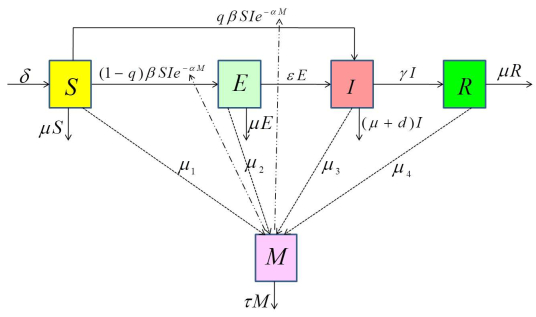
Citation: Ya-Dong Zhang, Hai-Feng Huo, Hong Xiang. Dynamics of tuberculosis with fast and slow progression and media coverage[J]. Mathematical Biosciences and Engineering, 2019, 16(3): 1150-1170. doi: 10.3934/mbe.2019055
[1] | C. Connell Mccluskey . Lyapunov functions for tuberculosis models with fast and slow progression. Mathematical Biosciences and Engineering, 2006, 3(4): 603-614. doi: 10.3934/mbe.2006.3.603 |
[2] | Xuejuan Lu, Shaokai Wang, Shengqiang Liu, Jia Li . An SEI infection model incorporating media impact. Mathematical Biosciences and Engineering, 2017, 14(5&6): 1317-1335. doi: 10.3934/mbe.2017068 |
[3] | Jingli Xie, Hongli Guo, Meiyang Zhang . Dynamics of an SEIR model with media coverage mediated nonlinear infectious force. Mathematical Biosciences and Engineering, 2023, 20(8): 14616-14633. doi: 10.3934/mbe.2023654 |
[4] | Weike Zhou, Aili Wang, Fan Xia, Yanni Xiao, Sanyi Tang . Effects of media reporting on mitigating spread of COVID-19 in the early phase of the outbreak. Mathematical Biosciences and Engineering, 2020, 17(3): 2693-2707. doi: 10.3934/mbe.2020147 |
[5] | Na Pang . Nonlinear neural networks adaptive control for a class of fractional-order tuberculosis model. Mathematical Biosciences and Engineering, 2023, 20(6): 10464-10478. doi: 10.3934/mbe.2023461 |
[6] | Shanjing Ren, Lingling Li . Global stability mathematical analysis for virus transmission model with latent age structure. Mathematical Biosciences and Engineering, 2022, 19(4): 3337-3349. doi: 10.3934/mbe.2022154 |
[7] | Wenjie Qin, Jiamin Zhang, Zhengjun Dong . Media impact research: a discrete SIR epidemic model with threshold switching and nonlinear infection forces. Mathematical Biosciences and Engineering, 2023, 20(10): 17783-17802. doi: 10.3934/mbe.2023790 |
[8] | Xiaojing Wang, Yu Liang, Jiahui Li, Maoxing Liu . Modeling COVID-19 transmission dynamics incorporating media coverage and vaccination. Mathematical Biosciences and Engineering, 2023, 20(6): 10392-10403. doi: 10.3934/mbe.2023456 |
[9] | Cunjuan Dong, Changcheng Xiang, Wenjin Qin, Yi Yang . Global dynamics for a Filippov system with media effects. Mathematical Biosciences and Engineering, 2022, 19(3): 2835-2852. doi: 10.3934/mbe.2022130 |
[10] | Georgi Kapitanov . A double age-structured model of the co-infection of tuberculosis and HIV. Mathematical Biosciences and Engineering, 2015, 12(1): 23-40. doi: 10.3934/mbe.2015.12.23 |
Tuberculosis (TB) is a common and fatal infectious disease. It has become a chronic infectious disease that threatens human health worldwide. Globally, in 2016 there were an estimated 10.4 million incident cases of TB, equivalent to 140 cases per 100000 population. Meanwhile, the proportion of people who develop TB and die from the disease (the case fatality ratio) was 16 [1]. Therefore, TB has become a global concern for social and public health issues.
Many scholars have carried out a lot of excellent researches on the transmission mechanism and prevention strategies of TB [2,3,4,5,6]. Silva et al. [4] introduced delays in a TB model, and studied optimal control of TB with state and control delays. Huo et al. [5] presented a two-strain TB model with general contact rate which allows TB patients with the drug sensitive of strain Mycobacterium tuberculosis to be treated and gave a detailed qualitative analysis about positivity, boundedness, existence, uniqueness and global stability of the equilibria of the model. Huo and Zou [6] studied a TB model with two kinds of treatment, that is, treatment at home and treatment in hospital and showed that the treatment at home has a negative influence on the spread of TB.
The susceptible individuals who carry the pathogen developing into infectious individuals are different from the progression of TB transmission. Some people may become symptomatic infectious individuals after a few days or months, and some people may occur after several years or even decades. For the former, it is considered that the susceptible individuals directly develop symptomatic infectious individuals without going through the latency period after infection, which is called the fast progression of TB transmission. For the latter, it is considered that the susceptible individuals become the latent individuals carrying the pathogen after infection with Mycobacterium tuberculosis, and they can become infected by exogenous reinfection or endogenous infection, which is called a slow progression of TB transmission. Huo and Feng [7] constructed an HIV/AIDS epidemic model with different latent stages and treatment. The model allowed for the latent individuals to have the fast and slow latent compartments. Mccluskey [8] introduced the spread of TB through two models which included fast and slow progression to the infected class. Berge et al. [9] considered a two patch cholera model with the aim of investigating the impact of human population movements between two cities(patches). Song et al. [10] studied TB models with fast and slow dynamics. Many scholars have studied infectious diseases related to the fast and slow progression (see e.g. [11] and references cited therein).
Media coverage is changing the way that we communicate with each other in our daily life, work and study. The media may be the most important source of public health information. At the same time, it also plays an important role in the spread and control of epidemics by providing some health information. Cui et al. [12] proposed a general contact rate β(I)=c1−c2f(I) to reflect some intrinsic characters of media coverage. Huo and Zhang [13] introduced a novel alcoholism model which involves impact of Twitter, and showed that Twitter can serve as a good indicator of alcoholism model and affect the spread of the drinking. Huo et al. [14] presented a SEIS epidemic model with the impact of media coverage. Their results manifested that media can be regarded as a good indicator in controlling the emergence and spread of the epidemic disease. Many scholars have done a lot of researches on infectious diseases with or without media coverage [15,16,17,18,19,20,21].
Motivated by the above, we construct a new TB model which not only involves fast and slow progression but also incorporates the impact of media coverage in this paper. We study the stability of all the equilibria. Furthermore, we also investigate the occurrence of backward and forward bifurcation. Our results show that media coverage can encourage people to take countermeasures to avoid potential infections.
