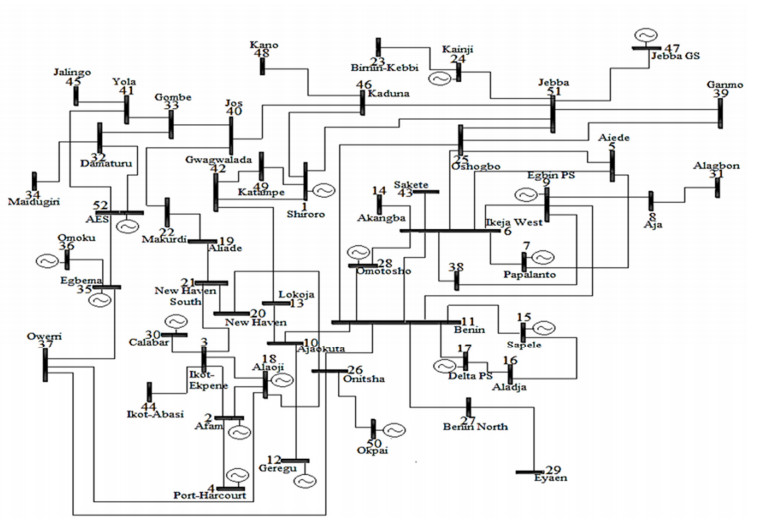
Investment has a crucial impact on the economic development of territories. Traditionally, scholars studied many factors influencing investment (cost of interest rate, labor productivity, GDP growth, financial firm's performance). At present, the existing body of literature demonstrates the shift of factors related to territorial investment attractiveness. Besides economic dimension, the ecological and social matters take key stages (the ESG concept and the triple bottom line concept). Our study is aimed at exploring the relationship between investment at municipal level and ecological factors, considering regional specifics and settlement patterns in Russia. We applied hierarchical (multilevel) modeling with spatial effects to accomplish a twofold goal: to estimate what share of investment variance attributed to municipal and regional scale; to distinguish between spatial error at regional level and influence of average investment in neighboring municipalities at municipal level. Our findings show that 32% total investment variance accounted for regional scale; investment is positively associated with population, production per capita and the ratio of circulating water and sequentially used water to used fresh water. The results of this paper could be useful in developing policy for attracting investment at regional and municipal levels. Given vast national territories, a variety of different regions and heterogeneous settlement patterns, our study lays an initial ground of assessing environmental impact on investment in Russia.
Citation: Dina Krasnoselskaya, Venera Timiryanova. Exploring the impact of ecological dimension on municipal investment: empirical evidence from Russia[J]. National Accounting Review, 2023, 5(3): 227-244. doi: 10.3934/NAR.2023014
[1] | Abshir Ashour, Taib Iskandar Mohamad, Kamaruzzaman Sopian, Norasikin Ahmad Ludin, Khaled Alzahrani, Adnan Ibrahim . Performance optimization of a photovoltaic-diesel hybrid power system for Yanbu, Saudi Arabia. AIMS Energy, 2021, 9(6): 1260-1273. doi: 10.3934/energy.2021058 |
[2] | Mulualem T. Yeshalem, Baseem Khan . Design of an off-grid hybrid PV/wind power system for remote mobile base station: A case study. AIMS Energy, 2017, 5(1): 96-112. doi: 10.3934/energy.2017.1.96 |
[3] | Z. Ismaila, O. A. Falode, C. J. Diji, R. A. Kazeem, O. M. Ikumapayi, M. O. Petinrin, A. A. Awonusi, S. O. Adejuwon, T-C. Jen, S. A. Akinlabi, E. T. Akinlabi . Evaluation of a hybrid solar power system as a potential replacement for urban residential and medical economic activity areas in southern Nigeria. AIMS Energy, 2023, 11(2): 319-336. doi: 10.3934/energy.2023017 |
[4] | Md. Mehadi Hasan Shamim, Sidratul Montaha Silmee, Md. Mamun Sikder . Optimization and cost-benefit analysis of a grid-connected solar photovoltaic system. AIMS Energy, 2022, 10(3): 434-457. doi: 10.3934/energy.2022022 |
[5] | Muti Ur Rehman Tahir, Adil Amin, Ateeq Ahmed Baig, Sajjad Manzoor, Anwar ul Haq, Muhammad Awais Asgha, Wahab Ali Gulzar Khawaja . Design and optimization of grid Integrated hybrid on-site energy generation system for rural area in AJK-Pakistan using HOMER software. AIMS Energy, 2021, 9(6): 1113-1135. doi: 10.3934/energy.2021051 |
[6] | S. Vinoth John Prakash, P.K. Dhal . Cost optimization and optimal sizing of standalone biomass/diesel generator/wind turbine/solar microgrid system. AIMS Energy, 2022, 10(4): 665-694. doi: 10.3934/energy.2022032 |
[7] | Aaron St. Leger . Demand response impacts on off-grid hybrid photovoltaic-diesel generator microgrids. AIMS Energy, 2015, 3(3): 360-376. doi: 10.3934/energy.2015.3.360 |
[8] | Khuthadzo Kgopana, Olawale Popoola . Improved utilization of hybrid energy for low-income houses based on energy consumption pattern. AIMS Energy, 2023, 11(1): 79-109. doi: 10.3934/energy.2023005 |
[9] | Nadwan Majeed Ali, Handri Ammari . Design of a hybrid wind-solar street lighting system to power LED lights on highway poles. AIMS Energy, 2022, 10(2): 177-190. doi: 10.3934/energy.2022010 |
[10] | Abdulrahman Th. Mohammad, Amer Ibrahim Ismael . An equivalent photovoltaic solar system to solve the problems of electricity in Iraqi houses. AIMS Energy, 2019, 7(5): 660-670. doi: 10.3934/energy.2019.5.660 |
Investment has a crucial impact on the economic development of territories. Traditionally, scholars studied many factors influencing investment (cost of interest rate, labor productivity, GDP growth, financial firm's performance). At present, the existing body of literature demonstrates the shift of factors related to territorial investment attractiveness. Besides economic dimension, the ecological and social matters take key stages (the ESG concept and the triple bottom line concept). Our study is aimed at exploring the relationship between investment at municipal level and ecological factors, considering regional specifics and settlement patterns in Russia. We applied hierarchical (multilevel) modeling with spatial effects to accomplish a twofold goal: to estimate what share of investment variance attributed to municipal and regional scale; to distinguish between spatial error at regional level and influence of average investment in neighboring municipalities at municipal level. Our findings show that 32% total investment variance accounted for regional scale; investment is positively associated with population, production per capita and the ratio of circulating water and sequentially used water to used fresh water. The results of this paper could be useful in developing policy for attracting investment at regional and municipal levels. Given vast national territories, a variety of different regions and heterogeneous settlement patterns, our study lays an initial ground of assessing environmental impact on investment in Russia.
Abbreviations: ABC: Artificial Bee Colony; AC: Alternative Current; ACC: Annual Capital Cost; AFC: Annual Fuel Cost of Diesel Generator; AIT: Artificial Intelligence Techniques; ANFIS: Adaptive Neuro Fuzzy Inference System; ANN: Artificial Neural Network; AOM: Annual Operation Maintenance cost; ARC: Annual Replacement Cost; BTS: Base Transceiver Station; CC: Cycle Charging; CD: Combined Dispatch; COE: Cost of Energy; CRF: Capital Recovery Factor; CRITIC: Criteria Importance; CSP/CST: Concentrating Solar-Thermal Power; DC: Direct Current; DF: Duty Factor; DG: Diesel Generator; DSM: Demand-Side Management; ECN: Electricity Corporation of Nigeria; EE: Excess Energy; FC: Fuel Cell; FDI: Foreign direct investment; FEC: Federal Executive Council; FPA: Flower Pollination Algorithm; GA: Genetic Algorithms; GHG: Green House Gases; GIS: Geographic Information System; GSM: Global System for Mobile; GWO: Grey Wolf Optimizer; HES: Hybrid Energy System; h-GAPS: hybrid Genetic Algorithm and Pattern Search; HILR: High Investment with Low Rating; HMS: Hybrid Microgrid System; HOMER: Hybrid Optimization of Multiple Energy Resources; HPS: Hybrid Power System; HRES: Hybrid renewable energy system; HS: Harmony Search; ICC: Initial Capital Cost; IPP: Independent Power Project; IRES: Integrated Renewable Energy System; IRR: Internal Rate of Return; LA: Lead Acid; LCC: Life Cycle Cost; LCE: Low Carbon electrification; LCOE: Levelized cost of energy; LF: Load Following; LIHR: Low Investment with High Rating; LLP: Loss of Load Probability; LOLP: Loss of Load Probability; LSPS: Loss of Power Supply Probability; LUCE: Levelized Unit Cost of Energy; MILP: Mixed Integer Linear Problem; MIMR: Medium Investment with Moderate Rating; MLI: Multi-Level Inverter; MMG: Multi-Microgrid; MOSaDE: Multi-Objective Self-Adaptive Differential Evolution; NDA: Nigeria Dams Authority; NEP: National Energy Policy; NEPA: National Electric Power Authority; NIPP: National Integrated Power Project; NPC: Net Present Cost; NREL: National Renewable Energy Laboratory; O & M: Operating & Maintenance; OCE: Overall Cost of Energy; OPF: Optimization Power Factor; PBP: Payback Period; PEE: Percentage of the Excess Energy; PEM: Proton Exchange Membrane; PFWHS: PV/FC/Wind Hybrid System; PHCN: Power Holding Company of Nigeria; PM: Particulate Matter; PSO: Particle Swarm Optimization; PSR: Power System Reliability; PV: Photovoltaic; RE: Renewable Energy; REMP: Renewable Energy Master Plan; RF: Renewable Friction; RPS: Renewable portfolio standards; RSM: Response Surface Methodology; SCSM: South China Sea, Malaysia; TAC: Total Annual Cost; TCN: Transmission Company of Nigeria; TED: Total Energy Deficit Through Inter-criteria Correlation; TLCC: Total Life Cycle Cost; TNPC: Total Net Present Cost; TNPV: Total Net Present Value; TOPSIS: Technique for Order of Preference by Similarity to Ideal Solution; UCOE: Unit Cost of Energy; WDHS: Wind Diesel Hybrid System; WT: Wind Turbine
Highlights:
● Most research conducted in Africa and Asia is done in remote and isolated places.
● There is more research conducted in Asia than in Africa with the latter having more people without power supply.
● COE is the most common cost component in most studies.
● Diesel fuel is more present than storage devices in HRES for most research.
● Most HRES with diesel fuel fails to conduct CO2 emission impact.
Energy overwhelms every aspect of our lives and is an important element of financial growth [1]. Electrical power is involved in the economic development and improvement of any country. For every country that is serious about industrial development, electricity is a must-have. This is because industrial progress leads to socioeconomic growth, which many countries desire [2,3,4,5]. Many nations have recognized that a contemporary energy system entails a shift to renewable energy sources (RES), which allow for greater economic development while having a lower environmental impact. RESs contribute to energy security by reducing reliance on fossil fuel markets and saving money [6]. It's an important part of economic policy. Because of the diversity of RES, practically every country has the option of relying on conventional energy resources only partially or entirely. As a result, the growth of renewable energy is seen as having several geopolitical benefits.
Developed countries in European Union and the Americas have diversified into different sources of sustainable and renewable energy to suit their energy needs [7,8]. Due to its energy policy, the European Union is without a doubt the undisputed leader in the energy transition process. With the progressive stride in the adoption of Directive 2001/77/EC [9], Directive 2003/30/EC [10], and Directive 2009/28/EC [11], the EU's contribution of RES to savings from fossil fuel imports in 2015 was around EUR 15 billion. It is anticipated that by 2030, it will be worth EUR 58 billion [12]. In the Americas such as the United State, the total capacity of electricity and total annual generation accounted for renewable energy was 20.5% and 17.6% respectively in 2018 [13]. Despite the COVID pandemic, RES was the only energy source that exhibited rising demand in 2020, while all other fuel use decreased. In 2020, annual renewable capacity additions climbed by 45% to approximately 280 GW [14].
According to the 2019 Renewables Energy Global Status Report [15], the number of people living without electricity fell below one billion in 2017 where Asia accounts for 35% whereas Sub-Saharan Africa accounts for 61%. This is due to a variety of causes including dispersion, which is characterized by low electrical energy consumption making grid extension impractical, population density, a lack of financial capability to extend the grid, and other social and cultural features. For instance, Nigeria with inhabitants of more than 150 million, is tasked with the expansion of economic activity and rising energy demands. However, the supply of electricity is still relatively static. The interest for electricity over 40,000 MW is currently much smaller than the 2,900–4,000 MW range accessible [16,17]. It is therefore obvious that the electricity demand is well beyond its availability. The lack of production and the lack of transmission facilities to enhance the supply of power have also been a major cause of the rising discrepancy between demand and supply of energy, which has resulted in overloading and stressing the grid beyond its stability and thermal limits [18,19].
For 15.3 million households, there is presently inaccessibility to grid power; but for anyone linked to the national grid, the supply is erratic, associated with a high-power loss, damping fluctuations, steady and transient system instability-state production, transmission, and distribution states [16]. The consumption of power per unit was far from 150 kW hr per year. 286 kW hr in Ghana, 4,000 kW hr in South Africa, 3347 kW hr in Libya, and 929 kW hr in Algeria [20] can be compared with other nations of the same continent. Remote communities are experiencing the biggest shortfall of power generation, insufficient power supply, and unexpected power failures [21]. In Nigeria, power starvation goes beyond the absence of availability of electric power. Approximately 72% of the citizens of the country solely rely on wood for preparing food as a fuel source. In contrast to the aspirations of the 2003 National Energy Policy, the deterioration of poverty has led to a re-evaluation of the shift to contemporary, effective forms of energy. So many Nigerians are already descending the energy hierarchy from electric power, gas, and kerosene to timber and other ancient forms of bioenergy [22].
The installed output of the generating plants is 7,914.4 MW. Seven out of the fourteen generating stations were more than 20 years old and the mean daily output of power is well below the generation capacity. Of the Seventeen operational electricity generation plants, 8 (functioning) with a generation capacity of 6,256 Mw are primarily managed by the Federal Government, but 2,484 MW are accessible. The leftover nine are from the NIPP and the IPP with a total installed capacity of 2,809 MW, of which 1,336.5 MW are accessible. The peak load ever attained was just barely beyond 4000 MW for a population of about 160 million. In reality, meeting market demand for power is significantly inadequate [23,24]. Many generating stations are old and require a complete upgrade. For factors of corruption, politics, totally inadequate financing, and weak management, total electricity production amounted to about 2,800 MW of daily purchase around December 2011. Due to insufficient resources required to achieve the necessary maintenance standard, most power plants have degraded. Accordingly, because of the low energy capacity of the few generating units currently in operation, the power grid has been defined as a persistent system failure [25]. Consequently, the country is witnessing significant load shedding.
Suppliers of electricity generators always seem to infiltrate the Nigerian market with inferior generators breaking down before real-life time. The generator companies in the country are among the few companies with major foreign exchange discharges associated with generator supplies, and the high cost of running these generators has had substantial negative social, cultural, and ecological consequences on the citizens of the country. Groups of businesses spend a large proportion of their setup costs on the purchase of generators, whereas maintenance of generators is an essential operating expense. In 2005, Nigeria officially was Africa's biggest generator importer, spending 152 million on generator purchases [20]. The present state of electricity production in Nigeria poses the following problems through the expected generation capacity projects for a brighter future (ⅰ) The insufficient availability for production (ⅱ) Insufficient and overdue maintenance of the equipment. (ⅲ). Inadequate financing of power stations. (ⅳ) Outdated services, instruments, protective facilities, and operating vehicles (ⅴ) Poor and outdated communication facilities (ⅵ) Total absence of discovery to tap all energy sources from the resources available, and (ⅶ) Poor morale of workers [26].
In Nigeria, the very first electricity interconnection was a 132 kV link installed between Lagos and Ibadan in 1962. The very first national power grid structure emanated in 1968 with the completion of the Kainji hydro station that delivered power to the 3132 kV sub-systems then operating in the eastern, northern, and western regions of Nigeria via a 330 kV, primary radial type transmission network. Two independent bodies—the NDA and the ECN respectively initially controlled the 330 kV and 132 kV systems. From the Kainji energy supply control room, the central control for the 330 kV network was controlled. Whereas, the 132 kV network was managed by the Lagos power supply load dispatcher situated at Ijora. In 1972, these two entities were officially combined into a single power utility known as NEPA, thus initiating centralized regulation and coordination of the entire rapidly growing national 330 kV and 132 kV network. The radial transmission grid (330 kV and 132 kV) is currently operated by the TCN, which is responsible for the maintenance of the system and the market settlement roles [27]. Such networks are marked by multiple disruptions, creating numerous challenges and outages [24,28]. The new transmission system in the country includes 330 kV 5523.8 km, 132 kV 6801.49 km, 32 No 330/132 kV substations with a total transformation power of 7688 MVA built 105 No. 132/33/11 kV substations with 9130 MVA gross installed capacity for transformation. The average power available at 330/132 kV is 7364 MVA and at 132/33 kV is 8448 MVA [29,30]. Because of the long transmission lines, the Nigerian 330 kV transmission grid is attributed to its high-power losses. Power losses result in a lower supply of power to customers, resulting in insufficient power to run their appliances.
Steadily increasing electricity demand forces the power transmission network to and beyond its maximum limit, leading to a shortened network life or complete failure of the network [31,32]. Before the unbundling of the current power network in the country, it consisted of 11,000 km (330 kV) transmission lines, faced with so many challenges such as; Failure to efficiently dispatch produced electricity to meet load demand, large numbers of unfinished transmission line projects, energy sector reinforcement, and expansion projects, weak voltage profile in most northern sections of the grid, failure to drive more than 4000 MW of power to exist power lines at present, operational issues, voltage frequency control [33,34,35]. The country's Power Grid transmission system does not reach every corner of the country. It presently has a maximum transmission capacity of approximately 4,000 MW and is technically delicate and radial in nature, hence very susceptible to major disruptions. This indicates that the transmission grid would not have the ability to manage the generated power efficiently if the generation system is to operate at maximum output [36,37,38].
To sum up, the key concerns listed are: (ⅰ) It is sponsored solely by the Federal Government, the allocation of resources of which is unable to satisfy all the demands adequately; (ⅱ) Many portions of the country have yet to be covered; (ⅲ) Its maximum available wheeling power for electricity is 4,000 MW, which is well below the national requirements needed; (ⅳ) Certain parts of the grid, as opposed to the necessary national needs, are obsolete with insufficient redundancies; (ⅴ) The Federal Govt needs the requisite funds to extend, upgrade, revitalize and maintain the infrastructure regularly; (ⅵ) Lines are routinely vandalized, with poor levels of monitoring and protection related to all power infrastructure; (ⅶ) Generally, the technology solutions used have very weak voltage stability and statuses; (ⅷ) Poor work tools and vehicles for running and sustaining the network have a high prevalence; (ⅸ) There is a significant absence of digital communication and monitoring technology solutions required; (ⅹ) In several service areas, the transformers installed are overloaded; (xi). Not enough spare parts for emergency repairs; and (xii). Bad recruiting of technical personnel, capacity building, and program preparation [25,39].
To fix these issues, PHCN was led to its privatization. Thus, the embedded Nigerian 330 kV network aims to boost the reliability of the grid and establish an efficient interconnection. Due to the extremely high demand for the current and ageing infrastructure, by installing more transmission lines and power stations via the IPPs, transmission strength is expected to increase. With the security system on the mind of the players, the restructured Nigeria electricity grid was launched, and the post-reformed grid presented solutions to the shortcomings involved with the pre-reform grid network [40]. The current grid lacks the technological adequacy to handle enormous electricity injection and meet potential system output requirements [41]. The grid links these stations with 64 dual or single-circuit transmission lines and 52 buses and has 4 control centers (1 national control centre at Osogbo and 3 supplementary control centres at Egbin, Benin, and Shiroro).
Ten (10) generating facilities, 32 transmission lines, and 23 load stations are comprised of the Nigerian 28-bus 330 kV transmission grid. Single Line System Diagram, Figure 1, demonstrates that the system is split into three main regions: the southeastern, southwestern, and northern regions. A triple circuit between Osogbo and Jebba links the South to the North, while the East is connected to the West via a single transmission line from Benin to Osogbo and the Ikeja to Benin double-circuit section [42,43,44]. It only has one big loop system involving Benin-Ikeja West-Ayede-Osogbo. The shortage of loops mainly contributes to the country's inadequate and slow power grid [35].
To draw investors to electricity and Nigerian energy industries, the NEP was introduced. This was first drafted by the ECN, approved in 2003 by the FEC, and subsequently unveiled by the Presidency in 2005 [45]. Its main objective was to guarantee the effective utilization of the energy resources of the country including tar, petroleum, sand/bitumen, coal, nuclear, and renewables (hydrogen, wind, solar, biomass, hydro), with the private sector's active involvement for sustainable national growth and development. The revised NEP maintains that the country shall seek, in an environmentally friendly manner, the effective utilization of all its existing fuel types and energy resources for various commercial, transport, domestic, agricultural, and industrial enterprises. This implies that to satisfy the energy demand of its teeming population, the nation must actively target the eventual utilization of all its existing energy resources, electricity, and other energy forms. In particular, the policy on solar energy in the NEP states: "The nation shall aggressively pursue the integration of solar energy into the nation's energy mix, which should be based on the established potentials and available technologies nationwide." Because of this, REMP is likely to raise the production of renewable power from thirteen percent of total power generation in 2015, to twenty-three percent in 2025 and thirty-six percent in 2030 [45,46].
In renewable electricity, only hydropower plays a significant role, while biomass and solar have yet to make a significant input to the energy supply mix of the nation, not even as of the close of 2017. There is no proof that, with solar thermal electricity making any significant contribution to the 2025 prediction, the REMP policy can be accomplished. To meet the objectives, in the NEPs, short-term, medium-term, and long-term development strategies were included. An illustration of this is the creation of a national database on energy use trends in various industries and the encouragement of the employment of energy-efficient devices in all energy usage technologies as the main short-term project initiatives in the policy document. In addition, the expansion of total transmission lines from under 14,000 km to about 39,000 km and the expansion of power production from 10 MW to approximately 350 GW and by 2043 is expected to require an estimated US$550 billion [46]. In addition to other energy sectors including coal and tar sand, oil, and gas, the discovery of renewable energy resources requires enormous amounts of financial resources for their full development and use, thereby encouraging international and local investment with private sector involvement. Globally, national grid electricity production is typically a combination of various sources, mixing non-renewable and renewable sources [47,48,49,50,51] not just for higher generation, but for a cleaner environment and lower electricity costs. Kenya has begun a 310 MW wind energy program in sub-Saharan Africa projected to be completed in mid-2017 [52]. Geothermal technologies produce electricity in Ethiopia and Kenya. Although pumped storage, hydro, solar, and wind form elements of the energy mix of South Africa, with a solar thermal plant (100 MW) commissioned in 2015, and 4 additional plants adding 300 MW to the grid finished later, the combustion of coal still plays a significant role. Although the population of Nigeria is high and there is an increasing electricity demand, renewable sources of clean energy such as biomass, geothermal, photovoltaic, wind, and solar thermal have not begun to play a role as hydropower in the national electricity mix. In this list, only PV technology has found inadequate application in the generation of private electricity, and few community-based pilots and PV street lighting projects (government-funded) in the nation, which are also afflicted by weak and inefficient installations undertaken by incompetent contractors.
