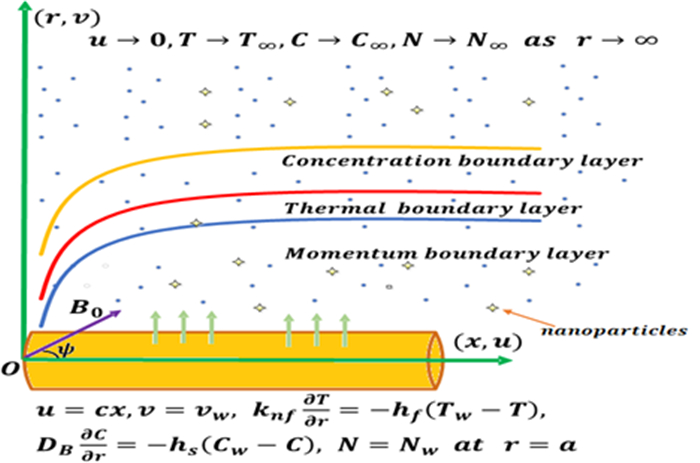
The mathematical formulation of fluid flow problems often results in coupled nonlinear partial differential equations (PDEs); hence, their solutions remain a challenging task for researchers. The present study offers a solution for the flow differential equations describing a bio-inspired flow field of non-Newtonian fluid with gyrotactic microorganisms. A methanol-based nanofluid with ferrous ferric oxide, copper, and silver nanoparticles was considered in a stretching permeable cylinder. The chemical reaction, activation energy, viscous dissipation, and convective boundary conditions were considered. The Casson fluid, a non-Newtonian fluid model, was used as flowing over a cylinder. The fundamental PDEs were established using boundary layer theory in a cylindrical coordinate system for concentration, mass, momentum, and microorganisms' field. These PDEs were then transformed into nonlinear ODEs by applying transforming variables. ODEs were then numerically solved in MATLAB software using the built-in solver bvp4c algorithm. We established an artificial neural network (ANN) model, incorporating Tan-Sig and Purelin transfer functions, to enhance the accuracy of predicting skin friction coefficient (SFC) values along the surface. The networks were trained using the Levenberg–Marquardt method. Quantitative results show that the ferrous ferric oxide nanofluid is superior in increasing Nusselt number, Sherwood number, velocity, and microorganism density number; silver nanofluid is superior in increasing skin friction coefficient, temperature, and concentration. Interestingly, heat transfer rate decreases with the magnetic and curvature parameters and Eckert number, whereas the skin friction coefficient increases with the magnetic parameter and Darcy–Forchheimer number. The present results are validated with the previous existing studies.
Citation: Khalil Ur Rehman, Nosheen Fatima, Wasfi Shatanawi, Nabeela Kousar. Mathematical solutions for coupled nonlinear equations based on bioconvection in MHD Casson nanofluid flow[J]. AIMS Mathematics, 2025, 10(1): 598-633. doi: 10.3934/math.2025027
[1] | Madeeha Tahir, Ayesha Naz, Muhammad Imran, Hasan Waqas, Ali Akgül, Hussein Shanak, Rabab Jarrar, Jihad Asad . Activation energy impact on unsteady Bio-convection nanomaterial flow over porous surface. AIMS Mathematics, 2022, 7(11): 19822-19845. doi: 10.3934/math.20221086 |
[2] | Khalil Ur Rehman, Wasfi Shatanawi, Zeeshan Asghar, Haitham M. S. Bahaidarah . Neural networking analysis for MHD mixed convection Casson flow past a multiple surfaces: A numerical solution. AIMS Mathematics, 2023, 8(7): 15805-15823. doi: 10.3934/math.2023807 |
[3] | Taqi A. M. Shatnawi, Nadeem Abbas, Wasfi Shatanawi . Comparative study of Casson hybrid nanofluid models with induced magnetic radiative flow over a vertical permeable exponentially stretching sheet. AIMS Mathematics, 2022, 7(12): 20545-20564. doi: 10.3934/math.20221126 |
[4] | Nadeem Abbas, Wasfi Shatanawi, Taqi A. M. Shatnawi . Innovation of prescribe conditions for radiative Casson micropolar hybrid nanofluid flow with inclined MHD over a stretching sheet/cylinder. AIMS Mathematics, 2025, 10(2): 3561-3580. doi: 10.3934/math.2025164 |
[5] | Muhammad Asif Zahoor Raja, Kottakkaran Sooppy Nisar, Muhammad Shoaib, Ajed Akbar, Hakeem Ullah, Saeed Islam . A predictive neuro-computing approach for micro-polar nanofluid flow along rotating disk in the presence of magnetic field and partial slip. AIMS Mathematics, 2023, 8(5): 12062-12092. doi: 10.3934/math.2023608 |
[6] | M. S. Alqarni . Thermo-bioconvection flow of Walter's B nanofluid over a Riga plate involving swimming motile microorganisms. AIMS Mathematics, 2022, 7(9): 16231-16248. doi: 10.3934/math.2022886 |
[7] | Kiran Sajjan, N. Ameer Ahammad, C. S. K. Raju, M. Karuna Prasad, Nehad Ali Shah, Thongchai Botmart . Study of nonlinear thermal convection of ternary nanofluid within Darcy-Brinkman porous structure with time dependent heat source/sink. AIMS Mathematics, 2023, 8(2): 4237-4260. doi: 10.3934/math.2023211 |
[8] | J. Kayalvizhi, A. G. Vijaya Kumar, Ndolane Sene, Ali Akgül, Mustafa Inc, Hanaa Abu-Zinadah, S. Abdel-Khalek . An exact solution of heat and mass transfer analysis on hydrodynamic magneto nanofluid over an infinite inclined plate using Caputo fractional derivative model. AIMS Mathematics, 2023, 8(2): 3542-3560. doi: 10.3934/math.2023180 |
[9] | Ali Raza, Umair Khan, Aurang Zaib, Wajaree Weera, Ahmed M. Galal . A comparative study for fractional simulations of Casson nanofluid flow with sinusoidal and slipping boundary conditions via a fractional approach. AIMS Mathematics, 2022, 7(11): 19954-19974. doi: 10.3934/math.20221092 |
[10] | Latifa I. Khayyat, Abdullah A. Abdullah . The onset of Marangoni bio-thermal convection in a layer of fluid containing gyrotactic microorganisms. AIMS Mathematics, 2021, 6(12): 13552-13565. doi: 10.3934/math.2021787 |
The mathematical formulation of fluid flow problems often results in coupled nonlinear partial differential equations (PDEs); hence, their solutions remain a challenging task for researchers. The present study offers a solution for the flow differential equations describing a bio-inspired flow field of non-Newtonian fluid with gyrotactic microorganisms. A methanol-based nanofluid with ferrous ferric oxide, copper, and silver nanoparticles was considered in a stretching permeable cylinder. The chemical reaction, activation energy, viscous dissipation, and convective boundary conditions were considered. The Casson fluid, a non-Newtonian fluid model, was used as flowing over a cylinder. The fundamental PDEs were established using boundary layer theory in a cylindrical coordinate system for concentration, mass, momentum, and microorganisms' field. These PDEs were then transformed into nonlinear ODEs by applying transforming variables. ODEs were then numerically solved in MATLAB software using the built-in solver bvp4c algorithm. We established an artificial neural network (ANN) model, incorporating Tan-Sig and Purelin transfer functions, to enhance the accuracy of predicting skin friction coefficient (SFC) values along the surface. The networks were trained using the Levenberg–Marquardt method. Quantitative results show that the ferrous ferric oxide nanofluid is superior in increasing Nusselt number, Sherwood number, velocity, and microorganism density number; silver nanofluid is superior in increasing skin friction coefficient, temperature, and concentration. Interestingly, heat transfer rate decreases with the magnetic and curvature parameters and Eckert number, whereas the skin friction coefficient increases with the magnetic parameter and Darcy–Forchheimer number. The present results are validated with the previous existing studies.
Nanofluids are generated when simple nanoparticles are dispersed in base fluids like ethylene glycol, oil, and water. These fluids have higher thermal conductivity than regular liquids. In 1995, at the Argonne National Laboratory, Choi and Eastman [1] were the first to show that solid nanoparticles dispersed in a base fluid make up a nanofluid. Nanofluids have applications in hybrid-powered engines, microelectronics, chemical catalytic reactors, particle storage facilities, pharmaceutical procedures, medicine diffusion, and electronic equipment cooling. To improve heat and mass transport properties, researchers have concentrated on nanofluids. Valipour et al. [2] numerically examined the CNT-polyethylene nanofluid around a stretching porous cylinder using the Runge–Kutta method and the impact of the Reynolds number, injection parameter, magnetic number, and volume fraction of CNT on flow style. They concluded that the CNT volume fraction and injection parameter had a direct relation with the velocity profile; on the other hand, the magnetic and Reynolds numbers had a reverse relation with the velocity profile. Mishra et al. [3] examined the heat characteristics of a silver-water nanofluid around a magnetized stretching cylinder. The investigation included the impacts of Joule heating, viscous dissipation, suction/injection, and slip boundary conditions. Two different models of thermal conductivity and dynamic viscosity were considered, corresponding to nanoparticles with different shapes: cylindrical and spherical (nanotubes). To solve the nonlinear equations, researchers employed a numerical approach, specifically the RKF45 order via the shooting algorithm, and found that when the nanofluid contains spherical nanoparticles, the fluid temperature is lower than when it contains cylindrical nanoparticles. Ramzan et al. [4] examined magnetohydrodynamic dusty Casson nanofluid flow over a deformable cylinder. They included the effects of Newtonian heating, heat sink/source, and binary chemical reactions together with activation energy to emphasize the novelty of their research. Numerically, they solved governing equations using the bvp4c technique. They found that the velocity field for the fluid flow decayed for high values of momentum dust particles, while a reverse pattern appeared for the dust phase. Rashed et al. [5] researched the nonhomogeneous Newtonian nanofluid flow over heated permeable cylinders and addressed Brownian and thermophoresis effects. The system of equations was computed numerically. Velocity, concentration, and temperature profiles of the nanofluid were boosted by increasing thermophoresis parameters. Recently, several studies were reported on nanofluid flow in cylinders [6,7,8].
The process by which fluid viscosity converts kinetic energy into internal energy is known as viscous dissipation. This process affects thermal distribution by acting like an energy source and changing heat transfer rates. Surface temperature influences viscous dissipation processes. Additionally, various factors, such as thermal radiation, Brownian diffusion, suction/injection, and viscous dissipation, may influence the enhancement of heat transfer. This characteristic has several real-world applications, such as in the production of electronic chips and plastics, food processing, electric power generation systems, cooling nuclear equipment, and groundwater contamination. Hsiao [9] analyzed the combined nanofluid and micropolar flow impacted by viscous dissipation and magnetohydrodynamic (MHD) through a stretching sheet. The finite difference technique was applied to discretize the resulting equations. The impact of important nondimensional parameters on temperature, velocity, and concentration was demonstrated. Temperature increased as the Eckect number increased, while the opposite was observed with the Prandtl number. Shahzad et al. [10] investigated the MHD Jeffery nanofluid flow over a stretching sheet with viscous dissipation aspects. They utilized copper nanoparticles dispersed with three various base fluids: polyvinyl alcohol (PVA) water, kerosene, and ethylene glycol. They found that the temperature profile improved as they increased the nanoparticle volume fraction, Eckert number, and magnetic parameter. Furthermore, when compared to the temperature profiles of Cu-kerosene nanofluids, the temperature distribution in the Cu-PVA-based Jeffrey nanofluid was higher. Zokri et al. [11] investigated the effect of viscous dissipation on Jeffrey flow over a horizontal circular cylinder, using the Keller-box approach to resolve numerically the nondimensional variables. The researchers discussed two specific cases: one where the cylinder was heated and another where it was cooled. They discovered that by increasing the Eckert number, there was a small increase in the skin friction coefficient (drag indicator) and a decrease in the Nusselt number (heat transfer indicator). Recent investigations on magnetic fields and viscous dissipation of nanofluid flow across surfaces may be found in [12,13,14,15].
In 1889, the scientist Svante Arrhenius defined the term activation energy as the smallest energy to start a chemical reaction. The definition of activation energy is derived from the Arrhenius equation, which describes how rate constants change with temperature. Mass equations that combine activation energy with binary chemical reactions have applications in geothermal and chemical engineering, food processing, oil reservoirs, and water and oil suspensions, among other fields. Bestman [16] first proposed this concept, and Azam et al. [17] modeled transient bioconvection Cross nanofluid flow over a contracting/expanding cylinder. The highly nonlinear system of ODEs was solved using the shooting Fehlberg approach to obtain numerical solutions for both when the cylinder was stretching and when it was shrinking. Accordingly, nanoparticle concentration increases with increasing values of the activation energy parameter for a stretching and contracting cylinder, but reverse outcomes are obtained for the chemical reaction parameter. For recent research on activation energy impact on various nanofluids under numerous conditions, see [18,19,20,21,22].
Bioconvection is a naturally occurring phenomenon caused by the erratic movement of microorganisms, either as single cells or in colony-like arrangements. For example, gyrotactic bacteria may move in the reverse direction of gravity when there is no movement. In the context of bioconvection, the migration of microorganisms is influenced by the asymmetric mass distribution balance. Bioconvection processes have various applications in many medical and industry sectors; they have been included in a variety of micro-biosystems encompassing enzyme biosensors, ethanol, biotechnology, hydrogen gas manufacturing, fertilizer synthesis, biofuel generation, and biodiesel processing, as well as the use of subpopulations, purified cultures, and swimming classes. The first theoretical bioconvection model including a wide range of mobile microorganisms was developed by Plesset and Winet [23]. Abdelmalek et al. [24] evaluated the Williamson bioconvection nanofluid flow toward a stretched cylinder with second-order slip and variable thermal conductivity. The numerical solution was computed with the shooting scheme. Researchers noticed that the activation energy, thermophoresis, and slip parameters had a significant influence on the variation of nanoparticle concentration. Furthermore, they observed an increased gyrotactic microorganism distribution for the Weissenberg number. Imran et al. [25] studied bioconvection in Cross nanofluid flow over a cylinder with motile microbes and convective conditions. The system of ODEs was numerically solved using the bvp4c technique. They determined that higher Peclet and Lewis values reduce the microorganism profile. Nabwey et al. [26] examined a magneto-Carreau nanofluid over an inclined cylinder with gyrotactic microorganism effects. They concluded that the concentration of nanoparticles in the nanofluid improves as the activation energy limitation increases. Recently, some scholars performed numerical analyses of bioconvection swimming microorganisms [27,28,29].