The rest of this paper is organized as follows. In Section 2, a new tuberculosis model with fast and slow progression and media coverage is constructed. In Section 3, we discuss the existence and stability of all the equilibria, then we analyze a forward and backward bifurcation. Some numerical simulations are presented in Section 4. Sensitivity analysis and some discussions are given in the last section.
The total population N(t) is divided into four compartments: S(t), E(t), I(t) and R(t). S(t) denotes susceptible individuals. E(t) is referred to as undetected non-symptomatic (latent) carriers. I(t) is symptomatic infectious individuals. R(t) represents recovered individuals. M(t) represents the number of message that all of them provide about TB at time t. The total population N(t) is given by
N(t)=S(t)+E(t)+I(t)+R(t). |
The transfer diagram of the model is shown in Figure 1. The transfer diagram leads to the following system of ordinary differential equations:
{dS(t)dt=δ−βSIe−αM−μS,dE(t)dt=(1−q)βSIe−αM−(μ+ε)E,dI(t)dt=qβSIe−αM+εE−(d+μ+γ)I,dR(t)dt=γI−μR,dM(t)dt=μ1S+μ2E+μ3I+μ4R−τM. | (2.1) |
All the parameters are positive constants. δ is the constant recruitment rate of the population. β is the transmission coefficient of TB. α is the coefficient that determines how effective the disease-related messages can influence the transmission rate and the transmission rate β is reduced by a factor e−αM (see [13,14]). μ is the natural death rate. q is the proportion of disease by fast progression. ε is the progression rate from the exposed individuals to the infected individuals. d is the disease-related death rate of TB. γ is the recovery rate of TB. τ is the rate that message become outdated. μ1, μ2, μ3 and μ4 are the rates that susceptible individuals, exposed individuals, infectious individuals, recovered individuals may send messages about TB, respectively.
In this section, we will show positivity and boundedness for system (2.1).
Lemma 1. If S(0)≥0,E(0)≥0,I(0)≥0,R(0)≥0,M(0)≥0, the solutions S(t), E(t), I(t), R(t), M(t) of system (2.1) are positive for all t>0.
Proof. If S(0)≥0, according to the first equation of system (2.1), we have
dS(t)dt=δ−[βI(t)e−αM(t)+μ]S(t). |
It can be rewritten as:
dS(t)dtexp{∫t0[βI(u)e−αM(u)+μ]du}+S(t)[βI(t)e−αM(t)+μ]exp{∫t0[βI(u)e−αM(u)+μ]du}=δexp{∫t0[βI(u)e−αM(u)+μ]du}. |
Therefore,
ddt(S(t)exp{∫t0[βI(u)e−αM(u)+μ]du})=δexp{∫t0[βI(u)e−αM(u)+μ]du}. |
Hence,
S(t)exp{∫t0[βI(u)e−αM(u)+μ]du}−S(0)=∫t0(δexp{∫u0[βI(v)e−αM(v)+μ]dv})du. |
So,
S(t)=S(0)exp{−∫t0[βI(u)e−αM(u)+μ]du}+exp{−∫t0[βI(u)e−αM(u)+μ]du}{∫t0(δexp{∫u0[βI(v)e−αM(v)+μ]dv})du}>0. |
Similarly, we can show that E(t)>0,I(t)>0,R(t)>0,M(t)>0. So the solutions S(t), E(t), I(t), R(t), M(t) of system (2.1) with initial conditions S(0)≥0,E(0)≥0,I(0)≥0,R(0)≥0,M(0)≥0 are positive for all t>0. This completes the proof of Lemma 1.
Lemma 2. The feasible region Ω defined by
Ω={(S,E,I,R,M)∈R5+:0≤S+E+I+R≤δμ,0≤M≤δ(μ1+μ2+μ3+μ4)μτ} |
with initial conditions S(0)≥0,E(0)≥0,I(0)≥0,R(0)≥0,M(0)≥0 is positively invariant for system (2.1).
Proof. Adding the former four equations of system (2.1), we obtain
dN(t)dt=δ−μN(t)−dI(t)≤δ−μN(t). |
It follows that
0≤N(t)≤δμ+N(0)e−μt, |
where N(0) is the initial value of total number of people. Thus,
limt→∞supN(t)≤δμ. |
Then
0≤S(t)+E(t)+I(t)+R(t)≤δμ. |
Further, from the last equation of system (2.1), we have
dM(t)dt=μ1S(t)+μ2E(t)+μ3I(t)+μ4R(t)−τM(t)≤δμ(μ1+μ2+μ3+μ4)−τM(t). |
It follows that
0≤M(t)≤δ(μ1+μ2+μ3+μ4)μτ+M(0)e−τt, |
where M(0) represents the initial value of cumulative density media coverage. Thus,
limt→∞supM(t)≤δ(μ1+μ2+μ3+μ4)μτ. |
It implies that the region
Ω={(S,E,I,R,M)∈R5+:0≤S+E+I+R≤δμ,0≤M≤δ(μ1+μ2+μ3+μ4)μτ} |
is a positively invariant set for system (2.1). So we consider dynamics of system (2.1) on the set Ω in this paper. This completes the proof of Lemma 2.
It is easy to see system (2.1) always has a disease-free equilibrium
P0=(S0,E0,I0,R0,M0)=(δμ,0,0,0,μ1δμτ). | (3.1) |
We can obtain the basic reproductive number R0 by using the next-generation method [22]. Let x=(E,I,R,S,M)T, then system (2.1) can be written as
dxdt=F(x)−V(x), |
where,
F(x)=((1−q)βSIe−αMqβSIe−αM000)andV(x)=((μ+ε)E−εE+(d+μ+γ)I−γI+μR−δ+βSIe−αM+μS−μ1S−μ2E−μ3I−μ4R+τM). |
The Jacobian matrices of F(x) and V(x) at the disease-free equilibrium P0 are, respectively,
DF(P0)=(F3×300000000),DV(P0)=(V3×3000βδμe−αμ1δμτ0μ0−μ2−μ3−μ4−μ1τ), |
where
F=(0(1−q)βδμe−αμ1δμτ00qβδμe−αμ1δμτ0000),V=(μ+ε00−εd+μ+γ00−γμ). |
The basic reproductive number, denoted by R0 is thus given by
R0=ρ(FV−1)=(ε+μq)βδe−αμ1δμτμ(μ+ε)(d+μ+γ). | (3.2) |
Theorem 1. The disease-free equilibrium P0=(δμ,0,0,0,μ1δμτ) of system (2.1) is locally asymptotically stable if R0<1, and is unstable if R0>1.