Given the growing interest in the use of RE in the generation of electricity in Nigeria, through research and development, there seems to be optimism in the electricity market. A thorough analysis of RE's current and future prospects in Nigeria was conducted by [53], highlighting the tremendous capacity of capital across the country. In the nation's energy mix. Some policy concerns in Nigeria's sustainable energy growth were discussed by [45]. They proposed increasing the attractiveness of FDI in the development of clean energy technologies and RPS as policies that could contribute to that. Although, there are rising interests in research and development in RE, increased pressure to variegate power generation, and increased government devotion to expanding PV power generation capacity, a few obstacles remain to be overcome in Nigeria's realization of solar thermal electricity generation. Solar thermal-based electricity will continue to be a pipedream until these are addressed.
Renewable energy (RE) sources are sources of energy that, by natural phenomena, are recycled periodically and are thus inexhaustible. Examples of renewable sources of energy include wave energy, biomass, wind, insolation, and hydro [54,55]. These RE sources are green and clean and have a low impact on the environment. Renewable resources, unlike fossil fuels, are roughly evenly spread across the world and are available free of cost. The world is still heavily dependent on fossil fuels for energy. Only a small portion of the globe's primary energy supply, approximately 12%, comes from renewable sources of energy. The decline of fossil fuel reserves and the rising energy demand have led to growth in the use of renewable sources of energy to meet energy needs. Increased demand for power, along with concerns regarding the appropriateness of other technologies and climate change, has increased interest in RE technologies. The effective use of a variety of sources of renewable energy will pave the way for sustainable growth [56]. For the sustainable growth of a social structure, careful planning and design for the use of renewable energy sources is therefore needed. RE sources are capable of fixing the global challenges of sustainable development, global warming, and energy security [57]. The development of renewable energy technologies, therefore, needs to be accelerated. To harness currently available renewable sources of energy, various technologies and techniques can be utilized. Two methods are widely used and discussed below: hybrid and integrated.
HRES merges multiple energy resources and transforms them into a single form of energy, usually electricity (AC or DC) for aggregation and distribution. Wind/biomass/fuel cell system, PV/wind/fuel cell hybrid system, diesel generator/wind system, PV/wind electric system, etc. are a few examples of HRES. As a backup generator, many hybrid systems use diesel generators. Hybrid power systems offer customers solutions and value which cannot be matched by individual technologies. The high capital costs and low end-user productivity of HRES make it inappropriate for rural applications. Figure 2 shows a typical hybrid system made up of a diesel generator, hydro turbine, and wind turbines with a battery backup system.
IRES is founded on the premise that various kinds of energy requirements need different types of energy and different energy qualities. The system uses end-use technologies and RE resources accessible locally to meet a range of demands. Some of these demands include irrigation water, low-grade thermal energy, potable and domestic water supply, cooking, cold storage, educational and communication devices, domestic and community lighting, and small-scale industries [58]. To maximize gains and end-use efficiencies, this strategy involves careful and systematic preparation for matching needs and available resources. IRES can potentially generate aggregate benefits from a synthesis of REs, energy efficiency, and conservation of energy [59]. This investigation uses the concept of IRES-MMG to assess the frugality of the integration of fossil-fuel and RE technologies in the Nigerian power sector using community microgrids and grid-connected facilities and the customer-owned business model, along with diverse case studies to verify the developed and selected, economic and technical business models.
Though the majority of studies on renewable energy systems have concentrated on HRES, there is a need for an article to comprehensively review these works, which explore the optimal planning of HRESs, which is the key aim of this paper. The review will be valuable in providing an insight into the study focus on the implementation and strategy of RESs in many continents, particularly developing continents such as Africa and Asia, which will be useful for researchers planning to employ these strategies in their regions. Does the paper give the necessary information concerning HRES planning, such as which hybrid systems were investigated for the RESs? What are the most important economic parameters in the planning? What methodologies are taken into account in the articles? Important remarks from each of the studies were also noted.
This section examines the current research on renewable energy (RE) in five continents, taking into account their methodologies, hybrid systems, economic parameters, location, and country. The viable renewable energy sources are determined by the geographical locations. Those living near the coast will have access to tidal or wave energy, while those living near the equator or in the Northern Hemisphere will benefit from intense solar radiation. High wind speeds and/or sun radiation are common in arid areas.
The implementation of one of the new GWO swarm intelligence algorithms influenced by gray wolves was introduced by Yahiaoui et al. [60]. The GWO algorithm mimics the management mechanism and the method in nature for murdering grey wolves. For optimum configuration, the desired strategy is applied and the overall cost of the fusion power generation system in the remote rural village called 'Djanet' in southern Algeria is reduced. A power system composed of a photovoltaic array panel, diesel generator, battery banks, and load is believed to be the possible answer. Table 1 shows the comparison to the PSO algorithm, the obtained results by this novel strategy show that the proposed technique can easily illustrate the optimum number of PV panels, diesel generators, and battery banks with rapid convergence, lower costs, and superior capacities of this suggested model.
Components | Grey wolf optimizer | Particle swarm optimization |
PV panels | 33 | 38 |
Battery banks | 90 | 90 |
Diesel generators | 2 | 3 |
Annual capital cost ($) | 10519.38 | 10214.95 |
Annual operation and management cost ($) | 170.46 | 160.81 |
Annual replacement cost ($) | 5638.5 | 6049 |
Annual cost system ($) | 16825.64 | 16872.66 |
Annual fuel cost ($) | 497.3 | 447.9 |
Muh and Tabet [61] used climate Wum data to assess the feasibility of offshore applications in Southern Cameroon of combined renewable energy systems. HOMER was used for the comparative analysis. 9 hybrid systems using the following elements were regarded in this research work: Inverters, charge controllers, wind turbines, micro-hydro turbines, diesel generators, batteries, and Photovoltaic modules. 2 energy sources and a battery bank were used to describe each device. For Southern Cameroon, the Photovoltaic/diesel/small hydro/battery with an energy cost of 0.443 dollars-per-kW-hr was identified to be the most viable economic process. The optimal solution is very resilient against fluctuations in streamflow, rate of interest, fuel price, and PV cost. For Cameroon's policy-makers and shareholders, this outcome proved to be extremely important.
A technique for maximizing and evaluating the responsiveness of an autonomous PV/diesel/battery hybrid energy system was proposed by Fodhil et al. [62]. To reduce overall device costs, unmet load, and Carbon dioxide emissions, PSO and the electron-constraint approach were used simultaneously. 1 optimal solution was chosen from among the proposals that were set and evaluated. The small Saharan village of Tiberkatine, in southern Algeria's Tamanrasset province, was used as a case study. The hybrid system has provided energy requirements for twenty households. The best solution was found to be able to supply energy without even an unmet load, with a minimum COE of 93 percent renewable fraction being 0.37 dollars-per-kW-hr. In an optimized system, the effect on system compatibility with 3 parameters was evaluated with a sensitivity analysis. A comparative analysis between PSO and HOMER was then performed, showing that the PSO-based method with more PV penetration than HOMER is much more cost-effective.
Diemuodeke et al. [63], which have taken into consideration energy storing and diesel-generator support in Warri, Yenagoa, Calabar, Port Harcourt, Uyo, and Benin, have optimized household mixed energy systems mapping in 6 sites in South-South Nigeria for the wind and PV market. Optima hybrid power systems can meet the household's electric demand at 7.23 kW-hr-per-day. The mixed energy system for each of the areas was efficiently implemented based on the HOMER technology computation and TOPSIS multi-criteria decision-making mechanism, which considers technological, economic, environmental, and socio-cultural criteria. For the 6 sites, the Weibull distribution function was also used to perform wind energy potential; the wind speed at an anemological height of 10 meters varies from 3.21 to 4.19 meters-per-seconds. The wind velocities and wind dynamics were extrapolated to the 30 metres and 50 meters hub heights. The solar resource capacity, ranging from 4.21 to 4.71 kiloWatts-hr-per-m2-per-day, is also provided throughout the 6 locations. For places in Yenagoa, Benin-city, and Port Harcourt, the diesel generator-PV/Wind/Battery model is the perfect hybrid system, whereas the PV/Wind/Battery model is the perfect hybrid system for places in Uyo, Calabar, and Warri. Benin-city, Yenagoa, and Port Harcourt's mixed systems emit carbon dioxide, just 8.47 percent, 15.02 percent, and 14.09 percent of the enterprise as usual (the diesel generator). The payback period is between 3.7 and 5.4 years, with standard COE earned for the company in the amount of 0.893 US dollars/kW-hr. The COE of hybrid systems varies between 0.459 and 0.562 US dollars/kW-hr, which is good compared to the public domain results.
Djelailia et al. [64] have used an environmental issue in Algeria by a hydroelectric pump storage network. This form of hybridisation must be managed explicitly to handle electricity. The suggested energy management takes into consideration the accessibility of temporary solar energy and relates it to the enhancement of the use and pumping limitations of online diesel fuel. The proposal illustrates the effectiveness of the double-effect hydroelectric pump storage system in irrigation and electrical power restoration, as well as fuel savings and Carbon dioxide emissions reductions.
For the capacity sizes of the different independent components of photovoltaic/wind-diesel/battery systems to be optimised for zero load energy deficits, Kaabeche and Ibtiouen [65] concentrated on the improvement of an efficient model for sizing. The model proposed takes into consideration the hybrid system submodels, the TED, the Overall NPC, and the COE. The optimal size design flow diagram for the combination is also shown. All setups that offer 0 percent of the TED rate are preserved using the defined model. The optimal setup is then calculated based on the minimum cost. The advanced system is compared to other energy sources using the site of Ghardaïa, Algeria, with solar radiation, atmospheric temperature, and wind speed data. The optimisation findings demonstrate that a photovoltaic/wind/diesel/battery choice is cheaper than a PV/wind/battery/power or diesel generator
Babatunde et al. [66], which supports a traditional rural health center throughout the 6 regions in Nigeria, propose an efficient hybrid renewable off-grid energy system. To evaluate the best blend of PV, wind, diesel generator, and battery-based off-grid hybrid energy network, a technological and economic assessment was conducted. As a result of government fuel subsidies, the cost of fuel pumps is decreased in many developing nations and does not reflect what can be bought on a deregulated market. To capture the fact, the study took into account the effect of the abolition of subsidies by analyzing the fuel pump price sensitivity. Therefore, the effect on optimum setup economic performance parameters from a shift in the diesel fuel pump price and interest rates is investigated. The findings demonstrate, with the net current and renewable cost of 12,779 USD to 13,646 USD, and 70–80%, that the PV/diesel/battery model is the most economically viable of all places. The COE varies between 0.507 and €0.542 per kW-hr as well.
A techno-economic feasibility study was performed by Samy et al. [67] for off-grid integrated solar PV/FC systems. A combined green energy system is under investigation to provide electricity to rural areas and remote urban areas in Egypt. All computer equipment is modeled and simulated and data on the region under study is collected to achieve this objective. With the TAC in mind, the goal function is formulated. The FPA has recommended estimating the optimal values of both PV panels and FC/electrolyzer/H2 storage tanks that are needed where the minimum present value is achieved as an efficient, recent metaheuristic optimization process [68]. It is perceived that the possibility of a loss of power supply increases the performance of the proposed design. The impact on the LCOE of the variation of FC, electrolyzer, H2 storage tanks, and the upfront cost of the PV power system will be presented in a comprehensive sensitivity analysis. The mathematical simulation results obtained by the FPA method were compared to the corresponding results through MATLAB through the use of the ABC) and PSO techniques. Based on the simulation outcomes review, the FPA algorithm has less full time and is a good representation of the other algorithms. Additionally, FPA with optimum hybridity of 27 PVs, 28 FCs, 58 electrolyzers, and 37 H2 storage tanks for 1.52 percent, 4.68 percent for the LPSP, and the PEE, guarantees the maximum system configuration. The system's overall current value is 3.244.897 dollars and the LCOE's € 0.334/kW-hr.
A technology-economic study of the Nigerian combined energy framework for the base transceiver plants was submitted by Ogunjuyigbe and Ayodele [69]. The GSM operator provides customers inside and away from urban centers with telecommunications networks of 2G, 3G, and 3.75G. The combined energy system is computed for total NPC over a selected 25-year life cycle of the project using a Homer simulation tool. The results of the simulation show that the best cost-benefit feature is the 5 economically viable Photovoltaic/Diesel/Battery hybrid energy systems. The machine is composed of 50 kW of PV, 10 kW of diesel, 300 Trojan LI6P, and 10 kW transducers. A 96 percent clean energy fraction of 401,000 USD NPC and 1.28 USD per-kW-hr electricity costs reflect the alternative. The outcome also shows savings of 60,595 kg per year of carbon dioxide and 149.77 kg per year of carbon monoxide compared to the non-renewable generation alternative (diesel only).
As an alternative to those threats, Tijani et al. [70] focused on the evaluation of the technology-economic viability of a mixed photovoltaic off-grid diesel/battery system (see Figure 3). For this reason, a case study considering an international institution with a peak demand of 90 kW was carried out in a remote location located far north of Nigeria. To assess the technical and economic feasibility of the planned energy infrastructure, the HOMER optimization tool is used to take account of variations in solar radiation and fuel prices, as found in most of Nigeria. The research demonstrates the possible capacity of the battery backup photovoltaic/diesel energy system as an alternative energy source for a single household replacement generator. However, there is high energy reliability and low carbon emissions in the planned network at reasonable energy prices.
Samy [68] intended to illustrate the viability, as contrasted to batteries, of implementing the RE-hydrogen concept for rural electrification in the remote communities of Egypt. Following the launch of a detailed literature review showing the benefits and drawbacks of stand-alone RE systems, which often need energy storage system assistance. It is regarded as the optimal economic model of the HPS that supplies the electrical load required of the tiny community of Mansheat Taher at Beni-Suef Governorate, Egypt. For this reason, 5 particular HPS setups are being tested, such as Photovoltaic/wind/battery, Photovoltaic/FC, wind/FC, Photovoltaic/wind/FC, and Photovoltaic/wind/battery/FC systems. The designs of various systems are optimally designed with HOMER technology, sized based on daily data for energy supply and demand. The HPS approach provides a cumulative net present cost of 1,233,317 USD from the simulation outcome viability analysis, which is regarded as the most economical and feasible solution feasible. The cost of electricity is 0.1424 USD-per-kW-hr, with an initial 916.728 USD capital allowance. For economic viability, a case study site, Monshaet Taher Village in the Governorate of Beni-Suef, Egypt (29° 1 '17.0718'N, 30° 52' 17.04'E), is identified in this study. With an annual average solar radiation measured at 5.93 (kW-hr-per-m2-per-day), the HOMER optimization model plan was designed with an average annual wind speed of 4.92 metres per second for the region.
Research by Salisu et al. [71] evaluated the feasibility analysis of the use of a composite solar-wind-battery-diesel system to provide power to a remote high school. To carry the same analysis to other regions of the world, a village (Moriki) in north-western Nigeria was adopted for this research. To have an optimal configuration in terms of NPC and COE, a HOMER simulation model is used to perform the feasibility study. Table 2 shows the various performance evaluation of the system configuration, the ideal design simulated by HOMER with NPC of 18,161USD and COE of 0.233 USD per kW hr for a nominal 6 percent sensitivity case discount rate is the composite solar photovoltaic battery system. Sensitivity analysis was carried out where all the sensitive variables for examining their effect on the NPC and COE were deemed to be wind speed, solar radiation, and nominal discount rate. The finding showed that the sensitivity parameters affect the NPC and COE. The findings also showed a tremendous reduction of greenhouse gas emissions by 100 percent, as the optimum setup is 100 percent renewable.
Configurations | PV-Battery | PV-Diesel-Battery | PV-Wind-Battery | PV-Wind-Diesel-Battery |
PV (kW) | 4 | 4 | 4 | 4 |
Wind turbine (kW) | - | - | 1 | 1 |
Gen-set (kW) | - | 10 | - | 10 |
Battery | 16 | 16 | 16 | 16 |
Converter (kW) | 5 | 5 | 5 | 5 |
NPC ($) | 18,161 | 21,671 | 26,824 | 29,225 |
COE ($) | 0.233 | 0.278 | 0.344 | 0.375 |
Initial cost ($) | 15,580 | 20,580 | 22,080 | 22,080 |
Operating cost | 163.87 | 69.23 | 301.15 | 517.04 |
The goal of Rao et al. [72] was to achieve an appropriately suitable configuration of the combined power generation system using different renewable energy sources to meet the requirements of the community load in a reliable, economical, endless, and sustainable way. Solar, wind, and conventional electric generators are part of the composite network. The study was conducted at Deleta village in the district of Meta Robi, Oromia region, Ethiopia in the year 2017–2018 for combined electrification. The required solar potential values and wind velocity statistics were taken by NASA. The data show that the project site has an average 10 metres anemometer wind speed of 2.9 meters-per-seconds and solar radiation of 5.81 kW-hr-per-m2-day. The expenses of the associated hybrid components were derived from different springs and the electrical load data were determined for the urban and public service requirements. HOMER software was considered using the renewable mixed off-grid framework to perform technology-economic research to meet the load requirement. Due to resource load, the hybrid scheme and the cost input data for the part were considered. For enhancement, sensitivity, and grid comparison, HOMER software offers performance. With a minimum percentage of around 195,974 kg per year of carbon dioxide and other GHG emissions, the device is distinct from an environmental point of view.
To define HRESs for the rural population, Babatunde and Ighravwe [73] used technology, economic and technology-economy benchmarks. The selection method involves requirements significance through similarity between CRITIC and TOPSIS as a framework for a solution. The use of 6 HRESs was evaluated based on economic and technical parameters. 10 technical and 9 economic parameters for HRESs with HOMER were simulated in the analysis. The findings of the HOMER program show that a renewable proportion of 1 was given by A5 (Photovoltaic/wind/battery) and A6 (Photovoltaic/battery). Findings from the CRITIC method showed that perhaps the diesel generator and the total fuel cost were the most significant technical and economic metrics, respectively. The perfect HRES was considered for the case studies in the economic sense A4 (diesel/battery), while in the technical and technology-economic sense, A3 (wind/diesel generator/batteries) was the better HRES.
Sanni et al. [74] discussed the optimal construction of a Photovoltaic/diesel hybrid solar power system in Kadawa village, the Nigerian state of Kano to promote irrigation agriculture and post-harvest cold storage. The program HOMER is used to perform the suggested hybrid power system technology-economic research. The simulated results have shown that the model suggested can be supported by a 25 kW Photovoltaic system and 10 kW diesel generators. The COE and NPC, pertaining to a sensitivity analysis, were some of the main reasons for the result. This optimal system was subject to sensitivities parameters such as solar irradiance and diesel fuel price effect (see Tables 3 and 4). The findings show that incorporating renewable energy into farming would go a long way toward ensuring food security.
Global solar (kWh/m2/d) | Total NPC ($) | Operating cost ($/yr.) | COE ($/kWh) | Ren fraction |
4.76 (–20%) | 83,915 | 1,263 | 0.355 | 0.84 |
5.95 | 74,836 | 2,584 | 0.316 | 0.96 |
7.14 (+20%) | 71,443 | 769 | 0.302 | 1 |
Diesel price ($/L) | Total NPC ($) | Operating cost ($/yr.) | COE $/kWh | Ren fraction |
0.52 (–20%) | 74,196 | 1,263 | 0.314 | 0.96 |
0.65 | 74,836 | 1,170 | 0.316 | 0.96 |
0.78 (+20%) | 75,477 | 1,356 | 0.319 | 0.96 |
The findings of the technology-economic combined system analysis comprising solar and wind energy for driving a particular remote mobile BTS in Nigeria were written by Olatomiwa et al. [75]. Any modeling, simulation, and technology-economic assessment needed are performed using the HOMER evaluation tools kit. 2 optimum system settings, namely Photovoltaic-diesel-battery and Photovoltaic-wind-diesel-battery systems, are compared with the conventional stand-alone diesel generator model. Observations have shown that the most financially feasible option is the Photovoltaic array (10 kW)-DG (5.5 kW)-battery (64 Trojan L16P units) with an overall present net cost of 69,811 USD and an energy cost of 0.409 USD-per-device. To determine the impact of potential fluctuations in solar radiation, wind speed, and fuel prices in ideal system settings, sensitivity analysis is also carried out. Ultimately, the environmental advantages of combined systems over traditional, stand-alone diesel systems have been identified. The results obtained demonstrate that the combined Photovoltaic-diesel-battery system provides a reduction in Carbon dioxide emissions of around 16.4 tons per year relative to the stand-alone diesel generator design.
Okundamiya et al. [76] investigated the feasibility of grid-connected HES for household electricity output in the developing world. It was aimed at evaluating the technology-economic advantages of operating a wind power conversion system. The HES includes management of the grid, conversion of wind power, power equipment, and storage units. A statistical approach based on opportunity is used by the grid distribution device. Using the wind turbine power model provided by the manufacturer, the simulation of the wind energy structure is predicated on the third-order polynomial piecewise. To solve the developed optimisation problem, an h-GAPS approach was used. To ensure efficient service, the h-GAPS-based method restricts power generation and distribution. Weather information for 6 areas, spread throughout Nigeria, was used for a typical residential region. The 10 kW/5.40 kW-hr wind/battery capacity system installed in Sokoto, in specific, can handle 95.4 percent of overall electricity demand, save more than 77 percent of electricity bills, and improve reliability by about 140 percent.
The technology-economic analysis of a combined power system with wind-diesel was given by Allali et al. [77]. In this scope, the impact envisaged in this study is to work together on the optimal design of a combined power system in South Algeria, along with a wind turbine generator, a diesel generator, and an energy storage system for the constant power supply of a remote site with a capacity of 120 kW. To handle different power sources (wind, diesel, and battery), this device has a high control method that relies on climatic conditions, especially wind speed values and the power required by the user's load.
