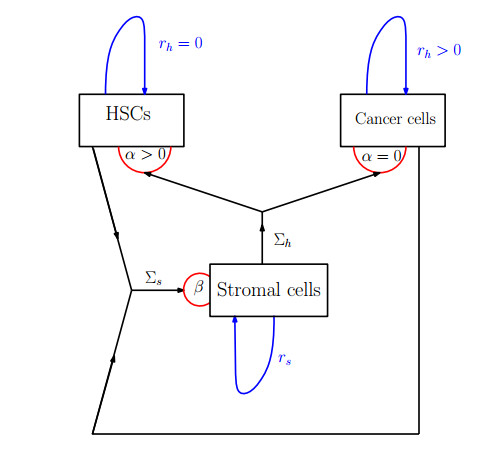
Citation: Thanh Nam Nguyen, Jean Clairambault, Thierry Jaffredo, Benoît Perthame, Delphine Salort. Adaptive dynamics of hematopoietic stem cells and their supporting stroma: a model and mathematical analysis[J]. Mathematical Biosciences and Engineering, 2019, 16(5): 4818-4845. doi: 10.3934/mbe.2019243
[1] | Fabien Crauste . Global Asymptotic Stability and Hopf Bifurcation for a Blood Cell Production Model. Mathematical Biosciences and Engineering, 2006, 3(2): 325-346. doi: 10.3934/mbe.2006.3.325 |
[2] | R. A. Everett, Y. Zhao, K. B. Flores, Yang Kuang . Data and implication based comparison of two chronic myeloid leukemia models. Mathematical Biosciences and Engineering, 2013, 10(5&6): 1501-1518. doi: 10.3934/mbe.2013.10.1501 |
[3] | Qiaojun Situ, Jinzhi Lei . A mathematical model of stem cell regeneration with epigenetic state transitions. Mathematical Biosciences and Engineering, 2017, 14(5&6): 1379-1397. doi: 10.3934/mbe.2017071 |
[4] | Katrine O. Bangsgaard, Morten Andersen, Vibe Skov, Lasse Kjær, Hans C. Hasselbalch, Johnny T. Ottesen . Dynamics of competing heterogeneous clones in blood cancers explains multiple observations - a mathematical modeling approach. Mathematical Biosciences and Engineering, 2020, 17(6): 7645-7670. doi: 10.3934/mbe.2020389 |
[5] | Awatif Jahman Alqarni, Azmin Sham Rambely, Sana Abdulkream Alharbi, Ishak Hashim . Dynamic behavior and stabilization of brain cell reconstitution after stroke under the proliferation and differentiation processes for stem cells. Mathematical Biosciences and Engineering, 2021, 18(5): 6288-6304. doi: 10.3934/mbe.2021314 |
[6] | Samantha L Elliott, Emek Kose, Allison L Lewis, Anna E Steinfeld, Elizabeth A Zollinger . Modeling the stem cell hypothesis: Investigating the effects of cancer stem cells and TGF−β on tumor growth. Mathematical Biosciences and Engineering, 2019, 16(6): 7177-7194. doi: 10.3934/mbe.2019360 |
[7] | Dane Patey, Nikolai Mushnikov, Grant Bowman, Rongsong Liu . Mathematical modeling of population structure in bioreactors seeded with light-controllable microbial stem cells. Mathematical Biosciences and Engineering, 2020, 17(6): 8182-8201. doi: 10.3934/mbe.2020415 |
[8] | Jihong Yang, Hao Xu, Congshu Li, Zhenhao Li, Zhe Hu . An explorative study for leveraging transcriptomic data of embryonic stem cells in mining cancer stemness genes, regulators, and networks. Mathematical Biosciences and Engineering, 2022, 19(12): 13949-13966. doi: 10.3934/mbe.2022650 |
[9] | J. Ignacio Tello . On a mathematical model of tumor growth based on cancer stem cells. Mathematical Biosciences and Engineering, 2013, 10(1): 263-278. doi: 10.3934/mbe.2013.10.263 |
[10] | Rujing Zhao, Xiulan Lai . Evolutionary analysis of replicator dynamics about anti-cancer combination therapy. Mathematical Biosciences and Engineering, 2023, 20(1): 656-682. doi: 10.3934/mbe.2023030 |
Hematopoietic stem cells (HSCs), developing in the bone marrow, are immature cells that are (the earliest in development) precursors of all lineages of blood cells: red blood cells, white blood cells and megacaryocytes (whose fragmentation gives rise to platelets). Blood cell formation, also called hematopoiesis, is a complex phenomenon basing on the self-renewal, differentiation and maturation of HSCs. It produces about 1011 blood cells per day in humans and is one of the most stable biological processes in vertebrate organisms. A dysfunction in the hematopoietic process may induce blood cancer diseases (usually named malignant hemopathies) such as leukaemia where blockade of maturation and of differentiation occurs in the hematopoietic tree. As a consequence, malignant cells, resulting from an accumulation of irregular genetic events, appear and proliferate abnormally.
Many mathematical models have been proposed to understand blood cell development and blood diseases. Mackey [1], inspired by Burns and Tannock [2] and Lajtha [3], have introduced a first mathematical model of the form of a system of delay differential equations for the dynamics of HSCs where the populations are divided into two groups (proliferating cells and quiescent cells) and the time delay corresponds to the proliferating phase duration. Further improvements both in modelling and mathematical analysis are investigated by many authors; see, for example, [5,6,7,8]—models in the form of ODEs or age-structured transport equations with applications to chronic myelogenous leukaemia, [9]—a diffusion model including spatial competition between cells–, reviews [4,10,11] and the references therein.
Despite extensive studies on the dynamics of HSCs and diseases of the hematopoietic system, none of the above-mentioned models takes into account the interactions between HSCs and the hematopoietic niche which is a specific microenvironment ensuring the maintenance and regulation of HCSs locally. It is worth noting that the interactions between HSCs and their niche, of which mesenchymal stem cells (MSCs) are the most important component, play a crucial role in the formation of mature blood cells. Also, alterations in the bidirectional exchanges between HSCs and MSCs may give rise among HSCs to blood cancer stem cells, i.e., leukemic stem cells, LSCs.
Note that healthy HSCs need the close presence of stromal cells for their development but stromal cells can proliferate without HSCs. Similar to HSCs, cancer cells in the early stages need stromal cells for their development whereas in the later stages they can proliferate without support cells. In other words, the more malignant a cell is, the more independent of stromal cells it is. Here, cancer cells in earlier stages are cells with few mutation events and cancer cells in later stages stand for the ones with more mutation events. We refer to, for example, [12,13,14] for reviews of the interaction between HSCs and stromal cells, [15] for acute myeloid leukemic cells.
In the present paper, we introduce a mathematical model for the interaction between HSCs and stromal cells with the aim to better understand the nature of the dialogue between them as well as their dynamics. We also perform a mathematical analysis for the long-time behaviour of the hepatopoietic and stromal cells in the framework of adaptive dynamics. Our mathematical model and some notions in the framework of adaptive dynamics, in particular, evolutionary stable distributions (ESDs) are given in the remaining part of this section.
Let nh(t,x) be the population density of hematopoietic stem cells (HSCs) and cancer cells at time t with phenotype x, continuous structure variable assumed to characterise the population heterogeneity in a way relevant to the question at stake. Here x will represent a malignancy potential of HSCs, from its minimal (representing a totally healthy state) to its maximal value (representing maximum malignancy), considered independently of their stromal support. From a biological point of view, x might represent a pathological combination of both high plasticity (i.e., ability to change phenotype; stem cells are plastic, but physiologically, they not proliferate much) and fecundity (i.e., ability to proliferate; differentiated cells are able to proliferate, but physiologically, they show little plasticity). Let ns(t,y) be their corresponding stromal cell population density - that we will sometimes call support cells - at time t and with phenotype y (here the continuous phenotype variable y will denote the supporting capacity of MSCs to HSCs). Assume for simplicity that x and y are real variables with x∈(a,b),y∈(c,d), where 0<a<b and 0<c<d. Totally healthy HSCs will thus have a phenotype x close to a, while agammaessive leukemic HSCs (i.e., LSCs) will have a phenotype x close to b. We consider a mathematical model of the form
{∂tnh(t,x)=[rh(x)−ρh(t)−ρs(t)+α(x)Σs(t)]nh,x∈(a,b),t>0,∂tns(t,y)=[rs(y)−ρh(t)−ρs(t)+β(y)Σh(t)]ns,y∈(c,d),t>0. | (1.1) |
This system is completed with initial data
nh(0,x)=nh0(x)≥0,ns(0,y)=ns0(y)≥0. | (1.2) |
Here our assumptions and notations are
● ρh(t):=∫banh(t,x)dx,ρs(t):=∫dcns(t,y)dy are the total populations of HSCs and their support cells, respectively.