Proof. The Jacobian matrix corresponding to system (2.1) about P0=(δμ,0,0,0,μ1δμτ) is obtained as follows:
J(P0)=(−μ0−βδμe−αμ1δμτ000−(μ+ε)(1−q)βδμe−αμ1δμτ000εqβδμe−αμ1δμτ−(d+μ+γ)0000γ−μ0μ1μ2μ3μ4−τ). |
The characteristic equation corresponding to the Jacobian matrix J(P0) is given by |λE−J(P0)|=0, where λ is the eigenvalue and E is the unit matrix. Thus, we get
(λ+τ)(λ+μ)2[λ2+(2μ+ε+d+γ−qβδμe−αμ1δμτ)λ+(μ+ε)(d+μ+γ)−βδ(ε+μq)μe−αμ1δμτ]=0. | (3.3) |
Obviously, Eq. (3.3) has three negative roots λ1=−τ, λ2=λ3=−μ, and the other two roots λ4 and λ5 are determined by
λ2+(2μ+ε+d+γ−qβδμe−αμ1δμτ)λ+(μ+ε)(d+μ+γ)−βδ(ε+μq)μe−αμ1δμτ=0. | (3.4) |
According to the above calculation and analysis, we can obtain
λ4λ5=(μ+ε)(d+μ+γ)−βδ(ε+μq)μe−αμ1δμτ=(μ+ε)(d+μ+γ)(1−R0), |
λ4+λ5=qβδμe−αμ1δμτ−(2μ+ε+d+γ)=(μ+ε)(d+μ+γ)qε+μq[(ε+μq)βδe−αμ1δμτμ(μ+ε)(d+μ+γ)−ε+μq(μ+ε)q−ε+μq(d+μ+γ)q]=(μ+ε)(d+μ+γ)qε+μq[R0−ε+μqεq+μq−ε+μq(d+μ+γ)q]<(μ+ε)(d+μ+γ)qε+μq[R0−1−ε+μq(d+μ+γ)q]. |
If R0<1, we have λ4λ5>0, λ4+λ5<0, hence λ4<0,λ5<0. Therefore, P0=(δμ,0,0,0,μ1δμτ) is locally asymptotically stable. If R0>1, Eq. (3.4) has two real roots that one is positive and another is negative. In this case, P0=(δμ,0,0,0,μ1δμτ) is unstable. This completes the proof of Theorem 1.
Theorem 2. The disease-free equilibrium P0=(δμ,0,0,0,μ1δμτ) of the system (2.1) is globally asymptotically stable if R0<1 and M(t)≥μ1δμτ.
Proof. Motivated by Huo and Zhang [13], we define the Lyapunov function
V(t)=εE(t)+(μ+ε)I(t). |
It is clear that V(t)≥0 and the equality holds if and only if E(t)=I(t)=0.
From the first equation of the system (2.1), we have
dSdt=δ−βSIe−αM−μS≤δ−μS, |
and then we can obtain S(t)≤δμ.
Differentiating V(t) with respect to time t yields:
dV(t)dt=εdE(t)dt+(μ+ε)dI(t)dt=βSIe−αM(ε+μq)−(μ+ε)(d+μ+γ)I≤βδ(ε+μq)e−αμ1δμτμI−(μ+ε)(d+μ+γ)I=(μ+ε)(d+μ+γ)I[βδ(ε+μq)e−αμ1δμτμ(μ+ε)(d+μ+γ)−1]=(μ+ε)(d+μ+γ)I(R0−1). |
It follows that V(t) is bounded and non-increasing. Therefore, limt→∞V(t) exists. Note that dV(t)dt=0 if and only if E=I=R=0,S=S0=δμ,M=M0=μ1δμτ. The maximum invariant set of the system (2.1) on the set {(S,E,I,R,M):dV(t)dt=0} is the singleton P0=(δμ,0,0,0,μ1δμτ). And note that R0<1 guarantees that dV(t)dt≤0 for all t≥0. By LaSalle's Invariance Principle [23], the disease-free equilibrium P0=(δμ,0,0,0,μ1δμτ) is globally asymptotically stable when R0<1 and M(t)≥μ1δμτ. This completes the proof of Theorem 2.
First, we introduce:
Φ=−αμτ(μq+ε){(μq+ε)(γμ4+μμ3)+(d+μ+γ)[μμ2(1−q)−μ1(μ+ε)]}, | (3.5) |
R01=δ(μq+ε)Φ(μ+ε)(d+μ+γ), | (3.6) |
Rc=R01e1−R01. | (3.7) |
Remark 1. It is clear to check that: R01>0 if and only if Φ>0; R01=0 if and only if Φ=0; R01<0 if and only if Φ<0.
Theorem 3. For system (2.1),
(ⅰ) If R0>max{1,R01}, there is a unique endemic equilibrium P∗1.
(ⅱ) If Rc=R0<min{1,R01} and R01>0, there is a unique endemic equilibrium P∗2.
(ⅲ) If Rc<R0<min{1,R01} and R01>0, there are two distinct endemic equilibria P∗3 and P∗4.
Proof. The endemic equilibrium P∗(S∗,E∗,I∗,R∗,M∗) of system (2.1) is determined by equations
{δ−βSIe−αM−μS=0,(1−q)βSIe−αM−(μ+ε)E=0,qβSIe−αM+εE−(d+μ+γ)I=0,γI−μR=0,μ1S+μ2E+μ3I+μ4R−τM=0. | (3.8) |
Further, we obtain
S=δμ−(μ+ε)(d+μ+γ)μ(μq+ε)I, | (3.9) |
E=(1−q)(d+μ+γ)μq+εI, | (3.10) |
R=γμI, | (3.11) |
M=μ1δμτ−ΦαI, | (3.12) |
where Φ is given by (3.5). Substituting S,M into the first equation of (3.8) yields
R0[1−(μ+ε)(d+μ+γ)δ(μq+ε)I]=e−ΦI. | (3.13) |
According to (3.6) and (3.13), we have
R0(1−ΦR01I)−e−ΦI=0. |
We consider a function F(I) defined by
F(I)=R0(1−ΦR01I)−e−ΦI. | (3.14) |
Then, we have
F(0)=R0−1,F(+∞)=−∞, |
F′(I)=−R0R01Φ+Φe−ΦI,F′(0)=−R0R01Φ+Φ, |
F″(I)=−Φ2e−ΦI. |
Case 1. When Φ=0, according to (3.13), we have
I=δ(μq+ε)(μ+ε)(d+μ+γ)(1−1R0). |
Therefore, there is a unique endemic equilibrium if Φ=0 and R0>1.
Case 2. When Φ≠0, we have
F″(I)=−Φ2e−ΦI<0. |
Thus, we get F′(I)<F′(0), which means Φe−ΦI<Φ.
(1) If R0>1, we have F(0)=R0−1>0, F(+∞)=−∞<0, and
F′(I)=−R0R01Φ+Φe−ΦI<−R0R01Φ+Φ=(1−R0R01)Φ. |
When R0>R01>0, we have Φ>0 and 1−R0R01<0, which means F′(I)<0.