To minimize their expenses and dependency on diesel fuel for energy production, Weis and Ilinca [78] have researched wind power systems for rural populations in Canada. Considering the high capital requirements, low-penetration wind-diesel hybrid systems (WDHS) be not financially practical. High-penetration WDHS benefit from improved economies of scale and large amounts of diesel fuel displacement but still have the drawback that when the wind turbines operate at a rated capacity, they cannot capture all the electricity generated. Two representative simulations of Canadian remote communities were built by HOMER, an NREL micro-power simulator, to simulate how a generic system for energy storage could contribute to the improvement of the economy of a high penetration WDHS. The overall performance of storage systems installed storage costs, diesel fuel prices, and average annual wind speed are the main variables influencing the optimum system. In isolated Canadian communities, wind generators are only effective whenever there is an annual average wind speed of at least 6.0 m/s, with an avoided cost of $0.30 Cdn/kWh of diesel fuel and existing installed costs. When annual average wind speeds surpass 7.0 m/s, where the installed storage system costs below $1000 Cdn/kW and can deliver an overall energy conversion capacity of at least 75 percent, wind energy storage systems are worth considering. The system for energy storage would require the supply of an additional 50 percent of the electricity from wind turbines for these situations.
Osaretin et al. [79] presented a new study that sought to optimally design a renewable energy system for operating an artificially elevated oil well. This presents a low-cost option for the closure and decommissioning of obsolete wells. This presents a low-cost solution to the closing and decommissioning of obsolete wells. To estimate the good energy needs, Sucker-rod-artificial-lift-simulators (QRodTM and PROSPERTM) are used, taking into consideration both intermittent and persistent pumping. Utilizing the HOMER optimizer, which produces various system configurations and implements possible configurations to maximize system costs, simulation and enhancement are carried out. On the grounds of economic and technical requirements, constant pumping using a combined renewable energy structure comprising solar, wind, and battery storage is selected as the most viable solution with 0 kW-hr-per-year of unmet demand, gross storage of 0.56 kW-hr-per-year, the net present cost of 145.150.5, leveled energy cost of 0.51-per-kW-hr and operating cost of 3.056.04 USD-per-year. Finally, to assess its reaction to the daily solar radiation fluctuations and mean wind speeds, the optimal configuration is checked. Also with the smallest average solar radiation of the day, it is shown to be the most required system configuration.
In research by Ciez and Whitacre [80], a device-level lifetime cost-of-use optimization framework was employed in a conceptual combined off-grid power system to contrast the effects of different battery technology. The authors analysed three distinct batteries and used a time-step model of battery degradation to account for device deterioration over a 20-year system lifetime. Evaluated parameters include: battery scale, allowed charge swing status during cycling, amount of battery replacements, criteria for fractional renewable energy, and discount rate implemented. Current research suggests that high-capacity; low-cost lithium-ion cell storage packs have the potential to compete in some circumstances with a non-renewable approach. The discount rate also shows to be crucial in determining the cost-effectiveness of hybrid systems: the LCOE is only slightly higher than the diesel supply at low discount rates, with prices veering away as the discount rate increases. The discount rate also defines the least energy costs provided by battery tech: lead-acid batteries are desirable at low prices; however high-energy lithium-ion batteries generate electricity at higher rates at cheaper prices. Likewise, the market conditions required to translate a hybrid scheme, including fuel and battery costs, feed-in tariffs, or carbohydrates, vary markedly from the discount rate.
For an isolated region called the "KLIA Sepang Station" in Selangor, Malaysia, Shezan et al. [81] assessed the performance of an off-grid PV-diesel-wind-battery HRES. Considering a 3.9 kW peak load and a 33 kWh/day average load demand, the system was both simulated and designed to serve a small population. To optimize and simulate device operations, the HOMER software used real-time field data of the wind speed and solar radiation of the region. The simulation guarantees that the method is suitable in terms of NPC intent and CO2 emission reduction. Results revealed that CO2 emissions and NPC can be lowered by around 29.6 percent and 16 tons annually respectively, compared to typical power plants. As shown in Table 5, the configured system's NPC was found at about USD 288,194.00, with the COE at about USD 1,877/kWh per device. The hybrid system studied could likely apply to other regions globally where weather conditions are comparable.
Optimization methods | Solar-Wind hybrid energy system | Conventional power station |
NPC/year ($) | 198,347.984 | 198,348.00 |
COE/year (kt) | 288,194.00 | 297,000.00 |
A new framework for ideal sizing and positioning of off-grid PV-diesel hybrid systems in rural communities has been deployed by Cai et al. [82]. In this context, a GIS module was employed to discover the best location based on environmental, social, reliability, economic and technological benchmarks. A hybrid optimization algorithm was used to determine the correct capacity through the cost reduction of the total life cycle to continuously meet the load. In South Khorasan, an actual case study is being contemplated to show the proposed structure and its efficacy. By comparing the findings with the results acquired from other heuristic approaches, the efficacy of the applied strategy is evaluated. The effects of variations in fuel prices, initial battery and PV costs, and interest rates on the hybrid scheme's economics are equally analyzed. Simulation outcomes demonstrated that the proposed hybrid algorithm generates more accurate (by 14.1%) results than other implemented algorithms and revealed that using a diesel generator with solar power yields a substantial reduction (by 59.6 percent) in greenhouse emissions and supply costs compared to the diesel-only approach (by 22.2 percent). Accordingly, the findings demonstrated the benefits of using the hybrid system framework proposed.
The techno-economic parameters of a hybrid battery/wind/PV/diesel system for the generation of power for a major user of non-residential power in southern Iran were analyzed by Baneshi and Hadianfard [83]. The practicability of running a hybrid system to meet the daily average load demand of 9911 kWh and the peak load demand of 725 kW in a non-residential city is a case study. Based on comparative environmental, economic and technological research, HOMER Pro software has been used to prototype the system's operation and to determine the correct system configuration. The models were based on both grid-linked and stand-alone systems. The effect of yearly load growth and government policies on energy such as carbon taxes, the provision of low-interest loans to RE sources, and the shift in system viability of grid electricity prices were addressed. Data indicated that with COE and the renewable fraction of 9.3–12.6 kWh and 0–43.9 percent respectively, wind turbine, PV Panel, and battery sizes of 0–600 kW, 0–1000 kW, and 1300 kWh are attained for off-grid systems. For grid systems lacking battery storage, with the same wind turbine and PV panel sizes, the COE and renewable fraction ranges are 5.7–8.4/kWh and 0–53 percent, respectively.
The overview of the articles written in the African continent is shown in Table 6 while the overview of the articles written in the Americas continent was shown in Table 7.
Author(s) | Hybrid System | Parameters | Location | Country | Remarks |
Yahiaoui et al. [60] | PV/Diesel/Battery | ACC, ARC, AFC, AOM | Remote village (Djanet) | Algeria | GWO offers higher capabilities, reduced costs, and faster convergence. |
Muh and Tabet [61] | PV/Hydro/Diesel/Battery | COE | Offshore | Cameroon | Southern Cameroons' most viable economic system. |
Fodhil et al. [62] | PV/Diesel/Battery | COE, RF | Small village | Algeria | The PSO-based strategy is less expensive and has a higher PV penetration than HOMER. |
Diemuodeke et al. [63] | Diesel/PV/Wind/Battery PV/Wind/Battery |
CO2 emission, Payback time, COE | South-South region | Nigeria | For renewable energy specialists, the methodology offered serves as an ideal design tool. |
Djelailia et al. [64] | PV/Diesel/ Power Storage Hydroelectric | Fuel Consumption, CO2 emission | Remote site (Adrar) | Algeria | The idea demonstrates the utility of pump storage hydropower in irrigation and power recovery. |
Kaabeche and Ibtiouen [65] | PV/wind/diesel/battery | TNPC, COE, CO2 emission | Ghardaïa | Algeria | When compared to a PV/wind/battery system or a diesel generator (DG), the PV/wind/diesel/battery option is more cost-effective. |
Babatunde et al. [66] | PV/diesel/battery system | NPC, RF, COE | Rural health centers | Nigeria | In terms of the parameters, a PV/diesel/battery system is the most cost-effective. |
Samy et al. [67] | PV/FC | TNPV and LCOE | Remote urban areas | Egypt | Among the other methods, the FPA Algorithm has the shortest fulfillment time and the best rendering. |
Ogunjuyigbe and Ayodele [69] | PV/Diesel/Battery | NPC, COE, CO2, and CO emission | Telecom company | Nigeria | Out of the five economically viable configurations, PV/Diesel/Battery provides the best cost-benefit ratio. |
Tijani et al. [70] | PV/ Diesel/ Battery | NPC, COE, Operation, and Initial cost | Remote location (north) | Nigeria | The suggested system has a high energy potential and reduced carbon emissions while maintaining an affordable electricity cost. |
Samy [68] | PV/wind/battery, PV/FC, wind/FC, PV/wind/FC, and PV/wind/battery/FC | NPC, COE, Initial capital | Monshaet Taher Village | Egypt | A cost-effective hybrid renewable energy system was created to fulfill the known electric load of a small town in Egypt. |
Salisu et al. [71] | PV/Wind/Battery/Diesel | NPC, COE | Moriki village | Nigeria | The results also revealed a spectacular reduction in greenhouse gas (GHG) emissions of 100 percent because the ideal arrangement contains a 100 percent renewable portion. |
Rao et al, [72] | PV/Wind/Conventional Electric generator | NPC, COE, and CO2 emission | Deleta village | Ethiopia | In terms of economics and the environment, the hybrid design is the best ideal hybrid configuration. |
Babatunde and Ighravwe [73] | PV/Wind/battery, PV/battery, Diesel/battery, Wind/Diesel/battery, PV/Wind/Diesel/battery, PV/Diesel/battery | NPC, RF, COE, Operating cost | Rural community (Abadam) | Nigeria | Diesel/batteries were the best HRES for the case study from an economic standpoint, while wind/diesel generator/batteries were the best HRES from a technological and techno-economic standpoint. |
Sanni et al [74] | PV/Diesel | NPC, COE, Operating cost, RF | Kadawa village | Nigeria | The findings demonstrate that using renewable energy in agricultural activities can help achieve food security. |
Olatomiwa et al. [75] | PV/Diesel/battery and PV/Wind/Diesel/battery | NPC, COE, and CO2 emission | Remote mobile BTS | Nigeria | When compared to a stand-alone diesel generator system, the hybrid PV–diesel–battery system gives a greater reduction in CO2 emissions each year. |
Okundamiya et al. [76] | Wind/battery | COE, PSR and performance index | Typical residential region (Sokoto) | Nigeria | The proposed power system might save money and increase electricity reliability, according to the findings. |
Allali et al. [77] | Wind/Diesel/battery | - | Remote site (south) | Algeria | This system features a sophisticated control technique for managing several power sources (wind, diesel, and battery) that is dependent on weather circumstances, particularly wind speed values. |
Author(s) | Hybrid system | Parameters | Location | Country | Remarks |
Weis and Ilinca [78] | Wind/Diesel | Rural communities | Canada | Wind turbines are only acceptable for use in distant Canadian towns when the average yearly wind speed is at least 6.0 m/s. | |
Osaretin et al. [79] | PV/Wind/battery | NPC, LCOE, and Operating cost | Remote oil and gas facility | Canada | Continuous pumping using a hybrid renewable energy system with solar, wind, and battery storage has been identified as the best practicable approach based on economic and technological benefits. |
Ciez and Whitacre [80] | PV/Diesel/battery | LCOE | - | USA | In some circumstances, studies demonstrate that storage packs with high-energy, low-cost lithium-ion cells can compete with a non-renewable alternative. |
Maleki et al. [84] analyzed modeling and designing an efficient system design of a hybrid energy model with various biodiesel fuels. The hybrid system is made up of photovoltaic panels, a battery storage system, and a diesel power generator for a standard Iranian townhouse. Norouzak (Salvia leriifolia) seed oil is recommended as a source in Iran of indigenous biodiesel. The seeds were obtained from the Davarzan Khorasan dry wilderness. The hybrid PV/diesel generator/battery systems with various biodiesel fuels are tested and compared in terms of technological efficiency and costs. Using a basic methanol trans-esterification process and a ratio of 6:1 and 1 percent potassium hydroxide as a catalyst, Norouzak biodiesel has been obtained with an Ultrasound Method at an amplitude of 60 percent and 9 metres. For cost analysis, the system component components are measured with a computational model, which was used to measure the system components optimally with a version of the harmony-search algorithm to meet the demand most cost-effectively. The results showed that Norouzak fuel was an excellent option for an alternative fuel-hybrid system and that using this hybrid photovoltaic/diesel generator/battery structure to suit the electrical needs of a rural house is advantageous as it reduces running costs and emissions of pollutants and increases efficiency.
The SCSM tourism industry relies entirely on the 24-hour power supply of diesel generators. Pollution from diesel-based power plants is dangerous for the environment in tourist spots. Hossain et al. [85] proposed a multi-optimal combination of a stand-alone HRES for a large resort site situated in SCSM, with detailed operational performance analysis. To test the system in economic and technical terms, HOMER software is used. The approximate peak and average daily load of the resort are 1185 kW and 13,048 kW. PV, wind, diesel, converter, and battery generators comprise the best integrated stand-alone hybrid energy system. The optimized system generated 17.15 million dollars NPC, 0.279 dollars/kW-hr COE, 41.6 percent RF, and 2,571.131 kg of carbon dioxide per annum. The diesel-only system, however, needs 21.09 million dollars in NPC, 0.343 dollars/kW-hr in COE, and 5,432.244 kg per year in carbon dioxide. The NPC, COE, and carbon dioxide emissions of the diesel system alone are higher than optimized by HRES. In all tourist places and distributed locations on SCSM and around the world with similar climatic conditions, the HRES model can be developed and analysed.
Wu et al. [86] intended to optimize the size of a reverse osmosis desalination-based diesel and photovoltaic power plant (see Figure 4) to boost freshwater supply and meet electrical power demands from a stand-alone region in Iran. To mitigate system life cycle expense, the battery bank sized, photovoltaic system area and diesel generator fuel usage are standardized within the proposed hybrid system. For this reason, a power management plan is developed and an efficient metaheuristic technique is used based on tabu quest. Compare results to those acquired by searching harmony and simulating algorithms for annealing. It also deals with the impacts on the macroeconomic variables of the hybrid system of differing fuel prices, interest rates, initial photovoltaic costs and initial battery costs. Results indicate that for a single diesel system or a single photovoltaic system in the studied area, the desalination system for photovoltaic/diesel/battery/reverse osmosis is economically and ecologically beneficial. Furthermore, tabu search offers more positive results than the other investigated algorithms.
A PV/WT/FC/diesel model (see Figure 5) with different fuel prices for electrification to a remote location was carried out and is configured by DSA Maleki [87]. By analyzing multiple hybrid systems and two situations related to the cost of diesel fuel, the sizing issue was fixed.
A phased strategy for diesel replacement has been proposed by Rodríguez-Gallegos et al. [88]. The policy began with smaller initial investments and eventually incorporated solar panels and batteries to lower diesel usage in the following years. To evaluate the suggested technique, a case study of an Indonesian island was used. Maybe if diesel generators are being used, the life-cycle cost over 25 years would be 10.53 mil. This is illustrated by optimization by lowering the initial expenditure to 0.25 mil. The cost of the life cycle is reduced to 6.62 mils. USD and the annual savings are ultimately used to reinvest in the procurement of extra solar panels and batteries. USD-USD (only 3.8 percent of the initial investment is required). This lowers the initial investment hurdle with the suggested solution and thus allows more renewable sources of energy to be integrated into the current diesel generator systems only, which makes electricity generation simpler.
Rodríguez-Gallegos et al. [89] suggested a technique for optimizing the dimensioning and positioning of solar PV panels and batteries in installations that use diesel generators to create a PV hybrid as their primary source of electrical energy. 3 objectives have been defined: reduction of the cost of levelized electricity (financial objective); levelized emissions of carbon dioxide-equivalent life cycle (ecological objective); and cumulative deviation of grid voltage (grid quality target). Since this is a multi-objective problem, the latest meta-heuristic algorithm, i.e. the undominated genetic sortation algorithm Ⅲ, is used. Furthermore, by taking into consideration the worst-case scenarios about weather conditions, a robust design was created. The effect is contrasted with unrobust designs afterwards. Optimal parallel fronts with different variations are constructed with great versatility to choose for the desired style. The results show the advantages of photovoltaic hybrid systems in remote areas from cost-efficacy, cumulative pollution reduction and even grid performance increase.
Das and Zaman [90] examined a standalone hybrid application that fulfills a charge of 350 kW-hr-per-day with a peak demand for peak power of 74.34 kW to achieve a Photovoltaics/Diesel/Battery system output in a remote locality in Bangladesh. Two main battery technologies are used to research the influence of different dispatch techniques on the COE and the NPC (LA and Lithium-ion). The study was also focused on efficiency measurements in the HOMER software to determine the impact of the choice of diesel generators. Tests show that, compared to the LF and CC strategies, the CD strategy has a substantially lower energy cost. Nevertheless, the operating emissions of the LF Strategy for both hybridized systems are substantially lower than for the other two methods. The RF is greater than the CD and CC approach at EE prices in LF. It is also evident from the results that the cost of capital and the discount rate have a major effect on energy pricing and net current prices. However, petrol, battery and PV module costs have marginal effects on all Photovoltaics/Diesel/LA and hybrid Photovoltaics/Diesel/Li-ion-based structures.
The possible future deployment of the hybridized energy (e.g., PV/Wind/Diesel) with battery storage systems in Northern Bangladesh was studied by Mandal et al. [91]. For different device setups, techno-economic viability is analyzed and an optimized device with HOMER software is chosen. The hybrid platform is built to satisfy the 242.56 kW-hr-per-day demand for load in a rural place with a peak demand of 51.52 kW. The results of the study reveal that the enhanced hybrid system includes a 73 kW photovoltaic array, a 57 kW diesel engine, a nominal 387 kW-hr battery power bank, and 28 kW inverters with the lowest possible COE of 0.37 USD per kW-hr, and an NPC of 357.284 USD. The result also showed that the enhanced system decreases carbon dioxide emissions by about 62 percent relative to the kerosene used in the current situation and 67 percent relative to the grid-connected network. Furthermore, the LCE (kilogram carbon dioxide-eq-per-year) output is substantially lower than in all the other setups of this system in the PV/Batt/ICE system. The DF of this system is lower than the Wind/Batt/Diesel system and Batt/Diesel. Although this scheme is not the equivalent of the grid tariff, its approach to the solar micro-utility system, Wind/Batt/Diesel, and the Diesel generation system alone is financially viable.
A mathematical model was developed by Javed et al. [92] to configure a prototype solar-wind energy network for a distant island with GA storage. The normal HOMER algorithm provides that the GA solution can produce a method that is more cost-effective and accurate than HOMER and the results of this are evaluated in 4 separate cases. Moreover, 2 systems are compared and their results show very little variation in system cost and efficiency, so the wind turbine has little impact on output. Wind turbine size is not considered very significant. Sensitivity analysis on certain important components indicates that the initial cost of capital (25 percent to 30 percent), operating costs (15 percent to 17 percent), and COE are significantly lowered, taking into account modest (1 percent to 5 percent) LPSP. The sensitivity analysis shows that the off-grid/isolated zones with minimal LPSP can be energised better than no electricity at all.
For the city of Yanbu and Saudi Arabia, Ramli et al. [93] conducted optimum battery storage sizing of a PV/wind/diesel HMS by using the MOSaDE algorithm. Using the multi-objective optimization technique, the objectives are interpreted simultaneously and independently, resulting in a reduction in computational time. The technique for energy management, which is necessary to coordinate the multiple units that make up the HMS, is among the primary factors to consider when designing and improving the HMS. The multi-objective optimal solution is then used in terms of price and productivity of HMS to determine the likelihood of loss of power supply, COE, and the renewable factor and is analyzed using 3 case studies featuring various house numbers. The findings affirm its role in the optimization and functional application of the HMS. In addition, optimization results using the suggested method presented a collection of HMS design solutions to help academicians and professionals select the right configuration for HMS. Moreover, it is important to choose optimally sized HMS modules to make sure that all load conditions were met at the minimal cost of energy and high reliability.
Maleki et al. [94] proposed a framework (shown in Figure 6) for combining geographical information systems, artificial bee swarm enhancement, and simulation to assess the optimum size and location of PV panels yearly. First of all, the GIS module is intended to select the exact location and capacity of a stand-alone PV system in rural areas, taking into consideration the technical, ecological, and socio-economic aspects influencing the extent of selecting stand-alone PV systems. An efficient heuristic approach, called artificial bee swarm optimization, is then recommended for the optimum dimensioning of a stand-alone PV structure to continually meet the demand for loads with reduced costs and increased energy network performance. For this purpose, the maximum permissible likelihood of power supply collapse is also viewed. The proposed device is applied to a particular research study in Eastern Iran and the results indicate it can be used efficiently for both power quality and cost factors for off-grid photovoltaic projects in isolated areas.
Hafeznia et al. [96] put forth a system to analyze the efficiency of solar photovoltaic energy for implementations on a utility scale. To achieve this aim, the framework integrates spatial planning and performance modeling of photovoltaic power systems into the potential assessment process. Birjand Region was chosen as a case study because owing to its environmental conditions, it is an ideal area for the implementation of photovoltaic technologies. It should be in line with land-use planning requirements to establish solar power plants as a subset of infrastructural development. During the first step, the list of factors (27 requirements) that influence the decision of on-site photovoltaic power plants was defined. Boolean logic had left them out of the county diagram after discovering unsuitable regions. Other areas have been categorized according to science, socio-economic and ecological requirements, based on different fluid membership roles. Those identified areas are then mapped. Fuzzy gamma operators were overlaid to select the optimum location for grid-connected photovoltaic systems. Then, the ultimate map pixels in their fuzzy value were listed in 5 clusters. Findings demonstrate that the territory of Birjand accounts for 0.5 percent (2005 hectares) of ideal photovoltaic power plant building locations. The results of the geospatial modeling of this study were verified by using satellite images. A photovoltaic device of 46.2 kW was developed to estimate the production capacity of electricity from photovoltaic power plants and to model its performance and energy outcome under the climatic and geographical conditions of Birjand. Not only can the estimated energy output of photovoltaic power plants at the ideal sites of Birjand County (1781.6 GW hr-per-year) meet the electricity demands of the Southern Khorasan Region, but the excess electricity generated can also be exported to other regions and neighbouring countries such as Pakistan and Afghanistan.
Photovoltaic/wind generator/diesel hybrid model configuration, size, and cost analysis of 2 storage systems, dependent on [95]: battery and fuel cell. In comparison to traditional Photovoltaic/Wind generator/diesel/battery structures wherein battery banks are being used as storage devices, Photovoltaic/Wind generators/diesel/Fuel cell systems integrate fuel cells, electrolyzer, and hydrogen storage tanks as the energy storage system. A mathematical model for the element of each system is implemented for cost analysis, and now a discrete version of the HS algorithm is constructed to efficiently scale the system components to meet the load demand most cost-effectively. As an effective search method, HS has a simple definition, is easily implementable, can escape local optimum through probabilistic mechanisms, and it requires just an initial search approach. For contrast and validation reasons, two robust optimization approaches, the SA and the HOMER are used. The economic aspects of Photovoltaic/Wind generators/diesel/Fuel cells are also contrasted with the traditional photovoltaic/wind generators/diesel/battery system, and the annual average cost for the systems is clarified in detail.
