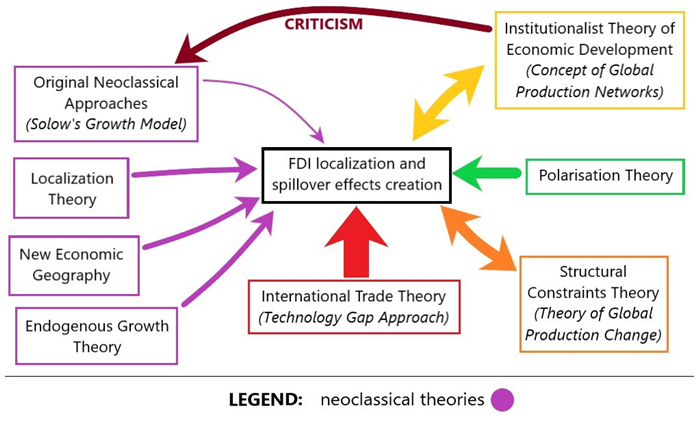
Citation: Zhiwei Huang, Gang Huang. Mathematical analysis on deterministic and stochastic lake ecosystem models[J]. Mathematical Biosciences and Engineering, 2019, 16(5): 4723-4740. doi: 10.3934/mbe.2019237
[1] | Dina Krasnoselskaya, Venera Timiryanova . Exploring the impact of ecological dimension on municipal investment: empirical evidence from Russia. National Accounting Review, 2023, 5(3): 227-244. doi: 10.3934/NAR.2023014 |
[2] | Sylvie Kotíková, Roman Vavrek . Development of foreign direct investment in the context of government aid: A case study of the Czech Republic since 1998. National Accounting Review, 2025, 7(2): 196-220. doi: 10.3934/NAR.2025009 |
[3] | Guido Ferrari, Yanyun Zhao, José Mondéjar Jiménez, Luca Secondi . The economy of Tuscany in the post Covid-19 era: struggling with energy crisis and inflation. What to do to resume the journey?1. National Accounting Review, 2024, 6(1): 1-26. doi: 10.3934/NAR.2024001 |
[4] | Rui Xie, Yuanyuan Zhao, Liming Chen . Structural path analysis and its applications: literature review. National Accounting Review, 2020, 2(1): 83-94. doi: 10.3934/NAR.2020005 |
[5] | Pavel Ivanov, Tatyana Altufeva . Stimulating the processes of attracting investments in industry of the constituent entities of the Russian Federation. National Accounting Review, 2024, 6(3): 352-366. doi: 10.3934/NAR.2024016 |
[6] | Ming He, Barnabé Walheer . Technology intensity and ownership in the Chinese manufacturing industry: A labor productivity decomposition approach. National Accounting Review, 2020, 2(2): 110-137. doi: 10.3934/NAR.2020007 |
[7] | Duncan O. Hongo, Fanglin Li, Max William Ssali, Maurice Simiyu Nyaranga, Zephaniah Moriaso Musamba, Brenda Nelima Lusaka . Inflation, unemployment and subjective wellbeing: nonlinear and asymmetric influences of economic growth. National Accounting Review, 2020, 2(1): 1-25. doi: 10.3934/NAR.2020001 |
[8] | Lorna Katusiime . International monetary spillovers and macroeconomic stability in developing countries. National Accounting Review, 2021, 3(3): 310-329. doi: 10.3934/NAR.2021016 |
[9] | Jinhong Wang, Yanting Xu . Factors influencing the transition of China's economic growth momentum. National Accounting Review, 2024, 6(2): 220-244. doi: 10.3934/NAR.2024010 |
[10] | Tsolmon Baatarzorig, Nyambaatar Batbayar, Ragchaasuren Galindev, Oyunzul Tserendorj . The 2014 Mongolian Social Accounting Matrix. National Accounting Review, 2020, 2(2): 138-154. doi: 10.3934/NAR.2020008 |
The spillover effect is referred to in literature as a situation where the domestic business environment benefits from the localization of foreign direct investment (FDI), and the presence of multinational companies (MNCs) and their FDI helps domestic firms to increase their productivity (Havránek and Iršová, 2013).
The pioneering study of this economic phenomenon is presented by Caves (1974). Blomström and Kokko (1997) laid down the basic methodology for analyzing indirect effects. When measuring indirect spillover effects, the impact of FDI on changes in labor productivity or on the aggregate productivity of production factors is monitored. There is a comparison over time between foreign and domestic business entities. This issue is derived from the production function.
In comparison — foreign productivity vs. domestic productivity is monitored to see if, or with a delay, "it spills over" towards the segment with lower labor productivity or production factors in general (Benáček et al., 2014). The importance of spillover effects on economic growth becomes more vital in the case of transforming economies, which, according to Benáček and Víšek (2000), are much better equipped with capital, both human and physical, compared to developing countries. Due to the capital equipment, the ability of transitive economies to absorb know-how and achieve the so-called imitation effect of FDI (one of the main channels of spillovers) should be much higher than it would be in the case of developing economies.
Other research related to the identification of effects on economic development was also reported by, for example, Konnings (2001), Mišun and Tomšík (2002), Bywalec (2019), Wehncke et al. (2023) and Popadynets et al. (2023).
Konings (2001) also researched the effects of FDI in Central and Eastern Europe using company data. Through a regression model of panel data, the crowding-out effect was identified in Bulgaria and Romania. The crowding-out effect is a situation where foreign investments crowd out domestic ones. Conversely, a positive spillover effect on increasing labor productivity was demonstrated in Poland.
Mišun and Tomšík (2002) modified the model of Agosin and Mayer (2000) to detect effects in the Czech Republic, Poland and Hungary. They identified a crowding-out effect in Poland in 1990–2000, crowding-in in Hungary in the same time series, and crowding-in in the Czech Republic in 1993–2000. By the term crowding-in effect, they understand the situation in the economy when FDI increases overall investment in the economy, e.g., by establishing supplier-customer relationships (feedback and direct links) or through investments in infrastructure, etc. According to the authors, even a negative crowding-out effect positively affects the economy, in which it manifested itself. Businesses that "survive" this selection logically become more competitive. In this direction, FDI can create pressure on innovative activity and increase other companies' production efficiency.
Popadynets et al. (2023) assessed the impact of FDI on the economic growth of Poland and Ukraine. The results showed that FDI positively affects the economic growth of both states. However, due to the security of investments in war and post-war Ukraine, investors are now distancing themselves from this country, which puts Ukraine at a disadvantage compared to Poland in terms of economic freedom, perception of corruption and ease of doing business.
Driffield and Hughes (2003) devoted themselves to the identification of the indirect effects of FDI both at the sectoral and regional level in the UK (Scotland, Wales and Northern Ireland) in the period 1984–1997. They were the first to highlight that differences within states can be more significant than between states. Therefore, it is necessary to focus on identifying local effects at lower territorial units.
The creation of FDI links with domestic enterprises is considered a key mechanism through which indirect effects are created — growth in employment, labor productivity and the creation of new domestic enterprises. The degree of integration of FDI into the host economy can vary significantly. A high degree of FDI penetration can represent a crucial element in the transformation of the local business environment. For example, a restructuring of the supply network can occur. On the other hand, FDI may not develop any or only negligible ties towards local businesses. The degree of FDI penetration into the host region is considered an indicator of investment stability (Pavlínek and Žížalová, 2016).
From the above, it can be concluded that the final impact of FDI on the host region and its business environment is ambiguous. The impact of FDI and interaction with other businesses in the region can take different forms, and the impact on the host business environment and regional competitiveness can occur in a positive as well as a negative way. This article seeks to identify the spillover effects of FDI on the business environment, directly affecting the localization of FDI. Although this is a significant way in which foreign investors affect the host region and its business environment, and the FDI represents a dynamic system of influencing the overall economic environment, the professional literature does not provide a uniform procedure or established methodology for its measurement. One of the reasons for this absence of professional studies evaluating the effects of FDI at the lower regional level is the complicated data collection. In order to identify the effects from the point of view of individual regions, it is necessary to obtain data from individual financial statements and annual reports of specific enterprises regarding, for example, the number of employees for the monitored period, economic results or gross value added (GVA). Subsequently, it is only possible to perform a more precise quantification of the effects on the local level.
This article is based on the work of Crespo and Fontoura (2007), who reviewed the literature on the determinants of FDI spillovers and discussed the numerous factors that may cause the spillover effects to vary. Whereas the survey of Crespo and Fontoura (2007) is narrative, this research examines spillover determinants using a quantitative method: shift-share analysis.
The shift-share analysis is a frequently used method in professional literature, especially for determining the development of employment dynamics, labor productivity or added value (Zdeněk and Střeleček, 2012). Its use is mainly in forecasting, strategic planning and political evaluations of individual areas (Dobrzanski, 2019; Lv et al., 2021; Sheng et al., 2021). It is, therefore, a suitable supporting tool for evaluating the benefits of FDI inflows and the localization of foreign capital in host economies (Ruault and Schaeffer, 2020).
This paper aims to measure the spillover effects of FDI localization, with the possibility of identifying differences in their size and development on the level of regions within the host economy, using the case of the Czech Republic. The spillover effect was evaluated based on five criteria: GVA, technology gap, investment in R&D, share of people with secondary and higher education and inflow of FDI.
This study presents an innovative methodological approach with an appropriate sample and novel application of the benchmarking method based on the shift-share analysis. The proposed methodology has the potential to enhance the understanding of the impact of FDI on regional business environments for policymakers and investors, facilitating more informed decision-making. In terms of the scientific field, the main contribution of the presented approach is the proposed methodology of the spillover effects identification, the construction of indicators and the assessment of the monitored effect at the regional level (of NUTS 3). This paper contributes to the existing scholarship by more explicitly dealing with the complexity of the relationship between the FDI inflow and the ability of regional business environments to deal with such internationalization activities.
The paper is structured as follows: Section 2 presents the theoretical anchoring of spillover effects within various theoretical approaches and directions. This is followed by Section 3, where the externality of spillover effects is emphasized. It is the nature of externalities that strongly contributes to the difficult measurability of this economic phenomenon. Furthermore, the chapter provides a comprehensive overview of the channels through which spillover effects spread in the host economy, including links to key studies and research evaluating the impact of spillover effects on economic growth and development of the business environment of the host market. Section 4 describes in detail the methodology for identifying and measuring spillover effects within the host economy. This section describes in detail the main method of shift-share analysis and the constructed indicators of the regional technology gap and the Spillover index. Subject indicators and research methodology are described in sufficient detail, including an interpretation of the results, possible modifications and research limits. Section 5 uses this methodology on data from the Czech Republic. The research sample is 211 FDI (with granted state investment incentives). Calculations showed that the strength and magnitude of spillover effects fully reflect the weaknesses of peripheral regions. The final Section 6 discusses the findings and policy implications, and presents a summary.
