logarithm of the Perron eigenvalue of . Is also equal to the logarithm of the growth rate of the admissible words of size .
Each movement is composed of patches of linear motions, through the plane, intertwined by changes of direction. The lengths and the directions of each patch are determined, in the Cartesian coordinates, by the iteration of the map
In an explicit way,
The position of the animal is at each step given by the pair . On the other hand, the pair codifies the future action of the animal and correspond to the displacements at step . The parameter determines the characteristics of the motion and is associated to a kneading invariant, , for . The parameter gives the scale of the motion and can be related with the energy spent during the motion. It will not be important for now, regarding the classification of types of movements, and we only include it for sake of generality and future work. Note that the absolute values of the maximal displacements is normalized to . Therefore, re-scales whenever needed to the appropriate size. Recall that we assume there is a maximal step possible, for a given animal.
In resume, the initial position of the animal is and the initial conditions for the state of the system, which determines the trajectory, is the pair .
The parameter , as referred above, is related to the typical size of the animal. The parameter is related with the type of trajectory. It is a parameter which characterizes the complexity of the motion, the average displacement, average area covered and other geometrical and statistical characteristics of the trajectory.
An animal, in different circumstances or time instants, produce different trajectories. We expect that under the same exterior conditions and with the same internal conditions (health, metabolism, ...) the animal produce, not equal, however similar trajectories. In our model this corresponds to consider different initial state conditions (typical, not special points).
If the same animal is in a different internal state (with hunger, exhausted, more agammaessive, etc.) we may expect that produce different type trajectories. This means that different behavior states are associated with different values of . Therefore, a specie can be characterized by a set of possible parameters according to its observed behavior states and a different specie can have a set of possible parameters , with some in common. Since there are different values of the parameter producing topological equivalent behavior, we in fact need to make the correspondence between kneading sequences and behaviors. It is this correspondence that may be meaningful. We, thus, expect to have a specie characterized by a finite set of kneading sequences
ordered by topological entropy which coincides with the symbolic order, which may be associated with the typical behaviors according to internal and/or special controlled external conditions. For example, may represents the type of behavior more simple possible (for example rest), next codifies directed trajectories, travelling, represents search for food, and so on, and the last may codify a random type behavior.
The fixed points for are for every , and , for . If the fixed point is repulsive, if is attractive. On the other hand the fixed points , for , are repulsive and for are attractive.
If , with the initial state , the animal remains in . If starts in any will travel with a certain transient motion and will eventually stop.
If , with the initial state , the animal remains in , however, any small perturbation will lead the animal a progressive walk until approaches the attractive fixed point, one or the other. If starts with will travel only in the -direction, maintaining constant. If starts in any will perform a certain transient motion and at some point starts to walk in one definite direction (similar to taking a decision), either along the displacement vector or . Note that this is descriptive of the type of motion. If we pretend, for some practical purposes, a different stable direction we simply apply an appropriate rotation.
For the values , we have the intervals and invariant and, consequently, there are no changes of direction - the positive (resp. negative) values of displacements are always followed by positive (resp. negative) values of displacements. This does not happen for . Therefore, the region is associated with search or hesitant motion (higher topological entropy). The region has a sub case of two uncoupled unimodal maps defined on the invariant intervals and . This means that we may have complex behavior, with topological entropy between and . However, for these values of the parameter there is no turning back. The chaotic oscillations lead, in average, to a definite constant direction.
The region is associated with decided motion (lower or topological entropy).
In this case there is only the fixed point for every , except in the case for which are repulsive fixed points.
For the fixed point is attractive, for is repulsive. The critical points are inside for . In the interval there is a unique attractive orbit of period .
Therefore, If for the initial state with , the animal remains in . If starts in any will travel with a certain transient motion and will be rapidly stops.
If for the initial state with , the animal remains in , however any small perturbation will lead the animal a spiral walk until approaches the attractive period . Therefore, if starts in any will travel in a spiral until reaches a trajectory which closes in itself.
The map in the periodic case is structurally stable, and small perturbations on the parameter gives topological equivalent dynamics. From a experimental point of view these are important cases, since we expect to observe approximate behavior which is consistent and reproducible. If we observe empirically irregular motion, we expect to have approximate values of the parameter corresponding to very long periods with kneading sequences with a certain symbolic structure.
