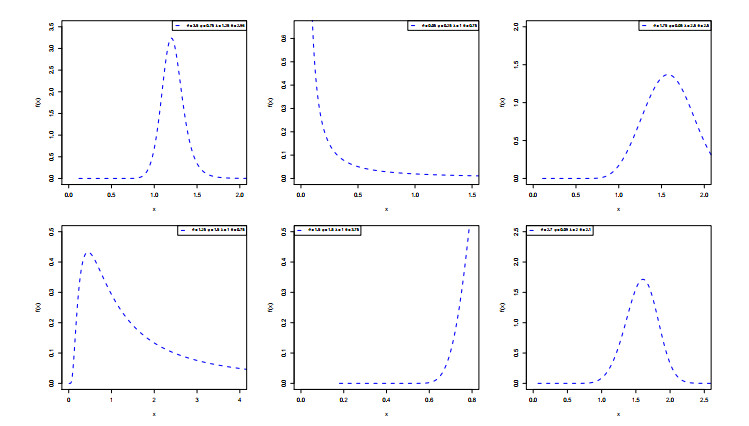
A nonautonomous logistic population model with a feature of an Allee threshold has been investigated in a periodically fluctuating environment. A slow periodicity of the harvesting effort was considered and may arise in response to relatively slow fluctuations of the environment. This assumption permits obtaining the analytical approximate solutions of such model using the perturbation approach based on the slow variation. Thus, the analytical expressions of the population evolution in the situation of subcritical and the supercritical harvesting were obtained and discussed in the framework of the Allee effect. Since the exact solution was not available due to the nonlinearity of the system, the numerical computation was considered to validate our analytical approximation. The comparison between the two methods showed a remarkable agreement as the time progressed, while such agreement fell off when the time was close to the initial density. Moreover, in the absence of the periodicity of the harvesting term, the expressions of the population evolution reduced to the exact solutions but in implicit forms. The finding results were appropriate for a wide range of parameter values, which lead to avoiding extensive recalculations while displaying the population behavior.
Citation: Fahad M. Alharbi. Harvesting a population model with Allee effect in a periodically varying environment[J]. AIMS Mathematics, 2024, 9(4): 8834-8847. doi: 10.3934/math.2024430
[1] | Mashail M. Al Sobhi . The modified Kies-Fréchet distribution: Properties, inference and application. AIMS Mathematics, 2021, 6(5): 4691-4714. doi: 10.3934/math.2021276 |
[2] | M. G. M. Ghazal, Yusra A. Tashkandy, Oluwafemi Samson Balogun, M. E. Bakr . Exponentiated extended extreme value distribution: Properties, estimation, and applications in applied fields. AIMS Mathematics, 2024, 9(7): 17634-17656. doi: 10.3934/math.2024857 |
[3] | Dawlah Alsulami . A new extension of the Rayleigh distribution: Properties, different methods of estimation, and an application to medical data. AIMS Mathematics, 2025, 10(4): 7636-7663. doi: 10.3934/math.2025350 |
[4] | Ahmed Sedky Eldeeb, Muhammad Ahsan-ul-Haq, Mohamed S. Eliwa . A discrete Ramos-Louzada distribution for asymmetric and over-dispersed data with leptokurtic-shaped: Properties and various estimation techniques with inference. AIMS Mathematics, 2022, 7(2): 1726-1741. doi: 10.3934/math.2022099 |
[5] | Fiaz Ahmad Bhatti, Azeem Ali, G. G. Hamedani, Mustafa Ç. Korkmaz, Munir Ahmad . The unit generalized log Burr XII distribution: properties and application. AIMS Mathematics, 2021, 6(9): 10222-10252. doi: 10.3934/math.2021592 |
[6] | Naif Alotaibi, A. S. Al-Moisheer, Ibrahim Elbatal, Salem A. Alyami, Ahmed M. Gemeay, Ehab M. Almetwally . Bivariate step-stress accelerated life test for a new three-parameter model under progressive censored schemes with application in medical. AIMS Mathematics, 2024, 9(2): 3521-3558. doi: 10.3934/math.2024173 |
[7] | Khaled M. Alqahtani, Mahmoud El-Morshedy, Hend S. Shahen, Mohamed S. Eliwa . A discrete extension of the Burr-Hatke distribution: Generalized hypergeometric functions, different inference techniques, simulation ranking with modeling and analysis of sustainable count data. AIMS Mathematics, 2024, 9(4): 9394-9418. doi: 10.3934/math.2024458 |
[8] | Ayed. R. A. Alanzi, Muhammad Imran, M. H. Tahir, Christophe Chesneau, Farrukh Jamal, Saima Shakoor, Waqas Sami . Simulation analysis, properties and applications on a new Burr XII model based on the Bell-X functionalities. AIMS Mathematics, 2023, 8(3): 6970-7004. doi: 10.3934/math.2023352 |
[9] | Nuran M. Hassan, M. Nagy, Subhankar Dutta . Statistical inference for the bathtub-shaped distribution using balanced and unbalanced sampling techniques. AIMS Mathematics, 2024, 9(9): 25049-25069. doi: 10.3934/math.20241221 |
[10] | SidAhmed Benchiha, Amer Ibrahim Al-Omari, Naif Alotaibi, Mansour Shrahili . Weighted generalized Quasi Lindley distribution: Different methods of estimation, applications for Covid-19 and engineering data. AIMS Mathematics, 2021, 6(11): 11850-11878. doi: 10.3934/math.2021688 |
A nonautonomous logistic population model with a feature of an Allee threshold has been investigated in a periodically fluctuating environment. A slow periodicity of the harvesting effort was considered and may arise in response to relatively slow fluctuations of the environment. This assumption permits obtaining the analytical approximate solutions of such model using the perturbation approach based on the slow variation. Thus, the analytical expressions of the population evolution in the situation of subcritical and the supercritical harvesting were obtained and discussed in the framework of the Allee effect. Since the exact solution was not available due to the nonlinearity of the system, the numerical computation was considered to validate our analytical approximation. The comparison between the two methods showed a remarkable agreement as the time progressed, while such agreement fell off when the time was close to the initial density. Moreover, in the absence of the periodicity of the harvesting term, the expressions of the population evolution reduced to the exact solutions but in implicit forms. The finding results were appropriate for a wide range of parameter values, which lead to avoiding extensive recalculations while displaying the population behavior.
Data analysis has been received a great interest in several applied fields such as medicine, reliability analysis, engineering, environmental studies, and economics. Several authors have proposed more flexible statistical distributions to model and predict various experimental and phenomenal data encountered in applied fields.
The Fréchet (Fr) distribution is also known as the inverse-Weibull distribution and it is one of the useful distributions in extreme value theory. The Fr model has some important applications in life testing, floods, earthquakes, and wind speeds, among others. Further information about the applications of the Fr distribution can be explored in [1,2,3,4,5].
The Fr distribution is specified by the following cumulative distribution function (CDF)
F(x;θ,λ)=e−λx−θ,θ,λ>0,x>0. | (1.1) |
Its probability density function (PDF) reduces to
f(x;θ,λ)=θλx−(θ+1)e−λx−θ,θ,λ>0,x>0, | (1.2) |
where θ and λ are, respectively, the shape and scale parameters. The PDF (1.2) exhibits a unimodal shape or a decreasing shape depending on θ while its hazard rate function (HRF) is always unimodal.
There is a clear need to define and develop more flexible versions of the Fr model using the well-known families to model several datasets encountered in many applied fields such as medicine, geology, engineering, and economics, among others. Hence, many authors have proposed several generalized forms of the Fr distribution to improve its flexibility and capability in modeling real-life data. Some notable extensions are the following: the exponentiated-Fr [6], beta-Fr [7], Marshall–Olkin Fr [8], transmuted Marshall–Olkin Fr [9], Weibull–Fr [10], beta exponential-Fr [11], Burr-X Fr [12], odd Lindley–Fr [13], logarithmic-transformed Fr [14], and modified Kies–Fr distributions [15].
This article introduces a new flexible extension of the Fr distribution called the extended Weibull–Fréchet (EWFr) distribution, which provides more flexibility to model real-life data than other competing distributions. Then, the first motivation to this article is devoted to introducing the EWFr distribution as a new extension of the Fr distribution via the extended Weibull-G (EW-G) family [16]. The useful characteristics of the EWFr distribution can be summarized as follows: The EWFr distribution is a more flexible version for the Fr distribution, and it improves the fitting of real-life data; it produces more flexible kurtosis than the baseline Fr model. The HRF of the EWFr distribution can exhibit an upside-down bathtub shape, an increasing shape, and a decreasing shape. Its density function can exhibit a symmetrical shape, a unimodal shape, an asymmetrical shape, a J shape, and a reversed-J shape. Furthermore, the EWFr distribution can be adopted to model various data in the medicine and engineering sciences. This fact was illustrated by modeling two real datasets from both fields, showing its superiority fit over other competing distributions.
Another motivation to this article is to show how several classical estimators of the EWFr distribution perform for different parameter combinations and several sample sizes. Hence, the EWFr parameters are estimated using different estimation approaches including: the maximum product of spacings estimators (MPSEs), least-squares estimators (LSEs), the right-tail Anderson-Darling estimators (RADEs), the maximum likelihood estimators (MLEs), the weighted least-squares estimators (WLSEs), the percentiles estimators (PCEs), the Cramér–von Mises estimators (CRVMEs), and the Anderson–Darling estimators (ADEs). Extensive simulation results were introduced to explore the performance of these estimators. Furthermore, these estimators are compared using partial and overall ranks to determine the best method for estimation the parameters of the EWFr distribution.
The paper is organized in six sections as follows: Section 2 introduces the EWFr distribution and its related functions. The distribution properties are determined in Section 3. Section 4 presents some classical estimators of the EWFr parameters. The simulation results for the classical methods are provided in the same section. Two real-life datasets are fitted using the EWFr distribution in Section 5. Some final remarks are presented in Section 6.
The EWFr distribution is constructed based on the EW-G family [16] which is specified, for any baseline CDF G(x;ζ), by the CDF
F(x;ϑ,φ,ζ)=1−{1+φ[G(x;ζ)1−G(x;ζ)]ϑ}−1φ,ϑ,φ>0,x∈ℜ. | (2.1) |
The corresponding PDF of (2.1) takes the form
f(x;ϑ,φ,ζ)=ϑg(x;ζ)G(x;ζ)ϑ−1[1−G(x;ζ)]ϑ+1{1+φ[G(x;ζ)1−G(x;ζ)]ϑ}−1φ−1. | (2.2) |
where g(x;ζ)=dG(x;ζ)/dx refers to the baseline density with parameter vector ζ.
To this end, by inserting Eq (1.1) in (2.1), the CDF of the EWFr model follows as
F(x;η)=1−{1+φ(eλx−θ−1)−ϑ}−1φ,ϑ,φ,λ,θ>0,x>0, | (2.3) |
where η=(ϑ,φ,λ,θ)⊺. The PDF of the EWFr model reduces to
f(x;η)=ϑθλx−(θ+1)eλx−θ(eλx−θ−1)−(ϑ+1){1+φ(eλx−θ−1)−ϑ}−(1φ+1), | (2.4) |
where ϑ,φ and θ are shape parameters whereas λ is a scale parameter.
The survival function (SF) of the EWFr distribution is given as
S(x;η)={1+φ(eλx−θ−1)−ϑ}−1φ. |
Long-term SF (LT-SF) is a useful feature in the modeling process, because a portion of the population may no longer be eligible to the event of interest with probability p (see [17,18]).
The general form of the LT-SF is SLT(x;p,η)=p+(1−p)S(x;η), where S(x;η) denotes the SF of any distribution and p denotes the probability of being cured. Hence, the PDF of the LT-SF can be derived as
fLT(x;p,η)=−∂∂xSLT(x;p,η)=(1−p)f(x;p,η),p∈(0,1). |
Using Eq (2.4), the PDF of the LT-SF of the EWFr distribution takes the form
fLT(x;p,η)=ϑθλ(1−p)eλx−θxθ+1(eλx−θ−1)−(ϑ+1){1+φ(eλx−θ−1)−ϑ}−(1φ+1). |
The HRF of the EWFr distribution takes the form
h(x;η)=ϑθλx−(θ+1)eλx−θ(eλx−θ−1)−(ϑ+1)1+φ(eλx−θ−1)−ϑ. |
Its reversed HRF has the form
r(x;η)=ϑθλx−(θ+1)eλx−θ(eλx−θ−1)−(ϑ+1){1+φ(eλx−θ−1)−ϑ}−(1φ+1)1−{1+φ(eλx−θ−1)−ϑ}−1φ. |
The odd ratio of the EWFr model is derived as
O(x;η)=F(x|η)S(x|η)={1+φ(eλx−θ−1)−ϑ}1φ−1. |
Figure 1 presents some possible shapes of the EWFr PDF for different values of its parameters. The EWFr PDF can be a symmetrical shape, a unimodal shape, an asymmetrical shape, a J shape, and a reversed-J shape. The hazard rate plots of the EWFr model are depicted in Figure 2. The EWFr HRF can be an increasing shape, a unimodal shape, and a decreasing shape.
The quantile function (QF) of the EWFr distribution, say, Q(u), can be calculated by solving F(x)=p in (2.3) in terms of p. Then, the EWFr QF follows as
Q(p)=λ1θ{ln([(1−p)−φ−1]1ϑφ1ϑ+[(1−p)−φ−1]1ϑ)}1θ,0<p<1. | (3.1) |
The median of the EWFr distribution follows by substituting p=0.5 in Eq (3.1).
A useful linear representation for the PDF of the EWFr model is provided based on [16]. Alizadeh et al. [16] introduced a simple representation for the density of the EW-G class as follows
f(x)=∞∑w,u=0ψw,uhϑw+u(x), | (3.2) |
where ψw,u=−φwΓ(ϑw+u)(−1φ)w/[w!u!Γ(ϑw)] and
hϑw+u(x)=(ϑw+u)g(x)G(x)ϑw+u−1, |
is the exponentiated-G PDF with a power parameter (ϑw+u)>0. Using Eqs (1.1) and (1.2) of the Fr distribution, Eq (3.2) can be expressed as
f(x)=∞∑w,u=0ψw,u(ϑw+u)θλx−(θ+1)e−(ϑw+u)λx−θ. | (3.3) |
Equation (3.3) can be rewritten as
f(x)=∞∑w,u=0ψw,ug(ϑw+u)(x), | (3.4) |
where g(ϑw+u)(x) denotes the Fr PDF with two-parameter θ and (ϑw+u)λ. Then, the density function of the EWFr model is expressed as a linear representation of Fr densities. Let Y be a random variable having the Fr distribution in (1.1). Hence, the r−th ordinary, μ′r,Y, and incomplete moments, ϕ(r,Y)(t), of Y are, respectively, given (for r<λ, ) by
μ′r,Y=λrθΓ(1−rθ)andϕ(r,Y)(t)=λrθγ(1−rθ,λt−θ), |
where Γ(s)=∫∞0ws−1e−wdw is the complete gamma function (GF) and γ(s,z)=∫z0ws−1e−wdw is the lower incomplete GF.
This section was devoted to deriving the r−th ordinary moment and incomplete moments of the EWFr distribution.
Proposition: Based on (3.4), the r−th moment of the EWFr distribution is defined by
μ′r=∞∑w,u=0ψw,u∫∞0xrgϑw+u(x)dxforr∈N. |
μ′r=∞∑w,u=0ψw,u(ϑw+u)rθΓ(1−rθ). | (3.5) |
Setting r=1 in Eq (3.5), we get the mean of x.
The s−th incomplete moment, say ϕs(x), of the EWFr distribution takes the form
ϕs(t)=∫t0xsf(x)dx=∞∑w,u=0ψw,u∫t0xsg(ϑw+u)(x)dx. |
Then, we obtain (fors<θ)
ϕs(t)=∞∑w,u=0ψw,u(ϑw+u)sθγ(1−sθ,(ϑw+u)λt−θ). |
The mean (μ), variance (σ2), skewness (ξ1(X)), and kurtosis (ξ2(X)) of the EWFr distribution are calculated numerically with λ=1 and different values of ϑ, φ and θ. Table 1 displays these numerical results. Table 1 shows that the EWFr model can be right-skewed and it can be leptokurtic (i.e., ξ2(X)>3).
η⊺ | μ | σ2 | ξ1(X) | ξ2(X) |
(ϑ=1.50,φ=0.50,θ=1.50) | 1.3511 | 0.4752 | 3.1421 | 56.2021 |
(ϑ=2.50,φ=0.75,θ=1.75) | 1.2701 | 0.1329 | 1.9395 | 16.1410 |
(ϑ=2.25,φ=1.00,θ=2.75) | 1.1904 | 0.0780 | 2.2648 | 19.2907 |
(ϑ=2.25,φ=0.25,θ=0.75) | 1.6154 | 0.7600 | 1.6132 | 9.0569 |
(ϑ=2.25,φ=1.25,θ=3.25) | 1.1831 | 0.0747 | 2.8068 | 27.8064 |
(ϑ=5.50,φ=1.50,θ=2.50) | 1.1927 | 0.0223 | 1.7507 | 11.7806 |
Let X1,X2,…Xn be a random sample from the EWFr (2.4) and X1:n≤X2:n≤⋯≤Xn:n be their corresponding order statistics (OS). The PDF and the CDF of the of r−th OS, say, Xr:n and 1≤r≤n are, respectively, defined by
fr:n(x)=n!(n−r)!(r−1)![F(x)]r−1[1−F(x)]n−rf(x)=n!(n−r)!(r−1)!n−r∑u=0(−1)u(n−ru)[F(x)]r−1+uf(x) | (3.6) |
and (for k=1,2,…,n)
Fr:n(x)=n∑l=k(nl)[F(x)]l[1−F(x)]n−l=n∑l=kn−r∑u=0(−1)u(nl)(n−ru)[F(x)]l+u. | (3.7) |
Using Eqs (3.6) and (3.7), the PDF and CDF of the r−th OS of the EWFr reduce to
fr:n(x)=ϑθλx−(θ+1)n!(r−1)!(n−r)!eλt−θ(eλx−θ−1)−(ϑ+1){1+φ(eλx−θ−1)−ϑ}−(1φ+1)n−r∑u=0(−1)u(n−ru)[1−{1+φ(eλx−θ−1)−ϑ}−1φ]r+u−1 |
and
Fr:n(x)=n∑l=kn−r∑u=0(−1)u(nl)(n−ru)[1−{1+φ(eλx−θ−1)−ϑ}−1φ]l+u. |
In this section, the EWFr parameters ϑ, λ, φ and θ are estimated using different frequentist approaches. We also provide detailed simulation results to compare and order their performances using partial and overall ranks.
The MLEs of the parameters ϑ, λ, φ and θ of the EWFr distribution are introduced in this sub-section. Let x1,…,xn be a sample from the EWFr distribution in (2.4). Hence, the log-likelihood function of η=(ϑ,φ,λ,θ)⊺ takes the form
l(η;x)=nlog(ϑ)+nlog(θ)+nlog(λ)−(θ+1)n∑i=1log(xi)−(ϑ+1)n∑i=1log(eλxi−θ−1)+λn∑i=1xi−θ−(1φ+1)n∑i=1log{1+φ(eλxi−θ−1)−ϑ}. |
The MLEs follow by maximizing the above equation by several programs such as {SAS} ({PROC NLMIXED}) or {R} ({optim} function).
Let x(1), x(2), ⋯, x(n) be the OS of a random sample from the PDF (2.4), then the LSEs [19] of the EWFr parameters are obtained by minimizing the function:
S(η)=n∑i=1[F(x(i))−in+1]2. |
Similarly, these estimators are also obtained by solving the following equation (for k=1,2,3,4)
n∑i=1({1+φ(eλxi−θ−1)−ϑ}−1φ−in+1)Ωk(x(i)|η)=0, |
where
Ω1(x(i)|η)=∂∂ϑF(x(i)|η)=1φ(1+φw−ϑi)−(1φ+1)−φw−ϑilnwi, |
Ω2(x(i)|η)=∂∂φF(x(i)|η)=(1+φw−ϑi)−1φln(1+φw−ϑi), |
Ω3(x(i)|η)=∂∂λF(x(i)|η)=1φ(1+φw−ϑi)−(1φ+1)−ϑφx−θiw−(ϑ+1)ieλxi−θ |
and
Ω4(x(i)|η)=∂∂θF(x(i)|η)=ϑλw−(ϑ+1)ieλxi−θx−θi(1+φw−ϑi)−(1φ+1)lnxi, |
where wi=eλxi−θ−1. The solution of Ωk for k=1,2,3,4 may be obtained numerically.
The WLSEs of the EWFr parameters can be determined by minimizing the equation (see [19]):
W(η)=n∑i=1(n+1)2(n+2)i(n−i+1)[F(x(i)|η)−in+1]2. |
The uniform spacings of a random sample from the EWFr distribution are defined (for i=1,2,…,n+1) by {Di(η)=F(x(i)|η)−F(x(i−1)|η)}, where F(x(0)|η)=0, F(x(n+1)|η)=1 and ∑n+1i=1Di(η)=1. The MPSEs of the EWFr parameters can be determined by maximizing the following geometric mean (GM) of spacings
G(η)=[n+1∏i=1Di(η)]1n+1 |
or by maximizing the logarithm of the GM of sample spacings
H(η)=1n+1n+1∑i=1logDi(η), |
The CRVMEs can be obtained using the difference between the estimated and empirical CDFs. The CRVMEs [20] of the EWFr parameters are determined by minimizing
C(η)=112n+n∑i=1[F(x(i)|η)−2i−12n]2. |
The ADEs [21] of the EWFr parameters are calculated by minimizing
A(η)=−n−1nn∑i=1(2i−1)[logF(x(i)|η)+logS(x(i)|η)]. |
The RADEs of the EWFr parameters are calculated by minimizing
R(η)=n2−2n∑i=1F(xi:n|η)−1nn∑i=1(2i−1)logS(xn+1−i:n|η). |
Consider the unbiased estimator of F(x(i)|η) which is defined by ui=i/(n+1). Hence, the PCEs of the EWFr parameters can be calculated by minimizing
P(η)=n∑i=1(x(i)−{ln([(1−ui)−φ−1]1ϑφ1ϑ+[(1−ui)−φ−1]1ϑ)λ}1θ)2. |
To compare and explore the behavior of different estimators of the EWFr parameters, we presented the numerical simulation results and ranked them with respect to their: average of absolute biases (|Bias(ˆη)|), |Bias(ˆη)|= 1N∑Ni=1|ˆη−η|, average of mean relative errors (MREs), MREs=1N∑Ni=1|ˆη−η|/η, and average mean square errors (MSEs), MSEs=1N∑Ni=1(ˆη−η)2.
The following algorithm can be adopted to explore the behavior of different estimators of the EWFr parameters:
Step 1: A random sample x1,x2,…,xn of sizes n=20, 80, 200, and 500 are generated from the QF (3.1).
Step 2: The required results are obtained based on eight combinations of the parameters ϑ={0.25,0.75,1.75,3.50,3.50}, φ={0.50,0.75,2.00,2.50,3.50}, λ={0.25,0.50,1.50,3.00,4.25} and θ={0.25,1.25,2.50}.
Step 3: Each sample is replicated N=5,000 times.
Step 4: Results of the biases, MSEs, and MREs are computed for the eight combinations, and to save more space, we present just the result of 5 combinations in Tables 2–6.