It is believed that the thermal conductivity of fluids can be enhanced using nanoparticles. This is essential for applications like energy systems, industrial processes, and cooling systems that need effective heat transfer. Copper and silver show high thermal conductivity and are well-suited for increasing heat transfer rates. Further, the fluid can be controlled by an external magnetic field with the assistance of the magnetic properties of ferrous ferric oxide. These properties, combined in a Casson fluid, may aid in drug delivery systems, magnetic cooling, and microfluidic devices. This will optimize the fluid behavior in specific industrial, medical, and environmental settings. Therefore, the novelty of this paper is to perform a comparative analysis of (Fe3O4/Cu/Ag−CH3OH) nanofluids regarding concentration, thermal, momentum, and motile microorganism density along a stretching cylinder. The dimensionless equations are computed using the bvp4c approach in MATLAB software, and an investigation is carried out on the impact of representative factors. Furthermore, three ANN models are built to predict SFC at the cylinder surface. The results of this study have numerous applications on bioconvection in magneto-Casson nanofluids and could improve current technologies in several domains, particularly those pertaining to fluid flow, heat transfer, and biological processes. According to this study, a ferrous ferric oxide nanofluid raises the Nusselt number, which is an indicator of heat transfer efficiency. Cooling systems can use this to improve heat dissipation and boost system performance.
The two-dimensional, steady, and incompressible laminar boundary layer flow for a non-Newtonian Casson nanofluid flowing through a permeable linearly stretching cylinder is considered here. Three different types of nanoparticles are considered: ferrous ferric oxide (Fe3O4), copper (Cu), and silver (Ag), together with the base fluid methanol (CH3OH). The cylindrical polar (x,r) coordinate system is considered, where x indicates the axial direction and r specifies the radial direction. At the boundary of a linearly stretched cylinder velocity is Uw(x)=cx. The constant strength B0 of the magnetic field is inserted in a radial direction with inclination ψ. Moreover, motile gyrotactic microorganisms are included in the flow, and their movement is caused by bioconvective phenomena. Convective-type boundary conditions are imposed at the cylinder surface, also known as Newton boundary conditions. The fluid motion is initiated in the axial direction of the stretching cylinder. The geometry and coordinate system of the model is labeled as in Figure 1.
The following equation represents the rheological relationship between the Cauchy stress tensor and an isotropic, incompressible flow of a Casson fluid [30]:
˜τ=˜τ0+μ˜α | (1) |
˜τij={2(μB+pr√2π)eij,π>πc,2(μB+pr√2π)eij,π<πc,} | (2) |
where ˜τ is shear stress, ˜α is shear rate, pr is a yield stress of fluid, ˜τ0 is a Casson yield stress, and μB is a plastic dynamic viscosity.
The governing mass, momentum, heat, and motile microorganism equations within the boundary layer are described as follows [31,32]:
∂(ru)∂x+∂(rv)∂r=0 | (3) |
u∂u∂x+v∂u∂r=νnf(1+1β)(∂2u∂r2+1r∂u∂r)−νnf1K∗u−1ρnfCb√K∗u2−σnfρnfB02Sin2ψu, | (4) |
u∂T∂x+v∂T∂r=knf(ρcp)nf(∂2T∂r2+1r∂T∂r)+νnf(cp)nf(1+1β)(∂u∂r)2+σnf(ρcp)nfBo2Sin2ψu2, | (5) |
u∂C∂x+v∂C∂r=DB(∂2C∂r2+1r∂C∂r)−Kr2(TT∞)noexp(−E∗k∗T)(C−C∞), | (6) |
u∂N∂x+v∂N∂r=Dn(∂2N∂r2+1r∂N∂r)−bWc(Cw−C∞)(∂C∂r∂N∂r+N∂2C∂r2). | (7) |
The following boundary conditions are suitable:
u=Uw(x)=cx,v=vw,knf∂T∂r=−hf(Tw−T),DB∂C∂r=−hs(Cw−C),N=Nwatr=a,u→0,T→T∞,C→C∞,N→N∞asr→∞. | (8) |
In Eq (6), the term Kr2(T/T∞)noexp(−E∗/k∗T) indicates a modified Arrhenius equation in which n0 is the fitted rate constant, taking values −1<n0<1, and k∗=8.61×10−5eV/K is the Boltzman constant.
The process to convert the PDEs into ODEs involves the utilization of a similarity transformation [31] in the following manner:
η=r2−a22a√cνf,u=cxf′(η),v=−ar√cνff(η),θ(η)=T−T∞Tw−T∞,ϕ(η)=C−C∞Cw−C∞,g(η)=N−N∞Nw−N∞ | (9) |
here, prime signifies differentiation with respect to η. The continuity equation in Eq (3) is fulfilled automatically, while Eqs (4)–(8) are simplified by using the similarity transformation given in Eq (9) as follows:
(1+1β)((1+2ηγ)f‴+2γf″)+C2C1(ff″−f′2)−(P0+C3C1M2Sin2ψ)f′−1C1Dff′2=0, | (10) |
(1+2ηγ)θ″+2γθ′+Pr(C5C4fθ′+C1C4(1+1β)(1+2ηγ)Ecf″2+C3C4M2EcSin2ψf′2)=0, | (11) |
(1+2ηγ)ϕ″+2γϕ′+Sc(fϕ′−ˆσ(1+δθ)noexp(−Ea(1+δθ))ϕ)=0, | (12) |
(1+2ηγ)g″+2γg′+Sbfg′−Pe((1+2ηγ)g′ϕ′+(g+Ω)(γϕ′+(1+2ηγ))ϕ″)=0, | (13) |
And the transform boundary conditions are expressed as:
f(η)=−α∗,f′(η)=1,θ′(η)=−1C4Bi(1−θ(η)),ϕ′(η)=−Bj(1−ϕ(η)),g(0)=1atη=0,f′(η)→0,θ(η)→0,ϕ(η)→0,g(η)→0asη→∞. | (14) |
Where
C1=μnfμf,C2=ρnfρf,C3=σnfσf,C4=knfkf,C5=(ρcp)nf(ρcp)f. | (15) |
μnf, σnf, ρnf, knf, and (ρcp)nf are the dynamic viscosity, electrical conductivity, density, thermal conductivity, and electrical conductivity, respectively. The thermophysical properties are presented in Table 1.
Properties | CH3OH | Fe3O4 | Cu | Ag |
ρ(kg/m3) | 792 | 5180 | 8933 | 10,500 |
σ(Ω−1m−1) | 0.5×10-6 | 0.74×106 | 5.96×107 | 6.3×107 |
k(W/mK) | 0.2035 | 9.7 | 400 | 429 |
cp(J/kgK) | 2545 | 670 | 385 | 235 |
Pr | 7.38 |
Numerous thermophysical correlations for nanofluids are given in Table 2, used to calculate the ρnf, μnf, (ρcp)nf, knf, and σnf for nanofluids. The solid volume fraction of nanofluids is represented by ϕ1. The dimensionless quantities involved in nondimensional equations and boundary conditions are displayed in Table 3.
Properties | Nanofluids |
Dynamic viscosity | μnfμf=1(1−ϕ1)2.5 |
Density | ρnfρf=(1−ϕ1)+ϕ1ρnfρf |
Heat capacitance | (ρcp)nf(ρcp)f=(1−ϕ1)+ϕ1(ρcp)nf(ρcp)f |
Thermal conductivity | knfkf=1−ϕ1+2ϕ1knfknf−kfln(knf+kf2kf)1−ϕ1+2ϕ1kfknf−kfln(knf+kf2kf) |
Electrical conductivity | σnfσf=1+3ϕ1(σnfσf−1)(σnfσf+2)−ϕ1(σnfσf−1) |
Parameters | Formula | Parameters | Formula |
Curvature parameter | γ=√νfca2 | Magnetic field parameter | M2=Bo2σfcρf |
Permeability parameter | P0=νfcK∗ | Darcy–Forchheimer number | Df=Cbxρf√K∗ |
Eckert number | Ec=Uw2(cp)f(Tw−T∞) | Prandtl number | Pr=μf(cp)fkf |
Bioconvection Peclet number | Pe=bWcDn | Bioconvection Schmidt number | Sb=νfDn |
Microorganism parameter | Ω=N∞Nw−N∞ | Schmidt number | Sc=νfDB |
Activation energy parameter | Ea=E∗k∗T∞ | Chemical reaction parameter | ˆσ=Kr2c |
Temperature difference parameter | δ=Tw−T∞T∞ | Suction parameter | α∗=vw√cνf |
Thermal Biot number | Bi=hfkf√νfc | Diffusion Biot number | Bj=hsDB√νfc |
For engineering, the skin friction coefficient Cfx, density number Dnx, Nusselt number Nux, and Sherwood number Shx are crucial physical variables and can be measured by the surface shear stress, heat/mass transfer effects, and microorganism diffusion of the system, respectively. These are written as follows:
Cfx=2τwρfUw2,Nux=xqwkf(Tw−T∞),Shx=xjwDB(Cw−C∞),Dnx=xiwDn(Nw−N∞), | (16) |
where τw, qw, iw, and jw are expressed as follows:
τw=μnf(1+1β)(∂u∂r)r=a,qw=−knf(∂T∂r)r=a,jw=−DB(∂C∂r)r=a,iw=−Dn(∂N∂r)r=a, | (17) |
using Eq (9), the dimensionless form of these quantities is written in Eq (17).
12Cfx√Rex=C1(1+1β)f″(0),Nux√Rex=−C4θ′(0),Shx√Rex=−ϕ′(0),Dnx√Rex=−g′(0), | (18) |
here, Rex=Uwxνf denotes the local Reynolds number.
Several techniques exist for solving equations in fluid flow problems [34,35,36,37,38,39,40]; here, the shooting method was selected because it is easy to use and effective at turning boundary-value problems into initial-value problems. For nonlinear differential equations that are frequently encountered in fluid mechanics, this method guarantees greater accuracy and quicker convergence. Eqs (10)−(14) are difficult to solve analytically since they are highly nonlinear ODEs. Because of this, we move toward numerical solutions using the bvp4c function in MATLAB software. The three-stage Lobatto IIIa finite difference technique is executed by Bvp4c with accuracy up to the fourth order. First, highly nonlinear ODEs are transformed into a system of first-order differential equations with the support of appropriate variables, as given in Eq (19).
y1=f,y2=f′,y3=f″,y4=θ,y5=θ′,y6=ϕ,y7=ϕ′,y8=g,y9=g′ | (19) |
by inserting Eq (19) into Eqs (10)−(14), we get
y1′=y2,y2′=y3,y3′=−1(1+1β)(1+2ηγ)((1+1β)2γy3+C2C1(y1y3−y22)−(P0+C3C1M2Sin2ψ)y2−1C1Dfy22),y4′=y5,y5′=−1(1+2ηγ)(2γy5+Pr(C5C4y1y5+C1C4(1+1β)(1+2ηγ)Ecy32+C3C4M2EcSin2ψy22)),y6′=y7,y7′=−1(1+2ηγ)(2γy7+Sc(y1y7−ˆσ(1+δy4)noexp(−Ea(1+δy4))y6)),y8′=y9,y9′=−1(1+2ηγ)(2γy9+Sby1y9−Pe((1+2ηγ)y7y9+(y8+Ω)(γy7+(1+2ηγ))y7′)), | (20) |
and
η=0:y1(0)=−α∗,y2(0)=1,y5(0)=−1C4Bi(1−y4),y7(0)=−Bj(1−y6),y8(0)=1, η→∞:y2(∞)→0,y4(∞)→0,y6(∞)→0,y8(∞)→0. | (21) |
It is important to note that the mesh size Δη=0.01 and the step size h=0.01 are frequently used interchangeably in the shooting method depending on how frequently the solution is calculated throughout the domain. All numerical outcomes are obtained with an 10−6 error tolerance in this problem.
The numerical results are compared under certain conditions with results from the literature with excellent agreement (Table 4). Outcomes agree with those of Khan and Pop [41], Ali et al. [42], and Ahmad et al. [43]. Excellent associations in the findings ensure the correctness of our numerical code and solutions.
Pr | Khan and Pop [41] | Ali et al. [42] | Ahmad et al. [43] | Present |
2 | 0.9113 | 0.9105 | 0.9105 | 0.9114 |
7 | 1.8954 | 1.8908 | 1.8908 | 1.8954 |
20 | 3.3539 | 3.3527 | 3.3527 | 3.3539 |
70 | 6.4621 | 6.4781 | 6.4781 | 6.4622 |
In this study, three distinct forms of nanofluids (Fe3O4−CH3OH, Cu−CH3OH, Ag−CH3OH) have been considered for 2D steady laminar Casson fluid flow above a stretching cylinder with inclined MHD and motile microorganisms. The impact of physical parameters on the dimensionless velocity f′(η), concentration ϕ(η), temperature θ(η), and motile microorganisms g(η) distributions is displayed in Figures 2–19, respectively for the magnetic parameter M, the Casson fluid parameter β, the permeability parameter P0, the magnetic field inclination ψ, the Darcy–Forchheimer number Df, the Eckert number Ec, the parameter of chemical reaction ˆσ, the activation energy parameter Ea, the bioconvective Peclet number Pe, the bioconvective Schmidt number Sb, the microorganism parameter Ω, the thermal Biot number Bi, the diffusion Biot number Bj, the suction parameter α∗, and the Schmidt number Sc. The fixed default values for fluid flow used in the simulation are as follows: γ=0.25,ϕ1=0.05,ψ=π/4,Df=P0=0.5,Pe=Ω=0.2,Ec=δ=n0=Ea=0.5,Sc=ˆσ=1,Bi=0.3, and Bj=0.3. The range of the flow parameters is carried within a convergence band of the adopted numerical scheme.
Figure 2 shows the increasing impact of the Casson fluid parameter β on f′(η). The fluids yield stress and the Casson fluid parameter are inversely correlated. A lower yield stress, which indicates that less force is needed to start the flow, is correlated with higher Casson parameter values. The fluid can move more easily as a result of the decreased flow resistance, which raises velocity.