The theoretical anchoring of spillover effects can be seen in those concepts that touch on FDI, which the effects create. FDI came to the center of professional discussions during the second half of the 20th century. Theories related to the issue itself can be considered relatively new, e.g., Vernon's theory of the production cycle (Vernon, 1966), Dunning's concept of OLI: ownership advantage, localization advantage and internationalization advantage (Dunning and Lundan, 2008a, 2008b).
The fundamental question is how a given economy or host business environment could profit as long as possible and as best as possible from the localization of a particular factor of production on its territory. Answers to this and related questions regarding the possible impacts of FDI on the business environment are provided by several theoretical approaches, including endogenous growth theory (Grossman and Helpman, 1994), localization theory, new economic geography (Krugman, 2011) and others (see Figure 1).
The original neoclassical models, e.g., Solow's growth model, consider foreign investments as only one source of capital equipment but do not pay more attention to their effects (Fagerberg et al., 2018).
In contrast to Solow's model, endogenous growth theories build into the production function the influence of external effects on knowledge and technological changes (Pan and Ngo, 2016). E.g., in Romer's (1986) learning by doing model, knowledge is a public good, where the author assumes that it cannot be kept secret. Knowledge creation in one company spills over into the entire economy (identification of the spillover effect).
According to Lucas (1988), the dynamics of economic development is linked to the stock of human capital. In this context, Lucas mentions the effect of the migration of skilled workers, as the availability of skilled workers is an important localization factor for firms producing goods with high added value. Workers are one of the channels for spreading spillover effects (Blomström and Kokko, 1998).
Fagerberg (1987) and Verspanger (1991) criticize theoretical approaches that are based on production functions and their modifications. They consider them too formalized. These authors interpret economic growth as a consequence of technological absorptive capacity and the size of the technological gap. They consider technological absorptive capacity to be a key factor in reducing the technological gap between the investor's country of origin — the FDI provider — and the FDI recipient. Because of a significant technological gap, FDI can function as so-called cathedrals in the desert. In such a case, they are large production plants that use relatively advanced technology (cathedral) with a low number of ties to the host region (desert). Prosperous are the regions (areas) without the dominance of these individual actors (cathedrals) or regions with a dense network of ties between subjects (Kotíková, 2019).
The second current economic approach, which, like the new economic geography, is based on the tradition of neoclassical economics, is the new growth theory. Unlike the new economic geography, this approach concentrates on the role of the importance of knowledge, information, innovation and their diffusion (Grossman and Helpman, 1994).
The essential identification feature of this contemporary theoretical approach is the crucial importance of human capital, innovation, technology, knowledge and the associated growing returns. Economic growth and competitiveness depend on these determinants.
A common feature with the new economic geography is the assumption of strong dependence on past development (path dependence). However, in contrast to traditional neoclassical economics, on which the new theory of growth is based, it emphasizes the role of institutional elements. These are understood broadly (e.g., education system, intellectual property protection). Institutions significantly influence the environment in which human capital, information, innovation and knowledge are formed and applied (Barasa et al., 2017; Cortright, 2001).
The new theory of growth is also referred to as endogenous growth theory in professional literature. Unlike neoclassical economics, it does not consider the factor of human capital and technology as exogenous factors but as endogenous. These factors do not appear randomly but can be accumulated through resources (Martin and Sunley, 1998). The endogenization of technological progress and human capital was primarily caused by the need to explain the so-called residual component of growth, i.e., the part of economic growth that the original neoclassical models did not explain. Modelling within theories of endogenous growth is problematic, as knowledge and innovation exhibit characteristics of public goods, e.g., the impossibility of benefit only for the creator due to the impossibility of achieving perfect protection of intellectual property. Several actors can use given knowledge or inventions at the same time without having to be created again. With repeated consumption, its size does not change (Blažek and Květoň, 2023).
According to the theory of endogenous growth, knowledge generated by FDI is a public good that can be well-disseminated under certain circumstances, but the basic condition is a sufficient supply of high-quality human capital, which can be the case in specific regions or economies that have an incompatible education structure with respect to labor market demand, a problem or an obstacle to economic growth. The Czech Republic and its structurally affected regions, such as the Karlovy Vary Region, can be cited as an example.
Neoclassical growth models prioritize supply-side factors, such as capital growth, technological changes or labor force growth. On the other hand, the influence of demand is, on the contrary, a characteristic feature of Keynesian theories of understanding the economy. Theories created after the Second World War can be classified into the so-called core-periphery group (Blažek and Uhlíř, 2011). The difference between polarization theories is the assumption that development is directed not towards a state of equilibrium but towards a deepening of imbalances. It can be argued that the polarization theory creates pressure or an appeal for economic policy to eliminate or erase regional disparities by appropriately setting institutional conditions (Kotíková, 2019).
Boudeville (1974) considers a growth center to be a set of intensively interconnected dynamic industries that are concentrated around a key industry. Regardless of distance, the spread of growth (and the source of spillover effects) occurs through customer-supplier linkages.
Due to rising personnel costs, energy prices and other inputs, regulatory measures in labor relations and environmental protection, more and more MNCs are locating part of their processes to less developed countries than the investor's country of origin (Bogataj et al., 2019). Often, only the management with key departments (e.g., research, development, design, marketing or financial management of the company) remains in the mother country. As a result of the transfer of part of the processes with lower added value to less developed countries, the parent (developed) economy experiences deindustrialization, the creation of GVA and employment growth in the tertiary sector.
The theory of changes in global production thus points to the growing dependence of less developed economies on foreign investors. On the other hand, the mentioned process can be considered as the first phase of the diffusion procedure, as it is possible to identify economies that have already passed this phase and managed to reach a higher development stage. An illustrative example is the so-called Asian tigers (Blažek and Uhlíř, 2011).
According to this theory and generally structuralist approaches, MNCs locate their FDI in regions with the lowest costs in terms of production fragmentation (Sako and Zylberberg, 2019). Given their capital strength, these investors logically have significant lobbying potential compared to small firms. They can obtain attractive investment benefits or incentives from states in the competition to attract FDI. Nevertheless, investment incentives should be appropriately set so that, at least to a certain extent, they prevent the deepening of regional disparities.
Institutionalists criticize the neoclassical for their ignorance of social, cultural, psychological, and political factors and the importance of the institutional framework in terms of the functioning and development of economies (Gambus and Almeida, 2018). At the same time, they criticize the neoclassical approach for the implicit assumption of the primacy of the market as the optimal means of efficient allocation of production factors (North and Weingast, 2000).
Due to the dynamically changing situation in the world, it can be assumed that MNCs will use the innovative capacity of the regions. For this reason, Amin and Thrift (1995) and Markowska et al. (2022) consider the adaptability of the region, the ability to incorporate new networks of contacts into the regional structure, to be a key capability. These approaches in terms of regional development are nothing new. It can be said that they seamlessly followed Alfred Marshall and his definition of agglomeration advantages, horizontal and vertical business ties (Šimanová and Trešl, 2011).
According to the theory of global production networks, the key actor for the emergence of spillover effects is the state, which defines and shapes the formal institutional environment in which other actors' activities occur. Due to the theory's global scale, international standards setting plays a significant role (Blažek and Květoň, 2023). For this reason, the theory deals with, e.g., the role of unions, environmental standards, the importance of international quality standards, such as the ISO 9000 certificate, etc. The theory of global networks also points to the growing importance of transnational standards defined by the corporate sphere itself. An illustrative example is the so-called Wintelism, i.e., the dominance of the Windows platform, with which software from other companies and Intel as the leading chip supplier must be compatible (Dicken et al., 2008).
A significant contribution of the theory of global networks is the emphasis on the role of the previous development. It works with the concept of dependence on development in the past (path dependence) or works with the idea that past development determines the current state and the situation in the future (Blažek and Květoň, 2023).
From the point of view of spillover effects, institutional directions represent an important theoretical basis, as a quality institutional environment is one of the basic conditions for the emergence of positive effects of FDI. At the same time, they create the potential for their persistence in the long term.
Theories of international trade deal with the effect of trade liberalization on the business environment. Posner (1961) defined the so-called technology gap theory, which he based on the importance of innovation. The company is developing a new product that will improve the market position of the given manufacturer, which will allow easier access to the foreign market. In the initial phase, profits and exports grow, logically motivating competitors to imitate. With the emergence of the so-called imitation effect (one of the channels of the spillover effect), the original producer loses his comparative advantage of the new production. Given the loss of competitive advantage, the producer will resort to innovation and regain the lost comparative advantage. The producer (investor, MNC) will have an absolute advantage only for a certain time or until other firms in other states or regions begin to produce more efficiently. As a result of innovation, there will be a time-limited technological gap between the original producer and all other firms.
The American professor Vernon (1966) followed Posner's technological gap theory with the production cycle theory. Vernon was convinced that the production cycle has four stages: innovation, growth, maturity and decline. Initially, the product is top-notch thanks to the technological maturity of the company; after some time, this advantage declines due to the so-called imitation effect, and the product becomes standard. A substandard product may be produced in technologically less advanced areas.
The evaluation of spillover effects and the impact of FDI on the business environment of the host economy and their influence on economic growth is based on various theoretical starting points The theories presented in this article, to a greater or lesser extent, define the conditions for the development of the potential for positive effects of FDI in the host economy (i.e., mainly the rooting or nesting of the investment and the development of other activities with local entities, the establishment of business or cooperative ties, the spillover effect, or the agglomeration effect or the effect of packing additional investments onto the original investment).
Research articles related to the economic growth of the host economy are concentrated in three supporting spheres, which are connected to the implementation of economic policy:
● Analysis of localization factors that determine the inflow of FDI into the host state or region (and verifying the significance of selected localization factors to FDI inflow; determining causality between selected localization factors and the level of FDI in the host economy or region; identifying regional disparities).
● Analysis of the influence of direct effects realized from the inflow of FDI in the host economy, e.g., direct impacts on the labor market, change in the composition of foreign trade, GDP growth, etc. (incl. determination of causality between labor market macroeconomic indicators and the development of FDI inflow; verification of the statistical significance of FDI on economic growth; testing of hypotheses of the impact of FDI on the living standard of host areas and regional development).
