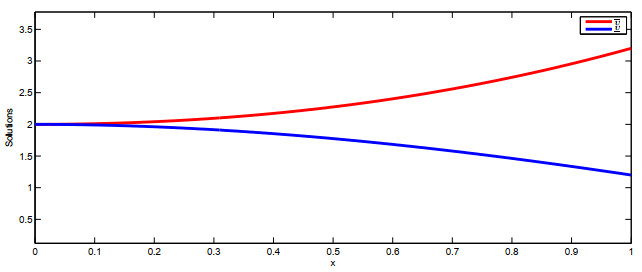
Citation: Ghazanfar Latif, Jaafar Alghazo, Majid Ali Khan, Ghassen Ben Brahim, Khaled Fawagreh, Nazeeruddin Mohammad. Deep convolutional neural network (CNN) model optimization techniques—Review for medical imaging[J]. AIMS Mathematics, 2024, 9(8): 20539-20571. doi: 10.3934/math.2024998
[1] | Xinyu Lu, Lifang Wang, Zejun Jiang, Shizhong Liu, Jiashi Lin . PEJL: A path-enhanced joint learning approach for knowledge graph completion. AIMS Mathematics, 2023, 8(9): 20966-20988. doi: 10.3934/math.20231067 |
[2] | Wenhui Feng, Xingfa Zhang, Yanshan Chen, Zefang Song . Linear regression estimation using intraday high frequency data. AIMS Mathematics, 2023, 8(6): 13123-13133. doi: 10.3934/math.2023662 |
[3] | Yan Wang, Ying Cao, Ziling Heng, Weiqiong Wang . Linear complexity and 2-adic complexity of binary interleaved sequences with optimal autocorrelation magnitude. AIMS Mathematics, 2022, 7(8): 13790-13802. doi: 10.3934/math.2022760 |
[4] | Rinko Miyazaki, Dohan Kim, Jong Son Shin . Uniform boundedness of solutions to linear difference equations with periodic forcing functions. AIMS Mathematics, 2023, 8(10): 24116-24131. doi: 10.3934/math.20231229 |
[5] | Gideon Simpson, Daniel Watkins . Relative entropy minimization over Hilbert spaces via Robbins-Monro. AIMS Mathematics, 2019, 4(3): 359-383. doi: 10.3934/math.2019.3.359 |
[6] | C. T. J. Dodson . Information distance estimation between mixtures of multivariate Gaussians. AIMS Mathematics, 2018, 3(4): 439-447. doi: 10.3934/Math.2018.4.439 |
[7] | Rashad M. Asharabi, Somaia M. Alhazmi . Accelerating the convergence of a two-dimensional periodic nonuniform sampling series through the incorporation of a bivariate Gaussian multiplier. AIMS Mathematics, 2024, 9(11): 30898-30921. doi: 10.3934/math.20241491 |
[8] | Zhengyan Luo, Lintao Ma, Yinghui Zhang . Optimal decay rates of higher–order derivatives of solutions for the compressible nematic liquid crystal flows in R3. AIMS Mathematics, 2022, 7(4): 6234-6258. doi: 10.3934/math.2022347 |
[9] | Xinyu Guan, Nan Kang . Stability for Cauchy problem of first order linear PDEs on Tm with forced frequency possessing finite uniform Diophantine exponent. AIMS Mathematics, 2024, 9(7): 17795-17826. doi: 10.3934/math.2024866 |
[10] | Myeongmin Kang, Miyoun Jung . Nonconvex fractional order total variation based image denoising model under mixed stripe and Gaussian noise. AIMS Mathematics, 2024, 9(8): 21094-21124. doi: 10.3934/math.20241025 |
Fractional differential equations (FDEs) have a profound physical background and rich theoretical connotations and have been particularly eye-catching in recent years. Fractional order differential equations refer to equations that contain fractional derivatives or integrals. Currently, fractional derivatives and integrals have a wide range of applications in many disciplines such as physics, biology, and chemistry, etc. For more information see [1,2,3,45].
Langevin equation is an important tool of many areas such as mathematical physics, protein dynamics [6], deuteron-cluster dynamics, and described anomalous diffusion [7]. In 1908, Langevin established first the Langevin equation with a view to describe the advancement of physical phenomena in fluctuating conditions [8]. Some evolution processes are characterized by the fact that they change of state abruptly at certain moments of time. These perturbations are short-term in comparison with the duration of the processes. So, the Langevin equations are a suitable tool to describe such problems. Besides the intensive improvement of fractional derivatives, the Langevin (FDEs) have been presented in 1990 by Mainardi and Pironi [9], which was trailed by numerous works interested in some properties of solutions like existence and uniqueness for Langevin FDEs [10,11,12,13,14,15,16,17,18,19]. We also refer here to some recent works that deal with a qualitative analysis of such problems, including the generalized Hilfer operator, see [20,21,22,23,24]. Recent works related to our work were done by [25,26,27,28,29,30]. The monotone iterative technique is one of the important techniques used to obtain explicit solutions for some differential equations. For more details about the monotone iterative technique, we refer the reader to the classical monographs [31,32].
Lakshmikantham and Vatsala [25] studied the general existence and uniqueness results for the following FDE
{Dμ0+(υ(ϰ)−υ(0))=f(ϰ,υ(ϰ)),ϰ∈[0,b],υ(0)=υ0, |
by the monotone iterative technique and comparison principle. Fazli et al. [26] investigated the existence of extremal solutions of a nonlinear Langevin FDE described as follows
{Dμ10+(Dμ20++λ)υ(ϰ)=f(ϰ,υ(ϰ)),ϰ∈[0,b],g(υ(0),υ(b))=0,Dμ20+υ(0)=υμ2, |
via a constructive technique that produces monotone sequences that converge to the extremal solutions. Wang et al. [27], used the monotone iterative method to prove the existence of extremal solutions for the following nonlinear Langevin FDE
{βDμ0+(γDμ0++λ)υ(ϰ)=f(ϰ,υ(ϰ),(γDμ0++λ)),ϰ∈(0,b],ϰμ(1−γ)υ(0)=τ1∫η0υ(s)ds+m∑i=1μiυ(σi),ϰμ(1−β)(γDμ0++λ)υ(0)=τ2∫η0 γDμ0+υ(s)ds+∑mi=1ργiDμ0+υ(σi), |
Motivated by the novel advancements of the Langevin equation and its applications, also by the above argumentations, in this work, we apply the monotone iterative method to investigate the lower and upper explicit monotone iterative sequences that converge to the extremal solution of a fractional Langevin equation (FLE) with multi-point sub-strip boundary conditions described by
{(HDμ1,β1;ϕ0++λ1)(HDμ2,β2;ϕ0++λ2)υ(ϰ)=f(ϰ,υ(ϰ)),ϰ∈(0,b],HDμ2,β2;ϕ0+υ(ϰ)|ϰ=0=0,υ(0)=0,υ(b)=∑mi=1δiIσi,ϕ0+υ(ζi), | (1.1) |
where HDμ1,β1;ϕ0+ and HDμ2,β2;ϕ0+ are the ϕ-Hilfer fractional derivatives of order μ1∈(0,1] and μ2∈(1,2] respectively, and type β1,β2∈[0,1],σi>0,λ1,λ2∈R+, δi>0, m≥1, 0<ζ1<ζ2<......<1, f:(0,b]×R→R is a given continuous function and ϕ is an increasing function, having a continuous derivative ϕ′ on (0,b) such that ϕ′(ϰ)≠0, for all ϰ∈(0,b]. Our main contributions to this work are as follows:
∙ By adopting the same techniques used in [26,27], we derive the formula of explicit solutions for ϕ-Hilfer-FLEs (1.1) involving two parameters Mittag-Leffler functions.
∙ We use the monotone iterative method to study the extremal of solutions of ϕ-Hilfer-FLE (1.1).
∙ We investigate the lower and upper explicit monotone iterative sequences that converge to the extremal solution.
∙ The proposed problem (1.1) covers some problems involving many classical fractional derivative operators, for different values of function ϕ and parameter μi,i=1,2. For instance:
∙ If ϕ(ϰ)=ϰ and μi=1, then the FLE (1.1) reduces to Caputo-type FLE.
∙ If ϕ(ϰ)=ϰ and μi=0, then the FLE (1.1) reduces to Riemann-Liouville-type FLE.
∙ If μi=0, then the FLE (1.1) reduces to FLE with the ϕ-Riemann-Liouville fractional derivative.
∙ If ϕ(ϰ)=ϰ, then the FLE (1.1) reduces to classical Hilfer-type FLE.
∙ If ϕ(ϰ)=logϰ, then the FLE (1.1) reduces to Hilfer-Hadamard-type FLE.
∙ If ϕ(ϰ)=ϰρ, then the FLE (1.1) reduces to Katugampola-type FLE.
∙ The results obtained in this work includes the results of Fazli et al. [26], Wang et al. [27] and cover many problems which do not study yet.
The structure of our paper is as follows: In the second section, we present some notations, auxiliary lemmas and some basic definitions which are used throughout the paper. Moreover, we derive the formula of the explicit solution for FLE (1.1) in the term of Mittag-Leffler with two parameters. In the third section, we discuss the existence of extremal solutions to our FLE (1.1) and prove lower and upper explicit monotone iterative sequences which converge to the extremal solution. In the fourth section, we provide a numerical example to illustrate the validity of our results. The concluding remarks will be given in the last section.
To achieve our main purpose, we present here some definitions and basic auxiliary results that are required throughout our paper. Let J:=[0,b], and C(J) be the Banach space of continuous functions υ:J→R equipped with the norm ‖υ‖=sup{|υ(ϰ)|:ϰ∈J}.
Definition 2.1. [2] Let f be an integrable function and μ>0. Also, let ϕ be an increasing and positive monotone function on (0,b), having a continuous derivative ϕ′ on (0,b) such that ϕ′(ϰ)≠0, for all ϰ∈J. Then the ϕ-Riemann-Liouville fractional integral of f of order μ is defined by
Iμ,ϕ0+f(ϰ)=∫ϰ0ϕ′(s)(ϕ(ϰ)−ϕ(s))μ−1Γ(μ)f(s)ds, 0<ϰ≤b. |
Definition 2.2. [33] Let n−1<μ<n, (n∈N), and f,ϕ∈Cn(J) such that ϕ′(ϰ) is continuous and satisfying ϕ′(ϰ)≠0 for all ϰ∈J. Then the left-sided ϕ-Hilfer fractional derivative of a function f of order μ and type β∈[0,1] is defined by
HDμ,β,ϕ0+f(ϰ)=Iβ(n−μ);ϕ0+Dγ;ϕa+f(ϰ),γ=μ+nβ−μβ, |
where
Dγ;ϕ0+f(ϰ)=f[n]ϕI(1−β)(n−μ);ϕ0+f(ϰ),andf[n]ϕ=[1ϕ′(ϰ)ddϰ]n. |
Lemma 2.3. [2,33] Let n−1<μ<n, 0≤β≤1, and n<δ∈R. For a given function f:J→R, we have
Iμ,ϕ0+Iβ,ϕ0+f(ϰ)=Iμ+β,ϕ0+f(ϰ), |
Iμ,ϕ0+(ϕ(ϰ)−ϕ(0))δ−1=Γ(δ)Γ(μ+δ)(ϕ(ϰ)−ϕ(0))μ+δ−1, |
and
HDμ,β,ϕ0+(ϕ(ϰ)−ϕ(0))δ−1=0,δ<n. |
Lemma 2.4. [33] Let f:J→R, n−1<μ<n, and 0≤β≤1. Then
(1) If f∈Cn−1(J), then
Iμ;ϕ0+HDμ,β,ϕ0+f(ϰ)=f(ϰ)−n−1∑k=1(ϕ(ϰ)−ϕ(0))γ−kΓ(γ−k+1)f[n−k]ϕI(1−β)(n−μ);ϕ0+f(0), |
(2) If f∈C(J), then
HDμ,β,ϕ0+Iμ;ϕ0+f(ϰ)=f(ϰ). |
Lemma 2.5. For μ,β,γ>0 and λ∈R, we have
Iμ,ϕ0+[ϕ(ϰ)−ϕ(0)]β−1Eγ,β[λ(ϕ(ϰ)−ϕ(0))γ]=[ϕ(ϰ)−ϕ(0)]β+μ−1Eγ,β+μ[λ(ϕ(ϰ)−ϕ(0))γ], |
where Eγ,β is Mittag-Leffler function with two-parameterdefined by
Eγ,β(υ)=∞∑i=1υiΓ(γi+β),υ∈C. |
Proof. See [34].
