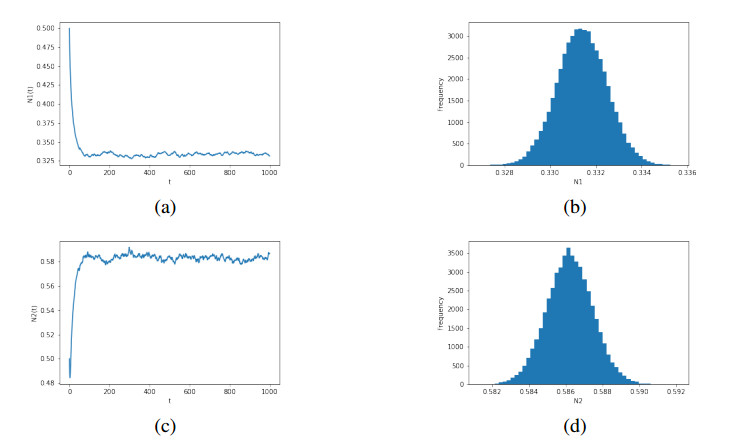
Citation: Eduardo Ibargüen-Mondragón, Lourdes Esteva, Edith Mariela Burbano-Rosero. Mathematical model for the growth of Mycobacterium tuberculosis in the granuloma[J]. Mathematical Biosciences and Engineering, 2018, 15(2): 407-428. doi: 10.3934/mbe.2018018
[1] | Virginia Giorno, Amelia G. Nobile . Exact solutions and asymptotic behaviors for the reflected Wiener, Ornstein-Uhlenbeck and Feller diffusion processes. Mathematical Biosciences and Engineering, 2023, 20(8): 13602-13637. doi: 10.3934/mbe.2023607 |
[2] | Buyu Wen, Bing Liu, Qianqian Cui . Analysis of a stochastic SIB cholera model with saturation recovery rate and Ornstein-Uhlenbeck process. Mathematical Biosciences and Engineering, 2023, 20(7): 11644-11655. doi: 10.3934/mbe.2023517 |
[3] | Junjing Xiong, Xiong Li, Hao Wang . The survival analysis of a stochastic Lotka-Volterra competition model with a coexistence equilibrium. Mathematical Biosciences and Engineering, 2019, 16(4): 2717-2737. doi: 10.3934/mbe.2019135 |
[4] | Virginia Giorno, Serena Spina . On the return process with refractoriness for a non-homogeneous Ornstein-Uhlenbeck neuronal model. Mathematical Biosciences and Engineering, 2014, 11(2): 285-302. doi: 10.3934/mbe.2014.11.285 |
[5] | Aniello Buonocore, Luigia Caputo, Enrica Pirozzi, Maria Francesca Carfora . A simple algorithm to generate firing times for leaky integrate-and-fire neuronal model. Mathematical Biosciences and Engineering, 2014, 11(1): 1-10. doi: 10.3934/mbe.2014.11.1 |
[6] | Yuanshi Wang, Donald L. DeAngelis . A mutualism-parasitism system modeling host and parasite with mutualism at low density. Mathematical Biosciences and Engineering, 2012, 9(2): 431-444. doi: 10.3934/mbe.2012.9.431 |
[7] | Meng Gao, Xiaohui Ai . A stochastic Gilpin-Ayala mutualism model driven by mean-reverting OU process with Lévy jumps. Mathematical Biosciences and Engineering, 2024, 21(3): 4117-4141. doi: 10.3934/mbe.2024182 |
[8] | Giuseppe D'Onofrio, Enrica Pirozzi . Successive spike times predicted by a stochastic neuronal model with a variable input signal. Mathematical Biosciences and Engineering, 2016, 13(3): 495-507. doi: 10.3934/mbe.2016003 |
[9] | Jinyu Wei, Bin Liu . Global dynamics of a Lotka-Volterra competition-diffusion-advection system for small diffusion rates in heterogenous environment. Mathematical Biosciences and Engineering, 2021, 18(1): 564-582. doi: 10.3934/mbe.2021031 |
[10] | Sheng Wang, Lijuan Dong, Zeyan Yue . Optimal harvesting strategy for stochastic hybrid delay Lotka-Volterra systems with Lévy noise in a polluted environment. Mathematical Biosciences and Engineering, 2023, 20(4): 6084-6109. doi: 10.3934/mbe.2023263 |
Competitive relationships between different species are common among populations of organisms. Interspecific competition is a phenomenon in which two or more populations living together struggle for ecological resources. For example, crops and weeds in the same field are two populations competing with each other, and there is also competition between cattle and sheep in the same grassland. As a result, competition models are also important objects of study in mathematical biology. Mathematical models of interspecific competition can effectively explain and predict populations' changing rules and development trends in the process of mutual competition.
In the 1920s, Volterra proposed the famous Lotka-Volterra model based on the predator-prey relationship [1]. (Lotka had also proposed this model in his chemical reaction studies.) This model has resulted in a breakthrough in population model study and piqued the interest of a wide range of academics. After the predator-prey model, the Lotka-Volterra competition model was proposed and a series of studies on competition models were obtained. We refer the reader to [2,3,4,5,6,7,8] and the references therein. Specifically, a classic two-species competition model can be expressed as follows.
{dN1(t)=N1(t)[r1−b11N1(t)−b12N2(t)]dt,dN2(t)=N2(t)[r2−b21N1(t)−b22N2(t)]dt, | (1.1) |
where Ni(t) (i=1,2) denotes the population size of the ith species at time t; ri is the intrinsic rate of increase; bii denotes the intraspecific competition rate of the species. Since two populations compete with each other, b12 denotes the effect of Population 2 on Population 1, and b21 denotes the effect of Population 1 on Population 2. In general, b12≠b21. All parameters in Eq (1.1) are positive. Regarding the equilibrium points of Eq (1.1), we have the following conclusions [9].
1) Equilibrium E0=(0,0) always exists.
2) Equilibrium E1=(r1b11,0) always exists.
3) Equilibrium E2=(0,r2b22) always exists.
4) Define α=b22r1−b12r2, β=−b21r1+b11r2 and γ=b11b22−b12b21. If the condition α>0, β>0 or α<0, β<0 holds, equilibrium E∗=(N∗1,N∗2)=(αγ,βγ) always exists.
The discussion of Eq (1.1) does not consider the effects of environmental disturbances, while in real life, the dynamic behaviors of biological populations are inevitably affected by environmental disturbances such as air humidity and weather changes. Several scholars' studies have proved this view. Mode and Jacobson [10] found that the probability of population extinction is sensitive to environmental perturbations. DuBowy [11] found that seasonal shifts affect population growth and community composition. And Mao et al. [12] showed that environmental noise can inhibit population size surges. Therefore, the introduction of stochastic factors is essential.