● The terms Σh(t):=∫baψh(x)nh(t,x)dx,Σs(t):=∫dcψs(y)ns(t,y)dy denote an assumed chemical signal (Σh) from the hematopoietic immature stem cells (HSCs) to their supporting stroma (MSCs), i.e., ``call for support'' and conversely, a trophic message (Σs) from MSCs to HSCs. The cytokine stem cell factor (SCF) and the C-X-C motif chemokine ligand 12 (CXCL12) are typical examples for such supporting messages [13,14]. The nonnegative functions ψh,ψs defined on (a,b) and (c,d) measure the contribution of each phenotype in the interactive messages. Assume that ψ′s≥0 (the higher the support phenotype in MSCs, the stronger the trophic message to HSCs); in the same way, unless otherwise specified, we shall assume that ψ′h≥0.
● The term rh≥0 represents the intrinsic (i.e., without contribution from trophic messages from MSCs, nor limitation by the non local logistic terms ρh and ρs, that represent competition for space and nutrients within the whole population of cells) proliferation rate of HSCs. Assume that rh is non-decreasing (the more malignant, the more proliferative), rh(a)=0 and rh(b)>0.
● The term α≥0, satisfying α′≤0 and α(b)=0, is the sensitivity of HSCs to the trophic messages from support cells
● For the term rs≥0, we assume that r′s(y)≤0 (there is a cost in proliferation for support cells to increase their support capacity). The term β(y)≥0 with β′(y)≥0 represents the sensitivity of the stromal cells MSCs to the (call for support) message coming from HSCs.
System (1.1) falls within the broader class of models for interacting populations where competitive, prey-predator and cooperative types are typical examples of such interaction; see, for example, [27,Chapter 3]. Apart from the cases mentioned in [27,Chapter 3], in the context of adaptive dynamics, the populations are often structured by phenotypical traits to take into account the heterogeneity in the population (e.g. [16]). We refer to [17] a related competitive system with healthy and cancer cells structured by a phenotypic variable related with their resistance to chemotherapy, to [18] an integro-differential Lotka-Volterra system for the interaction of N populations (N≥2). In our model, besides the competition terms between cells, we introduce new terms Σh,Σs,α,β to represent the interacting messages between HSCs and stromal cells. The presence of these terms makes the problem difficult to study since the nature of (1.1) is unknown and may vary in time. It could be competitive, co-operative or other types depending on the sign of the terms −ρs(t)+α(x)Σs(t) and −ρh(t)+β(y)Σh(t). Note that if Σh=Σs=0, our model reduces to the cases studied in [16,17]. Also when ψh=ψs=1, our problem becomes a particular case of [18].
Let us briefly sum up the meaning of our assumptions. From a biological point of view, the healthy HSCs cannot proliferate without support cells while cancer cells persist even without support cells. In our model nh(t,a) corresponds to healthy HSCs and nh(t,b) are leukemic cells since the intrinsic proliferation rate rh satisfies rh(a)=0,rh(b)>0. The monotonicity of rh implies that the higher x is, the more agammaessive is a HSC (in fact, a LSC, since it is then cancerous). Also the monotonicity of α (this function stands for the sensitivity of HSCs to the trophic messages coming from MSCs) indicates that the more agammaessive x is, the less sensitivity has a HSC to the trophic message sent by MSCs (i.e., the more independent it is from the surrounding stroma). Moreover, the condition α(b)=0 shows that n(t,b) (i.e., cancer cells in the latter stages) proliferate independently of the supporting stroma. Furthermore, the monotonicity of rs,β shows that the more supporting stromal capacity MSCs have, the less they proliferate and the more sensitive to messages from HSCs they are.
As a simple case, the parameters rh,α,rh,rh,ψh,ψs can be chosen as linear or quadratic functions. For example, rh, α are given by rh=r∗h(x−a) or rh=r∗h(x−a)2, α(x)=α∗(b−x) with positive constants r∗h,α∗, ψh(x)=x, ψs(y)=y.
A more general model which includes the possibility of mutations has the form:
{∂tnh(t,x)=μh(nh)xx+[rh(x)−c11(x)ρh(t)−c12(x)ρs(t)+α(x)Σs(t)]nh,∂tns(t,y)=μs(ns)yy+[rs(y)−c21(y)ρh(t)−c22(y)ρs(t)+β(y)Σh(t)]ns. | (1.3) |
Here the diffusion terms represent mutation with rates μh,μs and c11,c12,c22,c22 measure the strength of competition between cells. Problem (1.3) reduces to (1.1) by setting μh=μs=0 and c11(x)=c12(x)=1,c21(y)=c22(y)=1. Thus (1.1) can be considered as a good approximation of (1.3) in the regime μh,μs<<1 which is realistic since mutations occur rarely in physiology (to fix ideas, let us say between once every 106 and 109 cell divisions; of course, in evolved cancers, such low rates may increase).
Hereafter, we will use the notation ∫ to denote the integrals over [a,b] and [c,d], as long as there is no risk of confusion. Let ˆnh,ˆns be measures defined on [a,b],[c,d], respectively. We set
supp ˆnh=I⊂[a,b],supp ˆns=J⊂[c,d], |
ˆρh=∫ˆnh,ˆρs=∫ˆns,ˆΣh=∫ψh(x)ˆnh,ˆΣs=∫ψs(y)ˆns, |
Gh(x):=rh(x)−ˆρh−ˆρs+α(x)ˆΣs,Gs(y):=rs(y)−ˆρh−ˆρs+β(y)ˆΣh. | (1.4) |
Definition 1.1. The pair (ˆnh,ˆns) is a steady state of (1.1) if
Gh(x)=0,Gs(y)=0 for all x∈I,y∈J. | (1.5) |
Furthermore, ˆnh (resp. ˆns) is said
(ⅰ) to be monomorphic if supp ˆnh (resp. supp ˆns) is a singleton,
(ⅱ) to be dimorphic if supp ˆnh (resp. supp ˆns) is a set of two points.
Definition 1.2. We say that (ˆnh,ˆns) is an evolutionary stable distribution (ESD) of problem (1.1) if it is a steady state and the condition below is fulfilled
Gh(x)≤0 for all x∈[a,b]∖I,Gs(y)≤0 for all y∈[c,d]∖J. | (1.6) |
Remark 1.3. It follows from Definition 1.2 that any x∈I (resp. y∈J) is a maximum point of Gh (resp. Gs).
As our equation arises from biological cell population dynamics, we only consider non-negative steady states and ESDs in this paper.
In the context of adaptive dynamics, when (1.5) holds, we say that the phenotypes x∈I,y∈J are living in the stationary environment given by (ˆρh,ˆρs,ˆΣh,ˆΣs). The functions Gh,Gs are fitness functions associated with this environment. The quantities Gh(x),Gs(y) are growth rates of phenotypes x,y and they tell us whether (x,y) can invade this environment: If Gh(x)>0,Gs(y)>0, (x,y) can grow and the system will reach a new equilibrium. We refer to [20,25] for more details about the framework of adaptive dynamics.
We first present mathematical results to verify biological properties concerning the independence of stromal cells on HSCs and its vital support to HSCs in Section 2.1. A uniform bound in time and a well-posedness result are given in Section 2.2.
As generally only a finite number of traits is represented in the equilibrium, we study, for simplicity, in Section 3, equilibria with only one trait, i.e., those of the form of single Dirac mass. The linear stability result of single Dirac mass steady states (Theorem 3.2) exhibits the mechanisms by which another trait can or cannot invade the stationary state produced by a given trait.
ESDs—equilibria corresponding to optimal states of the evolution—are studied in Section 4. We study the impact of the parameters on the form of ESDs. Two cases are investigated: monomorphic situation (i.e., only one trait is represented in ESDs) and dimorphic one (two traits are represented in ESDs). More precisely, we provide sufficient conditions to guarantee that all ESDs are monomorphic or dimorphic (Proposition 4.1). Also in Theorem 4.2 we obtain a result on the uniqueness of ESDs and we show that this unique ESD is monomorphic. Another result on the uniqueness of ESD (Theorem 4.5) hold for rather than general functions rh,rs and under some homogeneity assumptions of stromal cells. This theorem is concerned with more general ESDs which are not necessary to be monomorphic or dimorphic.
Section 5 is concerned with dominant traits which are the best adapted ones to the environment and favored at high population densities. These traits are represented by maximum points of the population densities and change in time because of the variation of environment. We study the movement of these traits in a long time scale, hence make the change of variable, τ=εt, with small ε>0. We represent the dynamics of dominant traits (Theorems 5.1 and 5.2) in the regime as ε→0 (asymptotic analysis). Also we obtain the equation for dominant traits Eq (5.11).
In Section 6, we provide numerical simulations to illustrate our results and finally, some discussions are given in Section 7.