When R0>0>R01, we have Φ<0 and 1−R0R01>0, which means F′(I)<0.
Therefore, there is a unique endemic equilibrium if Φ≠0 and R0>max{1,R01}.
In conclusion, there is a unique endemic equilibrium P∗1 if R0>max{1,R01}.
(2) If R0<1, we have F(0)=R0−1<0, F(+∞)=−∞<0, Let's suppose
F′(I)=−R0R01Φ+Φe−ΦI=0. |
Then we obtain
Ic=1ΦlnR01R0. |
When R0<R01, we have Φ>0 and lnR01R0>0, which means Ic>0.
Substituting Ic into (3.14), we get
F(Ic)=R0+R0R01(lnR0R01−1). |
(a) When F(Ic)=0, we can obtain I∗2=Ic and R0=Rc, where Rc is given by (3.7).
Therefore, there is a unique endemic equilibrium P∗2 if Rc=R0<min{1,R01} and R01>0.
(b) When F(Ic)>0, we can obtain R0>Rc.
Since F(0)=R0−1<0, F(+∞)=−∞<0 and F(Ic)>0, we know that F(I)=0 has two different positive solutions I∗3 and I∗4. Let I∗3 and I∗4 satisfy I∗3<I∗2<I∗4. Therefore, there are two distinct endemic equilibria P∗3 and P∗4 if Rc<R0<min{1,R01} and R01>0. This completes the proof of Theorem 3.
Theorem 4. When q=0, the endemic equilibria P∗i(i=1,2,3,4) of system (2.1) have the following qualities:
(ⅰ) If R0>max{1,R01}, a1(I∗1)a2(I∗1)−a3(I∗1)>0, a3(I∗1)[a1(I∗1)a2(I∗1)−a3(I∗1)]−[a1(I∗1)]2a4(I∗1)>0 and a4(I∗1)>0, the endemic equilibrium P∗1 is locally asymptotically stable.
(ⅱ) If Rc=R0<min{1,R01} and R01>0, the endemic equilibrium P∗2 is unstable.
(ⅲ) If Rc<R0<min{1,R01} and R01>0, the endemic equilibrium P∗3 is unstable.
(ⅳ) If Rc<R0<min{1,R01} and R01>0, the stability of the endemic equilibrium P∗4 is uncertain.
Proof. When q=0, the Jacobian matrix corresponding to system (2.1) about P∗i(i=1,2,3,4) are obtained as follows:
J(P∗i)=(−βI∗ie−αM∗i−μ0−βS∗ie−αM∗i0αβS∗iI∗ie−αM∗iβI∗ie−αM∗i−(μ+ε)βS∗ie−αM∗i0−αβS∗iI∗ie−αM∗i0ε−(d+μ+γ)0000γ−μ0μ1μ2μ3μ4−τ). |
The characteristic equation corresponding to the Jacobian matrix J(P∗i) is given by |λE−J(P∗i)|=0, where λ is the eigenvalue and E is the unit matrix. Thus, we get
|λ+βI∗ie−αM∗i+μ0βS∗ie−αM∗i0−αβS∗iI∗ie−αM∗i−βI∗ie−αM∗iλ+μ+ε−βS∗ie−αM∗i0αβS∗iI∗ie−αM∗i0−ελ+d+μ+γ0000−γλ+μ0−μ1−μ2−μ3−μ4λ+τ|=0. |
We set Θ=βe−αM∗i, then
Θ=βe−αμ1δμτeΦI∗i=μ(μ+ε)(d+μ+γ)eΦI∗iR0εδ=μΦeΦI∗iR0R01. |
From the second equation of (3.8), we have
βS∗iI∗ie−αM∗i=(μ+ε)E∗i=(μ+ε)(d+μ+γ)εI∗i, |
then
ΘS∗i=βS∗ie−αM∗i=(μ+ε)(d+μ+γ)ε=δΦR01, |
and
ΘS∗iI∗i=βS∗iI∗ie−αM∗i=(μ+ε)(d+μ+γ)εI∗i=δΦR01I∗i. |
Therefore, the characteristic equation can be rewritten as:
(λ+μ)F(λ)=0, | (3.15) |
where
F(λ)=λ4+a1(I∗i)λ3+a2(I∗i)λ2+a3(I∗i)λ+a4(I∗i), | (3.16) |
where
a1(I∗i)=d+γ+3μ+ε+τ+ΘI∗i, | (3.17) |
a2(I∗i)=(d+γ+2μ+ε+τ)(μ+ΘI∗i)+τ(d+γ+2μ+ε)+αδΦR01I∗i(μ2−μ1), | (3.18) |
a3(I∗i)=μΘI∗i(d+γ+2τ+ε+μ)+τ(ε+d+γ)(μ+ΘI∗i)+ε(d+γ)ΘI∗i |
+αδΦR01I∗i[(d+γ+2μ)(μ2−μ1)+ε(μ3−μ1)], | (3.19) |
a4(I∗i)=τ(μ+ε)(d+γ+μ)[(μ+ΘI∗i)−μ(1+ΦI∗i)]. | (3.20) |
(ⅰ) According to (3.17)-(3.20), we have
a1(I∗1)=d+γ+3μ+ε+τ+ΘI∗1,a2(I∗1)=(d+γ+2μ+ε+τ)(μ+ΘI∗1)+τ(d+γ+2μ+ε)+αδΦR01I∗1(μ2−μ1),a3(I∗1)=μΘI∗1(d+γ+2τ+ε+μ)+τ(ε+d+γ)(μ+ΘI∗1)+ε(d+γ)ΘI∗1+αδΦR01I∗1[(d+γ+2μ)(μ2−μ1)+ε(μ3−μ1)],a4(I∗1)=τ(μ+ε)(d+γ+μ)[(μ+ΘI∗1)−μ(1+ΦI∗1)]. |
It is clear that a1(I∗1)>0, according to Routh−Hurwitz criteria [24], the proof (ⅰ) of Theorem 4 is obtained.
(ⅱ) According to the proof of (ⅱ) of Theorem 3, we have I∗2=1ΦlnR01R0. Then, we can get ΘI∗2=μΦI∗2. Therefore, based on (3.17)-(3.20), we can obtain
a1(I∗2)=d+γ+3μ+ε+τ+ΘI∗2>0,a4(I∗2)=τμ(μ+ε)(d+γ+μ)[(1+ΦI∗2)−(1+ΦI∗2)]=0. |
It is easy to know that a3(I∗2)≠0, and a1(I∗2)a2(I∗2)−a3(I∗2)<0. Therefore, we know that Eq. (3.15) has negative, positive and zero eigenvalues. So the endemic equilibrium P∗2 of system (2.1) is unstable.
