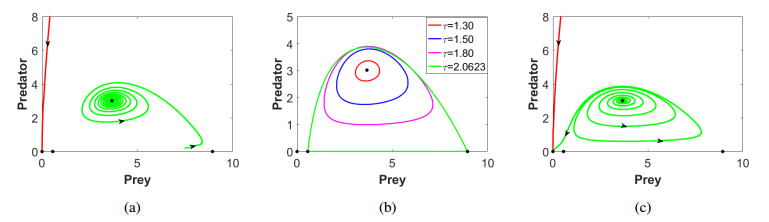
Citation: Kalyan Manna, Malay Banerjee. Stability of Hopf-bifurcating limit cycles in a diffusion-driven prey-predator system with Allee effect and time delay[J]. Mathematical Biosciences and Engineering, 2019, 16(4): 2411-2446. doi: 10.3934/mbe.2019121
[1] | Fang Liu, Yanfei Du . Spatiotemporal dynamics of a diffusive predator-prey model with delay and Allee effect in predator. Mathematical Biosciences and Engineering, 2023, 20(11): 19372-19400. doi: 10.3934/mbe.2023857 |
[2] | Yuhong Huo, Gourav Mandal, Lakshmi Narayan Guin, Santabrata Chakravarty, Renji Han . Allee effect-driven complexity in a spatiotemporal predator-prey system with fear factor. Mathematical Biosciences and Engineering, 2023, 20(10): 18820-18860. doi: 10.3934/mbe.2023834 |
[3] | Kawkab Al Amri, Qamar J. A Khan, David Greenhalgh . Combined impact of fear and Allee effect in predator-prey interaction models on their growth. Mathematical Biosciences and Engineering, 2024, 21(10): 7211-7252. doi: 10.3934/mbe.2024319 |
[4] | Mengyun Xing, Mengxin He, Zhong Li . Dynamics of a modified Leslie-Gower predator-prey model with double Allee effects. Mathematical Biosciences and Engineering, 2024, 21(1): 792-831. doi: 10.3934/mbe.2024034 |
[5] | Guangxun Sun, Binxiang Dai . Stability and bifurcation of a delayed diffusive predator-prey system with food-limited and nonlinear harvesting. Mathematical Biosciences and Engineering, 2020, 17(4): 3520-3552. doi: 10.3934/mbe.2020199 |
[6] | Moitri Sen, Malay Banerjee, Yasuhiro Takeuchi . Influence of Allee effect in prey populations on the dynamics of two-prey-one-predator model. Mathematical Biosciences and Engineering, 2018, 15(4): 883-904. doi: 10.3934/mbe.2018040 |
[7] | Yongli Cai, Malay Banerjee, Yun Kang, Weiming Wang . Spatiotemporal complexity in a predator--prey model with weak Allee effects. Mathematical Biosciences and Engineering, 2014, 11(6): 1247-1274. doi: 10.3934/mbe.2014.11.1247 |
[8] | Honghua Bin, Daifeng Duan, Junjie Wei . Bifurcation analysis of a reaction-diffusion-advection predator-prey system with delay. Mathematical Biosciences and Engineering, 2023, 20(7): 12194-12210. doi: 10.3934/mbe.2023543 |
[9] | Yue Xing, Weihua Jiang, Xun Cao . Multi-stable and spatiotemporal staggered patterns in a predator-prey model with predator-taxis and delay. Mathematical Biosciences and Engineering, 2023, 20(10): 18413-18444. doi: 10.3934/mbe.2023818 |
[10] | Yun Kang, Sourav Kumar Sasmal, Amiya Ranjan Bhowmick, Joydev Chattopadhyay . Dynamics of a predator-prey system with prey subject to Allee effects and disease. Mathematical Biosciences and Engineering, 2014, 11(4): 877-918. doi: 10.3934/mbe.2014.11.877 |
Incorporation of spatial components into a prey-predator system can potentially lead to very rich and complex spatiotemporal dynamics than the corresponding non-spatial counterpart which is in accordance with experimental observations and theoretical studies. For instance, Gause [1] performed a laboratory experiment regarding the growth of paramecium and didinum and identified the significance of heterogeneous spatial distribution on the stabilization and long term survival of certain species. Working on the theoretical setup introduced by Turing [2], Levin and Segel [3] explained the patchy distribution of plankton ecosystem and Klausmeier [4] investigated vegetation patterns in semiarid region. Diffusive prey-predator systems are able to produce a wide class of spatiotemporal patterns such as stationary (spots, labyrinthine, and mixture of stripes and spots) and non-stationary (periodic, quasi-periodic and chaotic etc.) patterns. Another class of non-stationary patterns includes traveling wave, periodic traveling wave and wave of invasion [5]. Prey-predator model with specialist predator, prey dependent functional response and linear death rate for predator is unable to produce stationary patterns through Turing instability. On the other hand, this type of models are capable to produce spatiotemporal chaotic patterns for equal diffusivity of both the species [6]. However, models with predator dependent functional response, density dependent death rate for predator or generalist predator are able to exhibit stationary patterns generated through Turing instability [7,8,9]. In this direction, transition from stationary pattern to different types of non-stationary patterns is getting lots of attention recently from researchers as the real instances of non-stationary patterns in ecological community are growing significantly. For example, plankton population in the Naroch Lakes in Belarus and vole population in northern Fennoscandia exhibit chaotic patterns [10,11].
There have been numerous evidences of Allee effect in ecological communities due to several ecological mechanisms such as difficulty in finding mates, habitat alteration and cooperative defense etc. [12,13,14]. Basically, Allee effect represents a positive correlation between population density and individual fitness of a species [12,13,14]. It is classified as the strong when the growth rate of a species becomes negative below a certain threshold density and the weak when the growth rate stays positive and increasing at low population density [13,15,16]. Incorporation of Allee effect in the modeling approach for prey-predator interactions is actually able to produce very rich temporal dynamics. For example, the classical Rosenzweig-MacArthur model exhibits temporal extinction scenario through homoclinic bifurcation in presence of the strong Allee effect [17]. Existence of two and three limit cycles in a temporal Leslie-Gower type prey-predator model with additive Allee effect in prey growth was shown in [18,19]. In case of two limit cycles, sub-critical Hopf bifurcation leads to an unstable limit cycle which is surrounded by a stable limit cycle [18]. In case of three limit cycles, homoclinic bifurcation leads to one limit cycle and Hopf bifurcation gives rise to other two [19]. Recently, we have identified that the temporal extinction for both the populations occurs through heteroclinic bifurcation in a prey-predator system with additive Allee effect, monotonic functional response with saturation and density dependent death rate for predators in [20].
Understanding the spatiotemporal dynamics of prey-predator interactions in presence of Allee effect and the corresponding spatial pattern formation has been emerging as an intriguing research theme in ecology and attracting the attention of many researchers. Du and Shi [21] studied a spatially heterogenous reaction-diffusion prey-predator system and found that the prey population can become extinct or persist or even blow-up depending on the initial conditions, heterogeneity of environment and certain parametric restrictions while the predator population maintains its density about a constant level. Further, they identified that heterogeneous environment can lead to the existence of Allee effect in case of strong prey growth. Wang et al. [22] considered a diffusive prey-predator system with strong Allee effect in prey growth and studied bifurcations of the spatially uniform, heterogeneous periodic and non-constant equilibrium solutions. In order to estimate the parametric region for spatial pattern formation, they examined the non-existence of the non-constant positive equilibrium solutions. In [23], the authors investigated the local and global asymptotic stability of positive homogeneous steady state and derived the conditions for Hopf bifurcation for a prey-predator model with Holling type Ⅱ functional response and additive Allee effect in prey growth. An extension of this model by incorporating the density dependent death rate for predators was presented in [24]. The authors studied the dissipation and persistence property, stability of the homogeneous steady states, and derived the conditions for the Hopf bifurcation of the positive homogeneous steady state. Further, they investigated the existence and non-existence of positive non-constant solutions of the system and presented some stationary Turing patterns only for the case of weak Allee effect. They found that density dependent death rate for predators plays a key role in producing different types of stationary patterns through Turing instability. Recently, a wide class of stationary and dynamic patterns for this model was reported in [20] in presence of both weak and strong Allee effect. They argued that the half-saturation constant plays a prominent role for the formation of this wide variety of patterns and identified the Hopf bifurcation as a necessary component to induce spatiotemporal chaos. Apart from these, they successfully found patchy invasion scenario for this model. Rao and Kang [25] studied a prey-predator system with multiplicative Allee effect in prey growth and Michaelis-Menten type functional response, and identified the strength of the Allee effect as the key component in formation of different types of stationary and dynamic patterns.
Mathematical modeling of ecological interactions is an evolving area where exposed discrepancies lead to alteration or reconstruction of the models. One important observation is almost all biological processes are not instantaneous in nature and require time delays such as time involved in the process of fertilization stage to birth and gestation period etc [26,27]. Time delay is incorporated into a prey-predator system with the understanding that the change in a specific population density at a particular time is regulated by both past and present population densities. Generally, the time delay is included in a prey-predator model through two different assumptions. For example, one can consider time delay in the prey growth term such that the prey population in absence of the predator population follows the well-known Hutchinson's equation which is actually the delayed logistic equation [28,29]. Another way to incorporate delay in a prey-predator model is to consider delayed numerical response term for the predator population and this type of time delay is known as the gestation delay [29,30]. That is, prey consumption does not immediately reflect in predator population in terms of offsprings but a time lag is required in order to take care of the gestation period. Kuang [27] has mentioned that non-delayed models of prey-predator interactions act as mere approximations of the real situations and ignore the reality. Therefore, it is necessary to investigate the consequences of time delay on the spatiotemporal pattern formation.
For the past few years, a considerable amount of efforts has been dedicated to investigate the dynamics of delayed spatiotemporal system for prey-predator interactions. According to the best of our knowledge, Roy Choudhury [31,32] first studied the spatial structure in a prey-predator model with Volterra-type distributed delays in the inter-species interaction terms by using linear analysis and multiple-scales perturbation method. Hadeler and Ruan [33] investigated the combined effects of delay and diffusion for delayed reaction-diffusion equations. Sen et al. [34] introduced a generalized approach to investigate the delay-induced spatiotemporal instability and reported the formation of spirals through Hopf-Turing transition. In [35], the authors considered a realistic modeling approach to explore the complex spatiotemporal behavior of populations and accordingly studied the stability and Hopf bifurcation for a spatiotemporal model with logistic type growth, nonlocal delay effect and Dirichlet boundary condition. Tian and Zhang [36] studied the spatiotemporal patterns in a delayed plankton system and identified that delay-induced Hopf bifurcation can lead to irregular spatial patterns. Banerjee and Zhang [37] investigated how Turing and delay-induced Hopf bifurcations together influence the spatial patterns for a ratio-dependent prey-predator model. They identified that comparatively large values of time delay and the ratio of diffusivity play prominent roles in destabilizing the system's dynamics. In a subsequent study [38], they explored the role of time delay in producing spatiotemporal chaotic patterns for a prey-predator model with Holling type Ⅱ functional response and density dependent death rate for predators. Apart from the chaotic patterns, the existence of stationary, quasi-periodic patterns were also reported in this study. Song et al. [39] presented results regarding persistence, stability and delay-induced Hopf bifurcation in a delayed diffusive ratio-dependent prey-predator model. They also calculated the formulae for determining the direction and stability of the Hopf-bifurcating periodic solutions and showed the existence of spatially homogeneous and inhomogeneous periodic solutions through numerical illustrations. For other related works on delay-induced Hopf bifurcation and its stability and direction for reaction-diffusion systems of prey-predator interactions, interested readers are referred to the articles [40,41,42,43,44,45,46,47,48,49,50,51,52,53,54,55,56]. Recently, Jankovic et al. [57] showed that the delay-induced spatiotemporal chaos is possible for single species population models. They considered the spatiotemporal dynamics of a single species population using two different mathematical formulations such as delayed diffusive logistic equation and integro-differential equation incorporating a spatial convolution. Also, spatiotemporal dynamics of delayed three-species food-chain models were investigated in [58,59,60].