Lemma 2.6. [27] Let μ∈(1,2] and β>0 be arbitrary. Then the functions Eμ(⋅), Eμ,μ(⋅) and Eμ,β(⋅) are nonnegative. Furthermore,
Eμ(χ):=Eμ,1(χ)≤1,Eμ,μ(χ)≤1Γ(μ),Eμ,β(χ)≤1Γ(β), |
for χ<0.
Lemma 2.7. Let μ,k,β>0, λ∈R and f∈C(J). Then
Ik,ϕ0+[Iμ,ϕ0+Eμ,μ(λ(ϕ(ϰ)−ϕ(0))μ)]=Iμ+k,ϕ0+Eμ,μ+k(λ(ϕ(ϰ)−ϕ(0))μ). |
Proof. See [34].
For some analysis techniques, we will suffice with indication to the classical Banach contraction principle (see [35]).
To transform the ϕ-Hilfer type FLE (1.1) into a fixed point problem, we will present the following Lemma.
Lemma 2.8. Let γj=μj+jβj−μjβj, (j=1,2) such that μ1∈(0,1],μ2∈(1,2], βj∈[0,1],λ1,λ2≥0 and ℏ is a functionin the space C(J). Then, υ is a solutionof the ϕ-Hilfer linear FLE of the form
{(HDμ1,β1;ϕ0++λ1)(HDμ2,β2;ϕ0++λ2)v(ϰ)=ℏ(ϰ),ϰ∈(0,b],HDμ2,β2;ϕ0+v(ϰ)|ϰ=0=0,v(0)=0,v(b)=∑mi=1δiIσi,ϕ0+v(ζi), | (2.1) |
if and only if υ satisfies the following equation
υ(ϰ)=[ϕ(ϰ)−ϕ(0)]γ2−1Eμ2,γ2(−λ2[ϕ(ϰ)−ϕ(0)]μ2)Θ[Γ(μ2)Iμ2,ϕ0+Eμ2,μ2(−λ2[ϕ(b)−ϕ(0)]μ2)(Γ(μ1)Iμ1,ϕ0+Eμ1,μ1(−λ1[ϕ(b)−ϕ(0)]μ1)ℏ(b))−m∑i=1δiΓ(μ2)Iμ2+σi,ϕ0+Eμ2,μ2+σi(−λ2[ϕ(ζi)−ϕ(0)]μ2)(Γ(μ1)Iμ1,ϕ0+Eμ1,μ1(−λ1[ϕ(ζi)−ϕ(0)]μ1)ℏ(ζi))]+Γ(μ2)Iμ2,ϕ0+Eμ2,μ2(−λ2[ϕ(ϰ)−ϕ(0)]μ2)Γ(μ1)Iμ1,ϕ0+[Eμ1,μ1(−λ1[ϕ(ϰ)−ϕ(0)]μ1)ℏ(ϰ)]. | (2.2) |
where
Θ:=(∑mi=1δi[ϕ(ζi)−ϕ(0)]γ2+σi−1Eμ2,γ2+σi(−λ2[ϕ(ζi)−ϕ(0)]μ2)−[ϕ(b)−ϕ(0)]γ2−1Eμ2,γ2(−λ2[ϕ(b)−ϕ(0)]μ2))≠0. | (2.3) |
Proof. Let (HDμ2,β2;ϕ0++λ2)υ(ϰ)=P(ϰ). Then, the problem (2.1) is equivalent to the following problem
{(HDμ1,β1;ϕ0++λ1)P(ϰ)=ℏ(ϰ),ϰ∈(0,b],P(0)=0. | (2.4) |
Applying the operator Iμ1,ϕ0+ to both sides of the first equation of (2.4) and using Lemma 2.4, we obtain
P(ϰ)=c0Γ(γ1)[ϕ(ϰ)−ϕ(0)]γ1−1−λ1Iμ1,ϕ0+P(ϰ)+Iμ1,ϕ0+ℏ(ϰ), | (2.5) |
where c0 is an arbitrary constant. For explicit solutions of Eq (2.4), we use the method of successive approximations, that is
P0(ϰ)=c0Γ(γ1)[ϕ(ϰ)−ϕ(0)]γ1−1, | (2.6) |
and
Pk(ϰ)=P0(ϰ)−λ1Iμ1,ϕ0+Pk−1(ϰ)+Iμ1,ϕ0+ℏ(ϰ). | (2.7) |
By Definition 2.1 and Lemma 2.3 along with Eq (2.6), we obtain
P1(ϰ)=P0(ϰ)−λ1Iμ1,ϕ0+P0(ϰ)+Iμ1,ϕ0+ℏ(ϰ)=c0Γ(γ1)[ϕ(ϰ)−ϕ(0)]γ1−1−λ1Iμ1,ϕ0+(c0Γ(γ1)[ϕ(ϰ)−ϕ(0)]γ1−1)+Iμ1,ϕ0+ℏ(ϰ)=c0Γ(γ1)[ϕ(ϰ)−ϕ(0)]γ1−1−λ1c0Γ(γ1+μ1)[ϕ(ϰ)−ϕ(0)]γ1+μ1−1+Iμ1,ϕ0+ℏ(ϰ)=c02∑i=1(−λ1)i−1[ϕ(ϰ)−ϕ(0)]iμ1+β1(1−μ1)−1Γ(iμ1+β1(1−μ1))+Iμ1,ϕ0+ℏ(ϰ). | (2.8) |
Similarly, by using Eqs (2.6)–(2.8), we get
P2(ϰ)=P0(ϰ)−λ1Iμ1,ϕ0+P1(ϰ)+Iμ1,ϕ0+ℏ(ϰ)=c0Γ(γ1)[ϕ(ϰ)−ϕ(0)]γ1−1−λ1Iμ1,ϕ0+(c02∑i=1(−λ1)i−1[ϕ(ϰ)−ϕ(0)]iμ1+β1(1−μ1)−1Γ(iμ1+β1(1−μ1))+Iμ1,ϕ0+ℏ(ϰ))+Iμ1,ϕ0+ℏ(ϰ)=c03∑i=1(−λ1)i−1[ϕ(ϰ)−ϕ(0)]iμ1+β1(1−μ1)−1Γ(iμ1+β1(1−μ1))+2∑i=1(−λ1)i−1Iiμ1,ϕ0+ℏ(ϰ). |
Repeating this process, we get Pk(ϰ) as
Pk(ϰ)=c0k+1∑i=1(−λ1)i−1[ϕ(ϰ)−ϕ(0)]iμ1+β1(1−μ1)−1Γ(iμ1+β1(1−μ1))+k∑i=1(−λ1)i−1Iiμ1,ϕ0+ℏ(ϰ). |
Taking the limit k→∞, we obtain the expression for Pk(ϰ), that is
P(ϰ)=c0∞∑i=1(−λ1)i−1[ϕ(ϰ)−ϕ(0)]iμ1+β1(1−μ1)−1Γ(iμ1+β1(1−μ1))+∞∑i=1(−λ1)i−1Iiμ1,ϕ0+ℏ(ϰ). |
Changing the summation index in the last expression, i→i+1, we have
P(ϰ)=c0∞∑i=0(−λ1)i[ϕ(ϰ)−ϕ(0)]iμ1+γ1−1Γ(iμ1+γ1)+∞∑i=0(−λ1)iIiμ1+μ1,ϕ0+ℏ(ϰ). |
From the definition of Mittag-Leffler function, we get
P(ϰ)=c0[ϕ(ϰ)−ϕ(0)]γ1−1Eμ1,γ1(−λ1[ϕ(ϰ)−ϕ(0)]μ1)+Γ(μ1)Iμ1,ϕ0+Eμ1,μ1(−λ1[ϕ(ϰ)−ϕ(0)]μ1)ℏ(ϰ). | (2.9) |
By the condition P(0)=0, we get c0=0 and hence
Equation (2.9) reduces to
P(ϰ)=Γ(μ1)Iμ1,ϕ0+Eμ1,μ1(−λ1[ϕ(ϰ)−ϕ(0)]μ1)ℏ(ϰ). | (2.10a) |
Similarly, the following equation
{(HDμ2,β2;ϕ0++λ2)υ(ϰ)=P(ϰ),ϰ∈(0,b],υ(0)=0,υ(b)=∑mi=1δiIσi,ϕ0+υ(ζi) |
is equivalent to
υ(ϰ)=c1[ϕ(ϰ)−ϕ(0)]γ2−1Eμ2,γ2(−λ2[ϕ(ϰ)−ϕ(0)]μ2)+c2[ϕ(ϰ)−ϕ(0)]γ2−2Eμ2,γ2−1(−λ2[ϕ(ϰ)−ϕ(0)]μ2)+Γ(μ2)Iμ2,ϕ0+Eμ2,μ2(−λ2[ϕ(ϰ)−ϕ(0)]μ2)P(ϰ). | (2.11) |
By the condition υ(0)=0, we obtain c2=0 and hence Eq (2.11) reduces to
υ(ϰ)=c1[ϕ(ϰ)−ϕ(0)]γ2−1Eμ2,γ2(−λ2[ϕ(ϰ)−ϕ(0)]μ2)+Γ(μ2)Iμ2,ϕ0+Eμ2,μ2(−λ2[ϕ(ϰ)−ϕ(0)]μ2)P(ϰ). | (2.12) |
By the condition υ(b)=∑mi=1δi Iσi,ϕ0+υ(ζi), we get
c1=1Θ(Γ(μ2)Iμ2,ϕ0+Eμ2,μ2(−λ2[ϕ(b)−ϕ(0)]μ2)P(b)−∑mi=1δiΓ(μ2)Iμ2+σi,ϕ0+Eμ2,μ2+σi(−λ2[ϕ(ζi)−ϕ(0)]μ2)P(ζi)). | (2.13) |
Put c0 in Eq (2.12), we obtain
υ(ϰ)=[ϕ(ϰ)−ϕ(0)]γ2−1Eμ2,γ2(−λ2[ϕ(ϰ)−ϕ(0)]μ2)Θ[Γ(μ2)Iμ2,ϕ0+Eμ2,μ2(−λ2[ϕ(b)−ϕ(0)]μ2)P(b)−m∑i=1δiΓ(μ2)Iμ2+σi,ϕ0+Eμ2,μ2+σi(−λ2[ϕ(ζi)−ϕ(0)]μ2)P(ζi)]+Γ(μ2)Iμ2,ϕ0+[Eμ2,μ2(−λ2[ϕ(ϰ)−ϕ(0)]μ2)P(ϰ)]. | (2.14) |
Substituting Eq (2.10a) into Eq (2.14), we can get Eq (2.2).