n | Est. | Est. Par. | MLEs | LSEs | WLSEs | CRVMEs | MPSEs | PCEs | ADEs | RADEs |
20 | |BIAS| | ˆϑ | 0.58150 {3} | 0.59477 {4} | 0.53422 {1} | 0.54541 {2} | 0.60555 {5} | 0.71685 {8} | 0.67445 {7} | 0.61466 {6} |
ˆφ | 0.47550 {4} | 0.54812 {7} | 0.46820 {2} | 0.53093 {6} | 0.19120 {1} | 0.55104 {8} | 0.46906 {3} | 0.51894 {5} | ||
ˆλ | 0.08930 {2} | 0.10351 {7} | 0.08402 {1} | 0.10057 {5} | 0.10077 {6} | 0.11204 {8} | 0.09758 {3} | 0.09866 {4} | ||
ˆθ | 0.49749 {7} | 0.47497 {4} | 0.46884 {3} | 0.48364 {5} | 0.45238 {1} | 0.53285 {8} | 0.46505 {2} | 0.48608 {6} | ||
MSEs | ˆϑ | 0.47425 {2} | 0.52401 {4} | 0.52337 {3} | 0.46463 {1} | 0.54588 {6} | 0.64689 {8} | 0.59726 {7} | 0.53428 {5} | |
ˆφ | 0.27624 {1} | 0.38734 {7} | 0.29294 {2} | 0.36212 {6} | 0.31849 {4} | 0.39577 {8} | 0.30160 {3} | 0.35513 {5} | ||
ˆλ | 0.01107 {2} | 0.01423 {7} | 0.00983 {1} | 0.01356 {5} | 0.01357 {6} | 0.01651 {8} | 0.01289 {3} | 0.01296 {4} | ||
ˆθ | 0.37764 {7} | 0.33320 {3} | 0.35778 {4} | 0.36101 {5} | 0.29381 {1} | 0.38887 {8} | 0.32193 {2} | 0.36311 {6} | ||
MREs | ˆϑ | 0.33229 {3} | 0.33987 {4} | 0.17807 {1} | 0.31166 {2} | 0.34603 {5} | 0.40963 {8} | 0.38540 {7} | 0.35123 {6} | |
ˆφ | 0.95101 {3} | 1.09624 {7} | 0.93641 {1} | 1.06186 {6} | 0.97231 {4} | 1.10208 {8} | 0.93811 {2} | 1.03788 {5} | ||
ˆλ | 0.35719 {2} | 0.41405 {7} | 0.33607 {1} | 0.40230 {5} | 0.40307 {6} | 0.44817 {8} | 0.39034 {3} | 0.39464 {4} | ||
ˆθ | 0.39799 {7} | 0.37998 {4} | 0.37507 {3} | 0.38691 {5} | 0.36190 {1} | 0.42628 {8} | 0.37204 {2} | 0.38886 {6} | ||
∑Ranks | 43 {2} | 65 {7} | 23 {1} | 53 {5} | 46 {4} | 96 {8} | 44 {3} | 62 {6} | ||
80 | |BIAS| | ˆϑ | 0.65720 {2} | 0.73260 {6} | 0.64523 {1} | 0.71780 {4} | 0.67014 {3} | 0.78730 {8} | 0.72979 {5} | 0.78285 {7} |
ˆφ | 0.29918 {4} | 0.36951 {7} | 0.27270 {1} | 0.36572 {6} | 0.28461 {2} | 0.43485 {8} | 0.29440 {3} | 0.31911 {5} | ||
ˆλ | 0.06946 {2} | 0.08089 {7} | 0.06755 {1} | 0.07955 {6} | 0.07162 {4} | 0.07099 {3} | 0.07301 {5} | 0.08347 {8} | ||
ˆθ | 0.41705 {6} | 0.41605 {5} | 0.41277 {4} | 0.43216 {7} | 0.34179 {1} | 0.38656 {3} | 0.37952 {2} | 0.45183 {8} | ||
MSEs | ˆϑ | 0.53699 {1} | 0.65792 {5} | 0.71214 {7} | 0.63722 {3} | 0.62257 {2} | 0.71659 {8} | 0.64185 {4} | 0.70948 {6} | |
ˆφ | 0.12478 {3} | 0.19502 {7} | 0.11097 {1} | 0.18881 {6} | 0.12320 {2} | 0.28084 {8} | 0.13150 {4} | 0.15268 {5} | ||
ˆλ | 0.00672 {1} | 0.00884 {7} | 0.00693 {2} | 0.00853 {6} | 0.00712 {4} | 0.00711 {3} | 0.00740 {5} | 0.00932 {8} | ||
ˆθ | 0.26049 {5} | 0.25434 {4} | 0.30456 {8} | 0.27845 {6} | 0.16695 {1} | 0.24032 {3} | 0.20942 {2} | 0.29980 {7} | ||
MREs | ˆϑ | 0.37554 {2} | 0.41863 {6} | 0.21508 {1} | 0.41017 {4} | 0.38294 {3} | 0.44989 {8} | 0.41702 {5} | 0.44734 {7} | |
ˆφ | 0.59835 {4} | 0.73902 {7} | 0.54540 {1} | 0.73144 {6} | 0.56922 {2} | 0.86970 {8} | 0.58881 {3} | 0.63822 {5} | ||
ˆλ | 0.27786 {2} | 0.32356 {7} | 0.27019 {1} | 0.31820 {6} | 0.28647 {4} | 0.28396 {3} | 0.29205 {5} | 0.33388 {8} | ||
ˆθ | 0.33364 {6} | 0.33284 {5} | 0.33022 {4} | 0.34573 {7} | 0.27343 {1} | 0.30924 {3} | 0.30361 {2} | 0.36147 {8} | ||
∑Ranks | 38 {3} | 73 {7} | 32 {2} | 67 {6} | 29 {1} | 66 {5} | 45 {4} | 82 {8} | ||
200 | |BIAS| | ˆϑ | 0.54244 {1} | 0.74060 {6} | 0.66029 {4} | 0.72065 {5} | 0.56860 {2} | 0.78528 {8} | 0.64417 {3} | 0.74318 {7} |
ˆφ | 0.20343 {3} | 0.27714 {7} | 0.18758 {1} | 0.27628 {6} | 0.19120 {2} | 0.33648 {8} | 0.21264 {4} | 0.21990 {5} | ||
ˆλ | 0.05329 {1} | 0.07033 {7} | 0.05985 {3} | 0.06898 {6} | 0.05669 {2} | 0.05992 {4} | 0.06059 {5} | 0.07617 {8} | ||
ˆθ | 0.30910 {2} | 0.38666 {6} | 0.37834 {5} | 0.39240 {7} | 0.26754 {1} | 0.34697 {4} | 0.32167 {3} | 0.42492 {8} | ||
MSEs | ˆϑ | 0.39682 {1} | 0.65105 {6} | 0.72704 {8} | 0.62292 {4} | 0.51529 {2} | 0.70871 {7} | 0.53035 {3} | 0.65051 {5} | |
ˆφ | 0.06226 {3} | 0.11401 {7} | 0.05424 {1} | 0.11214 {6} | 0.05643 {2} | 0.18593 {8} | 0.06927 {4} | 0.07447 {5} | ||
ˆλ | 0.00405 {1} | 0.00665 {7} | 0.00571 {5} | 0.00644 {6} | 0.00452 {2} | 0.00513 {4} | 0.00507 {3} | 0.00753 {8} | ||
ˆθ | 0.14395 {2} | 0.20851 {5} | 0.26568 {8} | 0.22075 {6} | 0.10322 {1} | 0.19203 {4} | 0.14560 {3} | 0.25309 {7} | ||
MREs | ˆϑ | 0.30997 {2} | 0.42320 {6} | 0.22010 {1} | 0.41180 {5} | 0.32491 {3} | 0.44873 {8} | 0.36810 {4} | 0.42468 {7} | |
ˆφ | 0.40685 {3} | 0.55429 {7} | 0.37516 {1} | 0.55256 {6} | 0.38240 {2} | 0.67295 {8} | 0.42528 {4} | 0.43980 {5} | ||
ˆλ | 0.21316 {1} | 0.28134 {7} | 0.23940 {3} | 0.27593 {6} | 0.22675 {2} | 0.23969 {4} | 0.24238 {5} | 0.30470 {8} | ||
ˆθ | 0.24728 {2} | 0.30933 {6} | 0.30267 {5} | 0.31392 {7} | 0.21403 {1} | 0.27758 {4} | 0.25734 {3} | 0.33994 {8} | ||
∑Ranks | 22 {1.5} | 77 {7} | 45 {4} | 70 {5} | 22 {1.5} | 71 {6} | 44 {3} | 81 {8} | ||
500 | |BIAS| | ˆϑ | 0.40816 {2} | 0.63822 {6} | 0.59712 {4} | 0.62303 {5} | 0.38723 {1} | 0.72351 {8} | 0.50869 {3} | 0.64537 {7} |
ˆφ | 0.13392 {3} | 0.19775 {6} | 0.12778 {1} | 0.19966 {7} | 0.13208 {2} | 0.26446 {8} | 0.14927 {4} | 0.15660 {5} | ||
ˆλ | 0.03902 {1} | 0.05985 {7} | 0.04987 {4} | 0.05913 {6} | 0.04014 {2} | 0.05408 {5} | 0.04806 {3} | 0.06618 {8} | ||
ˆθ | 0.21255 {2} | 0.32416 {6} | 0.31285 {5} | 0.33146 {7} | 0.18308 {1} | 0.30762 {4} | 0.24975 {3} | 0.35803 {8} | ||
MSEs | ˆϑ | 0.24842 {1} | 0.52025 {5} | 0.58302 {7} | 0.50041 {4} | 0.33081 {2} | 0.63280 {8} | 0.37071 {3} | 0.52827 {6} | |
ˆφ | 0.02762 {3} | 0.05937 {6} | 0.02573 {1} | 0.06052 {7} | 0.02727 {2} | 0.12045 {8} | 0.03399 {4} | 0.03724 {5} | ||
ˆλ | 0.00224 {1} | 0.00478 {7} | 0.00422 {5} | 0.00470 {6} | 0.00257 {2} | 0.00402 {4} | 0.00325 {3} | 0.00569 {8} | ||
ˆθ | 0.06777 {2} | 0.14391 {5} | 0.19157 {8} | 0.15413 {6} | 0.05743 {1} | 0.14128 {4} | 0.08776 {3} | 0.17743 {7} | ||
MREs | ˆϑ | 0.23323 {3} | 0.36470 {6} | 0.19904 {1} | 0.35602 {5} | 0.22127 {2} | 0.41343 {8} | 0.29068 {4} | 0.36879 {7} | |
ˆφ | 0.26784 {3} | 0.39550 {6} | 0.25556 {1} | 0.39932 {7} | 0.26417 {2} | 0.52891 {8} | 0.29853 {4} | 0.31320 {5} | ||
ˆλ | 0.15609 {1} | 0.23939 {7} | 0.19949 {4} | 0.23651 {6} | 0.16056 {2} | 0.21632 {5} | 0.19222 {3} | 0.26474 {8} | ||
ˆθ | 0.17004 {2} | 0.25933 {6} | 0.25028 {5} | 0.26517 {7} | 0.14646 {1} | 0.24610 {4} | 0.19980 {3} | 0.28642 {8} | ||
∑Ranks | 24 {2} | 73 {5.5} | 46 {4} | 73 {5.5} | 20 {1} | 74 {7} | 40 {3} | 82 {8} |
n | Est. | Est. Par. | MLEs | LSEs | WLSEs | CRVMEs | MPSEs | PCEs | ADEs | RADEs |
20 | |BIAS| | ˆϑ | 0.58249 {3} | 0.53932 {1} | 0.56241 {2} | 0.61397 {5} | 0.60739 {4} | 0.82341 {8} | 0.68123 {7} | 0.66592 {6} |
ˆφ | 0.70201 {2} | 0.74992 {4} | 0.71005 {3} | 0.99334 {7} | 0.41044 {1} | 1.11769 {8} | 0.88784 {5} | 0.97457 {6} | ||
ˆλ | 0.26246 {1} | 0.28717 {3} | 0.28400 {2} | 0.42881 {5} | 0.40138 {4} | 0.64545 {8} | 0.43589 {7} | 0.43050 {6} | ||
ˆθ | 0.83485 {6} | 0.91523 {7} | 0.92408 {8} | 0.76222 {3} | 0.77841 {4} | 0.78092 {5} | 0.74535 {1} | 0.74828 {2} | ||
MSEs | ˆϑ | 0.45340 {3} | 0.42087 {1} | 0.44427 {2} | 0.51220 {4} | 0.51720 {5} | 0.77654 {8} | 0.58720 {7} | 0.56968 {6} | |
ˆφ | 0.65182 {1} | 0.75540 {3} | 0.66110 {2} | 1.25282 {7} | 0.95641 {4} | 1.49737 {8} | 1.01553 {5} | 1.19476 {6} | ||
ˆλ | 0.07059 {1} | 0.08751 {3} | 0.08543 {2} | 0.29909 {7} | 0.24742 {4} | 0.52940 {8} | 0.29252 {6} | 0.29154 {5} | ||
ˆθ | 0.84612 {6} | 1.00860 {8} | 1.00532 {7} | 0.71906 {3} | 0.75325 {4} | 0.82231 {5} | 0.69565 {1} | 0.70768 {2} | ||
MREs | ˆϑ | 0.33285 {3} | 0.30818 {1} | 0.32138 {2} | 0.35084 {5} | 0.34708 {4} | 0.47052 {8} | 0.38927 {7} | 0.38052 {6} | |
ˆφ | 0.35100 {1} | 0.37496 {3} | 0.35503 {2} | 0.49667 {7} | 0.42863 {4} | 0.55884 {8} | 0.44392 {5} | 0.48728 {6} | ||
ˆλ | 0.17497 {1} | 0.19145 {3} | 0.18933 {2} | 0.28587 {5} | 0.26758 {4} | 0.43030 {8} | 0.29059 {7} | 0.28700 {6} | ||
ˆθ | 0.33394 {6} | 0.36609 {7} | 0.36963 {8} | 0.30489 {3} | 0.31136 {4} | 0.31237 {5} | 0.29814 {1} | 0.29931 {2} | ||
∑Ranks | 34 {1} | 44 {3} | 42 {2} | 61 {7} | 46 {4} | 87 {8} | 59 {5.5} | 59 {5.5} | ||
80 | |BIAS| | ˆϑ | 0.49925 {2} | 0.47135 {1} | 0.52766 {3} | 0.66329 {5} | 0.55966 {4} | 0.89224 {8} | 0.67228 {6} | 0.69447 {7} |
ˆφ | 0.52063 {1} | 0.55025 {3} | 0.52909 {2} | 0.70683 {7} | 0.56354 {4} | 0.93582 {8} | 0.63998 {5} | 0.69954 {6} | ||
ˆλ | 0.25198 {1} | 0.25883 {3} | 0.25641 {2} | 0.43311 {7} | 0.30034 {4} | 0.66563 {8} | 0.40607 {5} | 0.43206 {6} | ||
ˆθ | 0.78480 {6} | 0.82532 {7} | 0.83697 {8} | 0.69063 {3} | 0.58559 {1} | 0.72122 {5} | 0.63842 {2} | 0.69164 {4} | ||
MSEs | ˆϑ | 0.31719 {2} | 0.30026 {1} | 0.34946 {3} | 0.55815 {5} | 0.49857 {4} | 0.86629 {8} | 0.56483 {6} | 0.59417 {7} | |
ˆφ | 0.27881 {1} | 0.32553 {3} | 0.29109 {2} | 0.66883 {7} | 0.44978 {4} | 1.03760 {8} | 0.55511 {5} | 0.64550 {6} | ||
ˆλ | 0.06369 {1} | 0.06795 {3} | 0.06642 {2} | 0.29117 {7} | 0.15725 {4} | 0.54984 {8} | 0.25250 {5} | 0.28331 {6} | ||
ˆθ | 0.66344 {5} | 0.73666 {7} | 0.74889 {8} | 0.58730 {3} | 0.45144 {1} | 0.66717 {6} | 0.51377 {2} | 0.59201 {4} | ||
MREs | ˆϑ | 0.28529 {2} | 0.26934 {1} | 0.30152 {3} | 0.37902 {5} | 0.31981 {4} | 0.50985 {8} | 0.38416 {6} | 0.39684 {7} | |
ˆφ | 0.26032 {1} | 0.27512 {3} | 0.26454 {2} | 0.35341 {7} | 0.28177 {4} | 0.46791 {8} | 0.31999 {5} | 0.34977 {6} | ||
ˆλ | 0.16798 {1} | 0.17255 {3} | 0.17094 {2} | 0.28874 {7} | 0.20023 {4} | 0.44375 {8} | 0.27071 {5} | 0.28804 {6} | ||
ˆθ | 0.31392 {6} | 0.33013 {7} | 0.33479 {8} | 0.27625 {3} | 0.23423 {1} | 0.28849 {5} | 0.25537 {2} | 0.27666 {4} | ||
∑Ranks | 29 {1} | 42 {3} | 45 {4} | 66 {6} | 39 {2} | 88 {8} | 54 {5} | 69 {7} | ||
200 | |BIAS| | ˆϑ | 0.47555 {3} | 0.44985 {2} | 0.51341 {4} | 0.67707 {6} | 0.42436 {1} | 0.89805 {8} | 0.66134 {5} | 0.69895 {7} |
ˆφ | 0.50115 {2} | 0.50837 {4} | 0.50279 {3} | 0.56296 {7} | 0.41044 {1} | 0.81679 {8} | 0.51451 {5} | 0.55936 {6} | ||
ˆλ | 0.25019 {2} | 0.25187 {4} | 0.25076 {3} | 0.41917 {7} | 0.21499 {1} | 0.65544 {8} | 0.37297 {5} | 0.41435 {6} | ||
ˆθ | 0.79159 {6} | 0.80107 {7} | 0.82123 {8} | 0.64647 {3} | 0.43028 {1} | 0.75308 {5} | 0.57181 {2} | 0.66234 {4} | ||
MSEs | ˆϑ | 0.25888 {2} | 0.23847 {1} | 0.29413 {3} | 0.56097 {6} | 0.37531 {4} | 0.86783 {8} | 0.54545 {5} | 0.59107 {7} | |
ˆφ | 0.25134 {1} | 0.26082 {4} | 0.25349 {2} | 0.43952 {7} | 0.25968 {3} | 0.80523 {8} | 0.37160 {5} | 0.43084 {6} | ||
ˆλ | 0.06261 {1} | 0.06361 {3} | 0.06295 {2} | 0.25891 {7} | 0.09970 {4} | 0.52619 {8} | 0.20829 {5} | 0.25203 {6} | ||
ˆθ | 0.64670 {5} | 0.66161 {6} | 0.69240 {8} | 0.51309 {3} | 0.27527 {1} | 0.68347 {7} | 0.41304 {2} | 0.53268 {4} | ||
MREs | ˆϑ | 0.27175 {3} | 0.25705 {2} | 0.29338 {4} | 0.38690 {6} | 0.24249 {1} | 0.51317 {8} | 0.37791 {5} | 0.39940 {7} | |
ˆφ | 0.25057 {2} | 0.25419 {4} | 0.25139 {3} | 0.28148 {7} | 0.20522 {1} | 0.40839 {8} | 0.25725 {5} | 0.27968 {6} | ||
ˆλ | 0.16679 {2} | 0.16791 {4} | 0.16717 {3} | 0.27945 {7} | 0.14333 {1} | 0.43696 {8} | 0.24865 {5} | 0.27623 {6} | ||
ˆθ | 0.31664 {6} | 0.32043 {7} | 0.32849 {8} | 0.25859 {3} | 0.17211 {1} | 0.30123 {5} | 0.22872 {2} | 0.26494 {4} | ||
∑Ranks | 35 {2} | 48 {3} | 51 {4.5} | 69 {6.5} | 20 {1} | 89 {8} | 51 {4.5} | 69 {6.5} | ||
500 | |BIAS| | ˆϑ | 0.47314 {3} | 0.44590 {2} | 0.51215 {4} | 0.64549 {6} | 0.23589 {1} | 0.89642 {8} | 0.57765 {5} | 0.66728 {7} |
ˆφ | 0.50000 {5} | 0.50031 {7} | 0.50001 {6} | 0.44580 {4} | 0.25497 {1} | 0.66140 {8} | 0.39329 {2} | 0.42269 {3} | ||
ˆλ | 0.25000 {2} | 0.25003 {4} | 0.25001 {3} | 0.38068 {6} | 0.11500 {1} | 0.63857 {8} | 0.31593 {5} | 0.38454 {7} | ||
ˆθ | 0.79445 {5} | 0.80134 {6} | 0.82604 {8} | 0.59243 {3} | 0.25280 {1} | 0.80505 {7} | 0.49356 {2} | 0.61821 {4} | ||
MSEs | ˆϑ | 0.23703 {3} | 0.21187 {2} | 0.27432 {4} | 0.51809 {6} | 0.19898 {1} | 0.86166 {8} | 0.44538 {5} | 0.54549 {7} | |
ˆφ | 0.25000 {3} | 0.25034 {5} | 0.25001 {4} | 0.28548 {7} | 0.11705 {1} | 0.56366 {8} | 0.22462 {2} | 0.25796 {6} | ||
ˆλ | 0.06250 {2.5} | 0.06252 {4} | 0.06250 {2.5} | 0.20888 {6} | 0.03935 {1} | 0.50179 {8} | 0.14873 {5} | 0.20922 {7} | ||
ˆθ | 0.63903 {5} | 0.64949 {6} | 0.68943 {7} | 0.43597 {3} | 0.12546 {1} | 0.74499 {8} | 0.31912 {2} | 0.46804 {4} | ||
MREs | ˆϑ | 0.27037 {3} | 0.25480 {2} | 0.29266 {4} | 0.36885 {6} | 0.13479 {1} | 0.51224 {8} | 0.33008 {5} | 0.38130 {7} | |
ˆφ | 0.25000 {5.5} | 0.25015 {7} | 0.25000 {5.5} | 0.22290 {4} | 0.12748 {1} | 0.33070 {8} | 0.19665 {2} | 0.21134 {3} | ||
ˆλ | 0.16667 {2.5} | 0.16669 {4} | 0.16667 {2.5} | 0.25379 {6} | 0.07667 {1} | 0.42571 {8} | 0.21062 {5} | 0.25636 {7} | ||
ˆθ | 0.31778 {5} | 0.32053 {6} | 0.33042 {8} | 0.23697 {3} | 0.10112 {1} | 0.32202 {7} | 0.19742 {2} | 0.24728 {4} | ||
∑Ranks | 44.5 {3} | 55 {4} | 58.5 {5} | 60 {6} | 12 {1} | 94 {8} | 42 {2} | 66 {7} |
n | Est. | Est. Par. | MLEs | LSEs | WLSEs | CRVMEs | MPSEs | PCEs | ADEs | RADEs |
20 | |BIAS| | ˆϑ | 1.05821 {8} | 0.62985 {3} | 0.96882 {6} | 0.61879 {2} | 0.39728 {1} | 1.00062 {7} | 0.93184 {5} | 0.74851 {4} |
ˆφ | 0.58239 {4} | 0.61540 {8} | 0.57588 {3} | 0.61442 {7} | 0.16810 {1} | 0.59648 {6} | 0.54321 {2} | 0.58344 {5} | ||
ˆλ | 0.09205 {8} | 0.08984 {5} | 0.08749 {3} | 0.09161 {6} | 0.07490 {1} | 0.09181 {7} | 0.08360 {2} | 0.08890 {4} | ||
ˆθ | 0.65193 {8} | 0.48317 {2} | 0.52978 {5} | 0.51841 {4} | 0.37874 {1} | 0.57738 {7} | 0.51808 {3} | 0.53685 {6} | ||
MSEs | ˆϑ | 1.62863 {8} | 0.80009 {2} | 1.26747 {6} | 0.83422 {3} | 0.44242 {1} | 1.52407 {7} | 1.26323 {5} | 1.02485 {4} | |
ˆφ | 0.42299 {3} | 0.47351 {8} | 0.43072 {4} | 0.47310 {7} | 0.39842 {2} | 0.45399 {6} | 0.39008 {1} | 0.43912 {5} | ||
ˆλ | 0.01191 {6} | 0.01157 {5} | 0.01104 {3} | 0.01199 {7} | 0.00839 {1} | 0.01226 {8} | 0.01037 {2} | 0.01130 {4} | ||
ˆθ | 0.56633 {8} | 0.35145 {2} | 0.39993 {3} | 0.40530 {5} | 0.22597 {1} | 0.46238 {7} | 0.39998 {4} | 0.42492 {6} | ||
MREs | ˆϑ | 0.30235 {8} | 0.17996 {3} | 0.27681 {6} | 0.17680 {2} | 0.11351 {1} | 0.28589 {7} | 0.26624 {5} | 0.21386 {4} | |
ˆφ | 0.77652 {4} | 0.82054 {8} | 0.76784 {3} | 0.81923 {7} | 0.73001 {2} | 0.79531 {6} | 0.72428 {1} | 0.77792 {5} | ||
ˆλ | 0.18410 {8} | 0.17968 {5} | 0.17498 {3} | 0.18321 {6} | 0.14981 {1} | 0.18363 {7} | 0.16720 {2} | 0.17780 {4} | ||
ˆθ | 0.52155 {8} | 0.38654 {2} | 0.42382 {5} | 0.41473 {4} | 0.30299 {1} | 0.46190 {7} | 0.41446 {3} | 0.42948 {6} | ||
∑Ranks | 81 {7} | 53 {4} | 50 {3} | 60 {6} | 14 {1} | 82 {8} | 35 {2} | 57 {5} | ||
80 | |BIAS| | ˆϑ | 1.30458 {8} | 0.99944 {2} | 1.19014 {7} | 1.02885 {3} | 0.28553 {1} | 1.10670 {4} | 1.14568 {6} | 1.11457 {5} |
ˆφ | 0.32983 {3} | 0.40620 {6} | 0.34267 {4} | 0.40746 {7} | 0.27616 {1} | 0.42471 {8} | 0.32604 {2} | 0.35934 {5} | ||
ˆλ | 0.06496 {7} | 0.06255 {5} | 0.05976 {3} | 0.06558 {8} | 0.03810 {1} | 0.06030 {4} | 0.05724 {2} | 0.06303 {6} | ||
ˆθ | 0.60157 {8} | 0.45112 {2} | 0.47825 {5} | 0.49541 {6} | 0.19099 {1} | 0.45370 {3} | 0.45453 {4} | 0.50033 {7} | ||
MSEs | ˆϑ | 1.98067 {8} | 1.42246 {2} | 1.62721 {7} | 1.51028 {3} | 0.28600 {1} | 1.54773 {5} | 1.54350 {4} | 1.62460 {6} | |
ˆφ | 0.16347 {2} | 0.24145 {7} | 0.17842 {4} | 0.24070 {6} | 0.12275 {1} | 0.27309 {8} | 0.16427 {3} | 0.19753 {5} | ||
ˆλ | 0.00614 {7} | 0.00590 {5} | 0.00549 {3} | 0.00646 {8} | 0.00224 {1} | 0.00582 {4} | 0.00513 {2} | 0.00600 {6} | ||
ˆθ | 0.50150 {8} | 0.33442 {3} | 0.35764 {5} | 0.39078 {6} | 0.06108 {1} | 0.33595 {4} | 0.33240 {2} | 0.39877 {7} | ||
MREs | ˆϑ | 0.37274 {8} | 0.28555 {2} | 0.34004 {7} | 0.29396 {3} | 0.08158 {1} | 0.31620 {4} | 0.32734 {6} | 0.31845 {5} | |
ˆφ | 0.43977 {3} | 0.54161 {6} | 0.45690 {4} | 0.54328 {7} | 0.36821 {1} | 0.56628 {8} | 0.43472 {2} | 0.47912 {5} | ||
ˆλ | 0.12992 {7} | 0.12509 {5} | 0.11952 {3} | 0.13116 {8} | 0.07620 {1} | 0.12061 {4} | 0.11448 {2} | 0.12607 {6} | ||
ˆθ | 0.48126 {8} | 0.36089 {2} | 0.38260 {5} | 0.39633 {6} | 0.15279 {1} | 0.36296 {3} | 0.36362 {4} | 0.40027 {7} | ||
∑Ranks | 77 {8} | 47 {3} | 57 {4} | 71 {7} | 12 {1} | 59 {5} | 39 {2} | 70 {6} | ||
200 | |BIAS| | ˆϑ | 1.18071 {8} | 1.13329 {4} | 1.15680 {6} | 1.15101 {5} | 0.12881 {1} | 1.09310 {2} | 1.11947 {3} | 1.17399 {7} |
ˆφ | 0.21288 {2} | 0.27282 {6} | 0.22827 {4} | 0.28207 {7} | 0.16810 {1} | 0.31936 {8} | 0.21687 {3} | 0.23691 {5} | ||
ˆλ | 0.05455 {5} | 0.05483 {6} | 0.05139 {4} | 0.05648 {8} | 0.02373 {1} | 0.04586 {2} | 0.05000 {3} | 0.05607 {7} | ||
ˆθ | 0.50995 {8} | 0.46810 {5} | 0.45161 {4} | 0.48892 {7} | 0.11088 {1} | 0.36948 {2} | 0.42847 {3} | 0.48519 {6} | ||
MSEs | ˆϑ | 1.65210 {6} | 1.59933 {5} | 1.54359 {4} | 1.65457 {7} | 0.11230 {1} | 1.41356 {2} | 1.45126 {3} | 1.65710 {8} | |
ˆφ | 0.06995 {2} | 0.11564 {6} | 0.08167 {4} | 0.12209 {7} | 0.04607 {1} | 0.16683 {8} | 0.07397 {3} | 0.08772 {5} | ||
ˆλ | 0.00441 {5} | 0.00457 {6} | 0.00403 {4} | 0.00483 {8} | 0.00088 {1} | 0.00341 {2} | 0.00386 {3} | 0.00477 {7} | ||
ˆθ | 0.38578 {8} | 0.35447 {5} | 0.32071 {4} | 0.38130 {7} | 0.02076 {1} | 0.24604 {2} | 0.29346 {3} | 0.38069 {6} | ||
MREs | ˆϑ | 0.33734 {8} | 0.32380 {4} | 0.33052 {6} | 0.32886 {5} | 0.03680 {1} | 0.31232 {2} | 0.31985 {3} | 0.33543 {7} | |
ˆφ | 0.28384 {2} | 0.36376 {6} | 0.30437 {4} | 0.37610 {7} | 0.22413 {1} | 0.42582 {8} | 0.28917 {3} | 0.31587 {5} | ||
ˆλ | 0.10910 {5} | 0.10967 {6} | 0.10278 {4} | 0.11295 {8} | 0.04745 {1} | 0.09173 {2} | 0.10000 {3} | 0.11214 {7} | ||
ˆθ | 0.40796 {8} | 0.37448 {5} | 0.36129 {4} | 0.39114 {7} | 0.08871 {1} | 0.29559 {2} | 0.34277 {3} | 0.38815 {6} | ||
∑Ranks | 67 {6} | 64 {5} | 52 {4} | 83 {8} | 12 {1} | 42 {3} | 36 {2} | 76 {7} | ||
500 | |BIAS| | ˆϑ | 0.99609 {2} | 1.12481 {6} | 1.05940 {5} | 1.12860 {7} | 0.04256 {1} | 1.04542 {4} | 1.03887 {3} | 1.14079 {8} |
ˆφ | 0.14496 {2} | 0.18941 {6} | 0.15844 {4} | 0.19306 {7} | 0.10411 {1} | 0.23525 {8} | 0.15349 {3} | 0.16453 {5} | ||
ˆλ | 0.04309 {3} | 0.05010 {7} | 0.04425 {5} | 0.04998 {6} | 0.01474 {1} | 0.03603 {2} | 0.04334 {4} | 0.05109 {8} | ||
ˆθ | 0.38900 {4} | 0.44670 {6} | 0.39875 {5} | 0.45266 {7} | 0.06374 {1} | 0.31824 {2} | 0.38560 {3} | 0.45867 {8} | ||
MSEs | ˆϑ | 1.21294 {2} | 1.50352 {6} | 1.31440 {5} | 1.52047 {8} | 0.02893 {1} | 1.25338 {3} | 1.26686 {4} | 1.51668 {7} | |
ˆφ | 0.03263 {2} | 0.05612 {6} | 0.03902 {4} | 0.05818 {7} | 0.01736 {1} | 0.09221 ^{\left\{8 \right\}} | 0.03639 ^{\left\{3 \right\}} | 0.04120 ^{\left\{5 \right\}} | ||
\hat{\lambda} | 0.00278 ^{\left\{3 \right\}} | 0.00378 ^{\left\{6.5 \right\}} | 0.00295 ^{\left\{5 \right\}} | 0.00378 ^{\left\{6.5 \right\}} | 0.00034 ^{\left\{1 \right\}} | 0.00220 ^{\left\{2 \right\}} | 0.00283 ^{\left\{4 \right\}} | 0.00400 ^{\left\{8 \right\}} | ||
\hat{\theta} | 0.23678 ^{\left\{4 \right\}} | 0.31580 ^{\left\{6 \right\}} | 0.25000 ^{\left\{5 \right\}} | 0.32430 ^{\left\{7 \right\}} | 0.00703 ^{\left\{1 \right\}} | 0.18536 ^{\left\{2 \right\}} | 0.23280 ^{\left\{3 \right\}} | 0.33477 ^{\left\{8 \right\}} | ||
MREs | \hat{\vartheta} | 0.28460 ^{\left\{2 \right\}} | 0.32137 ^{\left\{6 \right\}} | 0.30269 ^{\left\{5 \right\}} | 0.32246 ^{\left\{7 \right\}} | 0.01216 ^{\left\{1 \right\}} | 0.29869 ^{\left\{4 \right\}} | 0.29682 ^{\left\{3 \right\}} | 0.32594 ^{\left\{8 \right\}} | |
\hat{\varphi} | 0.19328 ^{\left\{2 \right\}} | 0.25255 ^{\left\{6 \right\}} | 0.21126 ^{\left\{4 \right\}} | 0.25741 ^{\left\{7 \right\}} | 0.13881 ^{\left\{1 \right\}} | 0.31367 ^{\left\{8 \right\}} | 0.20466 ^{\left\{3 \right\}} | 0.21938 ^{\left\{5 \right\}} | ||
\hat{\lambda} | 0.08617 ^{\left\{3 \right\}} | 0.10020 ^{\left\{7 \right\}} | 0.08850 ^{\left\{5 \right\}} | 0.09997 ^{\left\{6 \right\}} | 0.02948 ^{\left\{1 \right\}} | 0.07207 ^{\left\{2 \right\}} | 0.08669 ^{\left\{4 \right\}} | 0.10217 ^{\left\{8 \right\}} | ||