Being motivated by the works discussed above, we aim to investigate the inherent relationship between time delay and emerging spatial patterns for a delayed diffusive prey-predator system with additive Allee effect in prey growth, monotonic functional response with saturation and density dependent death rate for predators. Majority of the existing literature on the delayed spatiotemporal dynamics of prey-predator interactions have considered one-dimensional spatial components and this simplification takes the outcome away from the reality. In this paper, our goal is to provide both the analytical and numerical results for the delayed spatiotemporal model with two-dimensional spatial components. Also as far as our knowledge is concerned, work on the delayed spatiotemporal dynamics in presence of the Allee effect, which is quite commonly observed for several ecological communities, is rare in literature. These facts actually make our investigation more complicated and interesting, and also set apart our proposed works in this paper from any other existing work in literature. We aim to provide verification of the analytical results regarding the direction and stability of the Hopf-bifurcating periodic solutions through numerical simulations and construction of relevant bifurcation diagram. In this study, we are especially interested in the onset of delay-mediated spatiotemporal chaos. Also, we would like to explore when the spatial and corresponding non-spatial systems will produce similar qualitative dynamical behaviors in presence of the time delay. We will present a comparative study between the spatial and non-spatial systems.
The remaining part of this paper is organized in the following fashion. We present the model and its description in the next section. Section 3 deals with the well-posedness of the problem and discusses about the associated spatially homogeneous steady states. In Section 4, we present linear analysis and derive the analytical expression for the delay induced Hopf bifurcation threshold. The succeeding section discusses about the analytical results regarding the properties of the Hopf bifurcating periodic solutions. In order to support our theoretical findings and to investigate the effect of time delay on stationary patterns, we present several numerical illustrations in Section 6. Finally, Section 7 ends this paper with a brief discussion.
In this section, we extend the spatiotemporal prey-predator model presented in [20,24] with additive Allee effect in prey growth, prey-dependent monotonic functional response with saturation and density dependent death rate for predator by incorporating the gestation delay for predator population as follows:
∂N(x,t)∂t=rN(x,t)(1−N(x,t)K)−mN(x,t)N(x,t)+b−cN(x,t)P(x,t)N(x,t)+a+d1ΔN(x,t), | (2.1) |
∂P(x,t)∂t=csN(x,t−τ)P(x,t−τ)N(x,t−τ)+a−sP(x,t)(q+δP(x,t))+d2ΔP(x,t), | (2.2) |
subjected to the non-negative initial conditions:
N(x,θ)=ϕ1(x,θ)≥0,P(x,θ)=ϕ2(x,θ)≥0,for(x,θ)∈¯Ω×[−τ,0], | (2.3) |
and zero-flux boundary conditions:
∂N∂ν=0,∂P∂ν=0,forx∈∂Ω,t>0. | (2.4) |
Here Ω is a bounded spatial domain in Rn with smooth boundary ∂Ω and ¯Ω=Ω∪∂Ω. The zero-flux boundary conditions indicate that no individual can move across the boundary with ∂∂ν being the outward drawn normal derivative on the boundary ∂Ω. The prey and predator population densities at position x∈Ω⊂Rn and time instant t are represented by N(x,t) and P(x,t), respectively. The parameters r and K stand for the intrinsic growth rate and the environmental carrying capacity in absence of the Allee effect, respectively, for the prey population. However, the effective environmental carrying capacity for prey population with additive Allee effect is actually different from K [61,62,63,64]. The expression of effective carrying capacity for prey population is explicitly given below. The rate of severity of the Allee effect and the prey population size at which fitness is half of its maximum value are respectively represented by the parameters m and b. Predator captures prey at a rate c while a denotes the half saturation constant. The parameter s represents feed concentration for predator with q and δ as natural and density dependent death rate for predator, respectively. Therefore, the quantities sq and sδ represent respectively the effective natural and density dependent death rates for predator. All these parameters are positive constants. Further, τ represents the gestation delay for predator population which reflects the fact that the consumption of prey can not lead to instantaneous reproduction of predator. The fractional terms mN+b and cNN+a in the model (2.1)-(2.2) represent additive Allee effect in prey growth and prey density dependent functional response for predator, respectively. The constant diffusion coefficients of prey and predator populations are respectively denoted by d1 and d2, and Δ represents the Laplacian operator.
Depending on the parameter values the Allee effect can act as weak or strong in nature, which has been thoroughly investigated in [24]. Here, we reiterate these parameter restrictions for weak and strong Allee effects briefly as follows:
(a) If the parameters obey the inequality b2rK<m<br, then the Allee effect is weak. In this case without any predator, prey population can survive for a very tiny positive initial prey population size and eventually approaches to N1=r(K−b)+√r2(K−b)2+4rK(br−m)2r. Also, N1 acts as the effective environmental carrying capacity in presence of the additive Allee effect for prey population [61,62,63,64].
(b) If the parameters obey the inequality b2rK<br<m<r2(K−b)2+4br2K4rK, then the Allee effect is strong. In this case without any predator, the prey population persists and approaches to N1 for any positive initial prey population size greater than a critical prey population threshold Ncrit and goes to extinction for any positive initial prey population size less than Ncrit, where Ncrit=r(K−b)−√r2(K−b)2+4rK(br−m)2r. This Ncrit acts as the Allee effect threshold for strong Allee effect.
In the following sections, we will examine the system (2.1)-(2.4) both analytically and numerically. We would like to mention here that numerical simulations will be carried out in two-dimensional space though all analytical results hold for n-dimensional space.
In this study, we are dealing with prey and predator populations and for this reason it is pertinent to establish the existence, uniqueness, non-negativity and boundedness of the solutions of the concerned system (2.1)-(2.4). In this section, we discuss these issues along with the existence of possible spatially homogeneous steady states. Before proceeding further, let us introduce some useful notations. Let X=C(¯Ω,R2) be a Banach space of continuous functions from ¯Ω to R2 and C=C([−τ,0],X) denote the Banach space of continuous functions from [−τ,0] to X equipped with the usual supremum norm. For the sake of convenience, an element ϕ∈C is a function from ¯Ω×[−τ,0] into R2 and is defined by ϕ(x,θ)=ϕ(θ)(x). We define ωt∈C by ωt(θ)=ω(t+θ), θ∈[−τ,0] for any continuous function ω:[−τ,T)→X where T>0.
Theorem 3.1. For any given ϕ∈C satisfying the initial conditions (2.3), the delayed spatiotemporal system (2.1)-(2.4) admits a unique solution defined on [0,+∞) and this solution remains non-negative and bounded for all t≥0.
Proof. For any ϕ=(ϕ1,ϕ2)T∈C and x∈¯Ω, we define F=(F1,F2):C→X by
F1(ϕ)(x)=rϕ1(x,0)(1−ϕ1(x,0)K)−mϕ1(x,0)ϕ1(x,0)+b−cϕ1(x,0)ϕ2(x,0)ϕ1(x,0)+a,F2(ϕ)(x)=csϕ1(x,−τ)ϕ2(x,−τ)ϕ1(x,−τ)+a−sϕ2(x,0)(q+δϕ2(x,0)). |
Then we can rewrite the system (2.1)-(2.4) in terms of the following abstract functional differential equation:
U′(t)=AU+F(Ut),t>0, | (3.1) |
U(0)=ϕ∈X, | (3.2) |
where U=(N,P)T, ϕ=(ϕ1,ϕ2)T and AU=(d1ΔN,d2ΔP)T. One can easily observe that F is locally Lipschitz in X. It follows from [65,66,67,68,69] that the system (3.1)-(3.2) admits a unique local solution on the interval [0,Tmax) where Tmax represents the maximal existential time for solution of the system (3.1)-(3.2). Also, since 0 = (0, 0) is a lower solution of the system (2.1)-(2.2), we can easily obtain that N(x,t)≥0 and P(x,t)≥0.
Now, it remains to prove the boundedness of the solutions of the system (2.1)-(2.4). From the equation (2.1), we can easily obtain that
∂N∂t−d1ΔN≤rN(1−NK),∂N∂ν=0,N(x,0)=ϕ1(x,0)≥0. |
Let ˆN(t) be a solution to the following ordinary differential equation:
dˆNdt=rˆN(1−ˆNK),ˆN(0)=maxx∈¯Ωϕ1(x,0). |
Thus, we have ˆN(t)≤max{K,maxx∈¯Ωϕ1(x,0)} for all t∈[0,Tmax) [24,70]. Then by using the comparison principle for parabolic partial differential equations [71], we obtain that N(x,t)≤ˆN(t). Therefore,
N(x,t)≤max{K,maxx∈¯Ωϕ1(x,0)},∀(x,t)∈¯Ω×[0,Tmax). |
Now, from the equation (2.2) and using the bound for N, we deduce that P(x,t) satisfies the following system:
∂P∂t−d2ΔP≤csρρ+aP(x,t−τ)−sP(x,t)(q+δP(x,t)),∂P∂ν=0,P(x,θ)=ϕ2(x,θ)≥0, |
where ρ=max{K,maxx∈¯Ωϕ1(x,0)}. Further, let ˆP(t) be a solution to the following delay differential equation:
dˆPdt=csρρ+aˆP(t−τ)−sˆP(t)(q+δˆP(t)),ˆP(θ)=maxx∈¯Ωϕ2(x,θ),θ∈[−τ,0]. |
In order to find a bound for ˆP(t), we recall a related result presented in [72,73]. This result states that for an equation of the form du(t)dt=Au(t−τ)−Bu(t)−Cu2(t) with A,B,C>0 and u(θ)>0 for θ∈[−τ,0], we get limt→+∞u(t)=A−BC when A>B and limt→+∞u(t)=0 when A<B. Now, we assume that M=max{0,(c−q)ρ−aqδ(ρ+a)}. Hence, using this result we obtain ˆP(t)≤max{M,max(x,θ)∈¯Ω×[−τ,0]ϕ2(x,θ)} for all t∈[0,Tmax). Then again using the comparison principle for parabolic partial differential equations [71], we obtain that P(x,t)≤ˆP(t). Therefore,
P(x,t)≤max{M,max(x,θ)∈¯Ω×[−τ,0]ϕ2(x,θ)},∀(x,t)∈¯Ω×[0,Tmax). |
From the above discussion, we obtain that N(x,t) and P(x,t) are bounded on ˉΩ×[0,Tmax) and also we have Tmax=+∞ from the standard theory of semi-linear parabolic partial differential equations [74]. This completes the proof.