In the absence of stromal cells, the system (1.1) reduces to the equation
∂tnh(t,x)=[rh(x)−ρh(t)]nh,x∈(a,b),t>0. | (2.1) |
Similarly, the behaviour of stromal cells without HSCs is given by
∂tns(t,y)=[rs(y)−ρs(t)]ns,y∈(c,d),t>0. | (2.2) |
In view of [28,Theorem 2.1,Page 29], we have the following selection principle:
Lemma 2.1. Suppose that rh is bounded and strictly increasing. Suppose furthermore that rs is bounded and strictly decreasing. Assume that nh0,ns0∈L1 are positive on [a,b] and [c,d], respectively.
(ⅰ) For (2.1), we have nh(t,x)→rh(b)δ{x=b} weakly in the sense of measures as t→∞.
(ⅱ) For (2.2), we have nh(t,y)→rs(c)δ{y=c} weakly in the sense of measures as t→∞.
These results confirm biological properties mentioned in Section 1 about the bi-directional interaction between HSCs and stromal cells. More precisely, in the absence of stromal cells for HSCs or of HSCs for stromal cells, the phenotypes of nh and ns, respectively, behave as monomorphic. Moreover, Lemma 2.1(ⅰ) implies that healthy hematopoietic cells eventually go extinct while cancer cells (with the phenotype y=b) are selected and persist. Furthermore, stromal cells persist without HSCs and stromal cells with the lowest capacity of support will be selected (cf. Lemma 2.1(ⅱ)).
For a function f defined on an interval I, we set
f_:=minx∈If(x),¯f:=maxx∈If(x). |
In the results below, we only need the boundedness of rh,rs,α,β,ψh,ψs. We do not need the monotonicity assumption of these functions.
Proposition 2.2. Assume that rh,rs,α,β,ψh,ψs are non-negative and bounded. Suppose furthermore that
ˉαˉψs+ˉβˉψh<4. | (2.3) |
Set
ρM:=max(ρh0+ρs0,4max(ˉrh,ˉrs)4−(ˉαˉψs+ˉβˉψh)). |
Then the solution of (1.1)- (1.2) is non-negative and satisfies
0≤ρh(t)+ρs(t)≤ρMfor all t≥0. | (2.4) |
Proof. First note that the solution of (1.1) can be written in the form
nh(t,x)=nh0(x)exp(∫t0A(σ,x)dσ),ns(t,y)=ns0(y)exp(∫t0B(σ,y)dσ), |
where
A(σ,x)=rh(x)−ρh(σ)−ρs(σ)+α(x)Σs(σ),B(σ,y)=rs(y)−ρh(σ)−ρs(σ)+β(y)Σh(σ). |
Thus nh(t,x)≥0,ns(t,y)≥0 since nh0(x)≥0,ns0(y)≥0. This yields the first inequality of (2.4).
Integrating the two equations in (1.1) yields
ddtρh=−ρ2h−ρhρs+∫rh(x)nh+Σs(t)∫α(x)nh,ddtρs=−ρ2s−ρhρs+∫rs(y)ns+Σh(t)∫β(y)ns. |
Summing up these two identities and using the non-negativity of nh,ns, we obtain
ddt(ρh+ρs)=−(ρh+ρs)2+∫rh(x)nh+Σs(t)∫α(x)nh+∫rs(y)ns+Σh(t)∫β(y)ns≤−(ρh+ρs)2+max(ˉrh,ˉrs)(ρh+ρs)+ˉαˉψsρhρs+ˉβˉψhρhρs≤−(ρh+ρs)2+max(ˉrh,ˉrs)(ρh+ρs)+ˉαˉψs+ˉβˉψh4(ρh+ρs)2=[max(ˉrh,ˉrs)−(1−ˉαˉψs+ˉβˉψh4)(ρh+ρs)](ρh+ρs). |
Hence the second inequality of (2.4) follows.
Proposition (2.2) and a standard argument imply the following result.
Theorem 2.3. Let (nh0,ns0)∈L1(a,b)×L1(c,d) be non-negative. Then (1.1) possesses a unique solution (nh,ns)∈C1([0,∞);L1(a,b)×L1(c,d)).
Hereafter, for simplicity, we assume that ψh(x)=x,ψs(y)=y. Then Problem (1.1) becomes
{∂tnh(t,x)=[rh(x)−ρh(t)−ρs(t)+α(x)∫yns(t,y)dy]nh,x∈(a,b),t>0,∂tns(t,y)=[rs(y)−ρh(t)−ρs(t)+β(y)∫xnh(t,x)dx]ns,y∈(c,d),t>0. | (3.1) |
Let ˆx∈[a,b],ˆy∈[c,d]. Consider a particular case where hematopoietic and support cells evolve as single Dirac masses concentrated at ˆx,ˆy. In other words, we focus on the behaviour of the size of the populations. In this case, nh,ns have the form
nh(t,x)=ρh(t)δ{x=ˆx},ns(t,y)=ρs(t)δ{y=ˆy}, |
where ρh(t),ρs(t) satisfy
{ddtρh=(rh(ˆx)−ρh−(1−α(ˆx)ˆy)ρs)ρh,ddtρs=(rs(ˆy)−(1−β(ˆy)ˆx)ρh−ρs)ρs. | (3.2) |
We can obtain an explicit form of single Dirac mass steady states.
Lemma 3.1. Let ˆx∈[a,b],ˆy∈[c,d] and assume that 1−(1−α(ˆx)ˆy)(1−β(ˆy)ˆx)≠0. Set
ˆρh:=rh(ˆx)−rs(ˆy)(1−α(ˆx)ˆy)1−(1−α(ˆx)ˆy)(1−β(ˆy)ˆx),ˆρs:=rs(ˆy)−rh(ˆx)(1−β(ˆy)ˆx)1−(1−α(ˆx)ˆy)(1−β(ˆy)ˆx). | (3.3) |
Then (ˆnh=ˆρhδ{x=ˆx},ˆns=ˆρsδ{y=ˆy}) is a steady state of problem (3.1). If the three conditions below hold
{1−(1−α(ˆx)ˆy)(1−β(ˆy)ˆx)>0,rh(ˆx)−rs(ˆy)(1−α(ˆx)ˆy)>0,rs(ˆy)−rh(ˆx)(1−β(ˆy)ˆx)>0, | (3.4) |
then ˆρh>0,ˆρs>0 and (ˆρh,ˆρs) is a linearly stable steady state of (3.2).
Proof. Single Dirac mass steady state solution yields
{rh(ˆx)−ˆρh−ˆρs+α(ˆx)ˆyˆρs=0,rs(ˆy)−ˆρh−ˆρs+β(ˆy)ˆxˆρh=0. | (3.5) |
An elementary calculation shows that (ˆρh,ˆρs) is a steady state of (3.2) and the corresponding Jacobian matrix is given by
J=(−ˆρh−(1−α(ˆx)ˆy)ˆρh−(1−β(ˆy)ˆx)ˆρs−ˆρs). | (3.6) |
In view of (3.4), tr(J)<0,det(J)>0 so that the two eigenvalues of J are negative. Thus the linear stability of (ˆρh,ˆρs) follows.
The results below are concerned with the linear stability of single Dirac mass steady states among perturbations of particular forms.
Theorem 3.2 (Stability of monomorphic steady states). Let (ˆnh=ˆρhδ{x=ˆx},ˆns=ˆρsδ{y=ˆy}) be a steady state of (3.1) as in Lemma 3.1. Assume that (3.4) holds. Let x∗,y∗ satisfy x∗≠ˆx,y∗≠ˆy and
Gh(x∗)<0,Gs(y∗)<0. | (3.7) |
Then (ˆnh,ˆns) is linearly stable among perturbations starting by
nh0:=ε1δ{x=x∗}+(ˆρh+ε2)δ{x=ˆx},ns0:=ε3δ{y=y∗}+(ˆρs+ε4)δ{y=ˆy}. |
Proof. We linearize the system at (ˆnh,ˆns). For gh(t,x):=nh(t,x)−ˆnh(x),gs(t,y):=ns(t,y)−ˆns(y) we obtain
{∂tgh=[rh(x)−ˆρh−ˆρs+α(x)∫yˆns]gh−ˆnh∫gh+ˆnh[−∫gs+α(x)∫ygs],∂tgs=[−∫gh+β(y)∫xgh]ˆns+[rs(y)−ˆρh−ˆρs+β(y)∫xˆnh]gs−ˆns∫gs. |
Note that gh,gs have the form
gh(t,x)=g1h(t)δ{x=x∗}+g2h(t)δ{x=ˆx},gs(t,y)=g1s(t)δ{y=y∗}+g2s(t)δ{y=ˆy}. |
Therefore, we obtain
{∂tg1h=[rh(x∗)−ˆρh−ˆρs+α(x∗)ˆyˆρs]g1h,∂tg1s=[rs(y∗)−ˆρh−ˆρs+β(y∗)ˆxˆρh]g1s,∂tg2h=−ˆρhg1h+ˆρh[α(ˆx)y∗−1]g1s−ˆρhg2h+ˆρh(α(ˆx)ˆy−1)g2s,∂tg2s=ˆρs(β(ˆy)x∗−1)g1h−ˆρsg1s+ˆρs(β(ˆy)ˆx−1)g2h−ˆρsg2s. |
The corresponding matrix is given by
(rh(x∗)−ˆρh−ˆρs+α(x∗)ˆyˆρs0000rs(y∗)−ˆρh−ˆρs+β(y∗)ˆxˆρh00−ˆρhˆρh[α(ˆx)y∗−1]−ˆρhˆρh(α(ˆx)ˆy−1)ˆρs(β(ˆy)x∗−1)−ˆρsˆρs(β(ˆy)ˆx−1)−ˆρs). |
The eigenvalues of the above matrix are
rh(x∗)−ˆρh−ˆρs+α(x∗)ˆyˆρs,rs(y∗)−ˆρh−ˆρs+β(y∗)ˆxˆρh |
and the two eigenvalues of the matrix J in (3.6). All are negative due to (3.7) and Lemma 3.1. Thus the stability of (ˆnh,ˆns) follows.