● Analysis of indirect effects on the host business environment (incl. testing the size of the level of foreign presence in selected (host) regions; if the size of the level of foreign presence in selected (host) regions represents the potential for (positive) spillover effects or not; verifying the hypothesis of the significance of the technological gap between FDI and domestic enterprises at the national or regional level).
Although the effects of FDI on economic growth are much discussed, quantifying the effects is very problematic. FDI is examined primarily in terms of its impact on GDP. A study evaluating the impact of FDI localization on the GDP of the host region was carried out by, for example, Naughton et al. (2016), Alfaro (2017), Govori and Fejzullahu (2020) and Tkalenko et al. (2022).
According to Leamer (1994), the last-mentioned sphere of analysis of indirect effects is of absolutely fundamental importance since indirect effects are considered in the international literature to be of dominant economic importance in terms of the economic growth and development of the host regions.
The economic effects of FDI can be divided into direct (primary) and indirect (secondary). Among other things, the inflow of long-term foreign capital can be characterized as direct: the creation of primary jobs in FDI, tax revenues to the state budget and others (Kotíková, 2018).
In professional literature, indirect positive effects are often referred to as spillovers (Benáček et al., 2014; Mišun and Tomšík, 2002). Indirect effects include so-called secondary effects — externalities that do not have a clearly specified link to FDI, but result from direct effects and the location of the FDI itself.
The indirect effects of FDI have the character of externalities, for the existence of which no one pays or gets paid. It is a similar principle that is considered in the theory of public goods (Benáček et al., 2014). Spillover effects are a type of "public interest". Their existence is supported by state institutions, which in many economies take the form of investment incentives (Pavlínek and Žížalová, 2016).
Benáček et al. (2014) point out that some fundamental benefits of FDI localization cannot be achieved through markets. Foreign investors are fully aware of this fact for two reasons. First, they deliver something positive to the host environment for free, for which they incurred significant costs in the past, in addition to the risk of a later imitation effect by domestic competitors. On the other hand, MNCs signal the possibility of "reselling" the externality to the host country if an institution in the given economy is willing to correct these market failures.
This feature of FDI is the reason for the commitment of the state or local authorities to the policy of the inflow of foreign capital in the sense of purchasing externalities in the form of investment incentives. Moreover, the spillover effects represent a positive economic externality or an external benefit flowing to the host economy that arises beyond the direct effects of FDI expressed in market transactions. It is assumed that the total benefit of FDI will be higher than the extent of institutional support granted. Foreign investors bring "something extra" to the host economy, which may turn against them in the future in the event of the a so-called FDI imitation effect. On the other hand, the government also carries the risk when creating suitable institutional conditions that FDI will bring not only positive, but also negative effects, which can cause the system of investment incentives to be counterproductive (Kotíková, 2018). Effects obtained through incentives can influence the business environment to a greater extent than effects associated with increasing the efficiency of specific companies forming the business environment (Benáček et al. 2014). For example, attracting investments with high added value, which will bind qualified workers, logically requires a certain level of education. In a broader context, it is pressure to build quality human capital.
Suppose a multinational firm implements FDI in the host economy. In that case, it transfers (according to Dunning's (1981) OLI concept) a competitive advantage in the form of ownership of specific company assets, thanks to which the FDI can better face competitive pressure from local firms that have the advantage of better knowledge of the given market, business practices, customer preferences, etc. There will, therefore, be a geographical dispersion of know-how and technologies, or technology transfer, but not automatically beyond the corporation's boundaries. Positive spillover effects can be identified if the presence of FDI increases the productivity of domestic enterprises (Havránek and Hampl, 2018; Lesher and Miroudot, 2008). The technology possessed by the FDI spills over into the host region's economy, is transferred to other enterprises and increases their productivity in the form of the so-called positive technological spillover effect (Hardy et al., 2011).
Positive spillover effects are considered the opposite of the dual economy, as they are identifiable through the increase in labor productivity of domestic companies in the host region. If FDI positively affects domestic enterprises within the same industry, so-called horizontal spillover effects are identified. Blomström and Kokko (1998) defined four ways in which local businesses can increase their productivity through the presence of FDI:
● imitation effect,
● human capital/labor mobility,
● competition,
● higher export performance.
Crespo et al. (2009) add, based on the approach of Markusen and Venables (1999), forward and backward linkages (see Figure 2).
The inflow of foreign investments unpleasantly impacts the investor itself — the so-called imitation effect. This effect is conditioned by the competition level and the host market's structure (Szent-Ivanyi and Vigvári, 2012). Competitors in the host country's businesses observe the new foreign company — its procedures, marketing, access to the public, and the way the company presents itself and behaves. By observing these methods, domestic businesses can learn and improve a lot, to be more competitive and productive (Crespo and Fontoura, 2007). The extent of this effect depends on the details and complexity of individual processes (Bitzer et al., 2008). Crespo and Fontoura (2007) consider the imitation effect the most visible spillover channel.
A technological transfer most often occurs through the acquisition of human capital. Domestic enterprises may hire workers who previously worked in FDI, or these people start their own businesses. Productivity improvements are the result of worker mobility. Even if the primary motive of the investor is the low cost of labor, MNCs spend funds on training programs and invest in human capital in various ways (Čuhlová and Potužáková, 2017). No company can 100% protect its investment in employees. Workers are carriers of intangible capital (Sinani and Meyer, 2004).
The transfer of know-how and overall knowledge and management procedures has an impact that is firmly reflected over the long term in the host country's economic growth. Employees who have worked in companies with foreign ownership and who have had to do with management transfer sophisticated management and a proven corporate culture to other companies, often becoming senior managers in domestic companies or capable entrepreneurs (Pavlínek, 2018).
Competition plays a key role in terms of spillover effects. The arrival of FDI in the host region will disrupt the market balance and cause local firms to fight for their existing market share. One of the ways to face new competition is to imitate FDI. If the imitation effect does not occur, domestic firms must use their existing technology more efficiently to maintain their position in the competitive struggle (Wang and Blomstörm, 1992). A more efficient use of technology leads to productivity growth, while competition can also change the speed of implementation of new production procedures (Havránek and Iršová, 2013; Kokko and Kravtsova, 2008).
According to Blomström and Kokko (1998), export represents spending on establishing a distribution network, obtaining information about consumers and foreign markets, or lobbying costs. The multinational company has already incurred these costs and has vital information upon entering the host country. This knowledge can be applied when exporting from the host economy, helping domestic companies in the field of exports (Greenaway et al., 2004; Crespo and Fontoura, 2007).
A foreign investor tries to prevent horizontal spillover effects upon entering a foreign market by internationalizing its activities or by setting up FDI instead of, for example, selling a license to a local business (Shaver and Flyer, 2000). On the other hand, MNCs can benefit from the existence of spillover effects. If they can use the quality production of domestic suppliers, they have no reason to prevent the spread of so-called vertical cross-industry spillovers. The basic difference with the horizontal spillover effect is that it does not originate from a competitive struggle but from cooperation (Lesher and Miroudot, 2008).
Vertical spillover effects occur through so-called backward linkages and forward linkages. Feedback occurs when FDI finds its suppliers among local businesses (Crespo and Fontoura, 2007). The emergence of feedback spillover effects depends on the willingness of MNCs to provide a technology transfer and the willingness of domestic firms to adapt to the MNCs' requirements (Lenaerts and Merlevede, 2012). In this case, productivity growth in home businesses and the spillover effects themselves can be manifested in several ways:
● knowledge transfer from a foreign investor to a local business partner;
● through higher quality requirements;
● strict compliance with business conditions (e.g., shortening delivery times);
● by creating access to the foreign market and the possibility of supplies to other FDI and headquarters (Watanabe, 1983; Hardy et al., 2011).
On the other hand, the localization of FDI in the host economy can result in negative effects. The entry of a foreign company could cause a decrease in sales of the production of domestic companies. Foreign investors can force domestic enterprises out of the domestic market; such a situation represents the emergence of a negative horizontal FDI effect, also referred to as the crowding-out effect (Choi 2018; Konstandina and Gachino, 2020; Liu et al., 2021). Negative vertical effects of FDI arise if foreign business partners replace domestic suppliers. On the other hand, this replacement leads to an inflow of additional FDI and an increase in the effect of creating primary jobs (Kotíková, 2018).
One of the first people who examined the secondary impact of FDI on the host economy development was Finlay (1978), who found that spillover effects were determined by so-called relative lagging, in other words, the technology gap between the parent and host economy. He claims that the larger the technology gap, the greater the space for the spillover effect to occur. His argument is based on the basic condition that a foreign investor always comes from a more advanced economy than the area where FDI is located.
On the other hand, Barrios et al. (2011), Cohen and Levinthal (2015), Cantwell (2017), Choi (2018), Konstandina and Gachino (2020) and Liu et al. (2021) have modified views on the position of the technology gap factor and its influence on the spillover effect and its impacts on economic growth. It follows from their conclusions that if the technology gap is too large, FDI could not increase economic growth through spillovers because domestic enterprises are unable to transfer technology in either the horizontal (e.g., through the FDI imitation effect) or vertical directions. A large technology gap can lead to a crowding-out effect when local firms are unable to compete with FDI. However, a too-small technology gap does not provide enough space for learning and technology transfer. Finally, it is possible to say that the positive spillover effect is most likely to occur when the technology gap is moderate (Damijan et. al., 2013).
The technology gap is closely related to the absorption capacity of domestic businesses. The absorption capacity is defined as the ability to acquire knowledge created by someone else and to modify it for one's own business needs (Pattnayak and Thangavelu, 2011). It is also considered to be a determinant of the occurrence of indirect FDI effects (Narula, 2017). Absorption capacity expresses the overall economic level of the given economy. The level of human capital is considered to be a key attribute because the inflow of FDI creates the potential for a technology transfer into the domestic business environment.
Szent-Ivanyi and Vigvári (2012) state that the identification and measurement of spillover effects is meaningful in such business environments where there are intense vertical links between domestic firms and foreign affiliates. At the same time, these affiliates must represent important employers (of the domestic local workforce), meaning an environment with an identifiable foreign presence.
Following methodology based on the findings of Blomström and Kokko (1998) and Görg and Greenway (2004), the findings of the leading researchers in the field of spillover effects Driffield and Hughes (2003) and Driffield and Love (2007) pointed out that differences within states can be more significant than between states. Therefore, it is necessary to focus on identifying local effects at lower territorial units.