Figure 3 presents the decreasing function between the magnetic parameter M and f′(η). The large values of the magnetic field parameter result in a decrease in fluid velocity because of the Lorentz force acting on the Casson fluid. An electrically conducting fluid experiences resistance to motion when a magnetic field is applied to it. This resistance is known as the Lorentz force.
Figure 4 reflects the impact of the magnetic field inclination ψ on dimensionless f′(η). The strength of applied magnetic field increases by increasing ψ. Therefore, f′(η) declines for rising values of ψ; when the angle increases, the magnetic field force improves, and thus velocity drops.
The impact of the permeability parameter P0 on f′(η) is exhibited in Figure 5. An inverse relation for velocity toward P0 is found. The resistance offered by the porous medium is responsible for the decrease in fluid velocity that occurs when the permeability parameter increases. Higher values of the permeability parameter signify a more restrictive porous medium, being inversely correlated with the resistance of the porous structure.
Figure 6 portrays the decreasing behavior of f′(η) for rising estimates of Darcy–Forchheimer Df. Physically inertial forces take over and produce a stronger opposing drag that prevents the fluid motion as the Darcy–Forchheimer parameter rises. This resistance leads to a noticeable velocity decrease in addition to lowering the momentum of flow. The larger Forchheimer parameter thus introduces stronger inertial drag forces, which are directly responsible for the decrease in fluid velocity.
Increasing values of the suction parameter α∗ reduce f′(η) (Figure 7). Physically, suction controls the flow of nanofluid, reducing drag on the nanoparticles in an external flow, causing a reduction in velocity. The improved removal of fluid particles from the boundary layer by suction causes a decrease in fluid velocity as the suction parameter increases. By physically drawing fluid away from the flow close to the surface suction, the total momentum in the boundary layer is lowered.
The nanofluid (Fe3O4−CH3OH) shows higher velocity than the other two nanofluids (Cu−CH3OH, Ag−CH3OH) in response to parameters M, ψ, P0, Df, and α∗, while the opposite is observed for β. The effect of several parameters on the temperature profile θ(η) for the three nanofluids is shown in Figures 8–12.
The impact of the Casson fluid parameter β on the temperature profile θ(η) is clearly shown in Figure 8. The temperature profile decreases with increasing β. Larger values of the Casson fluid parameter result in a drop in temperature because 𝛽 is inversely proportional to the fluid yield stress. When fluid resistance to deformation decreases, there is a smoother flow with less energy dissipation in response to an increase in 𝛽.
Figure 9 shows the impact of the magnetic parameter M on the temperature profile θ(η). The fluid generates more Joule heating when the magnetic field parameter increases; hence, the temperature rises. A magnetic field interaction with an electrically conducting fluid results in an induced Lorentz force that prevents the fluid from moving; this produces heat through electrical resistance.
Figure 10 illustrates the influence of a magnetic field inclination ψ on the temperature profile θ(η). Higher ψ increases θ(η).
The influence of the Eckert number Ec on temperature distribution θ(η) is displayed in Figure 11. The work done against a viscous fluid is often expressed as the Eckert number, a dissipation parameter. As Ec increases, the fluid temperature also increases. This phenomenon involves converting kinetic energy into internal energy through the work done against the forces within viscous fluids. Here, Ec depends upon the kinetic energy; as the kinetic energy increases, so will the Ec.
Figure 12 shows the interaction between the temperature of the nanofluids and the thermal Biot number Bi. The fluids' temperature close to the surface increases as Bi increases because of the improved efficiency of the heat exchange between the fluid and surface. This results in a thicker thermal boundary layer and a higher temperature profile across the convective heat transfer–affected region.
From Figures 8−12, it is clear that the temperature is higher for the nanofluid (Ag−CH3OH) than the other two nanofluids (Cu−CH3OH, Fe3O4−CH3OH).
The response of concentration distribution to several manipulating parameters is given in Figures 13–16. Figure 13 shows the influence of the Schmidt number Sc on the concentration profile ϕ(η) of nanofluids. The Schmidt number is the ratio of momentum to mass diffusivity; it ϕ(η) decreases as a function of Sc since higher Sc values correspond to lower mass diffusion and, as a consequence, a reduced concentration of the boundary layer.
The influence of the chemical reaction parameter ˆσ on the concentration gradient ϕ(η) is demonstrated in Figure 14. ϕ(η) decreases with increasing ˆσ; it consumes the chemical species in the fluid, and the chemical reaction lowers the concentration. Species depletion becomes more pronounced with the increase in the reaction rate, which lowers the concentration of fluid.
Figure 15 shows the variation of the concentration profile ϕ(η) in response to the activation energy parameter Ea. Increasing values of Ea lead to increased ϕ(η). Physically, the concentration profile will show an upward trend if a significant amount of energy is necessary to start the chemical reaction.
Figure 16 illustrates the influence of the Biot number Bj (sway of diffusion) on the concentration profile ϕ(η). ϕ(η) increases with increasing values of Bj. According to the definition of the Biot number, the transmitted mass will be dispersed throughout the surface by convection and thus, concentration distribution increases.
There is a higher influence of the relevant parameters on the mass distribution of the nanofluid Ag−CH3OH than of the other two nanofluids Cu/Fe3O4−CH3OH.
The variation in density of motile microorganisms g(η) in response to several parameters and nanofluids is shown in Figures 17–19. Figure 17 shows the effect of the bioconvective Schmidt number Sb on the density profile of motile microorganisms g(η). For higher values of Sb, the impact of this parameter on the rescaled g(η) is found to be insignificant. The variation of bioconvection Schmidt numbers tend to lower the boundary layer thickness. Figure 18 portrays the impact of bioconvective Peclet number Pe on the density distribution of motile microorganisms g(η). The Peclet number is a key parameter to recognize microorganisms that are swimming in a fluid. This number represents the ratio of the product of the maximum cell swimming speed and the chemotaxis constant to the diffusion coefficient of the microbes. Diffusion is the process by which the material travels from an area of high concentration to an area of low concentration, describing the movement of substances in the fluid. It has been discovered that an increase in Pe leads to an increase in the speed of fluid particles and a decrease in the diffusivity of microbes, thus reducing g(η).
Figure 19 shows the effect of the microorganism parameter Ω on the density profile g(η). Increasing Ω results in a decrease in the concentration of microorganisms in ambient fluid. The Ag nanoparticle shows a higher motile microorganisms profile than the Cu and Fe3O4 nanofluids.
The dimensionless skin friction coefficient 0.5Cfx√Rex, Nusselt number Nux/√Rex, density number Dnx/√Rex, and Sherwood number Shx/√Rex versus several parameters are calculated and presented in Tables 5–8. Table 5 highlights the impact of γ, β, M, P0, ψ, Df, and α∗ on the coefficient of skin friction. The coefficient increases by increased γ, M, ψ, P0, and Df; an opposite trend is observed for β and α∗. Moreover, the Ag−CH3OH nanofluid has higher 0.5Cfx√Rex than Cu−CH3OH and Fe3O4−CH3OH nanofluids. Table 6 depicts the influence of various physical parameters on the Nusselt number. The heat transfer rate (Nusselt number) decreases with increasing γ, ψ, M, and Ec; however, it increases with increasing β and Bi. This is a significant finding, as a higher Nusselt number would ultimately indicate a larger heat transfer from the surface to its surroundings, which aids to cool the system. Finally, the heat transfer rate for the Fe3O4−CH3OH nanofluid is higher than for the Cu−CH3OH and Ag−CH3OH nanofluids.
γ | β | M | ψ | P0 | Df | α∗ | 0.5Cfx√Rex Fe3O4−CH3OH Cu−CH3OH Ag−CH3OH |
||
0.1 | 5.0 | 0.5 | π/4 | 0.5 | 0.5 | -1.0 | 3.3536 | 3.6914 | 3.8326 |
0.2 | - | - | - | - | - | - | 3.4812 | 3.8187 | 3.9597 |
0.3 | - | - | - | - | - | - | 3.6085 | 3.9458 | 4.0867 |
0.1 | 5.0 | - | - | - | - | - | 3.3536 | 3.6914 | 3.8326 |
- | 6.0 | - | - | - | - | - | 3.1850 | 3.5045 | 3.6381 |
- | 7.0 | - | - | - | - | - | 3.0673 | 3.3742 | 3.5025 |
- | 5.0 | 0.1 | - | - | - | - | 3.2921 | 3.6341 | 3.7770 |
- | - | 0.3 | - | - | - | - | 3.3128 | 3.6534 | 3.7957 |
- | - | 0.6 | - | - | - | - | 3.3812 | 3.7171 | 3.8576 |
- | - | 0.5 | π/6 | - | - | - | 3.3218 | 3.6617 | 3.8038 |
- | - | - | π/3 | - | - | - | 3.3849 | 3.7206 | 3.8610 |
- | - | - | π/2 | - | - | - | 3.4158 | 3.7495 | 3.8891 |
- | - | - | π/4 | 0.8 | - | - | 3.4975 | 3.8260 | 3.9637 |
- | - | - | - | 1.8 | - | - | 3.9228 | 4.2294 | 4.3585 |
- | - | - | - | 2.8 | - | - | 4.2910 | 4.5831 | 4.7062 |
- | - | - | - | 0.5 | 0.6 | - | 3.3779 | 3.7138 | 3.8543 |
- | - | - | - | - | 0.8 | - | 3.4259 | 3.7581 | 3.8973 |
- | - | - | - | - | 1.0 | - | 3.4732 | 3.8019 | 3.9397 |
- | - | - | - | - | 0.5 | -0.9 | 3.2274 | 3.5372 | 3.6665 |
- | - | - | - | - | - | -0.7 | 2.9848 | 3.2413 | 3.3479 |
- | - | - | - | - | - | -0.5 | 2.7560 | 2.9634 | 3.0491 |
γ | β | M | ψ | Ec | Bi | Nux/√Rex Fe3O4−CH3OH Cu−CH3OH Ag−CH3OH |
||
0.1 | 1.0 | 0.5 | π/4 | 0.5 | 0.3 | 0.2160 | 0.2084 | 0.2028 |
0.2 | - | - | - | - | - | 0.2056 | 0.1980 | 0.1920 |
0.3 | - | - | - | - | - | 0.1950 | 0.1872 | 0.1810 |
0.1 | 1.2 | - | - | - | - | 0.2279 | 0.2217 | 0.2169 |
- | 1.4 | - | - | - | - | 0.2357 | 0.2303 | 0.2262 |
- | 1.6 | - | - | - | - | 0.2412 | 0.2364 | 0.2326 |
- | 1.0 | 0.1 | - | - | - | 0.2174 | 0.2097 | 0.2040 |
- | - | 0.3 | - | - | - | 0.2169 | 0.2093 | 0.2036 |
- | - | 0.6 | - | - | - | 0.2153 | 0.2078 | 0.2022 |
- | - | 0.5 | π/6 | - | - | 0.2167 | 0.2091 | 0.2034 |
- | - | - | π/3 | - | - | 0.2152 | 0.2078 | 0.2021 |
- | - | - | π/2 | - | - | 0.2145 | 0.2071 | 0.2015 |
- | - | - | π/4 | 0.1 | - | 0.2748 | 0.2734 | 0.2721 |
- | - | - | - | 0.2 | - | 0.2601 | 0.2571 | 0.2547 |
- | - | - | - | 0.3 | - | 0.2454 | 0.2409 | 0.2374 |
- | - | - | - | 0.5 | 0.2 | 0.1457 | 0.1406 | 0.1368 |
- | - | - | - | - | 0.4 | 0.2846 | 0.2747 | 0.2672 |
- | - | - | - | - | 0.6 | 0.4173 | 0.4029 | 0.3917 |
γ | β | M | Sc | ˆσ | Ea | Bj | Shx/√Rex Fe3O4−CH3OH Cu−CH3OH Ag−CH3OH |
||
0.1 | 1.0 | 0.5 | 1.0 | 1.0 | 0.5 | 0.3 | 0.2530 | 0.2529 | 0.2529 |
0.2 | - | - | - | - | - | - | 0.2537 | 0.2536 | 0.2536 |
0.3 | - | - | - | - | - | - | 0.2544 | 0.2544 | 0.2543 |
0.1 | 1.2 | - | - | - | - | - | 0.2532 | 0.2530 | 0.2530 |
- | 1.4 | - | - | - | - | - | 0.2532 | 0.2531 | 0.2531 |
- | 1.6 | - | - | - | - | - | 0.2533 | 0.2532 | 0.2531 |
- | 1.0 | 0.1 | - | - | - | - | 0.2531 | 0.2530 | 0.2529 |
- | - | 0.3 | - | - | - | - | 0.2531 | 0.2530 | 0.2529 |
- | - | 0.6 | - | - | - | - | 0.2530 | 0.2529 | 0.2529 |
- | - | 0.5 | 0.3 | - | - | - | 0.2096 | 0.2094 | 0.2094 |
- | - | - | 0.6 | - | - | - | 0.2362 | 0.2361 | 0.2360 |
- | - | - | 0.9 | - | - | - | 0.2498 | 0.2497 | 0.2497 |
- | - | - | 1.0 | 1.5 | - | - | 0.2565 | 0.2565 | 0.2565 |
- | - | - | - | 2.0 | - | - | 0.2592 | 0.2592 | 0.2592 |
- | - | - | - | 2.5 | - | - | 0.2613 | 0.2614 | 0.2614 |
- | - | - | - | 1.0 | 0.6 | - | 0.2523 | 0.2521 | 0.2521 |
- | - | - | - | - | 0.7 | - | 0.2515 | 0.2514 | 0.2513 |
- | - | - | - | - | 0.8 | - | 0.2508 | 0.2506 | 0.2506 |
- | - | - | - | - | 0.5 | 0.2 | 0.1780 | 0.1779 | 0.1779 |
- | - | - | - | - | - | 0.4 | 0.3207 | 0.3205 | 0.3205 |
- | - | - | - | - | - | 0.6 | 0.4376 | 0.4373 | 0.4372 |
γ | β | M | Sb | Pe | Ω | Dnx/√Rex Fe3O4−CH3OH Cu−CH3OH Ag−CH3OH |
||
0.1 | 1.0 | 0.5 | 0.1 | 0.2 | 0.2 | 0.2957 | 0.2943 | 0.2938 |
0.2 | - | - | - | - | - | 0.3591 | 0.3580 | 0.3576 |
0.3 | - | - | - | - | - | 0.4171 | 0.4162 | 0.4158 |
0.1 | 1.2 | - | - | - | - | 0.2970 | 0.2955 | 0.2949 |
- | 1.4 | - | - | - | - | 0.2980 | 0.2964 | 0.2958 |
- | 1.6 | - | - | - | - | 0.2989 | 0.2972 | 0.2966 |
- | 1.0 | 0.1 | - | - | - | 0.2959 | 0.2945 | 0.2939 |
- | - | 0.3 | - | - | - | 0.2959 | 0.2944 | 0.2939 |
- | - | 0.6 | - | - | - | 0.2956 | 0.2942 | 0.2937 |
- | - | 0.5 | 0.5 | - | - | 0.6931 | 0.6843 | 0.6810 |
- | - | - | 1.5 | - | - | 1.8345 | 1.8169 | 1.8102 |
- | - | - | 2.5 | - | - | 2.9361 | 2.9162 | 2.9084 |
- | - | - | 0.1 | 0.1 | - | 0.2647 | 0.2632 | 0.2627 |
- | - | - | - | 0.3 | - | 0.3268 | 0.3253 | 0.3248 |
- | - | - | - | 0.5 | - | 0.3888 | 0.3874 | 0.3869 |
- | - | - | - | 0.2 | 0.4 | 0.3061 | 0.3047 | 0.3042 |
- | - | - | - | - | 0.8 | 0.3269 | 0.3254 | 0.3249 |
- | - | - | - | - | 1.2 | 0.3476 | 0.3462 | 0.3457 |
Table 7 represents the impact of involved parameters on mass transfer rate (Sherwood number). A direct relation is observed with γ,β,Sc,ˆσ and Bj, while a reverse relation is found for M and Ea. Moreover, the mass transfer rate is smaller for Cu−CH3OH and Ag−CH3OH than Fe3O4−CH3OH. The impact of important parameters on the mass rate of microorganisms (density number) is presented in Table 8. Density increases with increased γ,β,Sb,Pe, and Ω but decreases with increased M. Moreover, the mass rate of microorganisms for the Fe3O4−CH3OH nanofluid is higher than for Cu−CH3OH and Ag−CH3OH nanofluids.