There are mainly two ways to characterize environmental disturbances. One method is to directly introduce environmental noises to a deterministic model, and it has been studied and used by many scholars [13,14,15,16,17,18]. Another approach is to simulate random environmental perturbations through the use of a stochastic process. Allen's study [19] illustrated that a stochastic Ornstein-Uhlenbeck process (also known as the mean-reverting process) possesses several advantages over linear functions of white noise in terms of being able to modify parameters for environmental variability, which indicated that the second way is a feasible and biologically meaningful approach. The study by Zhang and Yuan [20] showed that the Ornstein-Uhlenbeck process is an effective and reasonable way to introduce environmental noise into the continuous culture model of microorganisms. It was also found that the reversion speed and volatility intensity have essential effects on the extinction and persistence of microorganisms. Song and Zhang [21] proposed a new stochastic SVEIS model with the Ornstein-Uhlenbeck process and studied this model's stationary distribution and extinction. Yang et al. [22] introduced the Ornstein-Uhlenbeck process into a food chain system to simulate stochastic perturbation, and some valuable theoretical results were obtained. For the related study on the Ornstein-Uhlenbeck process, readers can refer to [19,20,21,22,23,24,25,26,27,28]. Previous studies have shown that introducing the Ornstein-Uhlenbeck process into the model is worth investigating in depth. However, as far as we know, no one has studied a competition model with the Ornstein-Uhlenbeck process.
In fact, the growth rate and death rate of the population are easily disturbed by environmental changes. Therefore, we consider a comparison of two methods to incorporate environmental variability into the intrinsic rates of increase. There are some differences between the Ornstein-Uhlenbeck noise and conventional Gaussian white noise, and these differences made us prefer to use the Ornstein-Uhlenbeck process to model this environmental variability.
1) The first approach is to introduce Gaussian white noise, specifically by making the following transformation to r1 and r2:
r1→r1(t)=θr1+ξr1dBr1(t)dt,r2→r2(t)=θr2+ξr2dBr2(t)dt, | (1.2) |
where Br1(t) and Br2(t) are mutually independent Brownian motions. By directly integrating Eq (1.2), the average per intrinsic rates of increase over an interval [0,T] is equal to
ˉr1=1T∫T0r1(t)dt=θr1+ξr1Br1(T)T∼N(θr1,ξ2r1T), |
ˉr2=1T∫T0r2(t)dt=θr2+ξr2Br2(T)T∼N(θr2,ξ2r2T). |
That is, the variances of the average intrinsic rates of increase ˉr1 and ˉr2 over an interval of length T both tend to infinity as T→0.
Specifically operating the second method, i.e., we assume that r1 and r2 have a transformation of the following form:
r1→r1+m1(t),r2→r2+m2(t). | (1.3) |
m1(t) and m2(t) are the Ornstein-Uhlenbeck processes which satisfy
dm1(t)=−θ1m1(t)dt+ξ1dB1(t),dm2(t)=−θ2m2(t)dt+ξ2dB2(t), | (1.4) |
where θi is the speed of reversion and ξi is the intensity of volatility of the process mi(t) (θi>0,ξi>0,i=1,2). B1(t) and B2(t) are mutually independent Brownian motions. After calculation, Eq (1.4) can be solved exactly to yield
mi(t)=mi0e−θit+∫t0ξie−θi(t−s)dBi(s), | (1.5) |
where mi0 is the initial value of the process mi(t). From Eq (1.5), we obtain that mi(t)∼N(mi0e−θit, θi22ξi(1−e−2θit)). It is not difficult to find that the process mi(t) follows the distribution N(0,θi22ξi) as t tends to infinity. By directly integrating Eq (1.5), the average per intrinsic rates of increase over an interval [0,T] is equal to
ˉri=ri+ˉmi=ri+1T∫T0mi(t)dt=ri+1T∫T0ξiθi(1−e−θi(T−s))mi(t)dBi(s). |
For small values of T, we have
Var(ˉri)=ξ2iT3+o(T2). |
It is not difficult to find that, unlike the results for Gaussian white noise, the variance goes to 0 rather than ∞ as T→0.
2) For a small time interval Δt, an Ornstein-Uhlenbeck process X(t) has a correlation coefficient ρ(X(t),X(t+Δt))=1−o(Δt) while ρ(X(t),X(t+Δt))=0 for Gaussian white noise. So white noise processes are often used to model random perturbations with very small correlation periods. However, the environment of many biological systems can be considered as continuously varying due to a large number of interacting variables. Therefore, the Ornstein-Uhlenbeck process is more suitable for modeling the parameters in this paper.
Motivated by the above discussion, we consider using the Ornstein-Uhlenbeck process to simulate the stochastic process. The interspecific competition model with the Ornstein-Uhlenbeck process studied in this paper is obtained by combining Eqs (1.1), (1.3) and (1.4):
{dN1(t)=N1(t)[r1+m1(t)−b11N1(t)−b12N2(t)]dt,dN2(t)=N2(t)[r2+m2(t)−b21N1(t)−b22N2(t)]dt,dm1(t)=−θ1m1(t)dt+ξ1dB1(t),dm2(t)=−θ2m2(t)dt+ξ2dB2(t). | (1.6) |
Throughout this paper, we let (Ω,F,{Ft}t≥0,P) be a complete probability space with a filtration {Ft}t≥0 satisfying the usual conditions (i.e., it is right continuous and increasing while F0 contains all P-null sets). And we denote Rn+={x∈Rn:xi>0,1≤i≤n}, R+=[0,+∞).
This paper is organized as follows. In the next section, we investigate the existence and uniqueness of the global solution to the model (1.6). In Section 3, we discuss the cases of population extinction. In Section 4, we obtain sufficient conditions for the existence of the stationary distribution; the expression for the mean and covariance of the density function of the linearized system corresponding to the stochastic model (1.6) around the original point are obtained in Section 5. In Section 6, the previous theoretical results are verified by numerical simulations. Finally, a brief conclusion is given.
Since N1(t) and N2(t) denote the number of individuals, they should be non-negative from the viewpoint of biology. To study the long-term dynamical behaviors of the stochastic model (1.6) proposed in this paper, we first need to consider whether the solution is global and non-negative, which is a fundamental condition for the follow-up study. So in this section, we obtain the following theorem, which guarantees the existence and uniqueness of the global positive solution for the model (1.6).
Theorem 1. For any given initial value (N1(0),N2(0),m1(0),m2(0))∈R2+×R2, the model (1.6) has a unique solution (N1(t),N2(t),m1(t),m2(t))∈R2+×R2 for all t≥0 with a probability of one.