Spillover effects represent a kind of accompanying effects of FDI, which, although they are not a substantial or fundamental decision-making factor on the part of foreign investors when deciding on the location of the investment, can have a very positive effect on the surroundings of the company and on the business environment of the host economy and the development of the region—so-called positive externalities. Of course, we cannot fail to mention again that FDI can also bring adverse effects—negative externalities, e.g., a possible increase in the ecological load in the region, various social problems or the crowding-out effect.
In both cases, we can talk about the so-called spillover effect, either in a positive or negative direction for the host region. With the influx of FDI into the region, in addition to economic growth, there is also a spillover of experience and capabilities resulting from the difference in the technological level between the country from which the investing company originates and the region to which the said company's investment is directed. The question then remains how to measure the spillover effect regularly.
The essential starting point of the measurement is the determination of a set of quantifiable factors on which FDI in the investigated regions have an influence, i.e., on which their direct or indirect (in the form of externalities) impact can be measured. The term region is used in this paper as an administrative unit. Based on the findings currently available to measure spillover effects and identify differences in their size and development at the level of regions within the host economy, the regional spillover effect index (SPE) has been constructed. It is composed of the following indicators, all of which positively reflect the economic growth of the host business environment:
1. Gross value added (GVA) — It can be assumed that FDI has a direct impact on the development of GVA. For investments coming from the countries with advanced technologies making high value-added products, this impact should be highly positive. However, the dependence can also be the opposite: regions with high GVA attract investment from higher GVA, i.e., FDI from countries with advanced technology. Hence, the development of GVA is both the cause of increasing foreign investment and the consequence of the inflow of foreign investment. Therefore, the weight of this factor in SPE will differ from the weight of other factors.
2. Investment in research and development (IR & D) — It can be expected that a higher level of FDI from countries with advanced technology brings new investment into research and development in the region for foreign companies to directly invest in this area. Furthermore, it is also logical to assume that areas with higher GVA will attract foreign investors with higher value-added production.
3. Proportion of the population with secondary or tertiary education (EDU) — reflects the level of human capital in the host business environment. It is possible to expect that high-tech businesses require highly qualified workers. This should, among other things, lead to cooperation between companies and schools in the region, which should consequently lead to an increase in the proportion of people with a higher level of education in the region. At the same time, this component is an indicator of human capital, which is an attribute of the absorption capability of the domestic business environment.
4. Inflow of foreign direct investment (FDII) — This is an indicator of the openness of the host business environment. It is significant that the spillover effect is directly dependent on the size of FDI in the region, which can greatly influence (above all in a positive way) the inflow of other FDI. This may happen for many reasons, including greater experience of the region with attracting new FDI, higher qualification of the population and thus a higher absorption capacity of the business environment, or the investments of buyers or suppliers in the investing companies.
5. Regional technology gap (RTG) — This is the region's ability to use the foreign presence. It can be said that the greater the inflow of FDI into a region and the more technologically advanced countries this investment comes from, the greater the region's experience and the greater the ability to use opportunities from this investment.
Each of the above-mentioned factors is considered in a relative measure, which is determined by:
1. Ratio of the absolute amount of the indicator to the population of the region (area) (per capita in the region). This will allow the relativization of the differences in the size of the economic space of the regions (analyzed areas).
2. Ratio to the benchmark. The benchmark will always be the simple arithmetic mean of the indicator of sums of values for individual regions.
3. Weight of the indicator of the relative GVA size will be half the weight in the SPE, other factors will have the same weight. The constructed final pattern of SPE (1) has the following form:
SPEn=GVAr∗1/GVABrINr+2(IRandDr∗1/IRandĐBrINr)+2(EDUrEDUB)+2(FDIIr∗1FDIIBINr)+2(RTGrRTGB)9 | (1) |
where, SPEn is the spillover effect in year n; GVAr is the relative gross value added that is ascertained from national statistics — in the case of the Czech Republic from the Czech Statistical Office (2023) — and calculated as the average level of gross value added per capita of region remeasured to the benchmark of regions (GVABr); INr is number of inhabitants of region r; IRandDr is the relative level of investment in research and development that is ascertained from national statistics — in the case of the Czech Republic from the Czech Statistical Office (2023) — and calculated as the average level of investment in research and development per capita of region r measured to the benchmark of regions (IRandDBr); EDUr is the proportion of secondary- and university-educated inhabitants of the region that is ascertained from national statistics — in the case of the Czech Republic from the Czech Statistical Office (2023) — and measured to the benchmark of regions (EDUB); FDIIr is the rate of the FDI inflow that is ascertained from national statistics — in the case of the Czech Republic from the Czech National Bank (2023) — and calculated as the FDI inflow into the region measured to the benchmark of regions (FDIB); RTGr is the regional technology gap measured to the benchmark of regions (RTGB) (Kotíková, 2018).
The constructed 'Regional Technology Gap Indicator' (RTG) from Kotíková (2019) tracks the difference between the technology levels of FDI and the regional business environment. The indicator also indicates the lag of the region behind the technology levels of MNCs, and identifies where this lag is generated: in the depth of lagging (the difference of labor productivity) or in the width of lagging (the extent of foreign presence). Based on the development of this indicator over time, it is possible to predict the future dynamics of productivity growth that a region with a high foreign presence (FP) can achieve compared to a region with a low FP.
The technology gap development is determined using the shift-share analysis method, more precisely the decomposition of the technology level of the regions to the technology effect, the employment effect and the combination of both effects. RTG is calculated according to Equation (2):
RTGn=∑ri=1(APFDILi−APregB)⋅FPregBAPregB+∑ri=1(FPFDIi−FPregB)⋅APregBAPregB+∑ri=1(APFDILi−APregB)⋅(FPFDIi−FPregB)APregB | (2) |
where APFDILi is the productivity of foreign companies of the given country in year n (OECD, 2023), which is expressed as the productivity of the investor's country of origin (reported by the OECD in USD constant prices of 2010, where the rate of use of labor inputs is measured by the total number of hours worked).APregB is the productivity of the benchmark in year n, which is expressed as the arithmetic average of the productivity of all the examined regions. Labor productivity is measured according to OECD (2023) as GDP per hour worked. This indicator is measured in USD (constant prices 2010 and PPPs).FPFDIi is the proportion of employees of the companies in the given country to the total number of people employed in the region in year n; FPregB is the benchmark of the proportion of employees in foreign companies in the examined regions in year n, which is expressed as the arithmetic average of the proportion of employees in the examined regions.
The described methodology was applied to the case of five regions of the Czech Republic. The CzechInvest (2023) records provided a list of FDI located in the Czech economy including information on regional location, investor country of origin, accorded investment incentives, sector, promises of the number of newly created jobs, field of branch and other information. This information was subsequently extended by data on the number of employees obtained from the annual reports by the analyzed companies for each year of the analyzed period. It was created using a unique data collection method completed by 211 foreign subsidiaries of MNCs, that have received an investment incentive from the state (Ministry of Justice, 2023). Data regarding the evaluation of the economic level of the business environment of the host economy (GVA, investment in science and research, proportion of the population with secondary or tertiary education), in the case of the Czech Republic, was drawn from the Czech Statistical Office (2023) and Czech National Bank (2023) report data regarding FDI inflows. The influence of these 211 FDIs is indicated on the economic development of the Czech business environment within five regions (Czech Statistical Office, 2023).
The shift-share analysis, which is the method of the RTG indicator construction, is often used in the professional literature to determine the dynamics of employment, labor productivity and value added (Zdeněk and Střeleček, 2012). Šimanová and Trešl (2011), Zdeněk and Střeleček (2012) and Dobrzanski, (2019), for example, research the shift-share analysis used in the field of labor productivity research. The decomposed variable may also be employment (Ruault and Schaeffer, 2020; Albuquerque and Lopes, 2015; Zdeněk and Střeleček, 2012) or value added (Esteban 2000). The applicability of the method is also possible in the case of other variables, where it is necessary to appraise the relationship of the monitored factors from both a static and a dynamic point of view (Sheng et al. 2021; Zdeněk and Střeleček 2012). The main advantage of the shift-share analysis is its use at any regional level (Kotíková, 2018; Lv et al., 2021).
Because of its decomposing character, a shift-share analysis is a suitable method for evaluating sustainable development. That is, whether (regional) development is driven or hampered by the economic, social or environmental component. A shift-share analysis assessing Polish regions was used by Cieślak et al. (2019), who observed abrupt changes over the years 2003 and 2013. They identified striking regional disparities and relatively low progress despite expectations and the starting position of the regions.
Dobrzanski (2019) dealt with the analysis of structural changes due to the change in labor productivity in the Czech Republic from 1996 to 2009 from the sector's point of view. With the use of a shift-share analysis, the growth of labor productivity was determined. The results showed that the growth of the structural component of productivity significantly impacted the country's economy. The highest decline in employment was found in forestry, hunting and agriculture. The main advantages of the shift-share analysis method are its ability to be used at any regional level and the option to choose the assessed components.
The above approach to determining the size (intensity) of the spillover effect has some essential features and benefits. According to the ascertained values, it is possible to determine the order of the examined regions in terms of spillover impact and to find out in which region the greatest spillover effect occurred. This approach also makes it possible to extend the set of the monitored regions and add a virtually unlimited number of other regions. Subsequently, such a set of regions can be sorted again by the intensity of the spillover effect. The differences between the single regions can be expressed in percentage points. It is also relatively easy to evaluate the trend of the SPE indicator series for individual regions.
Based on the above-mentioned methodology, the annual values of the spillover effect have been calculated for the case of the Czech Republic and its selected group of regions examined in a twenty-year time series (2002 to 2021)1.
1 2021 is the last monitored year due to current data availability.
The development of the productivity of labor during the monitored period is shown in Figure 3. The year 2002 was selected as the initial year of assessment because in that year the Czech Republic, on which the methodological procedure is demonstrated, changed the Act on Investment Incentives in order to intensify the support of foreign capital (CzechInvest, 2023).
The marked benchmark in Figure 3 shows the average productivity of the business environment of the investigated regions of the Czech Republic. It can be seen from Figure 3 that regional disparities in the Czech Republic are deepening over time. The gradual lagging of the peripheral Karlovy Vary region is evident. At the beginning of the monitored period (in 2002), the difference in labor productivity of the Karlovy Vary region compared to the benchmark was only 0.94 USD/worked hour. It was 1.56 USD/worked hour compared to the most productive region. In the last monitored year (2021), these differences were already more than three times USD 2.85/worked hour compared to the benchmark (average productivity of the regions) and USD 7/worked hour compared to the most productive region with the highest economic growth potential.