The solution of nonlinear partial differential equations (PDEs), which are computationally demanding, is frequently required for fluid flow problems. Frequently, the flow field is challenging to describe for a wide range of parameters because of singularities appearing in the solution scheme. Therefore, ANN can help us to predict the quantity of interest by learning and approximating these nonlinear systems. The mathematical modeling of Casson fluid flow over a horizontally stretched cylinder is studied here, and the resulting flow equations are solved using numerical methods. The skin friction coefficient (SFC) is computed using three distinct nanoparticles: Fe3O4−CH3OH, Cu−CH3OH, and Ag−CH3OH. In each scenario, ANN models are established to forecast the SFC values on the cylinder.
Researchers believe that the combination of the artificial neural networking models [44,45,46] and the multilayer perceptron (MLP) has outstanding learning capabilities, making it a popular choice for predicting various physical circumstances. The MLP network is composed of three separate layers. The first layer is responsible for accepting inputs; the hidden layer is centrally involved, and the last layer, known as the output layer, stores predicted data. Figure 20 illustrates the structural arrangement of this ANN model. Three separate ANN models were created and designated as ANN Model-Ⅰ, ANN Model-Ⅱ, and ANN Model-Ⅲ to forecast the SFC for the three nanofluids Fe3O4−CH3OH, Cu−CH3OH, and Ag−CH3OH, respectively. These ANN models utilize seven distinct flow parameters as inputs: Curvature parameter, Casson, suction, magnetic field inclination, permeability parameter, and Darcy–Forchheimer number.
Table 9 contains the symbolic details corresponding to the three different models. The desired output for all these models is the SFC. To train these models effectively, we have assembled a dataset comprising 70 different samples, each associated with its corresponding SFC value. The dataset is divided into 70%, 15%, and 15% for training, validation, and testing purposes, respectively. Complete information regarding this matter is demonstrated in Table 10.
Models | Nanoparticles | Input | Output | ||||||
Model-Ⅰ | Fe3O4−CH3OH | γ | β | M | ψ | P0 | Df | α∗ | SFC |
Model-Ⅱ | Cu−CH3OH | γ | β | M | ψ | P0 | Df | α∗ | SFC |
Model-Ⅲ | Ag−CH3OH | γ | β | M | ψ | P0 | Df | α∗ | SFC |
Samples | Model-Ⅰ | Model-Ⅱ | Model-Ⅲ |
Training data | 48 | 48 | 48 |
Validation data | 11 | 11 | 11 |
Testing data | 11 | 11 | 11 |
Total | 70 | 70 | 70 |
The impact of seven flow parameters on SFC for all three nanofluids is presented in Tables 11–17. The architecture of these models incorporates a hidden layer housing ten neurons. The training network is facilitated by adopting the Levenberg–Marquardt (LM) method. Compared to traditional gradient descent algorithms, the LM algorithm converges more rapidly especially when dealing with small-to-medium-sized datasets. In fluid dynamics where precise profile and coefficient predictions are essential, LM is particularly beneficial. The Purelin and Tan-Sig transfer functions used in the hidden and output layers are mathematically defined as:
FT(x)=11+e−x, | (22) |
Pureline(x)=x. | (23) |
γ | SFC (0.5Cfx√Rex) Fe3O4−CH3OH Cu−CH3OH Ag−CH3OH |
||
0.1 | -3.3536 | -3.6914 | -3.8326 |
0.2 | -3.4812 | -3.8187 | -3.9597 |
0.3 | -3.6085 | -3.9458 | -4.0867 |
0.4 | -3.7355 | -4.0726 | -4.2135 |
0.5 | -3.8622 | -4.1993 | -4.3401 |
0.6 | -3.9886 | -4.3257 | -4.4665 |
0.7 | -4.1148 | -4.4519 | -4.5927 |
0.8 | -4.2407 | -4.5779 | -4.7187 |
0.9 | -4.3664 | -4.7037 | -4.8445 |
1 | -4.4920 | -4.8294 | -4.9702 |
β | SFC (0.5Cfx√Rex) Fe3O4−CH3OH Cu−CH3OH Ag−CH3OH |
||
5.1 | -3.3335 | -3.6691 | -3.8094 |
5.2 | -3.3143 | -3.6478 | -3.7872 |
5.3 | -3.2958 | -3.6273 | -3.7659 |
5.4 | -3.2781 | -3.6077 | -3.7455 |
5.5 | -3.2611 | -3.5888 | -3.7259 |
5.6 | -3.2447 | -3.5707 | -3.7070 |
5.7 | -3.2289 | -3.5532 | -3.6888 |
5.8 | -3.2137 | -3.5364 | -3.6713 |
5.9 | -3.1991 | -3.5202 | -3.6544 |
6 | -3.1850 | -3.5045 | -3.6381 |
M | SFC (0.5Cfx√Rex) Fe3O4−CH3OH Cu−CH3OH Ag−CH3OH |
||
0.1 | -3.2921 | -3.6341 | -3.7770 |
0.2 | -3.2999 | -3.6414 | -3.7840 |
0.3 | -3.3128 | -3.6534 | -3.7957 |
0.4 | -3.3307 | -3.6701 | -3.8119 |
0.5 | -3.3536 | -3.6914 | -3.8326 |
0.6 | -3.3812 | -3.7171 | -3.8576 |
0.7 | -3.4133 | -3.7472 | -3.8869 |
0.8 | -3.4499 | -3.7814 | -3.9202 |
0.9 | -3.4906 | -3.8196 | -3.9574 |
1 | -3.5352 | -3.8616 | -3.9984 |
ψ | SFC (0.5Cfx√Rex) Fe3O4−CH3OH Cu−CH3OH Ag−CH3OH |
||
π/6 | -3.3218 | -3.6617 | -3.8038 |
π/4 | -3.3536 | -3.6914 | -3.8326 |
π/3 | -3.3849 | -3.7206 | -3.8610 |
π/2 | -3.4158 | -3.7495 | -3.8891 |
2π/3 | -3.3849 | -3.7206 | -3.8610 |
3π/4 | -3.3536 | -3.6914 | -3.8326 |
5π/6 | -3.3218 | -3.6617 | -3.8038 |
π | -3.2895 | -3.6317 | -3.7746 |
7π/6 | -3.3218 | -3.6617 | -3.3218 |
5π/4 | -3.3536 | -3.6914 | -3.3536 |
P0 | SFC (0.5Cfx√Rex) Fe3O4−CH3OH Cu−CH3OH Ag−CH3OH |
||
0.1 | -3.1449 | -3.4982 | -3.6452 |
0.2 | -3.1992 | -3.5481 | -3.6936 |
0.3 | -3.2520 | -3.5969 | -3.7409 |
0.4 | -3.3034 | -3.6447 | -3.7872 |
0.5 | -3.3536 | -3.6914 | -3.8326 |
0.6 | -3.4026 | -3.7371 | -3.8771 |
0.7 | -3.4505 | -3.7820 | -3.4505 |
0.8 | -3.4975 | -3.8260 | -3.4975 |
0.9 | -3.5434 | -3.8693 | -4.0060 |
1 | -3.5885 | -3.9118 | -4.0475 |
Df | SFC (0.5Cfx√Rex) Fe3O4−CH3OH Cu−CH3OH Ag−CH3OH |
||
0.1 | -3.2543 | -3.6001 | -3.7443 |
0.2 | -3.2794 | -3.6232 | -3.7666 |
0.3 | -3.3043 | -3.6461 | -3.7887 |
0.4 | -3.3291 | -3.6688 | -3.8107 |
0.5 | -3.3536 | -3.6914 | -3.8326 |
0.6 | -3.3779 | -3.7138 | -3.8543 |
0.7 | -3.4020 | -3.7360 | -3.8758 |
0.8 | -3.4259 | -3.7581 | -3.8973 |
0.9 | -3.4496 | -3.7801 | -3.9186 |
1.0 | -3.9397 | -3.8019 | -3.9397 |
α∗ | SFC (0.5Cfx√Rex) Fe3O4−CH3OH Cu−CH3OH Ag−CH3OH |
||
0.1 | -2.1593 | -2.2486 | -2.2844 |
0.2 | -2.0733 | -2.1473 | -2.1769 |
0.3 | -1.9909 | -2.0511 | -2.0750 |
0.4 | -1.9123 | -1.9598 | -1.9785 |
0.5 | -1.8374 | -1.8734 | -1.8874 |
0.6 | -1.7659 | -1.7916 | -1.8015 |
0.7 | -1.6980 | -1.7143 | -1.7205 |
0.8 | -1.6333 | -1.6413 | -1.6443 |
0.9 | -1.5718 | -1.5724 | -1.5725 |
1.0 | -1.5134 | -1.5074 | -1.5051 |
To calculate the effectiveness of the ANN models for predicting the SFC on a horizontal cylinder within three distinct nanofluids, we have considered specific performance indicators. These indicators include the mean squared error (MSE) and the coefficient of determination (R). The computation of these performance metrics involves the utilization of mathematical formulations, which can be expressed as:
MSE=1NN∑i=1(Xnum(i)−XANN(i))2, | (24) |
R=√1−N∑i=1(Xnum(i)−XANN(i))2N∑i=1(Xnum(i))2. | (25) |
The training process is a crucial stage in the development of ANN models. To perfect network training, the Levenberg–Marquardt algorithm was used. Within multi-layer perceptron (MLP) network structures, the training iteration continues until the error between target and prediction data generated at the output layer reaches a minimum level.
As the training advances through each epoch, the mean squared error (MSE) values, which were initially large, gradually drop, indicating that the network's performance is being refined. Figure 21(a)–(c) presents the training performances across all three ANN models. Notable observations occur throughout the training cycle (epoch) of an MLP network. The best validation performance for ANN model-Ⅰ is 0.0041131 at epoch 5. Similarly, the best validation performance for ANN model-Ⅱ is obtained at 0.013587 during epoch 4. Correspondingly, for ANN model-Ⅲ, the most favorable validation performance occurs at 0.012446 in epoch 6. Across all graphs, a consistent trend is apparent: the initial MSE values are relatively high, gradually decreasing as the training advances toward more refined stages.
The examination of error histograms is an additional stage for evaluating the training efficacy of ANN models. Throughout the training process, these histograms visually display the differences between predicted values and associated target values. In this regard, Figure 22(a)–(c) depicts the error histograms for the constructed ANN models intended to predict SFC values. Figure 22(a) provides the error histogram for ANN model-Ⅰ regarding the Fe3O4−CH3OH nanofluid on a horizontal cylinder. Figure 22(b) shows the error histogram for the ANN model-Ⅱ, focusing on the Cu−CH3OH nanofluid on a horizontal cylinder. Figure 22(c) displays the error histogram for the ANN model-Ⅲ regarding the Ag−CH3OH nanofluid on a horizontal cylinder. Each of these histograms was divided into 20 bins. A close examination shows that the errors obtained from each ANN model tend to cluster around the zero-error line. Furthermore, the magnitudes of these differences are generally small, which is an important point.
Figure 23(a)–(c) exhibits the regression values corresponding to the SFC data across all three ANN models. It is essential to understand that the regression value represents the correlation between the predicted and target values; a regression value close to 1 suggests a strong correlation. For ANN model-Ⅰ, the regression values were determined as R = 0.99698 for training, R = 0.99649 for validation, and R = 0.99362 for testing. The cumulative regression value for this model was computed as R = 0.99559 (Figure 23(a)). Regarding the ANN model-Ⅱ, the regression values were R = 0.99867 (training), R = 0.99434 (validation), and R = 0.9998 (testing), resulting in an overall regression value of R = 0.99764 (Figure 23(b)). For the ANN model-Ⅲ, the regression values were found to be R = 0.99822 (training), R = 0.98961 (validation), and R = 0.99598 (testing), resulting in an overall regression value of R = 0.99612 (Figure 23(c)). Based on the comprehensive statistical analysis presented in Figure 23(a)–(c), it is reasonable to conclude that the formulated ANN models are effective at predicting the SFC on a horizontal cylinder.
Figure 24(a)–(c) shows the mean square error (MSE) for each of the 70 data samples for the SFC predictions at the cylinder surface for the ANN Model-Ⅰ, ANN Model-Ⅱ, and ANN Model-Ⅲ, respectively. These figures collectively indicate the successful completion of the learning stages for the three models. A smaller MSE value, approaching zero, indicates fewer errors during the training of the ANN models to forecast SFC values. The average MSE is 0.000334 for ANN Model-Ⅰ, 0.012691 for ANN Model-Ⅱ, and 0.001038 for ANN Model-Ⅲ, all of which being notably low.