Interpretations of Theorem 3.2: With the notation (1.4), in view of (3.5), we have Gh(ˆx)=0,Gs(ˆy)=0. Also the conditions Gh(x∗)<0,Gs(y∗)<0 show that the phenotypes x∗,y∗ cannot invade the stable equilibrium (ˆnh,ˆns). As a consequence, no new equilibrium can be reached but a mutant invading the resident population (ˆx,ˆy).
Recall that from the defintion 1.2, an ESD (ˆnh,ˆns) is characterised by the conditions (1.5)– (1.6). Graphically, we plot the curve x∈[a,b]↦(Z=α(x),W=rh(x)) by the blue curve and the red straight line ZˆΣs+W=ˆρh+ˆρs; see Figure 2. Then the conditions (1.5)– (1.6) for ˆnh mean that the blue curve must be below the red line and that the pair (α(x),rh(x)) for all x∈I:=supp ˆnh are the coordinates of the intersection points between the blue curve and the red line. Similarly, we have the same illustration for ˆns.
If α (resp. rh) is strictly monotone and if rh(α−1) (resp. α(r−1h)) is concave on [0,α(a)] (resp. [0,rh(b)]). Then there is at most one intersection point satisfying the conditions (1.5)– (1.6) for ˆnh. Thus, the strict monotonicity of α implies that I is a singleton, hence ˆnh is monomorphic. In the case where rh(α−1(z)) (resp. α(r−1h)) is convex, I contains at most two points, thus ˆnh is at most dimorphic.
Note that by Remark 1.3, we can also check if an ESD is monomorphic or dimorphic by studying the set of maximum points of the corresponding fitness functions. We state the above results in the following proposition.
Proposition 4.1 (Conditions for monomorphism or dimorphism). Assume that (ˆnh,ˆns) is an ESD arbitrarily that does not vanish. Then ˆnh is monomorphic if one of the following hypotheses is fulfilled:
(ⅰ) either α is strictly monotone and rh(α−1) is concave on [0,α(a)],
(ⅱ) or rh is strictly monotone and α(r−1h)) is concave on [0,rh(b)],
(ⅲ) or rh,α are strictly concave.
Also ˆnh is at most dimorphic if one of the following hypotheses is fulfilled:
(ⅰ) either α is strictly monotone and rh(α−1) is convex on [0,α(a)],
(ⅱ) or rh is strictly monotone and α(r−1h)) is convex on [0,rh(b)],
(ⅲ) or rh,α are strictly convex.
Furthermore, the same conclusions as above hold for ˆns provided that similar assumptions on rs,β are supposed.
The next result is concerned with the existence and uniqueness of ESDs. We also show that the unique ESD is monomorphic and that the concentration points are endpoints of the intervals (a,b),(c,d). For simplicity, set (a,b):=(1,2),(c,d):=(3,4). Here we employ the two distinct sets (1,2) and (3,4) to insist on that fact that the two phenotypes of HSCs and of stromal cells are not the same. We will suppose two of the following assumptions:
(H1) r′h(x)+3α′(x)ˉrs<0 for all x∈(1,2) and β(y)≥1 for all y∈(3,4),
(H2) r′h(x)+4α′(x)ˉrs>0 for all x∈(1,2) and β(y)≤1/2 for all y∈(3,4),
(H3) β=β∗>0 is constant and rs is strictly decreasing,
(H4) β is strictly increasing and rs=r∗s>0 is constant.
Theorem 4.2 (Existence and uniqueness of ESDs). Set (a,b):=(1,2),(c,d):=(3,4). Suppose that rh,α∈C([1,2])∩C1((1,2)),rs,β∈C([3,4])∩C1((3,4)). Suppose furthermore that the pair (ˆnh,ˆns) below is non-negative and that the assumptions (depending on situations) mentioned below hold. Then there exists a unique (non-negative) ESD and it is monomorphic or vanishes.
(ⅰ) Under the assumptions (H1) and (H3), the unique ESD is given by
ˆnh=rs(3)(3α(1)−1)1+(3α(1)−1)(1−β∗)δ{x=1},ˆns=rs(3)1+(3α(1)−1)(1−β∗)δ{y=3}. |
(ⅱ) Under the assumptions (H2) and (H3), the unique ESD is given by
ˆnh=rh(2)−rs(3)2β∗δ{x=2},ˆns=rs(3)−rh(2)(1−2β∗)2β∗δ{y=3}. |
(ⅲ) Under the assumptions (H1) and (H4), the unique ESD is given by
ˆnh=r∗s(4α(1)−1)1+(4α(1)−1)(1−β(4))δ{x=1},ˆns=r∗s1+(4α(1)−1)(1−β(4))δ{y=4}. |
(ⅳ) Under the assumptions (H2) and (H4), the unique ESD is given by
ˆnh=rh(2)−r∗s2β(4)δ{x=2},ˆns=r∗s−rh(2)(1−2β(4))2β(4)δ{y=4}. |
Proof. We only prove (ⅰ). The other cases can be proved in the same way. First note that under the assumptions (H1) and (H3), β=β∗≥1. We have Gs(y)=rs(y)−ˆρh−ˆρs+β∗ˆΣh is strictly decreasing (by (H3)) so that it attains its global maximum only at y=3. This, in view of Remark 1.3, yields that supp ˆns={3}. As a consequence, Gs(3)=0 so that
rs(3)−ˆρs=ˆρh−β∗∫21xˆnh≤ˆρh−β∗∫21ˆnh=ˆρh−β∗ˆρh≤0. |
Therefore,
3rs(3)≤3ˆρs≤∫43yˆns=ˆΣs. |
This together with the property that α′≤0 implies that
G′h(x)=r′h(x)+α′(x)ˆΣs≤r′h(x)+3α′(x)rs(3)<0 for allx∈(1,2), |
where we used the hypothesis (H1) in the last inequality. Consequently, Gh is strictly decreasing on [1,2] so that it has only one maximum point x=1. Hence supp ˆnh={1}. The expression of (ˆnh,ˆns) follows from (3.3) with (ˆx,ˆy):=(1,3).
Below, we compute all ESDs explicitly. We also see that the dimorphic situation may occurs. For simplicity, we only consider the dimorphic distribution for hematopoietic stem cells.
Proposition 4.3 (Explicit formulas of all ESDs). Set (a,b):=(1,2),(c,d):=(3,4). Assume that rh is strictly convex and that α is convex. Suppose furthermore that (H3) is satisfied (i.e., β=β∗ is a positive constant and rs is strictly decreasing). Then all ESDs are given by
(ⅰ) (ˆnh=ˆρhδ{x=1},ˆns=ˆρsδ{y=3}) with
ˆρh=rs(3)(3α(1)−1)1+(3α(1)−1)(1−β∗),ˆρs=rs(3)1+(3α(1)−1)(1−β∗), |
provided that ˆρh≥0,ˆρs≥0 and
rh(2)≤3α(1)rs(3)1+(3α(1)−1)(1−β∗). | (4.1) |
(ⅱ) (ˆnh=ˆρhδ{x=2},ˆns=ˆρsδ{y=3}) with
ˆρh=rh(2)−rs(3)2β∗,ˆρs=rs(3)−rh(2)(1−2β∗)2β∗, |
provided that ˆρh≥0,ˆρs≥0 and
rh(2)≥3α(1)rs(3)−rh(2)(1−2β∗)2β∗. |
(ⅲ) (ˆnh=ˆρh1δ{x=1}+ˆρh2δ{x=2},ˆns=ˆρsδ{y=3}) with
ˆρh1=2rh(2)3α(1)−13α(1)−rh(2)−rs(3)β∗,ˆρh2=rh(2)−rs(3)β∗−rh(2)3α(1)−13α(1),ˆρs=rh(2)3α(1), |
provided that ˆρh1≥0,ˆρh2≥0,ˆρs≥0.