Regarding the countries of origin, FDI investors in the surveyed regions are dominated by Japan, Germany and the United Kingdom (UK). FDI investors in the Karlovy Vary region come from Germany, Japan, the Netherlands, Sweden, Switzerland, and the UK; in the Ústí nad Labem region from Austria, France, Germany, China, Japan, Israel, Luxembourg, Italy, Luxembourg, Mexico, the Netherlands, Poland, Norway, Slovenia, Spain, Sweden, Switzerland, the Republic of Korea, the UK, the USA, and Thailand; in the Liberec region from Belgium, Denmark, France, Germany, Japan, Spain, Switzerland, the UK, and the USA; in the Hradec Králové region from Austria, Belgium, France, Germany, Luxembourg, the Netherlands, Poland, the UK, and the USA; in the Pardubice region from Denmark, France, Germany, India, Italy, Japan, the Netherlands, Spain, Switzerland, and the UK.
Table 1 shows the results of the descriptive statistics of the technology gap calculation and Figure 4 tracks RTG and compares it for all five Czech regions. The amount of RTG is determined by the number of newly arrived and still-operating MNCs in the host economy.
Region | Standard deviation (RTG) (per region) | Average value (per region) | Minimum (per region) | Maximum (per region) | Variance (per region) | Median (per region) |
Karlovy Vary region | 0.0187 | 0.02548 | 0.00884 | 0.07040 | 0.00035 | 0.01897 |
Ústí nad Labem region | 0.0265 | 0.11074 | 0.04722 | 0.14493 | 0.00071 | 0.11686 |
Liberec region | 0.0369 | 0.08161 | 0.01731 | 0.13873 | 0.00136 | 0.09223 |
Hradec Králové region | 0.0375 | 0.07947 | 0.02782 | 0.14777 | 0.00141 | 0.08701 |
Pardubice region | 0.0337 | 0.10209 | 0.05659 | 0.16859 | 0.00114 | 0.10244 |
Average (per set of regions) | 0.0307 | 0.07988 | 0.03156 | 0.13408 | 0.00099 | 0.08350 |
Minimum (per set of regions) | 0.0187 | 0.02548 | 0.00884 | 0.07040 | 0.00035 | 0.01897 |
Maximum (per set of regions) | 0.0375 | 0.11074 | 0.05659 | 0.16859 | 0.00141 | 0.11686 |
Source: Own calculations based on company data of MNCs (Ministry of Justice, 2023), statistical data of regional economic development (Czech Statistical Office, 2023), FDI inflow data (Czech National Bank, 2023), labor productivity data (OECD, 2023). |
Regions unable or unwilling to attract foreign investors from advanced economies need less forfeited prospects for growth through technological transfers. Pardubice exhibits a deepening, nearly uninterrupted decline in RTG values over the period, notwithstanding growth in early 2003 and late 2014. This finding indicates that the region could realize growth potential through foreign technology transfers. Therefore, it is surprising that labor productivity there grew most dynamically of all the regions during the sampled period (Figure 3). The Pardubice region has the potential for self-reliant growth owing to its economic position in the Czech Republic and a wide representation of foreign companies. Thus, the region did not have to be in the center of public support both from the representation of the region itself and from the representation of the state. A small interest in growth potential using the transfer of technological knowledge can also be seen by foreign firms that have received investment incentives alongside the development of the RTG and of the proportion of employees of foreign firms with investment incentives in the total number of employees of the Pardubice region. The Pardubice region never exceeded 4%.
The calculated values of RTG of the Karlovy Vary region were the lowest. It can be said that while the other regions have undergone a relatively turbulent development, the Karlovy Vary region's RTG development curve is relatively (in comparison with other regions) consistent in the form of a nearly flat parabola. The size of the technology gap, expressed by the RTG indicator, in the Karlovy Vary region has gone through a period of initial decline that reached its minimum in the period that culminated in the European and global financial and economic crises. This corresponds to a global decline in MNCs' investment activities due to perceived uncertainty in this period. Since 2010, the RTG in the Karlovy Vary region has been increasing, including a notable fall in 2013 caused by a decrease in the proportion of employees in foreign companies with investment incentives. Specifically, this was the departure of a German investor operating in the plastics and rubber industry (CzechInvest, 2023).
The Ústí nad Labem region, a peripheral region, is often discussed because of its economic and social development. The monitoring of this region could also provide a response to the often-discussed question of whether the use of investment incentive policies is successful in attracting foreign investment in problematic regions, where the investment incentives should play a key role in improving the situation in the region, especially in the labor market but also in the overall economic development of the region.
Looking at Fig. 4 of the RTG development, it is possible to mention quite a positive finding — the region has been able to increase the value of the RTG over the entire monitored period. In 2013, the RTG fell sharply. The explanation is the correspondingly sharp fall in foreign presence (FP) due to a change in the recruitment of new employees (HR outsourcing is a current trend). FDI supplies employees through specialized agencies, though these employees are employees of the agency, not of the FDI subject. They are not reported in annual reports as ad hoc employees and they cannot be fully included in the foreign presence.
Table 2 shows descriptive statistics of the SPE index and Table 3 presents the resulting values of the SPE index for the evaluated group of regions.
Region | Average value (per region) | Minimum (per region) | Maximum (per region) | Variance (per region) | Standard deviation (per region) | Median (per region) |
Karlovarský | 0.52757 | 0.36431 | 1.05741 | 0.05053 | 0.22478 | 0.38881 |
Ústecký | 0.96545 | 0.63590 | 1.74404 | 0.08264 | 0.28747 | 0.85105 |
Liberecký | 0.95786 | 0.78842 | 1.44544 | 0.03779 | 0.19439 | 0.85827 |
Královehradecký | 0.84457 | 0.70036 | 1.22757 | 0.02047 | 0.14306 | 0.78858 |
Pardubický | 1.04174 | 0.86022 | 1.50283 | 0.03578 | 0.18915 | 0.97313 |
Average (per set of regions) | 0.86744 | 0.66984 | 1.39546 | 0.04544 | 0.20777 | 0.77197 |
Minimum (per set of regions) | 0.52757 | 0.36431 | 1.05741 | 0.02047 | 0.14306 | 0.38881 |
Maximum (per set of regions) | 1.04174 | 0.86022 | 1.74404 | 0.08264 | 0.28747 | 0.97313 |
Source: Own calculations based on company data of MNCs (Ministry of Justice, 2023), statistical data of regional economic development (Czech Statistical Office, 2023), FDI inflow data (Czech National Bank, 2023), labor productivity data (OECD, 2023). |
Year/Region | Karlovy Vary | Ústí nad Labem | Liberec | Hradec Králové | Pardubice |
2002 | 0.72774 | 0.91753 | 1.17554 | 1.04130 | 1.05141 |
2003 | 0.94477 | 0.63590 | 1.05951 | 1.22757 | 1.00846 |
2004 | 0.90959 | 0.81988 | 0.81525 | 0.92789 | 1.50283 |
2005 | 0.84676 | 1.08698 | 1.06097 | 0.84782 | 1.08596 |
2006 | 0.53845 | 1.10068 | 1.44544 | 0.81746 | 1.05127 |
2007 | 0.52572 | 0.90846 | 0.98133 | 1.06746 | 1.34932 |
2008 | 1.05741 | 0.73407 | 0.91111 | 0.75120 | 1.29566 |
2009 | 0.36431 | 0.78526 | 1.41975 | 0.83909 | 0.93663 |
2010 | 0.37142 | 0.98320 | 1.13346 | 0.85390 | 1.40367 |
2011 | 0.37932 | 1.70463 | 0.78842 | 0.74030 | 0.93002 |
2012 | 0.40945 | 1.26137 | 0.88000 | 1.05970 | 0.86022 |
2013 | 0.38474 | 0.78193 | 0.84383 | 0.70560 | 1.06413 |
2014 | 0.41497 | 1.74404 | 0.83737 | 0.83428 | 0.86895 |
2015 | 0.37665 | 0.84851 | 0.80085 | 0.70036 | 0.91540 |
2016 | 0.38089 | 0.83494 | 0.85681 | 0.70278 | 0.93780 |
2017 | 0.38641 | 0.77312 | 0.79581 | 0.75970 | 1.02261 |
2018 | 0.38031 | 0.82774 | 0.85973 | 0.75476 | 0.90407 |
2019 | 0.39121 | 0.85144 | 0.84610 | 0.75715 | 0.86965 |
2020 | 0.37976 | 0.85872 | 0.83862 | 0.75612 | 0.88560 |
2021 | 0.38158 | 0.85065 | 0.80734 | 0.74702 | 0.89107 |
Source: Own calculations based on company data of MNCs (Ministry of Justice, 2023) statistical data of regional economic development (Czech Statistical Office, 2023), FDI inflow data (Czech National Bank, 2023), labor productivity data (OECD, 2023). |
From Table 3, it is clear that within the analyzed period, the greatest rate of spillover increase was detected in the Pardubice region, while the Karlovy Vary region recorded the greatest rate of spillover decline.
At the same time, from the perspective of the individual indicators (GVA, FDII, IR & D, RTG, EDU), the Karlovy Vary region falls behind the other regions in all the analyzed values. On the contrary, Pardubice region has high labor productivity in the whole analyzed period.
An interesting fact is that the highest value of the spillover indicator of all the examined regions, 1.74, was reached in the Ústí nad Labem region in 2014 when the largest FDI entered the region so far within the entire Czech Republic — the FDI of the Korean company NEXEN Tires with 1,384 created new jobs. It was an investment worth almost EUR 850 million. At the same time, another 19 investors located their FDI in the region this year. Together, these investment actions created a similar number of new jobs as the FDI of NEXEN Tires, 1,396 (CzechInvest, 2023).
On the other hand, the least successful, the Karlovy Vary region, reached the lowest value of the spillover indicator in 2009 (specifically 0.36). This value indicates that in the mentioned year, the spillover effect in the region was half as small than the average of all the examined regions. A relatively stable to slightly volatile level of spillover effect can be seen in the Hradec Králové and Liberec regions. The values of the spillover effect in these regions were mostly around the average level. Strongly volatile development during the analyzed period was recorded in the Ústí nad Labem region. The strong lagging behind of the Karlovy Vary region is a very negative reality. However, the volatile development in Ústí nad Labem can also be evaluated as a negative feature. A graphical comparison of the spillover effect development for the individual regions is shown in Figure 5.