Now, we discuss about the spatially homogeneous steady states of the model (2.1)-(2.2). Any steady state of the model (2.1)-(2.2) satisfies the following system of coupled partial differential equations:
−d1ΔN=rN(1−NK)−mNN+b−cNPN+a,−d2ΔP=csNPN+a−sP(q+δP),∂N∂ν=∂P∂ν=0,x∈∂Ω. |
But in this study, we are only concerned with the spatially homogeneous steady states. Then, any non-negative solution of the following system of coupled algebraic equations corresponds to a spatially homogeneous steady state E=(ˆN,ˆP) of the model (2.1)-(2.2):
rˆN(1−ˆNK)−mˆNˆN+b−cˆNˆPˆN+a=0,csˆNˆPˆN+a−sˆP(q+δˆP)=0. |
From the above two algebraic equations, one can easily notice that there always exists a trivial extinction steady state E0=(0,0). For weak Allee effect, there exists a unique prey-only axial steady state E1=(r(K−b)+√r2(K−b)2+4rK(br−m)2r,0)≡(N1,0). But in case of strong Allee effect, apart from the steady state E1 there exists another additional prey-only axial steady state E2=(r(K−b)−√r2(K−b)2+4rK(br−m)2r,0)≡(Ncrit,0), whose prey component actually indicates the threshold value for prey population below which prey species can not persist in the non-spatial counterpart of the considered model (2.1)-(2.2). In order to find a spatially homogeneous coexisting steady state E∗=(N∗,P∗), we need to solve a quartic equation in N∗ as follows:
A4N4∗+A3N3∗+A2N2∗+A1N∗+A0=0, |
where A4=rδK, A3=(2a+b)rδK−rδ, A2=a(a+2b)rδK+mδ+c(c−q)−(2a+b)rδ, A1=a2brδK+2amδ+bc(c−q)−a(a+2b)rδ−acq and A0=a2mδ−a2brδ−abcq. The corresponding component for predator population, i.e. P∗, is evaluated through P∗=1δ(cN∗N∗+a−q). In this study, we use numerical computation for obtaining coexisting steady state since it is very difficult to compute coexisting steady state analytically from a quartic equation. Also, note that depending on the parameter values we can obtain one or more than one spatially homogeneous coexisting steady states for the model (2.1)-(2.2). For detailed discussion on steady states one can go through the works presented in [24]. In this study, we concentrate only on the case when there exists a unique spatially homogeneous coexisting steady state for the model (2.1)-(2.2).
In this section, we investigate the delay induced Hopf bifurcation in the delayed spatiotemporal system (2.1)-(2.4) around a typical spatially homogeneous coexisting steady state E∗=(N∗,P∗) through the linearization technique. Before proceeding further, let us introduce some useful notations for the sake of convenience. Let us consider 0=λ0<λ1<λ2<⋯<λn<⋯ be the eigenvalues of the operator −Δ on Ω under the zero-flux boundary conditions and let F(λi) be the space of eigenfunctions corresponding to λi in C1(Ω). Let {ψij:j=1,2,…,dimF(λi)} be an orthonormal basis of F(λi), Xij={κψij:κ∈R2} and X={U∈[C1(¯Ω)]2∣∂U∂ν=0on∂Ω}. Then we can write
X=∞⨁i=1XiandXi=dimF(λi)⨁j=1Xij. |
Linearizing the model (2.1)-(2.2) about the spatially homogeneous coexisting steady state E∗=(N∗,P∗), we obtain
∂U(x,t)∂t=DΔU(x,t)+J1U(x,t)+J2U(x,t−τ), | (4.1) |
where D=diag(d1,d2), U(x,t)≡(U1(x,t),U2(x,t)), U(x,t−τ)≡(U1(x,t−τ),U2(x,t−τ)) and
J1=[N∗(m(N∗+b)2−rK+cP∗(N∗+a)2)−cN∗(N∗+a)0−s(q+2δP∗)]≡[A11A12A21A22], |
J2=[00acsP∗(N∗+a)2csN∗N∗+a]≡[B11B12B21B22]. |
Let us define LU=DΔU(x,t)+J1U(x,t)+J2U(x,t−τ). Note that Xi is invariant under the operator L for each i≥0. Further, μ is an eigenvalue of the operator L if and only if μ satisfies the equation det(Ji−μI2)≡det(−λiD+J1+J2e−μτ−μI2)=0 for some i≥0 and there exists an eigenvector in Xi for this case. Since our model is subjected to the zero-flux boundary conditions, a typical eigenfunction of (4.1) is of the form
(U1,U2)=(η1,η2)eμtn∏j=1cos(kjxj), |
where x=(x1,x2,⋯,xn)∈Rn and k=(k1,k2,⋯,kn) represents the n-dimensional wave-vector. Then, the characteristic equation is given by
Δ(μ,τ)≡μ2+C1μ+C0+(D1μ+D0)e−μτ=0, | (4.2) |
where C1=−(A11+A22)+k2(d1+d2), C0=(A11−k2d1)(A22−k2d2), D1=−B22, D0=(A11B22−A12B21)−k2d1B22 and k2=k⋅k.
In absence of the time delay (that is, when τ=0), the characteristic equation reduces to the following quadratic equation:
Δ(μ,0)≡μ2+(C1+D1)μ+(C0+D0)=0. | (4.3) |
Working on the equation (4.3), one can obtain the conditions for Turing and spatiotemporal Hopf bifurcation for the corresponding non-delayed model which have been investigated earlier in [20,24]. Since the main goal of this study is to understand the destabilizing role of discrete time delay, we assume that the coexisting homogeneous steady state E∗=(N∗,P∗) is locally asymptotically stable for the non-delayed model. That is, we assume the following conditions hold: C1+D1>0 and C0+D0>0.
Now, we investigate the effect of time delay and deduce the conditions under which the model (2.1)-(2.2) undergoes delay induced Hopf bifurcation. In order to find the delay induced Hopf bifurcation threshold, we follow the procedure presented in [75]. Substituting μ=iω into the characteristic equation (4.2) and separating the real and imaginary parts, we arrive at the following two equations:
ω2−C0=D0cosωτ+D1ωsinωτ, | (4.4) |
C1ω=D0sinωτ−D1ωcosωτ. | (4.5) |
Eliminating the trigonometric functions from the above two equations, we arrive at the following quartic equation in ω:
ω4+(C21−2C0−D21)ω2+(C20−D20)=0. | (4.6) |
Now, note that one can visualize the equation (4.6) as a quadratic equation of ω2 and if both the values of ω2 are negative or imaginary, the time delay can not able to alter the stability property of the homogeneous coexisting steady state. But if we have at least one positive real value of ω2, then destabilization of the homogeneous coexisting steady state is possible through τ. In order to find the Hopf bifurcation threshold in terms of the delay parameter τ, we assume ω∗ denotes a positive root of the equation (4.6). The simplest possible assumptions that would give us a unique positive root ω∗ of the equation (4.6) is C21−2C0−D21>0 and C20−D20<0. The expression for the unique positive root ω∗ is given by
ω∗=1√2√−(C21−2C0−D21)+√(C21−2C0−D21)2−4(C20−D20). |
Then eliminating sinωτ from the equations (4.4)-(4.5) and solving for τ, we obtain
τ(n)=1ω∗arccos(D0(ω∗2−C0)−C1D1ω∗2D20+D21ω∗2)+2nπω∗, | (4.7) |
for n=0,1,2,3,⋯. Now, setting
τ∗=τ(0)=1ω∗arccos(D0(ω∗2−C0)−C1D1ω∗2D20+D21ω∗2), | (4.8) |
and by using the Butler's lemma [76], we obtain that the homogeneous coexisting steady state E∗=(N∗,P∗) is unstable for τ>τ∗. On the other hand, the characteristic equation (4.2) does not have any root on the imaginary axis for τ∈[0,τ∗). In conclusion, we obtain that E∗=(N∗,P∗) is locally asymptotically stable for τ∈[0,τ∗) and becomes unstable for τ>τ∗. But in order to ensure that the occurrence of instability is due to delay driven Hopf bifurcation, we need to verify the associated transversality condition:
ddτ(Re(μ(τ)))∣τ=τ∗>0. | (4.9) |
Differentiating the both sides of the characteristic equation (4.2) with respect to τ, we get
(2μ+C1)dμdτ−τ(D1μ+D0)e−μτdμdτ+D1e−μτdμdτ−μ(D1μ+D0)e−μτ=0, |
which eventually leads to
(dμdτ)−1=2μ+C1μ(D1μ+D0)e−μτ+D1μ(D1μ+D0)−τμ=2μ+C1−μ(μ2+C1μ+C0)+D1μ(D1μ+D0)−τμ. |
Thus, we obtain
Re(dμdτ|τ=τ∗)−1=Re[(2iω∗+C1)−iω∗(−ω∗2+iC1ω∗+C0)+D1iω∗(iD1ω∗+D0)−τ∗iω∗]=Re[C1+2iω∗C1ω∗2+iω∗(ω∗2−C0)+D1−D1ω∗2+iD0ω∗]=2ω∗2+C21−2C0C21ω∗2+(ω∗2−C0)2−D21D21ω∗2+D20. | (4.10) |
Now, from equations (4.4)-(4.5) we have
C21ω∗2+(ω∗2−C0)2=D21ω∗2+D20. | (4.11) |
Hence, equation (4.10) leads to
Re(dμdτ|τ=τ∗)−1=2ω∗2+C21−2C0−D21D20+D21ω∗2. | (4.12) |
Thus, from the assumptions for the occurrence of the unique positive root ω∗ of the equation (4.6), we obtain Re(dμdτ|τ=τ∗)−1>0. Further, we know that sign[ddτ(Re(μ(τ)))|τ=τ∗]=sign[Re(dμdτ|τ=τ∗)−1] and therefore, we conclude that the inequality (4.9) is satisfied.
Therefore, summarizing the above detailed analysis we arrive at the following theorem:
Theorem 4.1. The delayed spatiotemporal system (2.1)-(2.4) undergoes Hopf bifurcation around the spatially homogeneous coexisting steady state E∗=(N∗,P∗) at τ=τ∗ when C1+D1>0, C0+D0>0 and the assumptions for the unique positive ω∗ hold.
Remark 4.1. From the expression (4.8) for τ∗, we can notice that τ∗ depends on the value of k for the considered spatiotemporal system (2.1)-(2.4). In other words, for the considered system (2.1)-(2.4), there will exist a non-negative k for which we will have our theoretical Hopf bifurcation threshold τ∗. Also, it is important to note that for different values of system parameters the system (2.1)-(2.4) will need different non-negative k-values in order to achieve the Hopf bifurcation threshold. This fact will be demonstrated more clearly in Section 6. Here, we would like to mention that by considering k=0 we can eventually achieve the Hopf bifurcation threshold for the corresponding non-spatial counterpart of the spatial model (2.1)-(2.2). We denote this threshold by τ∗0, where the subscript \enquote{0} signifies the fact k=0. Hence, we conclude that the corresponding non-spatial model undergoes the Hopf bifurcation at the coexisting steady state E∗=(N∗,P∗) at τ=τ∗0 provided the conditions and assumptions given in Theorem 4.1 are true when k=0. Also, as per our knowledge there is no literature where the dynamics of the non-spatial counterpart of the model (2.1)-(2.2) have been investigated. Therefore, our findings in this section also provide key insights about the dynamics of the corresponding non-spatial model.