For distant locations in India, the optimum size and shifting of a hybrid photovoltaic/battery/diesel system are performed by Jeyaprabha and Selvakumar [97] with no meteorological information by using AIT. The optimal sizing and inclination angle analysis was initially planned for different cities across India, with the various meteorological data available for low cost and zero load discarding. By using latitude, longitude and elevation of any remote location, the optimum size of a hybrid system is identified by AIT. It is also anticipated that the tilt angle for the PV array to be placed in any distant area will reduce the hourly use of the diesel generator for all 4 seasons and that the number of manual surveillance visits through this study will be optimized to 3 per year. By using ANFIS and ANN approaches, the expected optimal values are compared with the measured values. The expense of the integrated hybrid system is contrasted with the standalone PV and also the cost of the diesel generator system to show its cost-efficiency. With a load probability loss of 0.00266, the efficiency of the sizing system for various load demands is also demonstrated.
In Sarawak, East Malaysia, Das et al. [98] debated the potential for renewable energy. The wind speed is ineffective, but the site has plenty of solar power. For loading, a long-housed village consisting of fifty families in Kapit, Sarawak, the viability of PV, battery and fuel cell-based systems is therefore examined. A tool for the study is used for HOMER software supplied by the NERL. The study also focuses on the operational strategy and sensitivity analysis of the hybrid model for optimum performance. With a net present cost of 335,297 USD, and no pollution energy cost of 0.323 USD/kW-hr, the findings of the HOMER optimization and sensitivity analysis suggest that the photovoltaic/battery system is economical and environmentally friendly. Therefore, a diesel-based network may be acceptable as an alternative.
In the Kollegal block of Chamarajanagar, State of Karnataka, India, Suresh et al. [99] carried out a study for electrifying a group of 3 villages using a grid hybrid renewable system. The process of optimizing the regulation, size and element preference of these hybrid energy systems is the provision of a cost-effective power solution for the company. The primary aim of this study is total system NPC, COE, unmet load, and Carbon dioxide using GA and HOMER Pro Technology. 4 variations of HRES are compared with the effects of both methods. In the end, GA and HOMER are subject to a comparative analysis. Especially in comparison to HOMER, the combination-1 GA-based HRES (biogas + biomass + solar + wind + battery-powered fuel cell) is the perfect solution to create power at a low energy cost of 0.163 USD per KW-Hr with an unmet load of 0 percent as shown in Table 8. PV saturation is also more cost-effective than HOMER in GA.
Optimization methods | GA | HOMER |
Total NPC ($) | 856013 | 890013 |
COE ($/kWh) | 0.163 | 0.214 |
Total production (kWh/yr) | 3,36,543 | 3,49,493 |
Total consumption (kWh/yr) | 3,16,428 | 3,28,266 |
CO2 emissions (kg/yr) | 3842 | 4089 |
For the report, in the Kollegal section of Chamarajanagar district, Vendoti et al. [100] recognized three (3) villages for electrification. Using GA, the key aim is to decrease the device's total net present cost and the lowest energy costs. For the usage of all renewable energy resources in various combinations, four (4) models have been selected. HRES-based solar PV-wind-biomass-biogas-fuel cell battery generates an overall NPC of 890,013 USD at an unmet 0 percent charge from the test.
In Chamarajanagar district, state of Karnataka, India on the optimisation of sustainable hybrid (solar-wind-diesel) structures in isolated rural Indiganatha. Vendoti et al. [101] goals are to reduce the present gross net production costs and energy costs with HOMER Pro. The sensitivity analysis is often conducted to determine the effects of insecurity or parameters and to find the best solution for the hybrid energy system.
At a specified remote village place in India, Vendoti et al. [102] planned to establish a power generation model based on various combinations of HRES with a renewable energy software program. The main objectives of this study are to identify the optimal configuration of a dynamic RE system from various combinations to fulfil the demands of the village load reliably, consistently and sustainably. The analysis also uses the multi-objective HOMER Pro software to decrease the device's average net current cost and the lowest energy prices. In this study, a resource assessment and demand calculation were conducted, and the COE per unit was measured for different systems and specifications. With a COE of 0.214 USD per kW-hr (see Table 9), the cheapest and most reliable solution was defined as a combination of PV/Wind/Biomass/Biogas/FC and battery.
Configurations | NPC ($) | COE ($/kWh) | Operating Cost ($) |
C1 (PV/Wind/Biomass/Biogas/FC and Battery) | 890,013 | 0.214 | 34,109 |
C2 (PV/Wind/Biomass/Biogas/FC without Battery) | 897,847 | 0.215 | 36,917 |
C3 (PV/Wind/Biomass/Biogas/Battery without FC) | 929,878 | 0.223 | 29,354 |
C4 (PV/Wind/Biomass/Biogas without storage) | 1,095,020 | 0.263 | 52,148 |
Two storage systems were included in a study conducted by Vendoti et al. [103], both electricity and power generation. Whilst a battery produces energy from the energy inside the unit, it uses fuel to create electricity from a fuel cell in an external fuel tank. These include the sequence PV, PEM and 7-stage MLI. First, in this research, the MATLAB/Simulink environment generated a dynamic model with the necessary processing. Finally, the results of the simulation for the renewable hybrid energy system suggested are discussed.
A hybrid energy system focused on renewable resources in a given area was suggested by He et al. [104]. HOMER software has been used to refine the system and incorporate the economic theory to achieve the optimal system setup. 80 kW PV20 sets of 10 kW wind turbine, 300 kW batteries and 30 kW generator are the perfect unit, with an investment amount of 709,471 USD, with a power shortage of 0 percent. The effect of the power failure was evaluated and the findings show that the battery capacity adjusted most as the reality of the system worsened, while the economic performance increased drastically with the COE ranging from 1.001 USD per kW-hr in capacity shortage of 0 percent to 0.619 USD/kW-hr in capacity shortage of 5 percent.
To maximize the size of PFWHS components, Nowdeh and Hajibeigy [105] carried out an optimization size study on a standalone PV/FC/Wind Hybrid System. Based on the PSO algorithm, an improved sizing method was developed to determine the efficient system configuration that can attain the load necessary probability of power supply (reliability) with a low OCE. Investment, repair and maintenance, as well as lack of load costs, entail costs for PFWHS. The wind and radiation data sets used belong to the Iranian Northwest Region of (Jolfa, latitude: 3856, longitude: 4537, altitude: 710 metres). The effect on optimum sizing of the accessibility of PFWHS components will be probed. The impact of the product availability rate on PFWHS costs and dependability due to malfunction and repair rate of PFWHS elements and wind speed and solar irradiance uncertainty is therefore categorised into 2 scenarios. Results indicate that the reliability indices are within an acceptable boundary of the reliability parameters even though the overall energy cost is reduced. Furthermore, the results indicate that taking into consideration the complete available components, the availability of components must be discussed in an appropriate way to accomplish the actual behavior of PFWHS. Rajanna and Saini [106] concentrated on the improvement of IRES optimal sizing models to meet the energy needs of the different load sectors from 4 distinct zones identified in the Chamarajanagar district of Karnataka, India. The study aims to reduce the overall cost of generation and energy prices by using a GA-based strategy. The optimized feasibility of the system was tested, taking into consideration the optimization power factor as well as the projected energy not supplied. IRES was known to be capable of offering a plausible solution between OPF values of 1.0 and 0.8 based on the test.
Off-grid electrification is expected to address the electrical and cooking requirements of the 7 non-electrified villages in the Almora district of Uttarakhand State, India, by the use of IRES. Four distinct scenarios are taken into account during IRES modeling and optimization in the Kanase-Patil et al. [107] analysis; to assure reliability variables such as EIR and estimated unsupplied energy. The optimum network performance, total device costs and COE were also calculated by the addition of the CIC. For the study area regarded, version 10 of the LINGO program was used to compare 4 different renewable energy situations. The best of the different choices was noticed to be the fourth renewable energy case, which accounts for 44.99 percent micro-hydro power, 30.07 percent biomass, 5.19 percent biogas and 4.16 percent solar energy, with additional wind (1.27 percent) and energy plantation (12.33 percent) capital. Furthermore, the best possible reliability of the fourth IRES system was 0.95 EIR for Rs 19.44 lakes with a mean COE of Rs 3.36 per kW-hr. The COE received by LINGO software and HOMER has also been compared in all 4 cases and discussed briefly. To verify the feasibility and costs of the system for different levels of biomass fuel, a sensitivity study was also underway, and a fourth case was discovered to be more feasible than the alternative suggested.
To resolve the load demand for a 4-zones group in the Indian state of Karnataka, Rajanna and Saini [108] focused on optimizing the size of an IRE with a battery bank (see Figure 7). Hours of demand for loads were simulated with HOMER software based on three scenarios: HILR, MIMR and LIHR. Optimum scale, total NPC and COE model results were generated with the aid of GA taking account of different situations, with the energy index at 1. 3 DSM-strategy situations were recorded in comparison to those without DSM. Based on the comparison of findings, the HILR situation and DSM strategy found that TNPC and COE were lowest for area 1 and area 2, while MIMR scenarios with DSM were found to be less TNPC and less COE for zone 3 and LIHR with DSM for zone 4. Moreover, results from GA are received. By comparing the PSO form, Total NPC has been assessed. GA and PSO outcomes were shown to be essentially the same, it was noted.
Dorji et al. [109] examined rural household energy requirements, resources accessible and existing policies and programs in the field of rural electrification. The HOMER for modeling distributed systems on-grid and off-grid was also used to identify the most inexpensive approaches for a variety of potential resources and to compare the cost of using them with traditional grid expansion systems. This research analyzes and compares possible choices for rural electrification in Bhutan with the extension of the grid to remote areas.
Baek et al. [110] evaluated and proposed the best hybrid renewable power network for Yeongjong Island by using empirical data (load data, meteorological data, equipment data and macroeconomics factors). Table 10 displayed the content of the suggested optimal renewable power generation system. The findings demonstrate a 100 percent hybrid technical, socially and economically achievable renewables-driven energy system for Yeongjong Island's electricity in academia. Firstly, there are lower costs and shorter constructions for renewable power stations on the island than for other power stations. Secondly, there is no carbon dioxide emitted by the island's renewable energy facility and no danger that the residents will be resilient to more emissions from the setting.
Components | Index |
Wind (numbers of turbines) | 11 |
PV (kW) | 357 |
Converter (kW) | 57 |
Battery (numbers of Battery) | 825 |
Grid (kW) | 0 |
Operating cost ($ per year) | 76,081 |
Initial capital ($) | 1,861,625 |
Total NPC ($) | 3,506,509 |
COE ($/kWh) | 0.545 |
Niazi et al. [111] have been working on technology and economic analysis for small-scale wind/solar combined systems users in domestic applications. Research is being carried out on the HOMER Ⅱ simulation software. Pakistan is also one of those developing countries experiencing a serious power crisis. HOMER Energy software program is used to analyse various systems and provide the most reliable and practicable possible system for the user. To discover a system that was economically and technologically possible, a simulation was carried out. Sensitivity analysis was modeled with hub height, de-rating factor for photovoltaic, life for photovoltaic, and life for the wind turbine. As the main variables of the system, shifts in hub height have had a significant effect on energy production and economic prospects. It can be of real benefit to address energy shortages by introducing such small hybrid systems at the local scale.
Tudu et al. [112] presented an improved edition of the strategy of particle swarm optimization to achieve unit sizing and technology-economic feasibility study of the off-grid hybrid energy system for the Sundarban area, the world's biggest mangrove forest partially situated near Bengal, India, taking into consideration the actual load data and other environmental variables. Originally, the combined energy system is designed with both the load pattern in view and the existence of that specific site's renewable sources in view. By incorporating various renewable energy sources like wind turbines, photovoltaic panels along with batteries and diesel generators, the combined system is planned to meet the regional load demand at varying times. To attain the best unit size of the system, NPC and COE for electricity generation were suggested. Pollution from the combined system is also analysed and compared to the conventional energy system based on emissions per unit of generation, and it is seen that, with the introduction of this combined model, 0.688 kg of Carbon dioxide emissions per unit of energy production can be decreased when fulfilling local demand. The enhanced version of the strategy of particle swarm optimization is proven to be extremely quite capable of fixing this complex non-linear optimization problem somewhat effectively.
An effective sizing strategy for enhancing the genetic algorithm-based setup of the HES was presented by Al-Shamma and Addoweesh [113]. The proposed optimization model was used to investigate the technology-economic potential of HES to fulfill the load demand of a rural place in northern Saudi Arabia. The optimum design is achieved not only by selecting the least expensive combination, but also by finding an appropriate proportion of renewable energy that meets the requirements of load demand with null rejected loads. Furthermore, the economic, technology and ecological characteristics of 9 distinct HES settings have been studied and their performance weighted. The simulated results showed that the optimal choice of WT is not only influenced by the WT speed or WT rating power variables, but also by the required renewable energy proportion. It was observed that the rated WT velocity had a major impact on the proper selection of WT, while the rated WT power had no consistent effect on the proper selection of WT. In addition, the findings clearly stated that the HES comprised of PV, WT, Battery Bank, and DG dominates all 9 ecological efficiency systems studied here. Only when there is so little wind power and high solar energy density is the PV/Batt/DG combined model viable. On the other side, the WT/Batt/DG combined model is only possible at strong wind speed and low solar density. It was also observed that the addition of batteries decreased the essential DG and thus decreased fuel consumption, as well as the cost of operation and maintenance.
Rajanna and Saini [114] emphasized optimal IRES sizing, considering the various commonly available renewable energy sources, including micro-hydro, solar, wind, biomass, and biogas with a distant electrification battery system in Karnataka, India. Considering the 3 decision variables, the GA was used to minimize the overall NPC and energy expense of the installed IRES model. Figure 8 displays the schematics of the developed IRE model: the total active sunshine region covered by the SPV modules, the total sweep area required to install windmills, and the charging level of the battery system. For the research area, 3 IRE scenarios based on optimization results are suggested. Zone 2 and Zone 3 of those three, scenario-S1. It is observed that for zone 1 and zone 4, scenario-S2 is most feasible for the study region. In addition, across all zones, the best schedule, resource combination and system type were also calculated.
Yuan et al. [115] designed a distributed HRES composed of PV arrays, a combined heat and power generator with wood syngas and backup batteries to drive a traditional semi-detached rural house in China that seek to satisfy the energy demand of a house and reduce fossil fuel greenhouse emissions. A system model is established using HOMER software centered on the house's annual load specifics and local weather parameters, like solar radiation, air temperature, etc., which is used to simulate all possible configurations for technological and economic evaluations. In different configurations, the performance of the entire HRES system and each part is measured. The system architecture is optimized.
From the various combinations of mixed power systems, Kirmani and Shadab [116] have found the optimum specification that will meet the electrical energy demands of the selected village economically and efficiently. The various systems under consideration are the linked solar-grid system, the solar-diesel system, the solar-wind power network, the linked wind-grid network, the linked solar-wind-grid system, and the linked wind-diesel-grid system and the linked solar-diesel-grid system. These models are compared in the terms of different economic metrics, like the internal rate of return, the net present cost, the payback period and the cost of electricity. For the case considered with the smallest energy cost as Rs. 2.34-per-kW-hr, the most appropriate hybrid model is from among the various systems examined in this research linked to the wind-grid system. This system's upfront cost is also lower than other hybrid systems.
Abujubbeh et al. [117] performed a techno-economic sustainability evaluation for an on-grid PV-Wind hybrid system to ensure the average annual energy demand of households in Amman, Jordan. The analysis indicates that there is a huge potential to satisfy the need for household energy for several months of the year and the system is capable of generating surplus power that can be sold to the national grid, which generates large project revenues. Moreover, in Table 11 which shows the proposed economic parameter, the hybrid model has an LCOE rather than a national grid tariff. As a result, this research contributes greatly to the nation's measures to cut reliance on imported fossil fuels to satisfy domestic energy requirements.
Parameter | Value |
LCOE ($/kWh) | 0.159 |
NPV ($) | 24,618 |
IRR (%) | 16.3 |
PBP (years) | 6.4 |
Annual life cycle saving ($/year) | 788 |
Ramli et al. [118] examined the capacity of the hybrid wind/solar power system in the west coast area of Saudi Arabia in this report. The inquiry focuses on the production of electricity and energy expenses from both wind turbines and PVs in the hybrid model. Consideration will be given to energy charges not met and to energy in abundance. The annual average solar irradiation and wind speeds in this study are 5.95 kW-hr-per-m2-per-day and 3.53 meters-per-seconds, respectively. The MATLAB and HOMER applications are used to perform technological and economic studies of the hybrid method. The photovoltaic arrays generate higher power output than the wind turbine generator when the wind turbine and photovoltaic arrays are being used with the same dimensions in the wind / solar hybrid system. Wind COE leverages are costlier than the solar energy of 0.0637 USD per kW hr, at a rate of 0.149 USD per kW hr. As shown in Figure 9, the hybrid system energy costs are dominated by batteries and wind turbine costs.
Taking into consideration an average load demand of 80 kW/hr/per/d with a peak load of 8.1 kW-hr-per-d, an off-grid hybrid power system and simulation was developed to support a limited population [119]. To simulate and enhance system operations, the HOMER program used real-time data sets of the solar radiation, wind speed, and biomass of that particular area. The study ensures that the system is economically and environmentally feasible in terms of NPC and Carbon dioxide emissions restrictions. The results indicate that NPC and Carbon dioxide emissions can be reduced by about 29.65 percent compared to conventional power plants, equivalent to 16 tons per year. The observed combined energy system could be relevant to other regions of the globe where similar climate patterns exist. The NPC of the configured system was found to be approximately 160,626.00 USD, with a COE per unit of 0.431 USD-per-kW-hr.
Alharthi et al. [120], based on a community mean demand of 15,000 kW-hr-per-day of community charges and a peak load of 2395 kW, was planned in a report. The program HOMER is used to estimate renewable energy resources capability and to perform technological and economic evaluations of the grid-linked composite system. The data on the climatic conditions were obtained from KACARE's Atlas of Renewable Resources. In the Kingdom of Saudi Arabia, 4 distinct cities were chosen to do the study, including the cities of Riyadh, Hafar Albatin, Sharurah, and Yanbu. The simulation experiments demonstrated that the proposed structure is economically and ecologically realistic in the Yanbu region. It has the least NPC and LCOE in the city network, the highest total power that can be marketed on the grid, and also the lowest Carbon dioxide emissions due to the incorporation of strongly renewable energy. For similar weather and environmental factors, this grid-connected hybrid model with the suggested design is relevant in the area and across the globe. Cutting certain greenhouse gases and reducing energy prices are the biggest contributors to these jobs.
A technology-economic study of microgrid operation in Jordan was carried out by Al-Asfar et al. [121] and the efficiency and economic effect of combined renewable energy systems for a chosen residence in the University of Jordan area were analyzed. Various situations based on the highest, lowest and mean data of solar radiation and wind speed were created between 2007 and 2016. Three combined microgrid renewable energy systems, namely: (1) photovoltaic, wind turbine, diesel and battery bank, (2) photovoltaic generation and wind turbine, and (3) diesel generator, have also been tested. The comparison showed that the lowest net present costs of 33,547 USD, and the lowest electricity costs of 0.237/kW-hr, have been identified in the first combined renewable energy system, under the scheme of peak solar radiation and wind speed. The ideal specification of the microgrid combined renewable energy system, therefore, comprises a photovoltaic generator of 1.3 kW, a wind turbine of 9 kW, a diesel generator of 1.6 kW and a battery bank of 6.
Tendo and Sirisamphanwong [122] received diagrams for a stand-alone photovoltaic-diesel hybrid model for maximizing system size. This is done using HOMER software to further develop device parameters such as output ratio, renewable energy proportion and also MATLAB. The goal is to achieve the optimum size that can satisfy the energy needs at the optimum price for a given length of the project. This study will be performed based on a system recently established at the Naresuan University Renewable Energy Institute, which has a capacity of 120 kW and is a combined Photovoltaic collection system, a diesel generator and a battery storage system. The cost variables to be analyzed are as follows: NPC, and COE (see Eqs 1 and 2). After modeling and simulating with HOMER software utilizing special designs, the first size for a hybrid system is the Photovoltaic-120 kW, Diesel-100 kW and Battery Storage-200 kW-hr. The ideal size is 100 kW Photovoltaic, 100 kW diesel and 100 kW-hr batteries in contrast with the initial COE and 10.01 USD-per-kW-hr. The optimum size of this system consists of a COE of 0.934 USD-per-kW-hr.
NPC=TotalAnnualisedCostCRF(i,l) | (1) |
COE=TotalCostEnergyyield | (2) |
where CRF is the Capital Recovery Factor, i is the annual real interest rate, l is the lifetime period of the project. Total annualised cost includes the total costs for each system component for each year which is the sum of the operating cost, the capital and replacement costs.
In the community of North-East Saudi Arabia, Rehman and El-Amin [123] suggested a wide range of hybrid wind, photovoltaic and diesel architectures for a settlement. Configurations 1) only diesel, 2) wind/diesel, 3) Photovoltaic/diesel and 4) wind/Photovoltaic/diesel generating systems are designed to take account of minimal energy costs and the lower environmental impact and are contrasted with the best alternative system. For scaling and enhancing the combined power systems, local hourly mean wind speed, hourly overall solar radiation, and hourly load details were used as inputs. Of the 4 configurations tested, the diesel-only framework is found to be the most cost-effective, with a COE of 0.037 USD per kW hr with a fuel cost of 0.067 USD/l. Among the combined energy systems, the most economical approach with a COE of 0.038 USD-per-kW-hr is the Photovoltaic-diesel hybrid model with 1,500 kW Photovoltaic capacity, equivalent inverter capacity, and 4 diesel generators with 1120 kW capacity each.
The off-grid renewable energy generation network has lower energy costs and greater performance compared to single solar photovoltaic or wind energy systems [124]. For different case studies, the UCOE carries out the principle of enhancement and compares the results achieved using the HOMER-Pro program. The combined energy system's optimal cash flow research is based on the charging patterns being discussed, site solar irradiance (kiloWatts-per-m2) at acceptable latitude and longitude, wind velocity, and diesel price collected from a remote village in Khurda District, Odisha, India. Furthermore, by changing the input variables such as solar radiation, wind speed, etc., the optimization and sensitivity results of the device are found.
Simulation results are obtained on the cross-use of a Photovoltaic/wind/battery/fuel-cell composite energy source to power an off-grid living area. The combined use of different renewable energy sources to operate off-grid installations is illustrated alongside battery and hydrogen energy storage solutions. To perform extensive technology-economic analysis, HOMER software from the National Laboratory for Renewable Energy was being used. It replicates all practicable system configurations that meet the proposed load for the sample locations under the constraints of the renewable resources given [125].