Proof. Apparently, coefficients of the model (1.6) satisfy the local Lipschitz condition; so, for any given initial value (N1(0),N2(0),m1(0),m2(0))∈R2+×R2, there exists a unique solution (N1(t),N2(t),m1(t),m2(t))∈R2+×R2, t∈[0,ρe), where ρe is the explosion time. To prove the global nature of the solution, we only need to prove that ρe=∞ a.s. Let l0>0 be sufficiently large such that N1(0),N2(0),em1(0) and em2(0) all lie within the interval [1l0,l0]. For each integer l>l0, define the stopping time [29] as follows:
τl=inf{t∈(0,ρe):min{N1(0),N2(0),em1(0),em2(0)}≤1l or max{N1(0),N2(0),em1(0),em2(0)}≥l}, |
where infϕ=∞. If we can show that τ∞=∞ a.s., then ρe=∞ a.s., and, further, Theorem 1 is proved.
Now, considering the contradiction, we assume that there exists a pair of constants T>0 and ε∈(0,1) such that P(τ∞≤T)>ε. Hence there is an integer l1≥l0 such that P(τl≤T)≥ε, ∀l≥l1. Define C2-function V:R2+×R2→R+,
V(N1(t),N2(t),m1(t),m2(t))=N1(t)−1−lnN1(t)+N2(t)−1−lnN2(t)+m41(t)4+m42(t)4. |
Applying Itô's formula to V, we get
dV=LVdt+m31ξ1dB1(t)+m32ξ2dB2(t), | (2.1) |
where
LV=(r1N1+m1N1−b11N21−b12N1N2−r1−m1+b11N1+b12N2) +(r2N2+m2N2−b21N1N2−b22N22−r2−m2+b21N1+b22N2) −θ1m41−θ2m42+32ξ21m21+32ξ22m22≤−b11N21−b22N22+(r1+b11+b21)N1+(r2+b12+b22)N2−m1−m2−r1 −r2+23N321+13|m1|3 +23N322+13|m2|3−θ1m41−θ2m42+32ξ21m21+32ξ22m22≤sup(m1,m2)∈R2 [ −θ1m41−θ2m42+13|m1|3+13|m2|3+32ξ21m21+32ξ22m22−m1−m2] +sup(N1,N2)∈R2+[−b11N21−b22N22+23N321+23N322+(r1+b11+b21)N1+(r2+b12+b22)N2] −r1−r2≤k1; |
k1 is a positive constant that is independent of the initial value. Integrating both sides of Eq (2.1) from 0 to τl∧T and then taking expectations, we get
EV(N1(τl∧T),N2(τl∧T),m1(τl∧T),m2(τl∧T)) ≤V(N1(0),N2(0),m1(0),m2(0))+k1E(τl∧T) ≤V(N1(0),N2(0),m1(0),m2(0))+k1T. |
Let ˜Ω={ϖ∈Ω:τl≤T}, where ϖ represents a sample point; then, we have P(˜Ω)≥ε, ε∈(0,1); thus,
V(N1(0),N2(0),m1(0),m2(0))+k1T ≥E[I˜ΩV(N1(τl∧T),N2(τl∧T),m1(τl∧T),m2(τl∧T))] ≥ε[(l−1−lnl)∧(1l−1+lnl)∧(lnl)44], |
where I˜Ω is the indicator function of ˜Ω. Let l→∞; we obtain
∞>V(N1(0),N2(0),m1(0),m2(0))+k1T=∞, |
which is a contradiction. This completes the proof of Theorem 1.
Theorem 2. For any given initial value (N1(0),N2(0),m1(0),m2(0))∈R2+×R2, the solution (N1(t),N2(t), m1(t),m2(t)) of the model (1.6) has the property that for any p≥1, there exists a constant k(p) such that
limsupt→∞ENpi≤k(p),i=1,2. |
Moreover,
limsupt→∞logNi(t)t≤0,i=1,2 a.s. |
Proof. Define a Lyapunov function as
V(N1(t),N2(t),m1(t),m2(t))=(N1(t)+N2(t))pp+m4p1(t)4p+m4p2(t)4p, |
where p≥1. Applying Itô's formula to V, we get
LV=(N1+N2)p−1[−b11N21−b22N22−(b12+b21)N1N2+r1N1+r2N2+m1N1+m2N2] −2∑i=1θim4pi+4p−122∑i=1ξ2im4p−2i≤(N1+N2)p−1[−12min{b11,b22}(N1+N2)2+max{r1,r2}(N1+N2)+(|m1|+|m2|)(N1+N2)] −2∑i=1θim4pi+4p−122∑i=1ξ2im4p−2i≤−12min{b11,b22}(N1+N2)p+1+max{r1,r2}(N1+N2)p+4p2p+1(N1+N2)1+12p −2∑i=1θim4pi+4p−122∑i=1ξ2im4p−2i+|m1|2p+12p+1+|m2|2p+12p+1≤−14min{b11,b22}(N1+N2)p+1−122∑i=1θim4pi+k2+k3, |
where
k2=sup(N1,N2)∈R2+[−14min{b11,b22}(N1+N2)p+1+max{r1,r2}(N1+N2)p+4p2p+1(N1+N2)1+12p]<+∞,k3=sup(m1,m2)∈R2[−122∑i=1θim4pi+4p−122∑i=1ξ2im4p−2i+|m1|2p+12p+1+|m2|2p+12p+1]<+∞. |
For any constant δ which satisfies 0<δ4p<θi2,i=1,2, we have
L(eδtV(N1,N2,m1,m2)=eδt(δV+LV)≤eδt(−14min{b11,b22}(N1+N2)p+1+δp(N1+N2)p −122∑i=1θim4pi +δ4p2∑i=1m4pi+k2+k3)≤ˉk(p)eδt, |
where
ˉk(p)=−14min{b11,b22}(N1+N2)p+1+δp(N1+N2)p−122∑i=1θim4pi +δ4p2∑i=1m4pi+k2+k3<+∞. |
Thus,
E(eδtV)=V(N1(0),N2(0),m1(0),m2(0))+E∫t0eδs(δV+LV)ds≤V(N1(0),N2(0),m1(0),m2(0))+E∫t0eδsˉk(p)ds≤V(N1(0),N2(0),m1(0),m2(0))+ˉk(p)δ(eδt−1), |
which implies
limsupt→∞EV(N1,N2,m1,m2)≤ˉk(p)δ:=k(p) a.s. |
There exists a constant h(p)>0 such that E[(N1(t)+N2(t))p]≤h(p),t≥0 a.s. For convenience, define N(t)=(N1(t)+N2(t))p=xp(t). Applying Itô's formula to N(t) yields
LN=pxp−1[−b11N21−b22N22−(b12+b21)N1N2+(r1+m1)N1+(r2+m2)N2]≤−p2min{b11,b22}xp+1+pmax{r1,r2}xp+4p22p+1x1+12p+|m1|2p+1+|m2|2p+12p+1. |
Let θ>0 be sufficiently small and satisfy nθ≤t≤(n+1)θ,n=1,2,…. It follows that
E[supnθ≤t≤(n+1)θxp(t)]=E[xp(nθ)]+I, |
where
I=E[supnθ≤t≤(n+1)θ|∫tnθLNds|]≤pmax{r1,r2}E∫(n+1)θnθxp(s)ds+4p22p+1E∫(n+1)θnθx1+12p(s)ds +p2p+1E∫(n+1)θnθ(|m1|2p+1(s)+|m2|2p+1(s))pds≤pmax{r1,r2}θE[supnθ≤t≤(n+1)θxp(t)]+4p22p+1θE[supnθ≤t≤(n+1)θx1+12p(t)] +p2p+1θE[supnθ≤t≤(n+1)θ(|m1|2p+1(t)+|m2|2p+1(t))p]. |
Choose θ sufficiently small such that I<h(p); therefore,
E[supnθ≤t≤(n+1)θxp(t)]<2h(p). |
Let ε be an arbitrary positive constant; then, based on Chebyshev's inequality [30], it follows that
P{supnθ≤t≤(n+1)θxp(t)>(nθ)1+ε}≤2h(p)(nθ)1+ε,n=1,2,…. |
There exists an integer-valued random variable n0(ω) such that for almost all ω∈Ω, when n≥n0, we have
supnθ≤t≤(n+1)θxp(t)≤(nθ)1+ε. |
If n≥n0 and nθ≤t≤(n+1)θ, we get
limsupt→∞logxp(t)logt≤limsupt→∞(1+ε)log(nθ)log(nθ)≤1+ε a.s. |
Let ε→0; we get
limsupt→∞logxp(t)logt≤1 a.s.; |
then
limsupt→∞logx(t)logt≤1p a.s. |
Thus,
limsupt→∞logx(t)t≤limsupt→∞logx(t)logt×limsupt→∞logtt≤0, |
and it follows that
limsupt→∞log(Ni(t))t≤0,i=1,2 a.s. |
In this section, the extinction of populations is discussed. First, we give the following lemma needed for the subsequent proof.