On the other hand, we assume that the solution υ satisfies Eq (2.2). Then, one can get υ(0)=0. Applying HDμ2,β2;ϕ0+ on both sides of Eq (2.2), we get
HDμ2,β2;ϕ0+υ(ϰ)=HDμ2,β2;ϕ0+[ϕ(ϰ)−ϕ(0)]γ2−1Eμ2,γ2(−λ2[ϕ(ϰ)−ϕ(0)]μ2)Θ[Γ(μ2)Iμ2,ϕ0+Eμ2,μ2(−λ2[ϕ(b)−ϕ(0)]μ2)(Γ(μ1)Iμ1,ϕ0+Eμ1,μ1(−λ1[ϕ(b)−ϕ(0)]μ1)ℏ(b))−m∑i=1δiΓ(μ2)Iμ2+σi,ϕ0+Eμ2,μ2+σi(−λ2[ϕ(ζi)−ϕ(0)]μ2)(Γ(μ1)Iμ1,ϕ0+Eμ1,μ1(−λ1[ϕ(ζi)−ϕ(0)]μ1)ℏ(ζi))]+HDμ2,β2;ϕ0+Γ(μ2)Iμ2,ϕ0+Eμ2,μ2(−λ2[ϕ(ϰ)−ϕ(0)]μ2)Γ(μ1)Iμ1,ϕ0+[Eμ1,μ1(−λ1[ϕ(ϰ)−ϕ(0)]μ1)ℏ(ϰ)]. | (2.15) |
Since γ2=μ2+β2−μ2β2, then, by Lemma 2.3, we have HDμ2,β2;ϕ0+[ϕ(ϰ)−ϕ(0)]γ2−1=0 and hence Eq (2.15) reduces to the following equation
HDμ2,β2;ϕ0+υ(ϰ)=HDμ2,β2;ϕ0+Γ(μ2)Iμ2,ϕ0+Eμ2,μ2(−λ2[ϕ(ϰ)−ϕ(0)]μ2)Γ(μ1)Iμ1,ϕ0+[Eμ1,μ1(−λ1[ϕ(ϰ)−ϕ(0)]μ1)ℏ(ϰ)]. |
By using some properties of Mittag-Leffler function and taking ϰ=0, we obtain
HDμ2,β2;ϕ0+υ(0)=0. |
Thus, the derivative condition is satisfied. The proof of Lemma 2.8 is completed.
Lemma 2.9. (Comparison Theorem). For j=1,2, let γj=μj+jβj−μjβj, μ1∈(0,1],μ2∈(1,2], βj∈[0,1],λ1≥0 and υ∈C(J) be acontinuous function satisfies
{(HDμ1,β1;ϕ0++λ1)(HDμ2,β2;ϕ0++λ2)v(ϰ)≥0,HDμ2,β2;ϕ0+v(ϰ)|ϰ=0≥0,v(0)≥0,v(b)≥0, |
then υ(ϰ)≥0, ϰ∈(0,b].
Proof. If z≥0, then from Lemma 2.6, we have Eμ,β(z)≥0. If z<0, then Eμ,β(z) is completely monotonic function [35], that means Eμ,β(z) possesses derivatives for all arbitrary integer order and (−1)ndndznEμ,β(z)≥0. Hence, Eμ,β(z)≥0 for all z∈R. In view of Eq (2.2), Eq (2.9), and from fact that Eμ1,γ1(⋅)≥0 and Eμ,μ(⋅)≥0 with help the definition of ϕ, we obtain υ(ϰ)≥0, for ϰ∈(0,b]. (Alternative proof). Let (HDμ2,β2;ϕ0++λ2)υ(ϰ)=P(ϰ). Then, we have
{(HDμ1,β1;ϕ0++λ1)P(ϰ)≥0,P(0)≥0. |
Assume that P(ϰ)≥0 (for all ϰ∈(0,b]) is not true. Then, there exist ϰ1,ϰ2, (0<ϰ1<ϰ2≤b) such that P(ϰ2)<0,P(ϰ1)=0 and
{P(ϰ)≥0,ϰ∈(0,ϰ1),P(ϰ)<0,ϰ∈(ϰ1,ϰ2). |
Since λ1≥0, we have (HDμ1,β1;ϕ0++λ1)P(ϰ)≥0 for all ϰ∈(ϰ1,ϰ2). In view of
HDμ1,β1,ϕ0+P(ϰ)=Iβ1(1−μ1);ϕ0+(1ϕ′(ϰ)ddϰ)I1−γ1;ϕ0+P(ϰ), |
the operator I1−γ1;ϕ0+P(ϰ) is nondecreasing on (ϰ1,ϰ2). Hence
I1−γ1;ϕ0+P(ϰ)−I1−γ1;ϕ0+P(ϰ1)≥0,ϰ∈(ϰ1,ϰ2). |
On the other hand, for all ϰ∈(ϰ1,ϰ2), we have
I1−γ1;ϕ0+P(ϰ)−I1−γ1;ϕ0+P(ϰ1)=1Γ(1−γ1)∫ϰ0ϕ′(s)(ϕ(ϰ)−ϕ(s))1−γ1−1P(s)ds−1Γ(1−γ1)∫ϰ10ϕ′(s)(ϕ(ϰ1)−ϕ(s))1−γ1−1P(s)ds=1Γ(1−γ1)∫ϰ10ϕ′(s)[(ϕ(ϰ)−ϕ(s))−γ1−(ϕ(ϰ1)−ϕ(s))−γ1]P(s)ds+1Γ(1−γ1)∫ϰϰ1ϕ′(s)(ϕ(ϰ)−ϕ(s))−γ1P(s)ds<0, for all ϰ∈(ϰ1,ϰ2), |
which is a contradiction. Therefore, P(ϰ)≥0 (ϰ∈(0,b]). By the same technique, one can prove that υ(ϰ)≥0, for all ϰ∈(0,b].
As a result of Lemma 2.8, we have the following Lemma.
Lemma 2.10. For j=1,2, let γj=μj+jβj−μjβj, μ1∈(0,1],μ2∈(1,2], βj∈[0,1] and f:J×R→R is continuous function . If υ∈C(J) satisfies the problem (1.1), then, υ satisfies thefollowing integral equation
υ(ϰ)=[ϕ(ϰ)−ϕ(0)]γ2−1Eμ2,γ2(−λ2[ϕ(ϰ)−ϕ(0)]μ2)Θ[Γ(μ2)Iμ2,ϕ0+Eμ2,μ2(−λ2[ϕ(b)−ϕ(0)]μ2)(Γ(μ1)Iμ1,ϕ0+Eμ1,μ1(−λ1[ϕ(b)−ϕ(0)]μ1)f(b,υ(b)))−m∑i=1δiΓ(μ2)Iμ2+σi,ϕ0+Eμ2,μ2+σi(−λ2[ϕ(ζi)−ϕ(0)]μ2)(Γ(μ1)Iμ1,ϕ0+Eμ1,μ1(−λ1[ϕ(ζi)−ϕ(0)]μ1)f(ζi,υ(ζi)))]+Γ(μ2)Iμ2,ϕ0+Eμ2,μ2(−λ2[ϕ(ϰ)−ϕ(0)]μ2)(Γ(μ1)Iμ1,ϕ0+Eμ1,μ1(−λ1[ϕ(ϰ)−ϕ(0)]μ1)f(ϰ,υ(ϰ))). |
In this part, we focus on the existence of lower and upper explicit monotone iterative sequences that converge to the extremal solution for the nonlinear ϕ-Hilfer FLE (1.1). The existence of unique solution for the problem (1.1) is based on Banach fixed point theorem. Now, let us give the following definitions:
Definition 3.1. For J= [0,b]⊂R+. Let υ∈C(J). Then, the upper and lower-control functions are defined by
¯f(ϰ,υ(ϰ))=sup0≤Y≤υ{f(ϰ,Y(ϰ))}, |
and
f_(ϰ,υ(ϰ))=infυ≤Y≤b{f(ϰ,Y(ϰ))}, |
respectively. Clearly, ¯f(ϰ,υ(ϰ)) and f_(ϰ,υ(ϰ)) are monotonous non-decreasing on [a,b] and
f_(ϰ,υ(ϰ))≤f(ϰ,υ(ϰ))≤¯f(ϰ,υ(ϰ)) |
Definition 3.2. Let ¯υ, υ_ ∈C(J) be upper and lower solutions of the problem (1.1) respectively. Then
{(HDμ1,β1;ϕ0++λ1)(HDμ2,β2;ϕ0++λ2)¯υ(ϰ)≥¯f(ϰ,¯υ(ϰ)),ϰ∈(0,b],HDμ2,β2;ϕ0+¯υ(ϰ)|ϰ=0≥0,¯υ(0)≥0,¯υ(b)≥∑mi=1δiIσi,ϕ0+¯υ(ζi), |
and
{(HDμ1,β1;ϕ0++λ1)(HDμ2,β2;ϕ0++λ2)υ_(ϰ)≤f_(ϰ,υ_(ϰ)),ϰ∈(0,b],HDμ2,β2;ϕ0+υ_(ϰ)|ϰ=0≤0,υ_(0)≤0,υ_(b)≤∑mi=1δiIσi,ϕ0+υ_(ζi). |
According to Lemma 2.8, we have
¯υ(ϰ)≥[ϕ(ϰ)−ϕ(0)]γ2−1Eμ2,γ2(−λ2[ϕ(ϰ)−ϕ(0)]μ2)Θ[Γ(μ2)Iμ2,ϕ0+Eμ2,μ2(−λ2[ϕ(b)−ϕ(0)]μ2)(Γ(μ1)Iμ1,ϕ0+Eμ1,μ1(−λ1[ϕ(b)−ϕ(0)]μ1)f(b,¯υ(b))−m∑i=1δiΓ(μ2)Iμ2+σi,ϕ0+Eμ2,μ2+σi(λ2[ϕ(ζi)−ϕ(0)]μ2)(Γ(μ1)Iμ1,ϕ0+Eμ1,μ1(−λ1[ϕ(ζi)−ϕ(0)]μ1)f(ζi,¯υ(ζi)))]+Γ(μ2)Iμ2,ϕ0+Eμ2,μ2(−λ2[ϕ(ϰ)−ϕ(0)]μ2)(Γ(μ1)Iμ1,ϕ0+Eμ1,μ1(−λ1[ϕ(ϰ)−ϕ(0)]μ1)f(ϰ,¯υ(ϰ))) |
and
υ_(ϰ)≤[ϕ(ϰ)−ϕ(0)]γ2−1Eμ2,γ2(−λ2[ϕ(ϰ)−ϕ(0)]μ2)Θ[Γ(μ2)Iμ2,ϕ0+Eμ2,μ2(−λ2[ϕ(b)−ϕ(0)]μ2)(Γ(μ1)Iμ1,ϕ0+Eμ1,μ1(−λ1[ϕ(b)−ϕ(0)]μ1)f(b,υ_(b))−m∑i=1δiΓ(μ2)Iμ2+σi,ϕ0+Eμ2,μ2+σi(−λ2[ϕ(ζi)−ϕ(0)]μ2)(Γ(μ1)Iμ1,ϕ0+Eμ1,μ1(−λ1[ϕ(ζi)−ϕ(0)]μ1)f(ζi,υ_(ζi)))]+Γ(μ2)Iμ2,ϕ0+Eμ2,μ2(−λ2[ϕ(ϰ)−ϕ(0)]μ2)(Γ(μ1)Iμ1,ϕ0+Eμ1,μ1(−λ1[ϕ(ϰ)−ϕ(0)]μ1)f(ϰ,υ_(ϰ))). |
Theorem 3.3. Let ¯υ(ϰ) and υ_(ϰ) be upper and lower solutions of the problem (1.1), respectively such that υ_ (ϰ)≤¯υ(ϰ) on J. Moreover, the function f(ϰ,υ) is continuouson J and there exists a constant number κ>0 such that |f(ϰ,υ)−f(ϰ,v)|≤κ|υ−v|, for υ,v∈R+, ϰ∈J. If
Q1=κ[ϕ(b)−ϕ(0)]γ2−1Γ(γ2)Θ[[ϕ(b)−ϕ(0)]μ2+μ1Γ(μ2+1)Γ(μ1+1)+m∑i=1δiΓ(μ2)[ϕ(ζi)−ϕ(0)]μ2+μ1+σiΓ(μ2+σi+1)Γ(μ2+σi)Γ(μ1+1)]+κ[ϕ(b)−ϕ(0)]μ2+μ1Γ(μ2+1)Γ(μ1+1)<1, |
then the problem (1.1) has a unique solution υ∈C(J).