The technical and economic viability of the combined energy system in the electricity grid of Oman at Masirah Island was evaluated by Al Ghaithi et al. [126]. The method relates to HOMER's use to optimize the suggested combined structure. The DIgSILENT simulation software program is used to model and simulate the incorporation of the suggested combined system into the existing network. In the estimation of the effects of combined energy system incorporation, various scenarios are taken into account in both the enhancement of the hybrid system and the different relation cases. The obtained results showed that the composite energy system consisting of diesel, photovoltaic and wind generator systems is the most financially viable alternative because it offers the lowest net current system costs, running costs and energy expenses, while at the same simultaneously enhancing the voltage profile of the connection point.
The objective of Lao and Chungpaibulpatana [127] was to evaluate the optimal scene of a combined system for supplying power to 1 distant district in Cambodia. Using HOMER as an approach for techno-economic analysis, this study discusses 3 situations: diesel only; diesel/Photovoltaic; and battery-system diesel/Photovoltaic. The results indicate that diesel/Photovoltaic with a battery is the ideal choice. 13 percent of 0.377 USD-per-kW-hr solar photovoltaic penetration with COE is made up of this hybrid unit. The initial capital cost and gross NPC are 2,260,000 USD and 16,661,344 USD respectively. If this model also removes nearly 720 tons of carbon dioxide per year in contrast with conventional diesel-only scenarios. The research also conducts an overview of sensitivity with fluctuations in variables such as fuel prices, component costs, real interest rates, and demand loads.
Using the smart grid load management program, Mohamed et al. [128] implemented an optimal sizing technique depending on the available generation for a mixed renewable energy system. This algorithm seeks to maximize the network's energy performance and meet load requirements with minimum cost and maximum dependability. The photovoltaic collection, the wind turbines, the storage batteries, and the diesel generator form this device as a secondary source of electricity. This study examined the forming of demand profiles, using load shifting priorities, as being one of the intelligent grid applications. Particle swarm optimisation (as presented in Figure 10) is being used in the technique to determine the optimum dimension of the components of the system. The findings from this approach are contrasted with the findings from the iterative technique of enhancement to determine the suitability of the suggested algorithm. The analysis is being conducted in some remote locations in Saudi Arabia and can be used in all relevant regions worldwide. This study draws several valuable conclusions that researchers and policymakers may benefit from.
Ahmad et al. [129] have focused on the technology-economic practicability and possibility of electricity generation through combined wind, photovoltaic, and biomass networks for local people of Kallar Kahar in Pakistan near Chakwal Province of Punjab. The extensive resource evaluation of wind, biomass and solar power is performed for network integration. For the simulation of a mixed microgrid network, Homer Pro software is used. The optimization results and sensitivity analysis were carried out to maintain the robustness and cost-effectiveness of the suggested combined microgrid system. For the low local load requirements, the overall load was optimally distributed between the wind-generated power and the excess electricity was distributed to the national grid. Technology-economic feasibility research findings demonstrate that the combined power system can produce more than 50 MW. Table 12 displayed the economic component of the two best system configurations. The COE is determined based on the high-demand profiles for both residential and industrial segments. The cost is 180.2 million USD for the 73.6 MW peak loads combined unit and the LCOE is 0.05744 USD per kW hr.
System Configuration | Cost Component | Value | Unit |
PV-Biomass-Wind-Grid | Total NPC | 180,290,247.40 | $ |
LCOE | 0.05744 | $/kWh | |
PV-Biomass-Grid | Total NPC | 178,790,678.90 | $ |
LCOE | 0.0614 | $/kWh |
Ayadi and Alsalhen [130] introduced a mixed system that provides the versatility of an organization with a fascinating financial standpoint and increases dispatch ability. A wind farm and an integrated solar power station with accumulated thermal energy are involved in this composite model. The effectiveness analysis was conducted in terms of the eventual yield and capacity factor, while the levelized electricity expense of the LCOE was analyzed in the economic analysis. To model and improve the combined plant, TRNSYS 17 energy simulation program was used, decreasing the LCOE and assuming a capacity factor greater than 65 percent as a goal. The decision parameters are solar multiple sizes and energy capacity. At the specified site in Jordan, the attractions of the selected combined system were highly complementary to the direct normal solar radiation. With a solar area of 2.6 solar multiples and 5-hour energy storage, the optimum CSP-wind network combined system configuration was achieved. The capacity factor obtained was 94 percent and the LCOE was smaller than for independent CSP installations.
In the research of Mudgal et al. [131] mathematical modeling, size optimization and technology-economic analysis of standalone IRES were performed. Figure 11 shows the operational strategy flow chart of the proposed Hybrid Renewable Energy. The composite system is planned to have a peak contribution from wind and solar energy with a low system NPC to meet the demand for electric charges of the CRC building, IIT Madras, India (13.01 ° N and 80.24 ° E). The results showed that the most feasible system configuration consists of 117,098 USD and 0.09 USD-per-kW-hr, 12 kW Photovoltaics, 3 kW wind turbine and 15 kW NPC biogas generator and COE respectively. To reach the AC load of 64,396 kW-hr, the IRES produces 71,826 kW-hr of energy per year. The power factor and percentage contribution of Photovoltaic, wind turbine and biogas generators were 17.8 percent, 6.57 percent, 39.1 percent and 26 percent, accordingly, 2.4 percent and 71.6 percent.
Under different microgrid scenarios based on RE, stand-alone and grid-connected, the technology-economic potential and optimum design were investigated [132]. HOMER software is used to determine the physical, functional and economic effectiveness of the parts of the system and to establish the most cost-effective sequence of configuration. The obtained results from the Beijing residential community case study will enable the decision-maker to recognize the best use of renewable energy sources, production of electricity and financial advantages. The gross NPC of the microgrid system would be at most 57 percent less of the power produced from the external grids than that completely of the grid-connected design. Findings have also indicated that if wind power is the main source of energy and coupled with medium solar photovoltaic performance in the RE mixed microgrid system, it would be more cost-effective. In conjunction, in both stand-alone and grid-connected systems, a device with a small battery size tends to be more ecologically effective and cost-effective than a battery-less network.
A heuristic optimization algorithm was developed and analyzed by Olamaei and Ghazvini [132] to address the optimal sizing of the combined PV/battery/diesel network concerning the controllable load for the V2G parking lot. The simulation results showed that the total cost of the PV/battery/diesel network optimization will be reduced by 5.21 percent with the V2G parking lot. Table 13 shows a summary of the published articles in the Asian continent.
Author(s) | Hybrid System | Parameters | Location | Country | Remarks |
Shezan et al. [81] | PV/Wind/Diesel/battery | NPC and COE | Remote region | Malaysia | The simulation ensures that the system is financially and environmentally feasible in terms of net present cost (NPC) and CO2 emission reduction. |
Cai et al. [82] | PV/battery/Diesel | TLCC, LCOE, operating and maintenance cost | South Khorasan | Iran | The results reveal that combining solar energy with a diesel generator cuts greenhouse gas emissions and supply costs significantly when compared to a diesel-only system. |
Baneshi and Hadianfard [83] | PV/Wind/battery | COE | Shiraz | Iran | Carbon taxes could be an effective policy for encouraging large-scale renewable energy installations. |
Maleki et al. [84] | PV/Diesel/Battery | LCC | Rural townhouse | Iran | The findings suggest that Norouzak fuel is a viable alternative fuel-hybrid system choice. |
Hossain et al. [85] | PV/Wind/Diesel/battery | NPC and COE | Large resort sites (12 islands) | Malaysia | In the settings, PV, wind, diesel generator, converter, and battery make up the greatest stand-alone hybrid energy system. |
Wu et al. [86] | PV/Diesel/battery/Reverse Osmosis Desalination | LCC and LCOE | Remote area (Khorasan) | Iran | PV/diesel/battery/ROD is more cost-effective and environmentally friendly than a single diesel or PV system. |
Maleki [87] | PV/Wind/FC/Diesel, PV/diesel/FC, WT/diesel/FC | Total Annual Cost and emission | Remote location | Iran | The PV/WT/diesel/FC system is the best option in terms of pollutant emissions. |
Rodríguez-Gallegos et al. [88] | PV/battery/Diesel | LCOE and CO2 emission | Island | Indonesia | The findings demonstrate the cost-effectiveness, reduction of cumulative emissions, and even improvement of grid quality of PV hybrid systems in remote places. |
Rodríguez-Gallegos et al. [89] | PV/battery/Diesel | LCC | Island | Indonesia | An investigation was conducted to develop a method for successfully hybridizing DG-only systems with solar panels and batteries. |
Das and Zaman [90] | PV/Diesel/battery types | COE and NPC | Remote locality | Bangladesh | The findings reveal that the discount rate and capital cost have a considerable impact on COE and NPC. |
Mandal et al [91] | PV/Wind/Diesel/Battery | COE, NPC, operational and Life Cycle Emission | Rural area (North) | Bangladesh | Solar micro utility systems, Wind/Batt/Diesel systems, and Diesel generator-only systems are all more expensive than the proposed solution. |
Javed et al. [92] | PV/Wind/battery | LPSP and COE | Isolated zones | China | After accounting for the various load needs, the effect of LPSP on COE stays the same. |
Ramli et al. [93] | PV/Wind | LCOE | Yanbu city | Saudi Arabia | All of these activities are aimed at lowering the cost of renewable energy supplies, particularly solar PV and wind energy. |
Maleki et al. [94] | PV/battery | LPSP and TLCC | Rural areas (East) | Iran | In terms of both power quality and cost, the suggested framework may be used efficiently for off-grid photovoltaic installations in rural locations. |
Hafeznia et al. [96] | PV | Birjand region | Iran | The fuzzy gamma operator was placed on the fuzzified maps to discover the best locations for grid-connected solar systems. | |
Maleki and Pourfayaz, [95] | PV/Wind/Diesel/battery, PV/Wind/Diesel/FC | Total Annual Cost | Remote area (Rafsanjan) | Iran | The standard PV/WG/diesel/battery system is more cost-effective than the PV/WG/diesel/FC system, according to the findings. |
Jeyaprabha and Selvakumar [97] | PV/Diesel/battery | LCC | Remote location | Iran | The validation of this sizing technique with LLP < 0.01 for various load demands demonstrates that the system sizing with a linear scale factor may be used for every site with any load requirement. |
Das et al. [98] | PV/Diesel/battery | COE and CO2 emission | Village (Sarawak) | Malaysia | The PV/Battery combination is both cost-effective and environmentally benign, according to the findings. |
Suresh et al.[99] | PV/Wind/BG/BM/FC/ battery, PV/Wind/BGG/BMG/FC, PV/Wind/BGG/BMG/battery, PV/Wind/BGG/BMG | NPC, COE and Operating cost | village hamlets | India | In comparison to the HOMER, the GA-based system has greater PV penetration and emits less CO2. |
Vendoti et al. [100] | PV/Wind/BMG/BGG/FC | NPC, COE and Operating cost | Villages | India | According to the findings, PV-wind-biomass-biogas-fuel cell-battery-based HRES) has the lowest NPC. |
Vendoti et al. [101] | PV/Wind/BGG/BMG/FC/battery, PV/Wind/BGG/BMG/FC, PV/Wind/BGG/BMG/ battery, PV/Wind/BGG/BMG | NPC, COE and Operating cost | Remote village | India | The cheapest and most reliable option has been established as a combination of PV–Wind–Biomass–Biogas–FC and batteries. |
Vendoti et al. [102] | PV/FC/battery | Efficiency, Cost energy density, and Power density | Isolated rural area | India | It is concerned with the modeling and simulation of a Hybrid Renewable Energy System for rural electrification. |
Vendoti et al. [103] | PV/Wind/Diesel/Battery | TNPC, Capital cost, COE | Rural village (Karnataka) | India | According to the sensitivity results, the proposed model (PV-Diesel) will provide a feasible solution for the research region. |
He et al. [104] | PV/Wind/battery | Initial cost, Replacement cost, Operating and maintenance cost | Subtropical monsoon community | China | The hybrid energy system has a good complementing feature, with higher PV power output in the spring and summer and more wind power output in the autumn and winter. |
Nowdeh and Hajibeigy [105] | PV/Wind/FC | COE | Jolfa | Iran | For reliable and cost-effective design, the rate of component availability must be taken into account. |
Rajanna and Saini [114] | MHG/BMG/BGG/PV/WTG/battery, MHG/BMG/BGG/WT/battery, MHG/BMG/BGG/PV/battery | TNPC and COE | Remote area | India | If biomass generators are used during time scheduling, the number of batteries and overall system cost can be lowered. |
Rajanna and Saini [108] | MHG/BMG/BGG/PV/WT/battery | TNPC and COE | Remote area | India | From HILR to LIHR scenarios, load density was shown to increase, increasing in system size and cost. |
Rajanna and Saini [106] | MHG/BMG/BGG/PV/WT | COE and OPF | Chamarajanagr (Karnataka) | India | It was discovered that IRES can give a viable solution for OPF values between 1.0 and 0.8. |
Kanase-Patil et al. [107] | MHP/BMG/BGG/PV, MHP/BMG/BGG/Wind/PV, MHP/BMG/BGG/energy plantation/PV, MHP/BMG/BGG/energy plantation/Wind/PV, | COE | Villages (Almora) | India | For variable biomass fuel prices, MHP/BMG/BGG/energy plantation/Wind/PV has been demonstrated to be more practical and sustainable than the other IRES systems. |
Dorji et al. [109] | PV/wind/Diesel/battery, PV/wind/ battery, PV/battery, Wind/battery | ICC, NPC and LUCE | - | Bhutan | The most cost-effective method was discovered to differ from one area to the next, depending on local renewable energy resources. |
Baek et al. [110] | PV/Wind/battery | Interest rate, NPC, COE and Project life | Island (Yeongjong) | South Korea | Combined cogeneration is cost-effective, although it emits a significant quantity of carbon dioxide per kWh. |
Niazi et al. [111] | PV/Wind | NPC, COE, and Operating cost | Nok Kundi and Ormara | Pakistan | The modeling of this hybrid system demonstrates that it is far superior to a single-source system. |
Tudu et al. [112] | PV/Wind/Battery/DG | NPC, COE and CO2 emission | Mangrove Forest | India | The upgraded version of the particle swarm optimization technique is capable of efficiently addressing this difficult non-linear optimization issue. |
Al-Shamma and Addoweesh [113] | PV/WT/Batt/DG, PV/Batt/DG, WT/Batt/DG, PV/WT/DG, WT/DG, DG, PV/WT/Batt, WT/Batt, PV/Batt | O & M and COE | Rural place | Saudi Arabia | The COE is not linearly reliant on the REF, as a little increase in the REF results in a large rise in the COE at high REF. |
Yuan et al. [115] | PV/Wood gas/battery | NPC, COE and CO2 emission | Rural house | China | The results revealed that the PV/WG/battery system with cycle charging mode had the lowest COE and NPC. |
Kirmani and Shadab [116] | PV/Wind/Diesel, PV/Grid, Wind/Grid, PV/Wind, and PV/Wind/Grid | Payback period, IRR, NPC, and COE | Selected village | India | In this analysis, the wind-grid connected system is the most matched hybrid system for the investigated instance, with the lowest energy and initial cost. |
Abujubbeh et al. [117] | PV/Wind | LCOE | Household (Amman) | Jordan | The hybrid system has a lower level of cost of electricity than the national grid tariff. |
Ramli et al.[118] | PV/Wind/Diesel/battery | COE and LPSP | West coastal area | Saudi Arabia | The hybrid system's energy costs are dominated by battery and wind turbine costs. |
Shezan and Ping [119] | PV-Diesel-Wind-battery | NPC, COE and CO2 emission | Offshore area | Bangladesh | The proposed hybrid system reduces CO2 emissions by approximately 1600 tons per year, indicating a major environmentally beneficial effort. |
Alharthi et al. [120] | PV-wind | NPC, COE and CO2 emission | Cities | Saudi Arabia | This study provides an overview of Saudi Arabia's current power generation status as well as a predicted growth in electricity consumption over the next 15 years. |
Al-Asfar et al. [121] | PV/Wind/Diesel/battery, PV/Wind/battery, Diesel |
NPC and COE | University | Jordan | In the scenario of maximum solar radiation and wind speed, the PV/Wind/Diesel/battery system had the lowest NPC and electricity cost. |
Tendo and Sirisamphanwong [122] | PV/Diesel | COE | University | Thailand | The primary aim was to design a PV/Diesel system of an optimal size that could meet load demand at the lowest possible cost. |
Rehman and El-Amin [123] | PV/Wind/Diesel, Wind/Diesel. PV/Diesel | COE | Remote Community (North-East) | Saudi Arabia | Among the hybrid power systems investigated in this scenario, PV-diesel was shown to be the most cost-effective. |
Pradhan et al. [124] | PV/Wind/Diesel/batteries | UCOE | Remote village (Khurda) | India | Presently, the best solution for stand-alone applications is a PV/wind/diesel/battery combination. |
Aziz [125] | PV/Wind/battery/FC | NPC, COE | Safari camp | UAE | The maximum electricity generated by the PV shows that it is more viable at the site than wind turbines. |
Al Ghaithi et al. [126] | Diesel, PV/Diesel, PV/Diesel/Wind | NPC, Operating cost, COE | Island (Masirah) | Oman | The results demonstrate that PV/Diesel/Wind is the most cost-effective alternative because it has the lowest NPC, OC, and COE for the system. |
Lao and Chungpaibulpatana [127] | Diesel, Diesel/PV, Diesel/PV/battery | Initial Capital cost, TNPC, and COE | Prasat Sambour | Cambodia | The best approach is a combination of diesel and PV with a battery, according to the findings. |
Mohamed et al. [128] | PV/Wind/Diesel/batteries | LCOE | Remote areas | Saudi Arabia | The purpose of the optimization was to reduce system costs while ensuring load demand and meeting a set of optimization constraints. |
Ahmad et al. [129] | Wind/PV/BMG | NPC and COE | Rural valley (Kallae Kahar) | Pakistan | When compared to an off-grid hybrid system with similar load patterns, the COE of a grid-connected hybrid system is lower. |
Ayadi and Alsalhen [130] | CSP/Wind | LCOE | - | Jordan | When wind and CSP energy sources are combined, the entire LCOE is around 0.045 JOD/kWh. |
Mudgal et al. [131] | PV/Wind/BGG | NPC and COE | Office building (Madras) | India | The results reveal that the integrated system is extremely sensitive to changes in component prices. |
Olamaei and Ghazvini [132] | PV/Battery/Diesel | Total cost | Parking lot | Iran | The best sizing for a PV/battery/diesel system was solved using a heuristic optimization technique that took into account the vehicle-to-grid parking lot as a controlled load. |
A new heuristic stochastic approach to optimizing the power supply of independent (off-grid) hybrid systems (PV-diesel-wind with battery storage) was illustrated by Dufo-Lopez et al. [133] in a study. The aim is the reduction of the net present expense of the device. Stochastic optimization is developed through the Monte Carlo simulation. It uses the variance-covariance matrix and probability density functions to take account of the uncertainties of wind speed, temperature, irradiation and load (correlated Gaussian random variables). Also considered was the uncertainty regarding the rate of inflation of diesel fuel prices. The heuristic approach utilizes a neural net to determine the optimal power system in a practical computational time (or a solution close to the optimal). A specific weighted all-throughput charging system with many control variables involves this approach, which can be installed in state-of-the-art chargers/inverters or battery controllers. As an example of applying this scientific method, a research paper is analyzed to achieve an optimal system close to that derived by deterministic optimization for stochastic optimization. It is advised to first carry out deterministic optimization (with minimal computation time), reduce the solution space and ultimately achieve stochastic optimization in a practicable computation time.
A responsive metamodel architecture and a C++ software implementation for the grassroots development of HRES were proposed by Roth et al. [134]. To find the optimal size of HRES, the metamodel allows one to create an optimization problem configured as a MILP with a customized objective function. The metamodel's flexibility depends on the ability to handle various variations of hybrid systems for 3 kinds of HRES applications, either for on-site demand, remote demand, or a combination. 2 distinct case studies, a stand-alone HRES composed of PV panels, battery array, and a diesel generator, and a tropical island plant, demonstrated this.
Ekren and Ekren [135] conducted a SA algorithm to enhance the size of a hybrid battery storage photovoltaic/wind integrated energy system. The proposed technique is a heuristic approach that uses a quest for the stochastic gradient to optimize the universe. The purpose is to decrease the total cost of the hybrid energy system. The range of output is PV scale, the region swept with the rotor of the wind turbine and the power of the battery. The findings of our initial findings are compared to the best results of the SA algorithm. The SA algorithm, therefore, runs better than the RSM. A study for the Turkish university area is being performed.
To minimize the high costs of using the standalone diesel system and achieve substantial fuel savings, Dursun et al. [136] have been looking at the possibility to supply electricity from solar/wind hybrid systems to isolated places without electricity in Edirne, the westernmost province of Turkey. The study uses HOMER's NREL program to conduct the technology-economic viability of such combined systems with solar data and wind data in mind. In addition, the impact of solar and wind on the generation of electricity, energy costs and total operating hours of the diesel generator is being examined for optimal hybrid systems. Furthermore, saving of fuel and reductions in carbon emissions from various hybrid systems are being examined. Undoubtedly, the suitability of using a hybrid solar-wind power system over a stand-alone diesel system is mainly discussed based on various global solar irradiances, wind speed and diesel efficiency.
Balikesir's solar radiation data in Turkey were examined in a study by Akyuz et al. [137] to assess the technology-economic feasibility and environmental efficiency of a combined photovoltaic-diesel-battery device to meet the load demands of a typical remote farmhouse. Via COE, diesel generator running hours, unfulfilled load, surplus electricity generation, fuel savings percentage, and so on, many aspects of the system are analyzed. For this form of the hybrid system, the COE is 1.245 USD per kW hr. Three instances are simulated (diesel-only, Photovoltaic-diesel, and Photovoltaic-diesel-battery). It is known that a diesel-only system produces 63,900 kW-hr of electricity and 69.7 tons of Carbon dioxide, 13.0 kg of PM and 1.53 tons of NOx emissions per annum. The use of photovoltaic-diesel and photovoltaic-diesel-battery systems helps minimize carbon dioxide emissions to 61.0 tons and 42.0 tons respectively, PM emissions to 11.4 and 7.83 kg and NOx emissions to 1.34 and 0.92. The diesel-only model is more economical if the fuel price stays below 2 USD-per-Litre. The cost-effectiveness of photovoltaic-diesel and photovoltaic-diesel-battery systems will otherwise be improved. For Photovoltaic-diesel and Photovoltaic-diesel-battery systems, the ecological impact improvement factor Eq 3 is also observed to be 0.127 and 0.399 for Carbon dioxide, 0.123 and 0.397 for PM and 0.124 and 0.398 for NOx, accordingly.
EIIF=(BCE−PSE)BCE | (3) |
where EIIF is the Environmental Impact Improvement Factor, BCE is Base-Case Emissions (here, it is the diesel system which is case 1) and PSE is the proposed system emission (PV-diesel and PV-diesel-battery as cases 2 and 3).