Lemma 1. [31] If f(t) is integrable, we define ⟨f⟩t=1t∫t0f(s)ds. Assume that z(t)∈C(Ω×[0,∞),R+).
1) If for all t>T, there are two positive constants T and δ0 satisfying
lnz(t)≤δt−δ0∫t0z(s)ds+n∑i=1αiB(t) a.s., |
where αi and δ are constants, we get
{limsupt→∞⟨z⟩t≤δδ0 a.s.,δ>0;limt→∞⟨z⟩t=0 a.s., δ<0. |
2) If for all t>T, there are three positive constants T, δ and δ0 satisfying
lnz(t)≥δt−δ0∫t0z(s)ds+n∑i=1αiB(t) a.s., |
where αi denotes constants, we get liminft→∞⟨z⟩t≥δδ0 a.s.
Theorem 3. For any given initial value (N1(0),N2(0),m1(0),m2(0))∈R2+×R2, the model (1.6) has the following properties:
1) if α>0 and β<0, then
limt→∞1t∫t0N1(s)ds=r1b11, limt→∞1t∫t0N2(s)ds=0 a.s., |
2) if α<0 and β>0, then
limt→∞1t∫t0N1(s)ds=0, limt→∞1t∫t0N2(s)ds=r2b22 a.s. |
Proof. 1) The discussion is divided into the following two cases.
Case 3.1 If α>0, β<0 and γ>0, by Itô's formula, we get
d(−b21b11lnN1(t)+lnN2(t))=[−b21b11(r1+m1(t)−b11N1(t)−b12N2(t)) +(r2+m2(t)−b21N1(t)−b22N2(t))]dt. |
According to Theorem 2, let ε1 satisfy −βb11=b21r1−b11r2b11>ε1>0 and there exist T1 which is sufficiently large such that b21b11tlnN1(t)N1(0)+1tlnN2(0)<ε1 holds for t>T1. It is easy to show that
1tlnN2(t)=b21b11tlnN1(t)N1(0)+1tlnN2(0)+−b21r1+b11r2b11−b11b22−b12b21b11⟨N2⟩t−b21b11⟨m1⟩t+⟨m2⟩t≤ε1+−b21r1+b11r2b11−b21b11⟨m1⟩t+⟨m2⟩t. |
Taking the superior limit on both sides, we have
limsupt→∞1tln(N2(t))≤ε1+−b21r1+b11r2b11<0. |
Therefore, we have that limt→∞N2(t)=0 a.s. Considering the equation
1tlnN1(t)N1(0)=r1+⟨m1⟩t−b11⟨N1⟩t−b12⟨N2⟩t, |
according to Lemma 1, we have
limt→∞⟨N1⟩t=r1b11 a.s. |
Case 3.2 Similar to Case 3.1, if α>0, β<0 and γ>0, by Itô's formula, we get
d(−lnN1(t)+b12b22lnN2(t))=[−(r1+m1t−b11N1t−b12N2t) +b12b22(r2+m2t−b21N1t−b22N2t)]dt. |
According to Theorem 2, let ε2 satisfy αb22=b22r1−b12r2b22>ε2>0 and there exist T2 which is sufficiently large such that 1tlnN1(t)N1(0)+b12b22tlnN2(0)<ε2 holds for t>T2. It is easy to show that
b12b22tlnN2(t)=1tlnN1(t)N1(0)+b12b22tlnN2(0)+−b22r1+b12r2b22+b11b22−b12b21b11⟨N1⟩t−⟨m1⟩t+b12b22⟨m2⟩t≤ε2+−b22r1+b12r2b22−⟨m1⟩t+b12b22⟨m2⟩t. |
Taking the superior limit on both sides, we have
limsupt→∞b12b22tln(N2(t))≤ε2+−b22r1+b12r2b22<0. |
Therefore, we have limt→∞N2(t)=0 a.s. Furthermore, we have
limt→∞⟨N1⟩t=r1b11 a.s. |
2) The discussion is divided into the following two cases.