Proof. Let Ξ=P−P_, where P(ϰ)=(HDμ2,β2;ϕ0++λ2)υ(ϰ) and P_(ϰ)=(HDμ2,β2;ϕ0++λ2)υ_(ϰ). Then, we get
{(HDμ1,β1;ϕ0++λ1)Ξ≥0,ϰ∈(0,b],Ξ(0)=0. |
In view of Lemma 2.9, we have Ξ(ϰ)≥0 on J and hence P_ (ϰ)≤P(ϰ). Since P(ϰ)=(HDμ2,β2;ϕ0++λ2)υ(ϰ) and P_(ϰ)=(HDμ2,β2;ϕ0++λ2)υ_(ϰ), by the same technique, we get υ_ (ϰ)≤υ(ϰ). Similarly, we can show that υ(ϰ)≤¯υ(ϰ). Consider the continuous operator G:C(J)→C(J) defined by
Gυ(ϰ)=[ϕ(ϰ)−ϕ(0)]γ2−1Eμ2,γ2(−λ2[ϕ(ϰ)−ϕ(0)]μ2)Θ[Γ(μ2)Iμ2,ϕ0+Eμ2,μ2(−λ2[ϕ(b)−ϕ(0)]μ2)(Γ(μ1)Iμ1,ϕ0+Eμ1,μ1(−λ1[ϕ(b)−ϕ(0)]μ1)f(b,υ(b)))−m∑i=1δiΓ(μ2)Iμ2+σi,ϕ0+Eμ2,μ2+σi(−λ2[ϕ(ζi)−ϕ(0)]μ2)(Γ(μ1)Iμ1,ϕ0+Eμ1,μ1(−λ1[ϕ(ζi)−ϕ(0)]μ1)f(ζi,υ(ζi)))]+Γ(μ2)Iμ2,ϕ0+Eμ2,μ2(−λ2[ϕ(ϰ)−ϕ(0)]μ2)(Γ(μ1)Iμ1,ϕ0+Eμ1,μ1(−λ1[ϕ(ϰ)−ϕ(0)]μ1)f(ϰ,υ(ϰ))). |
Clearly, the fixed point of G is a solution to problem (1.1). Define a closed ball BR as
BR={υ∈C(J):‖υ‖C(J)≤R,} |
with
R≥Q21−Q1, |
where
Q2=P[ϕ(b)−ϕ(0)]γ2−1Γ(γ2)Θ[[ϕ(b)−ϕ(0)]μ2+μ1Γ(μ2+1)Γ(μ1+1)+m∑i=1δiΓ(μ2)[ϕ(ζi)−ϕ(0)]μ2+μ1+σiΓ(μ2+σi+1)Γ(μ2+σi)Γ(μ1+1)]+P[ϕ(b)−ϕ(0)]μ2+μ1Γ(μ2+1)Γ(μ1+1) |
and P=sups∈J|f(s,0)|. Let υ∈BR and ϰ∈J. Then by Lemma 2.6, we have
|f(ϰ,υ(ϰ))|=|f(ϰ,υ(ϰ))−f(ϰ,0)+f(ϰ,0)|≤|f(ϰ,υ(ϰ))−f(ϰ,0)|+|f(ϰ,0)|≤κ|υ(ϰ)|+P≤(κ‖υ‖+P). |
Now, we will present the proof in two steps:
First step: We will show that G(BR)⊂BR. First, by Lemma 2.6 and Definition 2.1, we have
Iμ2,ϕ0+Eμ2,μ2(λ2[ϕ(ϰ)−ϕ(0)]μ2)≤[ϕ(ϰ)−ϕ(0)]μ2Γ(μ2+1)Γ(μ2). |
Next, for υ∈BR, we obtain
|Gυ(ϰ)|≤[ϕ(b)−ϕ(0)]γ2−1Γ(γ2)Θ[(κ‖υ‖+P)[ϕ(b)−ϕ(0)]μ2+μ1Γ(μ2+1)Γ(μ1+1)+m∑i=1δiΓ(μ2)[ϕ(ζi)−ϕ(0)]μ2+μ1+σiΓ(μ2+σi+1)Γ(μ2+σi)Γ(μ1+1)(κ‖υ‖+P)]+(κ‖υ‖+P)[ϕ(b)−ϕ(0)]μ2+μ1Γ(μ2+1)Γ(μ1+1)≤Q1R+Q2≤R. |
Thus G(BR)⊂BR.
Second step: We shall prove that G is contraction. Let υ,ˆυ∈BR and ϰ∈J. Then by Lemma 2.6 and Definition 2.1, we obtain
‖Gυ−Gˆυ‖≤κ‖υ−ˆυ‖(ϕ(bϰ)−ϕ(0))γ2−1Γ(γ2)Θ[[ϕ(b)−ϕ(0)]μ2+μ1Γ(μ2+1)Γ(μ1+1)+m∑i=1δiΓ(μ2)[ϕ(ζi)−ϕ(0)]μ2+μ1+σiΓ(μ2+σi+1)Γ(μ2+σi)Γ(μ1+1)]+κ‖υ−ˆυ‖[ϕ(b)−ϕ(0)]μ2+μ1Γ(μ2+1)Γ(μ1+1)≤Q1‖υ−ˆυ‖. |
Thus, G is a contraction. Hence, the Banach contraction principle theorem [35] shows that the problem (1.1) has a unique solution.
Theorem 3.4. Assume that ¯υ,υ_∈C(J) be upper and lower solutions of the problem (1.1), respectively, and υ_ (ϰ)≤¯υ(ϰ) on J. Inaddition, If the continuous function f: J×R→R satisfies f(ϰ,υ(ϰ))≤f(ϰ,y(ϰ)) for allυ_ (ϰ)≤υ(ϰ)≤y(ϰ)≤¯υ(ϰ),ϰ∈ J then there exist monotoneiterative sequences {υ_j}∞j=0 and {¯υj}∞j=0 which uniformly converges on J to the extremal solutions of problem (1.1) in Φ={υ∈C(J):υ_(ϰ)≤υ(ϰ)≤¯υ(ϰ),ϰ∈J}.
Proof. Step (1): Setting υ_0=υ_ and ¯υ0=¯υ, then given {υ_j}∞j=0 and {¯υj}∞j=0 inductively define υ_j+1 and ¯υj+1 to be the unique solutions of the following problem
{(HDμ1,β1;ϕ0++λ1)(HDμ2,β2;ϕ0++λ2)υ_j+1(ϰ)=f(ϰ,υ_j(ϰ)),ϰ∈J, HDμ2,β2;ϕ0+υ_j+1(ϰ)|ϰ=0=0,υ_j+1(0)=0,υ_j+1(b)=∑mi=1δiIσi,ϕ0+υ_j+1(ζi). | (3.1) |
and
{(HDμ1,β1;ϕ0++λ1)(HDμ2,β2;ϕ0++λ2)¯υj+1(ϰ)=f(ϰ,¯υj(ϰ)),ϰ∈J, HDμ2,β2;ϕ0+¯υj+1(ϰ)|ϰ=0=0,¯υj+1(0)=0,¯υj+1(b)=∑mi=1δiIσi,ϕ0+¯υj+1(ζi). | (3.2) |
By Theorem 3.3, we know that the above problems have a unique solutions in C(J).
Step (2): Now, for ϰ∈J, we claim that
υ_(ϰ)=υ_0(ϰ)≤υ_1(ϰ)≤........≤υ_j(ϰ)≤υ_j+1(ϰ)≤......≤¯υj+1(ϰ)≤¯υj(ϰ)≤......≤¯υ1(ϰ)≤¯υ0(ϰ)=¯υ(ϰ). | (3.3) |
To confirm this claim, from (3.1) for j=0, we have
{(HDμ1,β1;ϕ0++λ1)(HDμ2,β2;ϕ0++λ2)υ_1(ϰ)=f(ϰ,υ_0(ϰ)),j≥0,HDμ2,β2;ϕ0+υ_1(ϰ)|ϰ=0=0,υ_1(0)=0,υ_1(b)=∑mi=1δiIσi,ϕ0+υ_1(ζi). | (3.4) |
With reference to the definitions of the lower solution υ_(ϰ)=υ_0(ϰ) and putting Ξ(ϰ)=P1(ϰ)− P_ 0(ϰ), where P1(ϰ)=(HDμ2,β2;ϕ0++λ2)υ1(ϰ) and P_0(ϰ)=(HDμ2,β2;ϕ0++λ2)υ_0(ϰ). Then, we get
{(HDμ1,β1;ϕ0++λ1)Ξ≥0,ϰ∈(0,b],Ξ(0)≥0. |
Consequently, Lemma 2.9 implies Ξ(ϰ)≥0, that means P_ 0(ϰ)≤P1(ϰ),ϰ∈J and by the same technique, where P(ϰ)=(HDμ2,β2;ϕ0++λ2)υ(ϰ) we get υ(ϰ)≥0. Hence, υ_0(ϰ)≤υ_1(ϰ),ϰ∈J. Now, from Eq (3.4) and our assumptions, we infer that
(HDμ1,β1;ϕ0++λ1)(HDμ2,β2;ϕ0++λ2)υ_1(ϰ)=f(ϰ,υ_0(ϰ))≤f(ϰ,υ_1(ϰ)). |
Therefore, υ_1 is a lower solution of problem (1.1). In the same way of the above argument, we conclude that υ_1(ϰ)≤υ_2(ϰ),ϰ∈J. By mathematical induction, we get υ_j(ϰ)≤υ_j+1(ϰ),ϰ∈J,j≥2.