The viability of combined power generation systems utilizing different energy sources to fulfill the electricity demands of a residential population was examined by Mukherjee and Asthana [138]. The device's performance is calculated as capital expenditure, COE, emissions of Carbon dioxide and NPC. The findings demonstrate that, relative to the grid price, the mixed system decreases COE by 47 percent and has a negative Carbon dioxide emission of 24,603 kg per year (see Table 14) due to the supply of its excess energy to the grid. Renewable sources lead to 80.1 percent of the combined system's total power generated. The analysis found that total grid dependency could be replaced by hybrid systems.
Quality | Units | Hybrid system | Grid |
Carbon dioxide | kg/yr | –24,603 | 38,129 |
Sulfur dioxide | kg/yr | –107 | 165 |
Nitrogen oxides | kg/yr | –52, 2 | 80.8 |
Liu et al. [139] highlighted a self-service microgrid based on renewable energy in operating and economic optimisation. Due to the large size of the issue in time series models, the MILP model was developed. Because the restrictions needed in this model are typical of modest complexity, any non-linear variable relationship could be linearized, one by one. Furthermore, regulations have been implemented on the demand side. To reduce the overall level cost of electricity of a microgrid that involves a photovoltaic power source, a wind turbine, a diesel generator and an energy storage system, a two-stage MILP model was built here. The goal of the model was to focus on a combination of decision-making accuracy and computational effectiveness. With extra capacity control levers on the possible loss of power supply and load deferring allowance, model outputs were able to recognize both the optimum device size and planning for each network feature.
The optimal combination of technologies in an integrated system consisting of CST, solar PV, battery storage and diesel generators has been identified by Balaji and Gurgenci [140]. The optimisation is accomplished through an economic system that focuses on reducing the LCOE. The CST system considered here, which illustrates enhanced viability for small scales (less than 10 MW), uses a supercritical Carbon dioxide power loop. The performance and cost of the CST device are strongly related to the temperature at the inlet of the turbine. In this study, 3 temperatures are explored to find the correct condition. The findings shown in Table 15, indicate that the ideal systems are formed of CST (650 ℃) augmented by PV and diesel generation as the main source of baseload fuel. These solutions offer significant decreases in LCOE and emissions compared to the diesel base case and thus provide a successful remote-control solution.
Location | LCOE at 650 ℃ | LCOE at 800 ℃ | LCOE at 1000 ℃ |
Newman | 0.167 | 0.180 | 0.213 |
Port Augusta | 0.183 | 0.197 | 0.232 |
Halls Creek | 0.158 | 0.174 | 0.213 |
Lal and Raturi [141] explored the possibility of a wind/solar photovoltaic/diesel generator-powered combined power system in a distant area in the Fiji Islands. To model the program and to carry out system optimization analysis, HOMER software was used. In terms of optimal design, NPC and COE the machine characteristics were analyzed. If an annual capacity shortage of 10 percent is permitted, a completely renewable energy-based design is feasible, while a diesel generator is required for a situation with no capacity shortages. The introduction of renewable energy elements decreases greenhouse gas emissions and can be used in the exchange of carbon.
The overviews of the research in the European and Oceania continents are shown in Tables 16 and 17, respectively.
Author(s) | Hybrid System | Parameters | Location | Country | Remarks |
Dufo-Lopez et al. [133] | PV/Wind/Diesel/battery | NPC and LCOE | Navarra | Spain | The stochastic technique finds an optimal system that is remarkably close to the one identified by the deterministic approach. |
Roth et al. [134] | PV/Diesel/battery | TLCC | Tropical island | France | The metamodel utilized is versatile enough to solve a variety of problems, ranging from traditional on-site consumption to self-use with partial injection. |
Ekren and Ekren [135] | PV/Wind/battery | LLP | University | Turkey | The Simulated Annealing method outperforms the Response Surface Methodology, according to the findings. |
Dursum et al. [136] | PV/Wind/Diesel/battery | NPC and COE | Isolated place (Edirne) | Turkey | It has been demonstrated that a PV/wind/diesel system with batteries may be widely used in distant places to replace or upgrade existing stand-alone diesel systems. |
Mukherjee and Asthana [138] | PV/Wind | LCOE | Residential area | UK | The hybrid systems have the potential to completely replace grid dependency. |
Akyuz et al. [137] | PV/Diesel, PV/Diesel/battery | COE | Remote farmhouse | Turkey | The number of hours that diesel generators are operating for a particular hybrid system decreases as PV capacity increases. |
Author(s) | Hybrid System | Parameters | Location | Country | Remarks |
Liu et al. [139] | PV/Wind/Diesel/battery | LPSP | Isolated island | Australia | The possibility of maximizing the decrease of stand-alone renewable system costs with diesel and energy storage was investigated using a two-stage mixed-integer linear programming model. |
Balaji and Gurgenci [140] | CST/PV/Diesel/ Battery |
LCOE and CO2 emission | Remote locations | Australia | In comparison to the diesel base case, these systems have significantly lower LCOE and emissions, and so offer a feasible solution for remote electricity. |
Lal and Raturi [141] | PV/Wind/Diesel/battery | NPC, COE and CO2 emission | Island (Vanua Levu) | Fiji | If a 10% yearly capacity shortage is tolerated, a 100% renewable energy-based arrangement is possible. |
In this study, an overview of various renewable energy strategies done in developed and developing countries is implemented using different optimization methods, algorithms, and methodologies to arrive at the best renewable energy system setup and scheme. Criteria such as ICC ($), NPC/NPV/TNPC ($), Cost of Energy ($/kWh), RF (%) Operating cost ($/yr), and produced emissions (kg/yr) are estimated. In most of the strategies, a scheme with minimum NPC/NPV/TNPC and/or COE is selected; other criteria could be examined based on the investors' viewpoint. This issue is thoroughly covered in [142]. Research on renewable energy systems is understandably done in most developing countries, especially in rural and remote areas [143,144]. In developing countries such as Nigeria, research on renewable energy systems is mostly done as a necessity to complement the deficiency in power supply and not for its environmental advantages. Fuel price, solar radiation, and wind velocity are the most uncertain constraints on renewable energy system implementation. Constraints such as these are a very important consideration in a country such as Nigeria whose economy is very dependent on fossil fuels and regions of the country such as the Niger Delta and the Northeast where weather are mainly windy and sunny dependant respectively. Many researchers considered PV as the desired resource and more research on HRES was modelled in stand-alone than grid-connected.
The world may likely face a world energy crisis as a result of a drop in the abundance of cheap oil and suggestions to reduce reliance on fossil fuels. This has led to increasing interest in alternate power/fuel research such as wind, fuel cell technology, biodiesel, hydrogen fuel, tidal energy, solar energy, and geothermal energy. After a thorough review, it can be concluded that the hybrid combination seemed to be the most economical solution and the most reliable option for power generation electricity. The hybrid systems were also seen as the best for the environment as it reduces the quantity of carbon dioxide compared to the standalone diesel generators. The results showed that even with high initial costs, there was long-term profit and several advantages of using hybrid renewable energy systems compared to standalone systems because hybrid systems have lower operating and maintenance costs compared to the standalone options. The hybrid systems have so far produced the lowest cost of generating electricity. The hybrid systems were also seen as the best for the environment as it reduces the quantity of carbon dioxide compared to the standalone diesel generators.
The authors declare that there are no known conflicts of interest related to this study that can hinder its publication.
[1] |
Abdelmoula M, Etienne JM (2010) Determination of R & D investment in French firms: a two-part hierarchical model with correlated random effects. Econ Innov New Technol 19: 53–70. https://doi.org/10.1080/10438590903016435 doi: 10.1080/10438590903016435
![]() |
[2] |
Aghasafari H, Aminizadeh M, Karbasi A, et al. (2021) CO2 emissions, export and foreign direct investment: empirical evidence from Middle East and North Africa region. J Int Trade Econ Dev 30: 1054–1076. https://doi.org/10.1080/09638199.2021.1934087 doi: 10.1080/09638199.2021.1934087
![]() |
[3] |
Aguinis H, Gottfredson RK, Culpepper SA (2013) Best-practice recommendations for estimating cross-level interaction effects using multilevel modeling. J Manage 39: 1490–1528. https://doi.org/10.1177/0149206313478188 doi: 10.1177/0149206313478188
![]() |
[4] |
Alsan M, Bloom DE, Canning D (2006) The effect of population health on foreign direct investment inflows to low- and middle-income countries. World Dev 34: 613–630. https://doi.org/10.1016/j.worlddev.2005.09.00 doi: 10.1016/j.worlddev.2005.09.00
![]() |
[5] |
Andersson S, Svensson G, Molina-Castillo F, et al. (2022) Sustainable development—Direct and indirect effects between economic, social, and environmental dimensions in business practices. Corp Soc Responsib Environ Manag 29: 1158–1172. https://doi.org/10.1002/csr.2261 doi: 10.1002/csr.2261
![]() |
[6] | Brundtland G (1987) Our common future: The world commission on environment and development, Oxford, England: Oxford University Press. |
[7] |
Burger M, Meijers E (2012) Form follows function? Linking morphological and functional polycentricity. Urban Stud 49: 1127–1149. https://doi.org/10.1177/0042098011407095 doi: 10.1177/0042098011407095
![]() |
[8] |
Cheng Z, Li L, Liu J (2018) The spatial correlation and interaction between environmental regulation and foreign direct investment. J Regul Econ 54: 124–146. https://doi.org/10.1007/s11149-018-9366-x doi: 10.1007/s11149-018-9366-x
![]() |
[9] |
Cheng Z, Li L, Liu J (2020) The impact of foreign direct investment on urban PM2.5 pollution in China. J Environ Manage 265: 110532. https://doi.org/10.1016/j.jenvman.2020.110532 doi: 10.1016/j.jenvman.2020.110532
![]() |
[10] |
Cicea C, Marinescu C (2020) Bibliometric analysis of foreign direct investment and economic growth relationship. A research agenda. J Bus Econ Manag 22: 445–466. https://doi.org/10.3846/jbem.2020.14018 doi: 10.3846/jbem.2020.14018
![]() |
[11] | Elkington J (1997) Cannibals with forks – Triple bottom line of 21st century business. Stoney Creek, CT: New Society Publishers. |
[12] | Garson D (2013) Hierarchical linear modeling: guide and applications, New York: Sage Publications. |
[13] | Goldstein H (2010) Multilevel statistical models, 4th ed. Hoboken: Wiley. |
[14] |
Huta V (2014) When to use hierarchical linear modeling. Quant Meth Psychol 10: 13–28. https://doi.org/10.20982/tqmp.10.1.p013 doi: 10.20982/tqmp.10.1.p013
![]() |
[15] |
Kamenju AK, Olweny DrT (2021) The nexus between internal investment and economic growth in Kenya. J Appl Financ Bank 11: 29–93. https://doi.org/10.47260/jafb/1122 doi: 10.47260/jafb/1122
![]() |
[16] |
Kaneko Y, Nakagawa T, Phun VK, et al. (2019) Impacts of urban railway investment on regional economies: evidence from Tokyo using spatial difference-in-differences analysis. Transp Res Rec 2673: 129–140. https://doi.org/10.1177/0361198119846098 doi: 10.1177/0361198119846098
![]() |
[17] |
Kashefi-Pour E, Amini S, Uddin M, et al. (2020) Does Cultural Difference Affect Investment—Cash Flow Sensitivity? Evidence from OECD Countries. Br J Manag 31: 636–658. https://doi.org/10.1111/1467-8551.12394 doi: 10.1111/1467-8551.12394
![]() |
[18] |
Kleineick J, Ascani A, Smit M (2020) Multinational investments across Europe: a multilevel analysis. Rev Reg Res 40: 67–105. https://doi.org/10.1007/s10037-020-00139-2 doi: 10.1007/s10037-020-00139-2
![]() |
[19] |
Krasnoselskaya D, Timiryanova V (2022a) Do polycentric patterns influence the economic performance of municipalities? Empirical evidence from Russia. Reg Sci Policy Pract 14: 1201–1222. https://doi.org/10.1111/rsp3.12515 doi: 10.1111/rsp3.12515
![]() |
[20] |
Krasnoselskaya D, Timiryanova V (2022b) Estimate of settlement systems polycentricity: sensitivity to methods, number of observations and a level of data aggregation. Vestnik Magnitogorskogo Gosudarstvennogo Tekhnicheskogo Universiteta im. G.I. Nosova[Vestnik of Nosov Magnitogorsk State Technical University] 20: 144–153. https://doi.org/10.18503/1995-2732-2022-20-4-144-153 doi: 10.18503/1995-2732-2022-20-4-144-153
![]() |
[21] |
Ledyaeva S (2009) Spatial econometric analysis of foreign direct investment determinants in Russian regions. World Econ 32: 643–666. https://doi.org/10.1111/j.1467-9701.2008.01145.x doi: 10.1111/j.1467-9701.2008.01145.x
![]() |
[22] |
Liu X, Yu L, Zhang Y, et al. (2020) Spatial spillover effect of financial flexibility on investment in China's convention and exhibition listed companies. Math Probl Eng 3926747: 1–10. https://doi.org/10.1155/2020/3926747 doi: 10.1155/2020/3926747
![]() |
[23] |
Martini A (2021) Socially responsible investing: from the ethical origins to the sustainable development framework of the European Union. Environ Dev Sustain 23: 16874–16890. https://doi.org/10.1007/s10668-021-01375-3 doi: 10.1007/s10668-021-01375-3
![]() |
[24] |
Moran P (1948) The Interpretation of Statistical Maps. J R Stat Soc 10: 243–251. https://doi.org/10.1111/j.2517-6161.1948.tb00012.x doi: 10.1111/j.2517-6161.1948.tb00012.x
![]() |
[25] |
Ndikumana L (2000) Financial determinants of domestic investment in Sub-Saharan Africa: evidence from panel data. World Dev 28: 381–400. https://doi.org/10.1016/s0305-750x(99)00129-1 doi: 10.1016/s0305-750x(99)00129-1
![]() |
[26] |
Nogueira E, Gomes S, Lopes JM (2022) The key to sustainable economic development: a triple bottom line approach. Resources 11: 46. https://doi.org/10.3390/resources11050046 doi: 10.3390/resources11050046
![]() |
[27] |
Ofosu-Mensah Ababio J, Sarpong-Kumankoma E, Osei KA (2018) Financing cost and private investment in Ghana. Adv Econ Bus 6: 99–113. https://doi.org/10.13189/aeb.2018.060203 doi: 10.13189/aeb.2018.060203
![]() |
[28] |
Okuma K (2019) Potential mechanisms for the social regulation of economies on global and local scales: an institutional analysis of ESG investment and community renewables. Evolut Inst Econ Rev 16: 523–541. https://doi.org/10.1007/s40844-019-00133-5 doi: 10.1007/s40844-019-00133-5
![]() |
[29] |
Pain K, Shi Sh, Black D, et al. (2023) Real estate investment and urban density: exploring the polycentric urban region using a topological lens. Territ Politic Gov 11: 241–260. https://doi.org/10.1080/21622671.2020.1837665 doi: 10.1080/21622671.2020.1837665
![]() |
[30] |
Perovic-Randelovic M, Jankovic-Milic V, Kostadinovic I (2017) Market size as a determinant of the foreign direct investment inflows in the Western Balkans Countries. Facta Univ Ser Econ Organ 14: 93–104. https://doi.org/10.22190/FUEO1702093P doi: 10.22190/FUEO1702093P
![]() |
[31] | Raudenbush SW, Bryk AS, Cheong YF, et al. (2011) HLM 7: Hierarchical Linear and Nonlinear Modeling. Scientific Software International, Inc.: Linconwood, IL, USA. |
[32] |
Rossi MCT, Santos GF, Campos FAS (2016) Foreign direct investment and spatial linkages: third-country effects for Brazilian outward investment. Lat Am Bus Rev 17: 1–25. https://doi.org/10.1080/10978526.2015.1137460 doi: 10.1080/10978526.2015.1137460
![]() |
[33] |
Schweikert A, Espinet X, Chinowsky P (2018) The triple bottom line: bringing a sustainability framework to prioritize climate change investments for infrastructure planning. Sustain Sci 13: 377–391. https://doi.org/10.1007/s11625-017-0431-7 doi: 10.1007/s11625-017-0431-7
![]() |
[34] |
Stundziene A, Baliute A (2022) Link between tangible investment rate and labour productivity in the European manufacturing industry. Panoeconomicus 69: 609–633. https://doi.org/10.2298/PAN171208012S doi: 10.2298/PAN171208012S
![]() |
[35] |
Tafirenyika S (2023) The impact of foreign direct investment on Namibia's economic growth: A time series investigation. Cogent Econ Finance 11: 1. https://doi.org/10.1080/23322039.2023.2210857 doi: 10.1080/23322039.2023.2210857
![]() |
[36] |
Yang Y, Niu G, Tang D, et al. (2019) Does environmental regulation affect the introduction of foreign direct investment in China? — Empirical Research Based on the Spatial Durbin Model. Pol J Environ Stud 28: 415–424. https://doi.org/10.15244/pjoes/83692. doi: 10.15244/pjoes/83692
![]() |
[37] |
Zhang L, Zhang Z, Bieryt K, et al. (2023) Sustainable green financial system perspective of environmental protection investment and the government's environmental policy or public participation: evidence from Chinese A-share listed companies. Econ Res-Ekon Istraž 36: 2. https://doi.org/10.1080/1331677X.2023.2178019 doi: 10.1080/1331677X.2023.2178019
![]() |
[38] |
Zubarevich N (2019) Inequalities of regions and large cities of Russia: what was changed in the 2010? Obshchestvennye Nauki I Sovremennost 4: 57–70. https://doi.org/10.31857/S086904990005814-7 doi: 10.31857/S086904990005814-7
![]() |
![]() |
![]() |
1. | Mamdouh El Haj Assad, Siamak Hoseinzadeh, Editorial to the 'Special Issue-Analyzing energy storage systems for the applications of renewable energy sources' of AIMS Energy, 2022, 10, 2333-8334, 1074, 10.3934/energy.2022050 | |
2. | Z. Ismaila, O. A. Falode, C. J. Diji, R. A. Kazeem, O. M. Ikumapayi, M. O. Petinrin, A. A. Awonusi, S. O. Adejuwon, T-C. Jen, S. A. Akinlabi, E. T. Akinlabi, Evaluation of a hybrid solar power system as a potential replacement for urban residential and medical economic activity areas in southern Nigeria, 2023, 11, 2333-8334, 319, 10.3934/energy.2023017 | |
3. | Tarek I. Alanazi, Abdulaziz Alanazi, Ezzeddine Touti, Ahmed M. Agwa, Habib Kraiem, Mohana Alanazi, Abdulrahman M. Alanazi, Mona El Sabbagh, Proposal and Numerical Analysis of Organic/Sb2Se3 All-Thin-Film Tandem Solar Cell, 2023, 15, 2073-4360, 2578, 10.3390/polym15112578 | |
4. | Omolayo M. Ikumapayi, Opeyeolu T. Laseinde, Tin T. Ting, Sunday A. Afolalu, Adedotun O. Adetunla, Temitayo S. Ogedengbe, 2024, A Study on the Utilization of Green Hydrogen Energy - An Overview, 979-8-3503-5815-5, 1, 10.1109/SEB4SDG60871.2024.10630136 | |
5. | Oluwasina L. Rominiyi, Omolayo M. Ikumapayi, Johnson F. Eiche, Adeyemi W. Oke, Abdussami Giwa, Ugwushichika Alabo, 2024, Design and Construction of a Prototype Hybrid Solar-Wind Power System, 979-8-3503-5815-5, 1, 10.1109/SEB4SDG60871.2024.10630159 | |
6. | Yuqing Geng, Qinjun Xiang, Juan Gao, Yan Yan, Jianyi Li, Progress and framework of clean energy production: Bibliometric analysis from 2002 to 2022, 2024, 52, 2211467X, 101270, 10.1016/j.esr.2023.101270 | |
7. | Edward Dodzi Amekah, Emmanuel Wendsongre Ramde, David Ato Quansah, Elvis Twumasi, Stefanie Meilinger, Schneiders Thorsten, Analyzing the consequences of power factor degradation in grid-connected solar photovoltaic systems, 2024, 9, 27726711, 100715, 10.1016/j.prime.2024.100715 | |
8. | Edward Dodzi Amekah, Emmanuel Wendsongre Ramde, David Ato Quansah, Elvis Twumasi, Stefanie Meilinger, Thorsten Schneiders, Optimal Placement and Upgrade of Solar PV Integration in a Grid-Connected Solar Photovoltaic System., 2024, 27729400, 100099, 10.1016/j.solcom.2024.100099 | |
9. | Omolayo M. Ikumapayi, Temitayo S. Ogedengbe, Charles O. Ogunnigbo, Boluwaji G. Jaiyesimi, Abiodun Bayode, Opeyemi O. Olaitan, Remilekun R. Elewa, 2024, Bioenergy-A Review on Biofuels and Biomass Conversion, 979-8-3315-4255-9, 1, 10.1109/NIGERCON62786.2024.10927219 |
Components | Grey wolf optimizer | Particle swarm optimization |
PV panels | 33 | 38 |
Battery banks | 90 | 90 |
Diesel generators | 2 | 3 |
Annual capital cost ($) | 10519.38 | 10214.95 |
Annual operation and management cost ($) | 170.46 | 160.81 |
Annual replacement cost ($) | 5638.5 | 6049 |
Annual cost system ($) | 16825.64 | 16872.66 |
Annual fuel cost ($) | 497.3 | 447.9 |
Configurations | PV-Battery | PV-Diesel-Battery | PV-Wind-Battery | PV-Wind-Diesel-Battery |
PV (kW) | 4 | 4 | 4 | 4 |
Wind turbine (kW) | - | - | 1 | 1 |
Gen-set (kW) | - | 10 | - | 10 |
Battery | 16 | 16 | 16 | 16 |
Converter (kW) | 5 | 5 | 5 | 5 |
NPC ($) | 18,161 | 21,671 | 26,824 | 29,225 |
COE ($) | 0.233 | 0.278 | 0.344 | 0.375 |
Initial cost ($) | 15,580 | 20,580 | 22,080 | 22,080 |
Operating cost | 163.87 | 69.23 | 301.15 | 517.04 |
Global solar (kWh/m2/d) | Total NPC ($) | Operating cost ($/yr.) | COE ($/kWh) | Ren fraction |
4.76 (–20%) | 83,915 | 1,263 | 0.355 | 0.84 |
5.95 | 74,836 | 2,584 | 0.316 | 0.96 |
7.14 (+20%) | 71,443 | 769 | 0.302 | 1 |
Diesel price ($/L) | Total NPC ($) | Operating cost ($/yr.) | COE $/kWh | Ren fraction |