\hat{\theta} | 0.31120 ^{\left\{4 \right\}} | 0.35736 ^{\left\{6 \right\}} | 0.31900 ^{\left\{5 \right\}} | 0.36213 ^{\left\{7 \right\}} | 0.05100 ^{\left\{1 \right\}} | 0.25459 ^{\left\{2 \right\}} | 0.30848 ^{\left\{3 \right\}} | 0.36694 ^{\left\{8 \right\}} | ||
\sum{Ranks} | {\textbf{33}} ^{\left\{2 \right\}} | {\textbf{74.5}} ^{\left\{6 \right\}} | {\textbf{57}} ^{\left\{5 \right\}} | {\textbf{82.5}} ^{\left\{7 \right\}} | {\textbf{12}} ^{\left\{1 \right\}} | {\textbf{47}} ^{\left\{4 \right\}} | {\textbf{40}} ^{\left\{3 \right\}} | {\textbf{86}} ^{\left\{8 \right\}} |
n | Est. | Est. Par. | MLEs | LSEs | WLSEs | CRVMEs | MPSEs | PCEs | ADEs | RADEs |
20 | |BIAS| | \hat{\vartheta} | 0.98923 ^{\left\{7 \right\}} | 0.64331 ^{\left\{3 \right\}} | 0.95672 ^{\left\{6 \right\}} | 0.63413 ^{\left\{2 \right\}} | 0.44818 ^{\left\{1 \right\}} | 1.04755 ^{\left\{8 \right\}} | 0.90857 ^{\left\{5 \right\}} | 0.78916 ^{\left\{4 \right\}} |
\hat{\varphi} | 0.95367 ^{\left\{6 \right\}} | 0.90318 ^{\left\{4 \right\}} | 0.88553 ^{\left\{3 \right\}} | 0.95450 ^{\left\{7 \right\}} | 0.31253 ^{\left\{1 \right\}} | 0.97463 ^{\left\{8 \right\}} | 0.85825 ^{\left\{2 \right\}} | 0.93652 ^{\left\{5 \right\}} | ||
\hat{\lambda} | 0.50387 ^{\left\{8 \right\}} | 0.29370 ^{\left\{2 \right\}} | 0.38814 ^{\left\{5 \right\}} | 0.31558 ^{\left\{3 \right\}} | 0.23477 ^{\left\{1 \right\}} | 0.41787 ^{\left\{7 \right\}} | 0.38863 ^{\left\{6 \right\}} | 0.36144 ^{\left\{4 \right\}} | ||
\hat{\theta} | 0.83605 ^{\left\{8 \right\}} | 0.71444 ^{\left\{3 \right\}} | 0.81238 ^{\left\{7 \right\}} | 0.68991 ^{\left\{2 \right\}} | 0.62151 ^{\left\{1 \right\}} | 0.74404 ^{\left\{5 \right\}} | 0.75604 ^{\left\{6 \right\}} | 0.71561 ^{\left\{4 \right\}} | ||
MSEs | \hat{\vartheta} | 1.35323 ^{\left\{7 \right\}} | 0.71389 ^{\left\{2 \right\}} | 1.18225 ^{\left\{6 \right\}} | 0.73718 ^{\left\{3 \right\}} | 0.37586 ^{\left\{1 \right\}} | 1.57224 ^{\left\{8 \right\}} | 1.13154 ^{\left\{5 \right\}} | 1.02701 ^{\left\{4 \right\}} | |
\hat{\varphi} | 1.18857 ^{\left\{8 \right\}} | 1.06788 ^{\left\{4 \right\}} | 1.02750 ^{\left\{3 \right\}} | 1.18599 ^{\left\{7 \right\}} | 0.87512 ^{\left\{1 \right\}} | 1.15778 ^{\left\{6 \right\}} | 0.96462 ^{\left\{2 \right\}} | 1.13400 ^{\left\{5 \right\}} | ||
\hat{\lambda} | 0.36432 ^{\left\{8 \right\}} | 0.14714 ^{\left\{2 \right\}} | 0.22539 ^{\left\{5 \right\}} | 0.17712 ^{\left\{3 \right\}} | 0.09172 ^{\left\{1 \right\}} | 0.28638 ^{\left\{7 \right\}} | 0.23923 ^{\left\{6 \right\}} | 0.22267 ^{\left\{4 \right\}} | ||
\hat{\theta} | 0.77865 ^{\left\{8 \right\}} | 0.64926 ^{\left\{4 \right\}} | 0.77561 ^{\left\{7 \right\}} | 0.60519 ^{\left\{2 \right\}} | 0.52925 ^{\left\{1 \right\}} | 0.67762 ^{\left\{5 \right\}} | 0.68866 ^{\left\{6 \right\}} | 0.64046 ^{\left\{3 \right\}} | ||
MREs | \hat{\vartheta} | 0.28264 ^{\left\{7 \right\}} | 0.18380 ^{\left\{3 \right\}} | 0.27335 ^{\left\{6 \right\}} | 0.18118 ^{\left\{2 \right\}} | 0.12805 ^{\left\{1 \right\}} | 0.29930 ^{\left\{8 \right\}} | 0.25959 ^{\left\{5 \right\}} | 0.22547 ^{\left\{4 \right\}} | |
\hat{\varphi} | 0.47683 ^{\left\{6 \right\}} | 0.45159 ^{\left\{4 \right\}} | 0.44276 ^{\left\{3 \right\}} | 0.47725 ^{\left\{7 \right\}} | 0.40539 ^{\left\{1 \right\}} | 0.48731 ^{\left\{8 \right\}} | 0.42912 ^{\left\{2 \right\}} | 0.46826 ^{\left\{5 \right\}} | ||
\hat{\lambda} | 0.33591 ^{\left\{8 \right\}} | 0.19580 ^{\left\{2 \right\}} | 0.25876 ^{\left\{5 \right\}} | 0.21038 ^{\left\{3 \right\}} | 0.15651 ^{\left\{1 \right\}} | 0.27858 ^{\left\{7 \right\}} | 0.25908 ^{\left\{6 \right\}} | 0.24096 ^{\left\{4 \right\}} | ||
\hat{\theta} | 0.33442 ^{\left\{8 \right\}} | 0.28578 ^{\left\{3 \right\}} | 0.32495 ^{\left\{7 \right\}} | 0.27596 ^{\left\{2 \right\}} | 0.24860 ^{\left\{1 \right\}} | 0.29762 ^{\left\{5 \right\}} | 0.30242 ^{\left\{6 \right\}} | 0.28624 ^{\left\{4 \right\}} | ||
\sum{Ranks} | {\textbf{89}} ^{\left\{8 \right\}} | {\textbf{36}} ^{\left\{2 \right\}} | {\textbf{63}} ^{\left\{6 \right\}} | {\textbf{43}} ^{\left\{3 \right\}} | {\textbf{12}} ^{\left\{1 \right\}} | {\textbf{82}} ^{\left\{7 \right\}} | {\textbf{57}} ^{\left\{5 \right\}} | {\textbf{50}} ^{\left\{4 \right\}} | ||
80 | |BIAS| | \hat{\vartheta} | 1.00512 ^{\left\{8 \right\}} | 0.76116 ^{\left\{3 \right\}} | 0.98814 ^{\left\{7 \right\}} | 0.76068 ^{\left\{2 \right\}} | 0.22490 ^{\left\{1 \right\}} | 0.94177 ^{\left\{5 \right\}} | 0.95845 ^{\left\{6 \right\}} | 0.83406 ^{\left\{4 \right\}} |
\hat{\varphi} | 0.56247 ^{\left\{3 \right\}} | 0.64453 ^{\left\{6 \right\}} | 0.57353 ^{\left\{4 \right\}} | 0.64522 ^{\left\{7 \right\}} | 0.46925 ^{\left\{1 \right\}} | 0.76277 ^{\left\{8 \right\}} | 0.55915 ^{\left\{2 \right\}} | 0.63949 ^{\left\{5 \right\}} | ||
\hat{\lambda} | 0.45485 ^{\left\{8 \right\}} | 0.31115 ^{\left\{2 \right\}} | 0.36895 ^{\left\{6 \right\}} | 0.33000 ^{\left\{3 \right\}} | 0.11306 ^{\left\{1 \right\}} | 0.39112 ^{\left\{7 \right\}} | 0.36093 ^{\left\{5 \right\}} | 0.34405 ^{\left\{4 \right\}} | ||
\hat{\theta} | 0.76702 ^{\left\{8 \right\}} | 0.62063 ^{\left\{3 \right\}} | 0.68635 ^{\left\{7 \right\}} | 0.62598 ^{\left\{4 \right\}} | 0.33066 ^{\left\{1 \right\}} | 0.60384 ^{\left\{2 \right\}} | 0.66063 ^{\left\{6 \right\}} | 0.63130 ^{\left\{5 \right\}} | ||
MSEs | \hat{\vartheta} | 1.24539 ^{\left\{7 \right\}} | 0.92842 ^{\left\{2 \right\}} | 1.16588 ^{\left\{6 \right\}} | 0.94580 ^{\left\{3 \right\}} | 0.13919 ^{\left\{1 \right\}} | 1.25646 ^{\left\{8 \right\}} | 1.12647 ^{\left\{5 \right\}} | 1.05555 ^{\left\{4 \right\}} | |
\hat{\varphi} | 0.45689 ^{\left\{3 \right\}} | 0.57098 ^{\left\{6 \right\}} | 0.46410 ^{\left\{4 \right\}} | 0.57244 ^{\left\{7 \right\}} | 0.32482 ^{\left\{1 \right\}} | 0.71835 ^{\left\{8 \right\}} | 0.44181 ^{\left\{2 \right\}} | 0.55971 ^{\left\{5 \right\}} | ||
\hat{\lambda} | 0.28629 ^{\left\{8 \right\}} | 0.17260 ^{\left\{2 \right\}} | 0.20504 ^{\left\{5 \right\}} | 0.19582 ^{\left\{3 \right\}} | 0.02220 ^{\left\{1 \right\}} | 0.25122 ^{\left\{7 \right\}} | 0.20232 ^{\left\{4 \right\}} | 0.20735 ^{\left\{6 \right\}} | ||
\hat{\theta} | 0.68706 ^{\left\{8 \right\}} | 0.51170 ^{\left\{3 \right\}} | 0.58072 ^{\left\{7 \right\}} | 0.52071 ^{\left\{4 \right\}} | 0.16747 ^{\left\{1 \right\}} | 0.49400 ^{\left\{2 \right\}} | 0.55280 ^{\left\{6 \right\}} | 0.52733 ^{\left\{5 \right\}} | ||
MREs | \hat{\vartheta} | 0.28718 ^{\left\{8 \right\}} | 0.21747 ^{\left\{3 \right\}} | 0.28233 ^{\left\{7 \right\}} | 0.21734 ^{\left\{2 \right\}} | 0.06426 ^{\left\{1 \right\}} | 0.26908 ^{\left\{5 \right\}} | 0.27384 ^{\left\{6 \right\}} | 0.23830 ^{\left\{4 \right\}} | |
\hat{\varphi} | 0.28123 ^{\left\{3 \right\}} | 0.32226 ^{\left\{6 \right\}} | 0.28677 ^{\left\{4 \right\}} | 0.32261 ^{\left\{7 \right\}} | 0.23462 ^{\left\{1 \right\}} | 0.38138 ^{\left\{8 \right\}} | 0.27957 ^{\left\{2 \right\}} | 0.31974 ^{\left\{5 \right\}} | ||
\hat{\lambda} | 0.30323 ^{\left\{8 \right\}} | 0.20744 ^{\left\{2 \right\}} | 0.24597 ^{\left\{6 \right\}} | 0.22000 ^{\left\{3 \right\}} | 0.07538 ^{\left\{1 \right\}} | 0.26075 ^{\left\{7 \right\}} | 0.24062 ^{\left\{5 \right\}} | 0.22937 ^{\left\{4 \right\}} | ||
\hat{\theta} | 0.30681 ^{\left\{8 \right\}} | 0.24825 ^{\left\{3 \right\}} | 0.27454 ^{\left\{7 \right\}} | 0.25039 ^{\left\{4 \right\}} | 0.13226 ^{\left\{1 \right\}} | 0.24153 ^{\left\{2 \right\}} | 0.26425 ^{\left\{6 \right\}} | 0.25252 ^{\left\{5 \right\}} | ||
\sum{Ranks} | {\textbf{80}} ^{\left\{8 \right\}} | {\textbf{41}} ^{\left\{2 \right\}} | {\textbf{70}} ^{\left\{7 \right\}} | {\textbf{49}} ^{\left\{3 \right\}} | {\textbf{12}} ^{\left\{1 \right\}} | {\textbf{69}} ^{\left\{6 \right\}} | {\textbf{55}} ^{\left\{4 \right\}} | {\textbf{56}} ^{\left\{5 \right\}} | ||
200 | |BIAS| | \hat{\vartheta} | 0.98460 ^{\left\{6 \right\}} | 0.86500 ^{\left\{3 \right\}} | 1.00674 ^{\left\{7 \right\}} | 0.86298 ^{\left\{2 \right\}} | 0.12154 ^{\left\{1 \right\}} | 1.05269 ^{\left\{8 \right\}} | 0.98185 ^{\left\{5 \right\}} | 0.89235 ^{\left\{4 \right\}} |
\hat{\varphi} | 0.38859 ^{\left\{2 \right\}} | 0.45911 ^{\left\{5 \right\}} | 0.41129 ^{\left\{4 \right\}} | 0.46722 ^{\left\{6 \right\}} | 0.31253 ^{\left\{1 \right\}} | 0.67732 ^{\left\{8 \right\}} | 0.40329 ^{\left\{3 \right\}} | 0.46920 ^{\left\{7 \right\}} | ||
\hat{\lambda} | 0.39873 ^{\left\{8 \right\}} | 0.31023 ^{\left\{2 \right\}} | 0.35370 ^{\left\{6 \right\}} | 0.32546 ^{\left\{3 \right\}} | 0.06881 ^{\left\{1 \right\}} | 0.37938 ^{\left\{7 \right\}} | 0.34126 ^{\left\{5 \right\}} | 0.32900 ^{\left\{4 \right\}} | ||
\hat{\theta} | 0.68985 ^{\left\{8 \right\}} | 0.57483 ^{\left\{3 \right\}} | 0.64129 ^{\left\{7 \right\}} | 0.59868 ^{\left\{5 \right\}} | 0.21029 ^{\left\{1 \right\}} | 0.55195 ^{\left\{2 \right\}} | 0.61763 ^{\left\{6 \right\}} | 0.59808 ^{\left\{4 \right\}} | ||
MSEs | \hat{\vartheta} | 1.11626 ^{\left\{6 \right\}} | 1.01791 ^{\left\{2 \right\}} | 1.13048 ^{\left\{7 \right\}} | 1.02064 ^{\left\{3 \right\}} | 0.05498 ^{\left\{1 \right\}} | 1.30905 ^{\left\{8 \right\}} | 1.09868 ^{\left\{5 \right\}} | 1.05550 ^{\left\{4 \right\}} | |
\hat{\varphi} | 0.23053 ^{\left\{2 \right\}} | 0.31234 ^{\left\{5 \right\}} | 0.25573 ^{\left\{4 \right\}} | 0.32276 ^{\left\{6 \right\}} | 0.15678 ^{\left\{1 \right\}} | 0.58268 ^{\left\{8 \right\}} | 0.24649 ^{\left\{3 \right\}} | 0.32421 ^{\left\{7 \right\}} | ||
\hat{\lambda} | 0.22103 ^{\left\{7 \right\}} | 0.16583 ^{\left\{2 \right\}} | 0.18036 ^{\left\{5 \right\}} | 0.17985 ^{\left\{4 \right\}} | 0.00837 ^{\left\{1 \right\}} | 0.22479 ^{\left\{8 \right\}} | 0.17270 ^{\left\{3 \right\}} | 0.18259 ^{\left\{6 \right\}} | ||
\hat{\theta} | 0.58585 ^{\left\{8 \right\}} | 0.45832 ^{\left\{3 \right\}} | 0.51809 ^{\left\{7 \right\}} | 0.48976 ^{\left\{5 \right\}} | 0.07171 ^{\left\{1 \right\}} | 0.44322 ^{\left\{2 \right\}} | 0.49209 ^{\left\{6 \right\}} | 0.48968 ^{\left\{4 \right\}} | ||
MREs | \hat{\vartheta} | 0.28132 ^{\left\{6 \right\}} | 0.24714 ^{\left\{3 \right\}} | 0.28764 ^{\left\{7 \right\}} | 0.24657 ^{\left\{2 \right\}} | 0.03472 ^{\left\{1 \right\}} | 0.30077 ^{\left\{8 \right\}} | 0.28053 ^{\left\{5 \right\}} | 0.25496 ^{\left\{4 \right\}} | |
\hat{\varphi} | 0.19429 ^{\left\{2 \right\}} | 0.22956 ^{\left\{5 \right\}} | 0.20564 ^{\left\{4 \right\}} | 0.23361 ^{\left\{6 \right\}} | 0.15627 ^{\left\{1 \right\}} | 0.33866 ^{\left\{8 \right\}} | 0.20165 ^{\left\{3 \right\}} | 0.23460 ^{\left\{7 \right\}} | ||
\hat{\lambda} | 0.26582 ^{\left\{8 \right\}} | 0.20682 ^{\left\{2 \right\}} | 0.23580 ^{\left\{6 \right\}} | 0.21697 ^{\left\{3 \right\}} | 0.04587 ^{\left\{1 \right\}} | 0.25292 ^{\left\{7 \right\}} | 0.22751 ^{\left\{5 \right\}} | 0.21933 ^{\left\{4 \right\}} | ||
\hat{\theta} | 0.27594 ^{\left\{8 \right\}} | 0.22993 ^{\left\{3 \right\}} | 0.25652 ^{\left\{7 \right\}} | 0.23947 ^{\left\{5 \right\}} | 0.08412 ^{\left\{1 \right\}} | 0.22078 ^{\left\{2 \right\}} | 0.24705 ^{\left\{6 \right\}} | 0.23923 ^{\left\{4 \right\}} | ||
\sum{Ranks} | {\textbf{71}} ^{\left\{6.5 \right\}} | {\textbf{38}} ^{\left\{2 \right\}} | {\textbf{71}} ^{\left\{6.5 \right\}} | {\textbf{50}} ^{\left\{3 \right\}} | {\textbf{12}} ^{\left\{1 \right\}} | {\textbf{76}} ^{\left\{8 \right\}} | {\textbf{55}} ^{\left\{4 \right\}} | {\textbf{59}} ^{\left\{5 \right\}} | ||
500 | |BIAS| | \hat{\vartheta} | 0.93304 ^{\left\{5 \right\}} | 0.93263 ^{\left\{4 \right\}} | 0.97539 ^{\left\{7 \right\}} | 0.92961 ^{\left\{3 \right\}} | 0.05348 ^{\left\{1 \right\}} | 1.08240 ^{\left\{8 \right\}} | 0.95522 ^{\left\{6 \right\}} | 0.92527 ^{\left\{2 \right\}} |
\hat{\varphi} | 0.27572 ^{\left\{2 \right\}} | 0.32936 ^{\left\{5 \right\}} | 0.29210 ^{\left\{4 \right\}} | 0.33160 ^{\left\{6 \right\}} | 0.19113 ^{\left\{1 \right\}} | 0.58412 ^{\left\{8 \right\}} | 0.28766 ^{\left\{3 \right\}} | 0.33820 ^{\left\{7 \right\}} | ||
\hat{\lambda} | 0.34882 ^{\left\{7 \right\}} | 0.31976 ^{\left\{2 \right\}} | 0.33838 ^{\left\{6 \right\}} | 0.33117 ^{\left\{5 \right\}} | 0.03908 ^{\left\{1 \right\}} | 0.35124 ^{\left\{8 \right\}} | 0.32736 ^{\left\{3 \right\}} | 0.32969 ^{\left\{4 \right\}} | ||
\hat{\theta} | 0.61892 ^{\left\{8 \right\}} | 0.57580 ^{\left\{3 \right\}} | 0.60923 ^{\left\{7 \right\}} | 0.59341 ^{\left\{6 \right\}} | 0.12272 ^{\left\{1 \right\}} | 0.51040 ^{\left\{2 \right\}} | 0.59246 ^{\left\{5 \right\}} | 0.58416 ^{\left\{4 \right\}} | ||
MSEs | \hat{\vartheta} | 0.99386 ^{\left\{2 \right\}} | 1.04383 ^{\left\{6 \right\}} | 1.04849 ^{\left\{7 \right\}} | 1.04352 ^{\left\{5 \right\}} | 0.00732 ^{\left\{1 \right\}} | 1.28318 ^{\left\{8 \right\}} | 1.01861 ^{\left\{3 \right\}} | 1.03056 ^{\left\{4 \right\}} | |
\hat{\varphi} | 0.11591 ^{\left\{2 \right\}} | 0.16651 ^{\left\{5 \right\}} | 0.13079 ^{\left\{4 \right\}} | 0.16807 ^{\left\{6 \right\}} | 0.06394 ^{\left\{1 \right\}} | 0.45248 ^{\left\{8 \right\}} | 0.12739 ^{\left\{3 \right\}} | 0.17250 ^{\left\{7 \right\}} | ||
\hat{\lambda} | 0.17000 ^{\left\{7 \right\}} | 0.15789 ^{\left\{3 \right\}} | 0.15993 ^{\left\{4 \right\}} | 0.16663 ^{\left\{5 \right\}} | 0.00250 ^{\left\{1 \right\}} | 0.18698 ^{\left\{8 \right\}} | 0.15186 ^{\left\{2 \right\}} | 0.16915 ^{\left\{6 \right\}} | ||
\hat{\theta} | 0.48714 ^{\left\{8 \right\}} | 0.45146 ^{\left\{4 \right\}} | 0.47250 ^{\left\{6 \right\}} | 0.47497 ^{\left\{7 \right\}} | 0.02504 ^{\left\{1 \right\}} | 0.39915 ^{\left\{2 \right\}} | 0.45105 ^{\left\{3 \right\}} | 0.47078 ^{\left\{5 \right\}} | ||
MREs | \hat{\vartheta} | 0.26658 ^{\left\{5 \right\}} | 0.26647 ^{\left\{4 \right\}} | 0.27868 ^{\left\{7 \right\}} | 0.26560 ^{\left\{3 \right\}} | 0.01528 ^{\left\{1 \right\}} | 0.30926 ^{\left\{8 \right\}} | 0.27292 ^{\left\{6 \right\}} | 0.26436 ^{\left\{2 \right\}} | |
\hat{\varphi} | 0.13786 ^{\left\{2 \right\}} | 0.16468 ^{\left\{5 \right\}} | 0.14605 ^{\left\{4 \right\}} | 0.16580 ^{\left\{6 \right\}} | 0.09557 ^{\left\{1 \right\}} | 0.29206 ^{\left\{8 \right\}} | 0.14383 ^{\left\{3 \right\}} | 0.16910 ^{\left\{7 \right\}} | ||
\hat{\lambda} | 0.23255 ^{\left\{7 \right\}} | 0.21318 ^{\left\{2 \right\}} | 0.22559 ^{\left\{6 \right\}} | 0.22078 ^{\left\{5 \right\}} | 0.02606 ^{\left\{1 \right\}} | 0.23416 ^{\left\{8 \right\}} | 0.21824 ^{\left\{3 \right\}} | 0.21980 ^{\left\{4 \right\}} | ||
\hat{\theta} | 0.24757 ^{\left\{8 \right\}} | 0.23032 ^{\left\{3 \right\}} | 0.24369 ^{\left\{7 \right\}} | 0.23736 ^{\left\{6 \right\}} | 0.04909 ^{\left\{1 \right\}} | 0.20416 ^{\left\{2 \right\}} | 0.23698 ^{\left\{5 \right\}} | 0.23367 ^{\left\{4 \right\}} | ||
\sum{Ranks} | {\textbf{63}} ^{\left\{5.5 \right\}} | {\textbf{46}} ^{\left\{3 \right\}} | {\textbf{69}} ^{\left\{7 \right\}} | {\textbf{63}} ^{\left\{5.5 \right\}} | {\textbf{12}} ^{\left\{1 \right\}} | {\textbf{78}} ^{\left\{8 \right\}} | {\textbf{45}} ^{\left\{2 \right\}} | {\textbf{56}} ^{\left\{4 \right\}} |
n | Est. | Est. Par. | MLEs | LSEs | WLSEs | CRVMEs | MPSEs | PCEs | ADEs | RADEs |
20 | |BIAS| | \hat{\vartheta} | 1.25197 ^{\left\{8 \right\}} | 0.45438 ^{\left\{3 \right\}} | 1.06521 ^{\left\{7 \right\}} | 0.44888 ^{\left\{2 \right\}} | 0.20680 ^{\left\{1 \right\}} | 0.83805 ^{\left\{5 \right\}} | 1.02528 ^{\left\{6 \right\}} | 0.62513 ^{\left\{4 \right\}} |
\hat{\varphi} | 0.58123 ^{\left\{5 \right\}} | 0.60203 ^{\left\{7 \right\}} | 0.57995 ^{\left\{4 \right\}} | 0.61278 ^{\left\{8 \right\}} | 0.16575 ^{\left\{1 \right\}} | 0.57749 ^{\left\{3 \right\}} | 0.53508 ^{\left\{2 \right\}} | 0.58165 ^{\left\{6 \right\}} | ||
\hat{\lambda} | 0.08854 ^{\left\{8 \right\}} | 0.07652 ^{\left\{2 \right\}} | 0.08227 ^{\left\{7 \right\}} | 0.07831 ^{\left\{3 \right\}} | 0.06474 ^{\left\{1 \right\}} | 0.08065 ^{\left\{6 \right\}} | 0.07939 ^{\left\{4 \right\}} | 0.07980 ^{\left\{5 \right\}} | ||
\hat{\theta} | 0.66759 ^{\left\{8 \right\}} | 0.42262 ^{\left\{2 \right\}} | 0.53041 ^{\left\{7 \right\}} | 0.44839 ^{\left\{3 \right\}} | 0.32466 ^{\left\{1 \right\}} | 0.51024 ^{\left\{5 \right\}} | 0.52797 ^{\left\{6 \right\}} | 0.48959 ^{\left\{4 \right\}} | ||
MSEs | \hat{\vartheta} | 2.41625 ^{\left\{8 \right\}} | 0.60512 ^{\left\{2 \right\}} | 1.67131 ^{\left\{6 \right\}} | 0.67908 ^{\left\{3 \right\}} | 0.18285 ^{\left\{1 \right\}} | 1.58289 ^{\left\{5 \right\}} | 1.74298 ^{\left\{7 \right\}} | 1.01407 ^{\left\{4 \right\}} | |
\hat{\varphi} | 0.42148 ^{\left\{3 \right\}} | 0.46192 ^{\left\{7 \right\}} | 0.43565 ^{\left\{5 \right\}} | 0.46874 ^{\left\{8 \right\}} | 0.38218 ^{\left\{1 \right\}} | 0.43432 ^{\left\{4 \right\}} | 0.38271 ^{\left\{2 \right\}} | 0.43679 ^{\left\{6 \right\}} | ||
\hat{\lambda} | 0.01072 ^{\left\{8 \right\}} | 0.00870 ^{\left\{2 \right\}} | 0.00963 ^{\left\{7 \right\}} | 0.00906 ^{\left\{3 \right\}} | 0.00620 ^{\left\{1 \right\}} | 0.00950 ^{\left\{6 \right\}} | 0.00923 ^{\left\{5 \right\}} | 0.00919 ^{\left\{4 \right\}} | ||
\hat{\theta} | 0.58894 ^{\left\{8 \right\}} | 0.28693 ^{\left\{2 \right\}} | 0.40885 ^{\left\{6 \right\}} | 0.32249 ^{\left\{3 \right\}} | 0.16767 ^{\left\{1 \right\}} | 0.38646 ^{\left\{5 \right\}} | 0.41599 ^{\left\{7 \right\}} | 0.36863 ^{\left\{4 \right\}} | ||
MREs | \hat{\vartheta} | 0.27821 ^{\left\{8 \right\}} | 0.10097 ^{\left\{3 \right\}} | 0.23671 ^{\left\{7 \right\}} | 0.09975 ^{\left\{2 \right\}} | 0.04596 ^{\left\{1 \right\}} | 0.18623 ^{\left\{5 \right\}} | 0.22784 ^{\left\{6 \right\}} | 0.13892 ^{\left\{4 \right\}} | |
\hat{\varphi} | 0.77497 ^{\left\{5 \right\}} | 0.80271 ^{\left\{7 \right\}} | 0.77327 ^{\left\{4 \right\}} | 0.81704 ^{\left\{8 \right\}} | 0.70903 ^{\left\{1 \right\}} | 0.76998 ^{\left\{3 \right\}} | 0.71344 ^{\left\{2 \right\}} | 0.77554 ^{\left\{6 \right\}} | ||
\hat{\lambda} | 0.17707 ^{\left\{8 \right\}} | 0.15303 ^{\left\{2 \right\}} | 0.16453 ^{\left\{7 \right\}} | 0.15662 ^{\left\{3 \right\}} | 0.12948 ^{\left\{1 \right\}} | 0.16130 ^{\left\{6 \right\}} | 0.15879 ^{\left\{4 \right\}} | 0.15960 ^{\left\{5 \right\}} | ||
\hat{\theta} | 0.53407 ^{\left\{8 \right\}} | 0.33809 ^{\left\{2 \right\}} | 0.42432 ^{\left\{7 \right\}} | 0.35871 ^{\left\{3 \right\}} | 0.25973 ^{\left\{1 \right\}} | 0.40819 ^{\left\{5 \right\}} | 0.42237 ^{\left\{6 \right\}} | 0.39167 ^{\left\{4 \right\}} | ||
\sum{Ranks} | {\textbf{85}} ^{\left\{8 \right\}} | {\textbf{41}} ^{\left\{2 \right\}} | {\textbf{74}} ^{\left\{7 \right\}} | {\textbf{49}} ^{\left\{3 \right\}} | {\textbf{12}} ^{\left\{1 \right\}} | {\textbf{58}} ^{\left\{6 \right\}} | {\textbf{57}} ^{\left\{5 \right\}} | {\textbf{56}} ^{\left\{4 \right\}} | ||
80 | |BIAS| | \hat{\vartheta} | 1.62748 ^{\left\{8 \right\}} | 0.91367 ^{\left\{2 \right\}} | 1.37823 ^{\left\{7 \right\}} | 0.98139 ^{\left\{3 \right\}} | 0.11224 ^{\left\{1 \right\}} | 1.13720 ^{\left\{5 \right\}} | 1.31803 ^{\left\{6 \right\}} | 1.11805 ^{\left\{4 \right\}} |
\hat{\varphi} | 0.31837 ^{\left\{3 \right\}} | 0.39968 ^{\left\{7 \right\}} | 0.34474 ^{\left\{4 \right\}} | 0.40380 ^{\left\{8 \right\}} | 0.27352 ^{\left\{1 \right\}} | 0.37466 ^{\left\{6 \right\}} | 0.31821 ^{\left\{2 \right\}} | 0.35079 ^{\left\{5 \right\}} | ||
\hat{\lambda} | 0.07169 ^{\left\{8 \right\}} | 0.05804 ^{\left\{3 \right\}} | 0.06253 ^{\left\{7 \right\}} | 0.06113 ^{\left\{6 \right\}} | 0.03286 ^{\left\{1 \right\}} | 0.05699 ^{\left\{2 \right\}} | 0.05980 ^{\left\{5 \right\}} | 0.05870 ^{\left\{4 \right\}} | ||