(ⅲ) Due to I∗3<I∗2=1ΦlnR01R0, we can get ΘI∗3<μΦI∗3. Therefore, based on (3.17)-(3.20), we can obtain
a1(I∗3)=d+γ+3μ+ε+τ+ΘI∗3>0, |
and
a4(I∗3)<τμ(μ+ε)(d+γ+μ)[(1+ΦI∗3)−(1+ΦI∗3)]=0. |
Let gj(I∗3)(j=1,2,3,4) be the solutions of F(λ)=0, and we assume that the real parts satisfy Re(g1(I∗3))≤Re(g2(I∗3))≤Re(g3(I∗3))≤Re(g4(I∗3)), where Re means the real part of a complex number. Then we can obtain gj(I∗3)(j=1,2,3,4) satisfying
g1(I∗3)+g2(I∗3)+g3(I∗3)+g4(I∗3)=−a1(I∗3)<0, |
and
g1(I∗3)g2(I∗3)g3(I∗3)g4(I∗3)=a4(I∗3)<0. |
So, we have Re(g1(I∗3))<0 and Re(g4(I∗3))>0. Then, we know that the endemic equilibrium P∗3 of system (2.1) is unstable.
(ⅳ) Due to I∗4>I∗2=1ΦlnR01R0, we have ΘI∗4>μΦI∗4. Therefore, based on (3.17)-(3.20), we can obtain
a1(I∗4)=d+γ+3μ+ε+τ+ΘI∗4>0 |
and
a4(I∗4)>τμ(μ+ε)(d+γ+μ)[(1+ΦI∗4)−(1+ΦI∗4)]=0. |
Let gj(I∗4)(j=1,2,3,4) be the solutions of F(λ)=0, and we assume that the real parts satisfy Re(g1(I∗4))≤Re(g2(I∗4))≤Re(g3(I∗4))≤Re(g4(I∗4)). Then we can obtain gj(I∗4)(j=1,2,3,4) satisfying
g1(I∗4)+g2(I∗4)+g3(I∗4)+g4(I∗4)=−a1(I∗4)<0 | (3.21) |
and
g1(I∗4)g2(I∗4)g3(I∗4)g4(I∗4)=a4(I∗4)>0. |
Therefore, if Re(gj(I∗4))<0(j=1,2,3,4), the endemic equilibrium P∗4 of system (2.1) is stable. However, if Re(g1(I∗4))≤Re(g2(I∗4))<0<Re(g3(I∗4))≤Re(g4(I∗4)) and |Re(g1(I∗4))|+|Re(g2(I∗4))|>|Re(g3(I∗4))|+|Re(g4(I∗4))|, the endemic equilibrium P∗4 of system (2.1) is unstable. Thus, the stability of the endemic equilibrium P∗4 is uncertain. This completes the proof of Theorem 4.
Theorem 5. (ⅰ) If R01<1, system (2.1) exhibits a forward bifurcation at R0=1.
(ⅱ)If R01>1, system (2.1) exhibits a backward bifurcation at R0=1.
Proof. We suppose x1=S,x2=E,x3=I,x4=R,x5=M, system (2.1) becomes
{dx1dt=δ−βx1x3e−αx5−μx1:=f1,dx2dt=(1−q)βx1x3e−αx5−(μ+ε)x2:=f2,dx3dt=qβx1x3e−αx5+εx2−(d+μ+γ)x3:=f3,dx4dt=γx3−μx4:=f4,dx5dt=μ1x1+μ2x2+μ3x3+μ4x4−τx5:=f5. |
When R0=1, we obtain β=βc=μ(μ+ε)(d+μ+γ)δ(ε+μq)eαμ1δμτ. When β=βc, the Jacobian matrix corresponding to system (2.1) about the disease-free equilibrium P0=x0=(δμ,0,0,0,μ1δμτ) is given by
J(x0)=(−μ0−(μ+ε)(d+μ+γ)ε+μq000−(μ+ε)(1−q)(μ+ε)(d+μ+γ)ε+μq000εq(μ+ε)(d+μ+γ)ε+μq−(μ+d+γ)0000γ−μ0μ1μ2μ3μ4−τ). |
It is clear that 0 is a simple eigenvalue of J(x0). A right eigenvector ω corresponding to the 0 eigenvalue is ω=(ω1,ω2,ω3,ω4,ω5)T, where
ω1=−(μ+ε)(d+μ+γ)μ,ω2=(1−q)(d+μ+γ),ω3=ε+μq,ω4=γ(ε+μq)μ,ω5=−(ε+μq)Φα. |
The left eigenvector υ corresponding to the 0 eigenvalue satisfying υJ=0 and υω=1 is υ=(υ1,υ2,υ3,υ4,υ5), where
υ1=υ4=υ5=0,υ2=1(1−q)(d+μ+γ)+(ε+μq)(μ+ε), |
υ3=μ+εε[(1−q)(d+μ+γ)+(ε+μq)(μ+ε)]. |
Furthermore, we have a=5∑k,i,j=1υkωiωj∂2fk(x0)∂xi∂xj and b=5∑k,i=1υkωi∂2fk(x0)∂xi∂β. Substituting the values of all second order derivatives evaluated at the disease-free equilibrium x0=(δμ,0,0,0,μ1δμτ), we obtain
a=2υ2ω1ω3∂2f2(x0)∂x1∂x3+2υ2ω3ω5∂2f2(x0)∂x3∂x5+2υ3ω1ω3∂2f3(x0)∂x1∂x3+2υ3ω3ω5∂2f3(x0)∂x3∂x5=2βe−αμ1δμτ(ω1ω3−αδμω3ω5)(υ2(1−q)+υ3q)=2β(ε+μq)2(μ+ε)(d+μ+γ)(R01−1)e−αμ1δμτμε[(1−q)(d+μ+γ)+(ε+μq)(μ+ε)], |
and
b=υ2ω3∂2f2(x0)∂x3∂β+υ3ω3∂2f3(x0)∂x3∂β=ω3δμe−αμ1δμτ[(1−q)υ2+qυ3]=δ(ε+μq)2e−αμ1δμτμε[(1−q)(d+μ+γ)+(ε+μq)(μ+ε)]. |
According to Theorem 4.1 of [25], note that the coefficient b is always positive. If R01<1, the coefficient a is negative. In this case, the direction of the bifurcation of system (2.1) at R0=1 is forward. If R01>1, the coefficient a is positive. Under this circumstance, the direction of the bifurcation of system (2.1) at R—0=1 is backward. This completes the proof of Theorem 5.
In this section, we will give some simulations using the parameter values which are given in Table 1.