This graphical comparison also illustrates more clearly the increase in the difference of the size of the spillover effect between the examined regions in the analyzed time series. This development can be assessed negatively because it indicates the deepening of socio-economic disparities between regions, especially in the first half of the period. At the beginning of the monitored period, between 2002 and 2005, there was a comparable size of the spillover effect between all the individual regions. In the period between 2009 and 2015, the values of the spillover effect differed significantly between the regions. Given that in the first mentioned time period (2002–2005), the Czech economy had started to report strong economic growth culminating in 2006, and to the contrary, in the second period (2009–2013) the domestic economy was in a recession, it is possible to state that the development of any spillover effect is dependent of the economic development of the country. Since 2015, the development has been relatively stable but without growth tendencies.
The position of the Karlovy Vary region, which indicates a warning sign for economic policy makers, is worth mentioning — the Karlovy Vary region is the region with the lowest level of foreign presence and at the same time the region with the lowest labor productivity. The Karlovy Vary region has not managed to get away from the crisis and the values since 2009 have been more than alarming: The existing institutional support obviously does not contribute to the elimination of regional disparities; on the contrary, it is possible to identify a significant regional grouping of the core-periphery. However, the absorption capacity of the region to receive and benefit from foreign presence, including creating spillover effects, is at a very low level.
This article quantified the spillover effect of FDI for a given group of regions within the host economy. The spillover effect was evaluated based on five criteria: GVA, RTG, investment in R & D, share of people with secondary and higher education and inflow of FDI. Each of these indicators positively affects the economic growth of the host state and improves the quality of the business environment (Szent-Ivanyi and Vigvári, 2012). The higher the SPE index value is achieved in the region (economy), the higher the positive effect of FDI on the quality of the host business environment. However, previous studies also point to the negative effect of FDI inflows on the business environment. These effects mainly include the crowding-out effect, when domestic companies are displaced from the market by incoming foreign companies. Often, this effect also manifests itself in reducing employment in domestic companies.
This also includes the creation of a dual economy, when the influx of FDI causes the creation of two parallel markets. In one, foreign, heavily capitalized companies offering products with high added value operate, and in the other, less powerful domestic companies are unable to compete due to their lower productivity, producing lower quality products for different customer segments (Benáček et al., 2014). Even these, at first sight, adverse effects on the domestic business environment must be understood in the context of the possibility of increasing competitiveness in the examined market; resp., an increase in competition in the given market can always be perceived as a positive impact. Domestic companies are forced to improve the quality of their processes (production process, management, customer relations, corporate culture, PR, production efficiency, pricing policy, supplier-customer relations, etc.).
It can be stated that the inflow of FDI has a predominantly positive effect on the quality of the business environment, and the expected strength of this effect can be measured by the development of the creation of primary jobs due to the inflow of FDI or the spillover effect. The higher the influence of FDI on the creation of primary jobs in the region or the higher the spillover effect subsequently identified in the region, the more significant the positive effect of the inflow of FDI on the business environment can be expected.
The relationships mentioned above between economic growth and the development of the host market and between the development of the spillover effect and the quality of the business environment can also be examined in the set of investigated regions. For a given set of regions, the spillover effect was identified and measured by calculating the technological gap using the shift-share analysis method and the Spillover index in the twenty-year time series of 2002–2021.
The Karlovy Vary region is an outsider in the examined set of regions of the Czech Republic, achieving the lowest values in all monitored indicators. The results found for the Karlovy Vary Region correspond to the conclusions of Pavlínek and Žížalová (2016), who draw attention to the handicap of the educational infrastructure of this region and the organizational leanness of the regional innovation system, manifested by low awareness and the degree of cooperation and the technological lock-in effect (Šimanová and Trešl, 2011). This handicap is exacerbated over time by the persistent low foreign presence (FP) and low penetration of MNCs in the region.
This finding corresponds with the conclusions of Burger et al. (2017), Damijan et al. (2013) and Wolff and Blomström (1994), who agree that the low penetration of foreign firms creates a low potential for spillover effects and low competitive pressure, and therefore a lower possibility of stimulating the economic growth of the host economy.
Spillover effects are complicated to measure (Benáček et al., 2014; Crespo et al, 2009). Their quantification takes place through proxy variables (number of employees, size of FDI inflow, GVA or GDP, investments, educational indicators, labor productivity or aggregate productivity of factors, etc.). The main reasons why it is difficult to identify the impact of spillover effects on the economic development of the host market can be attributed to the behavior of the companies themselves. Foreign investors guard their specific advantages and purposefully prevent leakages to domestic companies. Therefore, the main tool by which domestic enterprises can increase their productivity in the presence of foreign economic entities is the competitive pressure that forces domestic firms to introduce more efficient production techniques. However, this often harms domestic productivity values in the short term.
Multinational companies tend to invest in sectors where they can maintain a dominant position or where domestic competitors are unable to imitate, i.e., in sectors or business environments with low absorptive capacity (Hardy et al., 2011), which corresponds to the results for the Ústí nad Labem region, where more than 100 MNCs invested from the examined sample of 211 companies). Moreover, at the same time, the labor productivity of this region does not even reach the average values of the benchmark and the values of the Spillover index increase only in the years of relatively significant investment inflows (2009–2011 and 2013–2015), but subsequently, the region and its business environment do not use the potential of this influx, and the values of the SPE index do not deviate in any way (see Figure 5 in period 2015–2021). The highest values of the SPE index were measured in the region in 2014, when the largest FDI was placed in the Czech Republic (CzechInvest, 2023). However, the region did not manage to use the potential of this capital inflow in the long term, and the value of the SPE index decreased over time. These results can be identified with the conclusions of Massey (2007) talking about the so-called cathedrals in the desert when the host economy fails to use the potential of significant FDI to support the sufficient creation of subcontracting links.
Inter-regional differences, significantly increasing differences in measured labor productivity, indicate growing inter-regional disparities, which institutions cannot erase effectively in time. These findings correspond to the conclusions of Driffield and Hughes (2003) and Driffield and Love (2007), who emphasize monitoring the impact of FDI at the regional level within the host state.
The positive spillover effect only applies to a particular group of companies. Thus, the quantification of the spillover effect, to some extent, underestimates the true significance of this effect (Hardy et al., 2011). The heterogeneity of enterprises in terms of absorptive capacity, size, productivity and level of technology influences spillover effects. Positive horizontal impacts are evenly distributed across firm size groups, while negative horizontal impacts are likely to increase for smaller firms. In addition, positive horizontal inflows occur in medium-sized or high-productivity firms with higher absorptive capacities, while negative horizontal spillovers are likely to affect low- to medium-productivity firms. At the same time, these results suggest that foreign presence may also affect smaller firms to a greater extent than larger firms (Damijan et al., 2013). Obtaining information about small companies is, however, connected to the complicated data collection related to the indicators in question.
The trade regime of the host economy conditions the nature of the spillover. Suppose a foreign company establishes itself in a country that stimulates exports. In that case, contacts with domestic firms will not be established to the extent that would occur in the opposite trade regime. Multinational firms will rely more on their advantages in global distribution networks or international marketing than on production technologies, which causes a lower potential for the spillover effect. On the contrary, in pro-import-oriented areas, the foreign firm must bring a higher range of technological advantages, which can stimulate spillover effects more (Bitzer et al., 2008; Du et al., 2023). Agosin and Mayer (2000) further point out that the evaluation of the effects of FDI is challenging because many influencing factors are connected with this issue—whether it is the policy of the host country, relations between the mother and the host country, the type of FDI, the power of MNCs and the strength of domestic enterprises, etc.
There is empirical evidence that policies that leave local governments free to design policies that attract FDI and thus provoke competition between them has been very beneficial and boosted economic growth in the country (Wang et al., 2016). Mariotti and Marzano (2021) add, based on data from 63 countries for the period 1980 to 2017, that institutional steps supporting the economic competition regime have a significant effect on attracting new FDI. The results showed that promoting actions to create competition among foreign investors supports attracting new investments, mainly in countries with a high-quality regulatory institutional environment. In addition to Asian and South American countries, the 63 countries that were the subject of the research also included European countries, including the Czech Republic. Colen et al. (2016) point out that, in addition to investment incentives, support for bilateral investment agreements, which can be more effective in attracting FDI, can help the host country's economic growth. Positive results in attracting investments using bilateral agreements were found mainly in the real estate and public services sector, as these sectors are characterized by high sunk costs in the case of countries of the former USSR, where economic transformation took place (including the Czech Republic).
Regarding the development of the values of RTG (see Figure 4), the greatest technology gap was recorded in the Ústí nad Labem region. In 2013 the RTG fell sharply. The explanation is a change in the recruitment of new employees. FDI supplies employees through specialized agencies, and these employees are employees of the agency, not of the FDI subject. They are not reported in annual reports as ad hoc employees and they cannot be fully included in the foreign presence.
The RTG values of the other regions decreased over time. Positive results were observed in larger regions. Gutierrez-Portilla et al. (2019) point out that the larger the market size, the more attractive the region and the more indirect effects may take place.
The best results were achieved by regions with larger market sizes, which correspond to the results of Regelink and Elhorst (2015). The regions with agglomeration effects have a greater potential for assuming the effects of FDI. Similar conclusions were also reached by Wu and Burge (2018), who analyzed the effects of FDI localization in Chinese provinces supported by government policies.
The results correspond with research dealing with absorptive capacity and FDI inflow by Popadynets et al. (2023) and Tkalenko et al. (2022) at the regional level in Ukraine. But attention must be paid to the significant limits of the Ukrainian regions to absorb localized foreign capital and the current security situation in this area.
The distribution of effects is strongly influenced by population distribution and GDP effects. This finding applies to spillover effects and socio-economic impacts on host markets, such as distribution key settings for refugee quotas (Grech, 2017).
Finally, it is also possible to mention the flexibility of the constructed SPE index. The indicator is modifiable — among these possible modifications, the following approaches can be included:
1. It would be possible to compare the productivity of a foreign company with the productivity of the region (instead of the productivity of the benchmark).
2. To simplify this approach, it would be possible to work with the average productivity of foreign companies operating in the region/country (instead of the productivity of each individual company by its country of origin). On the other hand, when looking at the country of origin, the research provides more comprehensive information on the behavior of MNCs by country of origin. As reported by Li et al. (2018), the country of origin of FDI plays an essential role in locating and maintaining investments in the host region.