Similarly, we put Ξ(ϰ)=¯P1(ϰ)−P_1(ϰ), where ¯P1(ϰ)=(HDμ2,β2;ϕ0++λ2)¯υ1(ϰ) and P_1(ϰ)=(HDμ2,β2;ϕ0++λ2)υ_1(ϰ). Then, we get
{(HDμ1,β1;ϕ0++λ1)Ξ(ϰ)≥0,ϰ∈(0,b],Ξ(0)≥0. |
Consequently, Lemma 2.9 implies Ξ(ϰ)≥0, that means ¯P1(ϰ)≤P_1(ϰ),ϰ∈J and by the same technique, we get ¯υ1(ϰ)≥υ_1(ϰ),ϰ∈J. By mathematical induction, we get ¯υj(ϰ)≥υ_j(ϰ), ϰ∈J, j≥0.
Step (3): In view of Eq (3.3), one can show that the sequences {υ_j}∞j=0 and {¯υj}∞j=0 are equicontinuous and uniformly bounded. In view of Arzela-Ascoli Theorem, we have limj→∞υ_j=υ∗ and limj→∞¯υj=υ∗ uniformly on J and the limit of the solutions υ∗ and υ∗ satisfy the problem (1.1). Moreover, υ∗, υ∗∈Φ.
Step (4): We will prove that υ∗ and υ∗ are the extremal solutions of the problem (1.1) in Φ. For this end, let υ∈Φ be a solution of the problem (1.1) such that ¯υj(ϰ)≥υ(ϰ)≥υ_j(ϰ),ϰ∈J, for some j∈N. Therefore, by our assumption, we find that
f(ϰ,¯υj(ϰ))≥f(ϰ,υ(ϰ))≥f(ϰ,υ_j(ϰ)). |
Hence
(HDμ1,β1;ϕ0++λ1)(HDμ2,β2;ϕ0++λ2)¯υj+1(ϰ)≥(HDμ1,β1;ϕ0++λ1)(HDμ2,β2;ϕ0++λ2)υ(ϰ)≥(HDμ1,β1;ϕ0++λ1)(HDμ2,β2;ϕ0++λ2)υ_j+1(ϰ), |
and
HDμ2,β2;ϕ0+¯υj+1(ϰ)|ϰ=0=HDμ2,β2;ϕ0+υ(ϰ)|ϰ=0=HDμ2,β2;ϕ0+υ_j+1(ϰ)|ϰ=0=0. |
Consequently, ¯υj+1(ϰ)≥υ(ϰ)≥υ_j+1(ϰ),ϰ∈J. It follows that
¯υj(ϰ)≥υ(ϰ)≥υ_j(ϰ),ϰ∈J, j∈N. | (3.5) |
Taking the limit of Eq (3.5) as j→∞, we get υ∗(ϰ)≥υ(ϰ)≥υ∗(ϰ), ϰ∈J. That is, υ∗ and υ∗ are the extremal solutions of the problem (1.1) in Φ.
Corollary 3.5. Assume that f:J×R+→R+ is continuous, and there exist \bmℵ1,\bmℵ2>0 such that
\bmℵ1≤f(ϰ,υ)≤\bmℵ2,∀(ϰ,υ)∈J×R+. | (3.6) |
Then the problem (1.1) has at least one solution υ(ϰ)∈C(J). Moreover
υ(ϰ)≤[ϕ(ϰ)−ϕ(0)]γ2−1Eμ2,γ2(−λ2[ϕ(ϰ)−ϕ(0)]μ2)Θ[Γ(μ2)Iμ2,ϕ0+Eμ2,μ2(−λ2[ϕ(b)−ϕ(0)]μ2)(Γ(μ1)Iμ1,ϕ0+Eμ1,μ1(−λ1[ϕ(b)−ϕ(0)]μ1)\bmℵ2)−m∑i=1δiΓ(μ2)Iμ2+σi,ϕ0+Eμ2,μ2+σi(λ2[ϕ(ζi)−ϕ(0)]μ2)(Γ(μ1)Iμ1,ϕ0+Eμ1,μ1(−λ1[ϕ(ζi)−ϕ(0)]μ1)\bmℵ2)]+Γ(μ2)Iμ2,ϕ0+Eμ2,μ2(−λ2[ϕ(ϰ)−ϕ(0)]μ2)(Γ(μ1)Iμ1,ϕ0+Eμ1,μ1(−λ1[ϕ(ϰ)−ϕ(0)]μ1)\bmℵ2) | (3.7) |
and
υ(ϰ)≥[ϕ(ϰ)−ϕ(0)]γ2−1Eμ2,γ2(−λ2[ϕ(ϰ)−ϕ(0)]μ2)Θ[Γ(μ2)Iμ2,ϕ0+Eμ2,μ2(−λ2[ϕ(b)−ϕ(0)]μ2)(Γ(μ1)Iμ1,ϕ0+Eμ1,μ1(−λ1[ϕ(b)−ϕ(0)]μ1)\bmℵ1)−m∑i=1δiΓ(μ2)Iμ2+σi,ϕ0+Eμ2,μ2+σi(λ2[ϕ(ζi)−ϕ(0)]μ2)(Γ(μ1)Iμ1,ϕ0+Eμ1,μ1(−λ1[ϕ(ζi)−ϕ(0)]μ1)\bmℵ1)]+Γ(μ2)Iμ2,ϕ0+Eμ2,μ2(−λ2[ϕ(ϰ)−ϕ(0)]μ2)(Γ(μ1)Iμ1,ϕ0+Eμ1,μ1(−λ1[ϕ(ϰ)−ϕ(0)]μ1)\bmℵ1). | (3.8) |
Proof. From Eq (3.6) and definition of control functions, we get
\bmℵ1≤f_(ϰ,υ(ϰ))≤¯f(ϰ,υ(ϰ))≤\bmℵ2, ∀(ϰ,υ)∈J×R+. | (3.9) |
Now, we consider the following problem
{(HDμ1,β1;ϕ0++λ1)(HDμ2,β2;ϕ0++λ2)¯υ(ϰ)=\bmℵ2,ϰ∈(0,b],HDμ2,β2;ϕ0+¯υ(ϰ)|ϰ=0=0, ¯υ(0)=0, ¯υ(b)=∑mi=1δiIσi,ϕ0+¯υ(ζi). | (3.10) |
In view of Lemma 2.8, the problem (3.10) has a solution
¯υ(ϰ)=[ϕ(ϰ)−ϕ(0)]γ2−1Eμ2,γ2(−λ2[ϕ(ϰ)−ϕ(0)]μ2)Θ[Γ(μ2)Iμ2,ϕ0+Eμ2,μ2(−λ2[ϕ(b)−ϕ(0)]μ2)(Γ(μ1)Iμ1,ϕ0+Eμ1,μ1(−λ1[ϕ(b)−ϕ(0)]μ1)\bmℵ2)−m∑i=1δiΓ(μ2)Iμ2+σi,ϕ0+Eμ2,μ2+σi(λ2[ϕ(ζi)−ϕ(0)]μ2)(Γ(μ1)Iμ1,ϕ0+Eμ1,μ1(−λ1[ϕ(ζi)−ϕ(0)]μ1)\bmℵ2)]+Γ(μ2)Iμ2,ϕ0+Eμ2,μ2(−λ2[ϕ(ϰ)−ϕ(0)]μ2)(Γ(μ1)Iμ1,ϕ0+Eμ1,μ1(−λ1[ϕ(ϰ)−ϕ(0)]μ1)\bmℵ2). |
Taking into account Eq (3.9), we obtain
¯υ(ϰ)≥[ϕ(ϰ)−ϕ(0)]γ2−1Eμ2,γ2(−λ2[ϕ(ϰ)−ϕ(0)]μ2)Θ[Γ(μ2)Iμ2,ϕ0+Eμ2,μ2(−λ2[ϕ(b)−ϕ(0)]μ2)(Γ(μ1)Iμ1,ϕ0+Eμ1,μ1(−λ1[ϕ(b)−ϕ(0)]μ1)¯f(b,¯υ(b)))−m∑i=1δiΓ(μ2)Iμ2+σi,ϕ0+Eμ2,μ2+σi(λ2[ϕ(ζi)−ϕ(0)]μ2)(Γ(μ1)Iμ1,ϕ0+Eμ1,μ1(−λ1[ϕ(ζi)−ϕ(0)]μ1)¯f(ζi,¯υ(ζi)))]+Γ(μ2)Iμ2,ϕ0+Eμ2,μ2(−λ2[ϕ(ϰ)−ϕ(0)]μ2)(Γ(μ1)Iμ1,ϕ0+Eμ1,μ1(−λ1[ϕ(ϰ)−ϕ(0)]μ1)¯f(ϰ,¯υ(ϰ))). |
It is obvious that ¯υ(ϰ) is the upper solution of problem (1.1). Also, we consider the following problem
{(HDμ1,β1;ϕ0++λ1)(HDμ2,β2;ϕ0++λ2)υ_(ϰ)=\bmℵ1,ϰ∈(0,b],HDμ2,β2;ϕ0+υ_(ϰ)|ϰ=0=0, υ_(0)=0, υ_(b)=∑mi=1δiIσi,ϕ0+υ_(ζi). | (3.11) |
In view of Lemma 2.8, the problem (3.11) has a solution
υ_(ϰ)=[ϕ(ϰ)−ϕ(0)]γ2−1Eμ2,γ2(−λ2[ϕ(ϰ)−ϕ(0)]μ2)Θ[Γ(μ2)Iμ2,ϕ0+Eμ2,μ2(−λ2[ϕ(b)−ϕ(0)]μ2)(Γ(μ1)Iμ1,ϕ0+Eμ1,μ1(−λ1[ϕ(b)−ϕ(0)]μ1)\bmℵ1)−m∑i=1δiΓ(μ2)Iμ2+σi,ϕ0+Eμ2,μ2+σi(λ2[ϕ(ζi)−ϕ(0)]μ2)(Γ(μ1)Iμ1,ϕ0+Eμ1,μ1(−λ1[ϕ(ζi)−ϕ(0)]μ1)\bmℵ1)]+Γ(μ2)Iμ2,ϕ0+Eμ2,μ2(−λ2[ϕ(ϰ)−ϕ(0)]μ2)(Γ(μ1)Iμ1,ϕ0+Eμ1,μ1(−λ1[ϕ(ϰ)−ϕ(0)]μ1)\bmℵ1). |
Taking into account Eq (3.9), we obtain
υ_(ϰ)≤[ϕ(ϰ)−ϕ(0)]γ2−1Eμ2,γ2(−λ2[ϕ(ϰ)−ϕ(0)]μ2)Θ[Γ(μ2)Iμ2,ϕ0+Eμ2,μ2(−λ2[ϕ(b)−ϕ(0)]μ2)(Γ(μ1)Iμ1,ϕ0+Eμ1,μ1(−λ1[ϕ(b)−ϕ(0)]μ1)f_(b,υ_(b)))−m∑i=1δiΓ(μ2)Iμ2+σi,ϕ0+Eμ2,μ2+σi(λ2[ϕ(ζi)−ϕ(0)]μ2)(Γ(μ1)Iμ1,ϕ0+Eμ1,μ1(−λ1[ϕ(ζi)−ϕ(0)]μ1)f_(ζi,υ_(ζi)))]+Γ(μ2)Iμ2,ϕ0+Eμ2,μ2(−λ2[ϕ(ϰ)−ϕ(0)]μ2)(Γ(μ1)Iμ1,ϕ0+Eμ1,μ1(−λ1[ϕ(ϰ)−ϕ(0)]μ1)f_(ϰ,υ_(ϰ))). |
Thus, υ_(ϰ) is the lower solution of problem (1.1).