Now we briefly corroborate the conclusions made about the dynamics of the non-spatial model corresponding to the model (2.1)-(2.2) in the above remark through numerical illustration. For numerical illustration, we restrict ourselves to the case of strong Allee effect. The three figures presented in the Figure 1 have been obtained by varying the value of the gestation delay parameter τ in an increasing manner while all other system parameters were kept fixed (all the parameter values are mentioned in the caption of the Figure 1). Figure 1(a) represents a bistable scenario for a lower value of τ (for example, τ=1.0), where the total extinction steady state E0=(0,0) is a stable node and the coexisting steady state E∗=(3.665,3.015) is a stable focus. In this case, the basins of attraction of these two steady states are kept apart by the stable manifold of the prey-only steady state E2=(0.559,0) whose prey component acts as a threshold prey population density characterizing the strong Allee effect. For the considered parameter values, we have computed the Hopf bifurcation threshold as τ∗0=1.275. Also, we have obtained dμdτ=0.0321−0.0674i at τ=τ∗0 which eventually confirms the occurrence of the Hopf bifurcation. Therefore, we can expect the existence of Hopf-bifurcating limit cycles by setting the value of τ to be lower or greater than 1.275 and Figure 1(b) shows the existence of the Hopf-bifurcating limit cycles for τ>1.275. From this figure we can observe that the size of the limit cycle is gradually increasing with the increment in the value of τ. Here, we would like to mention that the emerging limit cycles around the coexisting steady state E∗=(3.665,3.015) is stable in nature. Therefore, the Hopf bifurcation can be classified as the supercritical Hopf bifurcation. With this increasing limit cycles another interesting feature such as the heteroclinic orbit emerges and this orbit connects the two prey-only steady states E2=(0.559,0) and E1=(8.941,0) when τ=2.0623. We have estimated numerically the threshold value of τ for the heteroclinic bifurcation to be approximately τHO=2.0623 (see the green colored orbit presented in Figure 1(b)). Through this bifurcation the basin of attraction for the stable limit cycles around the coexisting steady state E∗=(3.665,3.015) is demolished and E0=(0,0) becomes the sole attractor. This scenario can be easily noticeable from the Figure 1(c). These scenarios are presented in a summarized fashion in Figure 2 by a one parameter (τ) bifurcation diagram. In this figure, the blue and black vertical straight lines represent the Hopf and heteroclinic bifurcation thresholds, respectively. Two completely red horizontal straight lines respectively stand for the prey-only steady states E1 and E2 which are always saddle points and hence unstable. The completely green horizontal straight line along the τ-axis stands for the extinction steady state E0=(0,0) which is always a stable equilibrium point. The partially green and partially red straight line in the middle denotes the coexisting steady state E∗=(3.665,3.015) which is a stable focus for τ less than τ∗0 and an unstable focus for τ greater than τ∗0. The green curve, which lies between the blue and black vertical lines, represents the existence of stable limit cycle that arises through Hopf bifurcation and disappears through heteroclinic bifurcation. Note that the green and red colors signify stability and instability properties of the steady states and limit cycles, respectively. In the next section, we derive the analytical conditions for the stability of the Hopf-bifurcating limit cycle and its direction for the system (2.1)-(2.4).
In this section, we investigate direction and stability of the Hopf bifurcating limit cycles by using center manifold reduction and normal form theory for partial functional differential equation [69,75,77].
Let us consider ˜N(⋅,t)=N(⋅,t)−N∗, ˜P(⋅,t)=P(⋅,t)−P∗, ˜U(t)≡(˜N(⋅,t),˜P(⋅,t)) and τ=τ∗+ς for ς∈R. Then, ς=0 corresponds to the Hopf bifurcation threshold of the system (2.1)-(2.4). In order to normalize the delay we rescale the time t by tτ and also recall the Banach space decomposition X=⨁∞i=1Xi. Now, applying these new variables in the system (2.1)-(2.2) and then dropping the tildes for the simplicity of notation, we obtain the following transformed system in space C=C([−1,0],R2):
dU(t)dt=Lς(Ut)+f(Ut,ς), | (5.1) |
where for φ=(φ1,φ2)T∈C, Lς:C→R2 and f:C×R→R2 are respectively given by
Lς(φ)=(τ∗+ς)[−d1k2+A11A120−d2k2+A22][φ1(0)φ2(0)]+(τ∗+ς)[00B21B22][φ1(−1)φ2(−1)], |
f(φ,τ)=(τ∗+ς)[∑i+j≥21i!j!f(1)ijφi1(0)φj2(0)∑i+j+l≥21i!j!l!f(2)ijlφi1(−1)φj2(0)φl2(−1)], |
where
f(1)=rN(1−NK)−mNN+b−cNPN+a,f(2)=csN1P1N1+a−sP(q+δP), |
and
f(1)ij=∂i+jf(1)∂Ni∂Pj(N∗,P∗),f(2)ijl=∂i+j+lf(2)∂Ni1∂Pj∂Pl1(N∗,P∗,P∗). |
Now by the Riesz representation theorem [75], there exists a 2×2 matrix function η(θ,ς) for −1≤θ≤0, whose elements are of bounded variation such that
Lς(φ)=∫0−1dη(θ,ς)φ(θ)forφ∈C. | (5.2) |
As a matter of fact, one can choose
η(θ,τ∗)=(τ∗+ς)[−d1k2+A11A120−d2k2+A22]δ(θ)+(τ∗+ς)[00B21B22]δ(θ+1), | (5.3) |
where δ represents a Dirac delta function. For φ∈C1([−1,0],R2), let us define
A(ς)φ={dφ(θ)dθ,forθ∈[−1,0),∫0−1dη(γ,ς)φ(γ),forθ=0, | (5.4) |
and
R(ς)φ={0,forθ∈[−1,0),f(ς,φ),forθ=0. | (5.5) |
Thus, the system (5.1) is equivalent to the following system:
˙Ut=A(ς)(Ut)+R(ς)(Ut), | (5.6) |
where Ut(θ)=U(t+θ) for θ∈[−1,0]. Now for ψ∈C1([0,1],(R2)∗), we define
A∗ψ(γ)={−dψ(γ)dγ,forγ∈(0,1],∫0−1ψ(−t)dηT(t,0),forγ=0, | (5.7) |
and a bilinear inner product
⟨ψ(γ),φ(θ)⟩=¯ψ(0)φ(0)−∫0−1∫θ0¯ψ(ξ−θ)dη(θ)φ(ξ)dξ, | (5.8) |
where η(θ)=η(θ,0). Then, we have A(0) and A∗ as adjoint operators. From the discussion in the previous section, we notice that ±iτ∗ω∗ are eigenvalues of A(0) and hence, they are also eigenvalues of A∗.
Let us assume that p(θ)=(p1,p2)Teiτ∗ω∗θ be the eigenvector of A(0) corresponding to the eigenvalue iτ∗ω∗. Then, we have A(0)p(θ)=iτ∗ω∗p(θ). Now, from the definition of A(0) we obtain
[−d1k2+A11−iω∗A12B21e−iτ∗ω∗−d2k2+A22+B22e−iτ∗ω∗−iω∗][p1p2]=[00]. |
Solving the above matrix equation, we have
p(θ)=(1,−d1k2−A11+iω∗A12)Teiτ∗ω∗θ. | (5.9) |
Similarly, let us assume that p∗(γ)=D(p∗1,p∗2)eiτ∗ω∗γ be the eigenvector of A∗ corresponding to the eigenvalue −iτ∗ω∗. Then, we have A∗p∗(γ)=−iτ∗ω∗p∗(γ). From the definition of A∗, we obtain
[−d1k2+A11+iω∗B21eiτ∗ω∗A12−d2k2+A22+B22eiτ∗ω∗+iω∗][p∗1p∗2]=[00]. |
Therefore, from the above matrix equation, we obtain
p∗(γ)=D(1,−−d1k2+A11+iω∗B21e−iτ∗ω∗)eiτ∗ω∗γ. | (5.10) |
In order to ensure ⟨p∗(γ),p(θ)⟩=1, we need to determine D. From the equation (5.8), we deduce that
⟨p∗(γ),p(θ)⟩=¯p∗(0)p(0)−∫0−1∫θξ=0¯p∗(ξ−θ)dη(θ)p(ξ)dξ=¯D{(¯p∗1,¯p∗2)(p1,p2)T−∫0−1∫θξ=0(¯p∗1,¯p∗2)e−iτ∗ω∗(ξ−θ)dη(θ)(p1,p2)Teiτ∗ω∗ξdξ}=¯D{¯p∗1p1+¯p∗2p2−∫0−1(¯p∗1,¯p∗2)θeiτ∗ω∗θdη(θ)(p1,p2)T}=¯D{¯p∗1p1+¯p∗2p2+τ∗e−iτ∗ω∗¯p∗2(B21p1+B22p2)}=¯D{1+¯p∗2p2+τ∗e−iτ∗ω∗¯p∗2(B21+B22p2)}. |
Therefore, we can take D as
D=11+p∗2¯p2+τ∗eiτ∗ω∗p∗2(B21+B22¯p2) |
such that ⟨p∗(γ),p(θ)⟩=1 and ⟨p∗(γ),¯p(θ)⟩=0.