Parameter | Description | Estimated value | Source |
δ | Constant recruitment rate of the population | 0.8day−1 | [14] |
β | Transmission coefficient of TB | 0.0099-0.8person−1day−1 | Estimate |
α | The coefficient that determines how effective TB | 0.00091-0.8day−1 | [14] |
information can influence the transmission rate | |||
μ | Nature death rate | 0.009-0.6year−1 | Estimate |
q | The proportion of disease by fast progression | 0-0.5year−1 | Estimate |
ε | The progression rate from E to I | 0.02-0.99day−1 | Estimate |
d | The disease-related death rate of TB | 0.002-0.5day−1 | Estimate |
γ | The recovery rate of TB | 0.006-0.99day−1 | Estimate |
μ1 | The rate that susceptible individuals may send | 0.04-0.99day−1 | [26] |
message about TB | |||
μ2 | The rate that exposed individuals may send | 0.008-0.8day−1 | [26] |
message about TB | |||
μ3 | The rate that infectious individuals may send | 0.08-0.8day−1 | [26] |
message about TB | |||
μ4 | The rate that recovered individuals may send | 0-1day−1 | Estimate |
message about TB | |||
τ | The rate that message become outdated | 0.03-0.6year−1 | [26] |
We choose a set of the following parameters: δ=0.8, β=0.8, α=0.08, μ=0.6, q=0.5, ε=0.09, d=0.02, γ=0.7, μ1=0.99, μ2=0.4, μ3=0.8, μ4=0.8, τ=0.6. It is easy to check that the basic reproductive number R0=0.383<1. Then the unique disease-free equilibrium P0=(1.3333,0,0,0,2.2) of system (2.1) is globally asymptotically stable (see Figure 2).
Next, we select a set of the following parameters: δ=0.8, β=0.8, α=0.08, μ=0.2, q=0.1, ε=0.4, d=0.02, γ=0.6, μ1=0.2, μ2=0.8, μ3=0.8, μ4=0.8, τ=0.6. It is easy to check that the basic reproductive number R0=2.4553>1. Then, from Theorem 4, the endemic equilibrium P∗1 of system (2.1) is locally asymptotically stable when R0>max(1,R01), where R01=−0.0158 (see Figure 3).
The backward and forward bifurcation diagram of system (2.1) is shown in Figure 4, and the direction of bifurcation depends upon the value of R01. As seen in the backward bifurcation diagram of Figure 4(a) when R01=4.4936>1, there is a threshold quantity Rc which is the value of R0. The disease-free equilibrium is globally asymptotically stable when R0<Rc, where Rc=0.1350. There are two endemic equilibria and a disease-free equilibrium when Rc<R0<1, the upper ones are stable, the middle ones are unstable and the lower ones is globally asymptotically stable. There is a stable endemic equilibrium and an unstable disease-free equilibrium when R0>1. As seen in the forward bifurcation diagram of Figure 4(b) when R01=0.5357<1, the disease-free equilibrium is globally asymptotically stable when R0<1. There are a stable endemic equilibrium and an unstable disease-free equilibrium when R0>1.
In this section, we discuss sensitivity analysis of the basic reproductive number R0 and the infectious individuals I at first. We study the influence of α, μ1 and β to R0. It is straightforward from (3.2) that R0 increases as β increases. This agrees with the intuition that higher transmission coefficient increases the basic reproduction number. In order to see the relationship of these parameters and R0, we regard R0 as a function about those parameters. Note that
∂R0∂α=−(ε+μq)βδ2μ1e−αμ1δμτμ2τ(μ+ε)(d+μ+γ)<0, |
∂R0∂μ1=−(ε+μq)αβδ2e−αμ1δμτμ2τ(μ+ε)(d+μ+γ)<0, |
∂R0∂q=μβδe−αμ1δμτμ(μ+ε)(d+μ+γ)>0. |
Therefore, we find that α and μ1 have a negative influence on the basic reproductive number R0. However, q has a positive influence on the basic reproductive number R0. The parameter values are δ=0.8, q=0.1, β=0.8, μ=0.2, ε=0.4, γ=0.6, d=0.02, μ2=0.8, μ3=0.8, μ4=0.8, τ=0.6. From Figure 5, we know that the basic reproductive number R0 will decrease when α and μ1 increase. However, the basic reproductive number R0 will increase when q increases.
Next, in order to evaluate the effect of media coverage on the dynamics of tuberculosis, we choose different values of α and τ (see Figure 6). The parameters are δ=0.8, q=0.1, β=0.8,μ=0.2, ε=0.4, γ=0.6, d=0.02, μ1=0.2, μ2=0.8, μ3=0.8, μ4=0.8.
From Figure 6, we know that infected number will decrease when α increase, and increase when τ increases. Therefore, we find that media coverage has a great impact on the transmission of tuberculosis.
Choosing β as a parameter, it is also observed that with β increasing, the positive equilibrium point P∗1 loses its stability and a Hopf bifurcation occurs when β passes a critical values β∗.
We select a set of the following parameters: δ=0.8, β=0.0099, α=0.007, μ=0.009, q=0.1, ε=0.99, d=0.5, γ=0.99, μ1=0.08, μ2=0.8, μ3=0.8, μ4=0.8, τ=0.6. The endemic equilibrium P∗1 of system (2.1) is locally asymptotically stable when R0>max{1,R01} and β<β∗ (see Figure 7).
To illustrate the existence of Hopf bifurcation, we choose a set of the following parameters: δ=0.8, α=0.007, μ=0.009, q=0.5, ε=0.99, d=0.02, γ=0.1, μ1=0.09, μ2=0.008, μ3=0.08, μ4=0.08, τ=0.03. When β passes through the critical value β∗, we find the positive endemic equilibrium P∗1 loses its stability and a Hopf bifurcation occurs (see Figure 8).
In this paper, we propose and analyse a TB model with fast and slow progression and media coverage. By means of the next-generation matrix, we obtain the basic reproductive number R0, which plays a crucial role in our model. By constructing Lyapunov function, we prove the global stability of the disease-free equilibrium. In addition, we obtain the existence and the local stability of the endemic equilibrium. By using the center manifold theory, we get a backward and forward bifurcation. Furthermore, we give a numerical result about a Hopf bifurcation occurs when β passes through the critical value β∗. At last, we also use numerical method to simulate outcomes which we have been proved.
The initially exposed individuals have a higher risk of developing active TB. They still have the possibility of progressing to infectious TB with time passing. The likelihood of becoming an active infectious case decreases with the age of the infection. Taking these factors into consideration, we set up a new tuberculosis with fast and slow progression and media coverage. Through simulations, we know that β plays an important role and induces Hopf bifucation in our model. Furthermore, we have done some simulations (not shown). We did not find other critical parameters (including q) for Hopf bifurcation. q is the proportion of disease by fast progression. Since R0=ρ(FV−1)=(ε+μq)βδe−αμ1δμτμ(μ+ε)(d+μ+γ), we can find the basic reproductive number R0 will increase when q increases. Tuberculosis may breakout due to the increase of q. The fast and slow progression can not induce Hopf bifurcation, but it still plays an important role in TB transmission and has a positive influence on the basic reproductive number R0.