Remark 4.4. We can also prove similar results as in Proposition 4.3 when we suppose the hypothesis (H4) instead of (H3).
Proof. First note that Gh(x) is strictly convex. Thus Gh attains its global maximum only at endpoints of the interval [1,2]. Note also that Gs(y)=rs(y)−ˆρh−ˆρs+β∗ˆΣh is strictly decreasing so that y=3 is its unique maximum point. The above observations and Remark 1.3 imply that supp ˆns=3 and either supp ˆnh={1} or supp ˆnh={2} or supp ˆnh={1,2}.
(ⅰ) The case supp ˆnh={1},supp ˆns={3}. The expressions of ˆρh,ˆρs follows from (3.3) with ˆx=1,ˆy=3. Because of the convexity of Gh and the decreasing monotonicity of Gs, the condition (1.6) is equivalent to Gh(1)≥Gh(2) which implies (4.1). Similarly, Item (ⅱ) — the case supp ˆnh={2},supp ˆns={3} — can be treated in the same way.
(ⅲ) The case supp ˆnh={1,2},supp ˆns={3}. The pair (ˆnh,ˆns) have the form
ˆnh=ˆρh1δ{x=1}+ˆρh2δ{x=2},ˆns=ˆρsδ{y=3}. |
Thus we have
ˆρh=ˆρh1+ˆρh2,ˆΣh=ˆρh1+2ˆρh2,ˆΣs=3ˆρs. |
The conditions in the definition 1.2 are equivalent to
Gh(1)=Gh(2)=0,Gs(3)=0, |
that is
{−ˆρh1−ˆρh2−ˆρs+3α(1)ˆρs=0,rh(2)−ˆρh1−ˆρh2−ˆρs=0,rs(3)−ρh1−ρh2−ˆρs+β∗(ˆρh1+2ˆρh2)=0. |
Solving this system yields the expressions of ˆρh1,ˆρh2,ˆρs.
Let us consider two concrete examples below.
Example 1: Suppose that rh,α,rs,β are given by
rh(x)=(x−1)2,α(x)=2−x,rs(y)=12+14(3−y),β=0.5. |
According to Proposition 4.3, there are only two positive ESDs which are
● Monomorphic distribution:
ˆnh(x)=12δ{x=1},ˆns(y)=12δ{y=3}, |
● Dimorphic distribution:
ˆnh(x)=13δ{x=1}+13δ{x=2},ˆns(y)=13δ{y=3}. |
Example 2: Suppose that rh,α,rs,β are given by
rh(x)=0.75(x−1)2,α(x)=0.625(2−x),rs(y)=12+14(3−y),β=0.5. |
Then, there is only one positive ESD. This ESD is dimorphic and has the form
ˆnh(x)=13δ{x=1}+16δ{x=2},ˆns(y)=25δ{y=3}. |
We suppose that all stromal cells have some similar properties. More precisely, we consider the case where the contribution of each phenotype y in the message from stromal cells to HSCs and the sensitivity of stromal cells to the message from HSCs are the same. Mathematically, assume that the weight function ψs(y)=1, and that β>0 is constant. Suppose furthermore that α(x)=b−x,ψh(x)=x. Problem (1.1) becomes
{∂tnh(t,x)=[rh(x)−ρh(t)−ρs(t)+(b−x)∫ns(t,y)dy]nh,x∈(a,b),t>0,∂tns(t,y)=[rs(y)−ρh(t)−ρs(t)+β∫xnh(t,x)dx]nsy∈(c,d),t>0. | (4.2) |
Below we state a result about the partial uniqueness of ESDs.
Theorem 4.5 (Partial uniqueness results of ESDs). Assume that (ˆnh,ˆns) and (˜nh,˜ns) are two (non-negative) ESDs of the system (4.2). Assume further that β is a positive constant satisfying
(β(1−b)+1)2<4β. | (4.3) |
Then
ˆρh=˜ρh=:H,ˆρs=˜ρs=:S, | (4.4) |
∫rh(x)ˆnh−S∫xˆnh=∫rh(x)˜nh−S∫x˜nh, | (4.5) |
∫rs(y)ˆns−βS∫xˆnh=∫rs(y)˜ns−βS∫x˜nh. | (4.6) |
Moreover,
(ⅰ) If rs is strictly decreasing, then ˆns=˜ns either is monomorphic concentrated at y=c or vanishes. Also we have
∫rh(x)ˆnh=∫rh(x)˜nh. | (4.7) |
(ⅱ) In addition to (i), if ˆns does not vanish, then
∫xˆnh=∫x˜nh. | (4.8) |
Proof. The definition of ESD and the non-negativity of ˆnh yield that
0≥∫[rh(x)−˜ρh−˜ρs+(b−x)∫˜ns]ˆnh=∫{[rh(x)−˜ρh−˜ρs+(b−x)∫˜ns]−[rh(x)−ˆρh−ˆρs+(b−x)∫ˆns]}ˆnh=[(ˆρh−˜ρh)+(ˆρs−˜ρs)]ˆρh+(˜ρs−ˆρs)∫(b−x)ˆnh. | (4.9) |
Similarly, it follows from the inequality
∫[rh(x)−ˆρh−ˆρs+(b−x)∫ˆns]˜nh≤0, |
that
[(˜ρh−ˆρh)+(˜ρs−ˆρs)]˜ρh+(ˆρs−˜ρs)∫(b−x)˜nh≤0. | (4.10) |
Summing up (4.9) and (4.10), we obtain
(ˆρh−˜ρh)2+(ˆρs−˜ρs)(ˆρh−˜ρh)+(˜ρs−ˆρs)∫(b−x)(ˆnh−˜nh)≤0. | (4.11) |
Similarly, we deduce from the inequality
∫[rs(y)−˜ρh−˜ρs+β∫x˜nh]ˆns+∫[rs(y)−ˆρh−ˆρs+β∫xˆnh]˜ns≤0, | (4.12) |
that
(ˆρs−˜ρs)2+(ˆρs−˜ρs)(ˆρh−˜ρh)+β∫x(˜nh−ˆnh)(ˆρs−˜ρs)≤0. |
This together with (4.11) yields
β(ˆρh−˜ρh)2+[β(1−b)+1](ˆρh−˜ρh)(ˆρs−˜ρs)+(ˆρs−˜ρs)2≤0. |
Equivalently,
(√β(ˆρh−˜ρh)+β(1−b)+12√β(ˆρs−˜ρs))2+(1−(β(1−b)+1)24β)(ˆρs−˜ρs)2≤0. | (4.13) |
Therefore, the hypothesis (4.3) yields that the equality in (4.13) (also in all above inequalities) holds. Thus each term in (4.13) vanishes so that (4.4) follows. The identities (4.5), (4.6) follow from the fact that
∫[rh(x)−˜ρh−˜ρs+(b−x)∫˜ns]ˆnh=∫[rh(x)−ˆρh−ˆρs+(b−x)∫ˆns]˜nh, |
∫[rs(y)−˜ρh−˜ρs+β∫x˜nh]ˆns=∫[rs(y)−ˆρh−ˆρs+β∫xˆnh]˜ns, | (4.14) |
respectively.
(ⅰ) If rs is strictly decreasing on [c,d], the fitness function Gs attains its global maximum at y=c. Thus ˆns and ˆns either vanish or are the Dirac mass concentrated at y=c. As a consequence we have ˆns=˜ns=Sδ{y=c} with S≥0. Using the formula for ˆns, (4.5) and (4.6), we obtain (4.7)
(ⅱ) The case ˆns=˜ns=Sδ{y=c} with S>0. Identity (4.8) follows from (4.6).
In the case αΣh=βΣs=0, an entropy functional has been found by Jabin and Raoul [16] and used to prove the convergence of the solution to the unique ESD. In the general form of the system (1.1), we do not expect to find an entropy functional due to the complexity of the terms αΣh,βΣs. However, we obtain an entropy functional similar to that of Jabin and Raoul for the system (4.2) corresponding to particular choices of αΣh and βΣs. We obtain below a partial information about the dynamics of the solution of (4.2) as t→∞: the entropy functional decreases monotonically on orbits. The question of the convergence of the solution to the unique ESD remains open but this functional could be an essential ingredient to solve this issue.