For the rest of this section, we will closely follow the notations as described in [75]. Now, we compute the coordinates to describe the center manifold C0 at ς=0. For this purpose, we define
z(t)=⟨p∗,Ut⟩,W(t,θ)=Ut(θ)−2Re{z(t)p(θ)}. | (5.11) |
On the center manifold C0, we have
W(t,θ)=W(z(t),¯z(t),θ), |
where
W(z(t),¯z(t),θ)=W20(θ)z22+W11(θ)z¯z+W02(θ)¯z22+⋯, | (5.12) |
and z and ¯z represent local coordinates for the center manifold C0 in the direction of p∗ and ¯p∗, respectively. We are only concerned with the real solutions and note that W is real if Ut is real. For the solution Ut∈C0 of the equation (5.6), since ς=0 and using (5.11), we obtain
˙z(t)=⟨p∗,˙Ut⟩=iτ∗ω∗z+¯p∗(0)f(0,W(z,¯z,θ)+2Re{zp(θ)})≜iτ∗ω∗z+¯p∗(0)f0(z,¯z). | (5.13) |
Now, we rewrite the above equation (5.13) as follows:
˙z(t)=iτ∗ω∗z+g(z,¯z), | (5.14) |
where
g(z,¯z)=¯p∗(0)f0(z,¯z)=g20z22+g11z¯z+g02¯z22+g21z2¯z2+⋯. | (5.15) |
Also from the equations (5.11) and (5.12), we get
Ut(θ)=W(t,θ)+2Re{z(t)p(θ)}=W20(θ)z22+W11(θ)z¯z+W02(θ)¯z22+zp+¯z¯p+⋯=W20(θ)z22+W11(θ)z¯z+W02(θ)¯z22+(p1,p2)Teiτ∗ω∗θz+(¯p1,¯p2)Te−iτ∗ω∗θ¯z+⋯. | (5.16) |
Then, it follows that
g(z,¯z)=¯p∗(0)f0(z,¯z)=¯Dτ∗(¯p∗1,¯p∗2)[∑i+j≥21i!j!f(1)ijφi1(0)φj2(0)∑i+j+l≥21i!j!l!f(2)ijlφi1(−1)φj2(0)φl2(−1)]=b1z2+b2z¯z+b3¯z2+b4z2¯z+⋯, | (5.17) |
where
b1=¯Dτ∗{(12f(1)20+f(1)11p2)+¯p∗2(f(2)101p2e−2iτ∗ω∗+12f(2)200e−2iτ∗ω∗+12f(2)020p22)},b2=¯Dτ∗{(f(1)20+f(1)11(p2+¯p2))+¯p∗2(f(2)101(p2+¯p2)+f(2)200+f(2)020p2¯p2)},b3=¯Dτ∗{(12f(1)20+f(1)11¯p2)+¯p∗2(f(2)101¯p2e2iτ∗ω∗+12f(2)200e2iτ∗ω∗+12f(2)020¯p22)},b4=¯Dτ∗[{f(1)20(W(1)11(0)+12W(1)20(0))+f(1)11(W(2)11(0)+12W(2)20(0)+p2W(1)11(0)+12¯p2W(1)20(0))+12f(1)30+f(1)21(p2+12¯p2)}+¯p∗2{f(2)101(e−iτ∗ω∗W(2)11(−1)+12eiτ∗ω∗W(2)20(−1)+p2e−iτ∗ω∗W(1)11(−1)+12¯p2eiτ∗ω∗W(1)20(−1))+f(2)200(e−iτ∗ω∗W(1)11(−1)+12eiτ∗ω∗W(1)20(−1))+f(2)020(p2W(2)11(0)+12¯p2W(2)20(0))+12f(2)300e−iτ∗ω∗+f(2)201(p2+12¯p2)e−iτ∗ω∗}]. |
Now, comparing the coefficients of the equations (5.15) and (5.17), we obtain
g20=2b1,g11=b2,g02=2b3,andg21=2b4. | (5.18) |
We need to compute the values of W11(θ) and W20(θ) as the expression for g21 involves both W11(θ) and W20(θ) for θ∈[−1,0]. It follows from the equations (5.6) and (5.11) that
˙W=˙Ut−˙zp−˙¯z¯p={A(0)W−2Re{¯p∗(0)f0p(θ)},θ∈[−1,0),A(0)W−2Re{¯p∗(0)f0p(θ)}+f0,θ=0≜A(0)W+H(z,¯z,θ), | (5.19) |
where
H(z,¯z,θ)=H20(θ)z22+H11(θ)z¯z+H02(θ)¯z22+⋯. | (5.20) |
On the other hand, from the equation (5.12) on C0, we obtain
˙W=Wz˙z+W¯z˙¯z=(W20(θ)z+W11(θ)¯z+⋯)(iτ∗ω∗z(t)+g(z,¯z))+(W11(θ)z+W02(θ)¯z+⋯)(−iτ∗ω∗¯z(t)+¯g(z,¯z)). | (5.21) |
Also, using the expressions (5.12) and (5.20) in the equation (5.19), we obtain
˙W=A(0)(W20(θ)z22+W11(θ)z¯z+W02(θ)¯z22+⋯)+H20(θ)z22+H11(θ)z¯z+H02(θ)¯z22+⋯=(A(0)W20(θ)+H20(θ))z22+(A(0)W11(θ)+H11(θ))z¯z+(A(0)W02(θ)+H02(θ))¯z22+⋯. | (5.22) |
Now, comparing the coefficients of z2 and z¯z from the equations (5.21) and (5.22), we obtain
(A(0)−2iτ∗ω∗I2)W20(θ)=−H20(θ),A(0)W11(θ)=−H11(θ). | (5.23) |
For θ∈[−1,0), it follows from the equations (5.15) and (5.19) that
H(z,¯z,θ)=−2Re{¯p∗(0)f0(z,¯z)p(θ)}=−2Re{g(z,¯z)p(θ)}=−g(z,¯z)p(θ)−¯g(z,¯z)¯p(θ)=−(g20z22+g11z¯z+g02¯z22+⋯)p(θ)−(¯g20¯z22+¯g11z¯z+¯g02z22+⋯)¯p(θ). | (5.24) |
Again comparing the coefficients of z2 and z¯z from the equations (5.20) and (5.24), for θ∈[−1,0) we obtain
H20(θ)=−g20p(θ)−¯g02¯p(θ), | (5.25) |
H11(θ)=−g11p(θ)−¯g11¯p(θ). | (5.26) |
From the equations (5.23) and (5.25) and using the definition of A(θ), we deduce that
˙W20(θ)=A(0)W20(θ)=2iτ∗ω∗W20(θ)+g20p(θ)+¯g02¯p(θ). | (5.27) |
The relation p(θ)=(p1,p2)Teiτ∗ω∗θ=p(0)eiτ∗ω∗θ leads the equation (5.27) to the following first order linear ordinary differential equation in W20(θ):
˙W20(θ)=2iτ∗ω∗W20(θ)+g20p(0)eiτ∗ω∗θ+¯g02¯p(0)e−iτ∗ω∗θ. | (5.28) |
Solving the above differential equation (5.28) for W20(θ), we obtain
W20(θ)=ig20τ∗ω∗p(0)eiτ∗ω∗θ+i¯g023τ∗ω∗¯p(0)e−iτ∗ω∗θ+V1e2iτ∗ω∗θ, | (5.29) |
where V1=(V(1)1,V(2)1)∈R2 denotes a two-dimensional constant vector. In a similar manner, from the equations (5.23) and (5.26) and using the definition of A(θ), we deduce that
W11(θ)=−ig11τ∗ω∗p(0)eiτ∗ω∗θ+i¯g11τ∗ω∗¯p(0)e−iτ∗ω∗θ+V2, | (5.30) |
where V2=(V(1)2,V(2)2)∈R2 also denotes a two-dimensional constant vector. Next, we need to find the appropriate values of the constant vectors V1 and V2. From the definition of A(0) and (5.23), we derive
∫0−1dη(θ)W20(θ)=2iτ∗ω∗W20(0)−H20(0), | (5.31) |
and
∫0−1dη(θ)W11(θ)=−H11(0), | (5.32) |
where η(θ)=η(θ,0). Taking θ=0, we obtain from the equation (5.19) that
H(z,¯z,0)=−2Re{¯p∗(0)f0(z,¯z)p(0)}+f0(z,¯z)=−g(z,¯z)p(0)−¯g(z,¯z)¯p(0)+f0(z,¯z)=−(g20z22+g11z¯z+g02¯z22+⋯)p(0)−(¯g20¯z22+¯g11z¯z+¯g02z22+⋯)¯p(0)+f0(z,¯z). | (5.33) |
Then, we have
H20(0)z22+H11(0)z¯z+H02(0)¯z22+⋯=−(g20z22+g11z¯z+g02¯z22+⋯)p(0)−(¯g20¯z22+¯g11z¯z+¯g02z22+⋯)¯p(0)+f0(z,¯z). | (5.34) |
Comparing the coefficients of z2 and z¯z from the both sides of the above equation (5.34), we obtain
H20(0)=−g20p(0)−¯g02¯p(0)+τ∗(ρ1,ρ2)T, | (5.35) |
H11(0)=−g11p(0)−¯g11¯p(0)+τ∗(ρ3,ρ4)T, | (5.36) |
where (ρ1,ρ2)T and (ρ3,ρ4)T represent the coefficients of z2 and z¯z of f0(z,¯z). Then, we have
ρ1=f(1)20+2f(1)11p2,ρ2=2f(2)101p2e−2iτ∗ω∗+f(2)200e−2iτ∗ω∗+f(2)020p22,ρ3=f(1)20+f(1)11(p2+¯p2),ρ4=f(2)101(p2+¯p2)+f(2)200+f(2)020p2¯p2. |
Since iτ∗ω∗ represents the eigenvalue of A(0) and p(0) denotes the corresponding eigenvector, we have
(iτ∗ω∗I2−∫0−1eiτ∗ω∗θdη(θ))p(0)=0, | (5.37) |
(−iτ∗ω∗I2−∫0−1e−iτ∗ω∗θdη(θ))¯p(0)=0. | (5.38) |
Now, substituting the equations (5.29) and (5.35) in equation (5.31) yields
(2iτ∗ω∗I2−∫0−1e2iτ∗ω∗θdη(θ))V1=τ∗(ρ1,ρ2)T, | (5.39) |
which eventually leads to
[2iω∗+d1k2−A11−A12−B21e−iτ∗ω∗2iω∗+d2k2−A22−B22e−iτ∗ω∗][V(1)1V(2)1]=[ρ1ρ2]. | (5.40) |
Solving the above matrix equation (5.40), we obtain
V(1)1=1△1|ρ1−A12ρ22iω∗+d2k2−A22−B22e−iτ∗ω∗|, |
and
V(2)1=1△1|2iω∗+d1k2−A11ρ1−B21e−iτ∗ω∗ρ2|, |
where
△1=|2iω∗+d1k2−A11−A12−B21e−iτ∗ω∗2iω∗+d2k2−A22−B22e−iτ∗ω∗|. |
In a similar manner, substituting the equations (5.30) and (5.36) in equation (5.32) we obtain
[d1k2−A11−A12−B21d2k2−A22−B22][V(1)2V(2)2]=[ρ3ρ4]. | (5.41) |
Solving the above matrix equation (5.41), we obtain
V(1)2=1△2|ρ3−A12ρ4d2k2−A22−B22|,V(2)2=1△2|d1k2−A11ρ3−B21ρ4|, |
where
△2=|d1k2−A11−A12−B21d2k2−A22−B22|. |
Thus, we can determine W20(θ) and W11(θ) from the equations (5.29) and (5.30). Furthermore, we can also compute g21. In order to determine properties of Hopf-bifurcating periodic solution of the system (2.1)-(2.4) at the critical value τ∗ on the center manifold, we compute the following quantities:
C1(0)=i2τ∗ω∗(g20g11−2|g11|2−|g02|23)+g212,ζ2=−Re{C1(0)}Re{μ′(τ∗)},β2=2Re{C1(0)},T2=−Im{C1(0)}+ζ2Im{μ′(τ∗)}τ∗ω∗. |
Here, ζ2 determines the direction of Hopf bifurcation, β2 determines the stability of the bifurcating periodic solution and T2 determines the period of the Hopf-bifurcating periodic solution. Therefore, following the results presented in [75], we summarize the properties of the Hopf bifurcation at the critical value τ∗ in the following theorem:
Theorem 5.1. Let us assume that the system (2.1)-(2.4) undergoes Hopf bifurcation at the coexisting steady state E∗=(N∗,P∗) when τ=τ∗. Then the following results hold:
(i) If ζ2>0(ζ2<0), then the Hopf bifurcation is forward (backward) and the bifurcating periodic solutions exist for τ>τ∗(τ<τ∗).
(ii) If β2<0(β2>0), then the bifurcating periodic solutions are stable (unstable).
(iii) If T2>0(T2<0), then the period of the periodic solutions increases (decreases).
Remark 5.1. In order to numerically compute the formulae derived in this section regarding the properties of the Hopf-bifurcating periodic solutions, we have to make use of the non-negative k-value for which we will get the Hopf bifurcation threshold τ∗ for a particular parameter set. Further, to match the numerical results presented in the preceding section, we have calculated the numerical values for these formulae. Our computations give C1(0)=−0.8435−0.3956i, ζ2=26.3009>0, β2=−1.6870<0 and T2=6.0453>0. Detailed computations of these quantities are presented in Appendix Ⅱ. Therefore, we can expect that the Hopf bifurcation is forward in direction, i.e., the bifurcating periodic solutions arise for τ>τ∗0; emerging periodic solutions are stable and their period increase. These conclusions can be easily verified from the figures presented in the preceding section.
In this section, we carry out numerical simulations in order to demonstrate the impact of gestation delay on the spatial structures exhibited by the prey and predator populations and to corroborate the theoretical results obtained. Numerical simulations were performed over a square spatial domain Ω=[0,200]×[0,200] along with grid sizes △x=1.0 and △y=1.0. We have considered time increment as △t=0.01 which is adequate in taking care of the numerical artifacts. For each numerical result, we have used small random perturbations about the homogeneous coexistence steady state as initial population distribution by using the usual Gaussian white noise terms. For the numerical integration of the reaction part, we employ the first order Euler scheme [38,59]. We discretize the Laplacian term at the lattice site (i,j) by the following formula
ΔN(i,j)=1(△x)2[Cl(i,j)N(i−1,j)+Cr(i,j)N(i+1,j)+Cd(i,j)N(i,j−1)+Cu(i,j)N(i,j+1)−4N(i,j)], |
where the matrix elements of Cl, Cr, Cd and Cu take the value 1 except at the boundary [59]. Further in order to guarantee the zero-flux boundary conditions, we define Cl(i,j)N(i−1,j)≡N(i+1,j) and we use similar definitions for Cr, Cd and Cu [59]. As we have observed that both the prey and predator populations exhibit patterns with the similar qualitative property and a change in scale, we chose to present the patterns only for the prey population. Also, in this section we restrict ourselves only to the case of strong Allee effect in prey growth. For this reason, we chose the following parameter values: r=1.0, K=10.0, m=1.0, b=0.5, c=1.0, q=0.35, δ=0.0425, s=2.0, d1=0.15 and d2=10.0 [20,24]. Here we would like to mention that we have chosen the value of the parameter b different from the value taken in [20,24] in order to make Ncrit visible better. For these parameter values, we obtain b2rK=0.025, br=0.5 and r2(K−b)2+4br2K4rK=2.756. Therefore, our choice of the parameter value m=1.0 gives rise to the strong Allee effect in prey growth.