Our results show that media coverage has a substantial influence on the dynamics of tuberculosis and it can greatly influence the spread of the tuberculosis, thus, it is crucial to remind people to take countermeasures to avoid potential infections by media coverage.
In our model (2.1), we only consider the form of ordinary equation. Note that all of the people have a time delay in releasing and receiving information, it is more realistic to explore a time delay in the rate that media coverage become outdated. On the other hand, as suggested by Styblo et al. [27], recovered individuals may only have partial immunity. Indeed, TB is one kind of chronic infectious diseases that has a certain relapse rate due to the drug-resistant tuberculosis and lack of combination drug regimen. Thus, it is a very interesting and more realistic to study our model with reinfection, that is some individuals in the recovered class can relapse back into the active TB state. We leave these interesting works for the future.
The authors are very grateful to the Editor-in-Chief and the anonymous referees for their valuable comments and suggestions which helped us to improve the paper. This work is supported by the National Natural Science Foundation of China (11861044 and 11661050), and the HongLiu first-class disciplines Development Program of Lanzhou University of Technology.
The authors declare there is no conflict of interest.
[1] | World Health Organization, Global tuberculosis report 2017, Available from: https://www. who.int/tb/publications/global_report/en. |
[2] | D.P. Gao and N.J. Huang, Optimal control analysis of a tuberculosis model, Appl. Math. Model., 58 (2018), 47–64. |
[3] | S. Kim, A.A. Reyes and E. Jung, Mathematical model and intervention strategies for mitigating tuberculosis in the Philippines, J. Theor. Biol., 443 (2018), 100–112. |
[4] | C.J. Silva, H. Maurer and D.F. Torres, Optimal control of a tuberculosis model with state and control delays, Math. Biosci. Eng., 14 (2016), 321–337. |
[5] | H.F. Huo, S.J. Dang and Y.N. Li, Stability of a two-strain tuberculosis model with general contact rate, Abstr. Appl. Anal., 2010 (2010), 1–31. |
[6] | H.F. Huo and M.X. Zou, Modelling effects of treatment at home on tuberculosis transmission dynamics, Appl. Math. Model., 40 (2016), 9474–9484. |
[7] | H.F. Huo and L.X. Feng, Global stability for an HIV/AIDS epidemic model with different latent stages and treatment, Appl. Math. Model., 37 (2013), 1480–1489. |
[8] | C.C. Mccluskey, Lyapunov functions for tuberculosis models with fast and slow progression, Math. Biosci. Eng., 3 (2006), 603–614. |
[9] | T. Bergeb, S. Bowonga and J.M. Lubuma, Global stability of a two-patch cholera model with fast and slow transmissions, Math. Comput. Simulat., 133 (2017), 124–164. |
[10] | B. Song, C. Castillo-Chavez and J.P. Aparicio, Tuberculosis models with fast and slow dynamics: the role of close and casual contacts, Math. Biosci., 180 (2002), 187–205. |
[11] | J. Li, Y.L. Zhao and S.M. Li, Fast and slow dynamics of malaria model with relapse, Math. Biosci., 246 (2013), 94–104. |
[12] | J.A. Cui, X. Tao and H.P. Zhu, An SIS infection model incorporating media coverage, Rocky Mt. J. Math., 38 (2008), 1323–1334. |
[13] | H.F. Huo and X.M. Zhang, Complex dynamics in an alcoholism model with the impact of twitter, Math. Biosci., 281 (2016), 24–35. |
[14] | H.F. Huo, P. Yang and H. Xiang, Stability and bifurcation for an SEIS epidemic model with the impact of media, Physica A, 490 (2018), 702–720. |
[15] | J.G. Cui, Y.H. Sun and H.P. Zhu, The impact of media on the control of infectious diseases, J. Differ. Equations, 20 (2008), 31–53. |
[16] | H. Xiang, Y.Y. Wang and H.F. Huo, Analysis of the binge drinking models with demographics and nonlinear infectivity on networks, J. Appl. Anal. Comput., 8 (2018), 1535–1554. |
[17] | H.F. Huo, F.F. Cui and H. Xiang, Dynamics of an SAITS alcoholism model on unweighted and weighted networks, Physica A, 496 (2018), 249–262. |
[18] | Y.L. Cai, J.J. Jiao, Z.J. Gui, Y.T. Liu and W.M. Wang, Environmental variability in a stochastic epidemic model, Appl. Math. Comput., 329 (2018), 210–226. |
[19] | Z. Du and Z. Feng, Existence and asymptotic behaviors of traveling waves of a modified vectordisease model, Commun. Pur. Appl. Anal., 17 (2018), 1899–1920. |
[20] | X.B. Zhang, Q.H. Shi, S.H. Ma, H.F. Huo and D.G. Li, Dynamic behavior of a stochastic SIQS epidemic model with levy jumps, Nonlinear Dynam., 93 (2018), 1481–1493. |
[21] | W.M. Wang, Y.L. Cai, Z.Q. Ding and Z.J. Gui, A stochastic differential equation SIS epidemic model incorporating Ornstein-Uhlenbeck process, Physica A, 509 (2018), 921–936. |
[22] | P.V. Driessche and J.Watmough, Reproduction numbers and sub-threshold endemic equilibria for compartmental models of disease transmission, Math. Biosci., 180 (2002), 29–48. |
[23] | J.P. LaSalle, The Stability of Dynamical Systems, Regional Conference Series in Applied Mathmatics, Society for Industrial and Applied Mathematics, Berlin, New Jersey, 1976. |
[24] | J.D. Murray, Mathematical Biology, Springer-Verlag, Berlin, 1998. |
[25] | C. Castillo-Chavez and B. Song, Dynamical models of tuberculosis and their applications, Math. Biosci. Eng., 1 (2004), 361–401. |
[26] | K.A. Pawelek, A. Oeldorfhirsch and L. Rong, Modeling the impact of twitter on influenza epidemics, Math. Biosci. Eng., 11 (2014), 1337–1356. |
[27] | K. Styblo, J. Meijer and I. Sutherland, The transmission of Tubercle Bacilli: its trend in a human population, Bull. World Health Organ., 41 (1969), 137–178. |