Proposition 4.6 (Entropy functional). Let (ˆnh,ˆns) be an (non-negative) ESD of problem (4.2). Set
E(t):=β∫nh−β∫ˆnhln(nh)+∫ns−∫ˆnsln(ns). |
Then E is an entropy functional for (4.2), i.e., ddtE(t)≤0 provide that β is a positive constant satisfying
(β(1−b)+1)2≤4β. |
Proof. Set
E1:=∫nh−∫ˆnhln(nh). |
We have
ddtE1(t)=∫(nh)t(nh−ˆnh)nh=∫[rh(x)−ρh−ρs+(b−x)∫ns](nh−ˆnh)=∫[(rh(x)−ρh−ρs+(b−x)∫ns)−(rh(x)−ˆρh−ˆρs+(b−x)∫ˆns)](nh−ˆnh)+∫[rh(x)−ˆρh−ˆρs+(b−x)∫ˆns)](nh−ˆnh)=−(ρh−ˆρh)2−(ρh−ˆρh)(ρs−ˆρs)+∫(b−x)(nh−ˆnh)∫(ns−ˆns)+∫[rh(x)−ˆρh−ˆρs+(b−x)∫ˆns)]nh≤−(ρh−ˆρh)2−(ρh−ˆρh)(ρs−ˆρs)+∫(b−x)(nh−ˆnh)∫(ns−ˆns). |
In the above inequality, we have used the definition of ESDs and the non-negativity of nh (cf. Proposition 2.2). Similarly, for E2:=∫ns−∫ˆnsln(ns), we have
ddtE2(t)≤−(ρs−ˆρs)2−(ρh−ˆρh)(ρs−ˆρs)+β∫x(nh−ˆnh)∫(ns−ˆns). |
Therefore, as E=βE1+E2, we have
dEdt≤−β(ρh−ˆρh)2−[β(1−b)+1](ρh−ˆρh)(ρs−ˆρs)−(ρs−ˆρs)2≤0, |
where the last inequality follows from the negativity of the discriminant of this polynomial:
(β(1−b)+1)2−4β≤0. |
We are interested in the dynamics of HSCs and stromal cells with initial data close to a monomorphic state and, in particular, in tracking the movements of concentration point towards an ESD. We follows the analysis in [23] and perform the time change variable τ=tε to accelerate time and observe the dynamics. The parameter ε is also used to measure how close is the distribution from the Dirac distribution.
The change of variable t↦τ converts the system (3.1) to
{∂τnεh(τ,x)=1ε[rh(x)−ρεh(τ)−ρεs(τ)+α(x)Σs(τ)]nεh,x∈(a,b),τ>0,∂τnεs(τ,y)=1ε[rs(y)−ρεh(τ)−ρεs(τ)+β(y)Σh(τ)]nεs,y∈(c,d),τ>0. |
This system is completed with the initial data
nεh(0,x)=nεh0(x)>0,nεs(0,y)=nεs0(y)>0. |
Rather than working on nεh,nεs directly, we define as usual [19,21,23] the functions uε,vε given by
uε(τ,x)=εln(nεh(τ,x)),uε0(x)=εln(nεh0(x)),vε(τ,y)=εln(nεs(τ,y)),vε0(y)=εln(nεs0(y)). |
The functions uε,vε satisfy
{∂τuε(τ,x)=rh(x)−ρεh(τ)−ρεs(τ)+α(x)∫ynεs(τ,y),x∈(a,b),τ>0,∂τvε(τ,y)=rs(y)−ρεh(τ)−ρεs(τ)+β(y)∫xnεh(τ,x),y∈(c,d),τ>0. | (5.1) |
Our purpose is to study the behaviour of uε,vε as ε→0 (at least with subsequences). In order to guarantee the existence of a global solution, suppose that (2.3) is fulfilled. Thus, under the assumption that ρεh0+ρεs0 is uniformly bounded, Proposition 2.2 yields that there exists (ˆnh,ˆns) such that as ε→0 (after extracting subsequences),
nεh∗⇀ˆnh in L∞(0,∞;M([a,b])), | (5.2) |
nεs∗⇀ˆns in L∞(0,∞;M([c,d])). | (5.3) |
Theorem 5.1. Assume that rh,α∈C1([a,b]),rs,β∈C1([c,d]) and that
ρεh0+ρεs0+‖uε0‖C1([a,b])+‖vε0‖C1([c,d])≤K0. | (5.4) |
Then
(ⅰ) The function uε (resp. vε) is uniformly Lipschitz continuous on [0,T]×[a,b] (resp. [0,T]×[c,d]) for all T>0.
(ⅱ) As ε→0 (after extractions of subsequences), the functions uε and vε converge locally uniformly to Lipschitz continuous functions u and v. Moreover, u,v satisfy
{u(τ,x)=u0(x)+rh(x)τ−∫τ0∫ˆnh−∫τ0∫ˆns+α(x)∫τ0∫yˆns,v(τ,y)=v0(y)+rs(y)τ−∫τ0∫ˆnh−∫τ0∫ˆns+β(y)∫τ0∫xˆnh,maxτ,xu(τ,x)≤0,maxτ,yv(τ,y)≤0 for all τ≥0. | (5.5) |
Furthermore we have for a.e. τ,
supp ˆnh(τ,⋅)⊂{u(τ,⋅)=0},supp ˆns(τ,⋅)⊂{v(τ,⋅)=0}. |
Proof. (ⅰ) First note that by Proposition 2.2, there is a constant K1>0 such that
‖nεh‖L∞(0,∞;L1(a,b))+‖nεs‖L∞(0,∞;L1(c,d))≤K1. | (5.6) |
Differentiating the equation for uε with respect to x yields
∂τuεx(τ,x)=r′h(x)+α′(x)∫ynεs(τ,y)dy. | (5.7) |
Thus, using (5.6), we obtain |∂τuεx(τ,x)|≤|¯r′h|+¯|α′|dK1 so that
|uεx(τ,x)|≤K0+(|¯r′h|+¯|α′|dK1)τ. |
On the other hand, in view of (5.1), we have
|∂τuε(τ,x)|≤ˉrh+2K1+αK1d. |
Hence uε is uniformly Lipschitz continuous on [0,T]×[a,b]. Similarly, the same property holds for vε.
(ⅱ) Using the point (ⅰ) and the Arzelà–Ascoli Theorem, we may extract subsequences (uε,vε) which converge as indicated in the statement. The equations for u and v are obtained by passing to the limit in (5.1). Moreover, u,v cannot take positive values. Otherwise (ρεh,ρεs) blows up in the limit as ε vanishes and this is in contradiction with (5.6).
We provide sufficient conditions so that (ˆnh,ˆns) defined in (5.2), (5.3) is a monomorphic state.
Theorem 5.2. Let all hypotheses as in Theorem 5.1 hold. Suppose furthermore that
{uε0xx≤−K∗,vε0yy≤−K∗,r″h(x)≤0,α″(x)≤0,r″s(y)≤0,β″(y)≤0. | (5.8) |
Then, in the distributional sense
uxx≤−K∗,vyy≤−K∗. |
Thus for all τ, the functions u(τ,⋅),v(τ,⋅) are concave so that they have a unique maximum point. As a consequence, ˆnh,ˆns have the form
ˆnh(τ,x)=ˆρh(τ)δ{x=ˆx(τ)},ˆns(τ,y)=ˆρs(τ)δ{y=ˆy(τ)}. |
Moreover,
If ˆρh(τ)>0, then maxxu(τ,x)=u(τ,ˆx(τ))=0,If ˆρs(τ)>0, then maxyv(τ,y)=v(τ,ˆy(τ))=0. |
Proof. Differentiating twice the equation of uε, we obtain
∂τuεxx(τ,x)=r″h(x)+α″(x)∫ynεs(τ,y)≤0. |
Thus uεxx(τ,x)≤uεxx(τ=0,x)≤−K∗. Therefore uxx≤−K∗. Similarly, vyy≤−K∗. Hence the theorem follows.