On the other hand, to determine at least a initial part of this symbolic sequence gives very valuable information, since gives us approximate value of the parameter and gives the possibility to determine approximate values of the topological entropy.
Note that periodic is not necessarily simple. If we consider very long periodic kneading sequence the behavior may be complex at some extend. For example
are admissible kneading sequences, relatively long and with some diversity regarding the type of constituent blocks. In this case, very small perturbation of the parameter gives the same kneading sequence except the symbols which are transformed in or . So we have some degree of stability in this complex/simple behavior. If, from observation, we obtain a long kneading sequence will not be clear if it is periodic or not, or which exact period is, since we must distinguish very small lengths to decide if we arrived to the critical points. However, this is not a problem because the topological entropy is continuous and monotone with respect to the parameter and the symbolic order. In the above sequence we could not identify and would consider it as a symbol , in this case what we collect empirically would be the sequence
and we could decide to finish it at any close point to , obtaining a very good characterization of the underlying dynamics.
The geometric structure of the patches of the trajectories are completely dependent on the patterns of the itineraries, and therefore dependent on the kneading sequences. The long blocks of the type means many consecutive small steps, (except the last two or three steps which are larger) increasing from the first to the last. This steps are in constant direction if , and in spiral if . The existence of blocks of the form or means change in direction on the corresponding axis. Therefore, sequences which have many blocks of those types are associated to trajectories with many turning points. On the other hand, sequences with blocks or means trajectories with steps maintaining the direction on that axis. A block is associated with a particular patch or pattern within a trajectory. A sequence which have many repeated blocks, eventually intertwined with other words correspond to trajectories with the pattern associated with the block repeated approximately. In the Figures 1–4, we see an example of perturbation of periodic kneading sequences. The square, associated with the period 4, is approximately repeated, through the perturbation. The parameter is , and produces the small changes in each case. The initial condition is, in every case, , . The kneading invariant, for the unperturbed case, , is .
In the Figure 5 another case, in which the kneading invariant is periodic, period 4, nevertheless, this case with higher topological entropy. Is given by
In this case we have the maximal value of the parameter or , with corresponding kneading invariant
The kneading sequences are not periodic, nevertheless have periodic tail (remind that the orbits of the critical points does not return to the critical points: , , , ). Any small perturbation of the parameter leads to non-equivalent topological behavior. There is also a Markov description which is simply given by the full-shift with states
Any word in the alphabet is allowed, the growth rate is and consequently the topological entropy is equal to . See the Figure 6, on the right side, where , very close to .
Let us consider the case . The critical points are , . Therefore, the intervals associated with the Markov partition , which coincide in this case with the partition of monotonicity of , through , , , are
The pre-images of the critical point are and the pre-images of the critical point are . Therefore, the intervals associated admissible words of size are
If we consider an itinerary for the initial displacement means that and that the next step will be in .
Numerical simulations shows that the average displacement in two dimension is, in this case, similar to the behavior of a random walk
In the Figure 7 we see a simulation showing the fit on the curve which gives the constant approximately equal to .
Let us focus on the case and that the intermittence refers to the symbol . We could consider any other word , , or even a block, which has a large occurrence rate in the itineraries of almost every initial condition repeated. Let us consider the following sub families of dynamics. Consider the values so that the kneading sequences are in the form
Recall that the form of one sequence implies the form of the other by the symmetry of .
In this case we obtain interesting examples of trajectories which are close to Levy walks: most of the time the iterates are in the interval If we have blocks of many repeated symbols , admissible, this mean that we have very frequent small displacements, interrupted by occasional larger displacements. If we assume that displacements smaller than a certain value must be disregarded, since are not observable, we have a distribution of lengths similar to a Levy distribution.
Consider the example in the Figure 8.
Let us analyze the case in detail.
Theorem 4. Let so that , with . Then the Markov state correspondence with admissible words is
with . The transition matrix is
of dimension . The topological entropy is the logarithm of the largest solution of the polynomial .
Proof. The boundary points are enumerated and ordered trough the shifts of the kneading sequences:
there is a permutation on the set which orders the previous sequences with respect to .