Case 3.3 If α<0, β>0 and γ>0, by Itô's formula, we get
d(b22b12lnN1(t)−lnN2(t))=[b22b12(r1+m1(t)−b11N1(t)−b12N2(t)) −(r2+m2(t)−b21N1(t)−b22N2(t))]dt. |
According to Theorem 2, let ε3 satisfy −αb12=−b22r1+b12r2b12>ε3>0 and there exist T3 which is sufficiently large such that b22b12tlnN1(0)+1tlnN2(t)N2(0)<ε3 holds for t>T3. It is easy to show that
b22b12tlnN1(t)=b22b12tlnN1(0)+1tlnN2(t)N2(0)+b22r1−b12r2b12−b11b22−b12b21b11⟨N1⟩t+b22b12⟨m1⟩t−⟨m2⟩t≤ε3+b22r1−b12r2b12+b22b12⟨m1⟩t−⟨m2⟩t. |
Taking the superior limit on both sides, we have
limsupt→∞b22b12tln(N1(t))≤ε3+b22r1−b12r2b12<0. |
Therefore, we have that limt→∞N1(t)=0 a.s. Considering the equation
1tlnN2(t)N2(0)=r2+⟨m2⟩t−b21⟨N1⟩t−b22⟨N2⟩t, |
according to Lemma 1, we have
limt→∞⟨N2⟩t=r2b22 a.s. |
Case 3.4 Similar to Case 3.3, if α<0, β>0 and γ<0, by Itô's formula, we get
d(lnN1(t)−b11b21lnN2(t))=[(r1+m1(t)−b11N1(t)−b12N2(t)) −b11b21(r2+m2(t)−b21N1(t)−b22N2(t))]dt. |
According to Theorem 2, let ε4 satisfy βb21=−b21r1+b11r2b21>ε4>0 and there exist T4 which is sufficiently large such that 1tlnN1(0)+b11b21tlnN2(t)N2(0)<ε4 holds for t>T4. It is easy to show that
1tlnN1(t)=1tlnN1(0)+b11b21tlnN2(t)N2(0)+b21r1−b11r2b21+b11b22−b12b21b11⟨N2⟩t+⟨m1⟩t−b11b21⟨m2⟩t≤ε4+b21r1−b11r2b21+⟨m1⟩t−b11b21⟨m2⟩t. |
Taking the superior limit on both sides, we have
limsupt→∞1tln(N1(t))≤ε4+b21r1−b11r2b21<0. |
Therefore, we have that limt→∞N1(t)=0 a.s. Furthermore, we have
limt→∞⟨N2⟩t=r2b22 a.s. |
In this section, a sufficient condition for the existence of the stationary distribution in the model (1.6) is obtained. Before giving the theorem, we present the following lemma needed for the subsequent proof.
Lemma 2. [32] X(t) is a homogeneous Markov process which is expressed as
dX(t)=b(X(t))dt+k∑r=1σr(X(t))dBr(t). |
Assume that b(X),σ1(X),⋯,σk(X)(t≥t0,x∈Rd) are continuous.
1) There is a constant B that satisfies
|b(X1)−b(X2)|+k∑r=1|σ1(X1)−σ2(X2)|≤B|X1−X2|,|b(X)|+k∑r=1|σr(X)|≤B(1+|X|). |
2) A non-negative U(x)∈C2 in Rd that satisfies LU(x)≤−1 outside of some compact set exists.
If Conditions (1) and (2) hold, X(t) is a stationary Markov process.
Theorem 4. For any given initial value (N1(0),N2(0),m1(0),m2(0))∈R2+×R2, suppose that the condition α>0,β>0 holds. If
w<min{(b11−b12+b212−ξ12)(N∗1)2,(b22−b12+b212−ξ22)(N∗2)2}, |
where w=ξ12θ1+ξ22θ2, then the model (1.6) has a stationary distribution.
Proof. For the model (1.6), Condition (1) of Lemma 2 holds. Thus, we only need to verify Condition (2). Consider the following function:
V(N1(t),N2(t),m1(t),m2(t))=(N1(t)−N∗1−N∗1lnN1(t)N∗1)+(N2(t)−N∗2−N∗2lnN2(t)N∗2) +1θ1ξ1m21(t)+1θ2ξ2m22(t), |
where N∗1=b22r1−b12r2b11b22−b12b21>0 and N∗2=−b21r1+b11r2b11b22−b12b21>0. For the sake of convenience, define
V1(t)=N1(t)−N∗1−N∗1lnN1(t)N∗1,V2(t)=N2(t)−N∗2−N∗2lnN2(t)N∗2,V3(t)=1θ1ξ1m21(t)+1θ2ξ2m22(t). |
Using Itô's formula, we get
LV1=(N1−N∗1)(r1+m1−b11N1−b12N2)=(N1−N∗1)(b11N∗1+b12N∗2+m1−b11N1−b12N2)≤−(b11−ξ12)(N1−N∗1)2−b12(N1−N∗1)(N2−N∗2)+m212ξ1≤−(b11−b122−ξ12)(N1−N∗1)2+b122(N2−N∗2)2+m212ξ1,LV2≤−(b22−b212−ξ22)(N2−N∗2)2+b212(N2−N∗2)2+m222ξ2,LV3=−1ξ1m21−1ξ2m22+ξ12θ1+ξ22θ2. |
Therefore,
LV≤−(b11−b12+b212−ξ12)(N1−N∗1)2−(b22−b12+b212−ξ22)(N2−N∗2)2−12ξ1m21−12ξ2m22+w, |
where w=ξ12θ1+ξ22θ2. If
w<min{(b11−b12+b212−ξ12)(N∗1)2,(b22−b12+b212−ξ22)(N∗2)2} |
holds, then the ellipsoid −(b11−b12+b212−ξ12)(N1−N∗1)2−(b22−b12+b212−ξ22)(N2−N∗2)2−12ξ1m21−12ξ2m22+w lies entirely in R2+×R2. We can take U to be a neighborhood of the ellipsoid with ˉU⊆R2+×R2 where ˉU represents the compact closure of U. Model (1.6) is known to satisfy Condition (1) of Lemma 2, and we have just proved that Condition (2) is true. Hence, the model (1.6) has a stationary distribution according to Lemma 2.
In this section, the formulas for the mean and the covariance of the probability density function of the corresponding linearized system near the equilibrium point are obtained. For the model (1.6), the equilibrium is E∗=(N∗1,N∗2,0,0). Let ui=Ni−N∗i,i=1,2; the corresponding linearized model of the model (1.6) is
{du1=(−a11u1−a12u2+N∗1m1)dt,du2=(−a21u1−a22u2+N∗2m2)dt,dm1(t)=−θ1m1(t)dt+ξ1dB1(t),dm2(t)=−θ2m2(t)dt+ξ2dB2(t), | (5.1) |
where a11=b11N∗1>0, a12=b12N∗1>0, a21=b21N∗2>0 and a22=b22N∗2>0.