\hat{\theta} | 0.64853 ^{\left\{8 \right\}} | 0.39956 ^{\left\{2 \right\}} | 0.49396 ^{\left\{7 \right\}} | 0.44278 ^{\left\{4 \right\}} | 0.16292 ^{\left\{1 \right\}} | 0.43570 ^{\left\{3 \right\}} | 0.47512 ^{\left\{6 \right\}} | 0.45276 ^{\left\{5 \right\}} | ||
MSEs | \hat{\vartheta} | 3.14585 ^{\left\{8 \right\}} | 1.58782 ^{\left\{2 \right\}} | 2.33956 ^{\left\{7 \right\}} | 1.79431 ^{\left\{3 \right\}} | 0.08679 ^{\left\{1 \right\}} | 1.96177 ^{\left\{4 \right\}} | 2.22845 ^{\left\{6 \right\}} | 2.05141 ^{\left\{5 \right\}} | |
\hat{\varphi} | 0.15254 ^{\left\{2 \right\}} | 0.23761 ^{\left\{7 \right\}} | 0.18156 ^{\left\{4 \right\}} | 0.24031 ^{\left\{8 \right\}} | 0.12335 ^{\left\{1 \right\}} | 0.22013 ^{\left\{6 \right\}} | 0.15798 ^{\left\{3 \right\}} | 0.19064 ^{\left\{5 \right\}} | ||
\hat{\lambda} | 0.00707 ^{\left\{8 \right\}} | 0.00520 ^{\left\{4 \right\}} | 0.00579 ^{\left\{7 \right\}} | 0.00566 ^{\left\{6 \right\}} | 0.00171 ^{\left\{1 \right\}} | 0.00514 ^{\left\{2 \right\}} | 0.00541 ^{\left\{5 \right\}} | 0.00519 ^{\left\{3 \right\}} | ||
\hat{\theta} | 0.56658 ^{\left\{8 \right\}} | 0.28713 ^{\left\{2 \right\}} | 0.38674 ^{\left\{7 \right\}} | 0.34041 ^{\left\{4 \right\}} | 0.04197 ^{\left\{1 \right\}} | 0.32550 ^{\left\{3 \right\}} | 0.36722 ^{\left\{6 \right\}} | 0.35156 ^{\left\{5 \right\}} | ||
MREs | \hat{\vartheta} | 0.36166 ^{\left\{8 \right\}} | 0.20304 ^{\left\{2 \right\}} | 0.30627 ^{\left\{7 \right\}} | 0.21809 ^{\left\{3 \right\}} | 0.02494 ^{\left\{1 \right\}} | 0.25271 ^{\left\{5 \right\}} | 0.29289 ^{\left\{6 \right\}} | 0.24846 ^{\left\{4 \right\}} | |
\hat{\varphi} | 0.42449 ^{\left\{3 \right\}} | 0.53291 ^{\left\{7 \right\}} | 0.45966 ^{\left\{4 \right\}} | 0.53840 ^{\left\{8 \right\}} | 0.36469 ^{\left\{1 \right\}} | 0.49955 ^{\left\{6 \right\}} | 0.42429 ^{\left\{2 \right\}} | 0.46771 ^{\left\{5 \right\}} | ||
\hat{\lambda} | 0.14337 ^{\left\{8 \right\}} | 0.11609 ^{\left\{3 \right\}} | 0.12506 ^{\left\{7 \right\}} | 0.12226 ^{\left\{6 \right\}} | 0.06572 ^{\left\{1 \right\}} | 0.11399 ^{\left\{2 \right\}} | 0.11961 ^{\left\{5 \right\}} | 0.11740 ^{\left\{4 \right\}} | ||
\hat{\theta} | 0.51882 ^{\left\{8 \right\}} | 0.31965 ^{\left\{2 \right\}} | 0.39517 ^{\left\{7 \right\}} | 0.35422 ^{\left\{4 \right\}} | 0.13034 ^{\left\{1 \right\}} | 0.34856 ^{\left\{3 \right\}} | 0.38010 ^{\left\{6 \right\}} | 0.36221 ^{\left\{5 \right\}} | ||
\sum{Ranks} | {\textbf{80}} ^{\left\{8 \right\}} | {\textbf{43}} ^{\left\{2 \right\}} | {\textbf{75}} ^{\left\{7 \right\}} | {\textbf{63}} ^{\left\{6 \right\}} | {\textbf{12}} ^{\left\{1 \right\}} | {\textbf{47}} ^{\left\{3 \right\}} | {\textbf{58}} ^{\left\{5 \right\}} | {\textbf{54}} ^{\left\{4 \right\}} | ||
200 | |BIAS| | \hat{\vartheta} | 1.48139 ^{\left\{8 \right\}} | 1.17284 ^{\left\{2 \right\}} | 1.40391 ^{\left\{7 \right\}} | 1.21294 ^{\left\{4 \right\}} | 0.04307 ^{\left\{1 \right\}} | 1.17950 ^{\left\{3 \right\}} | 1.33404 ^{\left\{6 \right\}} | 1.29174 ^{\left\{5 \right\}} |
\hat{\varphi} | 0.20186 ^{\left\{2 \right\}} | 0.26830 ^{\left\{7 \right\}} | 0.21385 ^{\left\{4 \right\}} | 0.26575 ^{\left\{6 \right\}} | 0.16575 ^{\left\{1 \right\}} | 0.27408 ^{\left\{8 \right\}} | 0.20558 ^{\left\{3 \right\}} | 0.23166 ^{\left\{5 \right\}} | ||
\hat{\lambda} | 0.06056 ^{\left\{8 \right\}} | 0.05260 ^{\left\{3 \right\}} | 0.05573 ^{\left\{7 \right\}} | 0.05571 ^{\left\{6 \right\}} | 0.02038 ^{\left\{1 \right\}} | 0.04542 ^{\left\{2 \right\}} | 0.05309 ^{\left\{4 \right\}} | 0.05470 ^{\left\{5 \right\}} | ||
\hat{\theta} | 0.54563 ^{\left\{8 \right\}} | 0.42847 ^{\left\{3 \right\}} | 0.47838 ^{\left\{7 \right\}} | 0.45810 ^{\left\{5 \right\}} | 0.09746 ^{\left\{1 \right\}} | 0.36816 ^{\left\{2 \right\}} | 0.44966 ^{\left\{4 \right\}} | 0.46232 ^{\left\{6 \right\}} | ||
MSEs | \hat{\vartheta} | 2.63270 ^{\left\{8 \right\}} | 2.08923 ^{\left\{3 \right\}} | 2.37558 ^{\left\{7 \right\}} | 2.20468 ^{\left\{5 \right\}} | 0.02535 ^{\left\{1 \right\}} | 1.86391 ^{\left\{2 \right\}} | 2.18628 ^{\left\{4 \right\}} | 2.32684 ^{\left\{6 \right\}} | |
\hat{\varphi} | 0.06343 ^{\left\{2 \right\}} | 0.11259 ^{\left\{7 \right\}} | 0.07126 ^{\left\{4 \right\}} | 0.11064 ^{\left\{6 \right\}} | 0.04499 ^{\left\{1 \right\}} | 0.12626 ^{\left\{8 \right\}} | 0.06649 ^{\left\{3 \right\}} | 0.08397 ^{\left\{5 \right\}} | ||
\hat{\lambda} | 0.00531 ^{\left\{8 \right\}} | 0.00437 ^{\left\{3 \right\}} | 0.00472 ^{\left\{6 \right\}} | 0.00486 ^{\left\{7 \right\}} | 0.00066 ^{\left\{1 \right\}} | 0.00347 ^{\left\{2 \right\}} | 0.00441 ^{\left\{4 \right\}} | 0.00470 ^{\left\{5 \right\}} | ||
\hat{\theta} | 0.44122 ^{\left\{8 \right\}} | 0.33416 ^{\left\{3 \right\}} | 0.37078 ^{\left\{5 \right\}} | 0.37139 ^{\left\{6 \right\}} | 0.01519 ^{\left\{1 \right\}} | 0.25838 ^{\left\{2 \right\}} | 0.33754 ^{\left\{4 \right\}} | 0.37704 ^{\left\{7 \right\}} | ||
MREs | \hat{\vartheta} | 0.32920 ^{\left\{8 \right\}} | 0.26063 ^{\left\{2 \right\}} | 0.31198 ^{\left\{7 \right\}} | 0.26954 ^{\left\{4 \right\}} | 0.00957 ^{\left\{1 \right\}} | 0.26211 ^{\left\{3 \right\}} | 0.29645 ^{\left\{6 \right\}} | 0.28705 ^{\left\{5 \right\}} | |
\hat{\varphi} | 0.26915 ^{\left\{2 \right\}} | 0.35774 ^{\left\{7 \right\}} | 0.28513 ^{\left\{4 \right\}} | 0.35434 ^{\left\{6 \right\}} | 0.22100 ^{\left\{1 \right\}} | 0.36544 ^{\left\{8 \right\}} | 0.27411 ^{\left\{3 \right\}} | 0.30888 ^{\left\{5 \right\}} | ||
\hat{\lambda} | 0.12113 ^{\left\{8 \right\}} | 0.10520 ^{\left\{3 \right\}} | 0.11146 ^{\left\{7 \right\}} | 0.11142 ^{\left\{6 \right\}} | 0.04075 ^{\left\{1 \right\}} | 0.09084 ^{\left\{2 \right\}} | 0.10618 ^{\left\{4 \right\}} | 0.10941 ^{\left\{5 \right\}} | ||
\hat{\theta} | 0.43650 ^{\left\{8 \right\}} | 0.34278 ^{\left\{3 \right\}} | 0.38270 ^{\left\{7 \right\}} | 0.36648 ^{\left\{5 \right\}} | 0.07797 ^{\left\{1 \right\}} | 0.29453 ^{\left\{2 \right\}} | 0.35973 ^{\left\{4 \right\}} | 0.36986 ^{\left\{6 \right\}} | ||
\sum{Ranks} | {\textbf{78}} ^{\left\{8 \right\}} | {\textbf{46}} ^{\left\{3 \right\}} | {\textbf{72}} ^{\left\{7 \right\}} | {\textbf{66}} ^{\left\{6 \right\}} | {\textbf{12}} ^{\left\{1 \right\}} | {\textbf{44}} ^{\left\{2 \right\}} | {\textbf{49}} ^{\left\{4 \right\}} | {\textbf{65}} ^{\left\{5 \right\}} | ||
500 | |BIAS| | \hat{\vartheta} | 1.29672 ^{\left\{5 \right\}} | 1.27235 ^{\left\{3 \right\}} | 1.31237 ^{\left\{6 \right\}} | 1.32513 ^{\left\{7 \right\}} | 0.01519 ^{\left\{1 \right\}} | 1.15120 ^{\left\{2 \right\}} | 1.28994 ^{\left\{4 \right\}} | 1.34189 ^{\left\{8 \right\}} |
\hat{\varphi} | 0.13744 ^{\left\{2 \right\}} | 0.17802 ^{\left\{6 \right\}} | 0.14815 ^{\left\{4 \right\}} | 0.18664 ^{\left\{7 \right\}} | 0.10227 ^{\left\{1 \right\}} | 0.19392 ^{\left\{8 \right\}} | 0.14640 ^{\left\{3 \right\}} | 0.15865 ^{\left\{5 \right\}} | ||
\hat{\lambda} | 0.04970 ^{\left\{5 \right\}} | 0.05107 ^{\left\{6 \right\}} | 0.04913 ^{\left\{4 \right\}} | 0.05400 ^{\left\{8 \right\}} | 0.01264 ^{\left\{1 \right\}} | 0.03665 ^{\left\{2 \right\}} | 0.04825 ^{\left\{3 \right\}} | 0.05335 ^{\left\{7 \right\}} | ||
\hat{\theta} | 0.44102 ^{\left\{5 \right\}} | 0.44509 ^{\left\{6 \right\}} | 0.43050 ^{\left\{4 \right\}} | 0.47518 ^{\left\{8 \right\}} | 0.05962 ^{\left\{1 \right\}} | 0.31796 ^{\left\{2 \right\}} | 0.42160 ^{\left\{3 \right\}} | 0.46945 ^{\left\{7 \right\}} | ||
MSEs | \hat{\vartheta} | 2.07266 ^{\left\{4 \right\}} | 2.19148 ^{\left\{6 \right\}} | 2.07839 ^{\left\{5 \right\}} | 2.34226 ^{\left\{8 \right\}} | 0.00344 ^{\left\{1 \right\}} | 1.67247 ^{\left\{2 \right\}} | 2.02330 ^{\left\{3 \right\}} | 2.31957 ^{\left\{7 \right\}} | |
\hat{\varphi} | 0.02955 ^{\left\{2 \right\}} | 0.05030 ^{\left\{6 \right\}} | 0.03417 ^{\left\{4 \right\}} | 0.05288 ^{\left\{7 \right\}} | 0.01679 ^{\left\{1 \right\}} | 0.06276 ^{\left\{8 \right\}} | 0.03295 ^{\left\{3 \right\}} | 0.03874 ^{\left\{5 \right\}} | ||
\hat{\lambda} | 0.00377 ^{\left\{4 \right\}} | 0.00418 ^{\left\{6 \right\}} | 0.00378 ^{\left\{5 \right\}} | 0.00452 ^{\left\{7 \right\}} | 0.00025 ^{\left\{1 \right\}} | 0.00242 ^{\left\{2 \right\}} | 0.00363 ^{\left\{3 \right\}} | 0.00454 ^{\left\{8 \right\}} | ||
\hat{\theta} | 0.30987 ^{\left\{5 \right\}} | 0.34423 ^{\left\{6 \right\}} | 0.30505 ^{\left\{4 \right\}} | 0.37795 ^{\left\{8 \right\}} | 0.00564 ^{\left\{1 \right\}} | 0.19875 ^{\left\{2 \right\}} | 0.29242 ^{\left\{3 \right\}} | 0.37470 ^{\left\{7 \right\}} | ||
MREs | \hat{\vartheta} | 0.28816 ^{\left\{5 \right\}} | 0.28275 ^{\left\{3 \right\}} | 0.29164 ^{\left\{6 \right\}} | 0.29447 ^{\left\{7 \right\}} | 0.00337 ^{\left\{1 \right\}} | 0.25582 ^{\left\{2 \right\}} | 0.28665 ^{\left\{4 \right\}} | 0.29820 ^{\left\{8 \right\}} | |
\hat{\varphi} | 0.18326 ^{\left\{2 \right\}} | 0.23736 ^{\left\{6 \right\}} | 0.19754 ^{\left\{4 \right\}} | 0.24885 ^{\left\{7 \right\}} | 0.13636 ^{\left\{1 \right\}} | 0.25856 ^{\left\{8 \right\}} | 0.19520 ^{\left\{3 \right\}} | 0.21153 ^{\left\{5 \right\}} | ||
\hat{\lambda} | 0.09940 ^{\left\{5 \right\}} | 0.10214 ^{\left\{6 \right\}} | 0.09825 ^{\left\{4 \right\}} | 0.10801 ^{\left\{8 \right\}} | 0.02528 ^{\left\{1 \right\}} | 0.07330 ^{\left\{2 \right\}} | 0.09651 ^{\left\{3 \right\}} | 0.10671 ^{\left\{7 \right\}} | ||
\hat{\theta} | 0.35281 ^{\left\{5 \right\}} | 0.35607 ^{\left\{6 \right\}} | 0.34440 ^{\left\{4 \right\}} | 0.38014 ^{\left\{8 \right\}} | 0.04770 ^{\left\{1 \right\}} | 0.25437 ^{\left\{2 \right\}} | 0.33728 ^{\left\{3 \right\}} | 0.37556 ^{\left\{7 \right\}} | ||
\sum{Ranks} | {\textbf{49}} ^{\left\{4 \right\}} | {\textbf{66}} ^{\left\{6 \right\}} | {\textbf{54}} ^{\left\{5 \right\}} | {\textbf{90}} ^{\left\{8 \right\}} | {\textbf{12}} ^{\left\{1 \right\}} | {\textbf{42}} ^{\left\{3 \right\}} | {\textbf{38}} ^{\left\{2 \right\}} | {\textbf{81}} ^{\left\{7 \right\}} |
All computations are obtained using R software (version 4.0.2) [22].
For each sample and each parametric combination, the EWFr parameters \vartheta , \varphi , \lambda and \theta are estimated using the eight estimators called MLEs, LSEs, WLSEs, MPSEs, CRVMEs, ADEs, PCEs, and RADEs. Simulated results are listed in Tables 2–6 which also indicate the ranks of each of the proposed estimators in each row, where the superscripts show the indicators, and the \sum Ranks illustrates the partial sum of ranks in each column for a particular sample size.
Table 7 lists the partial and overall ranks for all parametric combinations. From Tables 2–6, one can conclude that all methods of estimation illustrate the consistency property, that is, the MREs and MSEs decrease as the sample size increases, for all parametric combinations. Table 7 shows that the MPS approach has an overall score of 54, hence it outperforms other estimation methods. Therefore, we conclude and confirm the superiority of MPSEs for estimating the EWFr parameters. However, the ADEs and MLEs are approximately have a similar performance, where their overall scores are, respectively, 130.5 and 133.5.
\mathit{\boldsymbol{\eta}}^{\intercal} | n | MLEs | LSEs | WLSEs | CRVMEs | MPSEs | PCEs | ADEs | RADEs |
(\hat{\vartheta}=1.75, \hat{\varphi}=0.5, \hat{\lambda}=0.25, \hat{\theta}=1.25) | 20 | 2 | 7 | 1 | 5 | 4 | 8 | 3 | 6 |
80 | 3 | 7 | 2 | 6 | 1 | 5 | 4 | 8 | |
200 | 1.5 | 7 | 4 | 5 | 1.5 | 6 | 3 | 8 | |
500 | 2 | 5.5 | 4 | 5.5 | 1 | 7 | 3 | 8 | |
(\hat{\vartheta}=1.75, \hat{\varphi}=2, \hat{\lambda}=1.5, \hat{\theta}=2.5) | 20 | 1 | 3 | 2 | 7 | 4 | 8 | 5.5 | 5.5 |
80 | 1 | 3 | 4 | 6 | 2 | 8 | 5 | 7 | |
200 | 2 | 3 | 4.5 | 6.5 | 1 | 8 | 4.5 | 6.5 | |
500 | 3 | 4 | 5 | 6 | 1 | 8 | 2 | 7 | |
(\hat{\vartheta}=3.5, \hat{\varphi}=0.75, \hat{\lambda}=0.5, \hat{\theta}=1.25) | 20 | 7 | 4 | 3 | 6 | 1 | 8 | 2 | 5 |
80 | 8 | 3 | 4 | 7 | 1 | 5 | 2 | 6 | |
200 | 6 | 5 | 4 | 8 | 1 | 3 | 2 | 7 | |
500 | 2 | 6 | 5 | 7 | 1 | 4 | 3 | 8 | |
(\hat{\vartheta}=3.5, \hat{\varphi}=2, \hat{\lambda}=1.5, \hat{\theta}=2.5) | 20 | 8 | 2 | 6 | 3 | 1 | 7 | 5 | 4 |
80 | 8 | 2 | 7 | 3 | 1 | 6 | 4 | 5 | |
200 | 6.5 | 2 | 6.5 | 3 | 1 | 8 | 4 | 5 | |
500 | 5.5 | 3 | 7 | 5.5 | 1 | 8 | 2 | 4 | |
(\hat{\vartheta}=4.5, \hat{\varphi}=0.75, \hat{\lambda}=0.5, \hat{\theta}=1.25) | 20 | 8 | 2 | 7 | 3 | 1 | 6 | 5 | 4 |
80 | 8 | 2 | 7 | 6 | 1 | 3 | 5 | 4 | |
200 | 8 | 3 | 7 | 6 | 1 | 2 | 4 | 5 | |
500 | 4 | 6 | 5 | 8 | 1 | 3 | 2 | 7 | |
(\hat{\vartheta}=4.5, \hat{\varphi}=0.75, \hat{\lambda}=0.5, \hat{\theta}=1.25) | 20 | 8 | 2 | 5 | 3 | 1 | 7 | 6 | 4 |
80 | 6 | 2 | 4 | 3 | 1 | 8 | 7 | 5 | |
200 | 6 | 2 | 4 | 3 | 1 | 7 | 8 | 5 | |
500 | 6 | 2 | 5 | 3 | 1 | 8 | 7 | 4 | |
(\hat{\vartheta}=0.25, \hat{\varphi}=3.5, \hat{\lambda}=3, \hat{\theta}=0.25) | 20 | 1 | 6 | 8 | 2 | 7 | 5 | 4 | 3 |
80 | 1.5 | 5 | 8 | 1.5 | 6 | 4 | 3 | 7 | |
200 | 1 | 7 | 4 | 6 | 2 | 3 | 5 | 8 | |
500 | 2 | 8 | 6 | 7 | 1 | 3 | 4.5 | 4.5 | |
(\hat{\vartheta}=0.75, \hat{\varphi}=2.5, \hat{\lambda}=4.25, \hat{\theta}=0.25) | 20 | 2 | 8 | 6 | 5 | 3 | 1 | 4 | 7 |
80 | 1.5 | 7 | 5 | 6 | 1.5 | 3 | 4 | 8 | |
200 | 2 | 7 | 5 | 6 | 1 | 3 | 4 | 8 | |
500 | 2 | 8 | 5 | 6 | 1 | 3 | 4 | 7 | |
\sum Ranks | \textbf{133.5} | \textbf{143.5} | \textbf{160} | \textbf{164} | \textbf{54} | \textbf{176} | \textbf{130.5} | \textbf{190.5} | |
{\textbf{Overall Rank}} | \textbf{3} | \textbf{4} | \textbf{5} | \textbf{6} | \textbf{1} | \textbf{7} | \textbf{2} | \textbf{8} |
In this section, the flexibility and importance of the EWFr distribution in modeling real-life data are illustrated using two datasets from the medicine and engineering fields. The first dataset contains 128 observations and it refers to remission times (in months) for bladder cancer patients [23]. The second dataset contains 63 observations and it represents strengths for single carbon fibers of 10 mm of gauge lengths [24]. The fits of the EWFr distribution is checked and compared with some important extensions of the Fr distribution called the exponentiated-Fr (EFr) [6], beta-Fr (BFr) [7], odd Lomax-Fr (OLxFr) [25], Kumaraswamy Marshall–Olkin Fr (KMOFr) [26], gamma extended-Fr (GExFr) [27] and transmuted exponentiated-Fr (TEFr) [28] and Fr distributions.
We checked the competing distributions using some goodness-of-fit analytical measures such as AIC (Akaike information criterion), CAIC (consistent Akaike information criterion), HQIC (Hannan–Quinn information criterion), BIC (Bayesian information criterion), W^{\ast} (Cramér–Von Mises), A^{\ast} (Anderson–Darling), and KS (Kolmogorov–Smirnov) statistics with its PV (p-value).
The maximum likelihood (ML) estimates of the parameters of the fitted distributions, their standard errors (SEs), and the analytical measures are reported in Tables 8 and 9 for the cancer and gauge lengths data, respectively. The numbers in Tables 8 and 9 indicate that the EWFr distribution gives a superior fit over other competing models, since it has the lowest values for all measures and the largest PV.
Model | Par.. | Estimates | (SEs) | AIC | CAIC | BIC | HQIC | W^{*} | A^{*} | KS | (PV) |
EWFr | \hat{\vartheta} | 56.93662 | (38.42845) | 827.52150 | 827.84670 | 838.92960 | 832.15660 | 0.01991 | 0.13402 | 0.03757 | 0.99713 |
\hat{\varphi} | 0.46697 | (0.22382) | |||||||||
\hat{\lambda} | 0.71825 | (0.01713) | |||||||||
\hat{\theta} | 0.01794 | (0.01207) | |||||||||
OLxFr | \hat{\vartheta} | 2.18260 | (1.04763) | 827.70270 | 828.02790 | 839.11080 | 832.33780 | 0.02380 | 0.16520 | 0.04060 | 0.98433 |
\hat{\varphi} | 625.38000 | (622.71234) | |||||||||
\hat{a} | 0.12210 | (0.08023) | |||||||||
\hat{b} | 1.38560 | (0.17273) | |||||||||
KMOFr | \hat{\vartheta} | 43.87750 | (128.52731) | 831.05330 | 831.54510 | 845.31340 | 836.84730 | 0.26278 | 0.34950 | 0.04457 | 0.96120 |
\hat{\varphi} | 0.52028 | (0.41388) | |||||||||
\hat{\delta} | 0.01314 | (0.00808) | |||||||||
\hat{a} | 3.27359 | (2.90929) | |||||||||
\hat{b} | 19.93782 | (47.58906) | |||||||||
EFr | \hat{a} | 1426.40000 | (1440.19245) | 830.85880 | 831.05240 | 839.41490 | 834.33520 | 0.06720 | 0.46640 | 0.04910 | 0.91754 |
\hat{b} | 0.26240 | (0.02850) | |||||||||
\hat{\theta} | 46.24800 | (22.87321) | |||||||||
BFr | \hat{\vartheta} | 0.60970 | (0.32261) | 833.09830 | 833.42350 | 844.50640 | 837.73350 | 0.06900 | 0.47650 | 0.05540 | 0.82683 |
\hat{\varphi} | 36.60200 | (19.48341) | |||||||||
\hat{a} | 739.38700 | (629.24021) | |||||||||
\hat{b} | 0.32240 | (0.06044) | |||||||||
GExFr | \hat{\vartheta} | 226.67000 | (267.96324) | 840.21630 | 840.54150 | 851.62450 | 844.85150 | 0.14320 | 0.95970 | 0.06640 | 0.62593 |
\hat{\varphi} | 91.93900 | (133.94324) | |||||||||
\hat{a} | 22.27100 | (119.19139) | |||||||||
\hat{b} | 0.07090 | (0.04132) | |||||||||
TEFr | \hat{\vartheta} | 3.25820 | (0.40714) | 892.00150 | 892.09750 | 897.70560 | 894.31910 | 0.74430 | 4.54640 | 0.14080 | 0.01254 |
\hat{\varphi} | 0.75210 | (0.04224) | |||||||||
\hat{a} | 39.38721 | (40.24321) | |||||||||
\hat{b} | 0.25243 | (0.76041) | |||||||||
Fr | \hat{\theta} | 0.75208 | (0.04242) | 892.00150 | 892.09750 | 897.70560 | 894.31910 | 0.74432 | 4.54642 | 0.14079 | 0.01250 |
\hat{\lambda} | 2.43109 | (0.21928) |
Model | Par.. | Estimates | (SEs) | AIC | CAIC | BIC | HQIC | W^{*} | A^{*} | KS | (PV) |
EWFr | \hat{\vartheta} | 0.76563 | (0.3765631) | 119.68850 | 120.37820 | 128.26110 | 123.06020 | 0.04181 | 0.23988 | 0.06847 | 0.92920 |
\hat{\varphi} | 0.04349 | (0.3074545) | |||||||||
\hat{\lambda} | 145.67068 | (208.851634) | |||||||||
\hat{\theta} | 4.60846 | (1.2033681) | |||||||||
OLxFr | \hat{\vartheta} | 5.14301 | (9.9790719) | 119.94170 | 120.63140 | 128.51430 | 123.31340 | 0.04741 | 0.26530 | 0.07631 | 0.85664 |
\hat{\varphi} | 5.82568 | (7.6204045) | |||||||||
\hat{a} | 4.17374 | (2.671917) | |||||||||
\hat{b} | 2.82338 | (0.9308868) | |||||||||
KMOFr | \hat{\vartheta} | 31946.70000 | (837.7074) | 121.90480 | 122.95750 | 132.62050 | 126.11940 | 0.04616 | 0.26108 | 0.07450 | 0.87551 |
\hat{\varphi} | 3.40405 | (1.493079) | |||||||||
\hat{\delta} | 3.35291 | (0.9682264) | |||||||||
\hat{a} | 0.85381 | (0.1934628) | |||||||||
\hat{b} | 13724.50000 | (32837.29) | |||||||||
EFr | \hat{a} | 2.58672 | (2.2847576) | 122.19730 | 122.88700 | 130.76990 | 125.56900 | 0.07082 | 0.40707 | 0.08894 | 0.70136 |
\hat{b} | 0.50304 | (0.4780356) | |||||||||
\hat{\theta} | 6.25000 | (10.0300775) | |||||||||
BFr | \hat{\vartheta} | 2.38748 | (5.65075) | 120.58420 | 121.27380 | 129.15670 | 123.95580 | 0.06008 | 0.32203 | 0.07953 | 0.82045 |
\hat{\varphi} | 1.00168 | (5.322252) | |||||||||
\hat{a} | 22.38231 | (162.860747) | |||||||||
\hat{b} | 27.37096 | (295.790324) | |||||||||
GExFr | \hat{\vartheta} | 2.41716 | (8.509608) | 120.57980 | 121.26950 | 129.15240 | 123.95150 | 0.06064 | 0.32329 | 0.07962 | 0.81934 |
\hat{\varphi} | 1.20859 | (2.106142) | |||||||||
\hat{a} | 24.77784 | (82.649275) | |||||||||
\hat{b} | 15.60163 | (85.339807) | |||||||||
TEFr | \hat{\vartheta} | 4.04638 | (1.0119058) | 121.04420 | 121.73390 | 129.61670 | 124.41580 | 0.06547 | 0.34378 | 0.07500 | 0.87047 |
\hat{\varphi} | 2.50000 | (0.5973595) | |||||||||
\hat{a} | 5.25000 | (2.6300897) | |||||||||
\hat{b} | 0.09255 | (1.2750272) | |||||||||
Fr | \hat{\theta} | 5.43392 | (0.50788) | 121.80430 | 122.00430 | 126.09060 | 123.49010 | 0.11497 | 0.64202 | 0.10013 | 0.55274 |
\hat{\lambda} | 230.48644 | (110.91778) |
Some plots including the PDF, CDF, and SF along with the PP plots of the EWFr model are displayed in Figure 3 for both datasets. The PP plots of all studied distributions are displayed in Figure 4 for the two datasets, respectively.