Firstly, we aim to provide numerical results which support our theoretical findings on the Hopf bifurcation and the associated properties derived in the preceding sections. For this purpose, we have considered a=4.0 along with the other parameter values mentioned above. In this case, the corresponding non-delayed spatial system exhibits spatially homogeneous distribution of both the populations at the homogeneous coexistence steady state E∗=(3.665,3.015). For the sake of brevity, we restrict ourselves from displaying these numerical results. Thus, this choice of the parameter values acts as a desired parameter set to determine the Hopf bifurcation threshold induced by τ numerically. Our numerical investigations suggest that for lower values of τ, the system (2.1)-(2.4) retains the homogeneous distribution of both the populations as it was the case for the non-delayed system and we chose not to display them here. Gradually increasing the value of τ, we have achieved the space independent periodic in time population distribution through Hopf bifurcation. In order to check this space independence of the periodic distributions, we present the plots of the limit cycles for τ=1.7 in case of spatially averaged densities and densities at a particular spatial location (100,100) in Figure 3. From these two figures, we can easily observe that the limit cycles are exactly the same in both the cases. Also, one can get similar results for the plots of the density of prey against that of predator at other spatial locations instead of (100,100). This eventually confirms the space independence of the periodic patterns. Through numerical simulations we have arrived at the Hopf bifurcation threshold which is approximately τ∗=1.275 in this case and it is actually the same as the threshold τ∗0 for the corresponding non-spatial system. Therefore, in this case we can directly compute τ∗ by taking k=0 and any positive k does not have any role in determining τ∗. For the chosen parameter set, non-delayed counterpart of the considered spatial model and its non-spatial version exhibit that the coexistence steady state is stable in both the cases. Therefore, introduction of diffusion in non-delayed temporal model does not play any prominent role in the resulting dynamics for this set of parameter values. This is exactly the reason why any positive k does not contribute in determining the threshold τ∗ for the considered spatial model. Therefore, computations of the quantities regarding the properties of the Hopf bifurcation given in Section 5 follow directly from the computed values given in Remark 2 in this case. Hence, we can conclude from the computed values of the relevant quantities that the Hopf bifurcation is forward, i.e., the bifurcating periodic solutions emerge when τ>1.275, and these solutions are stable for our delayed spatial model.
In order to compare these periodic solutions emerging for the spatial model with that of the corresponding non-spatial model, we present Figure 4. Figure 4(a) shows that the limit cycles in both the cases are almost the same whereas Figures 4(b) and (c) exhibits the small deviations between these two limit cycles in different regions. We remark that this slight differences in these two limit cycles arise due to the presence of the spatial components. Even bigger value of τ eventually leads to the spatiotemporal chaotic pattern and these scenarios are depicted in Figure 5. Figure 5(a) exhibits the chaotic pattern for the prey population and Figures 5(b) and (c) give us assurance regarding the chaotic nature of this pattern. Also, we have cross-checked the chaotic patterns by examining the sensitivity to initial distributions, estimation of Lyapunov exponent and power spectrum [78,79,80,81] but did not present the results here for the sake of briefness. All these transitions are clearly visible from the bifurcation diagram presented in Figure 6. This bifurcation diagram (that is, Figure 6) has been prepared by plotting the global maximum and minimum of the average prey density for stable homogeneous and periodic solutions and the local maxima and minima of the average prey density for chaotic solutions after discarding the initial transients [57]. Also, from this figure we can observe that if τ is sufficiently large beyond the Hopf bifurcation threshold τ∗ (or τ∗0) but less than the heteroclinic bifurcation threshold τHO then the periodic solution for the corresponding non-spatial system turns out to be chaotic for the spatial counterpart. For this parameter set, chaotic distribution for both the populations emerges when τ>1.96. Comparing Figure 2 with Figure 6, we can easily notice that incorporation of diffusion enhances the persistence of both the species as in the τ-range where the non-spatial system exhibits extinction scenario for both the species, the corresponding spatial system leads to the chaotic coexistence.
Now we investigate numerically the impact of gestation delay on the stationary Turing patterns. For this reason, we chose the parameter value a=3.5 along with all other parameter values the same as above. From the conditions for Turing instability presented in [20,24], one can easily compute that these parameter values lie inside the Turing domain. A brief discussion about the analytical conditions for Turing instability and the justification for the fact that these parameter values lie inside the Turing domain of the non-delayed spatial system are presented in Appendix Ⅰ. For these parameter values, the non-delayed version of the system (2.1)-(2.4) exhibits stationary cold spot patterns for both the species. But for the sake of brevity of this paper, we did not incorporate these patterns here. Figure 7 represents the effect of delay on this stationary cold spot pattern. From Figure 7(a), we can observe that for lower values of τ the considered delayed system (2.1)-(2.4) preserves the cold spot pattern. If we increase the value of τ this cold spot pattern changes into mixture of stripes and cold spots pattern which is exhibited in Figure 7(b). Both these patterns are stationary in nature which can be noticed in Figures 8(a) and (b) for the corresponding temporal evolution of the spatial average densities of both the species. Also, we would like to mention here that for the stationary patterns of both the populations we have noticed almost one-to-one correspondence and accordingly we exhibit only the patterns for the prey population. Another interesting dynamical feature arises in this transition of one stationary pattern to another which is termed as the "loss of monotonicity". This feature is evident from the Figures 8(a) and (b). In Figure 8(a), we can see that the spatial average densities of both the populations approaches the stationary state monotonically (monotonically increasing in case of prey population and monotonically decreasing in case of predator population). Whereas in case of the stationary mixture pattern, there are initial fluctuations in the temporal evolution of the spatial average densities of both the populations before settling to the stationary state and this can be observed in the Figure 8(b). Therefore, we conclude that the transition of stationary patterns for our considered system (2.1)-(2.4) happens through the loss of monotonicity of the spatial average densities. Further gradual increments in τ leads to quasi-periodic and spatiotemporal chaotic patterns for both the populations respectively. These scenarios can be observed in Figures 7(c) and (d) for the prey population. The corresponding spatial average densities of both the populations are exhibited in Figures 8(c) and (d). In order to characterize these patterns efficiently we have incorporated the corresponding phase diagrams of the spatial average densities in Figure 9. Since the phase diagram presented in Figure 9(a) shows that the spatially averaged solution trajectory stays confined in an annular region, we have termed the corresponding pattern as the quasi-periodic pattern though it looked quite similar to a stationary pattern. Also, the annular domain will not change with the increase in the length of time interval. At this end, we would like to specify that all the patterns reported in this paper are self-organized as we have studied the considered system in homogeneous environment and there are no other external interferences. For the other choices of the parameter values in the Turing domain for the corresponding non-delayed spatial system, we have observed similar kind of transition of stationary patterns to spatiotemporal chaotic patterns due to the effect of the gestation delay. But we did not incorporate them here deliberately in order to make this paper concise.
Various research works were carried out to derive analytical expressions for the relevant quantities to determine the stability of the Hopf-bifurcating periodic solutions for delayed temporal and spatiotemporal prey-predator models [30,39,40,41,46,48,50,51,54,55,56,58,59,60]. In some of them, preliminary numerical simulations were carried out to explore the change in pattern formation scenario due to the presence of time delay. So far as our knowledge goes, there is no literature where the analytical results for the stability of Hopf-bifurcating periodic solutions for the non-spatial and spatial models have been verified numerically and the results are compared with the help of bifurcation diagrams for these two types of models.
In this paper, we have considered a delayed spatial prey-predator system with monotonic functional response with saturation to describe grazing phenomena and density dependent death rate for predator along with the additive Allee effect in prey growth. The consideration of density dependent death rate for predator is a realistic assumption and it is crucial for pattern formation as it allows the system to satisfy Turing instability conditions for certain choice of parameter values while models without it cannot produce Turing patterns [82]. The non-delayed version of our model cannot produce Turing patterns in absence of density dependent death rate for predators (i.e., δ=0) [24]. Also, one can go through the Note presented in Appendix Ⅰ for a brief justification of this observation. But it produces various kinds of Turing patterns in presence of density dependent death rate for predators (i.e., δ>0) [20,24]. We have incorporated the discrete time delay in the predator's growth to take care of gestation time period for predator species and studied the effect of time delay on the spatiotemporal pattern formation. The well-posedness of the problem in terms of existence, uniqueness, non-negativity and boundedness of the solutions has been established to ensure the mathematical model is appropriate. Theoretically we have derived the Hopf bifurcation threshold for the model under suitable assumptions and computed the analytical expressions in order to determine the properties of Hopf bifurcating periodic solutions with the help of center manifold reduction and normal form theory with discrete time delay as bifurcation parameter. Although the computed results are for spatial system, we have discussed on how one can actually obtain the associated results for the corresponding non-spatial system by taking k appropriately (i.e., k=0). Accordingly, we have discussed the Hopf bifurcation threshold and the corresponding properties of the limit cycle generated through Hopf bifurcation. Also, we have observed another bifurcation, which is a global bifurcation, the heteroclinic bifurcation for larger value of delay through which the periodic solution disappears and we have found the extinction scenario for both the species in the non-spatial model. Further, numerical illustrations have been provided in order to corroborate our theoretical results and to investigate the impact of time delay on the stationary patterns.
Our present study confirms that the stable Hopf bifurcating periodic solution changes to homogeneous in space and oscillatory in time solution and remains stable in the presence of spatial component. Further, we observe the emergence of the spatiotemporal chaotic distribution for both the species in presence of sufficiently large value of the gestation delay. Time delay changes the spatially uniform distribution of the populations to chaotic distribution through the periodic distribution. This periodic distribution emerges through Hopf bifurcation with time delay as the bifurcation parameter. Delay induced spatiotemporal pattern and enhancement of parametric domain for spatiotemporal chaotic pattern are available in literature [36,37,38,59] but its emergence from Hopf-bifurcating periodic solution is not well explained in the existing literature. For the model under consideration and for chosen parameter values, we have observed Hopf bifurcation threshold for the spatial system is the same as that for the corresponding non-spatial system. This phenomenon occurs since for the chosen parameter values the corresponding non-delayed temporal system admits asymptotically stable unique coexistence steady state and the corresponding non-delayed spatial system exhibits uniform distribution of populations at this stable steady state. Therefore, incorporation of diffusion does not play a prominent role on the destabilization of the dynamics when the parameter values are away from the Hopf bifurcation threshold for the temporal model and Turing bifurcation threshold for the spatial model. Hence, the Hopf bifurcation threshold (which has been calculated by considering k=0) is the same in both non-spatial and spatial models. In this regard, we remark that positive k-values come into picture in determining the Hopf bifurcation threshold when the unstable positive steady state for the non-delayed temporal system is stabilized by the incorporation of spatial components into the model. Also, our numerical results have indicated that incorporation of spatial components enhances the coexistence for both the species and this claim can be easily verified by comparing the results presented in the Figures 2 and 6. From these two figures, one can easily understand that the chaotic coexistence results in the spatial model where the corresponding non-spatial counterpart exhibits extinction of both the species. This chaotic coexistence appears in the spatial model due to the localized disappearance and restoration at other nearby favorable patches in the natural habitat. Another interesting fact is that we have obtained the chaotic pattern for much higher value of the ratio of predator's diffusion coefficient to that of the prey population. And we can safely associate the reason for this phenomenon to the presence of the time delay as it is generally a common practice in literature to take this ratio to be equal to unity (especially d1=d2=1) in order to produce the chaotic distribution of populations [6,8,20,78,83].