In this section we derive the equations for the concentration point ˆx(τ),ˆy(τ). Our equations are valid until the time T∗ where ˆρh(τ)>0,ˆρs(τ)>0 and that ˆx(τ),ˆy(τ) do not touch the boundary and that ˙ˆx,˙ˆy are smooth enough (see Remark 5.3 below for the regularity of ˆx,ˆy). For all τ∈(0,T∗) we have ˆx(τ)∈(a,b) is the maximum point of u(τ,⋅) on [a,b]. It follows that ux(τ,ˆx(τ))=0 so that
uxτ(τ,ˆx(τ))+uxx(τ,ˆx(τ))˙ˆx=0 with ˙ˆx:=dˆx/dτ. |
This implies
˙ˆx(τ)=−1uxx(τ,ˆx(τ))[r′h(ˆx(τ))+α′(ˆx(τ))∫yˆns]=−1uxx(τ,ˆx(τ))[r′h(ˆx(τ))+α′(ˆx(τ))ˆy(τ)ˆρs(τ)]. | (5.9) |
Similarly, we have
˙ˆy(τ)=−1vyy(τ,ˆy(τ))[r′s(ˆy(τ))+β′(ˆy(τ))ˆx(τ)ˆρh(τ)]. | (5.10) |
The equations (5.9) and (5.10) describe the dynamics of ˆx(τ),ˆy(τ). We also can obtain a more explicit form of (5.9) and (5.10) by representing ˆρh,ˆρs in terms of ˆx,ˆy. To that purpose, we first notice that u(τ,ˆx(τ))=0 for τ∈[0,T∗) so that
0=du(τ,ˆx(τ))dτ=uτ(τ,ˆx(τ))=rh(ˆx(τ))−ˆρh(τ)−ˆρs(τ)+α(ˆx(τ))ˆy(τ)ˆρs(τ). |
Similarly,
rs(ˆy(τ))−ˆρh(τ)−ˆρs(τ)+β(ˆy(τ)ˆx(τ)ˆρh(τ)=0. |
Therefore, under the assumption that 1−(1−α(ˆx(τ))ˆy(τ))(1−β(ˆy(τ))ˆx(τ))≠0, we have
ˆρh(τ)=rh(ˆx(τ))−rs(ˆy(τ))(1−α(ˆx(τ))ˆy(τ))1−(1−α(ˆx(τ))ˆy(τ))(1−β(ˆy(τ))ˆx(τ)), |
ˆρs(τ)=rs(ˆy(τ))−rh(ˆx(τ))(1−β(ˆy(τ))ˆx(τ))1−(1−α(ˆx(τ))ˆy(τ))(1−β(ˆy(τ))ˆx(τ)). |
Substituting these expressions of ˆρh(τ),ˆρs(τ) in (5.9) and (5.10) we obtain
Canonical equations
{˙ˆx=−1uxx(τ,ˆx)[r′h(ˆx)+α′(ˆx)ˆyrs(ˆy)−rh(ˆx)(1−β(ˆy)ˆx)1−(1−α(ˆx)ˆy)(1−β(ˆy)ˆx)],˙ˆy=−1vyy(τ,ˆy)[r′s(ˆy)+β′(ˆy)ˆxrh(ˆx)−rs(ˆy)(1−α(ˆx)ˆy)1−(1−α(ˆx)ˆy)(1−β(ˆy)ˆx)]. | (5.11) |
Remark 5.3. In view of the two first equations in (5.5), if u0,v0,rh,α,rs,β are smooth enough (e.g., C2 functions), then uxx is continuous. Thus the right-hand-sides of (5.11) are continuous as functions of ˆx,ˆy,τ. Therefore, the standard ordinary differential equation theory implies that the solution of (5.11) (ˆx,ˆy) is a C1 function of time. We refer to [26], for a results about the regularity of u,v in the case of Hamilton–Jacobi equations.
Let us illustrate numerically the convergence of the solution of (3.1) towards an ESD as well as the movement of concentration points. We employ the two distinct sets (a,b):=(1,2) and (c,d):=(3,4) to insist on that fact that the two phenotypes of HSCs and of stromal cells are not the same. The space and time steps are given by
δx=δy=0.005,δt=0.01. |
Define for 0≤k≤200 and p=0,1,2,...,
xk:=1+kδx,yk:=3+kδy,tp:=pδt,(nh)pk:=nh(tp,xk),(ns)pk:=nh(tp,yk). |
Our numerical simulations are performed in MATLAB and based on the implicit-explicit scheme below:
{(nh)p+1k−(nh)pkδt=max(0,Rh(xk,(ρh)p,(ρs)p,(Σs)p)(nh)pk−max(0,−Rh(xk,(ρh)p,(ρs)p,(Σs)p)(nh)p+1k,(ns)p+1k−(ns)pkδt=max(0,Rs(xk,(ρh)p,(ρs)p,(Σs)p)(ns)pk−max(0,−Rs(yk,(ρh)p,(ρs)p,(Σh)p)(ns)p+1k,Rh(xk,(ρh)p,(ρs)p,(Σs)p):=rh(xk)−(ρh)p−(ρs)p+α(xk)(Σs)p,Rs(yk,(ρh)p,(ρs)p,(Σs)p):=rs(yk)−(ρh)p−(ρs)p+β(yk)(Σh)p. | (6.1) |
Here (ρh)p,(ρs)p,(Σh)p,(Σs)p are the approximation of ρh,ρs,Σh,Σs at the time p. We use the initial conditions
nh0=exp(−(x−1.5)2/0.01),ns0=exp(−(y−3.4).2/0.01) |
for Figures 3, 4, 5 and 6. For the last figures, we choose
nh0=exp(−(x−1.45)2/0.002),ns0=exp(−(y−3.58).2/0.002). |
The parameters are chosen as in the table below.
Parameters | Figures 3 and 4 | Figures 5 and 6 | Figures 7 and 8 |
(monomorphic situation) | (monomorphic situation) | (dimorphic situation) | |
healthy case | leukemic case | co-existence case | |
Remark 6.1. The parameters in the three cases satisfy the assumptions of Theorem 4.2(ⅲ), (ⅱ) and Proposition 4.3(ⅲ), respectively. Also they are chosen small enough such that the condition (2.3) for the global existence of the solution holds. In the first case (Figures 3 and 4), we use the homogeneous proliferation rate and the inhomogeneous sensitivity of stromal cells. Conversely, in the last two cases, the parameters correspond to the inhomogeous proliferation rate and the homogeneous sensitivity of stromal cells. Note also that in the monomorphic situatations, the corresponding fitness functions are monotone while in the dimorphic situation, the fitness function (for HSCs) is strictly convex.
Figures 3 and 4 display the behavior of nh and ns in the time scale t:=10−2t. In Figure 3, the population densities for HSCs and their support cells nh,ns are monomorphic and behave as Dirac masses. The concentration point of nh moves towards x=1 and the one of ns moves towards the point y=4. In this situation, the phenotype (x,y)=(1,4) is selected. This represents a good scenario: healthy HSCs and stromal cells with the best support capacity are selected. The evolution of the corresponding dominant phenotypes are given in Figure 4. Figures 5 and 6 below show another monomorphic situation where stromal cells with lowest support capacity are selected. Healthy HSCs cannot survive and cancer cells are selected.
Figures 7 and 8 represent the dimorphic situation for HSCs in the time scale t:=10−2t. This situation corresponds to Proposition 4.3(ⅲ). Starting from an initial distribution with one peak at x=1.45, a branching process appears. There are two dominant phenotypes of HSCs. The first one moves to the left (only healthy cells selected about the time until t=10) and the second one move towards x=2 (and selected in a little bit later). In other words, cancerous cells invade the population of HSCs, however without occupying it in totality: healthy HSCs and cancerous cells (LSCs) coexist.
In this paper, we have introduced a mathematical model for the interaction between hematopoietic stem cells and their support cells. Leukemic stem cells are also taken into account in the model and the phenotype x, characterising the population heterogeneity in a way relevant to the question at stake, represents for both the intrinsic proliferation rate of HSCs and the malignancy potential of cancer cells (i.e., as mentioned in the introduction, a proposed pathological combination of both plasticity and fecundity, likely related to how many mutations are involved in cancer cells). Note also that the monotonicity assumption on rh means that we assumed that the malignant cells proliferate more than healthy HSCs.
We performed a study concerning the adaptive dynamics of HSCs and support cells, in particular, investigating Dirac masses (or sums of Dirac masses) that arose in the solutions of particular cases of the system. Linear stability results for single Dirac mass steady states, suggesting that another phenotype will invade the stationary environment corresponding to the steady state if the corresponding fitness function computed at that phenotype is positive. We also provided sufficient conditions to ensure that ESDs are dimorphic or monomorphic. These conditions are related to the convexity, concavity, monotonicity assumptions of the function parameters. In many cases, we could show the existence and uniqueness of ESDs as well as compute explicitly all ESDs in the case of non-uniqueness.
Applying an asymptotic approach, we showed that without extinction, the population density of HSCs and of their support cells behave as Dirac masses:
nεh(τ,x)≈ˆρh(τ)δ{x=ˆx(τ)} withˆρh(τ)>0⇔maxxu(τ,x)=0=u(τ,ˆx(τ))nεs(τ,y)≈ˆρs(τ)δ{y=ˆy(τ)} withˆρs(τ)>0⇔maxyv(τ,x)=0=v(τ,ˆy(τ)). |
Here the points of concentration ˆx(τ),ˆy(τ) represent well adapted phenotypes at the time τ. Also these points are maximum points of the phase functions u(⋅,τ) and v(⋅,τ). The system (5.11) gives us the dynamics of ˆx(τ),ˆy(τ), in other words, the adaptive process for HSCs and its support cells during their evolution. Our numerical illustrations provide the case of the existence of HSCs, or LCSs (only). Also, we illustrate the case of invasion of LCSs as well as the coexistence of HSCs and LSCs. This latter situation does not seem to be usually seen in the clinic of acute leukemias, which may be due to the fact that in reality, the competition for space and nutrients turns to the advantage of leukemic cells (The biological fact is that stromal cells change to adapt to healthy cells or malignant cells. Thus LSCs and HSCs have different hematopoietic niches so that the competitive strength of HSCs and LSCs for space and nutrients will be different). In our model, the advantage of leukemic cells in competition could be represented by a diversified non local logistic term in the equation for HSCs such as −k1∫(b−x)nh(t,x)dx−k2∫(x−a)nh(t,x)dx, with k2>k1, instead of the neutral term −ρh, thus attributing more importance in the competition to cells close to the malignant phenotype x=b. Or else, could it be that actual biological coexistence between HSCs and LSCs could come from the fact that leukemic cells may have been reduced to a state of dormancy? Note that this perspective of dormancy has recently been investigated in a rather different modelling setting (no adaptive dynamics, no interaction with stromal cells) in [22].