Therefore, the Markov partition is constituted by the intervals
The transitions can be explicitly computed, to obtain , through the semiconjugation of with the shift map:
Note the reversed order in this intervals , and since has negative derivative in those intervals. The polynomial is obtained using the expansion of the determinant of on the line , associated with the state .
Example 5. Let us consider the case described in the Theorem with . In this case the kneading invariant is and the Markov alphabet is . The parameter is approximately . The correspondence with admissible words are
The orbit of the critical point (and also of ) is, approximately
Reordered
The intervals
These are the possible lengths for each Markov state. This means for example, if we have then and since the only transition possible is to we know that the next step must be in .
In this section we illustrate the possible application of the model to movement in three dimension. The application of the model in 3D considers the same assumptions as the motion in the plane, in particular that the movement is composed of patches of linear motions, through the space, intertwined by changes of direction. Moreover, the characterization of the trajectory is accomplished by the parameter or the kneading invariant , and the parameter which gives the scale. The lengths and the directions of each patch are determined, in the Cartesian coordinates, by the iteration of the map
In an explicit way,
Let us consider a representative set of parameters, in the table below, considered also in examples and results in the two dimensional case. Note that the topological entropy refers to the corresponding interval map and characterizes both the and trajectories, for fixed . Note also that the kneading sequence is determined from by symmetry. See the Figures 9–14, for examples of trajectories for each case.
The author wishes to thanks the anonymous referee, whose comments and suggestions greatly improved this manuscript. This work was supported by FCT - Fundacao para a Ciencia e a Tecnologia under the project PEst-OE/MAT/UI0117/2014.
The author declares no conflict of interest.
[1] | P. E. Smouse, S. Focardi, P. R. Moorcroft, et al., Stochastic modelling of animal movement, Phil. Trans. R. Soc. B, 365 (2010), 2201–2211. |
[2] | R. Nathan, M. Getz, E. Revilla, et al., A movement ecology paradigm for unifying organismal movement research, Proc. Natl Acad. Sci. USA, 105 (2008), 19052–19059. |
[3] | W. Tang and D. A. Bennett, Agent-based modeling of animal movement: a review, Geography Compass, 4 (2010), 682–700. |
[4] | I. D. Jonsen, J. M. Flemming and R. A. Myers, Robust state–space modeling of animal movement data, Ecology, 86 (2005), 2874–2880. |
[5] | T. A. Patterson, L. Thomas, C. Wilcox, et al., State–space models of individual animal movement, Trends Ecol. Evol., 23 (2008), 87–94. |
[6] | B. T. McClintock, R. King, L. Thomas, et al., A general discrete-time modeling framework for animal movement using multistate random walks, Ecol. Monogr., 82 (2012), 335–349. |
[7] | R. Langrock, R. King, J Matthiopoulos, et al., Flexible and practical modeling of animal telemetry data: hidden Markov models and extensions, Ecology, 93 (2012), 2336–2342. |
[8] | S. Petrovskii, A. Mashanova and V. Jansen, Variation in individual walking behavior creates the impression of a Lévy flight, PNAS, 108 (2011), 8704–8707. |
[9] | P. Tilles, S. Petrovskii and P. Natti, A random walk description of individual animal movement accounting for periods of rest, R. Soc. Open Sci., 3 (2016), 28018645. |
[10] | J. P. Lampreia and J. Sousa Ramos, Symbolic dynamics of bimodal maps, Portugal. Math., 54 (1997), 1–18. |
[11] | J. P. Lampreia, R. Severino and J. Sousa Ramos, Renormalizations for cubic maps, Ann. Math. Silesianae, 13 (1999), 257–270. |
[12] | J. P. Lampreia, R. Severino and J Sousa Ramos, Irreducible complexity of iterated symmetrical bimodal maps, Discrete Dyn. Nat. Soc., 1 (2005), 69–85. |
[13] | J. Milnor and C. Tresser, On entropy and monotonicity of real cubic maps, Stony Brook, IMS 1998/9 (1998), arXiv:math/9809096. |
1. | Carlos Correia Ramos, Kinematics in Biology: Symbolic Dynamics Approach, 2020, 8, 2227-7390, 339, 10.3390/math8030339 |