The application of Theorem 3.4 results that problem (1.1) has at least one solution υ(ϰ)∈C(J) that satisfies the inequalities (3.7) and (3.8).
Example 4.1. Let us consider the following problem
{(HDμ1,β1;ϕ0++λ1)(HDμ2,β2;ϕ0++λ2)υ(ϰ)=f(ϰ,υ(ϰ)),ϰ∈[0,1],HDμ2,β2;ϕ0+υ(ϰ)|ϰ=0=0,υ(0)=0,υ(b)=∑mi=1δiIσi,ϕ0+υ(ζi), | (4.1) |
Here μ1=12,μ2=32,β1=β2=13,γ1=23,γ2=43,λ1=λ2=10,m=1,δ1=14,σ1=23,ζ1=34,b=1, ϕ=eϰ,λ1=λ2=10 and we set f(ϰ,υ(ϰ))=2+ϰ2+ϰ35(1+υ(ϰ))υ(ϰ). For υ,w∈R+, ϰ∈J, we have
|f(ϰ,υ)−f(ϰ,w)|=|(2+ϰ2+ϰ35(1+υ(ϰ))υ(ϰ))−(2+ϰ2+ϰ35(1+w(ϰ))w(ϰ))|≤15|υ(ϰ)−w(ϰ)|. |
By the given data, we get Q1≈0.9<1 and hence all conditions in Theorem 3.3 are satisfied with κ=15>0. Thus, the problem (4.1) has a unique solution υ∈C(J). On the other hand, from Theorem 3.4 and Theorem 3.3, the sequences {υ_n}∞n=0 and {¯υn}∞n=0 can be obtained as
¯υn+1(ϰ)=Γ(32)I32,eϰ0+E32,32(10[eϰ−1]32)(Γ(12)I12,eϰ0+E12,12(10[eϰ−1]12)(2+ϰ2+15(1+¯υn(ϰ))ϰ3¯υn(ϰ))). | (4.2) |
and
υ_n+1(ϰ)=Γ(32)I32,eϰ0+E32,32(10[eϰ−1]32)(Γ(12)I12,eϰ0+E12,12(10[eϰ−1]12)(2+ϰ2+15(1+υ_n(ϰ))ϰ3υ_n(ϰ))). | (4.3) |
Moreover, for any υ∈R+ and ϰ∈[0,1], we have
limυ→+∞f(ϰ,υ(ϰ))=limυ→+∞(2+ϰ2+ϰ35(1+υ(ϰ))υ(ϰ))=2+ϰ2+ϰ35. |
It follows that
2<f(ϰ,υ(ϰ))<165. |
Thus, by Corollary 3.5, we get \bmℵ1=2 and \bmℵ2=165. Then by Definitions 3.1 and 3.2, the problem (4.1) has a solution which verifies υ_ (ϰ)≤υ(ϰ)≤¯υ(ϰ) where
¯υ(ϰ)=(eϰ−1)43−1E32,43(−10(eϰ−1)32)Θ2[Γ(32)Γ(12)(e−1)2E32,3(−10(e−1)32)E12,1(−10(e−1)12)−45Γ(32)Γ(12)(e34−1)73E32,216(−10(e34−1)32)E12,1(−10(e34−1)12)]+165Γ(32)Γ(12)(eϰ−1)2E32,3(−10(e−1)32)E12,1(−10(eϰ−1)12), | (4.4) |
and
υ_(ϰ)=(eϰ−1)43−1E32,43(−10(eϰ−1)32)Θ165[Γ(32)Γ(12)(e−1)2E32,3(−10(e−1)32)E12,1(−10(e−1)12)−12Γ(32)Γ(12)(e34−1)73E32,216(−10(e34−1)32)E12,1(−10(e34−1)12)]+2Γ(32)Γ(12)(eϰ−1)2E32,3(−10(e−1)32)E12,1(−10(eϰ−1)12), | (4.5) |
are respectively the upper and lower solutions of the problem (4.1) and
Θ:=(14[e34−1]1E32,2(−10(e34−1)32)−[e−1]43−1E32,43(−10(e−1)32))≠0. |
Let us see graphically, we plot in Figure 1 the behavior of the upper solution ¯υ and lower solution υ_ of the problem (4.1) with given data above.
In this work, we have proved successfully the monotone iterative method is an effective method to study FLEs in the frame of ϕ-Hilfer fractional derivative with multi-point boundary conditions. Firstly, the formula of explicit solution of ϕ-Hilfer type FLE (1.1) in the term of Mittag-Leffler function has been derived. Next, we have investigated the lower and upper explicit monotone iterative sequences and proved that converge to the extremal solution of boundary value problems with multi-point boundary conditions. Finally, a numerical example has been given in order to illustrate the validity of our results.
Furthermore, it will be very important to study the present problem in this article regarding the Mittag-Leffler power low [36], the generalized Mittag-Leffler power low with another function [37,38], and the fractal-fractional operators [39].
Researchers would like to thank the Deanship of Scientific Research, Qassim University for funding the publication of this project. The authors are also grateful to the anonymous referees for suggestions that have improved manuscript.
The authors declare that they have no competing interests.
[1] |
V. Sharma, M. G. Dastidar, S. Sutradhar, V. Raj, K. De Silva, S. Roy, A step toward better sample management of COVID-19: On-spot detection by biometric technology and artificial intelligence, COVID-19 Sustain, Develop. Goals, 2022 (2022), 349–380. https://doi.org/10.1016/B978-0-323-91307-2.00017-1 doi: 10.1016/B978-0-323-91307-2.00017-1
![]() |
[2] |
G. Latif, H. Morsy, A. Hassan, J. Alghazo, Novel coronavirus and common pneumonia detection from CT scans using deep learning-based extracted features, Viruses, 14 (2022), 1667. https://doi.org/10.3390/v14081667 doi: 10.3390/v14081667
![]() |
[3] |
A. Islam, T. Rahim, M. Masuduzzaman, S. Y. Shin, A blockchain-based artificial intelligence-empowered contagious pandemic situation supervision scheme using internet of drone things, IEEE Wirel. Commun., 28 (2021), 166–173. https://doi.org/10.1109/MWC.001.2000429 doi: 10.1109/MWC.001.2000429
![]() |
[4] |
T. Rahim, M. A. Usman, S. Y. Shin, A survey on contemporary computer-aided tumor, polyp, and ulcer detection methods in wireless capsule endoscopy imaging, Comput. Med. Imag. Grap., 85 (2020), 101767. https://doi.org/10.1016/j.compmedimag.2020.101767 doi: 10.1016/j.compmedimag.2020.101767
![]() |
[5] |
G. Latif, DeepTumor: Framework for brain MR image classification, segmentation and tumor detection, Diagnostics, 12 (2022), 2888. https://doi.org/10.3390/diagnostics12112888 doi: 10.3390/diagnostics12112888
![]() |
[6] |
T. Rahim, S. A. Hassan, S. Y. Shin, A deep convolutional neural network for the detection of polyps in colonoscopy images, Biomed. Signal Proces., 68 (2021), 102654. https://doi.org/10.1016/j.bspc.2021.102654 doi: 10.1016/j.bspc.2021.102654
![]() |
[7] |
A. Bashar, G. Latif, G. Ben Brahim, N. Mohammad, J. Alghazo, COVID-19 pneumonia detection using optimized deep learning techniques, Diagnostics, 11 (2021), 1972. https://doi.org/10.3390/diagnostics11111972 doi: 10.3390/diagnostics11111972
![]() |
[8] |
E. Hussain, M. Hasan, M. A. Rahman, I. Lee, T. Tamanna, M. Z. Parvez, CoroDet: A deep learning based classification for COVID-19 detection using chest X-ray images, Chaos Soliton. Fract., 142 (2021), 110495. https://doi.org/10.1016/j.chaos.2020.110495 doi: 10.1016/j.chaos.2020.110495
![]() |
[9] |
G. Latif, G. Ben Brahim, D. N. F. A. Iskandar, A. Bashar, J. Alghazo, Glioma tumors' classification using deep-neural-network-based features with SVM classifier, Diagnostics, 12 (2022), 1018. https://doi.org/10.3390/diagnostics12041018 doi: 10.3390/diagnostics12041018
![]() |
[10] |
I. Iqbal, M. Younus, K. Walayat, M. U. Kakar, J. Ma, Automated multi-class classification of skin lesions through deep convolutional neural network with dermoscopic images, Comput. Med. Imag. grap, 88 (2021), 101843. https://doi.org/10.1016/j.compmedimag.2020.101843 doi: 10.1016/j.compmedimag.2020.101843
![]() |
[11] |
I. Iqbal, K. Walayat, M. U. Kakar, J. Ma, Automated identification of human gastrointestinal tract abnormalities based on deep convolutional neural network with endoscopic images, Intell. Syst. Appl., 16 (2022), 200149. https://doi.org/10.1016/j.iswa.2022.200149 doi: 10.1016/j.iswa.2022.200149
![]() |
[12] |
V. Shah, R. Keniya, A. Shridharani, M. Punjabi, J. Shah, N. Mehendale, Diagnosis of COVID-19 using CT scan images and deep learning techniques, Emerg. Radiol., 28 (2021), 497–505. https://doi.org/10.1007/s10140-020-01886-y doi: 10.1007/s10140-020-01886-y
![]() |
[13] |
M. M. Rahaman, C. Li, Y. Yao, K. Frank, M. A. Rahman, Q. Wang, et al., Identification of COVID-19 samples from chest X-Ray images using deep learning: A comparison of transfer learning approaches, J. X-Ray Sci. Technol., 28 (2020), 821–839. https://doi.org/10.3233/XST-200715 doi: 10.3233/XST-200715
![]() |
[14] |
A. S. Al-Waisy, S. Al-Fahdawi, M. A. Mohammed, K. H. Abdulkareem, S. A. Mostafa, M. S. Maashi, et al., COVID-CheXNet: Hybrid deep learning framework for identifying COVID-19 virus in chest X-rays images, Soft Comput., 27 (2020), 2657–2672. https://doi.org/10.1007/s00500-020-05424-3 doi: 10.1007/s00500-020-05424-3
![]() |
[15] |
Y. Chang, X. Jing, Z. Ren, B. Schuller, CovNet: A transfer learning framework for automatic COVID-19 detection from crowd-sourced cough sounds, Front. Digit. Health, 3 (2022), 799067. https://doi.org/10.3389/fdgth.2021.799067 doi: 10.3389/fdgth.2021.799067
![]() |
[16] |
M. Elpeltagy, H. Sallam, Automatic prediction of COVID-19 from chest images using modified ResNet50, Multimed. Tools Appl., 80 (2021), 26451–26463. https://doi.org/10.1007/s11042-021-10783-6 doi: 10.1007/s11042-021-10783-6
![]() |
[17] |
R. K. Patel, M. Kashyap, Automated diagnosis of COVID stages from lung CT images using statistical features in 2-dimensional flexible analytic wavelet transform, Biocybern. Biomed. Eng., 42 (2022), 829–841. https://doi.org/10.1016/j.bbe.2022.06.005 doi: 10.1016/j.bbe.2022.06.005
![]() |
[18] |
D. K. Redie, A. E. Sirko, T. M. Demissie, S. S. Teferi, V. K. Shrivastava, O. P. Verma, et al., Diagnosis of COVID-19 using chest X-ray images based on modified DarkCovidNet model, Evol Intell., 16 (2022), 729–738. https://doi.org/10.1007/s12065-021-00679-7 doi: 10.1007/s12065-021-00679-7
![]() |
[19] |
F. Özyurt, Efficient deep feature selection for remote sensing image recognition with fused deep learning architectures, J. Supercomput, 76 (2020), 8413–8431. https://doi.org/10.1007/s11227-019-03106-y doi: 10.1007/s11227-019-03106-y
![]() |
[20] |
D. H. Hubel, T. N. Wiesel, Receptive fields, binocular interaction and functional architecture in the cat's visual cortex, J. Physiol., 160 (1962), 106–154. https://doi.org/10.1113/jphysiol.1962.sp006837 doi: 10.1113/jphysiol.1962.sp006837