The described approach has to be taken in space and time with certain limitations and notes:
1. It does not take into account the interconnectedness of the regions (e.g., the transfer of acquired technology knowledge gained from FDI between regions).
2. It does not take into account the interaction between companies in the region or between regions. The so-called third-country (region) effect (Fonseca and Llamosas-Rosas, 2019) is not taken into account.
3. It does not consider the absolute rate of contribution of foreign companies to the region. The absolute benefit of the presence of foreign companies in the region could grow over time even if the RTG falls or stagnates — which would be due to the over-proportional growth of the foreign presence (FP) in the other regions of the benchmark, in the extreme case also assuming that labor productivity in the region has grown at a much higher rate than the productivity of incoming foreign companies (the country of origin of investors). A region with a historically high rate of foreign presence of FDI/MNCs may, in terms of the RTG indicator, stagnate or decrease due to the saturation of the region by the foreign presence.
4. It does not consider outsourcing in the area of human resources. Only employees reported in the annual reports enter the foreign presence. It does not take into account the delivery of agency employees.
5. The absence of data is an important limitation for the expansion of research. So far, there is no specified database or system to monitor FDI flows at the regional level, which would make it possible to conduct a comprehensive comparison not only within one country but also between countries, for example, when evaluating technology transfers in border regions in the areas of three‑terrain, etc.
Whether the overall indirect effect of FDI in the host region is positive or negative, and whether any spillover effects arise at all, depends on many factors. These are mainly the size of the host market and the level of competition there, the absorptive capacity of domestic companies, the adaptability of workers, the institutional environment, the size of the foreign presence and others (Kotíková, 2018; Szent‑Ivanyi and Vigvári, 2012).
Individual governments spend considerable funds (including in the form of investment incentives) to obtain positive effects that are considered self-evident but, in reality, are very difficult to measure (Benáček et al., 2014; Kotíková, 2019). On the other hand, it may not even manifest itself in the host region. The investment incentives granted may cause the emergence of the crowding-out effect of FDI or the emergence of a so-called dual economy, where, on the one hand, there are capital-strong foreign companies with advanced technologies, quality management and the efficient organization of production, and on the other hand, domestic producers who, due to their availability of production factors, are not able to compete with them, nor to cooperate with them and join supply chains.
The current setting of investment incentives (also in the case of the Czech Republic) is based on Keynesian core-periphery concepts (Blažek and Květoň, 2023). It attracts investors primarily to areas with high unemployment and low economic performance. This setting already weakens the potential positive effects both in core and peripheral regions. Peripheral regions achieve low economic performance themselves. The results achieved in this research correspond precisely to this, where the Ústí (peripheral) region achieves a low value of the spillover effect compared to other regions, even though it is a region where more than 50% of investment incentives were directed (101 MNCs from the examined sample placed its FDI in this region). The question remains as to what kind of inflow of investment the investment incentives would have to attract to the region for the growth of spillover effects or for the growth of the potential for these effects to occur. Without the dispersion of business activities into peripheral areas, which occurs due to the setting of investment incentives, the effects in the so-called core regions would intensify.
The proposed methodology has the potential to enhance the understanding of the impact of FDI on regional business environments for policymakers and investors, facilitating more informed decision-making. A certain filling of the current gap in the existing economic research focused on the identification of the FDI effects at the lower regional level can also be considered a contribution to the scientific field. This approach allows the virtually unlimited expansion of the set of the monitored regions. All the constructed indicators are applicable also to other regional units in other countries.
The research provides recommendations for economic policy makers regarding the regions they should focus on in the field of investment support for foreign investment attraction to increase economic growth, as well as which regions are able to benefit from, and to what extent, the presence of FDI in their business environment.
This methodology and constructed SPE index represent a supporting tool for economic policymakers to evaluate the quality of the business environment of the given market. For, as Fonseca and Llamosas-Rosas (2019) agree, understanding the determinants of FDI helps to modify future public strategies toward regional development.
Future research should be also focused on evaluating other regional units from special geographical areas. In so doing, it may be possible to offer more complex recommendations and comparisons of the investment incentives systems of analyzed countries, which could reflect or indicate comparative advantages. However, the problem with extending research in this direction continues to come in the form of a lack of data.
The author declares they have not used Artificial Intelligence (AI) tools in the creation of this article.
The author declares no conflicts of interest in this paper.
[1] | M. Rautio, H. Mariash and L. Forsstrom, Seasonal shifts between autochthonous and allochthon-ous carbon contributions to zooplankton diets in a subarctic lake, Limnol. Oceanogr., 56 (2011), 1513–1524. |
[2] | S. E. Jones, C. T. Solomon and B. C. Weidel, Subsidy or subtraction: how do terrestrial inputs influence consumer production in lakes, Freshwater Rev., 5 (2012), 37–49. |
[3] | K. A. Emery, G. M. Wilkinson, M. L. Pace, et al., Use of allochthonous resources by zooplankton in reservoirs, Hydrobiologia, 758 (2015), 257–269. |
[4] | J. Cole, S. R. Carpenter, J. F. Kitchell, et al., Differential support of lake food webs by three types of terrestrial organic carbon, Ecol. Lett., 9 (2006), 558–568. |
[5] | M. Berggren, S. E. Ziegler, N. F. Gelais, et al., Contrasting patterns of allochthony among three major groups of crustacean zooplankton in boreal and temperate lakes, Ecology, 95 (2014), 1947–1959. |
[6] | M. T. Brett, M. Kainz, S. J. Taipale, et al., Phytoplankton, not allochthonous carbon, sustains herbivorous zooplankton production, Proc. Natl. Acad. Sci. USA, 21 (2009), 197–201. |
[7] | M. Berggren, A. Bergstrom and J. Karlsson, Intraspecific autochthonous and allochthonous resource use by zooplankton in a humic lake during the transitions between winter, summer and fall, Plos One, 10 (2014), e0120575. |
[8] | A. D. Persaud and P. J. Dillon, Differences in zooplankton feeding rates and isotopic signatures from three temperate lakes, Aquat. SCI., 73 (2011), 261–273. |
[9] | N. D. Lewis, M. N. Breckels, M. Steinke, et al., Role of infochemical mediated zooplankton grazing in a phytoplankton competition model, Ecol. Complex., 16 (2013), 41–50. |
[10] | Y. Lv and Y. Pei, Harvesting of a phytoplankton-zooplankton model, Nonlinear Anal. Real. World. Appl., 11 (2010), 3608–3619. |
[11] | A. Sharma, A. K. Sharma and K. Agnihotri, Analysis of a toxin producing phytoplankton zooplankton interaction with Holling IV type scheme and time delay, Nonlinear Dynam., 81 (2015), 13–25. |
[12] | J. E. Truscott and J. Brindley, Ocean plankton population as excitable media, B. Math. Biol., 56 (1994), 981–998. |
[13] | Y. Pei, Y. Lv and C. Li, Evolutionary consequences of harvesting for a two-zooplankton one-phytoplankton system, Appl. Math. Model, 36 (2012), 1752–1765. |
[14] | L. Qian, Q. Lu, Q. Meng et al., Dynamical behaviors of a prey-predator system with impulsive control, J. Math. Anal. Appl., 1 (2010), 345–356. |
[15] | S. R. Jang and E. J. Allen, Deterministic and stochastic nutrient phytoplankton zooplankton models with periodic toxin producing phytoplankton, Appl. Math. Comput., 271 (2015), 52–67. |
[16] | S. Ruan, Persistence and coexistence in zooplankton-phytoplankton nutrient models with instantaneous nutrient recycling, J. Math. Biol., 31 (1993), 633–654. |
[17] | J. S. Wroblewski, S. L. Sarmiento and G. R. Flierl, An ocean basin scale model of plankton dynamics in the North Atlantic, solutions for the climatological oceanographic condition in May, Global Biogeochem. CY., 2 (1988), 199–218. |
[18] | A. J. Tanentzap, B. W. Kielstra, G. M. Wilkinson, et al., Terrestrial support of lake food webs: synthesis reveals controls over cross-ecosystem resource use, Sci. Adv., 3 (2017), e1601765. |