0.52 (–20%) | 74,196 | 1,263 | 0.314 | 0.96 |
0.65 | 74,836 | 1,170 | 0.316 | 0.96 |
0.78 (+20%) | 75,477 | 1,356 | 0.319 | 0.96 |
Optimization methods | Solar-Wind hybrid energy system | Conventional power station |
NPC/year ($) | 198,347.984 | 198,348.00 |
COE/year (kt) | 288,194.00 | 297,000.00 |
Author(s) | Hybrid System | Parameters | Location | Country | Remarks |
Yahiaoui et al. [60] | PV/Diesel/Battery | ACC, ARC, AFC, AOM | Remote village (Djanet) | Algeria | GWO offers higher capabilities, reduced costs, and faster convergence. |
Muh and Tabet [61] | PV/Hydro/Diesel/Battery | COE | Offshore | Cameroon | Southern Cameroons' most viable economic system. |
Fodhil et al. [62] | PV/Diesel/Battery | COE, RF | Small village | Algeria | The PSO-based strategy is less expensive and has a higher PV penetration than HOMER. |
Diemuodeke et al. [63] | Diesel/PV/Wind/Battery PV/Wind/Battery |
CO2 emission, Payback time, COE | South-South region | Nigeria | For renewable energy specialists, the methodology offered serves as an ideal design tool. |
Djelailia et al. [64] | PV/Diesel/ Power Storage Hydroelectric | Fuel Consumption, CO2 emission | Remote site (Adrar) | Algeria | The idea demonstrates the utility of pump storage hydropower in irrigation and power recovery. |
Kaabeche and Ibtiouen [65] | PV/wind/diesel/battery | TNPC, COE, CO2 emission | Ghardaïa | Algeria | When compared to a PV/wind/battery system or a diesel generator (DG), the PV/wind/diesel/battery option is more cost-effective. |
Babatunde et al. [66] | PV/diesel/battery system | NPC, RF, COE | Rural health centers | Nigeria | In terms of the parameters, a PV/diesel/battery system is the most cost-effective. |
Samy et al. [67] | PV/FC | TNPV and LCOE | Remote urban areas | Egypt | Among the other methods, the FPA Algorithm has the shortest fulfillment time and the best rendering. |
Ogunjuyigbe and Ayodele [69] | PV/Diesel/Battery | NPC, COE, CO2, and CO emission | Telecom company | Nigeria | Out of the five economically viable configurations, PV/Diesel/Battery provides the best cost-benefit ratio. |
Tijani et al. [70] | PV/ Diesel/ Battery | NPC, COE, Operation, and Initial cost | Remote location (north) | Nigeria | The suggested system has a high energy potential and reduced carbon emissions while maintaining an affordable electricity cost. |
Samy [68] | PV/wind/battery, PV/FC, wind/FC, PV/wind/FC, and PV/wind/battery/FC | NPC, COE, Initial capital | Monshaet Taher Village | Egypt | A cost-effective hybrid renewable energy system was created to fulfill the known electric load of a small town in Egypt. |
Salisu et al. [71] | PV/Wind/Battery/Diesel | NPC, COE | Moriki village | Nigeria | The results also revealed a spectacular reduction in greenhouse gas (GHG) emissions of 100 percent because the ideal arrangement contains a 100 percent renewable portion. |
Rao et al, [72] | PV/Wind/Conventional Electric generator | NPC, COE, and CO2 emission | Deleta village | Ethiopia | In terms of economics and the environment, the hybrid design is the best ideal hybrid configuration. |
Babatunde and Ighravwe [73] | PV/Wind/battery, PV/battery, Diesel/battery, Wind/Diesel/battery, PV/Wind/Diesel/battery, PV/Diesel/battery | NPC, RF, COE, Operating cost | Rural community (Abadam) | Nigeria | Diesel/batteries were the best HRES for the case study from an economic standpoint, while wind/diesel generator/batteries were the best HRES from a technological and techno-economic standpoint. |
Sanni et al [74] | PV/Diesel | NPC, COE, Operating cost, RF | Kadawa village | Nigeria | The findings demonstrate that using renewable energy in agricultural activities can help achieve food security. |
Olatomiwa et al. [75] | PV/Diesel/battery and PV/Wind/Diesel/battery | NPC, COE, and CO2 emission | Remote mobile BTS | Nigeria | When compared to a stand-alone diesel generator system, the hybrid PV–diesel–battery system gives a greater reduction in CO2 emissions each year. |
Okundamiya et al. [76] | Wind/battery | COE, PSR and performance index | Typical residential region (Sokoto) | Nigeria | The proposed power system might save money and increase electricity reliability, according to the findings. |
Allali et al. [77] | Wind/Diesel/battery | - | Remote site (south) | Algeria | This system features a sophisticated control technique for managing several power sources (wind, diesel, and battery) that is dependent on weather circumstances, particularly wind speed values. |
Author(s) | Hybrid system | Parameters | Location | Country | Remarks |
Weis and Ilinca [78] | Wind/Diesel | Rural communities | Canada | Wind turbines are only acceptable for use in distant Canadian towns when the average yearly wind speed is at least 6.0 m/s. | |
Osaretin et al. [79] | PV/Wind/battery | NPC, LCOE, and Operating cost | Remote oil and gas facility | Canada | Continuous pumping using a hybrid renewable energy system with solar, wind, and battery storage has been identified as the best practicable approach based on economic and technological benefits. |
Ciez and Whitacre [80] | PV/Diesel/battery | LCOE | - | USA | In some circumstances, studies demonstrate that storage packs with high-energy, low-cost lithium-ion cells can compete with a non-renewable alternative. |
Optimization methods | GA | HOMER |
Total NPC ($) | 856013 | 890013 |
COE ($/kWh) | 0.163 | 0.214 |
Total production (kWh/yr) | 3,36,543 | 3,49,493 |
Total consumption (kWh/yr) | 3,16,428 | 3,28,266 |
CO2 emissions (kg/yr) | 3842 | 4089 |
Configurations | NPC ($) | COE ($/kWh) | Operating Cost ($) |
C1 (PV/Wind/Biomass/Biogas/FC and Battery) | 890,013 | 0.214 | 34,109 |
C2 (PV/Wind/Biomass/Biogas/FC without Battery) | 897,847 | 0.215 | 36,917 |
C3 (PV/Wind/Biomass/Biogas/Battery without FC) | 929,878 | 0.223 | 29,354 |
C4 (PV/Wind/Biomass/Biogas without storage) | 1,095,020 | 0.263 | 52,148 |
Components | Index |
Wind (numbers of turbines) | 11 |
PV (kW) | 357 |
Converter (kW) | 57 |
Battery (numbers of Battery) | 825 |
Grid (kW) | 0 |
Operating cost ($ per year) | 76,081 |
Initial capital ($) | 1,861,625 |
Total NPC ($) | 3,506,509 |
COE ($/kWh) | 0.545 |
Parameter | Value |
LCOE ($/kWh) | 0.159 |
NPV ($) | 24,618 |
IRR (%) | 16.3 |
PBP (years) | 6.4 |
Annual life cycle saving ($/year) | 788 |
System Configuration | Cost Component | Value | Unit |
PV-Biomass-Wind-Grid | Total NPC | 180,290,247.40 | $ |
LCOE | 0.05744 | $/kWh | |
PV-Biomass-Grid | Total NPC | 178,790,678.90 | $ |
LCOE | 0.0614 | $/kWh |
Author(s) | Hybrid System | Parameters | Location | Country | Remarks |
Shezan et al. [81] | PV/Wind/Diesel/battery | NPC and COE | Remote region | Malaysia | The simulation ensures that the system is financially and environmentally feasible in terms of net present cost (NPC) and CO2 emission reduction. |
Cai et al. [82] | PV/battery/Diesel | TLCC, LCOE, operating and maintenance cost | South Khorasan | Iran | The results reveal that combining solar energy with a diesel generator cuts greenhouse gas emissions and supply costs significantly when compared to a diesel-only system. |
Baneshi and Hadianfard [83] | PV/Wind/battery | COE | Shiraz | Iran | Carbon taxes could be an effective policy for encouraging large-scale renewable energy installations. |
Maleki et al. [84] | PV/Diesel/Battery | LCC | Rural townhouse | Iran | The findings suggest that Norouzak fuel is a viable alternative fuel-hybrid system choice. |
Hossain et al. [85] | PV/Wind/Diesel/battery | NPC and COE | Large resort sites (12 islands) | Malaysia | In the settings, PV, wind, diesel generator, converter, and battery make up the greatest stand-alone hybrid energy system. |
Wu et al. [86] | PV/Diesel/battery/Reverse Osmosis Desalination | LCC and LCOE | Remote area (Khorasan) | Iran | PV/diesel/battery/ROD is more cost-effective and environmentally friendly than a single diesel or PV system. |
Maleki [87] | PV/Wind/FC/Diesel, PV/diesel/FC, WT/diesel/FC | Total Annual Cost and emission | Remote location | Iran | The PV/WT/diesel/FC system is the best option in terms of pollutant emissions. |
Rodríguez-Gallegos et al. [88] | PV/battery/Diesel | LCOE and CO2 emission | Island | Indonesia | The findings demonstrate the cost-effectiveness, reduction of cumulative emissions, and even improvement of grid quality of PV hybrid systems in remote places. |
Rodríguez-Gallegos et al. [89] | PV/battery/Diesel | LCC | Island | Indonesia | An investigation was conducted to develop a method for successfully hybridizing DG-only systems with solar panels and batteries. |
Das and Zaman [90] | PV/Diesel/battery types | COE and NPC | Remote locality | Bangladesh | The findings reveal that the discount rate and capital cost have a considerable impact on COE and NPC. |
Mandal et al [91] | PV/Wind/Diesel/Battery | COE, NPC, operational and Life Cycle Emission | Rural area (North) | Bangladesh | Solar micro utility systems, Wind/Batt/Diesel systems, and Diesel generator-only systems are all more expensive than the proposed solution. |
Javed et al. [92] | PV/Wind/battery | LPSP and COE | Isolated zones | China | After accounting for the various load needs, the effect of LPSP on COE stays the same. |
Ramli et al. [93] | PV/Wind | LCOE | Yanbu city | Saudi Arabia | All of these activities are aimed at lowering the cost of renewable energy supplies, particularly solar PV and wind energy. |
Maleki et al. [94] | PV/battery | LPSP and TLCC | Rural areas (East) | Iran | In terms of both power quality and cost, the suggested framework may be used efficiently for off-grid photovoltaic installations in rural locations. |
Hafeznia et al. [96] | PV | Birjand region | Iran | The fuzzy gamma operator was placed on the fuzzified maps to discover the best locations for grid-connected solar systems. | |
Maleki and Pourfayaz, [95] | PV/Wind/Diesel/battery, PV/Wind/Diesel/FC | Total Annual Cost | Remote area (Rafsanjan) | Iran | The standard PV/WG/diesel/battery system is more cost-effective than the PV/WG/diesel/FC system, according to the findings. |
Jeyaprabha and Selvakumar [97] | PV/Diesel/battery | LCC | Remote location | Iran | The validation of this sizing technique with LLP < 0.01 for various load demands demonstrates that the system sizing with a linear scale factor may be used for every site with any load requirement. |
Das et al. [98] | PV/Diesel/battery | COE and CO2 emission | Village (Sarawak) | Malaysia | The PV/Battery combination is both cost-effective and environmentally benign, according to the findings. |
Suresh et al.[99] | PV/Wind/BG/BM/FC/ battery, PV/Wind/BGG/BMG/FC, PV/Wind/BGG/BMG/battery, PV/Wind/BGG/BMG | NPC, COE and Operating cost | village hamlets | India | In comparison to the HOMER, the GA-based system has greater PV penetration and emits less CO2. |
Vendoti et al. [100] | PV/Wind/BMG/BGG/FC | NPC, COE and Operating cost | Villages | India | According to the findings, PV-wind-biomass-biogas-fuel cell-battery-based HRES) has the lowest NPC. |
Vendoti et al. [101] | PV/Wind/BGG/BMG/FC/battery, PV/Wind/BGG/BMG/FC, PV/Wind/BGG/BMG/ battery, PV/Wind/BGG/BMG | NPC, COE and Operating cost | Remote village | India | The cheapest and most reliable option has been established as a combination of PV–Wind–Biomass–Biogas–FC and batteries. |
Vendoti et al. [102] | PV/FC/battery | Efficiency, Cost energy density, and Power density | Isolated rural area | India | It is concerned with the modeling and simulation of a Hybrid Renewable Energy System for rural electrification. |
Vendoti et al. [103] | PV/Wind/Diesel/Battery | TNPC, Capital cost, COE | Rural village (Karnataka) | India | According to the sensitivity results, the proposed model (PV-Diesel) will provide a feasible solution for the research region. |
He et al. [104] | PV/Wind/battery | Initial cost, Replacement cost, Operating and maintenance cost | Subtropical monsoon community | China | The hybrid energy system has a good complementing feature, with higher PV power output in the spring and summer and more wind power output in the autumn and winter. |
Nowdeh and Hajibeigy [105] | PV/Wind/FC | COE | Jolfa | Iran | For reliable and cost-effective design, the rate of component availability must be taken into account. |
Rajanna and Saini [114] | MHG/BMG/BGG/PV/WTG/battery, MHG/BMG/BGG/WT/battery, MHG/BMG/BGG/PV/battery | TNPC and COE | Remote area | India | If biomass generators are used during time scheduling, the number of batteries and overall system cost can be lowered. |
Rajanna and Saini [108] | MHG/BMG/BGG/PV/WT/battery | TNPC and COE | Remote area | India | From HILR to LIHR scenarios, load density was shown to increase, increasing in system size and cost. |
Rajanna and Saini [106] | MHG/BMG/BGG/PV/WT | COE and OPF | Chamarajanagr (Karnataka) | India | It was discovered that IRES can give a viable solution for OPF values between 1.0 and 0.8. |
Kanase-Patil et al. [107] | MHP/BMG/BGG/PV, MHP/BMG/BGG/Wind/PV, MHP/BMG/BGG/energy plantation/PV, MHP/BMG/BGG/energy plantation/Wind/PV, | COE | Villages (Almora) | India | For variable biomass fuel prices, MHP/BMG/BGG/energy plantation/Wind/PV has been demonstrated to be more practical and sustainable than the other IRES systems. |
Dorji et al. [109] | PV/wind/Diesel/battery, PV/wind/ battery, PV/battery, Wind/battery | ICC, NPC and LUCE | - | Bhutan | The most cost-effective method was discovered to differ from one area to the next, depending on local renewable energy resources. |
Baek et al. [110] | PV/Wind/battery | Interest rate, NPC, COE and Project life | Island (Yeongjong) | South Korea | Combined cogeneration is cost-effective, although it emits a significant quantity of carbon dioxide per kWh. |
Niazi et al. [111] | PV/Wind | NPC, COE, and Operating cost | Nok Kundi and Ormara | Pakistan | The modeling of this hybrid system demonstrates that it is far superior to a single-source system. |
Tudu et al. [112] | PV/Wind/Battery/DG | NPC, COE and CO2 emission | Mangrove Forest | India | The upgraded version of the particle swarm optimization technique is capable of efficiently addressing this difficult non-linear optimization issue. |
Al-Shamma and Addoweesh [113] | PV/WT/Batt/DG, PV/Batt/DG, WT/Batt/DG, PV/WT/DG, WT/DG, DG, PV/WT/Batt, WT/Batt, PV/Batt | O & M and COE | Rural place | Saudi Arabia | The COE is not linearly reliant on the REF, as a little increase in the REF results in a large rise in the COE at high REF. |
Yuan et al. [115] | PV/Wood gas/battery | NPC, COE and CO2 emission | Rural house | China | The results revealed that the PV/WG/battery system with cycle charging mode had the lowest COE and NPC. |
Kirmani and Shadab [116] | PV/Wind/Diesel, PV/Grid, Wind/Grid, PV/Wind, and PV/Wind/Grid | Payback period, IRR, NPC, and COE | Selected village | India | In this analysis, the wind-grid connected system is the most matched hybrid system for the investigated instance, with the lowest energy and initial cost. |
Abujubbeh et al. [117] | PV/Wind | LCOE | Household (Amman) | Jordan | The hybrid system has a lower level of cost of electricity than the national grid tariff. |
Ramli et al.[118] | PV/Wind/Diesel/battery | COE and LPSP | West coastal area | Saudi Arabia | The hybrid system's energy costs are dominated by battery and wind turbine costs. |
Shezan and Ping [119] | PV-Diesel-Wind-battery | NPC, COE and CO2 emission | Offshore area | Bangladesh | The proposed hybrid system reduces CO2 emissions by approximately 1600 tons per year, indicating a major environmentally beneficial effort. |
Alharthi et al. [120] | PV-wind | NPC, COE and CO2 emission | Cities | Saudi Arabia | This study provides an overview of Saudi Arabia's current power generation status as well as a predicted growth in electricity consumption over the next 15 years. |
Al-Asfar et al. [121] | PV/Wind/Diesel/battery, PV/Wind/battery, Diesel |
NPC and COE | University | Jordan | In the scenario of maximum solar radiation and wind speed, the PV/Wind/Diesel/battery system had the lowest NPC and electricity cost. |
Tendo and Sirisamphanwong [122] | PV/Diesel | COE | University | Thailand | The primary aim was to design a PV/Diesel system of an optimal size that could meet load demand at the lowest possible cost. |
Rehman and El-Amin [123] | PV/Wind/Diesel, Wind/Diesel. PV/Diesel | COE | Remote Community (North-East) | Saudi Arabia | Among the hybrid power systems investigated in this scenario, PV-diesel was shown to be the most cost-effective. |
Pradhan et al. [124] | PV/Wind/Diesel/batteries | UCOE | Remote village (Khurda) | India | Presently, the best solution for stand-alone applications is a PV/wind/diesel/battery combination. |
Aziz [125] | PV/Wind/battery/FC | NPC, COE | Safari camp | UAE | The maximum electricity generated by the PV shows that it is more viable at the site than wind turbines. |
Al Ghaithi et al. [126] | Diesel, PV/Diesel, PV/Diesel/Wind | NPC, Operating cost, COE | Island (Masirah) | Oman | The results demonstrate that PV/Diesel/Wind is the most cost-effective alternative because it has the lowest NPC, OC, and COE for the system. |
Lao and Chungpaibulpatana [127] | Diesel, Diesel/PV, Diesel/PV/battery | Initial Capital cost, TNPC, and COE | Prasat Sambour | Cambodia | The best approach is a combination of diesel and PV with a battery, according to the findings. |
Mohamed et al. [128] | PV/Wind/Diesel/batteries | LCOE | Remote areas | Saudi Arabia | The purpose of the optimization was to reduce system costs while ensuring load demand and meeting a set of optimization constraints. |
Ahmad et al. [129] | Wind/PV/BMG | NPC and COE | Rural valley (Kallae Kahar) | Pakistan | When compared to an off-grid hybrid system with similar load patterns, the COE of a grid-connected hybrid system is lower. |
Ayadi and Alsalhen [130] | CSP/Wind | LCOE | - | Jordan | When wind and CSP energy sources are combined, the entire LCOE is around 0.045 JOD/kWh. |
Mudgal et al. [131] | PV/Wind/BGG | NPC and COE | Office building (Madras) | India | The results reveal that the integrated system is extremely sensitive to changes in component prices. |
Olamaei and Ghazvini [132] | PV/Battery/Diesel | Total cost | Parking lot | Iran | The best sizing for a PV/battery/diesel system was solved using a heuristic optimization technique that took into account the vehicle-to-grid parking lot as a controlled load. |
Quality | Units | Hybrid system | Grid |
Carbon dioxide | kg/yr | –24,603 | 38,129 |
Sulfur dioxide | kg/yr | –107 | 165 |
Nitrogen oxides | kg/yr | –52, 2 | 80.8 |
Location | LCOE at 650 ℃ | LCOE at 800 ℃ | LCOE at 1000 ℃ |
Newman | 0.167 | 0.180 | 0.213 |
Port Augusta | 0.183 | 0.197 | 0.232 |
Halls Creek | 0.158 | 0.174 | 0.213 |
Author(s) | Hybrid System | Parameters | Location | Country | Remarks |
Dufo-Lopez et al. [133] | PV/Wind/Diesel/battery | NPC and LCOE | Navarra | Spain | The stochastic technique finds an optimal system that is remarkably close to the one identified by the deterministic approach. |
Roth et al. [134] | PV/Diesel/battery | TLCC | Tropical island | France | The metamodel utilized is versatile enough to solve a variety of problems, ranging from traditional on-site consumption to self-use with partial injection. |
Ekren and Ekren [135] | PV/Wind/battery | LLP | University | Turkey | The Simulated Annealing method outperforms the Response Surface Methodology, according to the findings. |
Dursum et al. [136] | PV/Wind/Diesel/battery | NPC and COE | Isolated place (Edirne) | Turkey | It has been demonstrated that a PV/wind/diesel system with batteries may be widely used in distant places to replace or upgrade existing stand-alone diesel systems. |
Mukherjee and Asthana [138] | PV/Wind | LCOE | Residential area | UK | The hybrid systems have the potential to completely replace grid dependency. |
Akyuz et al. [137] | PV/Diesel, PV/Diesel/battery | COE | Remote farmhouse | Turkey | The number of hours that diesel generators are operating for a particular hybrid system decreases as PV capacity increases. |
Author(s) | Hybrid System | Parameters | Location | Country | Remarks |
Liu et al. [139] | PV/Wind/Diesel/battery | LPSP | Isolated island | Australia | The possibility of maximizing the decrease of stand-alone renewable system costs with diesel and energy storage was investigated using a two-stage mixed-integer linear programming model. |
Balaji and Gurgenci [140] | CST/PV/Diesel/ Battery |
LCOE and CO2 emission | Remote locations | Australia | In comparison to the diesel base case, these systems have significantly lower LCOE and emissions, and so offer a feasible solution for remote electricity. |
Lal and Raturi [141] | PV/Wind/Diesel/battery | NPC, COE and CO2 emission | Island (Vanua Levu) | Fiji | If a 10% yearly capacity shortage is tolerated, a 100% renewable energy-based arrangement is possible. |
Components | Grey wolf optimizer | Particle swarm optimization |
PV panels | 33 | 38 |
Battery banks | 90 | 90 |
Diesel generators | 2 | 3 |
Annual capital cost ($) | 10519.38 | 10214.95 |
Annual operation and management cost ($) | 170.46 | 160.81 |
Annual replacement cost ($) | 5638.5 | 6049 |
Annual cost system ($) | 16825.64 | 16872.66 |
Annual fuel cost ($) | 497.3 | 447.9 |
Configurations | PV-Battery | PV-Diesel-Battery | PV-Wind-Battery | PV-Wind-Diesel-Battery |
PV (kW) | 4 | 4 | 4 | 4 |
Wind turbine (kW) | - | - | 1 | 1 |
Gen-set (kW) | - | 10 | - | 10 |