The estimates of the EWFr parameters under several estimation approaches and the goodness-of-fit measures for both datasets are listed in Tables 10 and 11, respectively. Based on the values of PV in Tables 10 and 11, the Anderson-Darling approach is recommended to estimate the EWFr parameters for cancer data, while the least-squares approach is recommended for gauge lengths data.
Methods | \hat{\vartheta} | \hat{\varphi} | \hat{\lambda} | \hat{\theta} | W^{*} | A^{*} | KS | PV |
MLEs | 56.93661 | 0.46697 | 0.71825 | 0.01794 | 0.01991 | 0.13402 | 0.03757 | 0.99713 |
LSEs | 56.93660 | 0.55502 | 0.71932 | 0.01871 | 0.01850 | 0.12986 | 0.03334 | 0.99918 |
MPSEs | 56.93660 | 0.46698 | 0.71750 | 0.01717 | 0.01916 | 0.13069 | 0.03617 | 0.99708 |
WLSEs | 56.94615 | 0.51962 | 0.71884 | 0.01825 | 0.01815 | 0.12600 | 0.03251 | 0.99947 |
CRVMEs | 56.93659 | 0.55064 | 0.71962 | 0.01891 | 0.01795 | 0.12584 | 0.03352 | 0.99910 |
ADEs | 56.93668 | 0.47772 | 0.71887 | 0.01815 | 0.01763 | 0.12016 | 0.03167 | 0.99968 |
PCEs | 57.47389 | 0.81500 | 0.72219 | 0.02155 | 0.03320 | 0.23254 | 0.03830 | 0.99367 |
RADEs | 56.93805 | 0.56319 | 0.71967 | 0.01899 | 0.01830 | 0.12878 | 0.03385 | 0.99895 |
\textbf{Methods} | \hat{\vartheta} | \hat{\varphi} | \hat{\lambda} | \hat{\theta} | W^{*} | A^{*} | \textbf{KS} | \textbf{PV} |
\textbf{MLEs} | 0.76563 | 0.04349 | 145.67068 | 4.60846 | 0.04181 | 0.23988 | 0.06847 | 0.92920 |
\textbf{LSEs} | 0.69421 | 0.08110 | 145.67010 | 4.56661 | 0.04850 | 0.26973 | 0.06486 | 0.94154 |
\textbf{MPSEs} | 0.75534 | 0.13947 | 145.66920 | 4.65631 | 0.05531 | 0.29551 | 0.07780 | 0.81897 |
\textbf{WLSEs} | 0.71596 | 0.01530 | 145.66740 | 4.57637 | 0.04747 | 0.26675 | 0.06895 | 0.90999 |
\textbf{CRVMEs} | 0.71398 | 0.00100 | 145.67030 | 4.57259 | 0.04761 | 0.26713 | 0.06733 | 0.92336 |
\textbf{ADEs} | 0.72963 | 0.00010 | 145.67980 | 4.57988 | 0.04696 | 0.26562 | 0.07047 | 0.89642 |
\textbf{PCEs} | 0.75075 | 0.02596 | 145.67020 | 4.59649 | 0.04775 | 0.26737 | 0.07311 | 0.87060 |
\textbf{RADEs} | 0.74062 | 0.00010 | 145.66970 | 4.57722 | 0.04668 | 0.26516 | 0.07058 | 0.89538 |
In this paper, we introduced a more flexible four-parameter model called the extended Weibull–Fréchet (EWFr) distribution. Its basic mathematical properties are explored. The EWFr parameters are estimated using eight classical estimation methods. The simulation results showed that the maximum product of spacings approach outperforms other considered methods based on overall ranks. The importance and flexibility of the EWFr distribution over some competing extensions of the Fréchet distribution are addressed by analyzing two real-life datasets from the medicine and engineering fields. The analytical measures showed that our EWFr model returned an adequate fit in comparison with other distributions.
This study was funded by Taif University Researchers Supporting Project number (TURSP-2020/279), Taif University, Taif, Saudi Arabia. The authors would like to thank the editorial board and the three referees for their constructive comments that improved the final version of the paper.
The authors declare no conflict of interest.
[1] | R. B. Banks, Growth and diffusion phenomena: Mathematical frameworks and applications, Springer Science & Business Media, 14 (1993). |
[2] | M. Braun, M. Golubitsky, Differential equations and their applications, Springer, 1 (1983). https://doi.org/10.1007/978-1-4684-0164-6-1 |
[3] |
F. Courchamp, T. C. Brock, B. Grenfell, Inverse density dependence and the Allee effect, Trends Ecol. Evol., 14 (1999), 405–410. https://doi.org/10.1016/S0169-5347(99)01683-3 doi: 10.1016/S0169-5347(99)01683-3
![]() |
[4] |
P. A. Stephens, W. J. Sutherland, R. P. Freckleton, What is the Allee effect? Oikos, 1999,185–190. https://doi.org/10.2307/3547011 doi: 10.2307/3547011
![]() |
[5] | M. J. Panik, Stochastic differential equations: An introduction with applications in population dynamics modeling, John Wiley & Sons, 2017. https://doi.org/10.1002/9781119377399 |
[6] |
M. Krstić, M. Jovanović, On stochastic population model with the Allee effect, Math. Comput. Model., 52 (2010), 370–379. https://doi.org/10.1016/j.mcm.2010.02.051 doi: 10.1016/j.mcm.2010.02.051
![]() |
[7] |
Q. Yang, D. Jiang, A note on asymptotic behaviors of stochastic population model with Allee effect, Appl. Math. Model., 35 (2011), 4611–4619. https://doi.org/10.1016/j.apm.2011.03.034 doi: 10.1016/j.apm.2011.03.034
![]() |
[8] |
J. R. Graef, S. Padhi, S. Pati, Periodic solutions of some models with strong Allee effects, Nonlinear Anal.-Real, 13 (2012), 569–581. https://doi.org/10.1016/j.nonrwa.2011.07.044 doi: 10.1016/j.nonrwa.2011.07.044
![]() |
[9] |
B. F. Brockett, M. Hassall, The existence of an Allee effect in populations of porcellio scaber (isopoda: Oniscidea), Eur. J. Soil Biol., 41 (2005), 123–127. https://doi.org/10.1016/j.ejsobi.2005.09.004 doi: 10.1016/j.ejsobi.2005.09.004
![]() |
[10] |
F. Courchamp, T. C. Brock, B. Grenfell, Inverse density dependence and the Allee effect, Trends Ecol. Evol., 14 (1999), 405–410. https://doi.org/10.1016/S0169-5347(99)01683-3 doi: 10.1016/S0169-5347(99)01683-3
![]() |
[11] |
F. M. Hilker, M. Langlais, S. V. Petrovskii, H. Malchow, A diffusive si model with Allee effect and application to FIV, Math. Biol., 206 (2007), 61–80. https://doi.org/10.1016/j.mbs.2005.10.003 doi: 10.1016/j.mbs.2005.10.003
![]() |
[12] |
A. Hurford, M. Hebblewhite, M. A. Lewis, A spatially explicit model for an Allee effect: Why wolves recolonize so slowly in greater yellowstone, Theor. Popul. Biol., 70 (2006), 244–254. https://doi.org/10.1016/j.tpb.2006.06.009 doi: 10.1016/j.tpb.2006.06.009
![]() |
[13] |
P. Amarasekare, Allee effects in metapopulation dynamics, Am. Nat., 152 (1998), 298–302. https://doi.org/10.1086/286169 doi: 10.1086/286169
![]() |
[14] |
M. A. Idlango, J. J. Shepherd, J. A. Gear, Multiscaling analysis of a slowly varying single species population model displaying an Allee effect, Math. Method. Appl. Sci., 37 (2014), 1561–1569. https://doi.org/10.1002/mma.2911 doi: 10.1002/mma.2911
![]() |
[15] |
A. Tesfay, D. Tesfay, J. Brannan, J. Duan, A logistic-harvest model with Allee effect under multiplicative noise, Stoch. Dynam., 21 (2021), 2150044. https://doi.org/10.1142/S0219493721500441 doi: 10.1142/S0219493721500441
![]() |
[16] |
F. B. Rizaner, S. P. Rogovchenko, Dynamics of a single species under periodic habitat fluctuations and Allee effect, Nonlinear Anal.-Real, 13 (2012), 141–157. https://doi.org/10.1016/j.nonrwa.2011.07.021 doi: 10.1016/j.nonrwa.2011.07.021
![]() |
[17] |
S. Rosenblat, Population models in a periodically fluctuating environment, J. Math. Biol., 9 (1980) 23–36. https://doi.org/10.1007/BF00276033 doi: 10.1007/BF00276033
![]() |
[18] |
T. Legović, G. Perić, Harvesting population in a periodic environment, Ecol. Model., 24 (1984), 221–229. https://doi.org/10.1016/0304-3800(84)90042-5 doi: 10.1016/0304-3800(84)90042-5
![]() |
[19] |
F. M. Alharbi, A slow single-species model with non-symmetric variation of the coefficients, Fractal Fract., 6 (2022), 72. https://doi.org/10.3390/fractalfract6020072 doi: 10.3390/fractalfract6020072
![]() |
[20] |
F. M. Alharbi, The general analytic expression of a harvested logistic model with slowly varying coefficients, Axioms, 11 (2022), 585. https://doi.org/10.3390/axioms11110585 doi: 10.3390/axioms11110585
![]() |
[21] |
A. K. Alsharidi, A. A. Khan, J. J. Shepherd, A. J. Stacey, Multiscaling analysis of a slowly varying anaerobic digestion model, Math. Method. Appl. Sci., 43 (2020), 5729–5743. https://doi.org/10.1002/mma.6315 doi: 10.1002/mma.6315
![]() |
[22] |
M. A. Idlango, J. J. Shepherd, J. A. Gear, Multiscaling analysis of a slowly varying single species population model displaying an Allee effect, Math. Method. Appl. Sci., 37 (2014), 1561–1569. https://doi.org/10.1002/mma.2911 doi: 10.1002/mma.2911
![]() |
[23] |
M. A. Idlango, J. J. Shepherd, J. A. Gear, Logistic growth with a slowly varying holling type Ⅱ harvesting term, Commun. Nonlinear Sci., 49 (2017), 81–92. https://doi.org/10.1016/j.cnsns.2017.02.005 doi: 10.1016/j.cnsns.2017.02.005
![]() |
[24] |
T. Cromer, Harvesting in a seasonal environment, Math. Comput. Model., 10 (1988), 445–450. https://doi.org/10.1016/0895-7177(88)90034-9 doi: 10.1016/0895-7177(88)90034-9
![]() |
[25] |
P. S. Meyer, J. H. Ausubel, Carrying capacity: A model with logistically varying limits, Technol. Forecast. Soc., 61 (1999), 209–214. https://doi.org/10.1016/S0040-1625(99)00022-0 doi: 10.1016/S0040-1625(99)00022-0
![]() |
[26] |
D. Ludwig, D. D. Jones, C. S. Holling, Qualitative analysis of insect outbreak systems: The spruce budworm and forest, J. Anim. Ecol., 47 (1978), 315–332. https://doi.org/10.2307/3939 doi: 10.2307/3939
![]() |
[27] |
J. J. Shepherd, L. Stojkov, The logistic population model with slowly varying carrying capacity, Anziam J., 47 (2005), C492–C506. https://doi.org/10.21914/anziamj.v47i0.1058 doi: 10.21914/anziamj.v47i0.1058
![]() |
[28] |
T. Grozdanovski, J. J. Shepherd, A. Stacey, Multi-scaling analysis of a logistic model with slowly varying coefficients, Appl. Math. Lett., 22 (2009), 1091–1095. https://doi.org/10.1016/j.aml.2008.10.002 doi: 10.1016/j.aml.2008.10.002
![]() |
[29] |
R. M. May, Thresholds and breakpoints in ecosystems with a multiplicity of stable states, Nature, 269 (1977), 471–477. https://doi.org/10.1038/269471a0 doi: 10.1038/269471a0
![]() |
1. | Selasi Kwaku Ocloo, Lewis Brew, Suleman Nasiru, Benjamin Odoi, Jewgeni Dshalalow, Harmonic Mixture Fréchet Distribution: Properties and Applications to Lifetime Data, 2022, 2022, 1687-0425, 1, 10.1155/2022/6460362 | |
2. | Xueyu Wu, Zubair Ahmad, Eslam Hussam, Marwan H. Alhelali, Ramy Aldallal, Muqrin A. Almuqrin, Fathy H. Riad, A new Cosine-Weibull model: Distributional properties with applications to basketball and medical sectors, 2023, 66, 11100168, 751, 10.1016/j.aej.2022.10.068 | |
3. | Abdul Ghaniyyu Abubakari, Chin-Chia Wu, Actuarial Measures, Regression, and Applications of Exponentiated Fréchet Loss Distribution, 2022, 2022, 1687-0425, 1, 10.1155/2022/3155188 | |
4. | Adam Braima S. Mastor, Oscar Ngesa, Joseph Mung’atu, Nada M. Alfaer, Ahmed Z. Afify, M. De Aguiar, The Extended Exponential Weibull Distribution: Properties, Inference, and Applications to Real-Life Data, 2022, 2022, 1099-0526, 1, 10.1155/2022/4068842 | |
5. | Otieno Kevin Okumu, Omondi Joseph Ouno, Anthony Nyutu Karanjah, Samuel Nganga Muthiga, Three-parameters Gumbel Distribution: Formulation and Parameter Estimation, 2024, 10, 2472-3509, 25, 10.11648/j.ijsd.20241002.12 | |
6. | Fatimah E. Almuhayfith, Talha Arslan, Hassan S. Bakouch, Aminh M. Alnaghmosh, A versatile model for lifetime of a component under stress, 2023, 13, 2045-2322, 10.1038/s41598-023-47313-3 | |
7. | Hassan M. Aljohani, The single and double inverse Pareto–Burr XII distribution: Properties, estimation methods, and real-life data applications, 2024, 14, 2158-3226, 10.1063/5.0203919 | |
8. | M. G. M. Ghazal, Yusra A. Tashkandy, Oluwafemi Samson Balogun, M. E. Bakr, Exponentiated extended extreme value distribution: Properties, estimation, and applications in applied fields, 2024, 9, 2473-6988, 17634, 10.3934/math.2024857 | |
9. | Ahmed Z. Afify, Ekramy A. Hussein, Hazem Al-Mofleh, Rehab Alsultan, Hassan M. Aljohani, Nian-Sheng Tang, The Flexible Fréchet Distribution: Properties, Inference, and Medical Applications, 2024, 2024, 2314-4629, 10.1155/jom/2600560 | |
10. | Hatem Semary, Ahmad Abubakar Suleiman, Aliyu Ismail Ishaq, Jamilu Yunusa Falgore, Umar Kabir Abdullahi, Hanita Daud, Mohamed A. Abd Elgawad, Mohammad Elgarhy, A new modified Sine-Weibull distribution for modeling medical data with dynamic structures, 2025, 18, 16878507, 101427, 10.1016/j.jrras.2025.101427 | |
11. | Dawlah Alsulami, A new extension of the Rayleigh distribution: Properties, different methods of estimation, and an application to medical data, 2025, 10, 2473-6988, 7636, 10.3934/math.2025350 |
\mathit{\boldsymbol{\eta}}^{\intercal} | \mu | \sigma^{2} | \xi_{1}(X) | \xi_{2}(X) |
(\vartheta=1.50, \; \varphi=0.50, \; \theta=1.50) | 1.3511 | 0.4752 | 3.1421 | 56.2021 |
(\vartheta=2.50, \; \varphi=0.75, \; \theta=1.75) | 1.2701 | 0.1329 | 1.9395 | 16.1410 |
(\vartheta=2.25, \; \varphi=1.00, \; \theta=2.75) | 1.1904 | 0.0780 | 2.2648 | 19.2907 |
(\vartheta=2.25, \; \varphi=0.25, \; \theta=0.75) | 1.6154 | 0.7600 | 1.6132 | 9.0569 |
(\vartheta=2.25, \; \varphi=1.25, \; \theta=3.25) | 1.1831 | 0.0747 | 2.8068 | 27.8064 |
(\vartheta=5.50, \; \varphi=1.50, \; \theta=2.50) | 1.1927 | 0.0223 | 1.7507 | 11.7806 |
n | Est. | Est. Par. | MLEs | LSEs | WLSEs | CRVMEs | MPSEs | PCEs | ADEs | RADEs |
20 | |BIAS| | \hat{\vartheta} | 0.58150 ^{\{3\}} | 0.59477 ^{\{4\}} | 0.53422 ^{\{1\}} | 0.54541 ^{\{2\}} | 0.60555 ^{\{5\}} | 0.71685 ^{\{8\}} | 0.67445 ^{\{7\}} | 0.61466 ^{\{6\}} |
\hat{\varphi} | 0.47550 ^{\{4\}} | 0.54812 ^{\{7\}} | 0.46820 ^{\{2\}} | 0.53093 ^{\{6\}} | 0.19120 ^{\{1\}} | 0.55104 ^{\{8\}} | 0.46906 ^{\{3\}} | 0.51894 ^{\{5\}} | ||
\hat{\lambda} | 0.08930 ^{\{2\}} | 0.10351 ^{\{7\}} | 0.08402 ^{\{1\}} | 0.10057 ^{\{5\}} | 0.10077 ^{\{6\}} | 0.11204 ^{\{8\}} | 0.09758 ^{\{3\}} | 0.09866 ^{\{4\}} | ||
\hat{\theta} | 0.49749 ^{\{7\}} | 0.47497 ^{\{4\}} | 0.46884 ^{\{3\}} | 0.48364 ^{\{5\}} | 0.45238 ^{\{1\}} | 0.53285 ^{\{8\}} | 0.46505 ^{\{2\}} | 0.48608 ^{\{6\}} | ||
MSEs | \hat{\vartheta} | 0.47425 ^{\{2\}} | 0.52401 ^{\{4\}} | 0.52337 ^{\{3\}} | 0.46463 ^{\{1\}} | 0.54588 ^{\{6\}} | 0.64689 ^{\{8\}} | 0.59726 ^{\{7\}} | 0.53428 ^{\{5\}} | |
\hat{\varphi} | 0.27624 ^{\{1\}} | 0.38734 ^{\{7\}} | 0.29294 ^{\{2\}} | 0.36212 ^{\{6\}} | 0.31849 ^{\{4\}} | 0.39577 ^{\{8\}} | 0.30160 ^{\{3\}} | 0.35513 ^{\{5\}} | ||
\hat{\lambda} | 0.01107 ^{\{2\}} | 0.01423 ^{\{7\}} | 0.00983 ^{\{1\}} | 0.01356 ^{\{5\}} | 0.01357 ^{\{6\}} | 0.01651 ^{\{8\}} | 0.01289 ^{\{3\}} | 0.01296 ^{\{4\}} | ||
\hat{\theta} | 0.37764 ^{\{7\}} | 0.33320 ^{\{3\}} | 0.35778 ^{\{4\}} | 0.36101 ^{\{5\}} | 0.29381 ^{\{1\}} | 0.38887 ^{\{8\}} | 0.32193 ^{\{2\}} | 0.36311 ^{\{6\}} | ||
MREs | \hat{\vartheta} | 0.33229 ^{\{3\}} | 0.33987 ^{\{4\}} | 0.17807 ^{\{1\}} | 0.31166 ^{\{2\}} | 0.34603 ^{\{5\}} | 0.40963 ^{\{8\}} | 0.38540 ^{\{7\}} | 0.35123 ^{\{6\}} | |
\hat{\varphi} | 0.95101 ^{\{3\}} | 1.09624 ^{\{7\}} | 0.93641 ^{\{1\}} | 1.06186 ^{\{6\}} | 0.97231 ^{\{4\}} | 1.10208 ^{\{8\}} | 0.93811 ^{\{2\}} | 1.03788 ^{\{5\}} | ||
\hat{\lambda} | 0.35719 ^{\{2\}} | 0.41405 ^{\{7\}} | 0.33607 ^{\{1\}} | 0.40230 ^{\{5\}} | 0.40307 ^{\{6\}} | 0.44817 ^{\{8\}} | 0.39034 ^{\{3\}} | 0.39464 ^{\{4\}} | ||
\hat{\theta} | 0.39799 ^{\{7\}} | 0.37998 ^{\{4\}} | 0.37507 ^{\{3\}} | 0.38691 ^{\{5\}} | 0.36190 ^{\{1\}} | 0.42628 ^{\{8\}} | 0.37204 ^{\{2\}} | 0.38886 ^{\{6\}} | ||
\sum{Ranks} | 43 ^{\{2\}} | 65 ^{\{7\}} | 23 ^{\{1\}} | 53 ^{\{5\}} | 46 ^{\{4\}} | 96 ^{\{8\}} | 44 ^{\{3\}} | 62 ^{\{6\}} | ||
80 | |BIAS| | \hat{\vartheta} | 0.65720 ^{\{2\}} | 0.73260 ^{\{6\}} | 0.64523 ^{\{1\}} | 0.71780 ^{\{4\}} | 0.67014 ^{\{3\}} | 0.78730 ^{\{8\}} | 0.72979 ^{\{5\}} | 0.78285 ^{\{7\}} |
\hat{\varphi} | 0.29918 ^{\{4\}} | 0.36951 ^{\{7\}} | 0.27270 ^{\{1\}} | 0.36572 ^{\{6\}} | 0.28461 ^{\{2\}} | 0.43485 ^{\{8\}} | 0.29440 ^{\{3\}} | 0.31911 ^{\{5\}} | ||
\hat{\lambda} | 0.06946 ^{\{2\}} | 0.08089 ^{\{7\}} | 0.06755 ^{\{1\}} | 0.07955 ^{\{6\}} | 0.07162 ^{\{4\}} | 0.07099 ^{\{3\}} | 0.07301 ^{\{5\}} | 0.08347 ^{\{8\}} | ||
\hat{\theta} | 0.41705 ^{\{6\}} | 0.41605 ^{\{5\}} | 0.41277 ^{\{4\}} | 0.43216 ^{\{7\}} | 0.34179 ^{\{1\}} | 0.38656 ^{\{3\}} | 0.37952 ^{\{2\}} | 0.45183 ^{\{8\}} | ||
MSEs | \hat{\vartheta} | 0.53699 ^{\{1\}} | 0.65792 ^{\{5\}} | 0.71214 ^{\{7\}} | 0.63722 ^{\{3\}} | 0.62257 ^{\{2\}} | 0.71659 ^{\{8\}} | 0.64185 ^{\{4\}} | 0.70948 ^{\{6\}} | |
\hat{\varphi} | 0.12478 ^{\{3\}} | 0.19502 ^{\{7\}} | 0.11097 ^{\{1\}} | 0.18881 ^{\{6\}} | 0.12320 ^{\{2\}} | 0.28084 ^{\{8\}} | 0.13150 ^{\{4\}} | 0.15268 ^{\{5\}} | ||
\hat{\lambda} | 0.00672 ^{\{1\}} | 0.00884 ^{\{7\}} | 0.00693 ^{\{2\}} | 0.00853 ^{\{6\}} | 0.00712 ^{\{4\}} | 0.00711 ^{\{3\}} | 0.00740 ^{\{5\}} | 0.00932 ^{\{8\}} | ||
\hat{\theta} | 0.26049 ^{\{5\}} | 0.25434 ^{\{4\}} | 0.30456 ^{\{8\}} | 0.27845 ^{\{6\}} | 0.16695 ^{\{1\}} | 0.24032 ^{\{3\}} | 0.20942 ^{\{2\}} | 0.29980 ^{\{7\}} | ||
MREs | \hat{\vartheta} | 0.37554 ^{\{2\}} | 0.41863 ^{\{6\}} | 0.21508 ^{\{1\}} | 0.41017 ^{\{4\}} | 0.38294 ^{\{3\}} | 0.44989 ^{\{8\}} | 0.41702 ^{\{5\}} | 0.44734 ^{\{7\}} | |
\hat{\varphi} | 0.59835 ^{\{4\}} | 0.73902 ^{\{7\}} | 0.54540 ^{\{1\}} | 0.73144 ^{\{6\}} | 0.56922 ^{\{2\}} | 0.86970 ^{\{8\}} | 0.58881 ^{\{3\}} | 0.63822 ^{\{5\}} | ||
\hat{\lambda} | 0.27786 ^{\{2\}} | 0.32356 ^{\{7\}} | 0.27019 ^{\{1\}} | 0.31820 ^{\{6\}} | 0.28647 ^{\{4\}} | 0.28396 ^{\{3\}} | 0.29205 ^{\{5\}} | 0.33388 ^{\{8\}} | ||
\hat{\theta} | 0.33364 ^{\{6\}} | 0.33284 ^{\{5\}} | 0.33022 ^{\{4\}} | 0.34573 ^{\{7\}} | 0.27343 ^{\{1\}} | 0.30924 ^{\{3\}} | 0.30361 ^{\{2\}} | 0.36147 ^{\{8\}} | ||
\sum{Ranks} | 38 ^{\{3\}} | 73 ^{\{7\}} | 32 ^{\{2\}} | 67 ^{\{6\}} | 29 ^{\{1\}} | 66 ^{\{5\}} | 45 ^{\{4\}} | 82 ^{\{8\}} | ||
200 | |BIAS| | \hat{\vartheta} | 0.54244 ^{\{1\}} | 0.74060 ^{\{6\}} | 0.66029 ^{\{4\}} | 0.72065 ^{\{5\}} | 0.56860 ^{\{2\}} | 0.78528 ^{\{8\}} | 0.64417 ^{\{3\}} | 0.74318 ^{\{7\}} |
\hat{\varphi} | 0.20343 ^{\{3\}} | 0.27714 ^{\{7\}} | 0.18758 ^{\{1\}} | 0.27628 ^{\{6\}} | 0.19120 ^{\{2\}} | 0.33648 ^{\{8\}} | 0.21264 ^{\{4\}} | 0.21990 ^{\{5\}} | ||
\hat{\lambda} | 0.05329 ^{\{1\}} | 0.07033 ^{\{7\}} | 0.05985 ^{\{3\}} | 0.06898 ^{\{6\}} | 0.05669 ^{\{2\}} | 0.05992 ^{\{4\}} | 0.06059 ^{\{5\}} | 0.07617 ^{\{8\}} | ||
\hat{\theta} | 0.30910 ^{\{2\}} | 0.38666 ^{\{6\}} | 0.37834 ^{\{5\}} | 0.39240 ^{\{7\}} | 0.26754 ^{\{1\}} | 0.34697 ^{\{4\}} | 0.32167 ^{\{3\}} | 0.42492 ^{\{8\}} | ||
MSEs | \hat{\vartheta} | 0.39682 ^{\{1\}} | 0.65105 ^{\{6\}} | 0.72704 ^{\{8\}} | 0.62292 ^{\{4\}} | 0.51529 ^{\{2\}} | 0.70871 ^{\{7\}} | 0.53035 ^{\{3\}} | 0.65051 ^{\{5\}} | |