Theorem 5. For any given initial value (N1(0),N2(0),m1(0),m2(0))∈R2+×R2, if the conditions of Theorem 4 hold, then the model (5.1) has a stationary distribution around the original point. The mean vector is (0,0,0,0), and the covariance matrix has the following form:
Σ = α21(I3I2I1)−1Σ01[(I3I2I1)−1]T+α22(J3J2J1)−1Σ02[(J3J2J1)−1]T, |
where
p1=a11+a22, p2=a11a22−a21a12, α1=a21ξ1N∗1, α2=a12ξ2N∗2, η1 = 2θ21p1+2θ1p21+2p1p2, η2 = 2θ22p1+2θ1p21+2p1p2,
I1=(0010100001000001),I2=(10000−a21−a22000100001),I3=(−a21N∗1−p1−p2−a22N∗2010000100001),J1=(0001010010000010),J2=(10000−a12−a11000100001),J3=(−a12N∗2−p1−p2−a11N∗1010000100001),Σ01=(θ1p1+p2η10−1η1001η100−1η10θ1+p1θ1p2η100000),Σ02=(θ2p1+p2η20−1η2001η200−1η20θ2+p1θ2p2η200000). |
Proof. Let X=(u1,u2,m1,m2)T, B(t)=(0,0,B1(t),B2(t))T and
A=(−a11−a12N∗10−a21−a220N∗200−θ10000−θ2), H=(0000000000ξ10000ξ2); |
then the model (5.1) is transformed into dX=AX(t)dt+HdB(t). Then we get
H2+AΣ+ΣAT=0. | (5.2) |
Since B1(t) and B2(t) are mutually independent, Equation (5.2) can be equivalently transformed into the following algebraic sub-equations:
H2i+AΣi+ΣiAT=0,i=1,2, |
where H21=diag(0,0,ξ21,0), H22=diag(0,0,0,ξ22) and Σ=Σ1+Σ2. Let B=(−a11−a12−a21−a22); the characteristic polynomial of matrix B is
ϕB(λ)=λ2+(a11+a22)λ+a11a22−a12a21, |
where p1=a11+a22>0, p2=a11a22−a12a21>0 and p1p2=(a11+a22)(a11a22−a12a21)>0. According to the Routh-Hurwitz criterion [33], matrix B has all negative real-part eigenvalues. Next, we prove Theorem 5 in two cases.
Case 5.1 Consider the equation
H21+AΣ1+Σ1AT=0. | (5.3) |
Let A1=I1AI−11, where I1=(0010100001000001); we have
A1=(−θ1000N∗1−a11−a1200−a21−a22N∗2000−θ2). |
Next, let A2=I2A1I−12, where I2=(10000−a21−a22000100001); we therefore have
A2=(−θ1000−a21N∗1−p1−p2−a22N∗2010N∗2000−θ2). |
Let A3=I3A2I−13, where I3=(−a21N∗1−p1−p2−a22N∗2010000100001); thus,
A3=(−θ1−p1−θ1p1−p2−θ1p2(θ2−θ1)a22N∗2−p2N∗21000010N∗2000−θ2). |
Equation (5.3) is transformed into
(I3I2I1)H21(I3I2I1)T+A3(I3I2I1)Σ1(I3I2I1)T+(I3I2I1)Σ1(I3I2I1)TAT3=0. |
Denote Σ01 = 1α21(I3I2I1)Σ1(I3I2I1)T, where α1=a21ξ1N∗1; we can get
Σ01=(θ1p1+p2η10−1η1001η100−1η10θ1+p1θ1p2η100000), |
where η1 = 2θ21p1+2θ1p21+2p1p2.
Σ01 is a positive semi-definite matrix, and Σ1 = α21(I3I2I1)−1Σ01[(I3I2I1)−1]T is also a positive semi-definite matrix.
Case 5.2 Consider the equation
H22+AΣ2+Σ2AT=0. | (5.4) |
Let B1=J1AJ−11, where J1=(0001010010000010); we have
B1=(−θ2000N∗2−a22−a2100−a12−a11N∗1000−θ1). |
Next, let B2=J2B1J−12, where J2=(10000−a12−a11000100001); we therefore have
B2=(−θ2000−a12N∗2−p1−p2−a11N∗1010N∗1000−θ1). |
Let B3=J3B2J−13, where J3=(−a12N∗2−p1−p2−a11N∗1010000100001); thus,
B3=(−θ2−p1−θ2p1−p2−θ2p2(θ1−θ2)a11N∗1−p2N∗11000010N∗1000−θ1). |
Equation (5.4) is transformed into
(J3J2J1)H22(J3J2J1)T+B3(J3J2J1)Σ2(J3J2J1)T+(J3J2J1)Σ2(J3J2J1)TBT3=0. |
Denote Σ02 = 1α22(J3J2J1)Σ2(J3J2J1)T, where α2=a12ξ2N∗2; we can get
Σ02=(θ2p1+p2η20−1η2001η200−1η20θ2+p1θ2p2η200000), |
where η2 = 2θ22p1+2θ1p21+2p1p2.
Σ02 is a positive semi-definite matrix, and Σ2 = α22(J3J2J1)−1Σ02[(J3J2J1)−1]T is also a positive semi-definite matrix. The proof is complete.
This section gives examples of numerical simulations to verify the previous results. Specifically, we will verify the following results:
1) The effect of the change of ξi in the Ornstein-Uhlenbeck process.
2) When the conditions of Theorems 3 or 4 are satisfied, observe whether the images resulting from numerical simulation agree with the theory.
3) Compare and observe the images of the deterministic and stochastic models.
The corresponding discrete model of the model (1.6) is
{Nk+11=Nk1+Nk1[r1+mk1−b11Nk1−b12Nk2]Δt,Nk+12=Nk2+Nk2[r2+mk2−b21Nk1−b22Nk2]Δt,mk+11=mk1−θ1mk1Δt+ξ1√Δtνk+ξ212(ν2k−1)Δt,mk+12=mk2−θ2mk2Δt+ξ2√Δtlk+ξ222(l2k−1)Δt, | (6.1) |
where Δt>0 is the time increment and νk and lk are the mutually independent Gaussian random variables with distribution N(0,1) for k=1,2,…,n. Nk1,Nk2, mk1 and mk2 denote the corresponding values of the kth iteration of the model (6.1).
And the corresponding discrete model of the deterministic model (1.1) is
{Nk+11=Nk1+Nk1[r1−b11Nk1−b12Nk2]Δt,Nk+12=Nk2+Nk2[r2−b21Nk1−b22Nk2]Δt, | (6.2) |
where Δt>0 is the time increment and Nk1 and Nk2 denote the corresponding values of the kth iteration of the model (6.2).
1) We will verify the correctness of Theorem 4 and consider the effect of the change of ξi in the Ornstein-Uhlenbeck process.
Example 1. Let r1=0.4,r2=0.5,b11=0.5,b12=0.4,b21=0.45,b22=0.6,θ1=θ2=0.5,N1(0)=0.5,N2(0)=0.5,M1(0)=0.001 and M2(0)=0.001. If ξ1=ξ2=0.001, then α=0.04>0, β=0.07>0 and w=0.002<min{0.0083,0.0594}. The corresponding image can be seen in Figure 1.
Example 2. Similarly, let r1=0.4,r2=0.5,b11=0.5,b12=0.4,b21=0.45,b22=0.6,θ1=θ2=0.5,N1(0)=0.5,N2(0)=0.5,M1(0)=0.001 and M2(0)=0.001. If ξ1=0.005 and ξ2=0.001, then α=0.04>0, β=0.07>0 and w=0.006<min{0.0081,0.0594}. The corresponding image can be seen in Figure 2.