Our analytic results, except in Section 4.2, hold for more general choices of the weight functions ψh,ψs. However, we present our results here mainly for the case ψh=x,ψs=y to clarify the ideas and avoid complex computations. The mathematical question related to the convergence of the solution of (1.1) to its limit (which is an ESD) remains open. The BV-method (see, for example, [24,28]) seems not amenable be applied due to the complexity of function parameters. However, we could find an entropy functional for a simplified system (4.2). This functional decreases to −∞, however it could be an essential ingredient to solve this issue.
The present model and its mathematical analysis represent to the best of our knowledge a first attempt to study the interactions between HSCs and their supporting stromal cells in the framework of adaptive dynamics. One could certainly complicate it to introduce multidimensional phenotypes related to refined cell functionalities such as fecundity, viability, plasticity, in the two cell populations, but even simple as it is, it relies on many unknown functions that should first be experimentally evaluated to go further in this modelling work, which we actually plan to do in the future.
B.P. has received funding from the European Research Council (ERC) under the European Union's Horizon 2020 research and innovation programme (grant agreement No 740623). The authors acknowledge funding by Plan Cancer HTE programm EcoAML.
The authors declare there is no conflict of interest.
[1] | M. C. Mackey, Unified hypothesis for the origin of aplastic anemia and periodic hematopoiesis, Blood, 51 (1978), 941–956. |
[2] | F. Burns and I. Tannock, On the existence of a G 0 -phase in the cell cycle, Cell Prolif., 3 (1970), 321–334. |
[3] | L. Lajtha, On DNA labeling in the study of the dynamics of bone marrow cell populations, F. StohlmanJr.(Ed.), The KineticsofCellularProliferation, Gruneand Stratton, NewYork, 173–182. |
[4] | M. Adimy, S. Bernard, J. Clairambault, et al., Modélisation de la dynamique de l'hématopoïèse normale et pathologique, Hématologie, 14 (2008), 339–350. |
[5] | M. C. Mackey, C. Ou, L. Pujo-Menjouet, et al., Periodic oscillations of blood cell populations in chronic myelogenous leukemia, SIAM J. Math. Anal., 38 (2006), 166–187. |
[6] | M. Adimy, F. Crauste and S. Ruan, A mathematical study of the hematopoiesis process with applications to chronic myelogenous leukemia, SIAM J. Appl. Math., 65 (2005), 1328–1352. |
[7] | L. Pujo-Menjouet, S. Bernard and M. C. Mackey, Long period oscillations in a G 0 model of hematopoietic stem cells, SIAM J. Appl. Dyn. Syst., 4 (2005), 312–332. |
[8] | M. Adimy, F. Crauste and S. Ruan, Periodic oscillations in leukopoiesis models with two delays, J. Theor. Biol., 242 (2006), 288–299. |
[9] | A. Ducrot and V. Volpert, On a model of Leukemia development with a spatial cell distribution, Math. Model. Nat. Phenom., 2 (2007), 101–120. |
[10] | G. Clapp and D. Levy, A review of mathematical models for leukemia and lymphoma, Drug Discov. Today Dis. Models, 16 (2015), 1–6. |
[11] | L. Pujo-Menjouet, Blood cell dynamics: half of a century of modelling, Math. Model. Nat. Phenom., 11 (2016), 92–115. |
[12] | B. A. Anthony and D. C. Link, Regulation of hematopoietic stem cells by bone marrow stromal cells, Trends Immunol., 35 (2014), 32–37. |
[13] | G. Stik, L. Petit, P. Charbord, et al., Vésicules extracellulaires stromales et régulation des cellules souches et progéniteurs hématopoï étiques, médecine/sciences, 34 (2018), 114–116. |
[14] | P. Charbord, T. Jaffredo and C. Durand, Le cœur moléculaire de la fonction de la niche des cellules souches hématopoï étiques, médecine/sciences, 31 (2015), 12–14. |
[15] | P. Hirsch, Y. Zhang, R. Tang, et al., Genetic hierarchy and temporal variegation in the clonal history of acute myeloid leukaemia, Nat. commun., 7 (2016), 12475. |
[16] | P.-E. Jabin and G. Raoul, On selection dynamics for competitive interactions, J. Math. Biol., 63 (2011), 493–517. |
[17] | C. Pouchol, J. Clairambault, A. Lorz, et al., Asymptotic analysis and optimal control of an integro- differential system modelling healthy and cancer cells exposed to chemotherapy, J. Math. Pures Appl., 116 (2018), 268–308. |
[18] | C. Pouchol and E. Trélat, Global stability with selection in integro-differential Lotka-Volterra systems modelling trait-structured populations, J. Biol.l Dyn., 12 (2018), 872–893. |
[19] | G. Barles and B. Perthame, Dirac concentrations in Lotka-Volterra parabolic PDEs, Indiana Univ. Math. J., 57 (2008), 3275–3301. |
[20] | O. Diekmann, A beginner's guide to adaptive dynamics, Banach Center Publications, 63 (2003), 47–86. |
[21] | O. Diekmann, P.-E. Jabin, S. Mischler, et al., The dynamics of adaptation: An illuminating example and a hamilton–jacobi approach, Theor. Popul. Biol., 67 (2005), 257–271. |
[22] | W. Djema, C. Bonnet, F. Mazenc, et al., Control in dormancy or eradication of cancer stem cells: Mathematical modeling and stability issues, J. Theor. Biol., 449 (2018), 103–123. |
[23] | A. Lorz, S. Mirrahimi and B. Perthame, Dirac mass dynamics in multidimensional nonlocal parabolic equations, Commun Part. Diff. Eq., 36 (2011), 1071–1098. |
[24] | A. Lorz and B. Perthame, Long-term behaviour of phenotypically structured models, Proc. R. Soc. Lond. Ser. A Math. Phys. Eng. Sci., 470 (2014), 20140089, 10. |
[25] | G. Meszéna, I. Czibula and S. Geritz, Adaptive dynamics in a 2-patch environment: A toy model for allopatric and parapatric speciation, J. Biol. Syst., 5 (1997), 265–284. |
[26] | S. Mirrahimi and J.-M. Roquejoffre, A class of Hamilton-Jacobi equations with constraint: uniqueness and constructive approach, J. Differ. Equations, 260 (2016), 4717–4738. |
[27] | J. D. Murray, Mathematical biology. I, vol. 17 of Interdisciplinary Applied Mathematics, 3rd edition, Springer-Verlag, New York, 2002, An introduction. |
[28] | B. Perthame, Transport equations in biology, Frontiers in Mathematics, Birkhäuser Verlag, Basel, 2007. |
1. | Shensi Shen, Jean Clairambault, Cell plasticity in cancer cell populations, 2020, 9, 2046-1402, 635, 10.12688/f1000research.24803.1 | |
2. | Jean Clairambault, Stepping From Modeling Cancer Plasticity to the Philosophy of Cancer, 2020, 11, 1664-8021, 10.3389/fgene.2020.579738 | |
3. | Giada Fiandaca, Marcello Delitala, Tommaso Lorenzi, A Mathematical Study of the Influence of Hypoxia and Acidity on the Evolutionary Dynamics of Cancer, 2021, 83, 0092-8240, 10.1007/s11538-021-00914-3 | |
4. | Jean Clairambault, 2023, Mathematical Modelling of Cancer Growth and Drug Treatments: Taking Into Account Cell Population Heterogeneity and Plasticity, 978-3-907144-08-4, 1, 10.23919/ECC57647.2023.10178266 | |
5. | Thomas Stiehl, 2023, Chapter 73, 978-3-031-28048-1, 327, 10.1007/16618_2023_73 | |
6. | Frederick R. Adler, A modelling framework for cancer ecology and evolution, 2024, 21, 1742-5662, 10.1098/rsif.2024.0099 | |
7. | Xiyin Liang, Jinzhi Lei, Oscillatory Dynamics of Heterogeneous Stem Cell Regeneration, 2024, 6, 2096-6385, 431, 10.1007/s42967-023-00263-z |
Parameters | Figures 3 and 4 | Figures 5 and 6 | Figures 7 and 8 |
(monomorphic situation) | (monomorphic situation) | (dimorphic situation) | |
healthy case | leukemic case | co-existence case | |