\hat{\varphi} | 0.06226 ^{\{3\}} | 0.11401 ^{\{7\}} | 0.05424 ^{\{1\}} | 0.11214 ^{\{6\}} | 0.05643 ^{\{2\}} | 0.18593 ^{\{8\}} | 0.06927 ^{\{4\}} | 0.07447 ^{\{5\}} | ||
\hat{\lambda} | 0.00405 ^{\{1\}} | 0.00665 ^{\{7\}} | 0.00571 ^{\{5\}} | 0.00644 ^{\{6\}} | 0.00452 ^{\{2\}} | 0.00513 ^{\{4\}} | 0.00507 ^{\{3\}} | 0.00753 ^{\{8\}} | ||
\hat{\theta} | 0.14395 ^{\{2\}} | 0.20851 ^{\{5\}} | 0.26568 ^{\{8\}} | 0.22075 ^{\{6\}} | 0.10322 ^{\{1\}} | 0.19203 ^{\{4\}} | 0.14560 ^{\{3\}} | 0.25309 ^{\{7\}} | ||
MREs | \hat{\vartheta} | 0.30997 ^{\{2\}} | 0.42320 ^{\{6\}} | 0.22010 ^{\{1\}} | 0.41180 ^{\{5\}} | 0.32491 ^{\{3\}} | 0.44873 ^{\{8\}} | 0.36810 ^{\{4\}} | 0.42468 ^{\{7\}} | |
\hat{\varphi} | 0.40685 ^{\{3\}} | 0.55429 ^{\{7\}} | 0.37516 ^{\{1\}} | 0.55256 ^{\{6\}} | 0.38240 ^{\{2\}} | 0.67295 ^{\{8\}} | 0.42528 ^{\{4\}} | 0.43980 ^{\{5\}} | ||
\hat{\lambda} | 0.21316 ^{\{1\}} | 0.28134 ^{\{7\}} | 0.23940 ^{\{3\}} | 0.27593 ^{\{6\}} | 0.22675 ^{\{2\}} | 0.23969 ^{\{4\}} | 0.24238 ^{\{5\}} | 0.30470 ^{\{8\}} | ||
\hat{\theta} | 0.24728 ^{\{2\}} | 0.30933 ^{\{6\}} | 0.30267 ^{\{5\}} | 0.31392 ^{\{7\}} | 0.21403 ^{\{1\}} | 0.27758 ^{\{4\}} | 0.25734 ^{\{3\}} | 0.33994 ^{\{8\}} | ||
\sum{Ranks} | 22 ^{\{1.5\}} | 77 ^{\{7\}} | 45 ^{\{4\}} | 70 ^{\{5\}} | 22 ^{\{1.5\}} | 71 ^{\{6\}} | 44 ^{\{3\}} | 81 ^{\{8\}} | ||
500 | |BIAS| | \hat{\vartheta} | 0.40816 ^{\{2\}} | 0.63822 ^{\{6\}} | 0.59712 ^{\{4\}} | 0.62303 ^{\{5\}} | 0.38723 ^{\{1\}} | 0.72351 ^{\{8\}} | 0.50869 ^{\{3\}} | 0.64537 ^{\{7\}} |
\hat{\varphi} | 0.13392 ^{\{3\}} | 0.19775 ^{\{6\}} | 0.12778 ^{\{1\}} | 0.19966 ^{\{7\}} | 0.13208 ^{\{2\}} | 0.26446 ^{\{8\}} | 0.14927 ^{\{4\}} | 0.15660 ^{\{5\}} | ||
\hat{\lambda} | 0.03902 ^{\{1\}} | 0.05985 ^{\{7\}} | 0.04987 ^{\{4\}} | 0.05913 ^{\{6\}} | 0.04014 ^{\{2\}} | 0.05408 ^{\{5\}} | 0.04806 ^{\{3\}} | 0.06618 ^{\{8\}} | ||
\hat{\theta} | 0.21255 ^{\{2\}} | 0.32416 ^{\{6\}} | 0.31285 ^{\{5\}} | 0.33146 ^{\{7\}} | 0.18308 ^{\{1\}} | 0.30762 ^{\{4\}} | 0.24975 ^{\{3\}} | 0.35803 ^{\{8\}} | ||
MSEs | \hat{\vartheta} | 0.24842 ^{\{1\}} | 0.52025 ^{\{5\}} | 0.58302 ^{\{7\}} | 0.50041 ^{\{4\}} | 0.33081 ^{\{2\}} | 0.63280 ^{\{8\}} | 0.37071 ^{\{3\}} | 0.52827 ^{\{6\}} | |
\hat{\varphi} | 0.02762 ^{\{3\}} | 0.05937 ^{\{6\}} | 0.02573 ^{\{1\}} | 0.06052 ^{\{7\}} | 0.02727 ^{\{2\}} | 0.12045 ^{\{8\}} | 0.03399 ^{\{4\}} | 0.03724 ^{\{5\}} | ||
\hat{\lambda} | 0.00224 ^{\{1\}} | 0.00478 ^{\{7\}} | 0.00422 ^{\{5\}} | 0.00470 ^{\{6\}} | 0.00257 ^{\{2\}} | 0.00402 ^{\{4\}} | 0.00325 ^{\{3\}} | 0.00569 ^{\{8\}} | ||
\hat{\theta} | 0.06777 ^{\{2\}} | 0.14391 ^{\{5\}} | 0.19157 ^{\{8\}} | 0.15413 ^{\{6\}} | 0.05743 ^{\{1\}} | 0.14128 ^{\{4\}} | 0.08776 ^{\{3\}} | 0.17743 ^{\{7\}} | ||
MREs | \hat{\vartheta} | 0.23323 ^{\{3\}} | 0.36470 ^{\{6\}} | 0.19904 ^{\{1\}} | 0.35602 ^{\{5\}} | 0.22127 ^{\{2\}} | 0.41343 ^{\{8\}} | 0.29068 ^{\{4\}} | 0.36879 ^{\{7\}} | |
\hat{\varphi} | 0.26784 ^{\{3\}} | 0.39550 ^{\{6\}} | 0.25556 ^{\{1\}} | 0.39932 ^{\{7\}} | 0.26417 ^{\{2\}} | 0.52891 ^{\{8\}} | 0.29853 ^{\{4\}} | 0.31320 ^{\{5\}} | ||
\hat{\lambda} | 0.15609 ^{\{1\}} | 0.23939 ^{\{7\}} | 0.19949 ^{\{4\}} | 0.23651 ^{\{6\}} | 0.16056 ^{\{2\}} | 0.21632 ^{\{5\}} | 0.19222 ^{\{3\}} | 0.26474 ^{\{8\}} | ||
\hat{\theta} | 0.17004 ^{\{2\}} | 0.25933 ^{\{6\}} | 0.25028 ^{\{5\}} | 0.26517 ^{\{7\}} | 0.14646 ^{\{1\}} | 0.24610 ^{\{4\}} | 0.19980 ^{\{3\}} | 0.28642 ^{\{8\}} | ||
\sum{Ranks} | 24 ^{\{2\}} | 73 ^{\{5.5\}} | 46 ^{\{4\}} | 73 ^{\{5.5\}} | 20 ^{\{1\}} | 74 ^{\{7\}} | 40 ^{\{3\}} | 82 ^{\{8\}} |
n | Est. | Est. Par. | MLEs | LSEs | WLSEs | CRVMEs | MPSEs | PCEs | ADEs | RADEs |
20 | |BIAS| | \hat{\vartheta} | 0.58249 ^{\left\{3 \right\}} | 0.53932 ^{\left\{1 \right\}} | 0.56241 ^{\left\{2 \right\}} | 0.61397 ^{\left\{5 \right\}} | 0.60739 ^{\left\{4 \right\}} | 0.82341 ^{\left\{8 \right\}} | 0.68123 ^{\left\{7 \right\}} | 0.66592 ^{\left\{6 \right\}} |
\hat{\varphi} | 0.70201 ^{\left\{2 \right\}} | 0.74992 ^{\left\{4 \right\}} | 0.71005 ^{\left\{3 \right\}} | 0.99334 ^{\left\{7 \right\}} | 0.41044 ^{\left\{1 \right\}} | 1.11769 ^{\left\{8 \right\}} | 0.88784 ^{\left\{5 \right\}} | 0.97457 ^{\left\{6 \right\}} | ||
\hat{\lambda} | 0.26246 ^{\left\{1 \right\}} | 0.28717 ^{\left\{3 \right\}} | 0.28400 ^{\left\{2 \right\}} | 0.42881 ^{\left\{5 \right\}} | 0.40138 ^{\left\{4 \right\}} | 0.64545 ^{\left\{8 \right\}} | 0.43589 ^{\left\{7 \right\}} | 0.43050 ^{\left\{6 \right\}} | ||
\hat{\theta} | 0.83485 ^{\left\{6 \right\}} | 0.91523 ^{\left\{7 \right\}} | 0.92408 ^{\left\{8 \right\}} | 0.76222 ^{\left\{3 \right\}} | 0.77841 ^{\left\{4 \right\}} | 0.78092 ^{\left\{5 \right\}} | 0.74535 ^{\left\{1 \right\}} | 0.74828 ^{\left\{2 \right\}} | ||
MSEs | \hat{\vartheta} | 0.45340 ^{\left\{3 \right\}} | 0.42087 ^{\left\{1 \right\}} | 0.44427 ^{\left\{2 \right\}} | 0.51220 ^{\left\{4 \right\}} | 0.51720 ^{\left\{5 \right\}} | 0.77654 ^{\left\{8 \right\}} | 0.58720 ^{\left\{7 \right\}} | 0.56968 ^{\left\{6 \right\}} | |
\hat{\varphi} | 0.65182 ^{\left\{1 \right\}} | 0.75540 ^{\left\{3 \right\}} | 0.66110 ^{\left\{2 \right\}} | 1.25282 ^{\left\{7 \right\}} | 0.95641 ^{\left\{4 \right\}} | 1.49737 ^{\left\{8 \right\}} | 1.01553 ^{\left\{5 \right\}} | 1.19476 ^{\left\{6 \right\}} | ||
\hat{\lambda} | 0.07059 ^{\left\{1 \right\}} | 0.08751 ^{\left\{3 \right\}} | 0.08543 ^{\left\{2 \right\}} | 0.29909 ^{\left\{7 \right\}} | 0.24742 ^{\left\{4 \right\}} | 0.52940 ^{\left\{8 \right\}} | 0.29252 ^{\left\{6 \right\}} | 0.29154 ^{\left\{5 \right\}} | ||
\hat{\theta} | 0.84612 ^{\left\{6 \right\}} | 1.00860 ^{\left\{8 \right\}} | 1.00532 ^{\left\{7 \right\}} | 0.71906 ^{\left\{3 \right\}} | 0.75325 ^{\left\{4 \right\}} | 0.82231 ^{\left\{5 \right\}} | 0.69565 ^{\left\{1 \right\}} | 0.70768 ^{\left\{2 \right\}} | ||
MREs | \hat{\vartheta} | 0.33285 ^{\left\{3 \right\}} | 0.30818 ^{\left\{1 \right\}} | 0.32138 ^{\left\{2 \right\}} | 0.35084 ^{\left\{5 \right\}} | 0.34708 ^{\left\{4 \right\}} | 0.47052 ^{\left\{8 \right\}} | 0.38927 ^{\left\{7 \right\}} | 0.38052 ^{\left\{6 \right\}} | |
\hat{\varphi} | 0.35100 ^{\left\{1 \right\}} | 0.37496 ^{\left\{3 \right\}} | 0.35503 ^{\left\{2 \right\}} | 0.49667 ^{\left\{7 \right\}} | 0.42863 ^{\left\{4 \right\}} | 0.55884 ^{\left\{8 \right\}} | 0.44392 ^{\left\{5 \right\}} | 0.48728 ^{\left\{6 \right\}} | ||
\hat{\lambda} | 0.17497 ^{\left\{1 \right\}} | 0.19145 ^{\left\{3 \right\}} | 0.18933 ^{\left\{2 \right\}} | 0.28587 ^{\left\{5 \right\}} | 0.26758 ^{\left\{4 \right\}} | 0.43030 ^{\left\{8 \right\}} | 0.29059 ^{\left\{7 \right\}} | 0.28700 ^{\left\{6 \right\}} | ||
\hat{\theta} | 0.33394 ^{\left\{6 \right\}} | 0.36609 ^{\left\{7 \right\}} | 0.36963 ^{\left\{8 \right\}} | 0.30489 ^{\left\{3 \right\}} | 0.31136 ^{\left\{4 \right\}} | 0.31237 ^{\left\{5 \right\}} | 0.29814 ^{\left\{1 \right\}} | 0.29931 ^{\left\{2 \right\}} | ||
\sum{Ranks} | {\textbf{34}} ^{\left\{1 \right\}} | {\textbf{44}} ^{\left\{3 \right\}} | {\textbf{42}} ^{\left\{2 \right\}} | {\textbf{61}} ^{\left\{7 \right\}} | {\textbf{46}} ^{\left\{4 \right\}} | {\textbf{87}} ^{\left\{8 \right\}} | {\textbf{59}} ^{\left\{5.5 \right\}} | {\textbf{59}} ^{\left\{5.5 \right\}} | ||
80 | |BIAS| | \hat{\vartheta} | 0.49925 ^{\left\{2 \right\}} | 0.47135 ^{\left\{1 \right\}} | 0.52766 ^{\left\{3 \right\}} | 0.66329 ^{\left\{5 \right\}} | 0.55966 ^{\left\{4 \right\}} | 0.89224 ^{\left\{8 \right\}} | 0.67228 ^{\left\{6 \right\}} | 0.69447 ^{\left\{7 \right\}} |
\hat{\varphi} | 0.52063 ^{\left\{1 \right\}} | 0.55025 ^{\left\{3 \right\}} | 0.52909 ^{\left\{2 \right\}} | 0.70683 ^{\left\{7 \right\}} | 0.56354 ^{\left\{4 \right\}} | 0.93582 ^{\left\{8 \right\}} | 0.63998 ^{\left\{5 \right\}} | 0.69954 ^{\left\{6 \right\}} | ||
\hat{\lambda} | 0.25198 ^{\left\{1 \right\}} | 0.25883 ^{\left\{3 \right\}} | 0.25641 ^{\left\{2 \right\}} | 0.43311 ^{\left\{7 \right\}} | 0.30034 ^{\left\{4 \right\}} | 0.66563 ^{\left\{8 \right\}} | 0.40607 ^{\left\{5 \right\}} | 0.43206 ^{\left\{6 \right\}} | ||
\hat{\theta} | 0.78480 ^{\left\{6 \right\}} | 0.82532 ^{\left\{7 \right\}} | 0.83697 ^{\left\{8 \right\}} | 0.69063 ^{\left\{3 \right\}} | 0.58559 ^{\left\{1 \right\}} | 0.72122 ^{\left\{5 \right\}} | 0.63842 ^{\left\{2 \right\}} | 0.69164 ^{\left\{4 \right\}} | ||
MSEs | \hat{\vartheta} | 0.31719 ^{\left\{2 \right\}} | 0.30026 ^{\left\{1 \right\}} | 0.34946 ^{\left\{3 \right\}} | 0.55815 ^{\left\{5 \right\}} | 0.49857 ^{\left\{4 \right\}} | 0.86629 ^{\left\{8 \right\}} | 0.56483 ^{\left\{6 \right\}} | 0.59417 ^{\left\{7 \right\}} | |
\hat{\varphi} | 0.27881 ^{\left\{1 \right\}} | 0.32553 ^{\left\{3 \right\}} | 0.29109 ^{\left\{2 \right\}} | 0.66883 ^{\left\{7 \right\}} | 0.44978 ^{\left\{4 \right\}} | 1.03760 ^{\left\{8 \right\}} | 0.55511 ^{\left\{5 \right\}} | 0.64550 ^{\left\{6 \right\}} | ||
\hat{\lambda} | 0.06369 ^{\left\{1 \right\}} | 0.06795 ^{\left\{3 \right\}} | 0.06642 ^{\left\{2 \right\}} | 0.29117 ^{\left\{7 \right\}} | 0.15725 ^{\left\{4 \right\}} | 0.54984 ^{\left\{8 \right\}} | 0.25250 ^{\left\{5 \right\}} | 0.28331 ^{\left\{6 \right\}} | ||
\hat{\theta} | 0.66344 ^{\left\{5 \right\}} | 0.73666 ^{\left\{7 \right\}} | 0.74889 ^{\left\{8 \right\}} | 0.58730 ^{\left\{3 \right\}} | 0.45144 ^{\left\{1 \right\}} | 0.66717 ^{\left\{6 \right\}} | 0.51377 ^{\left\{2 \right\}} | 0.59201 ^{\left\{4 \right\}} | ||
MREs | \hat{\vartheta} | 0.28529 ^{\left\{2 \right\}} | 0.26934 ^{\left\{1 \right\}} | 0.30152 ^{\left\{3 \right\}} | 0.37902 ^{\left\{5 \right\}} | 0.31981 ^{\left\{4 \right\}} | 0.50985 ^{\left\{8 \right\}} | 0.38416 ^{\left\{6 \right\}} | 0.39684 ^{\left\{7 \right\}} | |
\hat{\varphi} | 0.26032 ^{\left\{1 \right\}} | 0.27512 ^{\left\{3 \right\}} | 0.26454 ^{\left\{2 \right\}} | 0.35341 ^{\left\{7 \right\}} | 0.28177 ^{\left\{4 \right\}} | 0.46791 ^{\left\{8 \right\}} | 0.31999 ^{\left\{5 \right\}} | 0.34977 ^{\left\{6 \right\}} | ||
\hat{\lambda} | 0.16798 ^{\left\{1 \right\}} | 0.17255 ^{\left\{3 \right\}} | 0.17094 ^{\left\{2 \right\}} | 0.28874 ^{\left\{7 \right\}} | 0.20023 ^{\left\{4 \right\}} | 0.44375 ^{\left\{8 \right\}} | 0.27071 ^{\left\{5 \right\}} | 0.28804 ^{\left\{6 \right\}} | ||
\hat{\theta} | 0.31392 ^{\left\{6 \right\}} | 0.33013 ^{\left\{7 \right\}} | 0.33479 ^{\left\{8 \right\}} | 0.27625 ^{\left\{3 \right\}} | 0.23423 ^{\left\{1 \right\}} | 0.28849 ^{\left\{5 \right\}} | 0.25537 ^{\left\{2 \right\}} | 0.27666 ^{\left\{4 \right\}} | ||
\sum{Ranks} | {\textbf{29}} ^{\left\{1 \right\}} | {\textbf{42}} ^{\left\{3 \right\}} | {\textbf{45}} ^{\left\{4 \right\}} | {\textbf{66}} ^{\left\{6 \right\}} | {\textbf{39}} ^{\left\{2 \right\}} | {\textbf{88}} ^{\left\{8 \right\}} | {\textbf{54}} ^{\left\{5 \right\}} | {\textbf{69}} ^{\left\{7 \right\}} | ||
200 | |BIAS| | \hat{\vartheta} | 0.47555 ^{\left\{3 \right\}} | 0.44985 ^{\left\{2 \right\}} | 0.51341 ^{\left\{4 \right\}} | 0.67707 ^{\left\{6 \right\}} | 0.42436 ^{\left\{1 \right\}} | 0.89805 ^{\left\{8 \right\}} | 0.66134 ^{\left\{5 \right\}} | 0.69895 ^{\left\{7 \right\}} |
\hat{\varphi} | 0.50115 ^{\left\{2 \right\}} | 0.50837 ^{\left\{4 \right\}} | 0.50279 ^{\left\{3 \right\}} | 0.56296 ^{\left\{7 \right\}} | 0.41044 ^{\left\{1 \right\}} | 0.81679 ^{\left\{8 \right\}} | 0.51451 ^{\left\{5 \right\}} | 0.55936 ^{\left\{6 \right\}} | ||
\hat{\lambda} | 0.25019 ^{\left\{2 \right\}} | 0.25187 ^{\left\{4 \right\}} | 0.25076 ^{\left\{3 \right\}} | 0.41917 ^{\left\{7 \right\}} | 0.21499 ^{\left\{1 \right\}} | 0.65544 ^{\left\{8 \right\}} | 0.37297 ^{\left\{5 \right\}} | 0.41435 ^{\left\{6 \right\}} | ||
\hat{\theta} | 0.79159 ^{\left\{6 \right\}} | 0.80107 ^{\left\{7 \right\}} | 0.82123 ^{\left\{8 \right\}} | 0.64647 ^{\left\{3 \right\}} | 0.43028 ^{\left\{1 \right\}} | 0.75308 ^{\left\{5 \right\}} | 0.57181 ^{\left\{2 \right\}} | 0.66234 ^{\left\{4 \right\}} | ||
MSEs | \hat{\vartheta} | 0.25888 ^{\left\{2 \right\}} | 0.23847 ^{\left\{1 \right\}} | 0.29413 ^{\left\{3 \right\}} | 0.56097 ^{\left\{6 \right\}} | 0.37531 ^{\left\{4 \right\}} | 0.86783 ^{\left\{8 \right\}} | 0.54545 ^{\left\{5 \right\}} | 0.59107 ^{\left\{7 \right\}} | |
\hat{\varphi} | 0.25134 ^{\left\{1 \right\}} | 0.26082 ^{\left\{4 \right\}} | 0.25349 ^{\left\{2 \right\}} | 0.43952 ^{\left\{7 \right\}} | 0.25968 ^{\left\{3 \right\}} | 0.80523 ^{\left\{8 \right\}} | 0.37160 ^{\left\{5 \right\}} | 0.43084 ^{\left\{6 \right\}} | ||
\hat{\lambda} | 0.06261 ^{\left\{1 \right\}} | 0.06361 ^{\left\{3 \right\}} | 0.06295 ^{\left\{2 \right\}} | 0.25891 ^{\left\{7 \right\}} | 0.09970 ^{\left\{4 \right\}} | 0.52619 ^{\left\{8 \right\}} | 0.20829 ^{\left\{5 \right\}} | 0.25203 ^{\left\{6 \right\}} | ||
\hat{\theta} | 0.64670 ^{\left\{5 \right\}} | 0.66161 ^{\left\{6 \right\}} | 0.69240 ^{\left\{8 \right\}} | 0.51309 ^{\left\{3 \right\}} | 0.27527 ^{\left\{1 \right\}} | 0.68347 ^{\left\{7 \right\}} | 0.41304 ^{\left\{2 \right\}} | 0.53268 ^{\left\{4 \right\}} | ||
MREs | \hat{\vartheta} | 0.27175 ^{\left\{3 \right\}} | 0.25705 ^{\left\{2 \right\}} | 0.29338 ^{\left\{4 \right\}} | 0.38690 ^{\left\{6 \right\}} | 0.24249 ^{\left\{1 \right\}} | 0.51317 ^{\left\{8 \right\}} | 0.37791 ^{\left\{5 \right\}} | 0.39940 ^{\left\{7 \right\}} | |
\hat{\varphi} | 0.25057 ^{\left\{2 \right\}} | 0.25419 ^{\left\{4 \right\}} | 0.25139 ^{\left\{3 \right\}} | 0.28148 ^{\left\{7 \right\}} | 0.20522 ^{\left\{1 \right\}} | 0.40839 ^{\left\{8 \right\}} | 0.25725 ^{\left\{5 \right\}} | 0.27968 ^{\left\{6 \right\}} | ||
\hat{\lambda} | 0.16679 ^{\left\{2 \right\}} | 0.16791 ^{\left\{4 \right\}} | 0.16717 ^{\left\{3 \right\}} | 0.27945 ^{\left\{7 \right\}} | 0.14333 ^{\left\{1 \right\}} | 0.43696 ^{\left\{8 \right\}} | 0.24865 ^{\left\{5 \right\}} | 0.27623 ^{\left\{6 \right\}} | ||
\hat{\theta} | 0.31664 ^{\left\{6 \right\}} | 0.32043 ^{\left\{7 \right\}} | 0.32849 ^{\left\{8 \right\}} | 0.25859 ^{\left\{3 \right\}} | 0.17211 ^{\left\{1 \right\}} | 0.30123 ^{\left\{5 \right\}} | 0.22872 ^{\left\{2 \right\}} | 0.26494 ^{\left\{4 \right\}} | ||
\sum{Ranks} | {\textbf{35}} ^{\left\{2 \right\}} | {\textbf{48}} ^{\left\{3 \right\}} | {\textbf{51}} ^{\left\{4.5 \right\}} | {\textbf{69}} ^{\left\{6.5 \right\}} | {\textbf{20}} ^{\left\{1 \right\}} | {\textbf{89}} ^{\left\{8 \right\}} | {\textbf{51}} ^{\left\{4.5 \right\}} | {\textbf{69}} ^{\left\{6.5 \right\}} | ||
500 | |BIAS| | \hat{\vartheta} | 0.47314 ^{\left\{3 \right\}} | 0.44590 ^{\left\{2 \right\}} | 0.51215 ^{\left\{4 \right\}} | 0.64549 ^{\left\{6 \right\}} | 0.23589 ^{\left\{1 \right\}} | 0.89642 ^{\left\{8 \right\}} | 0.57765 ^{\left\{5 \right\}} | 0.66728 ^{\left\{7 \right\}} |
\hat{\varphi} | 0.50000 ^{\left\{5 \right\}} | 0.50031 ^{\left\{7 \right\}} | 0.50001 ^{\left\{6 \right\}} | 0.44580 ^{\left\{4 \right\}} | 0.25497 ^{\left\{1 \right\}} | 0.66140 ^{\left\{8 \right\}} | 0.39329 ^{\left\{2 \right\}} | 0.42269 ^{\left\{3 \right\}} | ||
\hat{\lambda} | 0.25000 ^{\left\{2 \right\}} | 0.25003 ^{\left\{4 \right\}} | 0.25001 ^{\left\{3 \right\}} | 0.38068 ^{\left\{6 \right\}} | 0.11500 ^{\left\{1 \right\}} | 0.63857 ^{\left\{8 \right\}} | 0.31593 ^{\left\{5 \right\}} | 0.38454 ^{\left\{7 \right\}} | ||
\hat{\theta} | 0.79445 ^{\left\{5 \right\}} | 0.80134 ^{\left\{6 \right\}} | 0.82604 ^{\left\{8 \right\}} | 0.59243 ^{\left\{3 \right\}} | 0.25280 ^{\left\{1 \right\}} | 0.80505 ^{\left\{7 \right\}} | 0.49356 ^{\left\{2 \right\}} | 0.61821 ^{\left\{4 \right\}} | ||
MSEs | \hat{\vartheta} | 0.23703 ^{\left\{3 \right\}} | 0.21187 ^{\left\{2 \right\}} | 0.27432 ^{\left\{4 \right\}} | 0.51809 ^{\left\{6 \right\}} | 0.19898 ^{\left\{1 \right\}} | 0.86166 ^{\left\{8 \right\}} | 0.44538 ^{\left\{5 \right\}} | 0.54549 ^{\left\{7 \right\}} | |
\hat{\varphi} | 0.25000 ^{\left\{3 \right\}} | 0.25034 ^{\left\{5 \right\}} | 0.25001 ^{\left\{4 \right\}} | 0.28548 ^{\left\{7 \right\}} | 0.11705 ^{\left\{1 \right\}} | 0.56366 ^{\left\{8 \right\}} | 0.22462 ^{\left\{2 \right\}} | 0.25796 ^{\left\{6 \right\}} | ||
\hat{\lambda} | 0.06250 ^{\left\{2.5 \right\}} | 0.06252 ^{\left\{4 \right\}} | 0.06250 ^{\left\{2.5 \right\}} | 0.20888 ^{\left\{6 \right\}} | 0.03935 ^{\left\{1 \right\}} | 0.50179 ^{\left\{8 \right\}} | 0.14873 ^{\left\{5 \right\}} | 0.20922 ^{\left\{7 \right\}} | ||
\hat{\theta} | 0.63903 ^{\left\{5 \right\}} | 0.64949 ^{\left\{6 \right\}} | 0.68943 ^{\left\{7 \right\}} | 0.43597 ^{\left\{3 \right\}} | 0.12546 ^{\left\{1 \right\}} | 0.74499 ^{\left\{8 \right\}} | 0.31912 ^{\left\{2 \right\}} | 0.46804 ^{\left\{4 \right\}} | ||
MREs | \hat{\vartheta} | 0.27037 ^{\left\{3 \right\}} | 0.25480 ^{\left\{2 \right\}} | 0.29266 ^{\left\{4 \right\}} | 0.36885 ^{\left\{6 \right\}} | 0.13479 ^{\left\{1 \right\}} | 0.51224 ^{\left\{8 \right\}} | 0.33008 ^{\left\{5 \right\}} | 0.38130 ^{\left\{7 \right\}} | |
\hat{\varphi} | 0.25000 ^{\left\{5.5 \right\}} | 0.25015 ^{\left\{7 \right\}} | 0.25000 ^{\left\{5.5 \right\}} | 0.22290 ^{\left\{4 \right\}} | 0.12748 ^{\left\{1 \right\}} | 0.33070 ^{\left\{8 \right\}} | 0.19665 ^{\left\{2 \right\}} | 0.21134 ^{\left\{3 \right\}} | ||
\hat{\lambda} | 0.16667 ^{\left\{2.5 \right\}} | 0.16669 ^{\left\{4 \right\}} | 0.16667 ^{\left\{2.5 \right\}} | 0.25379 ^{\left\{6 \right\}} | 0.07667 ^{\left\{1 \right\}} | 0.42571 ^{\left\{8 \right\}} | 0.21062 ^{\left\{5 \right\}} | 0.25636 ^{\left\{7 \right\}} | ||
\hat{\theta} | 0.31778 ^{\left\{5 \right\}} | 0.32053 ^{\left\{6 \right\}} | 0.33042 ^{\left\{8 \right\}} | 0.23697 ^{\left\{3 \right\}} | 0.10112 ^{\left\{1 \right\}} | 0.32202 ^{\left\{7 \right\}} | 0.19742 ^{\left\{2 \right\}} | 0.24728 ^{\left\{4 \right\}} | ||
\sum{Ranks} | {\textbf{44.5}} ^{\left\{3 \right\}} | {\textbf{55}} ^{\left\{4 \right\}} | {\textbf{58.5}} ^{\left\{5 \right\}} | {\textbf{60}} ^{\left\{6 \right\}} | {\textbf{12}} ^{\left\{1 \right\}} | {\textbf{94}} ^{\left\{8 \right\}} | {\textbf{42}} ^{\left\{2 \right\}} | {\textbf{66}} ^{\left\{7 \right\}} |
n | Est. | Est. Par. | MLEs | LSEs | WLSEs | CRVMEs | MPSEs | PCEs | ADEs | RADEs |