1. | Shuang-Lin Jing, Hai-Feng Huo, Hong Xiang, Modeling the Effects of Meteorological Factors and Unreported Cases on Seasonal Influenza Outbreaks in Gansu Province, China, 2020, 82, 0092-8240, 10.1007/s11538-020-00747-6 | |
2. | Hai-Feng Huo, Shuang-Lin Jing, Xun-Yang Wang, Hong Xiang, Modeling and analysis of a H1N1 model with relapse and effect of Twitter, 2020, 560, 03784371, 125136, 10.1016/j.physa.2020.125136 | |
3. | Zhong-Kai Guo, Hong Xiang, Hai-Feng Huo, Analysis of an age-structured tuberculosis model with treatment and relapse, 2021, 82, 0303-6812, 10.1007/s00285-021-01595-1 | |
4. | Dipo Aldila, Joseph Páez Chávez, Karunia Putra Wijaya, Naleen Chaminda Ganegoda, Gracia Monalisa Simorangkir, Hengki Tasman, Edy Soewono, A tuberculosis epidemic model as a proxy for the assessment of the novel M72/AS01E vaccine, 2023, 120, 10075704, 107162, 10.1016/j.cnsns.2023.107162 | |
5. | Cheng-Cheng Zhu, Jiang Zhu, Spread trend of COVID-19 epidemic outbreak in China: using exponential attractor method in a spatial heterogeneous SEIQR model, 2020, 17, 1551-0018, 3062, 10.3934/mbe.2020174 | |
6. | Ling Xue, Shuanglin Jing, Hao Wang, Evaluating Strategies For Tuberculosis to Achieve the Goals of WHO in China: A Seasonal Age-Structured Model Study, 2022, 84, 0092-8240, 10.1007/s11538-022-01019-1 | |
7. | Peng Yang, Transmission dynamics to a spatially diffusive Tuberculosis model subject to age-since-infection, 2022, 73, 0044-2275, 10.1007/s00033-022-01872-z | |
8. | Yaping Wang, Lin Hu, Linfei Nie, Dynamics of a Hybrid HIV/AIDS Model with Age-Structured, Self-Protection and Media Coverage, 2022, 11, 2227-7390, 82, 10.3390/math11010082 | |
9. | Qian Yang, Hai-Feng Huo, Hong Xiang, Analysis of an edge-based SEIR epidemic model with sexual and non-sexual transmission routes, 2023, 609, 03784371, 128340, 10.1016/j.physa.2022.128340 | |
10. | Na Pang, Nonlinear neural networks adaptive control for a class of fractional-order tuberculosis model, 2023, 20, 1551-0018, 10464, 10.3934/mbe.2023461 | |
11. | Yuan Sang, Long Zhang, Bing Song, Yuru Zhang, Global Stability for an Endogenous-Reactivated Tuberculosis Model with Beddington–DeAngelis Incidence, Distributed Delay and Relapse, 2023, 22, 1575-5460, 10.1007/s12346-023-00788-x | |
12. | Lin Hu, Linfei Nie, Stability and Hopf Bifurcation Analysis of a Multi-Delay Vector-Borne Disease Model with Presence Awareness and Media Effect, 2023, 7, 2504-3110, 831, 10.3390/fractalfract7120831 | |
13. | Wei Li, Yi Wang, Jinde Cao, Mahmoud Abdel-Aty, Dynamics and backward bifurcations of SEI tuberculosis models in homogeneous and heterogeneous populations, 2025, 543, 0022247X, 128924, 10.1016/j.jmaa.2024.128924 | |
14. | Abhineshwary Bhalraj, Amirah Azmi, 2024, 3016, 0094-243X, 020012, 10.1063/5.0192515 | |
15. | Saduri Das, Prashant K. Srivastava, Pankaj Biswas, The role of social media in a tuberculosis compartmental model: Exploring Hopf-bifurcation and nonlinear oscillations, 2024, 03784754, 10.1016/j.matcom.2024.11.015 | |
16. | Chiganga. S. Ruoja, Maranya Mayengo, Nkuba Nyerere, Farai Nyabadza, Modeling the influence of fear and patients’ attitudes on the transmission dynamics of tuberculosis, 2025, 11, 2363-6203, 10.1007/s40808-024-02257-z | |
17. | Kumneger Tadesse Mulugeta, Mohammed Yiha Dawed, Shewafera Wondimagegnhu Teklu, Joshua Kiddy K. Asamoah, Impact of media coverage on the transmission dynamics of TB with vaccines and treatment, 2025, 20, 1932-6203, e0314324, 10.1371/journal.pone.0314324 |
Parameter | Description | Estimated value | Source |
δ | Constant recruitment rate of the population | 0.8day−1 | [14] |
β | Transmission coefficient of TB | 0.0099-0.8person−1day−1 | Estimate |
α | The coefficient that determines how effective TB | 0.00091-0.8day−1 | [14] |
information can influence the transmission rate | |||
μ | Nature death rate | 0.009-0.6year−1 | Estimate |
q | The proportion of disease by fast progression | 0-0.5year−1 | Estimate |
ε | The progression rate from E to I | 0.02-0.99day−1 | Estimate |
d | The disease-related death rate of TB | 0.002-0.5day−1 | Estimate |
γ | The recovery rate of TB | 0.006-0.99day−1 | Estimate |
μ1 | The rate that susceptible individuals may send | 0.04-0.99day−1 | [26] |
message about TB | |||
μ2 | The rate that exposed individuals may send | 0.008-0.8day−1 | [26] |
message about TB | |||
μ3 | The rate that infectious individuals may send | 0.08-0.8day−1 | [26] |
message about TB | |||
μ4 | The rate that recovered individuals may send | 0-1day−1 | Estimate |
message about TB | |||
τ | The rate that message become outdated | 0.03-0.6year−1 | [26] |
Parameter | Description | Estimated value | Source |
δ | Constant recruitment rate of the population | 0.8day−1 | [14] |
β | Transmission coefficient of TB | 0.0099-0.8person−1day−1 | Estimate |
α | The coefficient that determines how effective TB | 0.00091-0.8day−1 | [14] |
information can influence the transmission rate | |||
μ | Nature death rate | 0.009-0.6year−1 | Estimate |
q | The proportion of disease by fast progression | 0-0.5year−1 | Estimate |
ε | The progression rate from E to I | 0.02-0.99day−1 | Estimate |
d | The disease-related death rate of TB | 0.002-0.5day−1 | Estimate |
γ | The recovery rate of TB | 0.006-0.99day−1 | Estimate |
μ1 | The rate that susceptible individuals may send | 0.04-0.99day−1 | [26] |
message about TB | |||
μ2 | The rate that exposed individuals may send | 0.008-0.8day−1 | [26] |
message about TB | |||
μ3 | The rate that infectious individuals may send | 0.08-0.8day−1 | [26] |
message about TB | |||
μ4 | The rate that recovered individuals may send | 0-1day−1 | Estimate |
message about TB | |||
τ | The rate that message become outdated | 0.03-0.6year−1 | [26] |