Another interesting finding in this study is that, apart from the emergence of the chaotic patterns, time delay can actually alter a stationary pattern to another stationary pattern. For smaller values of the time delay the considered system retains the stationary pattern exhibited by the corresponding non-delayed system in its Turing domain. With the increment in time delay the stationary pattern gets converted into another one which eventually turns into chaotic pattern for sufficiently large time delay. In our study, we have shown this transition where cold spot pattern turns into a stationary mixture pattern (mixture of cold spots and stripes). In this case, the mixture pattern eventually settles into a chaotic pattern through the quasi-periodic one with the increase in the magnitude of time delay. Further, we have identified the loss of monotonicity property for the spatially averaged densities in this transition from one stationary pattern to another. Generally, it is believed that both the local and external factors acts together in shaping the spatial pattern formation for ecological community [84,85]. By chemical-physical limitations, environmental heterogeneity plays a crucial role in shaping population dynamics as one of the most common external component [86]. However, there exist sufficient amount of empirical manifestations where it is often very difficult to justify the observed spatial patterns through environmental heterogeneity solely [57,87,88]. This fact eventually leads to the well-known concept of "self-organized" spatiotemporal pattern formation. This type of patterns emerges due to internal factors alone in an absolutely homogeneous environment [3,83,89,90,91]. Therefore, all the patterns reported in this study has been classified as the self-organized patterns since we have considered our system in a uniform environment and in absence of any other external factors like periodic and/or fluctuating environmental conditions and stochasticity.
The works presented in this paper give rise to various relevant and interesting problems to investigate regarding prey and predator interactions. An immediate future direction is to study the problem with predator density dependent functional responses, namely, Beddington-DeAngelis and Michaelis-Menten types of functional responses. Another important and interesting problem will be to consider the system in presence of both time delay and external influencing factors (especially the environmental heterogeneity) and study the corresponding dynamical behaviors. Also in reality, predator's movement is generally guided by some specific ingredients passed off by prey species such as pheromones and odour etc. [92]. Therefore, it will be a problem of immense potential to study the problem presented in this paper in presence of prey-taxis. We will take up and explore these problems as our future studies.
The first author gratefully acknowledges the financial support provided by Science and Engineering Research Board, Government of India for pursuing his post-doctoral research. The authors express their gratitude to the learned reviewers for their insightful comments and suggestions.
The authors declare there is no conflict of interest.
[1] | G. F. Gause, The Struggle for Existence, Williams and Wilkins: Baltimore, MD, USA, 1935. |
[2] | A. M. Turing, The chemical basis of morphogenesis, Philos. Trans. R. Soc. Lond., B, Biol. Sci., 237 (1952), 37–72. |
[3] | S. A. Levin and L. A. Segel, Hypothesis for origin of planktonic patchiness, Nature, 259 (1976), 659. |
[4] | C. A. Klausmeier, Regular and irregular patterns in semiarid vegetation, Science, 284 (1999), 1826–1828. |
[5] | H. Malchow, S. V. Petrovskii and E. Venturino, Spatiotemporal Patterns in Ecology and Epidemiology: Theory, Models, and Simulations, Chapman & Hall, London, 2008. |
[6] | S. V. Petrovskii and H. Malchow, A minimal model of pattern formation in a prey-predator system, Math. Comput. Model., 29 (1999), 49–63. |
[7] | D. Alonso, F. Bartumeus and J. Catalan, Mutual interference between predators can give rise to Turing spatial patterns, Ecology, 83 (2002), 28–34. |
[8] | M. Banerjee and S. Petrovskii, Self-organized spatial patterns and chaos in a ratio-dependent predator-prey system, Theor. Ecol., 4 (2011), 37–53. |
[9] | B. Miao, Persistence and Turing instability in a cross-diffusive predator-prey system with generalist predator, Adv. Differ. Equ., 2018 (2018), 260. |
[10] | A. B. Medvinsky, B. V. Adamovich and A. Chakraborty, et al., Chaos far away from the edge of chaos: A recurrence quantification analysis of plankton time series, Ecol. Complex., 23 (2015), 61–67. |
[11] | P. Turchin and S. P. Ellner, Living on the edge of chaos: Population dynamics of Fennoscandian voles, Ecology, 81 (2000), 3099–3116. |
[12] | W. C. Allee, Animal aggregations: A study in general sociology, University of Chicago Press, Chicago, USA, 1931. |
[13] | B. Dennis, Allee effect: population growth, critical density, and chance of extinction, Nat. Resour. Model., 3 (1989), 481–538. |
[14] | P. A. Stephens, W. J. Sutherland and R. P. Freckleton, What is the Allee effect? Oikos, 87 (1999), 185–190. |
[15] | M. A. Lewis and P. Kareiva, Allee Dynamics and the Spread of Invading Organisms, Theor. Popul. Biol., 43 (1993), 141–158. |
[16] | E. Odum and G. W. Barrett, Fundamentals of Ecology, Thomson Brooks/Cole, Belmont, CA, 2004. |
[17] | J. Wang, J. Shi and J. Wei, Predator-prey system with strong Allee effect in prey, J. Math. Biol., 62 (2011), 291–331. |
[18] | P. Aguirre, E. González-Olivares and E. Sáez, Two limit cycles in a Leslie-Gower predator-prey model with additive Allee effect, Nonlinear Anal. Real World Appl., 10 (2009), 1401–1416. |
[19] | P. Aguirre, E. González-Olivares and E. Sáez, Three Limit Cycles in a Leslie-Gower Predator-Prey Model with Additive Allee Effect, SIAM J. Appl. Math., 69 (2009), 1244–1262. |
[20] | K. Manna and M. Banerjee, Stationary, non-stationary and invasive patterns for a prey-predator system with additive Allee effect in prey growth, Ecol. Complex., 36 (2018), 206–217. |
[21] | Y. Du and J. Shi, Allee effect and bistability in a spatially heterogeneous predator-prey model, Trans. Am. Math. Soc., 359 (2007), 4557–4593. |
[22] | J. Wang, J. Shi and J. Wei, Dynamics and pattern formation in a diffusive predator-prey system with strong Allee effect in prey, J. Differ. Equ., 251 (2011), 1276–1304. |
[23] | Y. Cai, W. Wang and J. Wang, Dynamics of a diffusive predator-prey model with additive Allee effect, Int. J. Biomath., 5 (2012), 1250023(11 pages). |
[24] | Y. Cai, M. Banerjee and Y. Kang, et al., Spatiotemporal complexity in a predator-prey model with weak Allee effects, Math. Biosci. Eng., 11 (2014), 1247–1274. |
[25] | F. Rao and Y. Kang, The complex dynamics of a diffusive prey-predator model with an Allee effect in prey, Ecol. Complex., 28 (2016), 123–144. |
[26] | K. Gopalsamy, Stability and Oscillations in Delay Differential Equations of Population Dynamics, Kluwer Academic, Dordrecht, 1992. |
[27] | Y. Kuang, Delay Differential Equations with Applications in Population Dynamics, Academic Press, New York, 1993. |
[28] | R. M. May, Time-delay versus stability in population models with two and three trophic levels, Ecology, 54 (1973), 315–325. |
[29] | S. Ruan, On nonlinear dynamics of predator-prey models with discrete delay, Math. Model. Nat. Pheno., 4 (2009), 140–188. |
[30] | P. J. Pal, T. Saha and M. Sen, et al., A delayed predator-prey model with strong Allee effect in prey population growth, Nonlinear Dyn., 68 (2012), 23–42. |
[31] | S. Roy Choudhury, Turing instability in competition models with delay I: Linear theory, SIAM J. Appl. Math., 54 (1994), 1425–1450. |
[32] | S. Roy Choudhury, Analysis of spatial structure in a predator-prey model with delay II: Nonlinear theory, SIAM J. Appl. Math., 54 (1994), 1451–1467. |
[33] | K. P. Hadeler and S. Ruan, Interaction of diffusion and delay, Discrete Contin. Dyn. Syst. Ser. B, 8 (2007), 95–105. |
[34] | S. Sen, P. Ghosh and S. S. Riaz, et al., Time-delay-induced instabilities in reaction-diffusion systems, Phys. Rev. E, 80 (2009), 046212. |
[35] | S. Chen and J. Shi, Stability and Hopf bifurcation in a diffusive logistic population model with nonlcal delay effect, J. Differ. Equ., 253 (2012), 3440–3470. |
[36] | C. Tian and L. Zhang, Delay-driven irregular spatiotemporal patterns in a plankton system, Phys. Rev. E, 88 (2013), 012713. |
[37] | M. Banerjee and L. Zhang, Influence of discrete delay on pattern formation in a ratio-dependent prey-predator model, Chaos Solitons Fractals, 67 (2014), 73–81. |
[38] | M. Banerjee and L. Zhang, Time delay can enhance spatio-temporal chaos in a prey-predator model, Ecol. Complex., 27 (2016), 17–28. |
[39] | Y. Song, Y. Peng and X. Zou, Persistence, Stability and Hopf bifurcation in a diffusive ratiodependent predator-prey model with delay, Int. J. Bifurc. Chaos, 24 (2014), 1450093. |
[40] | S. Chen, J. Shi and J. Wei, Global stability and Hopf bifurcation in a delayed diffusive Leslie- Gower predator-prey system, Int. J. Bifurc. Chaos, 22 (2012), 1250061. |
[41] | H. Fang, L. Hu and Y.Wu, Delay-induced Hopf bifurcation in a diffusive Holling-Tanner predatorprey model with ratio-dependent response and Smith growth, Adv. Differ. Equ., 2018 (2018), 285. |
[42] | T. Faria, Stability and bifurcation for a delayed predator-prey model and the effect of diffusion, J. Math. Anal. Appl., 254 (2001), 433–463. |
[43] | Z. Ge and Y. He, Diffusion effect and stability analysis of a predator-prey system descried by a delayed reaction-diffusion equations, J. Math. Anal. Appl., 339 (2008), 1432–1450. |
[44] | G. P. Hu andW. T. Li, Hopf bifurcation analysis for a delayed predator-prey system with diffusion effects, Nonlinear Anal. Real World Appl., 11 (2010), 819–826. |
[45] | J. Li, Z. Jin and G. Q. Sun, Periodic solutions of a spatiotemporal predator-prey system with additional food, Chaos Solitons Fractals, 91 (2016), 350–359. |