20 | |BIAS| | \hat{\vartheta} | 1.05821 ^{\left\{8 \right\}} | 0.62985 ^{\left\{3 \right\}} | 0.96882 ^{\left\{6 \right\}} | 0.61879 ^{\left\{2 \right\}} | 0.39728 ^{\left\{1 \right\}} | 1.00062 ^{\left\{7 \right\}} | 0.93184 ^{\left\{5 \right\}} | 0.74851 ^{\left\{4 \right\}} |
\hat{\varphi} | 0.58239 ^{\left\{4 \right\}} | 0.61540 ^{\left\{8 \right\}} | 0.57588 ^{\left\{3 \right\}} | 0.61442 ^{\left\{7 \right\}} | 0.16810 ^{\left\{1 \right\}} | 0.59648 ^{\left\{6 \right\}} | 0.54321 ^{\left\{2 \right\}} | 0.58344 ^{\left\{5 \right\}} | ||
\hat{\lambda} | 0.09205 ^{\left\{8 \right\}} | 0.08984 ^{\left\{5 \right\}} | 0.08749 ^{\left\{3 \right\}} | 0.09161 ^{\left\{6 \right\}} | 0.07490 ^{\left\{1 \right\}} | 0.09181 ^{\left\{7 \right\}} | 0.08360 ^{\left\{2 \right\}} | 0.08890 ^{\left\{4 \right\}} | ||
\hat{\theta} | 0.65193 ^{\left\{8 \right\}} | 0.48317 ^{\left\{2 \right\}} | 0.52978 ^{\left\{5 \right\}} | 0.51841 ^{\left\{4 \right\}} | 0.37874 ^{\left\{1 \right\}} | 0.57738 ^{\left\{7 \right\}} | 0.51808 ^{\left\{3 \right\}} | 0.53685 ^{\left\{6 \right\}} | ||
MSEs | \hat{\vartheta} | 1.62863 ^{\left\{8 \right\}} | 0.80009 ^{\left\{2 \right\}} | 1.26747 ^{\left\{6 \right\}} | 0.83422 ^{\left\{3 \right\}} | 0.44242 ^{\left\{1 \right\}} | 1.52407 ^{\left\{7 \right\}} | 1.26323 ^{\left\{5 \right\}} | 1.02485 ^{\left\{4 \right\}} | |
\hat{\varphi} | 0.42299 ^{\left\{3 \right\}} | 0.47351 ^{\left\{8 \right\}} | 0.43072 ^{\left\{4 \right\}} | 0.47310 ^{\left\{7 \right\}} | 0.39842 ^{\left\{2 \right\}} | 0.45399 ^{\left\{6 \right\}} | 0.39008 ^{\left\{1 \right\}} | 0.43912 ^{\left\{5 \right\}} | ||
\hat{\lambda} | 0.01191 ^{\left\{6 \right\}} | 0.01157 ^{\left\{5 \right\}} | 0.01104 ^{\left\{3 \right\}} | 0.01199 ^{\left\{7 \right\}} | 0.00839 ^{\left\{1 \right\}} | 0.01226 ^{\left\{8 \right\}} | 0.01037 ^{\left\{2 \right\}} | 0.01130 ^{\left\{4 \right\}} | ||
\hat{\theta} | 0.56633 ^{\left\{8 \right\}} | 0.35145 ^{\left\{2 \right\}} | 0.39993 ^{\left\{3 \right\}} | 0.40530 ^{\left\{5 \right\}} | 0.22597 ^{\left\{1 \right\}} | 0.46238 ^{\left\{7 \right\}} | 0.39998 ^{\left\{4 \right\}} | 0.42492 ^{\left\{6 \right\}} | ||
MREs | \hat{\vartheta} | 0.30235 ^{\left\{8 \right\}} | 0.17996 ^{\left\{3 \right\}} | 0.27681 ^{\left\{6 \right\}} | 0.17680 ^{\left\{2 \right\}} | 0.11351 ^{\left\{1 \right\}} | 0.28589 ^{\left\{7 \right\}} | 0.26624 ^{\left\{5 \right\}} | 0.21386 ^{\left\{4 \right\}} | |
\hat{\varphi} | 0.77652 ^{\left\{4 \right\}} | 0.82054 ^{\left\{8 \right\}} | 0.76784 ^{\left\{3 \right\}} | 0.81923 ^{\left\{7 \right\}} | 0.73001 ^{\left\{2 \right\}} | 0.79531 ^{\left\{6 \right\}} | 0.72428 ^{\left\{1 \right\}} | 0.77792 ^{\left\{5 \right\}} | ||
\hat{\lambda} | 0.18410 ^{\left\{8 \right\}} | 0.17968 ^{\left\{5 \right\}} | 0.17498 ^{\left\{3 \right\}} | 0.18321 ^{\left\{6 \right\}} | 0.14981 ^{\left\{1 \right\}} | 0.18363 ^{\left\{7 \right\}} | 0.16720 ^{\left\{2 \right\}} | 0.17780 ^{\left\{4 \right\}} | ||
\hat{\theta} | 0.52155 ^{\left\{8 \right\}} | 0.38654 ^{\left\{2 \right\}} | 0.42382 ^{\left\{5 \right\}} | 0.41473 ^{\left\{4 \right\}} | 0.30299 ^{\left\{1 \right\}} | 0.46190 ^{\left\{7 \right\}} | 0.41446 ^{\left\{3 \right\}} | 0.42948 ^{\left\{6 \right\}} | ||
\sum{Ranks} | {\textbf{81}} ^{\left\{7 \right\}} | {\textbf{53}} ^{\left\{4 \right\}} | {\textbf{50}} ^{\left\{3 \right\}} | {\textbf{60}} ^{\left\{6 \right\}} | {\textbf{14}} ^{\left\{1 \right\}} | {\textbf{82}} ^{\left\{8 \right\}} | {\textbf{35}} ^{\left\{2 \right\}} | {\textbf{57}} ^{\left\{5 \right\}} | ||
80 | |BIAS| | \hat{\vartheta} | 1.30458 ^{\left\{8 \right\}} | 0.99944 ^{\left\{2 \right\}} | 1.19014 ^{\left\{7 \right\}} | 1.02885 ^{\left\{3 \right\}} | 0.28553 ^{\left\{1 \right\}} | 1.10670 ^{\left\{4 \right\}} | 1.14568 ^{\left\{6 \right\}} | 1.11457 ^{\left\{5 \right\}} |
\hat{\varphi} | 0.32983 ^{\left\{3 \right\}} | 0.40620 ^{\left\{6 \right\}} | 0.34267 ^{\left\{4 \right\}} | 0.40746 ^{\left\{7 \right\}} | 0.27616 ^{\left\{1 \right\}} | 0.42471 ^{\left\{8 \right\}} | 0.32604 ^{\left\{2 \right\}} | 0.35934 ^{\left\{5 \right\}} | ||
\hat{\lambda} | 0.06496 ^{\left\{7 \right\}} | 0.06255 ^{\left\{5 \right\}} | 0.05976 ^{\left\{3 \right\}} | 0.06558 ^{\left\{8 \right\}} | 0.03810 ^{\left\{1 \right\}} | 0.06030 ^{\left\{4 \right\}} | 0.05724 ^{\left\{2 \right\}} | 0.06303 ^{\left\{6 \right\}} | ||
\hat{\theta} | 0.60157 ^{\left\{8 \right\}} | 0.45112 ^{\left\{2 \right\}} | 0.47825 ^{\left\{5 \right\}} | 0.49541 ^{\left\{6 \right\}} | 0.19099 ^{\left\{1 \right\}} | 0.45370 ^{\left\{3 \right\}} | 0.45453 ^{\left\{4 \right\}} | 0.50033 ^{\left\{7 \right\}} | ||
MSEs | \hat{\vartheta} | 1.98067 ^{\left\{8 \right\}} | 1.42246 ^{\left\{2 \right\}} | 1.62721 ^{\left\{7 \right\}} | 1.51028 ^{\left\{3 \right\}} | 0.28600 ^{\left\{1 \right\}} | 1.54773 ^{\left\{5 \right\}} | 1.54350 ^{\left\{4 \right\}} | 1.62460 ^{\left\{6 \right\}} | |
\hat{\varphi} | 0.16347 ^{\left\{2 \right\}} | 0.24145 ^{\left\{7 \right\}} | 0.17842 ^{\left\{4 \right\}} | 0.24070 ^{\left\{6 \right\}} | 0.12275 ^{\left\{1 \right\}} | 0.27309 ^{\left\{8 \right\}} | 0.16427 ^{\left\{3 \right\}} | 0.19753 ^{\left\{5 \right\}} | ||
\hat{\lambda} | 0.00614 ^{\left\{7 \right\}} | 0.00590 ^{\left\{5 \right\}} | 0.00549 ^{\left\{3 \right\}} | 0.00646 ^{\left\{8 \right\}} | 0.00224 ^{\left\{1 \right\}} | 0.00582 ^{\left\{4 \right\}} | 0.00513 ^{\left\{2 \right\}} | 0.00600 ^{\left\{6 \right\}} | ||
\hat{\theta} | 0.50150 ^{\left\{8 \right\}} | 0.33442 ^{\left\{3 \right\}} | 0.35764 ^{\left\{5 \right\}} | 0.39078 ^{\left\{6 \right\}} | 0.06108 ^{\left\{1 \right\}} | 0.33595 ^{\left\{4 \right\}} | 0.33240 ^{\left\{2 \right\}} | 0.39877 ^{\left\{7 \right\}} | ||
MREs | \hat{\vartheta} | 0.37274 ^{\left\{8 \right\}} | 0.28555 ^{\left\{2 \right\}} | 0.34004 ^{\left\{7 \right\}} | 0.29396 ^{\left\{3 \right\}} | 0.08158 ^{\left\{1 \right\}} | 0.31620 ^{\left\{4 \right\}} | 0.32734 ^{\left\{6 \right\}} | 0.31845 ^{\left\{5 \right\}} | |
\hat{\varphi} | 0.43977 ^{\left\{3 \right\}} | 0.54161 ^{\left\{6 \right\}} | 0.45690 ^{\left\{4 \right\}} | 0.54328 ^{\left\{7 \right\}} | 0.36821 ^{\left\{1 \right\}} | 0.56628 ^{\left\{8 \right\}} | 0.43472 ^{\left\{2 \right\}} | 0.47912 ^{\left\{5 \right\}} | ||
\hat{\lambda} | 0.12992 ^{\left\{7 \right\}} | 0.12509 ^{\left\{5 \right\}} | 0.11952 ^{\left\{3 \right\}} | 0.13116 ^{\left\{8 \right\}} | 0.07620 ^{\left\{1 \right\}} | 0.12061 ^{\left\{4 \right\}} | 0.11448 ^{\left\{2 \right\}} | 0.12607 ^{\left\{6 \right\}} | ||
\hat{\theta} | 0.48126 ^{\left\{8 \right\}} | 0.36089 ^{\left\{2 \right\}} | 0.38260 ^{\left\{5 \right\}} | 0.39633 ^{\left\{6 \right\}} | 0.15279 ^{\left\{1 \right\}} | 0.36296 ^{\left\{3 \right\}} | 0.36362 ^{\left\{4 \right\}} | 0.40027 ^{\left\{7 \right\}} | ||
\sum{Ranks} | {\textbf{77}} ^{\left\{8 \right\}} | {\textbf{47}} ^{\left\{3 \right\}} | {\textbf{57}} ^{\left\{4 \right\}} | {\textbf{71}} ^{\left\{7 \right\}} | {\textbf{12}} ^{\left\{1 \right\}} | {\textbf{59}} ^{\left\{5 \right\}} | {\textbf{39}} ^{\left\{2 \right\}} | {\textbf{70}} ^{\left\{6 \right\}} | ||
200 | |BIAS| | \hat{\vartheta} | 1.18071 ^{\left\{8 \right\}} | 1.13329 ^{\left\{4 \right\}} | 1.15680 ^{\left\{6 \right\}} | 1.15101 ^{\left\{5 \right\}} | 0.12881 ^{\left\{1 \right\}} | 1.09310 ^{\left\{2 \right\}} | 1.11947 ^{\left\{3 \right\}} | 1.17399 ^{\left\{7 \right\}} |
\hat{\varphi} | 0.21288 ^{\left\{2 \right\}} | 0.27282 ^{\left\{6 \right\}} | 0.22827 ^{\left\{4 \right\}} | 0.28207 ^{\left\{7 \right\}} | 0.16810 ^{\left\{1 \right\}} | 0.31936 ^{\left\{8 \right\}} | 0.21687 ^{\left\{3 \right\}} | 0.23691 ^{\left\{5 \right\}} | ||
\hat{\lambda} | 0.05455 ^{\left\{5 \right\}} | 0.05483 ^{\left\{6 \right\}} | 0.05139 ^{\left\{4 \right\}} | 0.05648 ^{\left\{8 \right\}} | 0.02373 ^{\left\{1 \right\}} | 0.04586 ^{\left\{2 \right\}} | 0.05000 ^{\left\{3 \right\}} | 0.05607 ^{\left\{7 \right\}} | ||
\hat{\theta} | 0.50995 ^{\left\{8 \right\}} | 0.46810 ^{\left\{5 \right\}} | 0.45161 ^{\left\{4 \right\}} | 0.48892 ^{\left\{7 \right\}} | 0.11088 ^{\left\{1 \right\}} | 0.36948 ^{\left\{2 \right\}} | 0.42847 ^{\left\{3 \right\}} | 0.48519 ^{\left\{6 \right\}} | ||
MSEs | \hat{\vartheta} | 1.65210 ^{\left\{6 \right\}} | 1.59933 ^{\left\{5 \right\}} | 1.54359 ^{\left\{4 \right\}} | 1.65457 ^{\left\{7 \right\}} | 0.11230 ^{\left\{1 \right\}} | 1.41356 ^{\left\{2 \right\}} | 1.45126 ^{\left\{3 \right\}} | 1.65710 ^{\left\{8 \right\}} | |
\hat{\varphi} | 0.06995 ^{\left\{2 \right\}} | 0.11564 ^{\left\{6 \right\}} | 0.08167 ^{\left\{4 \right\}} | 0.12209 ^{\left\{7 \right\}} | 0.04607 ^{\left\{1 \right\}} | 0.16683 ^{\left\{8 \right\}} | 0.07397 ^{\left\{3 \right\}} | 0.08772 ^{\left\{5 \right\}} | ||
\hat{\lambda} | 0.00441 ^{\left\{5 \right\}} | 0.00457 ^{\left\{6 \right\}} | 0.00403 ^{\left\{4 \right\}} | 0.00483 ^{\left\{8 \right\}} | 0.00088 ^{\left\{1 \right\}} | 0.00341 ^{\left\{2 \right\}} | 0.00386 ^{\left\{3 \right\}} | 0.00477 ^{\left\{7 \right\}} | ||
\hat{\theta} | 0.38578 ^{\left\{8 \right\}} | 0.35447 ^{\left\{5 \right\}} | 0.32071 ^{\left\{4 \right\}} | 0.38130 ^{\left\{7 \right\}} | 0.02076 ^{\left\{1 \right\}} | 0.24604 ^{\left\{2 \right\}} | 0.29346 ^{\left\{3 \right\}} | 0.38069 ^{\left\{6 \right\}} | ||
MREs | \hat{\vartheta} | 0.33734 ^{\left\{8 \right\}} | 0.32380 ^{\left\{4 \right\}} | 0.33052 ^{\left\{6 \right\}} | 0.32886 ^{\left\{5 \right\}} | 0.03680 ^{\left\{1 \right\}} | 0.31232 ^{\left\{2 \right\}} | 0.31985 ^{\left\{3 \right\}} | 0.33543 ^{\left\{7 \right\}} | |
\hat{\varphi} | 0.28384 ^{\left\{2 \right\}} | 0.36376 ^{\left\{6 \right\}} | 0.30437 ^{\left\{4 \right\}} | 0.37610 ^{\left\{7 \right\}} | 0.22413 ^{\left\{1 \right\}} | 0.42582 ^{\left\{8 \right\}} | 0.28917 ^{\left\{3 \right\}} | 0.31587 ^{\left\{5 \right\}} | ||
\hat{\lambda} | 0.10910 ^{\left\{5 \right\}} | 0.10967 ^{\left\{6 \right\}} | 0.10278 ^{\left\{4 \right\}} | 0.11295 ^{\left\{8 \right\}} | 0.04745 ^{\left\{1 \right\}} | 0.09173 ^{\left\{2 \right\}} | 0.10000 ^{\left\{3 \right\}} | 0.11214 ^{\left\{7 \right\}} | ||
\hat{\theta} | 0.40796 ^{\left\{8 \right\}} | 0.37448 ^{\left\{5 \right\}} | 0.36129 ^{\left\{4 \right\}} | 0.39114 ^{\left\{7 \right\}} | 0.08871 ^{\left\{1 \right\}} | 0.29559 ^{\left\{2 \right\}} | 0.34277 ^{\left\{3 \right\}} | 0.38815 ^{\left\{6 \right\}} | ||
\sum{Ranks} | {\textbf{67}} ^{\left\{6 \right\}} | {\textbf{64}} ^{\left\{5 \right\}} | {\textbf{52}} ^{\left\{4 \right\}} | {\textbf{83}} ^{\left\{8 \right\}} | {\textbf{12}} ^{\left\{1 \right\}} | {\textbf{42}} ^{\left\{3 \right\}} | {\textbf{36}} ^{\left\{2 \right\}} | {\textbf{76}} ^{\left\{7 \right\}} | ||
500 | |BIAS| | \hat{\vartheta} | 0.99609 ^{\left\{2 \right\}} | 1.12481 ^{\left\{6 \right\}} | 1.05940 ^{\left\{5 \right\}} | 1.12860 ^{\left\{7 \right\}} | 0.04256 ^{\left\{1 \right\}} | 1.04542 ^{\left\{4 \right\}} | 1.03887 ^{\left\{3 \right\}} | 1.14079 ^{\left\{8 \right\}} |
\hat{\varphi} | 0.14496 ^{\left\{2 \right\}} | 0.18941 ^{\left\{6 \right\}} | 0.15844 ^{\left\{4 \right\}} | 0.19306 ^{\left\{7 \right\}} | 0.10411 ^{\left\{1 \right\}} | 0.23525 ^{\left\{8 \right\}} | 0.15349 ^{\left\{3 \right\}} | 0.16453 ^{\left\{5 \right\}} | ||
\hat{\lambda} | 0.04309 ^{\left\{3 \right\}} | 0.05010 ^{\left\{7 \right\}} | 0.04425 ^{\left\{5 \right\}} | 0.04998 ^{\left\{6 \right\}} | 0.01474 ^{\left\{1 \right\}} | 0.03603 ^{\left\{2 \right\}} | 0.04334 ^{\left\{4 \right\}} | 0.05109 ^{\left\{8 \right\}} | ||
\hat{\theta} | 0.38900 ^{\left\{4 \right\}} | 0.44670 ^{\left\{6 \right\}} | 0.39875 ^{\left\{5 \right\}} | 0.45266 ^{\left\{7 \right\}} | 0.06374 ^{\left\{1 \right\}} | 0.31824 ^{\left\{2 \right\}} | 0.38560 ^{\left\{3 \right\}} | 0.45867 ^{\left\{8 \right\}} | ||
MSEs | \hat{\vartheta} | 1.21294 ^{\left\{2 \right\}} | 1.50352 ^{\left\{6 \right\}} | 1.31440 ^{\left\{5 \right\}} | 1.52047 ^{\left\{8 \right\}} | 0.02893 ^{\left\{1 \right\}} | 1.25338 ^{\left\{3 \right\}} | 1.26686 ^{\left\{4 \right\}} | 1.51668 ^{\left\{7 \right\}} | |
\hat{\varphi} | 0.03263 ^{\left\{2 \right\}} | 0.05612 ^{\left\{6 \right\}} | 0.03902 ^{\left\{4 \right\}} | 0.05818 ^{\left\{7 \right\}} | 0.01736 ^{\left\{1 \right\}} | 0.09221 ^{\left\{8 \right\}} | 0.03639 ^{\left\{3 \right\}} | 0.04120 ^{\left\{5 \right\}} | ||
\hat{\lambda} | 0.00278 ^{\left\{3 \right\}} | 0.00378 ^{\left\{6.5 \right\}} | 0.00295 ^{\left\{5 \right\}} | 0.00378 ^{\left\{6.5 \right\}} | 0.00034 ^{\left\{1 \right\}} | 0.00220 ^{\left\{2 \right\}} | 0.00283 ^{\left\{4 \right\}} | 0.00400 ^{\left\{8 \right\}} | ||
\hat{\theta} | 0.23678 ^{\left\{4 \right\}} | 0.31580 ^{\left\{6 \right\}} | 0.25000 ^{\left\{5 \right\}} | 0.32430 ^{\left\{7 \right\}} | 0.00703 ^{\left\{1 \right\}} | 0.18536 ^{\left\{2 \right\}} | 0.23280 ^{\left\{3 \right\}} | 0.33477 ^{\left\{8 \right\}} | ||
MREs | \hat{\vartheta} | 0.28460 ^{\left\{2 \right\}} | 0.32137 ^{\left\{6 \right\}} | 0.30269 ^{\left\{5 \right\}} | 0.32246 ^{\left\{7 \right\}} | 0.01216 ^{\left\{1 \right\}} | 0.29869 ^{\left\{4 \right\}} | 0.29682 ^{\left\{3 \right\}} | 0.32594 ^{\left\{8 \right\}} | |
\hat{\varphi} | 0.19328 ^{\left\{2 \right\}} | 0.25255 ^{\left\{6 \right\}} | 0.21126 ^{\left\{4 \right\}} | 0.25741 ^{\left\{7 \right\}} | 0.13881 ^{\left\{1 \right\}} | 0.31367 ^{\left\{8 \right\}} | 0.20466 ^{\left\{3 \right\}} | 0.21938 ^{\left\{5 \right\}} | ||
\hat{\lambda} | 0.08617 ^{\left\{3 \right\}} | 0.10020 ^{\left\{7 \right\}} | 0.08850 ^{\left\{5 \right\}} | 0.09997 ^{\left\{6 \right\}} | 0.02948 ^{\left\{1 \right\}} | 0.07207 ^{\left\{2 \right\}} | 0.08669 ^{\left\{4 \right\}} | 0.10217 ^{\left\{8 \right\}} | ||
\hat{\theta} | 0.31120 ^{\left\{4 \right\}} | 0.35736 ^{\left\{6 \right\}} | 0.31900 ^{\left\{5 \right\}} | 0.36213 ^{\left\{7 \right\}} | 0.05100 ^{\left\{1 \right\}} | 0.25459 ^{\left\{2 \right\}} | 0.30848 ^{\left\{3 \right\}} | 0.36694 ^{\left\{8 \right\}} | ||
\sum{Ranks} | {\textbf{33}} ^{\left\{2 \right\}} | {\textbf{74.5}} ^{\left\{6 \right\}} | {\textbf{57}} ^{\left\{5 \right\}} | {\textbf{82.5}} ^{\left\{7 \right\}} | {\textbf{12}} ^{\left\{1 \right\}} | {\textbf{47}} ^{\left\{4 \right\}} | {\textbf{40}} ^{\left\{3 \right\}} | {\textbf{86}} ^{\left\{8 \right\}} |
n | Est. | Est. Par. | MLEs | LSEs | WLSEs | CRVMEs | MPSEs | PCEs | ADEs | RADEs |
20 | |BIAS| | \hat{\vartheta} | 0.98923 ^{\left\{7 \right\}} | 0.64331 ^{\left\{3 \right\}} | 0.95672 ^{\left\{6 \right\}} | 0.63413 ^{\left\{2 \right\}} | 0.44818 ^{\left\{1 \right\}} | 1.04755 ^{\left\{8 \right\}} | 0.90857 ^{\left\{5 \right\}} | 0.78916 ^{\left\{4 \right\}} |
\hat{\varphi} | 0.95367 ^{\left\{6 \right\}} | 0.90318 ^{\left\{4 \right\}} | 0.88553 ^{\left\{3 \right\}} | 0.95450 ^{\left\{7 \right\}} | 0.31253 ^{\left\{1 \right\}} | 0.97463 ^{\left\{8 \right\}} | 0.85825 ^{\left\{2 \right\}} | 0.93652 ^{\left\{5 \right\}} | ||
\hat{\lambda} | 0.50387 ^{\left\{8 \right\}} | 0.29370 ^{\left\{2 \right\}} | 0.38814 ^{\left\{5 \right\}} | 0.31558 ^{\left\{3 \right\}} | 0.23477 ^{\left\{1 \right\}} | 0.41787 ^{\left\{7 \right\}} | 0.38863 ^{\left\{6 \right\}} | 0.36144 ^{\left\{4 \right\}} | ||
\hat{\theta} | 0.83605 ^{\left\{8 \right\}} | 0.71444 ^{\left\{3 \right\}} | 0.81238 ^{\left\{7 \right\}} | 0.68991 ^{\left\{2 \right\}} | 0.62151 ^{\left\{1 \right\}} | 0.74404 ^{\left\{5 \right\}} | 0.75604 ^{\left\{6 \right\}} | 0.71561 ^{\left\{4 \right\}} | ||
MSEs | \hat{\vartheta} | 1.35323 ^{\left\{7 \right\}} | 0.71389 ^{\left\{2 \right\}} | 1.18225 ^{\left\{6 \right\}} | 0.73718 ^{\left\{3 \right\}} | 0.37586 ^{\left\{1 \right\}} | 1.57224 ^{\left\{8 \right\}} | 1.13154 ^{\left\{5 \right\}} | 1.02701 ^{\left\{4 \right\}} | |
\hat{\varphi} | 1.18857 ^{\left\{8 \right\}} | 1.06788 ^{\left\{4 \right\}} | 1.02750 ^{\left\{3 \right\}} | 1.18599 ^{\left\{7 \right\}} | 0.87512 ^{\left\{1 \right\}} | 1.15778 ^{\left\{6 \right\}} | 0.96462 ^{\left\{2 \right\}} | 1.13400 ^{\left\{5 \right\}} | ||
\hat{\lambda} | 0.36432 ^{\left\{8 \right\}} | 0.14714 ^{\left\{2 \right\}} | 0.22539 ^{\left\{5 \right\}} | 0.17712 ^{\left\{3 \right\}} | 0.09172 ^{\left\{1 \right\}} | 0.28638 ^{\left\{7 \right\}} | 0.23923 ^{\left\{6 \right\}} | 0.22267 ^{\left\{4 \right\}} | ||
\hat{\theta} | 0.77865 ^{\left\{8 \right\}} | 0.64926 ^{\left\{4 \right\}} | 0.77561 ^{\left\{7 \right\}} | 0.60519 ^{\left\{2 \right\}} | 0.52925 ^{\left\{1 \right\}} | 0.67762 ^{\left\{5 \right\}} | 0.68866 ^{\left\{6 \right\}} | 0.64046 ^{\left\{3 \right\}} | ||
MREs | \hat{\vartheta} | 0.28264 ^{\left\{7 \right\}} | 0.18380 ^{\left\{3 \right\}} | 0.27335 ^{\left\{6 \right\}} | 0.18118 ^{\left\{2 \right\}} | 0.12805 ^{\left\{1 \right\}} | 0.29930 ^{\left\{8 \right\}} | 0.25959 ^{\left\{5 \right\}} | 0.22547 ^{\left\{4 \right\}} | |
\hat{\varphi} | 0.47683 ^{\left\{6 \right\}} | 0.45159 ^{\left\{4 \right\}} | 0.44276 ^{\left\{3 \right\}} | 0.47725 ^{\left\{7 \right\}} | 0.40539 ^{\left\{1 \right\}} | 0.48731 ^{\left\{8 \right\}} | 0.42912 ^{\left\{2 \right\}} | 0.46826 ^{\left\{5 \right\}} | ||
\hat{\lambda} | 0.33591 ^{\left\{8 \right\}} | 0.19580 ^{\left\{2 \right\}} | 0.25876 ^{\left\{5 \right\}} | 0.21038 ^{\left\{3 \right\}} | 0.15651 ^{\left\{1 \right\}} | 0.27858 ^{\left\{7 \right\}} | 0.25908 ^{\left\{6 \right\}} | 0.24096 ^{\left\{4 \right\}} | ||
\hat{\theta} | 0.33442 ^{\left\{8 \right\}} | 0.28578 ^{\left\{3 \right\}} | 0.32495 ^{\left\{7 \right\}} | 0.27596 ^{\left\{2 \right\}} | 0.24860 ^{\left\{1 \right\}} | 0.29762 ^{\left\{5 \right\}} | 0.30242 ^{\left\{6 \right\}} | 0.28624 ^{\left\{4 \right\}} | ||
\sum{Ranks} | {\textbf{89}} ^{\left\{8 \right\}} | {\textbf{36}} ^{\left\{2 \right\}} | {\textbf{63}} ^{\left\{6 \right\}} | {\textbf{43}} ^{\left\{3 \right\}} | {\textbf{12}} ^{\left\{1 \right\}} | {\textbf{82}} ^{\left\{7 \right\}} | {\textbf{57}} ^{\left\{5 \right\}} | {\textbf{50}} ^{\left\{4 \right\}} | ||
80 | |BIAS| | \hat{\vartheta} | 1.00512 ^{\left\{8 \right\}} | 0.76116 ^{\left\{3 \right\}} | 0.98814 ^{\left\{7 \right\}} | 0.76068 ^{\left\{2 \right\}} | 0.22490 ^{\left\{1 \right\}} | 0.94177 ^{\left\{5 \right\}} | 0.95845 ^{\left\{6 \right\}} | 0.83406 ^{\left\{4 \right\}} |