![]() |
[21] | Y. LeCun, Y. Bengio, Convolutional networks for images, speech, and time series, In: The handbook of brain theory and neural networks, 1995. |
[22] | G. Latif, J. Alghazo, L. Alzubaidi, M. N. Nasser, Y. Alghazo, Deep convolutional neural network for recognition of unified multi-language handwritten numerals, In: 2018 IEEE 2nd International Workshop on Arabic and Derived Script Analysis and Recognition (ASAR), 2018. https://doi.org/10.1109/ASAR.2018.8480289 |
[23] |
A. Krizhevsky, I. Sutskever, G. E. Hinton, ImageNet classification with deep convolutional neural networks. Commun. ACM, 60 (2017), 84–90. https://doi.org/10.1145/3065386 doi: 10.1145/3065386
![]() |
[24] | C. Szegedy, W. Liu, Y. Jia, P. Sermanet, S. Reed, D. Anguelov, et al., Going deeper with convolutions, 2014, arXiv: 1409.4842. |
[25] | S. Alghamdi, M. Alabkari, F. Aljishi, G. Latif, A. Bashar, Lung cancer detection from LDCT images using deep convolutional neural networks, In: International Conference on Communication, Computing and Electronics Systems, Singapore: Springer, 733 (2021), 363–374. https://doi.org/10.1007/978-981-33-4909-4_27 |
[26] |
D. A. Alghmgham, G. Latif, J. Alghazo, L. Alzubaidi, Autonomous traffic sign (ATSR) detection and recognition using deep CNN, Procedia Comput. Sci., 163 (2019), 266–274. https://doi.org/10.1016/j.procs.2019.12.108 doi: 10.1016/j.procs.2019.12.108
![]() |
[27] |
G. Latif, N. Mohammad, R. AlKhalaf, R. AlKhalaf, J. Alghazo, M. Khan, An automatic arabic sign language recognition system based on deep CNN: An assistive system for the deaf and hard of hearing, Int. J. Comput. Digital Syst., 9 (2020), 715–724. http://doi.org/10.12785/ijcds/090418 doi: 10.12785/ijcds/090418
![]() |
[28] | B. Zhou, A. Lapedriza, J. Xiao, A. Torralba, A. Oliva, Learning deep features for scene recognition using places database, In: NIPS'14: Proceedings of the 27th International Conference on Neural Information Processing Systems, 1 (2014), 487–495. |
[29] |
M. M. Butt, G. Latif, D. N. F. A. Iskandar, J. Alghazo, A. H. Khan, Multi-channel convolutions neural network based diabetic retinopathy detection from fundus images, Procedia Comput. Sci., 163 (2019), 283–291. https://doi.org/10.1016/j.procs.2019.12.110 doi: 10.1016/j.procs.2019.12.110
![]() |
[30] | D. C. Cireşan, U. Meier, J. Masci, L. Gambardella, J. Schmidhuber, Flexible, high performance convolutional neural networks for image classification, In: Proceedings of the Twenty-Second International Joint Conference on Artificial Intelligence, 2011, 1237–1242. https://doi.org/10.5591/978-1-57735-516-8/IJCAI11-210 |
[31] |
G. Lokku, G. H. Reddy, M. N. G. Prasad, OPFaceNet: Optimized face recognition network for noise and occlusion affected face images using hyperparameters tuned convolutional neural network, Appl. Soft Comput., 117 (2022), 108365. https://doi.org/10.1016/j.asoc.2021.108365 doi: 10.1016/j.asoc.2021.108365
![]() |
[32] |
S. Y. Kim, Z. W. Geem, G. Han, Hyperparameter optimization method based on harmony search algorithm to improve performance of 1D CNN human respiration pattern recognition system, Sensors, 20 (2020), 3697. https://doi.org/10.3390/s20133697 doi: 10.3390/s20133697
![]() |
[33] |
G. Latif, K. Bouchard, J. Maitre, A. Back, L. P. Bedard, Deep-learning-based automatic mineral grain segmentation and recognition, Minerals, 12 (2022), 455. https://doi.org/10.3390/min12040455 doi: 10.3390/min12040455
![]() |
[34] |
J. Bruna, S. Mallat, Invariant scattering convolution networks, IEEE T. Pattern Anal., 35 (2013), 1872–1886. https://doi.org/10.1109/TPAMI.2012.230 doi: 10.1109/TPAMI.2012.230
![]() |
[35] | S. Lawrence, C. L. Giles, A. C. Tsoi, What size neural network gives optimal generalization? Convergence properties of backpropagation, In: Digital Repository at the University of Maryland, 1998. |
[36] | L. Wan, M. Zeiler, S. Zhang, Y. Cun, R. Fergus, Regularization of neural networks using dropconnect, In: ICML'13: Proceedings of the 30th International Conference on International Conference on Machine Learning, 28 (2013), 1058–1066. |
[37] |
Q. Xu, M. Zhang, Z. Gu, G. Pan, Overfitting remedy by sparsifying regularization on fully-connected layers of CNNs, Neurocomputing, 328 (2019), 69–74. https://doi.org/10.1016/j.neucom.2018.03.080 doi: 10.1016/j.neucom.2018.03.080
![]() |
[38] |
S. R. Dubey, S. K. Singh, B. B. Chaudhuri, Activation functions in deep learning: A comprehensive survey and benchmark, Neurocomputing, 503 (2022), 92–108. https://doi.org/10.1016/j.neucom.2022.06.111 doi: 10.1016/j.neucom.2022.06.111
![]() |
[39] |
S. Akbar, M. Peikari, S. Salama, S. Nofech-Mozes, A. Martel, The transition module: A method for preventing overfitting in convolutional neural networks, Comput. Methods Biomech. Biomed. Eng.: Imaging Vis., 7 (2019), 260–265. https://doi.org/10.1080/21681163.2018.1427148 doi: 10.1080/21681163.2018.1427148
![]() |
[40] |
H. Wu, X. Gu, Towards dropout training for convolutional neural networks, Neural Networks, 71 (2015), 1–10. https://doi.org/10.1016/j.neunet.2015.07.007 doi: 10.1016/j.neunet.2015.07.007
![]() |
[41] |
M. Anthimopoulos, S. Christodoulidis, L. Ebner, A. Christe, S. Mougiakakou, Lung pattern classification for interstitial lung diseases using a deep convolutional neural network, IEEE T. Med. Imaging, 35 (2016), 1207–1216. https://doi.org/10.1109/TMI.2016.2535865 doi: 10.1109/TMI.2016.2535865
![]() |
[42] | J. Chen, Y. Shen, The effect of kernel size of CNNs for lung nodule classification, In: 2017 9th International Conference on Advanced Infocomm Technology (ICAIT), 2017,340–344. https://doi.org/10.1109/ICAIT.2017.8388942 |
[43] | B. Chen, W. Deng, J. Du, Noisy softmax: Improving the generalization ability of DCNN via postponing the early softmax saturation, In: 2017 IEEE Conference on Computer Vision and Pattern Recognition (CVPR), 2017, 4021–4030. https://doi.org/10.1109/CVPR.2017.428 |
[44] | Y. Bengio, P. Lamblin, D. Popovici, H. Larochelle, Greedy layer-wise training of deep networks, In: Advances in Neural Information Processing Systems 19 (NIPS 2006), 2006. |
[45] | K. He, X. Zhang, S. Ren, J. Sun, Deep residual learning for image recognition, In: 2016 IEEE Conference on Computer Vision and Pattern Recognition (CVPR), 2016,770–778. https://doi.org/10.1109/CVPR.2016.90 |
[46] | S. Han, J. Pool, J. Tran, W. J. Dally, Learning both weights and connections for efficient neural network, In: NIPS'15: Proceedings of the 28th International Conference on Neural Information Processing Systems, 1 (2015), 1135–1143. |
[47] |
P. Ochs, A. Dosovitskiy, T. Brox, T. Pock, On iteratively reweighted algorithms for nonsmooth nonconvex optimization in computer vision, SIAM J. Imaging Sci., 8 (2015), 331–372. https://doi.org/10.1137/140971518 doi: 10.1137/140971518
![]() |
[48] | P. Murugan, S. Durairaj, Regularization and optimization strategies in deep convolutional neural network, 2017, arXiv: 1712.04711. |
[49] | J. Snoek, O. Rippel, K. Swersky, R. Kiros, N. Satish, N. Sundaram, et al., Scalable Bayesian optimization using deep neural networks, In: ICML'15: Proceedings of the 32nd International Conference on International Conference on Machine Learning, 37 (2015), 2171–2180. |
[50] | D. Cheng, Y. Gong, S. Zhou, J. Wang, N. Zheng, Person re-identification by multi-channel parts-based CNN with improved triplet loss function, In: 2016 IEEE Conference on Computer Vision and Pattern Recognition (CVPR), 2016, 1335–1344. https://doi.org/10.1109/CVPR.2016.149 |
[51] |
Y. S. Aurelio, G. M. de Almeida, C. L. de Castro, A. P. Braga, Learning from imbalanced data sets with weighted cross-entropy function, Neural Process Lett., 50 (2019), 1937–1949. https://doi.org/10.1007/s11063-018-09977-1 doi: 10.1007/s11063-018-09977-1
![]() |
[52] |
M. Bouten, J. Schietse, C. Van. den Broeck, Gradient descent learning in perceptrons: A review of its possibilities, Phys. Rev. E, 52 (1995), 1958–1967. https://doi.org/10.1103/PhysRevE.52.1958 doi: 10.1103/PhysRevE.52.1958
![]() |
[53] | A. El-Sawy, M. Loey, H. El-Bakry, Arabic handwritten characters recognition using convolutional neural network, WSEAS Trans. Comput. Res., 5 (2017), 11–19. |
[54] |
Y. Sun, W. Zhang, H. Gu, C. Liu, S. Hong, W. Xu, et al., Convolutional neural network based models for improving super-resolution imaging, IEEE Access, 7 (2019), 43042–43051. https://doi.org/10.1109/ACCESS.2019.2908501 doi: 10.1109/ACCESS.2019.2908501
![]() |
[55] |
G. D. Rubin, C. J. Ryerson, L. B. Haramati, N. Sverzellati, J. P. Kanne, S. Raoof, et al., The role of chest imaging in patient management during the COVID-19 pandemic: A multinational consensus statement from the Fleischner society, Radiology, 296 (2020), 172–180. https://doi.org/10.1148/radiol.2020201365 doi: 10.1148/radiol.2020201365
![]() |
[56] |