As a result, the training process of the neural network models is considered highly effective in accurately predicting SFC values at the horizontal cylinder for all three types of nanoparticles. Figure 25(a)–(c) shows a comprehensive comparison between the actual and predicted SFC values for each ANN model: ANN model-Ⅰ, ANN model-Ⅱ, and ANN model-Ⅲ, respectively. It is clear that the ANN model outputs closely align with the SFC target values. With such an overlap, we can conclude that the three constructed models can accurately predict SFC.
Table 18 shows the performance information needed to compute the MSE and R values across each of the ANN models during the training, validation, and testing stages. It is evident that the MSE values are minimal, and the R-values closely approach 1. This underscores the capability of the constructed models to predict SFC values on the surface of a horizontal cylinder with high accuracy.
Models | Nanoparticles | MSE | R | ||||
Training | Validation | Test | Training | Validation | Test | ||
ANN Model-Ⅰ | Fe3O4−CH3OH | 0.0021 | 0.0041 | 0.0101 | 0.9970 | 0.9964 | 0.9936 |
ANN Model-Ⅱ | Cu−CH3OH | 0.0037 | 0.0136 | 0.0049 | 0.9987 | 0.9943 | 0.9998 |
ANN Model-Ⅲ | Ag−CH3OH | 0.0022 | 0.01245 | 0.0075 | 0.9982 | 0.9896 | 0.9960 |
The objective of the current paper is to explore inclined magneto-Casson fluid flow over a stretching cylinder consisting of Darcy–Forchheimer medium and gyrotactic microorganisms. Additionally, the impact of joule heating, activation energy, viscous dissipation, binary chemical reaction rate, and convective boundary conditions are considered. The nanoparticles used in this research are Fe3O4, Cu, and Ag with CH3OH as the base fluid. These nanofluids are compared based on relevant parameters, and quantities are simulated numerically using tabular and graphical ways. The skin friction coefficient (SFC) values are calculated for all three types of nanoparticles using artificial neural network models along the cylinder's surface. The main outcomes are summarized as follows:
o Velocity decreases with magnetic, permeability, inclination, Darcy–Forchheimer number, and suction parameters and increases with the Casson fluid parameter.
o Temperature increases with magnetic field inclination, magnetic parameter, Eckert number, and thermal Biot number but decreases with the Casson fluid parameter.
o The concentration is enhanced by increasing diffusion Biot number and activation energy parameter and decreases with rising chemical reaction parameter and Schmidt number.
o The density distribution of motile microorganisms decreases with larger values of bioconvective Schmidt number, bioconvective Peclet number, and microorganism parameter.
o The skin friction coefficient increases with curvature parameter, magnetic parameter, permeability parameter, magnetic field inclination, and Darcy–Forchheimer number; an opposite behavior is observed with Casson fluid and suction parameters.
o The heat transfer rate decreases with the curvature parameter, magnetic parameter, Eckert number, and magnetic field inclination; an opposite influence can be noted with the Casson fluid parameter.
o The thermal Biot number has a positive impact on the heat transfer rate; the diffusion Biot number has a positive impact on mass transfer rate.
o The silver nanofluid has a dominant effect on the coefficient of skin friction, temperature, concentration, and motile microorganisms profiles; the ferrous ferric oxide nanofluid has a dominant impact on density number, Sherwood number, Nusselt number, and velocity profiles.
o The MSE values for all ANN Models Ⅰ, Ⅱ, and Ⅲ are 0.000334, 0.012691, and 0.001038, respectively, indicating that these possess the capability to accurately predict the SFC.
o This idea can be extended to more extensive interactions between nanofluids and microorganisms by refining flow systems inspired by biotechnology, verifying computational models using experimental data, and applying results to real-world biotechnology and engineering problems.
K. U. Rehman: Writing – original draft, validation, investigation, formal analysis; N. Fatima: Writing – review & editing, visualization, validation, investigation; W. Shatanawi: Visualization, supervision, investigation, conceptualization; N. Kousar: Investigation, data curation, conceptualization. All authors have read and approved the final version of the manuscript for publication.
The authors declare they have not used Artificial Intelligence (AI) tools in the creation of this article.
The authors would like to thank Prince Sultan University, Saudi Arabia, for the technical support through the TAS research lab.
The authors declare no conflict of interest.
[1] | S. U. S. Choi, J. A. Eastman, Enhancing thermal conductivity of fluids with nanoparticles, Argonne National Lab. (ANL), Argonne, IL (United States), 1995. |
[2] |
P. Valipour, F. S. Aski, M. Mirparizi, Influence of magnetic field on CNT-Polyethylene nanofluid flow over a permeable cylinder, J. Mol. Liq., 225 (2017), 592−597. https://doi.org/10.1016/j.molliq.2016.11.111 doi: 10.1016/j.molliq.2016.11.111
![]() |
[3] |
A. Mishra, M. Kumar, Velocity and thermal slip effects on MHD nanofluid flow past a stretching cylinder with viscous dissipation and Joule heating, SN Appl. Sci., 2 (2020), 1350. https://doi.org/10.1007/s42452-020-3156-7 doi: 10.1007/s42452-020-3156-7
![]() |
[4] |
M. Ramzan, N. Shaheen, J. D. Chung, S. Kadry, Y. M. Chu, F. Howari, Impact of Newtonian heating and Fourier and Fick's laws on a magnetohydrodynamic dusty Casson nanofluid flow with variable heat source/sink over a stretching cylinder, Sci. Rep., 11 (2021), 1−19. https://doi.org/10.1038/s41598-021-81747-x doi: 10.1038/s41598-021-81747-x
![]() |
[5] |
A. S. Rashed, T. A. Mahmoud, A. M. Wazwaz, Axisymmetric forced flow of nonhomogeneous nanofluid over heated permeable cylinders, Wave. Random Complex, 2022, 1−29. https://doi.org/10.1080/17455030.2022.2053611 doi: 10.1080/17455030.2022.2053611
![]() |
[6] |
S. Gouran, S. Mohsenian, S. E. Ghasemi, Theoretical analysis on MHD nanofluid flow between two concentric cylinders using efficient computational techniques, Alex. Eng. J., 61 (2022), 3237−3248. https://doi.org/10.1016/j.aej.2021.08.047 doi: 10.1016/j.aej.2021.08.047
![]() |
[7] |
F. Hussain, A. Hussain, S. Nadeem, Unsteady shear-thinning behaviour of nanofluid flow over exponential stretching/shrinking cylinder, J. Mol. Liq., 345 (2022), 117894. https://doi.org/10.1016/j.molliq.2021.117894 doi: 10.1016/j.molliq.2021.117894
![]() |
[8] |
P. Kumar, H. Poonia, L. Ali, S. Areekara, A. Mathew, Effects of different nanoparticles Cu, TiO2, and Ag on fluid flow and heat transfer over cylindrical surface subject to non-fourier heat flux model, Numer. Heat Tr. B-Fund., 85 (2024), 1−19. https://doi.org/10.1080/10407790.2023.2235077 doi: 10.1080/10407790.2023.2235077
![]() |
[9] |
K. L. Hsiao, Micropolar nanofluid flow with MHD and viscous dissipation effects towards a stretching sheet with multimedia feature, Int. J. Heat Mass Tr., 112 (2017), 983−990. https://doi.org/10.1016/j.ijheatmasstransfer.2017.05.042 doi: 10.1016/j.ijheatmasstransfer.2017.05.042
![]() |
[10] |
F. Shahzad, W. Jamshed, K. S. Nisar, M. M. Khashan, A. H. Abdel-Aty, Computational analysis of Ohmic and viscous dissipation effects on MHD heat transfer flow of Cu-PVA Jeffrey nanofluid through a stretchable surface, Case Stud. Therm. Eng., 26 (2021), 101148. https://doi.org/10.1016/j.csite.2021.101148 doi: 10.1016/j.csite.2021.101148
![]() |
[11] |
S. M. Zokri, N. S. Arifin, M. K. A. Mohamed, A. R. M. Kasim, N. F. Mohammad, M. Z. Salleh, Mathematical model of mixed convection boundary layer flow over a horizontal circular cylinder filled in a Jeffrey fluid with viscous dissipation effect, Sains Malays., 47 (2018), 1607−1615. https://doi.org/10.17576/jsm-2018-4707-32 doi: 10.17576/jsm-2018-4707-32
![]() |
[12] |
H. U. Rasheed, Z. Khan, E. R. El-Zahar, N. A. Shah, S. Islam, T. Abbas, Homotopic solutions of an unsteady magnetohydrodynamic flow of Casson nanofluid flow by a vertical cylinder with Brownian and viscous dissipation effects, Wave. Random Complex, 2022, 1−14. https://doi.org/10.1080/17455030.2022.2105979 doi: 10.1080/17455030.2022.2105979
![]() |
[13] |
S. Nandi, B. Kumbhakar, Entropy generation in MHD nanofluid flow induced by a slendering stretching sheet with activation energy, viscous dissipation and Joule heating impacts, Indian J. Phys., 96 (2022), 2873−2892. https://doi.org/10.1007/s12648-021-02206-x doi: 10.1007/s12648-021-02206-x
![]() |
[14] |
G. R. Ganesh, W. Sridhar, K. Al-Farhany, S. E. Ahmed, Electrically MHD Casson nanofluid flow and entropy exploration under the influence of the viscous dissipation, radiation, and higher-order chemical reaction, Phys. Scripta, 97 (2022), 065208. https://doi.org/10.1088/1402-4896/ac6e51 doi: 10.1088/1402-4896/ac6e51
![]() |
[15] |
S. K. Saini, R. Agrawal, P. Kaswan, Activation energy and convective heat transfer effects on the radiative Williamson nanofluid flow over a radially stretching surface containing Joule heating and viscous dissipation, Numer. Heat Tr. A-Appl., 85 (2024), 1−24. https://doi.org/10.1080/10407782.2023.2226815 doi: 10.1080/10407782.2023.2226815
![]() |
[16] |
R. Bestman, Natural convection boundary layer with suction and mass transfer in a porous medium, Int. J. Energ. Res., 14 (1990), 389−396. https://doi.org/10.1002/er.4440140403 doi: 10.1002/er.4440140403
![]() |
[17] |