Battery | 16 | 16 | 16 | 16 |
Converter (kW) | 5 | 5 | 5 | 5 |
NPC ($) | 18,161 | 21,671 | 26,824 | 29,225 |
COE ($) | 0.233 | 0.278 | 0.344 | 0.375 |
Initial cost ($) | 15,580 | 20,580 | 22,080 | 22,080 |
Operating cost | 163.87 | 69.23 | 301.15 | 517.04 |
Global solar (kWh/m2/d) | Total NPC ($) | Operating cost ($/yr.) | COE ($/kWh) | Ren fraction |
4.76 (–20%) | 83,915 | 1,263 | 0.355 | 0.84 |
5.95 | 74,836 | 2,584 | 0.316 | 0.96 |
7.14 (+20%) | 71,443 | 769 | 0.302 | 1 |
Diesel price ($/L) | Total NPC ($) | Operating cost ($/yr.) | COE $/kWh | Ren fraction |
0.52 (–20%) | 74,196 | 1,263 | 0.314 | 0.96 |
0.65 | 74,836 | 1,170 | 0.316 | 0.96 |
0.78 (+20%) | 75,477 | 1,356 | 0.319 | 0.96 |
Optimization methods | Solar-Wind hybrid energy system | Conventional power station |
NPC/year ($) | 198,347.984 | 198,348.00 |
COE/year (kt) | 288,194.00 | 297,000.00 |
Author(s) | Hybrid System | Parameters | Location | Country | Remarks |
Yahiaoui et al. [60] | PV/Diesel/Battery | ACC, ARC, AFC, AOM | Remote village (Djanet) | Algeria | GWO offers higher capabilities, reduced costs, and faster convergence. |
Muh and Tabet [61] | PV/Hydro/Diesel/Battery | COE | Offshore | Cameroon | Southern Cameroons' most viable economic system. |
Fodhil et al. [62] | PV/Diesel/Battery | COE, RF | Small village | Algeria | The PSO-based strategy is less expensive and has a higher PV penetration than HOMER. |
Diemuodeke et al. [63] | Diesel/PV/Wind/Battery PV/Wind/Battery |
CO2 emission, Payback time, COE | South-South region | Nigeria | For renewable energy specialists, the methodology offered serves as an ideal design tool. |
Djelailia et al. [64] | PV/Diesel/ Power Storage Hydroelectric | Fuel Consumption, CO2 emission | Remote site (Adrar) | Algeria | The idea demonstrates the utility of pump storage hydropower in irrigation and power recovery. |
Kaabeche and Ibtiouen [65] | PV/wind/diesel/battery | TNPC, COE, CO2 emission | Ghardaïa | Algeria | When compared to a PV/wind/battery system or a diesel generator (DG), the PV/wind/diesel/battery option is more cost-effective. |
Babatunde et al. [66] | PV/diesel/battery system | NPC, RF, COE | Rural health centers | Nigeria | In terms of the parameters, a PV/diesel/battery system is the most cost-effective. |
Samy et al. [67] | PV/FC | TNPV and LCOE | Remote urban areas | Egypt | Among the other methods, the FPA Algorithm has the shortest fulfillment time and the best rendering. |
Ogunjuyigbe and Ayodele [69] | PV/Diesel/Battery | NPC, COE, CO2, and CO emission | Telecom company | Nigeria | Out of the five economically viable configurations, PV/Diesel/Battery provides the best cost-benefit ratio. |
Tijani et al. [70] | PV/ Diesel/ Battery | NPC, COE, Operation, and Initial cost | Remote location (north) | Nigeria | The suggested system has a high energy potential and reduced carbon emissions while maintaining an affordable electricity cost. |
Samy [68] | PV/wind/battery, PV/FC, wind/FC, PV/wind/FC, and PV/wind/battery/FC | NPC, COE, Initial capital | Monshaet Taher Village | Egypt | A cost-effective hybrid renewable energy system was created to fulfill the known electric load of a small town in Egypt. |
Salisu et al. [71] | PV/Wind/Battery/Diesel | NPC, COE | Moriki village | Nigeria | The results also revealed a spectacular reduction in greenhouse gas (GHG) emissions of 100 percent because the ideal arrangement contains a 100 percent renewable portion. |
Rao et al, [72] | PV/Wind/Conventional Electric generator | NPC, COE, and CO2 emission | Deleta village | Ethiopia | In terms of economics and the environment, the hybrid design is the best ideal hybrid configuration. |
Babatunde and Ighravwe [73] | PV/Wind/battery, PV/battery, Diesel/battery, Wind/Diesel/battery, PV/Wind/Diesel/battery, PV/Diesel/battery | NPC, RF, COE, Operating cost | Rural community (Abadam) | Nigeria | Diesel/batteries were the best HRES for the case study from an economic standpoint, while wind/diesel generator/batteries were the best HRES from a technological and techno-economic standpoint. |
Sanni et al [74] | PV/Diesel | NPC, COE, Operating cost, RF | Kadawa village | Nigeria | The findings demonstrate that using renewable energy in agricultural activities can help achieve food security. |
Olatomiwa et al. [75] | PV/Diesel/battery and PV/Wind/Diesel/battery | NPC, COE, and CO2 emission | Remote mobile BTS | Nigeria | When compared to a stand-alone diesel generator system, the hybrid PV–diesel–battery system gives a greater reduction in CO2 emissions each year. |
Okundamiya et al. [76] | Wind/battery | COE, PSR and performance index | Typical residential region (Sokoto) | Nigeria | The proposed power system might save money and increase electricity reliability, according to the findings. |
Allali et al. [77] | Wind/Diesel/battery | - | Remote site (south) | Algeria | This system features a sophisticated control technique for managing several power sources (wind, diesel, and battery) that is dependent on weather circumstances, particularly wind speed values. |
Author(s) | Hybrid system | Parameters | Location | Country | Remarks |
Weis and Ilinca [78] | Wind/Diesel | Rural communities | Canada | Wind turbines are only acceptable for use in distant Canadian towns when the average yearly wind speed is at least 6.0 m/s. | |
Osaretin et al. [79] | PV/Wind/battery | NPC, LCOE, and Operating cost | Remote oil and gas facility | Canada | Continuous pumping using a hybrid renewable energy system with solar, wind, and battery storage has been identified as the best practicable approach based on economic and technological benefits. |
Ciez and Whitacre [80] | PV/Diesel/battery | LCOE | - | USA | In some circumstances, studies demonstrate that storage packs with high-energy, low-cost lithium-ion cells can compete with a non-renewable alternative. |
Optimization methods | GA | HOMER |
Total NPC ($) | 856013 | 890013 |
COE ($/kWh) | 0.163 | 0.214 |
Total production (kWh/yr) | 3,36,543 | 3,49,493 |
Total consumption (kWh/yr) | 3,16,428 | 3,28,266 |
CO2 emissions (kg/yr) | 3842 | 4089 |
Configurations | NPC ($) | COE ($/kWh) | Operating Cost ($) |
C1 (PV/Wind/Biomass/Biogas/FC and Battery) | 890,013 | 0.214 | 34,109 |
C2 (PV/Wind/Biomass/Biogas/FC without Battery) | 897,847 | 0.215 | 36,917 |
C3 (PV/Wind/Biomass/Biogas/Battery without FC) | 929,878 | 0.223 | 29,354 |
C4 (PV/Wind/Biomass/Biogas without storage) | 1,095,020 | 0.263 | 52,148 |
Components | Index |
Wind (numbers of turbines) | 11 |
PV (kW) | 357 |
Converter (kW) | 57 |
Battery (numbers of Battery) | 825 |
Grid (kW) | 0 |
Operating cost ($ per year) | 76,081 |
Initial capital ($) | 1,861,625 |
Total NPC ($) | 3,506,509 |
COE ($/kWh) | 0.545 |
Parameter | Value |
LCOE ($/kWh) | 0.159 |
NPV ($) | 24,618 |
IRR (%) | 16.3 |
PBP (years) | 6.4 |
Annual life cycle saving ($/year) | 788 |
System Configuration | Cost Component | Value | Unit |
PV-Biomass-Wind-Grid | Total NPC | 180,290,247.40 | $ |
LCOE | 0.05744 | $/kWh | |
PV-Biomass-Grid | Total NPC | 178,790,678.90 | $ |
LCOE | 0.0614 | $/kWh |
Author(s) | Hybrid System | Parameters | Location | Country | Remarks |
Shezan et al. [81] | PV/Wind/Diesel/battery | NPC and COE | Remote region | Malaysia | The simulation ensures that the system is financially and environmentally feasible in terms of net present cost (NPC) and CO2 emission reduction. |
Cai et al. [82] | PV/battery/Diesel | TLCC, LCOE, operating and maintenance cost | South Khorasan | Iran | The results reveal that combining solar energy with a diesel generator cuts greenhouse gas emissions and supply costs significantly when compared to a diesel-only system. |
Baneshi and Hadianfard [83] | PV/Wind/battery | COE | Shiraz | Iran | Carbon taxes could be an effective policy for encouraging large-scale renewable energy installations. |
Maleki et al. [84] | PV/Diesel/Battery | LCC | Rural townhouse | Iran | The findings suggest that Norouzak fuel is a viable alternative fuel-hybrid system choice. |
Hossain et al. [85] | PV/Wind/Diesel/battery | NPC and COE | Large resort sites (12 islands) | Malaysia | In the settings, PV, wind, diesel generator, converter, and battery make up the greatest stand-alone hybrid energy system. |
Wu et al. [86] | PV/Diesel/battery/Reverse Osmosis Desalination | LCC and LCOE | Remote area (Khorasan) | Iran | PV/diesel/battery/ROD is more cost-effective and environmentally friendly than a single diesel or PV system. |
Maleki [87] | PV/Wind/FC/Diesel, PV/diesel/FC, WT/diesel/FC | Total Annual Cost and emission | Remote location | Iran | The PV/WT/diesel/FC system is the best option in terms of pollutant emissions. |
Rodríguez-Gallegos et al. [88] | PV/battery/Diesel | LCOE and CO2 emission | Island | Indonesia | The findings demonstrate the cost-effectiveness, reduction of cumulative emissions, and even improvement of grid quality of PV hybrid systems in remote places. |
Rodríguez-Gallegos et al. [89] | PV/battery/Diesel | LCC | Island | Indonesia | An investigation was conducted to develop a method for successfully hybridizing DG-only systems with solar panels and batteries. |
Das and Zaman [90] | PV/Diesel/battery types | COE and NPC | Remote locality | Bangladesh | The findings reveal that the discount rate and capital cost have a considerable impact on COE and NPC. |
Mandal et al [91] | PV/Wind/Diesel/Battery | COE, NPC, operational and Life Cycle Emission | Rural area (North) | Bangladesh | Solar micro utility systems, Wind/Batt/Diesel systems, and Diesel generator-only systems are all more expensive than the proposed solution. |
Javed et al. [92] | PV/Wind/battery | LPSP and COE | Isolated zones | China | After accounting for the various load needs, the effect of LPSP on COE stays the same. |
Ramli et al. [93] | PV/Wind | LCOE | Yanbu city | Saudi Arabia | All of these activities are aimed at lowering the cost of renewable energy supplies, particularly solar PV and wind energy. |
Maleki et al. [94] | PV/battery | LPSP and TLCC | Rural areas (East) | Iran | In terms of both power quality and cost, the suggested framework may be used efficiently for off-grid photovoltaic installations in rural locations. |
Hafeznia et al. [96] | PV | Birjand region | Iran | The fuzzy gamma operator was placed on the fuzzified maps to discover the best locations for grid-connected solar systems. | |
Maleki and Pourfayaz, [95] | PV/Wind/Diesel/battery, PV/Wind/Diesel/FC | Total Annual Cost | Remote area (Rafsanjan) | Iran | The standard PV/WG/diesel/battery system is more cost-effective than the PV/WG/diesel/FC system, according to the findings. |
Jeyaprabha and Selvakumar [97] | PV/Diesel/battery | LCC | Remote location | Iran | The validation of this sizing technique with LLP < 0.01 for various load demands demonstrates that the system sizing with a linear scale factor may be used for every site with any load requirement. |
Das et al. [98] | PV/Diesel/battery | COE and CO2 emission | Village (Sarawak) | Malaysia | The PV/Battery combination is both cost-effective and environmentally benign, according to the findings. |
Suresh et al.[99] | PV/Wind/BG/BM/FC/ battery, PV/Wind/BGG/BMG/FC, PV/Wind/BGG/BMG/battery, PV/Wind/BGG/BMG | NPC, COE and Operating cost | village hamlets | India | In comparison to the HOMER, the GA-based system has greater PV penetration and emits less CO2. |
Vendoti et al. [100] | PV/Wind/BMG/BGG/FC | NPC, COE and Operating cost | Villages | India | According to the findings, PV-wind-biomass-biogas-fuel cell-battery-based HRES) has the lowest NPC. |
Vendoti et al. [101] | PV/Wind/BGG/BMG/FC/battery, PV/Wind/BGG/BMG/FC, PV/Wind/BGG/BMG/ battery, PV/Wind/BGG/BMG | NPC, COE and Operating cost | Remote village | India | The cheapest and most reliable option has been established as a combination of PV–Wind–Biomass–Biogas–FC and batteries. |
Vendoti et al. [102] | PV/FC/battery | Efficiency, Cost energy density, and Power density | Isolated rural area | India | It is concerned with the modeling and simulation of a Hybrid Renewable Energy System for rural electrification. |
Vendoti et al. [103] | PV/Wind/Diesel/Battery | TNPC, Capital cost, COE | Rural village (Karnataka) | India | According to the sensitivity results, the proposed model (PV-Diesel) will provide a feasible solution for the research region. |
He et al. [104] | PV/Wind/battery | Initial cost, Replacement cost, Operating and maintenance cost | Subtropical monsoon community | China | The hybrid energy system has a good complementing feature, with higher PV power output in the spring and summer and more wind power output in the autumn and winter. |
Nowdeh and Hajibeigy [105] | PV/Wind/FC | COE | Jolfa | Iran | For reliable and cost-effective design, the rate of component availability must be taken into account. |
Rajanna and Saini [114] | MHG/BMG/BGG/PV/WTG/battery, MHG/BMG/BGG/WT/battery, MHG/BMG/BGG/PV/battery | TNPC and COE | Remote area | India | If biomass generators are used during time scheduling, the number of batteries and overall system cost can be lowered. |
Rajanna and Saini [108] | MHG/BMG/BGG/PV/WT/battery | TNPC and COE | Remote area | India | From HILR to LIHR scenarios, load density was shown to increase, increasing in system size and cost. |
Rajanna and Saini [106] | MHG/BMG/BGG/PV/WT | COE and OPF | Chamarajanagr (Karnataka) | India | It was discovered that IRES can give a viable solution for OPF values between 1.0 and 0.8. |
Kanase-Patil et al. [107] | MHP/BMG/BGG/PV, MHP/BMG/BGG/Wind/PV, MHP/BMG/BGG/energy plantation/PV, MHP/BMG/BGG/energy plantation/Wind/PV, | COE | Villages (Almora) | India | For variable biomass fuel prices, MHP/BMG/BGG/energy plantation/Wind/PV has been demonstrated to be more practical and sustainable than the other IRES systems. |
Dorji et al. [109] | PV/wind/Diesel/battery, PV/wind/ battery, PV/battery, Wind/battery | ICC, NPC and LUCE | - | Bhutan | The most cost-effective method was discovered to differ from one area to the next, depending on local renewable energy resources. |
Baek et al. [110] | PV/Wind/battery | Interest rate, NPC, COE and Project life | Island (Yeongjong) | South Korea | Combined cogeneration is cost-effective, although it emits a significant quantity of carbon dioxide per kWh. |
Niazi et al. [111] | PV/Wind | NPC, COE, and Operating cost | Nok Kundi and Ormara | Pakistan | The modeling of this hybrid system demonstrates that it is far superior to a single-source system. |
Tudu et al. [112] | PV/Wind/Battery/DG | NPC, COE and CO2 emission | Mangrove Forest | India | The upgraded version of the particle swarm optimization technique is capable of efficiently addressing this difficult non-linear optimization issue. |
Al-Shamma and Addoweesh [113] | PV/WT/Batt/DG, PV/Batt/DG, WT/Batt/DG, PV/WT/DG, WT/DG, DG, PV/WT/Batt, WT/Batt, PV/Batt | O & M and COE | Rural place | Saudi Arabia | The COE is not linearly reliant on the REF, as a little increase in the REF results in a large rise in the COE at high REF. |
Yuan et al. [115] | PV/Wood gas/battery | NPC, COE and CO2 emission | Rural house | China | The results revealed that the PV/WG/battery system with cycle charging mode had the lowest COE and NPC. |
Kirmani and Shadab [116] | PV/Wind/Diesel, PV/Grid, Wind/Grid, PV/Wind, and PV/Wind/Grid | Payback period, IRR, NPC, and COE | Selected village | India | In this analysis, the wind-grid connected system is the most matched hybrid system for the investigated instance, with the lowest energy and initial cost. |
Abujubbeh et al. [117] | PV/Wind | LCOE | Household (Amman) | Jordan | The hybrid system has a lower level of cost of electricity than the national grid tariff. |
Ramli et al.[118] | PV/Wind/Diesel/battery | COE and LPSP | West coastal area | Saudi Arabia | The hybrid system's energy costs are dominated by battery and wind turbine costs. |
Shezan and Ping [119] | PV-Diesel-Wind-battery | NPC, COE and CO2 emission | Offshore area | Bangladesh | The proposed hybrid system reduces CO2 emissions by approximately 1600 tons per year, indicating a major environmentally beneficial effort. |
Alharthi et al. [120] | PV-wind | NPC, COE and CO2 emission | Cities | Saudi Arabia | This study provides an overview of Saudi Arabia's current power generation status as well as a predicted growth in electricity consumption over the next 15 years. |
Al-Asfar et al. [121] | PV/Wind/Diesel/battery, PV/Wind/battery, Diesel |
NPC and COE | University | Jordan | In the scenario of maximum solar radiation and wind speed, the PV/Wind/Diesel/battery system had the lowest NPC and electricity cost. |
Tendo and Sirisamphanwong [122] | PV/Diesel | COE | University | Thailand | The primary aim was to design a PV/Diesel system of an optimal size that could meet load demand at the lowest possible cost. |
Rehman and El-Amin [123] | PV/Wind/Diesel, Wind/Diesel. PV/Diesel | COE | Remote Community (North-East) | Saudi Arabia | Among the hybrid power systems investigated in this scenario, PV-diesel was shown to be the most cost-effective. |
Pradhan et al. [124] | PV/Wind/Diesel/batteries | UCOE | Remote village (Khurda) | India | Presently, the best solution for stand-alone applications is a PV/wind/diesel/battery combination. |
Aziz [125] | PV/Wind/battery/FC | NPC, COE | Safari camp | UAE | The maximum electricity generated by the PV shows that it is more viable at the site than wind turbines. |
Al Ghaithi et al. [126] | Diesel, PV/Diesel, PV/Diesel/Wind | NPC, Operating cost, COE | Island (Masirah) | Oman | The results demonstrate that PV/Diesel/Wind is the most cost-effective alternative because it has the lowest NPC, OC, and COE for the system. |
Lao and Chungpaibulpatana [127] | Diesel, Diesel/PV, Diesel/PV/battery | Initial Capital cost, TNPC, and COE | Prasat Sambour | Cambodia | The best approach is a combination of diesel and PV with a battery, according to the findings. |
Mohamed et al. [128] | PV/Wind/Diesel/batteries | LCOE | Remote areas | Saudi Arabia | The purpose of the optimization was to reduce system costs while ensuring load demand and meeting a set of optimization constraints. |
Ahmad et al. [129] | Wind/PV/BMG | NPC and COE | Rural valley (Kallae Kahar) | Pakistan | When compared to an off-grid hybrid system with similar load patterns, the COE of a grid-connected hybrid system is lower. |
Ayadi and Alsalhen [130] | CSP/Wind | LCOE | - | Jordan | When wind and CSP energy sources are combined, the entire LCOE is around 0.045 JOD/kWh. |
Mudgal et al. [131] | PV/Wind/BGG | NPC and COE | Office building (Madras) | India | The results reveal that the integrated system is extremely sensitive to changes in component prices. |
Olamaei and Ghazvini [132] | PV/Battery/Diesel | Total cost | Parking lot | Iran | The best sizing for a PV/battery/diesel system was solved using a heuristic optimization technique that took into account the vehicle-to-grid parking lot as a controlled load. |
Quality | Units | Hybrid system | Grid |
Carbon dioxide | kg/yr | –24,603 | 38,129 |
Sulfur dioxide | kg/yr | –107 | 165 |
Nitrogen oxides | kg/yr | –52, 2 | 80.8 |
Location | LCOE at 650 ℃ | LCOE at 800 ℃ | LCOE at 1000 ℃ |
Newman | 0.167 | 0.180 | 0.213 |
Port Augusta | 0.183 | 0.197 | 0.232 |
Halls Creek | 0.158 | 0.174 | 0.213 |
Author(s) | Hybrid System | Parameters | Location | Country | Remarks |
Dufo-Lopez et al. [133] | PV/Wind/Diesel/battery | NPC and LCOE | Navarra | Spain | The stochastic technique finds an optimal system that is remarkably close to the one identified by the deterministic approach. |
Roth et al. [134] | PV/Diesel/battery | TLCC | Tropical island | France | The metamodel utilized is versatile enough to solve a variety of problems, ranging from traditional on-site consumption to self-use with partial injection. |
Ekren and Ekren [135] | PV/Wind/battery | LLP | University | Turkey | The Simulated Annealing method outperforms the Response Surface Methodology, according to the findings. |
Dursum et al. [136] | PV/Wind/Diesel/battery | NPC and COE | Isolated place (Edirne) | Turkey | It has been demonstrated that a PV/wind/diesel system with batteries may be widely used in distant places to replace or upgrade existing stand-alone diesel systems. |
Mukherjee and Asthana [138] | PV/Wind | LCOE | Residential area | UK | The hybrid systems have the potential to completely replace grid dependency. |
Akyuz et al. [137] | PV/Diesel, PV/Diesel/battery | COE | Remote farmhouse | Turkey | The number of hours that diesel generators are operating for a particular hybrid system decreases as PV capacity increases. |
Author(s) | Hybrid System | Parameters | Location | Country | Remarks |
Liu et al. [139] | PV/Wind/Diesel/battery | LPSP | Isolated island | Australia | The possibility of maximizing the decrease of stand-alone renewable system costs with diesel and energy storage was investigated using a two-stage mixed-integer linear programming model. |
Balaji and Gurgenci [140] | CST/PV/Diesel/ Battery |
LCOE and CO2 emission | Remote locations | Australia | In comparison to the diesel base case, these systems have significantly lower LCOE and emissions, and so offer a feasible solution for remote electricity. |
Lal and Raturi [141] | PV/Wind/Diesel/battery | NPC, COE and CO2 emission | Island (Vanua Levu) | Fiji | If a 10% yearly capacity shortage is tolerated, a 100% renewable energy-based arrangement is possible. |