Example 3. Similarly, let r1=0.4,r2=0.5,b11=0.5,b12=0.4,b21=0.45,b22=0.6,θ1=θ2=0.5,N1(0)=0.5,N2(0)=0.5,M1(0)=0.001 and M2(0)=0.001. If ξ1=ξ2=0.005, then α=0.04>0, β=0.07>0 and w=0.01>min{0.0081,0.0587}. The corresponding image can be seen in Figure 3.
Figures 1 and 2 show that if the conditions of Theorem 4 hold, the two species of the model (1.6) can coexist in the long term. By comparing Figures 1–3, we find that when other parameters and initial values remain the same, the intensity of the fluctuations in the plot increases if ξi, i.e., is the intensity of the volatility of the process increases.
2) There are two examples of extinction.
Example 4. Let r1=0.4, r2=0.5, b11=0.5, b12=0.4, b21=0.7, b22=0.6, θ1=θ2=0.5, ξ1=ξ2=0.01, N1(0)=0.5, N2(0)=0.5, M1(0)=0.001 and M2(0)=0.001; then, α=0.04>0 and β=−0.03<0.
By Theorem 3, Population 2 will go extinct eventually and
limt→∞1t∫t0N1(s)ds=0.8. |
The corresponding image can be seen in Figure 4.
Example 5. Let r1=0.4, r2=0.5, b11=0.5, b12=0.5, b21=0.61, b22=0.6, θ1=θ2=0.5, ξ1=ξ2=0.01, N1(0)=0.5, N2(0)=0.5, M1(0)=0.001 and M2(0)=0.001; then, α=−0.01<0 and β=0.01>0.
By Theorem 3, Population 1 will go extinct eventually and
limt→∞1t∫t0N2(s)ds=0.8333. |
The corresponding image can be seen in Figure 5.
From Figures 4 and 5, it is not difficult to find that the result is in line with Theorem 3.
3) We consider the comparison of the deterministic model (1.1) and the stochastic model (1.6).
Example 6. Consider the discrete models (6.1) and (6.2), take the parameters as r1=0.4, r2=0.5, b11=0.5, b12=0.4, b21=0.45, b22=0.6, θ1=θ2=0.5 and ξ1=ξ2=0.0006 and set the initial values as N1(0)=0.5, N2(0)=0.5, M1(0)=0.001 and M2(0)=0.001. The corresponding image can be seen in Figure 6.
It is not difficult to find that when the intensity of the volatility of the Ornstein-Uhlenbeck process is relatively small, the trends of the stochastic and deterministic model populations are similar.
Competition models are an important medium for us to study the extinction and survival of competing species in ecosystems, and they have theoretical support for the study of ecosystem balance. This paper studies the dynamical behaviors of two population competition models with the Ornstein-Uhlenbeck process. The existence and uniqueness of the system solution have been verified, the cases of population extinction have been discussed and sufficient conditions for the stationary distribution of the system have been obtained. Specifically, if α>0 and β<0 or α<0 and β>0, then one population goes extinct while the other survives. Otherwise, if α>0, β>0 and the parameters θi and ξi of the Ornstein-Uhlenbeck process meet certain conditions, then the model (1.6) exists as a stable distribution, which means that the two populations coexist in this case.
In addition to proving the existence and uniqueness of global positive solutions and obtaining sufficient conditions for population extinction and stationary distribution, the innovation of this paper is that we further obtained the results of the mathematical analysis of the density function of the stochastic model (1.6), which is challenging work. Finally, the numerical simulations with examples have been provided to verify the theoretical results of extinction and stationary distribution. It is also proved that the stochastic and the corresponding deterministic models have similar properties when the intensity of the volatility of the Ornstein-Uhlenbeck process is relatively small.
Previously, although using the Ornstein-Uhlenbeck process to model stochastic processes is an effective way to introduce environmental noise into stochastic models, there are fewer related studies. Moreover, no one has studied the dynamical behaviors of stochastic competition models with Ornstein-Uhlenbeck processes, so this paper has some reference significance for studying stochastic competition models.
Thanks to the Reviewers for their helpful comments and suggestions. This work was supported by the National Natural Science Foundation of China Tianyuan Mathematical Foundation (No. 12126312, No. 12126328). The authors gratefully acknowledge the Natural Science Foundation of Heilongjiang Province (No. LH2022E023) for the support in publishing this paper.
The authors declare that there is no conflict of interest.
[1] | [ J. Alavez,R. Avendao,L. Esteva,J. A. Flores,J. L. Fuentes-Allen,G. Garca-Ramos,G. Gmez,J. Lpez Estrada, Population dynamics of antibiotic resistant M. tuberculosis, Math Med Biol, 24 (2007): 35-56. |
[2] | [ R. Antia,J. C. Koella,V. Perrot, Model of the Within-host dynamics of persistent mycobacterial infections, Proc R Soc Lond B, 263 (1996): 257-263. |
[3] | [ M. A. Behr,W. R. Waters, Is tuberculosis a lymphatic disease with a pulmonary portal?, Lancet, 14 (2004): 250-255. |
[4] | [ S. M. Blower,T. Chou, Modeling the emergence of the hot zones: Tuberculosis and the amplification dynamics of drug resistance, Nat Med, 10 (2004): 1111-1116. |
[5] | [ C. Castillo-Chávez,B. Song, Dynamical models of tuberculosis and their applications, Math Biosci Eng, 1 (2004): 361-404. |
[6] | [ T. Cohen,M. Murray, Modelling epidemics of multidrug-resistant M. tuberculosis of heterogeneous fitness, Nat Med, 10 (2004): 1117-1121. |
[7] | [ A. M. Cooper, Cell-mediated immune responses in tuberculosis, Annu Rev Immunol, 27 (2009): 393-422. |
[8] | [ C. Dye,M. A. Espinal, Will tuberculosis become resistant to all antibiotics?, Proc R Soc Lond B, 268 (2001): 45-52. |
[9] | [ F. R. Gantmacher, The Theory of Matrices, AMS Chelsea Publishing, Providence, RI, 1998. |
[10] | [ E. Guirado,L. S. Schlesinger, Modeling the Mycobacterium tuberculosis granuloma-the critical battlefield in host immunity and disease, Frontiers in Immunology, 4 (2013): 1-7. |