M. Azam, T. Xu, F. Mabood, M. Khan, Non-linear radiative bioconvection flow of cross nano-material with gyrotatic microorganisms and activation energy, Int. Commun. Heat Mass, 127 (2021), 105530. https://doi.org/10.1016/j.icheatmasstransfer.2021.105530 doi: 10.1016/j.icheatmasstransfer.2021.105530
![]() |
[18] |
S. A. A. Shah, N. A. Ahammad, B. Ali, K. Guedri, A. U. Awan, F. Gamaoun, et al., Significance of bio-convection, MHD, thermal radiation and activation energy across Prandtl nanofluid flow: A case of stretching cylinder, Int. Commun. Heat Mass, 137 (2022), 106299. https://doi.org/10.1016/j.icheatmasstransfer.2022.106299 doi: 10.1016/j.icheatmasstransfer.2022.106299
![]() |
[19] |
A. Ali, S. Sarkar, S. Das, R. N. Jana, A report on entropy generation and Arrhenius kinetics in magneto-bioconvective flow of Cross nanofluid over a cylinder with wall slip, Int. J. Ambient Energy, 2022, 1−16. https://doi.org/10.1080/01430750.2022.2031292 doi: 10.1080/01430750.2022.2031292
![]() |
[20] |
E. Sangeetha, D. Poulomi, Gyrotactic microorganisms suspended in MHD nanofluid with activation energy and binary chemical reaction over a non-Darcian porous medium, Wave. Random Complex, 2022, 1−17. https://doi.org/10.1080/17455030.2022.2112114 doi: 10.1080/17455030.2022.2112114
![]() |
[21] |
Y. S. Kumar, S. Hussain, K. Raghunath, F. Ali, K. Guedri, S. M. Eldin, et al., Numerical analysis of magnetohydrodynamics Casson nanofluid flow with activation energy, Hall current and thermal radiation, Sci. Rep., 13 (2023), 4021. https://doi.org/10.1038/s41598-023-28379-5 doi: 10.1038/s41598-023-28379-5
![]() |
[22] |
N. Fatima, N. Kousar, K. U. Rehman, W. Shatanawi, Computational analysis of heat and mass transfer in magnetized Darcy-Forchheimer hybrid nanofluid flow with porous medium and slip effects, CMES-Comput. Model. Eng., 137 (2023). https://doi.org/10.32604/cmes.2023.026994 doi: 10.32604/cmes.2023.026994
![]() |
[23] |
M. S. Plesset, H. Winet, Bioconvection patterns in swimming microorganism cultures as an example of Rayleigh-Taylor instability, Nature, 248 (1974), 441−443. https://doi.org/10.1038/248441a0 doi: 10.1038/248441a0
![]() |
[24] |
Z. Abdelmalek, S. U. Khan, H. Waqas, A. Riaz, I. A. Khan, I. Tlili, A mathematical model for bioconvection flow of Williamson nanofluid over a stretching cylinder featuring variable thermal conductivity, activation energy and second-order slip, J. Therm. Anal. Calorim., 144 (2021), 205−217. https://doi.org/10.1007/s10973-020-09450-z doi: 10.1007/s10973-020-09450-z
![]() |
[25] |
M. Imran, U. Farooq, H. Waqas, A. E. Anqi, M. R. Safaei, Numerical performance of thermal conductivity in Bioconvection flow of cross nanofluid containing swimming microorganisms over a cylinder with melting phenomenon, Case Stud. Therm. Eng., 26 (2021), 101181. https://doi.org/10.1016/j.csite.2021.101181 doi: 10.1016/j.csite.2021.101181
![]() |
[26] |
H. A. Nabwey, S. I. Alshber, A. M. Rashad, A. E. N. Mahdy, Influence of bioconvection and chemical reaction on magneto—Carreau nanofluid flow through an inclined cylinder, Mathematics, 10 (2022), 504. https://doi.org/10.3390/math10030504 doi: 10.3390/math10030504
![]() |
[27] |
T. Muhammad, H. Waqas, U. Manzoor, U. Farooq, Z. F. Rizvi, On doubly stratified bioconvective transport of Jeffrey nanofluid with gyrotactic motile microorganisms, Alex. Eng. J., 61 (2022), 1571−1583. https://doi.org/10.1016/j.aej.2021.06.059 doi: 10.1016/j.aej.2021.06.059
![]() |
[28] |
E. M. A. Elbashbeshy, H. G. Asker, B. Nagy, The effects of heat generation absorption on boundary layer flow of a nanofluid containing gyrotactic microorganisms over an inclined stretching cylinder, Ain Shams Eng. J., 13 (2022), 101690. https://doi.org/10.1016/j.asej.2022.101690 doi: 10.1016/j.asej.2022.101690
![]() |
[29] |
M. S. Alqarni, H. Waqas, U. Manzoor, T. Muhammad, Marangoni transport of Jeffrey nanofluid due to circular horizontal cylinder with motile microorganisms, Wave. Random Complex, 2022, 1−20. https://doi.org/10.1080/17455030.2022.2067368 doi: 10.1080/17455030.2022.2067368
![]() |
[30] | N. Casson, Flow equation for pigment-oil suspensions of the printing ink-type, Rheol. Disperse Syst., 1959, 84−104. |
[31] |
A. Zeeshan, O. U. Mehmood, F. Mabood, F. Alzahrani, Numerical analysis of hydromagnetic transport of Casson nanofluid over permeable linearly stretched cylinder with Arrhenius activation energy, Int. Commun. Heat Mass, 130 (2022), 105736. https://doi.org/10.1016/j.icheatmasstransfer.2021.105736 doi: 10.1016/j.icheatmasstransfer.2021.105736
![]() |
[32] | G. Kumar, S. M. K. Rizvi, Casson fluid flow past on vertical cylinder in the presence of chemical reaction and magnetic field, Appl. Appl. Math., 16 (2021), 28. |
[33] |
S. Nandi, B. Kumbhakar, S. Sarkar, MHD stagnation point flow of Fe3O4/Cu/Ag-CH3OH nanofluid along a convectively heated stretching sheet with partial slip and activation energy: Numerical and statistical approach, Int. Commun. Heat Mass, 130 (2022), 105791. https://doi.org/10.1016/j.icheatmasstransfer.2021.105791 doi: 10.1016/j.icheatmasstransfer.2021.105791
![]() |
[34] |
S. M. Hussain, Dynamics of ethylene glycol-based graphene and molybdenum disulfide hybrid nanofluid over a stretchable surface with slip conditions, Sci. Rep., 12 (2022), 1751. https://doi.org/10.1038/s41598-022-05703-z doi: 10.1038/s41598-022-05703-z
![]() |
[35] |
S. M. Hussain, R. Sharma, M. K. Mishra, G. S. Seth, Radiative magneto-nanofluid over an accelerated moving ramped temperature plate with Hall effects, J. Nanofluids, 6 (2017), 840−851. https://doi.org/10.1166/jon.2017.1381 doi: 10.1166/jon.2017.1381
![]() |
[36] |
Y. Nawaz, M. S. Arif, K. Abodayeh, A. H. Soori, A two-stage multi-step numerical scheme for mixed convective Williamson nanofluid flow over flat and oscillatory sheets, Int. J. Mod. Phys. B, 38 (2024), 2450298. https://doi.org/10.1142/S0217979224502989 doi: 10.1142/S0217979224502989
![]() |
[37] |
S. Ullah, I. Ullah, A. Ali, K. Shah, T. Abdeljawad, Investigation of cross-diffusion effect on radiative Jeffery-Hamel flow in convergent/divergent stretchable channel with Lorentz force and Joule heating, Alex. Eng. J., 86 (2024), 289−297. https://doi.org/10.1016/j.aej.2023.11.054 doi: 10.1016/j.aej.2023.11.054
![]() |
[38] |
S. Shaheen, H. Huang, F. A. M. Al-Yarimi, M. B. Arain, Theoretical analysis of Ellis fluid flow in two layers due to metachronal propulsion subject to heat and mass transfer: Application in biological function, Case Stud. Therm. Eng., 59 (2024), 104446. https://doi.org/10.1016/j.csite.2024.104446 doi: 10.1016/j.csite.2024.104446
![]() |
[39] |
M. B. Arain, S. Shaheen, F. A. M. Al-Yarimi, N. Ijaz, J. Hu, Sensitivity analysis for acoustic-driven gas bubble dynamics in tangent hyperbolic fluid, J. Mol. Liq., 395 (2024), 123894. https://doi.org/10.1016/j.molliq.2023.123894 doi: 10.1016/j.molliq.2023.123894
![]() |
[40] |
S. M. Isa, R. Mahat, N. M. Katbar, B. S. Goud, I. Ullah, W. Jamshed, Thermal radiative and Hall current effects on magneto-natural convective flow of dusty fluid: Numerical Runge–Kutta–Fehlberg technique, Numer. Heat Tr. B-Fund., 2024, 1−23. https://doi.org/10.1080/10407790.2024.2318452 doi: 10.1080/10407790.2024.2318452
![]() |
[41] |
W. A. Khan, I. Pop, Boundary-layer flow of a nanofluid past a stretching sheet, Int. J. Heat Mass Tran., 53 (2010), 2477−2483. https://doi.org/10.1016/j.ijheatmasstransfer.2010.01.032 doi: 10.1016/j.ijheatmasstransfer.2010.01.032
![]() |
[42] |
K. Ali, S. Ahmad, K. S. Nisar, A. A. Faridi, M. Ashraf, Simulation analysis of MHD hybrid CuAl2O3/H2O nanofluid flow with heat generation through a porous media, Int. J. Energy Res., 45 (2021), 19165−19179. https://doi.org/10.1002/er.7016 doi: 10.1002/er.7016
![]() |
[43] |
S. Ahmad, S. Akhter, M. I. Shahid, K. Ali, M. Akhtar, M. Ashraf, Novel thermal aspects of hybrid nanofluid flow comprising of manganese zinc ferrite MnZnFe2O4, nickel zinc ferrite NiZnFe2O4 and motile microorganisms, Ain Shams Eng. J., 13 (2022), 101668. https://doi.org/10.1016/j.asej.2021.101668 doi: 10.1016/j.asej.2021.101668
![]() |
[44] |
Z. Said, P. Sharma, R. M. Elavarasan, A. K. Tiwari, M. K. Rathod, Exploring the specific heat capacity of water-based hybrid nanofluids for solar energy applications: A comparative evaluation of modern ensemble machine learning techniques, J. Energy Storage, 54 (2022), 105230. https://doi.org/10.1016/j.est.2022.105230 doi: 10.1016/j.est.2022.105230
![]() |
[45] |
K. U. Rehman, W. Shatanawi, Non-newtonian mixed convection magnetized flow with heat generation and viscous dissipation effects: A prediction application of artificial intelligence, Processes, 11 (2023), 986. https://doi.org/10.3390/pr11040986 doi: 10.3390/pr11040986
![]() |
[46] |
A. S. Baazeem, M. S. Arif, K. Abodayeh, An efficient and accurate approach to electrical boundary layer nanofluid flow simulation: A use of artificial intelligence, Processes, 11 (2023), 2736. https://doi.org/10.3390/pr11092736 doi: 10.3390/pr11092736
![]() |
1. | Muhammad Sohail, Muhammad Hussain Ali, Kamaleldin Abodayeh, Syed Tehseen Abbas, Bio-convective boundary layer flow of Maxwell nanofluid via optimal homotopic procedure with radiation and Darcy-Forchheimer impacts over a stretched sheet, 2025, 46, 0143-0750, 10.1080/01430750.2025.2462583 |
Properties | CH3OH | Fe3O4 | Cu | Ag |
ρ(kg/m3) | 792 | 5180 | 8933 | 10,500 |
σ(Ω−1m−1) | 0.5×10-6 | 0.74×106 | 5.96×107 | 6.3×107 |
k(W/mK) | 0.2035 | 9.7 | 400 | 429 |
cp(J/kgK) | 2545 | 670 | 385 | 235 |
Pr | 7.38 |
Properties | Nanofluids |
Dynamic viscosity | μnfμf=1(1−ϕ1)2.5 |
Density | ρnfρf=(1−ϕ1)+ϕ1ρnfρf |