B. Xu, Y. Xing, J. Peng, Z. Zheng, W. Tang, Y. Sun, et al., Chest CT for detecting COVID-19: A systematic review and meta-analysis of diagnostic accuracy, Eur Radiol, 30 (2020), 5720–5727. https://doi.org/10.1007/s00330-020-06934-2 doi: 10.1007/s00330-020-06934-2
![]() |
[57] |
A. M. Rahmani, E. Azhir, M. Naserbakht, M. Mohammadi, A. H. M. Aldalwie, M. K. Majeed, et al., Automatic COVID-19 detection mechanisms and approaches from medical images: A systematic review, Multimed. Tools Appl., 81 (2022), 28779–28798. https://doi.org/10.1007/s11042-022-12952-7 doi: 10.1007/s11042-022-12952-7
![]() |
[58] | E. E. Hemdan, M. A. Shouman, M. E. Karar, COVIDX-Net: A framework of deep learning classifiers to diagnose COVID-19 in X-ray images, 2020, arXiv: 2003.11055. |
[59] |
M. Polsinelli, L. Cinque, G. Placidi, A light CNN for detecting COVID-19 from CT scans of the chest, Pattern Recogn. Lett., 140 (2020), 95–100. https://doi.org/10.1016/j.patrec.2020.10.001 doi: 10.1016/j.patrec.2020.10.001
![]() |
[60] |
A. Narin, C. Kaya, Z. Pamuk, Automatic detection of coronavirus disease (COVID-19) using X-ray images and deep convolutional neural networks, Pattern Anal, Appl., 24 (2021), 1207–1220. https://doi.org/10.1007/s10044-021-00984-y doi: 10.1007/s10044-021-00984-y
![]() |
[61] | P. Mooney, Chest X-ray images (Pneumonia), 2018. Available from: https://www.kaggle.com/datasets/paultimothymooney/chest-xray-pneumonia. |
[62] | T. Rahman, COVID-19 radiography database, 2020. Available from: https://www.kaggle.com/datasets/tawsifurrahman/covid19-radiography-database. |
[63] |
I. D. Apostolopoulos, T. A. Mpesiana, Covid-19: Automatic detection from X-ray images utilizing transfer learning with convolutional neural networks, Phys. Eng. Sci. Med., 43 (2020), 635–640. https://doi.org/10.1007/s13246-020-00865-4 doi: 10.1007/s13246-020-00865-4
![]() |
[64] |
P. K. Sethy, S. K. Behera, P. K. Ratha, P. Biswas, Detection of coronavirus disease (COVID-19) based on deep features and support vector machine, Int. J. Math. Eng. Manage. Sci., 5 (2020), 643–651. https://doi.org/10.33889/IJMEMS.2020.5.4.052 doi: 10.33889/IJMEMS.2020.5.4.052
![]() |
[65] | Y. Wang, M. Hu, Q. Li, X. Zhang, G. Zhai, N. Yao, Abnormal respiratory patterns classifier may contribute to large-scale screening of people infected with COVID-19 in an accurate and unobtrusive manner, 2020, arXiv: 2002.05534. |
[66] |
J. Zhang, Y. Xie, G. Pang, Z. Liao, J. Verjans, W. Li, et al., Viral pneumonia screening on chest X-rays using confidence-aware anomaly detection, IEEE T. Med. Imaging, 40 (2021), 879–890. https://doi.org/10.1109/TMI.2020.3040950 doi: 10.1109/TMI.2020.3040950
![]() |
[67] |
P. Afshar, S. Heidarian, F. Naderkhani, A. Oikonomou, K. N. Plataniotis, A. Mohammadi, COVID-CAPS: A capsule network-based framework for identification of COVID-19 cases from X-ray images, Pattern Recogn. Lett., 138 (2020), 638–643. https://doi.org/10.1016/j.patrec.2020.09.010 doi: 10.1016/j.patrec.2020.09.010
![]() |
[68] |
M. E. H. Chowdhury, T. Rahman, A. Khandakar, R. Mazhar, M. A. Kadir, Z. B. Mahbub, et al., Can AI help in screening viral and COVID-19 pneumonia? IEEE Access, 8 (2020), 132665–132676. https://doi.org/10.1109/ACCESS.2020.3010287 doi: 10.1109/ACCESS.2020.3010287
![]() |
[69] | L. O. Hall, R. Paul, D. B. Goldgof, G. M. Goldgof, Finding Covid-19 from chest X-rays using deep learning on a small dataset, 2020, arXiv: 2004.02060. |
[70] |
T. Ozturk, M. Talo, E. A. Yildirim, U. B. Baloglu, O. Yildirim, U. R. Acharya, Automated detection of COVID-19 cases using deep neural networks with X-ray images, Comput. Biol. Med., 121 (2020), 103792. https://doi.org/10.1016/j.compbiomed.2020.103792 doi: 10.1016/j.compbiomed.2020.103792
![]() |
[71] |
R. M. Pereira, D. Bertolini, L. O. Teixeira, C. N. Silla, Y. M. G. Costa, COVID-19 identification in chest X-ray images on flat and hierarchical classification scenarios, Comput. Meth. Prog. Bio., 194 (2020), 105532. https://doi.org/10.1016/j.cmpb.2020.105532 doi: 10.1016/j.cmpb.2020.105532
![]() |
[72] | L. Mahdy, K. Ezzat, H. Elmousalami, H. Ella, A. Hassanien, Automatic X-ray COVID-19 lung image classification system based on multi-level thresholding and support vector machine, 2020, medRxiv preprint. https://doi.org/10.1101/2020.03.30.20047787 |
[73] | K. El Asnaoui, Y. Chawki, A. Idri, Automated methods for detection and classification pneumonia based on X-ray images using deep learning, In: Artificial intelligence and blockchain for future cybersecurity applications, Springer, Cham, 2021,257–284. https://doi.org/10.1007/978-3-030-74575-2_14 |
[74] |
D. Singh, V. Kumar, V. Kaur, M. Kaur, Classification of COVID-19 patients from chest CT images using multi-objective differential evolution–based convolutional neural networks, Eur. J. Clin. Microbiol. Infect. Dis., 39 (2020), 1379–1389. https://doi.org/10.1007/s10096-020-03901-z doi: 10.1007/s10096-020-03901-z
![]() |
[75] |
M. Yamac, M. Ahishali, A. Degerli, S. Kiranyaz, M. E. H. Chowdhury, M. Gabbouj, Convolutional sparse support estimator-based COVID-19 recognition from X-ray images, IEEE T. Neur. Net. Lear. Syst., 32 (2021), 1810–1820. https://doi.org/10.1109/TNNLS.2021.3070467 doi: 10.1109/TNNLS.2021.3070467
![]() |
[76] | U. Özkaya, Ş. Öztürk, M. Barstugan, Coronavirus (COVID-19) classification using deep features fusion and ranking technique, In: Big Data Analytics and Artificial Intelligence Against COVID-19: Innovation Vision and Approach, Springer, Cham, 2020,281–295. https://doi.org/10.1007/978-3-030-55258-9_17 |
[77] |
C. Salvatore, M. Interlenghi, C. Monti, D. Ippolito, D. Capra, A. Cozzi, et al., Artificial intelligence applied to chest X-ray for differential diagnosis of COVID-19 pneumonia, Diagnostics, 11 (2021), 530. https://doi.org/10.3390/diagnostics11030530 doi: 10.3390/diagnostics11030530
![]() |
[78] | T. T. Nguyen, Q. V. H. Nguyen, D. T. Nguyen, S. Yang, P. W. Eklund, T. Huynh-The, et al., Artificial intelligence in the battle against coronavirus (COVID-19): A survey and future research directions, 2020, arXiv: 2008.07343. |
[79] |
E. Neri, V. Miele, F. Coppola, R. Grassi, Use of CT and artificial intelligence in suspected or COVID-19 positive patients: Statement of the Italian society of medical and interventional radiology, La radiologia medica, 125 (2020), 505–508. https://doi.org/10.1007/s11547-020-01197-9 doi: 10.1007/s11547-020-01197-9
![]() |
[80] |
L. Li, L. Qin, Z. Xu, Y. Yin, X. Wang, B. Kong, et al., Using artificial intelligence to detect COVID-19 and community-acquired pneumonia based on pulmonary CT: Evaluation of the diagnostic accuracy, Radiology, 296 (2020), E65–E71. https://doi.org/10.1148/radiol.2020200905 doi: 10.1148/radiol.2020200905
![]() |
[81] |
M. A. Amou, K. Xia, S. Kamhi, M. Mouhafid, A novel MRI diagnosis method for brain tumor classification based on CNN and Bayesian optimization, Healthcare, 10 (2022), 494. https://doi.org/10.3390/healthcare10030494 doi: 10.3390/healthcare10030494
![]() |
[82] |
S. Z. Kurdi, M. H. Ali, M. M. Jaber, T. Saba, A. Rehman, R. Damaševičius, Brain tumor classification using meta-heuristic optimized convolutional neural networks, J. Pers. Med, 13 (2023), 181. https://doi.org/10.3390/jpm13020181 doi: 10.3390/jpm13020181
![]() |
[83] |
E. Irmak, Multi-classification of brain tumor MRI images using deep convolutional neural network with fully optimized framework, Iran. J. Sci. Technol. Trans. Electr. Eng., 45 (2021), 1015–1036. https://doi.org/10.1007/s40998-021-00426-9 doi: 10.1007/s40998-021-00426-9
![]() |
[84] |
C. Venkatesh, K. Ramana, S. Y. Lakkisetty, S. S. Band, S. Agarwal, A. Mosavi, A neural network and optimization based lung cancer detection system in CT images, Front. Public Health, 10 (2022), 769692. https://doi.org/10.3389/fpubh.2022.769692 doi: 10.3389/fpubh.2022.769692
![]() |
[85] |
D. Paikaray, A. K. Mehta, D. A. Khan, Optimized convolutional neural network for the classification of lung cancer, J. Supercomput., 80 (2024), 1973–1989. https://doi.org/10.1007/s11227-023-05550-3 doi: 10.1007/s11227-023-05550-3
![]() |
[86] |
C. Lin, S. Jeng, M. Chen, Using 2D CNN with Taguchi parametric optimization for lung cancer recognition from CT images, Appl. Sci., 10 (2020), 2591. https://doi.org/10.3390/app10072591 doi: 10.3390/app10072591
![]() |
1. | Muhammad Aslam, Florentin Smarandache, Chi-square test for imprecise data in consistency table, 2023, 9, 2297-4687, 10.3389/fams.2023.1279638 | |
2. | Adewale F. Lukman, Rasha A. Farghali, B. M. Golam Kibria, Okunlola A. Oluyemi, Robust-stein estimator for overcoming outliers and multicollinearity, 2023, 13, 2045-2322, 10.1038/s41598-023-36053-z | |
3. | Maciej Neugebauer, Cengiz Akdeniz, Vedat Demir, Hüseyin Yurdem, Fuzzy logic control for watering system, 2023, 13, 2045-2322, 10.1038/s41598-023-45203-2 | |
4. | Muhammad Aslam, Neutrosophic Chi-Square Test for Analyzing Population Variances with Uncertain Data, 2025, 19, 1559-8608, 10.1007/s42519-025-00436-4 |