[11] | [ T. Gumbo,A. Louie,M. R. Deziel,L. M. Parsons,M. Salfinger,G. L. Drusano, Drusano, Selection of a moxifloxacin dose that suppresses drug resistance in Mycobacterium tuberculosis, by use of an in vitro pharmacodynamic infection model and mathematical modeling, J Infect Dis, 190 (2004): 1642-1651. |
[12] | [ E. G. Hoal-Van Helden,D. Hon,L. A. Lewis,N. Beyers,P. D. Van Helden, Mycobacterial growth in human macrophages: Variation according to donor, inoculum and bacterial strain, Cell Biol Int, 25 (2001): 71-81. |
[13] | [ E. Ibargüen-Mondragón,L. Esteva,L. Chávez-Galán, A mathematical model for cellular immunology of tuberculosis, Math Biosci Eng, 8 (2011): 973-986. |
[14] | [ E. Ibargüen-Mondragón,L. Esteva, Un modelo matemático sobre la dinámica del Mycobacterium tuberculosis en el granuloma, Revista Colombiana de Matemáticas, 46 (2012): 39-65. |
[15] | [ E. Ibargüen-Mondragón,J. P. Romero-Leiton,L. Esteva,E. M. Burbano-Rosero, Mathematical modeling of bacterial resistance to antibiotics by mutations and plasmids, J Biol Syst, 24 (2016): 129-146. |
[16] | [ E. Ibargüen-Mondragón,S. Mosqueraa,M. Cerón,E. M. Burbano-Rosero,S. P. Hidalgo-Bonilla,L. Esteva,J. P. Romero-Leiton, Mathematical modeling on bacterial resistance to multiple antibiotics caused by spontaneous mutations, BioSystems, 117 (2014): 60-67. |
[17] | [ S. Kaufmann, How can immunology contribute to the control of tuberculosis?, Nat Rev Immunol, 1 (2001): 20-30. |
[18] | [ D. Kirschner, Dynamics of Co-infection with M. tuberculosis and HIV-1, Theor Popul Biol, 55 (1999): 94-109. |
[19] | [ H. Koppensteiner,R. Brack-Werner,M. Schindler, Macrophages and their relevance in Human Immunodeficiency Virus Type Ⅰ infection, Retrovirology, 9 (2012): p82. |
[20] | [ Q. Li,C. C. Whalen,J. M. Albert,R. Larkin,L. Zukowsy,M. D. Cave,R. F. Silver, Differences in rate and variability of intracellular growth of a panel of Mycobacterium tuberculosis clinical isolates within monocyte model, Infect Immun, 70 (2002): 6489-6493. |
[21] | [ G. Magombedze,W. Garira,E. Mwenje, Modellingthe human immune response mechanisms to mycobacterium tuberculosis infection in the lungs, Math Biosci Eng, 3 (2006): 661-682. |
[22] | [ S. Marino,D. Kirschner, The human immune response to the Mycobacterium tuberculosis in lung and lymph node, J Theor Biol, 227 (2004): 463-486. |
[23] | [ J. Murphy,R. Summer,A. A. Wilson,D. N. Kotton,A. Fine, The prolonged life-span of alveolar macrophages, Am J Respir Cell Mol Biol, 38 (2008): 380-385. |
[24] | [ G. Pedruzzi,K. V. Rao,S. Chatterjee, Mathematical model of mycobacterium-host interaction describes physiology of persistence, J Theor Biol, 376 (2015): 105-117. |
[25] | [ L. Ramakrishnan, Revisiting the role of the granuloma in tuberculosis, Nat Rev Immunol, 12 (2012): 352-366. |
[26] | [ D. Russell, Who puts the tubercle in tuberculosis?, Nat Rev Microbiol, 5 (2007): 39-47. |
[27] | [ A. Saltelli,M. Ratto,S. Tarantola,F. Campolongo, Sensitivity analysis for chemical models, Chem Rev, 105 (2005): 2811-2828. |
[28] | [ M. Sandor,J. V. Weinstock,T. A. Wynn, Granulomas in schistosome and mycobacterial infections: A model of local immune responses, Trends Immunol, 24 (2003): 44-52. |
[29] | [ R. Shi,Y. Li,S. Tang, A mathematical model with optimal constrols for cellular immunology of tuberculosis, Taiwan J Math, 18 (2014): 575-597. |
[30] | [ D. Sud,C. Bigbee,J. L. Flynn,D. E. Kirschner, Contribution of CD8+ T cells to control of Mycobacterium tuberculosis infection, J Immunol, 176 (2006): 4296-4314. |
[31] | [ D. F. Tough,J. Sprent, Life span of naive and memory T cells, Stem Cells, 13 (1995): 242-249. |
[32] | [ M. C. Tsai,S. Chakravarty,G. Zhu,J. Xu,K. Tanaka,C. Koch,J. Tufariello,J. Flynn,J. Chan, Characterization of the tuberculous granuloma in murine and human lungs: cellular composition and relative tissue oxygen tension, Cell Microbiol, 8 (2006): 218-232. |
[33] | [ S. Umekia,Y. Kusunokia, Lifespan of human memory T-cells in the absence of T-cell receptor expression, Immunol Lettt, 62 (1998): 99-104. |
[34] | [ L. Westera,J. Drylewicz, Closing the gap between T-cell life span estimates from stable isotope-labeling studies in mice and humans, BLOOD, 122 (2013): 2205-2212. |
[35] | [ J. E. Wigginton,D. E. Kischner, A model to predict cell mediated immune regulatory mechanisms during human infection with Mycobacterium tuberculosis, J Immunol, 166 (2001): 1951-1967. |
[36] | [ Word Health Organization (WHO), Global tuberculosis report 2015,2003. Available from: http://apps.who.int/iris/bitstream/10665/191102/1/9789241565059_eng.pdf. |
[37] | [ Word Health Organization (WHO), Global tuberculosis report 2016,2003. Available from: http://apps.who.int/iris/bitstream/10665/250441/1/9789241565394-eng.pdf?ua=1. |
[38] | [ M. Zhang,J. Gong,Z. Yang,B. Samten,M. D. Cave,P. F. Barnes, Enhanced capacity of a widespread strain of Mycobacterium tuberculosis to grow in human monocytes, J Infect Dis, 179 (1998): 1213-1217. |
[39] | [ M. Zhang,S. Dhandayuthapani,V. Deretic, Molecular basis for the exquisite sensitivity of Mycobacterium tuberculosis to isoniazid, Proc Natl Acad Sci U S A, 93 (1996): 13212-13216. |
1. | Xiaojie Mu, Daqing Jiang, Dynamics caused by the mean-reverting Ornstein–Uhlenbeck process in a stochastic predator–prey model with stage structure, 2024, 179, 09600779, 114445, 10.1016/j.chaos.2023.114445 | |
2. | Xin Xu, Baodan Tian, Xingzhi Chen, Yanhong Qiu, Dynamics of a stochastic food chain chemostat model with Monod–Haldane functional response and Ornstein–Uhlenbeck process, 2024, 225, 03784754, 495, 10.1016/j.matcom.2024.05.014 | |
3. | Meng Gao, Xiaohui Ai, A stochastic Gilpin-Ayala nonautonomous competition model driven by mean-reverting OU process with finite Markov chain and Lévy jumps, 2024, 32, 2688-1594, 1873, 10.3934/era.2024086 | |
4. | Lev Ryashko, Anna Otman, Irina Bashkirtseva, Multirhythmicity, Synchronization, and Noise-Induced Dynamic Diversity in a Discrete Population Model with Competition, 2025, 13, 2227-7390, 857, 10.3390/math13050857 |