Heat capacitance | (ρcp)nf(ρcp)f=(1−ϕ1)+ϕ1(ρcp)nf(ρcp)f |
Thermal conductivity | knfkf=1−ϕ1+2ϕ1knfknf−kfln(knf+kf2kf)1−ϕ1+2ϕ1kfknf−kfln(knf+kf2kf) |
Electrical conductivity | σnfσf=1+3ϕ1(σnfσf−1)(σnfσf+2)−ϕ1(σnfσf−1) |
Parameters | Formula | Parameters | Formula |
Curvature parameter | γ=√νfca2 | Magnetic field parameter | M2=Bo2σfcρf |
Permeability parameter | P0=νfcK∗ | Darcy–Forchheimer number | Df=Cbxρf√K∗ |
Eckert number | Ec=Uw2(cp)f(Tw−T∞) | Prandtl number | Pr=μf(cp)fkf |
Bioconvection Peclet number | Pe=bWcDn | Bioconvection Schmidt number | Sb=νfDn |
Microorganism parameter | Ω=N∞Nw−N∞ | Schmidt number | Sc=νfDB |
Activation energy parameter | Ea=E∗k∗T∞ | Chemical reaction parameter | ˆσ=Kr2c |
Temperature difference parameter | δ=Tw−T∞T∞ | Suction parameter | α∗=vw√cνf |
Thermal Biot number | Bi=hfkf√νfc | Diffusion Biot number | Bj=hsDB√νfc |
Pr | Khan and Pop [41] | Ali et al. [42] | Ahmad et al. [43] | Present |
2 | 0.9113 | 0.9105 | 0.9105 | 0.9114 |
7 | 1.8954 | 1.8908 | 1.8908 | 1.8954 |
20 | 3.3539 | 3.3527 | 3.3527 | 3.3539 |
70 | 6.4621 | 6.4781 | 6.4781 | 6.4622 |
γ | β | M | ψ | P0 | Df | α∗ | 0.5Cfx√Rex Fe3O4−CH3OH Cu−CH3OH Ag−CH3OH |
||
0.1 | 5.0 | 0.5 | π/4 | 0.5 | 0.5 | -1.0 | 3.3536 | 3.6914 | 3.8326 |
0.2 | - | - | - | - | - | - | 3.4812 | 3.8187 | 3.9597 |
0.3 | - | - | - | - | - | - | 3.6085 | 3.9458 | 4.0867 |
0.1 | 5.0 | - | - | - | - | - | 3.3536 | 3.6914 | 3.8326 |
- | 6.0 | - | - | - | - | - | 3.1850 | 3.5045 | 3.6381 |
- | 7.0 | - | - | - | - | - | 3.0673 | 3.3742 | 3.5025 |
- | 5.0 | 0.1 | - | - | - | - | 3.2921 | 3.6341 | 3.7770 |
- | - | 0.3 | - | - | - | - | 3.3128 | 3.6534 | 3.7957 |
- | - | 0.6 | - | - | - | - | 3.3812 | 3.7171 | 3.8576 |
- | - | 0.5 | π/6 | - | - | - | 3.3218 | 3.6617 | 3.8038 |
- | - | - | π/3 | - | - | - | 3.3849 | 3.7206 | 3.8610 |
- | - | - | π/2 | - | - | - | 3.4158 | 3.7495 | 3.8891 |
- | - | - | π/4 | 0.8 | - | - | 3.4975 | 3.8260 | 3.9637 |
- | - | - | - | 1.8 | - | - | 3.9228 | 4.2294 | 4.3585 |
- | - | - | - | 2.8 | - | - | 4.2910 | 4.5831 | 4.7062 |
- | - | - | - | 0.5 | 0.6 | - | 3.3779 | 3.7138 | 3.8543 |
- | - | - | - | - | 0.8 | - | 3.4259 | 3.7581 | 3.8973 |
- | - | - | - | - | 1.0 | - | 3.4732 | 3.8019 | 3.9397 |
- | - | - | - | - | 0.5 | -0.9 | 3.2274 | 3.5372 | 3.6665 |
- | - | - | - | - | - | -0.7 | 2.9848 | 3.2413 | 3.3479 |
- | - | - | - | - | - | -0.5 | 2.7560 | 2.9634 | 3.0491 |
γ | β | M | ψ | Ec | Bi | Nux/√Rex Fe3O4−CH3OH Cu−CH3OH Ag−CH3OH |
||
0.1 | 1.0 | 0.5 | π/4 | 0.5 | 0.3 | 0.2160 | 0.2084 | 0.2028 |
0.2 | - | - | - | - | - | 0.2056 | 0.1980 | 0.1920 |
0.3 | - | - | - | - | - | 0.1950 | 0.1872 | 0.1810 |
0.1 | 1.2 | - | - | - | - | 0.2279 | 0.2217 | 0.2169 |
- | 1.4 | - | - | - | - | 0.2357 | 0.2303 | 0.2262 |
- | 1.6 | - | - | - | - | 0.2412 | 0.2364 | 0.2326 |
- | 1.0 | 0.1 | - | - | - | 0.2174 | 0.2097 | 0.2040 |
- | - | 0.3 | - | - | - | 0.2169 | 0.2093 | 0.2036 |
- | - | 0.6 | - | - | - | 0.2153 | 0.2078 | 0.2022 |
- | - | 0.5 | π/6 | - | - | 0.2167 | 0.2091 | 0.2034 |
- | - | - | π/3 | - | - | 0.2152 | 0.2078 | 0.2021 |
- | - | - | π/2 | - | - | 0.2145 | 0.2071 | 0.2015 |
- | - | - | π/4 | 0.1 | - | 0.2748 | 0.2734 | 0.2721 |
- | - | - | - | 0.2 | - | 0.2601 | 0.2571 | 0.2547 |
- | - | - | - | 0.3 | - | 0.2454 | 0.2409 | 0.2374 |
- | - | - | - | 0.5 | 0.2 | 0.1457 | 0.1406 | 0.1368 |
- | - | - | - | - | 0.4 | 0.2846 | 0.2747 | 0.2672 |
- | - | - | - | - | 0.6 | 0.4173 | 0.4029 | 0.3917 |
γ | β | M | Sc | ˆσ | Ea | Bj | Shx/√Rex Fe3O4−CH3OH Cu−CH3OH Ag−CH3OH |
||
0.1 | 1.0 | 0.5 | 1.0 | 1.0 | 0.5 | 0.3 | 0.2530 | 0.2529 | 0.2529 |
0.2 | - | - | - | - | - | - | 0.2537 | 0.2536 | 0.2536 |
0.3 | - | - | - | - | - | - | 0.2544 | 0.2544 | 0.2543 |
0.1 | 1.2 | - | - | - | - | - | 0.2532 | 0.2530 | 0.2530 |
- | 1.4 | - | - | - | - | - | 0.2532 | 0.2531 | 0.2531 |
- | 1.6 | - | - | - | - | - | 0.2533 | 0.2532 | 0.2531 |
- | 1.0 | 0.1 | - | - | - | - | 0.2531 | 0.2530 | 0.2529 |
- | - | 0.3 | - | - | - | - | 0.2531 | 0.2530 | 0.2529 |
- | - | 0.6 | - | - | - | - | 0.2530 | 0.2529 | 0.2529 |
- | - | 0.5 | 0.3 | - | - | - | 0.2096 | 0.2094 | 0.2094 |
- | - | - | 0.6 | - | - | - | 0.2362 | 0.2361 | 0.2360 |
- | - | - | 0.9 | - | - | - | 0.2498 | 0.2497 | 0.2497 |
- | - | - | 1.0 | 1.5 | - | - | 0.2565 | 0.2565 | 0.2565 |
- | - | - | - | 2.0 | - | - | 0.2592 | 0.2592 | 0.2592 |
- | - | - | - | 2.5 | - | - | 0.2613 | 0.2614 | 0.2614 |
- | - | - | - | 1.0 | 0.6 | - | 0.2523 | 0.2521 | 0.2521 |
- | - | - | - | - | 0.7 | - | 0.2515 | 0.2514 | 0.2513 |
- | - | - | - | - | 0.8 | - | 0.2508 | 0.2506 | 0.2506 |
- | - | - | - | - | 0.5 | 0.2 | 0.1780 | 0.1779 | 0.1779 |
- | - | - | - | - | - | 0.4 | 0.3207 | 0.3205 | 0.3205 |
- | - | - | - | - | - | 0.6 | 0.4376 | 0.4373 | 0.4372 |
γ | β | M | Sb | Pe | Ω | Dnx/√Rex Fe3O4−CH3OH Cu−CH3OH Ag−CH3OH |
||
0.1 | 1.0 | 0.5 | 0.1 | 0.2 | 0.2 | 0.2957 | 0.2943 | 0.2938 |
0.2 | - | - | - | - | - | 0.3591 | 0.3580 | 0.3576 |
0.3 | - | - | - | - | - | 0.4171 | 0.4162 | 0.4158 |
0.1 | 1.2 | - | - | - | - | 0.2970 | 0.2955 | 0.2949 |
- | 1.4 | - | - | - | - | 0.2980 | 0.2964 | 0.2958 |
- | 1.6 | - | - | - | - | 0.2989 | 0.2972 | 0.2966 |
- | 1.0 | 0.1 | - | - | - | 0.2959 | 0.2945 | 0.2939 |
- | - | 0.3 | - | - | - | 0.2959 | 0.2944 | 0.2939 |
- | - | 0.6 | - | - | - | 0.2956 | 0.2942 | 0.2937 |
- | - | 0.5 | 0.5 | - | - | 0.6931 | 0.6843 | 0.6810 |
- | - | - | 1.5 | - | - | 1.8345 | 1.8169 | 1.8102 |
- | - | - | 2.5 | - | - | 2.9361 | 2.9162 | 2.9084 |
- | - | - | 0.1 | 0.1 | - | 0.2647 | 0.2632 | 0.2627 |
- | - | - | - | 0.3 | - | 0.3268 | 0.3253 | 0.3248 |
- | - | - | - | 0.5 | - | 0.3888 | 0.3874 | 0.3869 |
- | - | - | - | 0.2 | 0.4 | 0.3061 | 0.3047 | 0.3042 |
- | - | - | - | - | 0.8 | 0.3269 | 0.3254 | 0.3249 |
- | - | - | - | - | 1.2 | 0.3476 | 0.3462 | 0.3457 |
Models | Nanoparticles | Input | Output | ||||||
Model-Ⅰ | Fe3O4−CH3OH | γ | β | M | ψ | P0 | Df | α∗ | SFC |
Model-Ⅱ | Cu−CH3OH | γ | β | M | ψ | P0 | Df | α∗ | SFC |
Model-Ⅲ | Ag−CH3OH | γ | β | M | ψ | P0 | Df | α∗ | SFC |
Samples | Model-Ⅰ | Model-Ⅱ | Model-Ⅲ |
Training data | 48 | 48 | 48 |
Validation data | 11 | 11 | 11 |
Testing data | 11 | 11 | 11 |
Total | 70 | 70 | 70 |
γ | SFC (0.5Cfx√Rex) Fe3O4−CH3OH Cu−CH3OH Ag−CH3OH |
||
0.1 | -3.3536 | -3.6914 | -3.8326 |
0.2 | -3.4812 | -3.8187 | -3.9597 |
0.3 | -3.6085 | -3.9458 | -4.0867 |
0.4 | -3.7355 | -4.0726 | -4.2135 |
0.5 | -3.8622 | -4.1993 | -4.3401 |
0.6 | -3.9886 | -4.3257 | -4.4665 |
0.7 | -4.1148 | -4.4519 | -4.5927 |
0.8 | -4.2407 | -4.5779 | -4.7187 |
0.9 | -4.3664 | -4.7037 | -4.8445 |
1 | -4.4920 | -4.8294 | -4.9702 |
β | SFC (0.5Cfx√Rex) Fe3O4−CH3OH Cu−CH3OH Ag−CH3OH |
||
5.1 | -3.3335 | -3.6691 | -3.8094 |
5.2 | -3.3143 | -3.6478 | -3.7872 |
5.3 | -3.2958 | -3.6273 | -3.7659 |
5.4 | -3.2781 | -3.6077 | -3.7455 |
5.5 | -3.2611 | -3.5888 | -3.7259 |
5.6 | -3.2447 | -3.5707 | -3.7070 |
5.7 | -3.2289 | -3.5532 | -3.6888 |
5.8 | -3.2137 | -3.5364 | -3.6713 |
5.9 | -3.1991 | -3.5202 | -3.6544 |
6 | -3.1850 | -3.5045 | -3.6381 |
M | SFC (0.5Cfx√Rex) Fe3O4−CH3OH Cu−CH3OH Ag−CH3OH |
||
0.1 | -3.2921 | -3.6341 | -3.7770 |
0.2 | -3.2999 | -3.6414 | -3.7840 |
0.3 | -3.3128 | -3.6534 | -3.7957 |
0.4 | -3.3307 | -3.6701 | -3.8119 |
0.5 | -3.3536 | -3.6914 | -3.8326 |
0.6 | -3.3812 | -3.7171 | -3.8576 |
0.7 | -3.4133 | -3.7472 | -3.8869 |
0.8 | -3.4499 | -3.7814 | -3.9202 |
0.9 | -3.4906 | -3.8196 | -3.9574 |
1 | -3.5352 | -3.8616 | -3.9984 |
ψ | SFC (0.5Cfx√Rex) Fe3O4−CH3OH Cu−CH3OH Ag−CH3OH |
||
π/6 | -3.3218 | -3.6617 | -3.8038 |
π/4 | -3.3536 | -3.6914 | -3.8326 |
π/3 | -3.3849 | -3.7206 | -3.8610 |
π/2 | -3.4158 | -3.7495 | -3.8891 |
2π/3 | -3.3849 | -3.7206 | -3.8610 |
3π/4 | -3.3536 | -3.6914 | -3.8326 |
5π/6 | -3.3218 | -3.6617 | -3.8038 |
π | -3.2895 | -3.6317 | -3.7746 |
7π/6 | -3.3218 | -3.6617 | -3.3218 |
5π/4 | -3.3536 | -3.6914 | -3.3536 |
P0 | SFC (0.5Cfx√Rex) Fe3O4−CH3OH Cu−CH3OH Ag−CH3OH |
||
0.1 | -3.1449 | -3.4982 | -3.6452 |
0.2 | -3.1992 | -3.5481 | -3.6936 |
0.3 | -3.2520 | -3.5969 | -3.7409 |
0.4 | -3.3034 | -3.6447 | -3.7872 |
0.5 | -3.3536 | -3.6914 | -3.8326 |
0.6 | -3.4026 | -3.7371 | -3.8771 |
0.7 | -3.4505 | -3.7820 | -3.4505 |
0.8 | -3.4975 | -3.8260 | -3.4975 |
0.9 | -3.5434 | -3.8693 | -4.0060 |
1 | -3.5885 | -3.9118 | -4.0475 |
Df | SFC (0.5Cfx√Rex) Fe3O4−CH3OH Cu−CH3OH Ag−CH3OH |
||
0.1 | -3.2543 | -3.6001 | -3.7443 |
0.2 | -3.2794 | -3.6232 | -3.7666 |
0.3 | -3.3043 | -3.6461 | -3.7887 |
0.4 | -3.3291 | -3.6688 | -3.8107 |
0.5 | -3.3536 | -3.6914 | -3.8326 |
0.6 | -3.3779 | -3.7138 | -3.8543 |
0.7 | -3.4020 | -3.7360 | -3.8758 |
0.8 | -3.4259 | -3.7581 | -3.8973 |
0.9 | -3.4496 | -3.7801 | -3.9186 |
1.0 | -3.9397 | -3.8019 | -3.9397 |
α∗ | SFC (0.5Cfx√Rex) Fe3O4−CH3OH Cu−CH3OH Ag−CH3OH |
||
0.1 | -2.1593 | -2.2486 | -2.2844 |
0.2 | -2.0733 | -2.1473 | -2.1769 |
0.3 | -1.9909 | -2.0511 | -2.0750 |
0.4 | -1.9123 | -1.9598 | -1.9785 |
0.5 | -1.8374 | -1.8734 | -1.8874 |
0.6 | -1.7659 | -1.7916 | -1.8015 |
0.7 | -1.6980 | -1.7143 | -1.7205 |
0.8 | -1.6333 | -1.6413 | -1.6443 |
0.9 | -1.5718 | -1.5724 | -1.5725 |
1.0 | -1.5134 | -1.5074 | -1.5051 |
Models | Nanoparticles | MSE | R | ||||
Training | Validation | Test | Training | Validation | Test | ||
ANN Model-Ⅰ | Fe3O4−CH3OH | 0.0021 | 0.0041 | 0.0101 | 0.9970 | 0.9964 | 0.9936 |
ANN Model-Ⅱ | Cu−CH3OH | 0.0037 | 0.0136 | 0.0049 | 0.9987 | 0.9943 | 0.9998 |
ANN Model-Ⅲ | Ag−CH3OH | 0.0022 | 0.01245 | 0.0075 | 0.9982 | 0.9896 | 0.9960 |
Properties | CH3OH | Fe3O4 | Cu | Ag |
ρ(kg/m3) | 792 | 5180 | 8933 | 10,500 |
σ(Ω−1m−1) | 0.5×10-6 | 0.74×106 | 5.96×107 | 6.3×107 |
k(W/mK) | 0.2035 | 9.7 | 400 | 429 |