[19] | B. C. Mcmeans, A. Koussoroplis, M. T. Arts, et al., Terrestrial dissolved organic matter supports growth and reproduction of Daphnia magna when algae are limiting, J. Plankton. Res., 0 (2015), 1–9. |
[20] | U. Forys, M. Qiao and A. Liu, Asymptotic dynamics of a deterministic and stochastic predator- prey model with disease in the prey species, Math. Method. Appl. Sci., 37 (2014), 306–320. |
[21] | G. Ge, H. Wang and J. Xu, A stochastic analysis for a phytoplankton zooplankton model, JPCS, 96 (2008), 012168. |
[22] | P. Ghosh, P. Das and D. Mukherjee, Persistence and stability of a seasonally perturbed three species stochastic model of salmonoid aquaculture, Differ. Equ. Dyn. Syst., 2016. Available from: https://doi.org/10.1007/s12591-016-0283-0. |
[23] | C. Ji, D. Jiang and N. Shi, A note on a predator-prey model with modified Leslie-Gower and Holling-type II schemes with stochastic perturbation, J. Math. Anal. Appl., 377 (2011), 435–440. |
[24] | J. Djordjevic, C. J. Silva and D. F. Torres, A stochastic SICA epidemic model for HIV transmission, Appl. Math. Lett., 84 (2018), 168–175. |
[25] | R. Rajaji and M. Pitchaimani, Analysis of stochastic viral infection model with immune impairment, Int. J. Ap. Mat. Com-Pol., 3 (2017), 3561–3574. |
[26] | F. C. Klebaner, Introduction to Stochastic Calculus with Applications, 2nd edition, World Scientific Publishing, London, 2005. |
[27] | X. Mao, Stochastic Diferential Equations and Applications, 1nd edition, Horwood Publishing, Chichester, UK, 2008. |
[28] | N. D. Lewis, M. N. Breckels, S. D. Archer, et al., Grazing-induced production of DMS can stabilize food-web dynamics and promote the formation of phytoplankton blooms in a multitrophic plankton model, Biogeochem., 110 (2012), 303–313. |
[29] | D. J. Higham, An algorithmic introduction to numerical simulation of stochastic differential equations, SIAM Rev., 43 (2001), 525–546. |
[30] | N. Dalal, D. Greenhalgh and X. Mao, A stochastic model for internal HIV dynamics, J. Math. Anal. Appl., 341 (2008), 1084–1101. |
[31] | L. Shaikheta and A. Korobeinikov, Stability of a stochastic model for HIV-1 dynamics within a host, Appl. Anal., 6 (2016), 1228–1238. |
Region | Standard deviation (RTG) (per region) | Average value (per region) | Minimum (per region) | Maximum (per region) | Variance (per region) | Median (per region) |
Karlovy Vary region | 0.0187 | 0.02548 | 0.00884 | 0.07040 | 0.00035 | 0.01897 |
Ústí nad Labem region | 0.0265 | 0.11074 | 0.04722 | 0.14493 | 0.00071 | 0.11686 |
Liberec region | 0.0369 | 0.08161 | 0.01731 | 0.13873 | 0.00136 | 0.09223 |
Hradec Králové region | 0.0375 | 0.07947 | 0.02782 | 0.14777 | 0.00141 | 0.08701 |
Pardubice region | 0.0337 | 0.10209 | 0.05659 | 0.16859 | 0.00114 | 0.10244 |
Average (per set of regions) | 0.0307 | 0.07988 | 0.03156 | 0.13408 | 0.00099 | 0.08350 |
Minimum (per set of regions) | 0.0187 | 0.02548 | 0.00884 | 0.07040 | 0.00035 | 0.01897 |
Maximum (per set of regions) | 0.0375 | 0.11074 | 0.05659 | 0.16859 | 0.00141 | 0.11686 |
Source: Own calculations based on company data of MNCs (Ministry of Justice, 2023), statistical data of regional economic development (Czech Statistical Office, 2023), FDI inflow data (Czech National Bank, 2023), labor productivity data (OECD, 2023). |
Region | Average value (per region) | Minimum (per region) | Maximum (per region) | Variance (per region) | Standard deviation (per region) | Median (per region) |
Karlovarský | 0.52757 | 0.36431 | 1.05741 | 0.05053 | 0.22478 | 0.38881 |
Ústecký | 0.96545 | 0.63590 | 1.74404 | 0.08264 | 0.28747 | 0.85105 |
Liberecký | 0.95786 | 0.78842 | 1.44544 | 0.03779 | 0.19439 | 0.85827 |
Královehradecký | 0.84457 | 0.70036 | 1.22757 | 0.02047 | 0.14306 | 0.78858 |
Pardubický | 1.04174 | 0.86022 | 1.50283 | 0.03578 | 0.18915 | 0.97313 |
Average (per set of regions) | 0.86744 | 0.66984 | 1.39546 | 0.04544 | 0.20777 | 0.77197 |
Minimum (per set of regions) | 0.52757 | 0.36431 | 1.05741 | 0.02047 | 0.14306 | 0.38881 |
Maximum (per set of regions) | 1.04174 | 0.86022 | 1.74404 | 0.08264 | 0.28747 | 0.97313 |
Source: Own calculations based on company data of MNCs (Ministry of Justice, 2023), statistical data of regional economic development (Czech Statistical Office, 2023), FDI inflow data (Czech National Bank, 2023), labor productivity data (OECD, 2023). |
Year/Region | Karlovy Vary | Ústí nad Labem | Liberec | Hradec Králové | Pardubice |
2002 | 0.72774 | 0.91753 | 1.17554 | 1.04130 | 1.05141 |
2003 | 0.94477 | 0.63590 | 1.05951 | 1.22757 | 1.00846 |
2004 | 0.90959 | 0.81988 | 0.81525 | 0.92789 | 1.50283 |
2005 | 0.84676 | 1.08698 | 1.06097 | 0.84782 | 1.08596 |
2006 | 0.53845 | 1.10068 | 1.44544 | 0.81746 | 1.05127 |
2007 | 0.52572 | 0.90846 | 0.98133 | 1.06746 | 1.34932 |
2008 | 1.05741 | 0.73407 | 0.91111 | 0.75120 | 1.29566 |
2009 | 0.36431 | 0.78526 | 1.41975 | 0.83909 | 0.93663 |
2010 | 0.37142 | 0.98320 | 1.13346 | 0.85390 | 1.40367 |
2011 | 0.37932 | 1.70463 | 0.78842 | 0.74030 | 0.93002 |
2012 | 0.40945 | 1.26137 | 0.88000 | 1.05970 | 0.86022 |
2013 | 0.38474 | 0.78193 | 0.84383 | 0.70560 | 1.06413 |
2014 | 0.41497 | 1.74404 | 0.83737 | 0.83428 | 0.86895 |
2015 | 0.37665 | 0.84851 | 0.80085 | 0.70036 | 0.91540 |
2016 | 0.38089 | 0.83494 | 0.85681 | 0.70278 | 0.93780 |
2017 | 0.38641 | 0.77312 | 0.79581 | 0.75970 | 1.02261 |
2018 | 0.38031 | 0.82774 | 0.85973 | 0.75476 | 0.90407 |
2019 | 0.39121 | 0.85144 | 0.84610 | 0.75715 | 0.86965 |
2020 | 0.37976 | 0.85872 | 0.83862 | 0.75612 | 0.88560 |
2021 | 0.38158 | 0.85065 | 0.80734 | 0.74702 | 0.89107 |
Source: Own calculations based on company data of MNCs (Ministry of Justice, 2023) statistical data of regional economic development (Czech Statistical Office, 2023), FDI inflow data (Czech National Bank, 2023), labor productivity data (OECD, 2023). |
Region | Standard deviation (RTG) (per region) | Average value (per region) | Minimum (per region) | Maximum (per region) | Variance (per region) | Median (per region) |
Karlovy Vary region | 0.0187 | 0.02548 | 0.00884 | 0.07040 | 0.00035 | 0.01897 |
Ústí nad Labem region | 0.0265 | 0.11074 | 0.04722 | 0.14493 | 0.00071 | 0.11686 |
Liberec region | 0.0369 | 0.08161 | 0.01731 | 0.13873 | 0.00136 | 0.09223 |
Hradec Králové region | 0.0375 | 0.07947 | 0.02782 | 0.14777 | 0.00141 | 0.08701 |
Pardubice region | 0.0337 | 0.10209 | 0.05659 | 0.16859 | 0.00114 | 0.10244 |
Average (per set of regions) | 0.0307 | 0.07988 | 0.03156 | 0.13408 | 0.00099 | 0.08350 |
Minimum (per set of regions) | 0.0187 | 0.02548 | 0.00884 | 0.07040 | 0.00035 | 0.01897 |
Maximum (per set of regions) | 0.0375 | 0.11074 | 0.05659 | 0.16859 | 0.00141 | 0.11686 |
Source: Own calculations based on company data of MNCs (Ministry of Justice, 2023), statistical data of regional economic development (Czech Statistical Office, 2023), FDI inflow data (Czech National Bank, 2023), labor productivity data (OECD, 2023). |
Region | Average value (per region) | Minimum (per region) | Maximum (per region) | Variance (per region) | Standard deviation (per region) | Median (per region) |
Karlovarský | 0.52757 | 0.36431 | 1.05741 | 0.05053 | 0.22478 | 0.38881 |
Ústecký | 0.96545 | 0.63590 | 1.74404 | 0.08264 | 0.28747 | 0.85105 |
Liberecký | 0.95786 | 0.78842 | 1.44544 | 0.03779 | 0.19439 | 0.85827 |
Královehradecký | 0.84457 | 0.70036 | 1.22757 | 0.02047 | 0.14306 | 0.78858 |
Pardubický | 1.04174 | 0.86022 | 1.50283 | 0.03578 | 0.18915 | 0.97313 |
Average (per set of regions) | 0.86744 | 0.66984 | 1.39546 | 0.04544 | 0.20777 | 0.77197 |
Minimum (per set of regions) | 0.52757 | 0.36431 | 1.05741 | 0.02047 | 0.14306 | 0.38881 |
Maximum (per set of regions) | 1.04174 | 0.86022 | 1.74404 | 0.08264 | 0.28747 | 0.97313 |
Source: Own calculations based on company data of MNCs (Ministry of Justice, 2023), statistical data of regional economic development (Czech Statistical Office, 2023), FDI inflow data (Czech National Bank, 2023), labor productivity data (OECD, 2023). |
Year/Region | Karlovy Vary | Ústí nad Labem | Liberec | Hradec Králové | Pardubice |
2002 | 0.72774 | 0.91753 | 1.17554 | 1.04130 | 1.05141 |
2003 | 0.94477 | 0.63590 | 1.05951 | 1.22757 | 1.00846 |
2004 | 0.90959 | 0.81988 | 0.81525 | 0.92789 | 1.50283 |
2005 | 0.84676 | 1.08698 | 1.06097 | 0.84782 | 1.08596 |
2006 | 0.53845 | 1.10068 | 1.44544 | 0.81746 | 1.05127 |
2007 | 0.52572 | 0.90846 | 0.98133 | 1.06746 | 1.34932 |
2008 | 1.05741 | 0.73407 | 0.91111 | 0.75120 | 1.29566 |
2009 | 0.36431 | 0.78526 | 1.41975 | 0.83909 | 0.93663 |
2010 | 0.37142 | 0.98320 | 1.13346 | 0.85390 | 1.40367 |
2011 | 0.37932 | 1.70463 | 0.78842 | 0.74030 | 0.93002 |
2012 | 0.40945 | 1.26137 | 0.88000 | 1.05970 | 0.86022 |
2013 | 0.38474 | 0.78193 | 0.84383 | 0.70560 | 1.06413 |
2014 | 0.41497 | 1.74404 | 0.83737 | 0.83428 | 0.86895 |
2015 | 0.37665 | 0.84851 | 0.80085 | 0.70036 | 0.91540 |
2016 | 0.38089 | 0.83494 | 0.85681 | 0.70278 | 0.93780 |
2017 | 0.38641 | 0.77312 | 0.79581 | 0.75970 | 1.02261 |
2018 | 0.38031 | 0.82774 | 0.85973 | 0.75476 | 0.90407 |
2019 | 0.39121 | 0.85144 | 0.84610 | 0.75715 | 0.86965 |
2020 | 0.37976 | 0.85872 | 0.83862 | 0.75612 | 0.88560 |
2021 | 0.38158 | 0.85065 | 0.80734 | 0.74702 | 0.89107 |
Source: Own calculations based on company data of MNCs (Ministry of Justice, 2023) statistical data of regional economic development (Czech Statistical Office, 2023), FDI inflow data (Czech National Bank, 2023), labor productivity data (OECD, 2023). |