[46] | F. Rao, C. Castillo-Chavez and Y. Kang, Dynamics of a diffusion reaction prey-predator model with delay in prey: Effects of delay and spatial components, J. Math. Anal. Appl., 461 (2018), 1177–1214. |
[47] | S. Ruan and X. Q. Zhao, Persistence and Extinction in two species reaction-diffusion systems with delays, J. Differ. Equ., 156 (1999), 71–92. |
[48] | X. Tang and Y. Song, Stability, Hopf bifurcations and spatial patterns in a delayed diffusive predator-prey model with herd behavior, Appl. Math. Comput., 254 (2015), 375–391. |
[49] | B. Wang, A. L. Wang and Y. J. Liu, et al., Analysis of a spatial predator-prey model with delay, Nonlinear Dyn., 62 (2010), 601–608. |
[50] | C. Xu and S. Yuan, Spatial periodic solutions in a delayed diffusive predator-prey model with herd behavior, Int. J. Bifurc. Chaos, 25 (2015), 1550155. |
[51] | X. P. Yan, Stability and Hopf bifurcation for a delayed prey-predator system with diffusion effects, Appl. Math. Comput., 192 (2007), 552–566. |
[52] | R. Yang, H. Ren and X. Cheng, A diffusive predator-prey system with prey refuge and gestation delay, Adv. Differ. Equ., 2017 (2017), 158. |
[53] | J. F. Zhang, W. T. Li and X. P. Yan, Bifurcation and spatiotemporal patterns in a homogeneous diffusion-competition system with delays, Int. J. Biomath., 5 (2012), 1250049. |
[54] | J. Zhao and J. Wei, Persistence, Turing instability and Hopf bifurcation in a diffusive plankton system with delay and quadratic closure, Int. J. Bifurc. Chaos, 26 (2016), 1650047. |
[55] | W. Zuo, Global stability and Hopf bifurcations of a Beddington-DeAngelis type predator-prey system with diffusion and delays, Appl. Math. Comput., 223 (2013), 423–435. |
[56] | W. Zuo and J. Wei, Stability and Hopf bifurcation in a diffusive predator-prey system with delay effect, Nonlinear Anal. Real World Appl., 12 (2011), 1998–2011. |
[57] | M. Jankovic, S. Petrovskii and M. Banerjee, Delay driven spatiotemporal chaos in single species population dynamics models, Theor. Popul. Biol., 110 (2016), 51–62. |
[58] | Z. P. Ma, W. T. Li and X. P. Yan, Stability and Hopf bifurcation for a three-species food chain model with time delay and spatial diffusion, Appl. Math. Comput., 219 (2012), 2713–2731. |
[59] | C. Tian and L. Zhang, Hopf bifurcation analysis in a diffusive food-chain model with time delay, Comput. Math. Appl., 66 (2013), 2139–2153. |
[60] | G. X. Yang and J. Xu, Stability and Hopf bifurcation for a three-species reaction-diffusion predator-prey system with two delays, Int. J. Bifurc. Chaos, 23 (2013), 1350194. |
[61] | R. Arditi, L. F. Bersier and R. P. Rohr, The perfect mixing paradox and the logistic equation: Verhulst vs. Lotka, Ecosphere, 7 (2016), e01599. |
[62] | J. P. Gabriel, F. Saucy and L. F. Bersier, Paradoxes in the logistic equation? Ecol. Model., 185 (2005), 147–151. |
[63] | L. R. Ginzburg, Evolutionary consequences of basic growth equations, TREE, 7 (1992), 133. |
[64] | J. Mallet, The struggle for existence: How the notion of carrying capacity, K, obscures the links between demography, Darwinian evolution, and speciation, Evol. Ecol. Res., 14 (2012), 627–665. |
[65] | W. E. Fitzgibbon, Semilinear functional differential equations in Banach space, J. Differ. Equ., 29 (1978), 1–14. |
[66] | R. H. Martin and H. L. Smith, Abstract functional-differential equations and reaction-diffusion systems, Trans. Am. Math. Soc., 321 (1990), 1–44. |
[67] | R. H. Martin and H. L. Smith, Reaction-diffusion systems with time delays: Monotonicity, invariance, comparison and convergence, J. reine angew. Math., 413 (1991), 1–35. |
[68] | C. C. Travis and G. F. Webb, Existence and stability for partial functional differential equations, Trans. Am. Math. Soc., 200 (1974), 395–418. |
[69] | J. Wu, Theory and Applications of Partial Functional Differential Equations, Springer-Verlag, New York, 1996. |
[70] | Z. Wu, J. Yin and C. Wang, Elliptic and Parabolic Equations, World Scientific, 2006. |
[71] | M. H. Protter and H. F.Weinberger, Maximum Principles in Differential Equations, Prentice Hall, Englewood Cliffs, 1967. |
[72] | M. Sen, M. Banerjee and E. Venturino, A model for biological control in agriculture, Math. Comput. Simul., 87 (2013), 30–44. |
[73] | X. Song and L. Chen, Optimal harvesting and stability for a two-species competitive system with stage structure, Math. Biosci., 170 (2001), 173–186. |
[74] | D. Henry, Geometric Theory of Semilinear Parabolic Equations, Lecture Notes in Mathematics, Vol. 840, Springer-Verlag, Berlin, New York, 1981. |
[75] | B. Hassard, N. Kazarinoff and Y. Wan, Theory and applications of Hopf bifurcation, Cambridge University Press, Cambridge, 1981. |
[76] | H. I. Freedman and V. S. H. Rao, The trade-off between mutual interference and time lags in predator-prey system, Bull. Math. Biol., 45 (1983), 991–1004. |
[77] | T. Faria, Normal forms and Hopf bifurcation for partial differential equations with delays, Trans. Am. Math. Soc., 352 (2000), 2217–2238. |
[78] | A. Morozov, S. Petrovskii and B. L. Li, Spatiotemporal complexity of patchy invasion in a predator-prey system with the Allee effect, J. Theor. Biol., 238 (2006), 18–35. |
[79] | N. Mukherjee, S. Ghorai and M. Banerjee, Effects of density dependent cross-diffusion on the chaotic patterns in a ratio-dependent prey-predator model, Ecol. Complex., 36 (2018), 276–289. |
[80] | M. Pascual, Diffusion-induced chaos in a spatial predator-prey system, Proc. R. Soc. Lond., B, Biol. Sci., 251 (1993), 1–7. |
[81] | A. Wolf, J. B. Swift and H. L. Swinney, et al., Determining Lyapunov exponents from a time series, Physica D, 16 (1985), 285–317. |
[82] | M. Baurmann, T. Gross and U. Feudel, Instabilities in spatially extended predator-prey systems: Spatio-temporal paterns in the neighborhood of Turing-Hopf bifurcations, J. Theor. Biol., 245 (2007), 220–229. |
[83] | S. V. Petrovskii and H. Malchow, Wave of chaos: New mechanism of pattern formation in spatiotemporal population dynamics, Theor. Popul. Biol., 59 (2001), 157–174. |
[84] | E. Ranta, V. Kaitala and J. Lindström, et al., Synchrony in population dynamics, Proc. R. Soc. Lond., B, Biol. Sci., 262 (1995), 113–118. |
[85] | M. Rietkerk and J. van de Koppel, Regular pattern formation in real ecosystems, Trends Ecol. Evol., 23 (2008), 169–175. |
[86] | P. Kareiva, A. Mullen and R. Southwood, Population dynamics in spatially complex environments: Theory and data (and discussion), Philos. Trans. R. Soc. Lond., B, Biol. Sci., 330 (1990), 175–190. |
[87] | T. M. Powell, P. J. Richerson and T. M. Dillon, et al., Spatial scales of current speed and phytoplankton biomass fluctuations in Lake Tahoe, Science, 189 (1975), 1088–1090. |
[88] | A. A. Sharov, A. M. Liebhold and E. A. Roberts, Correlation of counts of gypsy moths (Lepidoptera: Lymantriidae) in pheromone traps with landscape characteristics, Forest Sci., 43 (1997), 483–490. |
[89] | A. Okubo, Diffusion and Ecological Problems: Mathematical Models, Springer-Verlag, Berlin, 1980. |
[90] | A. Okubo and S. A. Levin, Diffusion and Ecological Problems: Modern Perspectives, Springer, Berlin, 2001. |
[91] | L. A. Segel and J. L. Jackson, Dissipative structure: An explanation and an ecological example, J. Theor. Biol., 37 (1972), 545–559. |
[92] | Y. V. Tyutyunov, L. I. Titova and I. N. Senina, Prey-taxis destabilizes homogeneous stationary state in spatial Gause-Kolmogorov-type model for predator-prey system, Ecol. Complex., 31 (2017), 170–180. |
[93] | J. D. Murray, Mathematical Biology, Springer, Heidelberg, 1989. |
1. | Jingen Yang, Sanling Yuan, Tonghua Zhang, Complex dynamics of a predator–prey system with herd and schooling behavior: with or without delay and diffusion, 2021, 0924-090X, 10.1007/s11071-021-06343-0 | |
2. | Kalyan Manna, Swadesh Pal, Malay Banerjee, Analytical and numerical detection of traveling wave and wave-train solutions in a prey–predator model with weak Allee effect, 2020, 100, 0924-090X, 2989, 10.1007/s11071-020-05655-x | |
3. | Sourav Kumar Sasmal, Yasuhiro Takeuchi, Editorial: Mathematical Modeling to Solve the Problems in Life Sciences, 2020, 17, 1551-0018, 2967, 10.3934/mbe.2020167 | |
4. | Yongli Song, Yahong Peng, Tonghua Zhang, The spatially inhomogeneous Hopf bifurcation induced by memory delay in a memory-based diffusion system, 2021, 300, 00220396, 597, 10.1016/j.jde.2021.08.010 | |
5. | Balram Dubey, Sourav Kumar Sasmal, Anand Sudarshan, Consequences of fear effect and prey refuge on the Turing patterns in a delayed predator–prey system, 2022, 32, 1054-1500, 123132, 10.1063/5.0126782 | |
6. | Huimiao Dong, Tiansi Zhang, Xingbo Liu, BIFURCATIONS OF DOUBLE HETERODIMENSIONAL CYCLES WITH THREE SADDLE POINTS, 2022, 12, 2156-907X, 2143, 10.11948/20210082 | |
7. | Mengxin Chen, Qianqian Zheng, Ranchao Wu, Liping Chen, Hopf bifurcation in delayed nutrient-microorganism model with network structure, 2022, 16, 1751-3758, 1, 10.1080/17513758.2021.2020915 | |
8. | Malay Banerjee, Swadesh Pal, Pranali Roy Chowdhury, Stationary and non-stationary pattern formation over fragmented habitat, 2022, 162, 09600779, 112412, 10.1016/j.chaos.2022.112412 | |
9. | Yingzi Liu, Zhong Li, Mengxin He, Bifurcation analysis in a Holling-Tanner predator-prey model with strong Allee effect, 2023, 20, 1551-0018, 8632, 10.3934/mbe.2023379 | |
10. | R. Lavanya, S. Vinoth, K. Sathiyanathan, Zeric Njitacke Tabekoueng, P. Hammachukiattikul, R. Vadivel, Kenan Yildirim, Dynamical Behavior of a Delayed Holling Type-II Predator-Prey Model with Predator Cannibalism, 2022, 2022, 2314-4785, 1, 10.1155/2022/4071375 | |
11. | Balram Dubey, Spatiotemporal dynamics of a multi-delayed prey–predator system with variable carrying capacity, 2023, 33, 1054-1500, 10.1063/5.0173566 | |
12. | Ting Yu, Qinglong Wang, Shuqi Zhai, Exploration on dynamics in a ratio-dependent predator-prey bioeconomic model with time delay and additional food supply, 2023, 20, 1551-0018, 15094, 10.3934/mbe.2023676 | |
13. | Vikas Kumar, Pattern formation and delay-induced instability in a Leslie–Gower type prey–predator system with Smith growth function, 2024, 225, 03784754, 78, 10.1016/j.matcom.2024.05.004 |