cp(J/kgK) | 2545 | 670 | 385 | 235 |
Pr | 7.38 |
Properties | Nanofluids |
Dynamic viscosity | μnfμf=1(1−ϕ1)2.5 |
Density | ρnfρf=(1−ϕ1)+ϕ1ρnfρf |
Heat capacitance | (ρcp)nf(ρcp)f=(1−ϕ1)+ϕ1(ρcp)nf(ρcp)f |
Thermal conductivity | knfkf=1−ϕ1+2ϕ1knfknf−kfln(knf+kf2kf)1−ϕ1+2ϕ1kfknf−kfln(knf+kf2kf) |
Electrical conductivity | σnfσf=1+3ϕ1(σnfσf−1)(σnfσf+2)−ϕ1(σnfσf−1) |
Parameters | Formula | Parameters | Formula |
Curvature parameter | γ=√νfca2 | Magnetic field parameter | M2=Bo2σfcρf |
Permeability parameter | P0=νfcK∗ | Darcy–Forchheimer number | Df=Cbxρf√K∗ |
Eckert number | Ec=Uw2(cp)f(Tw−T∞) | Prandtl number | Pr=μf(cp)fkf |
Bioconvection Peclet number | Pe=bWcDn | Bioconvection Schmidt number | Sb=νfDn |
Microorganism parameter | Ω=N∞Nw−N∞ | Schmidt number | Sc=νfDB |
Activation energy parameter | Ea=E∗k∗T∞ | Chemical reaction parameter | ˆσ=Kr2c |
Temperature difference parameter | δ=Tw−T∞T∞ | Suction parameter | α∗=vw√cνf |
Thermal Biot number | Bi=hfkf√νfc | Diffusion Biot number | Bj=hsDB√νfc |
Pr | Khan and Pop [41] | Ali et al. [42] | Ahmad et al. [43] | Present |
2 | 0.9113 | 0.9105 | 0.9105 | 0.9114 |
7 | 1.8954 | 1.8908 | 1.8908 | 1.8954 |
20 | 3.3539 | 3.3527 | 3.3527 | 3.3539 |
70 | 6.4621 | 6.4781 | 6.4781 | 6.4622 |
γ | β | M | ψ | P0 | Df | α∗ | 0.5Cfx√Rex Fe3O4−CH3OH Cu−CH3OH Ag−CH3OH |
||
0.1 | 5.0 | 0.5 | π/4 | 0.5 | 0.5 | -1.0 | 3.3536 | 3.6914 | 3.8326 |
0.2 | - | - | - | - | - | - | 3.4812 | 3.8187 | 3.9597 |
0.3 | - | - | - | - | - | - | 3.6085 | 3.9458 | 4.0867 |
0.1 | 5.0 | - | - | - | - | - | 3.3536 | 3.6914 | 3.8326 |
- | 6.0 | - | - | - | - | - | 3.1850 | 3.5045 | 3.6381 |
- | 7.0 | - | - | - | - | - | 3.0673 | 3.3742 | 3.5025 |
- | 5.0 | 0.1 | - | - | - | - | 3.2921 | 3.6341 | 3.7770 |
- | - | 0.3 | - | - | - | - | 3.3128 | 3.6534 | 3.7957 |
- | - | 0.6 | - | - | - | - | 3.3812 | 3.7171 | 3.8576 |
- | - | 0.5 | π/6 | - | - | - | 3.3218 | 3.6617 | 3.8038 |
- | - | - | π/3 | - | - | - | 3.3849 | 3.7206 | 3.8610 |
- | - | - | π/2 | - | - | - | 3.4158 | 3.7495 | 3.8891 |
- | - | - | π/4 | 0.8 | - | - | 3.4975 | 3.8260 | 3.9637 |
- | - | - | - | 1.8 | - | - | 3.9228 | 4.2294 | 4.3585 |
- | - | - | - | 2.8 | - | - | 4.2910 | 4.5831 | 4.7062 |
- | - | - | - | 0.5 | 0.6 | - | 3.3779 | 3.7138 | 3.8543 |
- | - | - | - | - | 0.8 | - | 3.4259 | 3.7581 | 3.8973 |
- | - | - | - | - | 1.0 | - | 3.4732 | 3.8019 | 3.9397 |
- | - | - | - | - | 0.5 | -0.9 | 3.2274 | 3.5372 | 3.6665 |
- | - | - | - | - | - | -0.7 | 2.9848 | 3.2413 | 3.3479 |
- | - | - | - | - | - | -0.5 | 2.7560 | 2.9634 | 3.0491 |
γ | β | M | ψ | Ec | Bi | Nux/√Rex Fe3O4−CH3OH Cu−CH3OH Ag−CH3OH |
||
0.1 | 1.0 | 0.5 | π/4 | 0.5 | 0.3 | 0.2160 | 0.2084 | 0.2028 |
0.2 | - | - | - | - | - | 0.2056 | 0.1980 | 0.1920 |
0.3 | - | - | - | - | - | 0.1950 | 0.1872 | 0.1810 |
0.1 | 1.2 | - | - | - | - | 0.2279 | 0.2217 | 0.2169 |
- | 1.4 | - | - | - | - | 0.2357 | 0.2303 | 0.2262 |
- | 1.6 | - | - | - | - | 0.2412 | 0.2364 | 0.2326 |
- | 1.0 | 0.1 | - | - | - | 0.2174 | 0.2097 | 0.2040 |
- | - | 0.3 | - | - | - | 0.2169 | 0.2093 | 0.2036 |
- | - | 0.6 | - | - | - | 0.2153 | 0.2078 | 0.2022 |
- | - | 0.5 | π/6 | - | - | 0.2167 | 0.2091 | 0.2034 |
- | - | - | π/3 | - | - | 0.2152 | 0.2078 | 0.2021 |
- | - | - | π/2 | - | - | 0.2145 | 0.2071 | 0.2015 |
- | - | - | π/4 | 0.1 | - | 0.2748 | 0.2734 | 0.2721 |
- | - | - | - | 0.2 | - | 0.2601 | 0.2571 | 0.2547 |
- | - | - | - | 0.3 | - | 0.2454 | 0.2409 | 0.2374 |
- | - | - | - | 0.5 | 0.2 | 0.1457 | 0.1406 | 0.1368 |
- | - | - | - | - | 0.4 | 0.2846 | 0.2747 | 0.2672 |
- | - | - | - | - | 0.6 | 0.4173 | 0.4029 | 0.3917 |
γ | β | M | Sc | ˆσ | Ea | Bj | Shx/√Rex Fe3O4−CH3OH Cu−CH3OH Ag−CH3OH |
||
0.1 | 1.0 | 0.5 | 1.0 | 1.0 | 0.5 | 0.3 | 0.2530 | 0.2529 | 0.2529 |
0.2 | - | - | - | - | - | - | 0.2537 | 0.2536 | 0.2536 |
0.3 | - | - | - | - | - | - | 0.2544 | 0.2544 | 0.2543 |
0.1 | 1.2 | - | - | - | - | - | 0.2532 | 0.2530 | 0.2530 |
- | 1.4 | - | - | - | - | - | 0.2532 | 0.2531 | 0.2531 |
- | 1.6 | - | - | - | - | - | 0.2533 | 0.2532 | 0.2531 |
- | 1.0 | 0.1 | - | - | - | - | 0.2531 | 0.2530 | 0.2529 |
- | - | 0.3 | - | - | - | - | 0.2531 | 0.2530 | 0.2529 |
- | - | 0.6 | - | - | - | - | 0.2530 | 0.2529 | 0.2529 |
- | - | 0.5 | 0.3 | - | - | - | 0.2096 | 0.2094 | 0.2094 |
- | - | - | 0.6 | - | - | - | 0.2362 | 0.2361 | 0.2360 |
- | - | - | 0.9 | - | - | - | 0.2498 | 0.2497 | 0.2497 |
- | - | - | 1.0 | 1.5 | - | - | 0.2565 | 0.2565 | 0.2565 |
- | - | - | - | 2.0 | - | - | 0.2592 | 0.2592 | 0.2592 |
- | - | - | - | 2.5 | - | - | 0.2613 | 0.2614 | 0.2614 |
- | - | - | - | 1.0 | 0.6 | - | 0.2523 | 0.2521 | 0.2521 |
- | - | - | - | - | 0.7 | - | 0.2515 | 0.2514 | 0.2513 |
- | - | - | - | - | 0.8 | - | 0.2508 | 0.2506 | 0.2506 |
- | - | - | - | - | 0.5 | 0.2 | 0.1780 | 0.1779 | 0.1779 |
- | - | - | - | - | - | 0.4 | 0.3207 | 0.3205 | 0.3205 |
- | - | - | - | - | - | 0.6 | 0.4376 | 0.4373 | 0.4372 |
γ | β | M | Sb | Pe | Ω | Dnx/√Rex Fe3O4−CH3OH Cu−CH3OH Ag−CH3OH |
||
0.1 | 1.0 | 0.5 | 0.1 | 0.2 | 0.2 | 0.2957 | 0.2943 | 0.2938 |
0.2 | - | - | - | - | - | 0.3591 | 0.3580 | 0.3576 |
0.3 | - | - | - | - | - | 0.4171 | 0.4162 | 0.4158 |
0.1 | 1.2 | - | - | - | - | 0.2970 | 0.2955 | 0.2949 |
- | 1.4 | - | - | - | - | 0.2980 | 0.2964 | 0.2958 |
- | 1.6 | - | - | - | - | 0.2989 | 0.2972 | 0.2966 |
- | 1.0 | 0.1 | - | - | - | 0.2959 | 0.2945 | 0.2939 |
- | - | 0.3 | - | - | - | 0.2959 | 0.2944 | 0.2939 |
- | - | 0.6 | - | - | - | 0.2956 | 0.2942 | 0.2937 |
- | - | 0.5 | 0.5 | - | - | 0.6931 | 0.6843 | 0.6810 |
- | - | - | 1.5 | - | - | 1.8345 | 1.8169 | 1.8102 |
- | - | - | 2.5 | - | - | 2.9361 | 2.9162 | 2.9084 |
- | - | - | 0.1 | 0.1 | - | 0.2647 | 0.2632 | 0.2627 |
- | - | - | - | 0.3 | - | 0.3268 | 0.3253 | 0.3248 |
- | - | - | - | 0.5 | - | 0.3888 | 0.3874 | 0.3869 |
- | - | - | - | 0.2 | 0.4 | 0.3061 | 0.3047 | 0.3042 |
- | - | - | - | - | 0.8 | 0.3269 | 0.3254 | 0.3249 |
- | - | - | - | - | 1.2 | 0.3476 | 0.3462 | 0.3457 |
Models | Nanoparticles | Input | Output | ||||||
Model-Ⅰ | Fe3O4−CH3OH | γ | β | M | ψ | P0 | Df | α∗ | SFC |
Model-Ⅱ | Cu−CH3OH | γ | β | M | ψ | P0 | Df | α∗ | SFC |
Model-Ⅲ | Ag−CH3OH | γ | β | M | ψ | P0 | Df | α∗ | SFC |
Samples | Model-Ⅰ | Model-Ⅱ | Model-Ⅲ |
Training data | 48 | 48 | 48 |
Validation data | 11 | 11 | 11 |
Testing data | 11 | 11 | 11 |
Total | 70 | 70 | 70 |
γ | SFC (0.5Cfx√Rex) Fe3O4−CH3OH Cu−CH3OH Ag−CH3OH |
||
0.1 | -3.3536 | -3.6914 | -3.8326 |
0.2 | -3.4812 | -3.8187 | -3.9597 |
0.3 | -3.6085 | -3.9458 | -4.0867 |
0.4 | -3.7355 | -4.0726 | -4.2135 |
0.5 | -3.8622 | -4.1993 | -4.3401 |
0.6 | -3.9886 | -4.3257 | -4.4665 |
0.7 | -4.1148 | -4.4519 | -4.5927 |
0.8 | -4.2407 | -4.5779 | -4.7187 |
0.9 | -4.3664 | -4.7037 | -4.8445 |
1 | -4.4920 | -4.8294 | -4.9702 |
β | SFC (0.5Cfx√Rex) Fe3O4−CH3OH Cu−CH3OH Ag−CH3OH |
||
5.1 | -3.3335 | -3.6691 | -3.8094 |
5.2 | -3.3143 | -3.6478 | -3.7872 |
5.3 | -3.2958 | -3.6273 | -3.7659 |
5.4 | -3.2781 | -3.6077 | -3.7455 |
5.5 | -3.2611 | -3.5888 | -3.7259 |
5.6 | -3.2447 | -3.5707 | -3.7070 |
5.7 | -3.2289 | -3.5532 | -3.6888 |
5.8 | -3.2137 | -3.5364 | -3.6713 |
5.9 | -3.1991 | -3.5202 | -3.6544 |
6 | -3.1850 | -3.5045 | -3.6381 |
M | SFC (0.5Cfx√Rex) Fe3O4−CH3OH Cu−CH3OH Ag−CH3OH |
||
0.1 | -3.2921 | -3.6341 | -3.7770 |
0.2 | -3.2999 | -3.6414 | -3.7840 |
0.3 | -3.3128 | -3.6534 | -3.7957 |
0.4 | -3.3307 | -3.6701 | -3.8119 |
0.5 | -3.3536 | -3.6914 | -3.8326 |
0.6 | -3.3812 | -3.7171 | -3.8576 |
0.7 | -3.4133 | -3.7472 | -3.8869 |
0.8 | -3.4499 | -3.7814 | -3.9202 |
0.9 | -3.4906 | -3.8196 | -3.9574 |
1 | -3.5352 | -3.8616 | -3.9984 |
ψ | SFC (0.5Cfx√Rex) Fe3O4−CH3OH Cu−CH3OH Ag−CH3OH |
||
π/6 | -3.3218 | -3.6617 | -3.8038 |
π/4 | -3.3536 | -3.6914 | -3.8326 |
π/3 | -3.3849 | -3.7206 | -3.8610 |
π/2 | -3.4158 | -3.7495 | -3.8891 |
2π/3 | -3.3849 | -3.7206 | -3.8610 |
3π/4 | -3.3536 | -3.6914 | -3.8326 |
5π/6 | -3.3218 | -3.6617 | -3.8038 |
π | -3.2895 | -3.6317 | -3.7746 |
7π/6 | -3.3218 | -3.6617 | -3.3218 |
5π/4 | -3.3536 | -3.6914 | -3.3536 |
P0 | SFC (0.5Cfx√Rex) Fe3O4−CH3OH Cu−CH3OH Ag−CH3OH |
||
0.1 | -3.1449 | -3.4982 | -3.6452 |
0.2 | -3.1992 | -3.5481 | -3.6936 |
0.3 | -3.2520 | -3.5969 | -3.7409 |
0.4 | -3.3034 | -3.6447 | -3.7872 |
0.5 | -3.3536 | -3.6914 | -3.8326 |
0.6 | -3.4026 | -3.7371 | -3.8771 |
0.7 | -3.4505 | -3.7820 | -3.4505 |
0.8 | -3.4975 | -3.8260 | -3.4975 |
0.9 | -3.5434 | -3.8693 | -4.0060 |
1 | -3.5885 | -3.9118 | -4.0475 |
Df | SFC (0.5Cfx√Rex) Fe3O4−CH3OH Cu−CH3OH Ag−CH3OH |
||
0.1 | -3.2543 | -3.6001 | -3.7443 |
0.2 | -3.2794 | -3.6232 | -3.7666 |
0.3 | -3.3043 | -3.6461 | -3.7887 |
0.4 | -3.3291 | -3.6688 | -3.8107 |
0.5 | -3.3536 | -3.6914 | -3.8326 |
0.6 | -3.3779 | -3.7138 | -3.8543 |
0.7 | -3.4020 | -3.7360 | -3.8758 |
0.8 | -3.4259 | -3.7581 | -3.8973 |
0.9 | -3.4496 | -3.7801 | -3.9186 |
1.0 | -3.9397 | -3.8019 | -3.9397 |
α∗ | SFC (0.5Cfx√Rex) Fe3O4−CH3OH Cu−CH3OH Ag−CH3OH |
||
0.1 | -2.1593 | -2.2486 | -2.2844 |
0.2 | -2.0733 | -2.1473 | -2.1769 |
0.3 | -1.9909 | -2.0511 | -2.0750 |
0.4 | -1.9123 | -1.9598 | -1.9785 |
0.5 | -1.8374 | -1.8734 | -1.8874 |
0.6 | -1.7659 | -1.7916 | -1.8015 |
0.7 | -1.6980 | -1.7143 | -1.7205 |
0.8 | -1.6333 | -1.6413 | -1.6443 |
0.9 | -1.5718 | -1.5724 | -1.5725 |
1.0 | -1.5134 | -1.5074 | -1.5051 |
Models | Nanoparticles | MSE | R | ||||
Training | Validation | Test | Training | Validation | Test | ||
ANN Model-Ⅰ | Fe3O4−CH3OH | 0.0021 | 0.0041 | 0.0101 | 0.9970 | 0.9964 | 0.9936 |
ANN Model-Ⅱ | Cu−CH3OH | 0.0037 | 0.0136 | 0.0049 | 0.9987 | 0.9943 | 0.9998 |
ANN Model-Ⅲ | Ag−CH3OH | 0.0022 | 0.01245 | 0.0075 | 0.9982 | 0.9896 | 0.9960 |