\hat{\varphi} | 0.56247 ^{\left\{3 \right\}} | 0.64453 ^{\left\{6 \right\}} | 0.57353 ^{\left\{4 \right\}} | 0.64522 ^{\left\{7 \right\}} | 0.46925 ^{\left\{1 \right\}} | 0.76277 ^{\left\{8 \right\}} | 0.55915 ^{\left\{2 \right\}} | 0.63949 ^{\left\{5 \right\}} | ||
\hat{\lambda} | 0.45485 ^{\left\{8 \right\}} | 0.31115 ^{\left\{2 \right\}} | 0.36895 ^{\left\{6 \right\}} | 0.33000 ^{\left\{3 \right\}} | 0.11306 ^{\left\{1 \right\}} | 0.39112 ^{\left\{7 \right\}} | 0.36093 ^{\left\{5 \right\}} | 0.34405 ^{\left\{4 \right\}} | ||
\hat{\theta} | 0.76702 ^{\left\{8 \right\}} | 0.62063 ^{\left\{3 \right\}} | 0.68635 ^{\left\{7 \right\}} | 0.62598 ^{\left\{4 \right\}} | 0.33066 ^{\left\{1 \right\}} | 0.60384 ^{\left\{2 \right\}} | 0.66063 ^{\left\{6 \right\}} | 0.63130 ^{\left\{5 \right\}} | ||
MSEs | \hat{\vartheta} | 1.24539 ^{\left\{7 \right\}} | 0.92842 ^{\left\{2 \right\}} | 1.16588 ^{\left\{6 \right\}} | 0.94580 ^{\left\{3 \right\}} | 0.13919 ^{\left\{1 \right\}} | 1.25646 ^{\left\{8 \right\}} | 1.12647 ^{\left\{5 \right\}} | 1.05555 ^{\left\{4 \right\}} | |
\hat{\varphi} | 0.45689 ^{\left\{3 \right\}} | 0.57098 ^{\left\{6 \right\}} | 0.46410 ^{\left\{4 \right\}} | 0.57244 ^{\left\{7 \right\}} | 0.32482 ^{\left\{1 \right\}} | 0.71835 ^{\left\{8 \right\}} | 0.44181 ^{\left\{2 \right\}} | 0.55971 ^{\left\{5 \right\}} | ||
\hat{\lambda} | 0.28629 ^{\left\{8 \right\}} | 0.17260 ^{\left\{2 \right\}} | 0.20504 ^{\left\{5 \right\}} | 0.19582 ^{\left\{3 \right\}} | 0.02220 ^{\left\{1 \right\}} | 0.25122 ^{\left\{7 \right\}} | 0.20232 ^{\left\{4 \right\}} | 0.20735 ^{\left\{6 \right\}} | ||
\hat{\theta} | 0.68706 ^{\left\{8 \right\}} | 0.51170 ^{\left\{3 \right\}} | 0.58072 ^{\left\{7 \right\}} | 0.52071 ^{\left\{4 \right\}} | 0.16747 ^{\left\{1 \right\}} | 0.49400 ^{\left\{2 \right\}} | 0.55280 ^{\left\{6 \right\}} | 0.52733 ^{\left\{5 \right\}} | ||
MREs | \hat{\vartheta} | 0.28718 ^{\left\{8 \right\}} | 0.21747 ^{\left\{3 \right\}} | 0.28233 ^{\left\{7 \right\}} | 0.21734 ^{\left\{2 \right\}} | 0.06426 ^{\left\{1 \right\}} | 0.26908 ^{\left\{5 \right\}} | 0.27384 ^{\left\{6 \right\}} | 0.23830 ^{\left\{4 \right\}} | |
\hat{\varphi} | 0.28123 ^{\left\{3 \right\}} | 0.32226 ^{\left\{6 \right\}} | 0.28677 ^{\left\{4 \right\}} | 0.32261 ^{\left\{7 \right\}} | 0.23462 ^{\left\{1 \right\}} | 0.38138 ^{\left\{8 \right\}} | 0.27957 ^{\left\{2 \right\}} | 0.31974 ^{\left\{5 \right\}} | ||
\hat{\lambda} | 0.30323 ^{\left\{8 \right\}} | 0.20744 ^{\left\{2 \right\}} | 0.24597 ^{\left\{6 \right\}} | 0.22000 ^{\left\{3 \right\}} | 0.07538 ^{\left\{1 \right\}} | 0.26075 ^{\left\{7 \right\}} | 0.24062 ^{\left\{5 \right\}} | 0.22937 ^{\left\{4 \right\}} | ||
\hat{\theta} | 0.30681 ^{\left\{8 \right\}} | 0.24825 ^{\left\{3 \right\}} | 0.27454 ^{\left\{7 \right\}} | 0.25039 ^{\left\{4 \right\}} | 0.13226 ^{\left\{1 \right\}} | 0.24153 ^{\left\{2 \right\}} | 0.26425 ^{\left\{6 \right\}} | 0.25252 ^{\left\{5 \right\}} | ||
\sum{Ranks} | {\textbf{80}} ^{\left\{8 \right\}} | {\textbf{41}} ^{\left\{2 \right\}} | {\textbf{70}} ^{\left\{7 \right\}} | {\textbf{49}} ^{\left\{3 \right\}} | {\textbf{12}} ^{\left\{1 \right\}} | {\textbf{69}} ^{\left\{6 \right\}} | {\textbf{55}} ^{\left\{4 \right\}} | {\textbf{56}} ^{\left\{5 \right\}} | ||
200 | |BIAS| | \hat{\vartheta} | 0.98460 ^{\left\{6 \right\}} | 0.86500 ^{\left\{3 \right\}} | 1.00674 ^{\left\{7 \right\}} | 0.86298 ^{\left\{2 \right\}} | 0.12154 ^{\left\{1 \right\}} | 1.05269 ^{\left\{8 \right\}} | 0.98185 ^{\left\{5 \right\}} | 0.89235 ^{\left\{4 \right\}} |
\hat{\varphi} | 0.38859 ^{\left\{2 \right\}} | 0.45911 ^{\left\{5 \right\}} | 0.41129 ^{\left\{4 \right\}} | 0.46722 ^{\left\{6 \right\}} | 0.31253 ^{\left\{1 \right\}} | 0.67732 ^{\left\{8 \right\}} | 0.40329 ^{\left\{3 \right\}} | 0.46920 ^{\left\{7 \right\}} | ||
\hat{\lambda} | 0.39873 ^{\left\{8 \right\}} | 0.31023 ^{\left\{2 \right\}} | 0.35370 ^{\left\{6 \right\}} | 0.32546 ^{\left\{3 \right\}} | 0.06881 ^{\left\{1 \right\}} | 0.37938 ^{\left\{7 \right\}} | 0.34126 ^{\left\{5 \right\}} | 0.32900 ^{\left\{4 \right\}} | ||
\hat{\theta} | 0.68985 ^{\left\{8 \right\}} | 0.57483 ^{\left\{3 \right\}} | 0.64129 ^{\left\{7 \right\}} | 0.59868 ^{\left\{5 \right\}} | 0.21029 ^{\left\{1 \right\}} | 0.55195 ^{\left\{2 \right\}} | 0.61763 ^{\left\{6 \right\}} | 0.59808 ^{\left\{4 \right\}} | ||
MSEs | \hat{\vartheta} | 1.11626 ^{\left\{6 \right\}} | 1.01791 ^{\left\{2 \right\}} | 1.13048 ^{\left\{7 \right\}} | 1.02064 ^{\left\{3 \right\}} | 0.05498 ^{\left\{1 \right\}} | 1.30905 ^{\left\{8 \right\}} | 1.09868 ^{\left\{5 \right\}} | 1.05550 ^{\left\{4 \right\}} | |
\hat{\varphi} | 0.23053 ^{\left\{2 \right\}} | 0.31234 ^{\left\{5 \right\}} | 0.25573 ^{\left\{4 \right\}} | 0.32276 ^{\left\{6 \right\}} | 0.15678 ^{\left\{1 \right\}} | 0.58268 ^{\left\{8 \right\}} | 0.24649 ^{\left\{3 \right\}} | 0.32421 ^{\left\{7 \right\}} | ||
\hat{\lambda} | 0.22103 ^{\left\{7 \right\}} | 0.16583 ^{\left\{2 \right\}} | 0.18036 ^{\left\{5 \right\}} | 0.17985 ^{\left\{4 \right\}} | 0.00837 ^{\left\{1 \right\}} | 0.22479 ^{\left\{8 \right\}} | 0.17270 ^{\left\{3 \right\}} | 0.18259 ^{\left\{6 \right\}} | ||
\hat{\theta} | 0.58585 ^{\left\{8 \right\}} | 0.45832 ^{\left\{3 \right\}} | 0.51809 ^{\left\{7 \right\}} | 0.48976 ^{\left\{5 \right\}} | 0.07171 ^{\left\{1 \right\}} | 0.44322 ^{\left\{2 \right\}} | 0.49209 ^{\left\{6 \right\}} | 0.48968 ^{\left\{4 \right\}} | ||
MREs | \hat{\vartheta} | 0.28132 ^{\left\{6 \right\}} | 0.24714 ^{\left\{3 \right\}} | 0.28764 ^{\left\{7 \right\}} | 0.24657 ^{\left\{2 \right\}} | 0.03472 ^{\left\{1 \right\}} | 0.30077 ^{\left\{8 \right\}} | 0.28053 ^{\left\{5 \right\}} | 0.25496 ^{\left\{4 \right\}} | |
\hat{\varphi} | 0.19429 ^{\left\{2 \right\}} | 0.22956 ^{\left\{5 \right\}} | 0.20564 ^{\left\{4 \right\}} | 0.23361 ^{\left\{6 \right\}} | 0.15627 ^{\left\{1 \right\}} | 0.33866 ^{\left\{8 \right\}} | 0.20165 ^{\left\{3 \right\}} | 0.23460 ^{\left\{7 \right\}} | ||
\hat{\lambda} | 0.26582 ^{\left\{8 \right\}} | 0.20682 ^{\left\{2 \right\}} | 0.23580 ^{\left\{6 \right\}} | 0.21697 ^{\left\{3 \right\}} | 0.04587 ^{\left\{1 \right\}} | 0.25292 ^{\left\{7 \right\}} | 0.22751 ^{\left\{5 \right\}} | 0.21933 ^{\left\{4 \right\}} | ||
\hat{\theta} | 0.27594 ^{\left\{8 \right\}} | 0.22993 ^{\left\{3 \right\}} | 0.25652 ^{\left\{7 \right\}} | 0.23947 ^{\left\{5 \right\}} | 0.08412 ^{\left\{1 \right\}} | 0.22078 ^{\left\{2 \right\}} | 0.24705 ^{\left\{6 \right\}} | 0.23923 ^{\left\{4 \right\}} | ||
\sum{Ranks} | {\textbf{71}} ^{\left\{6.5 \right\}} | {\textbf{38}} ^{\left\{2 \right\}} | {\textbf{71}} ^{\left\{6.5 \right\}} | {\textbf{50}} ^{\left\{3 \right\}} | {\textbf{12}} ^{\left\{1 \right\}} | {\textbf{76}} ^{\left\{8 \right\}} | {\textbf{55}} ^{\left\{4 \right\}} | {\textbf{59}} ^{\left\{5 \right\}} | ||
500 | |BIAS| | \hat{\vartheta} | 0.93304 ^{\left\{5 \right\}} | 0.93263 ^{\left\{4 \right\}} | 0.97539 ^{\left\{7 \right\}} | 0.92961 ^{\left\{3 \right\}} | 0.05348 ^{\left\{1 \right\}} | 1.08240 ^{\left\{8 \right\}} | 0.95522 ^{\left\{6 \right\}} | 0.92527 ^{\left\{2 \right\}} |
\hat{\varphi} | 0.27572 ^{\left\{2 \right\}} | 0.32936 ^{\left\{5 \right\}} | 0.29210 ^{\left\{4 \right\}} | 0.33160 ^{\left\{6 \right\}} | 0.19113 ^{\left\{1 \right\}} | 0.58412 ^{\left\{8 \right\}} | 0.28766 ^{\left\{3 \right\}} | 0.33820 ^{\left\{7 \right\}} | ||
\hat{\lambda} | 0.34882 ^{\left\{7 \right\}} | 0.31976 ^{\left\{2 \right\}} | 0.33838 ^{\left\{6 \right\}} | 0.33117 ^{\left\{5 \right\}} | 0.03908 ^{\left\{1 \right\}} | 0.35124 ^{\left\{8 \right\}} | 0.32736 ^{\left\{3 \right\}} | 0.32969 ^{\left\{4 \right\}} | ||
\hat{\theta} | 0.61892 ^{\left\{8 \right\}} | 0.57580 ^{\left\{3 \right\}} | 0.60923 ^{\left\{7 \right\}} | 0.59341 ^{\left\{6 \right\}} | 0.12272 ^{\left\{1 \right\}} | 0.51040 ^{\left\{2 \right\}} | 0.59246 ^{\left\{5 \right\}} | 0.58416 ^{\left\{4 \right\}} | ||
MSEs | \hat{\vartheta} | 0.99386 ^{\left\{2 \right\}} | 1.04383 ^{\left\{6 \right\}} | 1.04849 ^{\left\{7 \right\}} | 1.04352 ^{\left\{5 \right\}} | 0.00732 ^{\left\{1 \right\}} | 1.28318 ^{\left\{8 \right\}} | 1.01861 ^{\left\{3 \right\}} | 1.03056 ^{\left\{4 \right\}} | |
\hat{\varphi} | 0.11591 ^{\left\{2 \right\}} | 0.16651 ^{\left\{5 \right\}} | 0.13079 ^{\left\{4 \right\}} | 0.16807 ^{\left\{6 \right\}} | 0.06394 ^{\left\{1 \right\}} | 0.45248 ^{\left\{8 \right\}} | 0.12739 ^{\left\{3 \right\}} | 0.17250 ^{\left\{7 \right\}} | ||
\hat{\lambda} | 0.17000 ^{\left\{7 \right\}} | 0.15789 ^{\left\{3 \right\}} | 0.15993 ^{\left\{4 \right\}} | 0.16663 ^{\left\{5 \right\}} | 0.00250 ^{\left\{1 \right\}} | 0.18698 ^{\left\{8 \right\}} | 0.15186 ^{\left\{2 \right\}} | 0.16915 ^{\left\{6 \right\}} | ||
\hat{\theta} | 0.48714 ^{\left\{8 \right\}} | 0.45146 ^{\left\{4 \right\}} | 0.47250 ^{\left\{6 \right\}} | 0.47497 ^{\left\{7 \right\}} | 0.02504 ^{\left\{1 \right\}} | 0.39915 ^{\left\{2 \right\}} | 0.45105 ^{\left\{3 \right\}} | 0.47078 ^{\left\{5 \right\}} | ||
MREs | \hat{\vartheta} | 0.26658 ^{\left\{5 \right\}} | 0.26647 ^{\left\{4 \right\}} | 0.27868 ^{\left\{7 \right\}} | 0.26560 ^{\left\{3 \right\}} | 0.01528 ^{\left\{1 \right\}} | 0.30926 ^{\left\{8 \right\}} | 0.27292 ^{\left\{6 \right\}} | 0.26436 ^{\left\{2 \right\}} | |
\hat{\varphi} | 0.13786 ^{\left\{2 \right\}} | 0.16468 ^{\left\{5 \right\}} | 0.14605 ^{\left\{4 \right\}} | 0.16580 ^{\left\{6 \right\}} | 0.09557 ^{\left\{1 \right\}} | 0.29206 ^{\left\{8 \right\}} | 0.14383 ^{\left\{3 \right\}} | 0.16910 ^{\left\{7 \right\}} | ||
\hat{\lambda} | 0.23255 ^{\left\{7 \right\}} | 0.21318 ^{\left\{2 \right\}} | 0.22559 ^{\left\{6 \right\}} | 0.22078 ^{\left\{5 \right\}} | 0.02606 ^{\left\{1 \right\}} | 0.23416 ^{\left\{8 \right\}} | 0.21824 ^{\left\{3 \right\}} | 0.21980 ^{\left\{4 \right\}} | ||
\hat{\theta} | 0.24757 ^{\left\{8 \right\}} | 0.23032 ^{\left\{3 \right\}} | 0.24369 ^{\left\{7 \right\}} | 0.23736 ^{\left\{6 \right\}} | 0.04909 ^{\left\{1 \right\}} | 0.20416 ^{\left\{2 \right\}} | 0.23698 ^{\left\{5 \right\}} | 0.23367 ^{\left\{4 \right\}} | ||
\sum{Ranks} | {\textbf{63}} ^{\left\{5.5 \right\}} | {\textbf{46}} ^{\left\{3 \right\}} | {\textbf{69}} ^{\left\{7 \right\}} | {\textbf{63}} ^{\left\{5.5 \right\}} | {\textbf{12}} ^{\left\{1 \right\}} | {\textbf{78}} ^{\left\{8 \right\}} | {\textbf{45}} ^{\left\{2 \right\}} | {\textbf{56}} ^{\left\{4 \right\}} |
n | Est. | Est. Par. | MLEs | LSEs | WLSEs | CRVMEs | MPSEs | PCEs | ADEs | RADEs |
20 | |BIAS| | \hat{\vartheta} | 1.25197 ^{\left\{8 \right\}} | 0.45438 ^{\left\{3 \right\}} | 1.06521 ^{\left\{7 \right\}} | 0.44888 ^{\left\{2 \right\}} | 0.20680 ^{\left\{1 \right\}} | 0.83805 ^{\left\{5 \right\}} | 1.02528 ^{\left\{6 \right\}} | 0.62513 ^{\left\{4 \right\}} |
\hat{\varphi} | 0.58123 ^{\left\{5 \right\}} | 0.60203 ^{\left\{7 \right\}} | 0.57995 ^{\left\{4 \right\}} | 0.61278 ^{\left\{8 \right\}} | 0.16575 ^{\left\{1 \right\}} | 0.57749 ^{\left\{3 \right\}} | 0.53508 ^{\left\{2 \right\}} | 0.58165 ^{\left\{6 \right\}} | ||
\hat{\lambda} | 0.08854 ^{\left\{8 \right\}} | 0.07652 ^{\left\{2 \right\}} | 0.08227 ^{\left\{7 \right\}} | 0.07831 ^{\left\{3 \right\}} | 0.06474 ^{\left\{1 \right\}} | 0.08065 ^{\left\{6 \right\}} | 0.07939 ^{\left\{4 \right\}} | 0.07980 ^{\left\{5 \right\}} | ||
\hat{\theta} | 0.66759 ^{\left\{8 \right\}} | 0.42262 ^{\left\{2 \right\}} | 0.53041 ^{\left\{7 \right\}} | 0.44839 ^{\left\{3 \right\}} | 0.32466 ^{\left\{1 \right\}} | 0.51024 ^{\left\{5 \right\}} | 0.52797 ^{\left\{6 \right\}} | 0.48959 ^{\left\{4 \right\}} | ||
MSEs | \hat{\vartheta} | 2.41625 ^{\left\{8 \right\}} | 0.60512 ^{\left\{2 \right\}} | 1.67131 ^{\left\{6 \right\}} | 0.67908 ^{\left\{3 \right\}} | 0.18285 ^{\left\{1 \right\}} | 1.58289 ^{\left\{5 \right\}} | 1.74298 ^{\left\{7 \right\}} | 1.01407 ^{\left\{4 \right\}} | |
\hat{\varphi} | 0.42148 ^{\left\{3 \right\}} | 0.46192 ^{\left\{7 \right\}} | 0.43565 ^{\left\{5 \right\}} | 0.46874 ^{\left\{8 \right\}} | 0.38218 ^{\left\{1 \right\}} | 0.43432 ^{\left\{4 \right\}} | 0.38271 ^{\left\{2 \right\}} | 0.43679 ^{\left\{6 \right\}} | ||
\hat{\lambda} | 0.01072 ^{\left\{8 \right\}} | 0.00870 ^{\left\{2 \right\}} | 0.00963 ^{\left\{7 \right\}} | 0.00906 ^{\left\{3 \right\}} | 0.00620 ^{\left\{1 \right\}} | 0.00950 ^{\left\{6 \right\}} | 0.00923 ^{\left\{5 \right\}} | 0.00919 ^{\left\{4 \right\}} | ||
\hat{\theta} | 0.58894 ^{\left\{8 \right\}} | 0.28693 ^{\left\{2 \right\}} | 0.40885 ^{\left\{6 \right\}} | 0.32249 ^{\left\{3 \right\}} | 0.16767 ^{\left\{1 \right\}} | 0.38646 ^{\left\{5 \right\}} | 0.41599 ^{\left\{7 \right\}} | 0.36863 ^{\left\{4 \right\}} | ||
MREs | \hat{\vartheta} | 0.27821 ^{\left\{8 \right\}} | 0.10097 ^{\left\{3 \right\}} | 0.23671 ^{\left\{7 \right\}} | 0.09975 ^{\left\{2 \right\}} | 0.04596 ^{\left\{1 \right\}} | 0.18623 ^{\left\{5 \right\}} | 0.22784 ^{\left\{6 \right\}} | 0.13892 ^{\left\{4 \right\}} | |
\hat{\varphi} | 0.77497 ^{\left\{5 \right\}} | 0.80271 ^{\left\{7 \right\}} | 0.77327 ^{\left\{4 \right\}} | 0.81704 ^{\left\{8 \right\}} | 0.70903 ^{\left\{1 \right\}} | 0.76998 ^{\left\{3 \right\}} | 0.71344 ^{\left\{2 \right\}} | 0.77554 ^{\left\{6 \right\}} | ||
\hat{\lambda} | 0.17707 ^{\left\{8 \right\}} | 0.15303 ^{\left\{2 \right\}} | 0.16453 ^{\left\{7 \right\}} | 0.15662 ^{\left\{3 \right\}} | 0.12948 ^{\left\{1 \right\}} | 0.16130 ^{\left\{6 \right\}} | 0.15879 ^{\left\{4 \right\}} | 0.15960 ^{\left\{5 \right\}} | ||
\hat{\theta} | 0.53407 ^{\left\{8 \right\}} | 0.33809 ^{\left\{2 \right\}} | 0.42432 ^{\left\{7 \right\}} | 0.35871 ^{\left\{3 \right\}} | 0.25973 ^{\left\{1 \right\}} | 0.40819 ^{\left\{5 \right\}} | 0.42237 ^{\left\{6 \right\}} | 0.39167 ^{\left\{4 \right\}} | ||
\sum{Ranks} | {\textbf{85}} ^{\left\{8 \right\}} | {\textbf{41}} ^{\left\{2 \right\}} | {\textbf{74}} ^{\left\{7 \right\}} | {\textbf{49}} ^{\left\{3 \right\}} | {\textbf{12}} ^{\left\{1 \right\}} | {\textbf{58}} ^{\left\{6 \right\}} | {\textbf{57}} ^{\left\{5 \right\}} | {\textbf{56}} ^{\left\{4 \right\}} | ||
80 | |BIAS| | \hat{\vartheta} | 1.62748 ^{\left\{8 \right\}} | 0.91367 ^{\left\{2 \right\}} | 1.37823 ^{\left\{7 \right\}} | 0.98139 ^{\left\{3 \right\}} | 0.11224 ^{\left\{1 \right\}} | 1.13720 ^{\left\{5 \right\}} | 1.31803 ^{\left\{6 \right\}} | 1.11805 ^{\left\{4 \right\}} |
\hat{\varphi} | 0.31837 ^{\left\{3 \right\}} | 0.39968 ^{\left\{7 \right\}} | 0.34474 ^{\left\{4 \right\}} | 0.40380 ^{\left\{8 \right\}} | 0.27352 ^{\left\{1 \right\}} | 0.37466 ^{\left\{6 \right\}} | 0.31821 ^{\left\{2 \right\}} | 0.35079 ^{\left\{5 \right\}} | ||
\hat{\lambda} | 0.07169 ^{\left\{8 \right\}} | 0.05804 ^{\left\{3 \right\}} | 0.06253 ^{\left\{7 \right\}} | 0.06113 ^{\left\{6 \right\}} | 0.03286 ^{\left\{1 \right\}} | 0.05699 ^{\left\{2 \right\}} | 0.05980 ^{\left\{5 \right\}} | 0.05870 ^{\left\{4 \right\}} | ||
\hat{\theta} | 0.64853 ^{\left\{8 \right\}} | 0.39956 ^{\left\{2 \right\}} | 0.49396 ^{\left\{7 \right\}} | 0.44278 ^{\left\{4 \right\}} | 0.16292 ^{\left\{1 \right\}} | 0.43570 ^{\left\{3 \right\}} | 0.47512 ^{\left\{6 \right\}} | 0.45276 ^{\left\{5 \right\}} | ||
MSEs | \hat{\vartheta} | 3.14585 ^{\left\{8 \right\}} | 1.58782 ^{\left\{2 \right\}} | 2.33956 ^{\left\{7 \right\}} | 1.79431 ^{\left\{3 \right\}} | 0.08679 ^{\left\{1 \right\}} | 1.96177 ^{\left\{4 \right\}} | 2.22845 ^{\left\{6 \right\}} | 2.05141 ^{\left\{5 \right\}} | |
\hat{\varphi} | 0.15254 ^{\left\{2 \right\}} | 0.23761 ^{\left\{7 \right\}} | 0.18156 ^{\left\{4 \right\}} | 0.24031 ^{\left\{8 \right\}} | 0.12335 ^{\left\{1 \right\}} | 0.22013 ^{\left\{6 \right\}} | 0.15798 ^{\left\{3 \right\}} | 0.19064 ^{\left\{5 \right\}} | ||
\hat{\lambda} | 0.00707 ^{\left\{8 \right\}} | 0.00520 ^{\left\{4 \right\}} | 0.00579 ^{\left\{7 \right\}} | 0.00566 ^{\left\{6 \right\}} | 0.00171 ^{\left\{1 \right\}} | 0.00514 ^{\left\{2 \right\}} | 0.00541 ^{\left\{5 \right\}} | 0.00519 ^{\left\{3 \right\}} | ||
\hat{\theta} | 0.56658 ^{\left\{8 \right\}} | 0.28713 ^{\left\{2 \right\}} | 0.38674 ^{\left\{7 \right\}} | 0.34041 ^{\left\{4 \right\}} | 0.04197 ^{\left\{1 \right\}} | 0.32550 ^{\left\{3 \right\}} | 0.36722 ^{\left\{6 \right\}} | 0.35156 ^{\left\{5 \right\}} | ||
MREs | \hat{\vartheta} | 0.36166 ^{\left\{8 \right\}} | 0.20304 ^{\left\{2 \right\}} | 0.30627 ^{\left\{7 \right\}} | 0.21809 ^{\left\{3 \right\}} | 0.02494 ^{\left\{1 \right\}} | 0.25271 ^{\left\{5 \right\}} | 0.29289 ^{\left\{6 \right\}} | 0.24846 ^{\left\{4 \right\}} | |
\hat{\varphi} | 0.42449 ^{\left\{3 \right\}} | 0.53291 ^{\left\{7 \right\}} | 0.45966 ^{\left\{4 \right\}} | 0.53840 ^{\left\{8 \right\}} | 0.36469 ^{\left\{1 \right\}} | 0.49955 ^{\left\{6 \right\}} | 0.42429 ^{\left\{2 \right\}} | 0.46771 ^{\left\{5 \right\}} | ||
\hat{\lambda} | 0.14337 ^{\left\{8 \right\}} | 0.11609 ^{\left\{3 \right\}} | 0.12506 ^{\left\{7 \right\}} | 0.12226 ^{\left\{6 \right\}} | 0.06572 ^{\left\{1 \right\}} | 0.11399 ^{\left\{2 \right\}} | 0.11961 ^{\left\{5 \right\}} | 0.11740 ^{\left\{4 \right\}} | ||
\hat{\theta} | 0.51882 ^{\left\{8 \right\}} | 0.31965 ^{\left\{2 \right\}} | 0.39517 ^{\left\{7 \right\}} | 0.35422 ^{\left\{4 \right\}} | 0.13034 ^{\left\{1 \right\}} | 0.34856 ^{\left\{3 \right\}} | 0.38010 ^{\left\{6 \right\}} | 0.36221 ^{\left\{5 \right\}} | ||
\sum{Ranks} | {\textbf{80}} ^{\left\{8 \right\}} | {\textbf{43}} ^{\left\{2 \right\}} | {\textbf{75}} ^{\left\{7 \right\}} | {\textbf{63}} ^{\left\{6 \right\}} | {\textbf{12}} ^{\left\{1 \right\}} | {\textbf{47}} ^{\left\{3 \right\}} | {\textbf{58}} ^{\left\{5 \right\}} | {\textbf{54}} ^{\left\{4 \right\}} | ||
200 | |BIAS| | \hat{\vartheta} | 1.48139 ^{\left\{8 \right\}} | 1.17284 ^{\left\{2 \right\}} | 1.40391 ^{\left\{7 \right\}} | 1.21294 ^{\left\{4 \right\}} | 0.04307 ^{\left\{1 \right\}} | 1.17950 ^{\left\{3 \right\}} | 1.33404 ^{\left\{6 \right\}} | 1.29174 ^{\left\{5 \right\}} |
\hat{\varphi} | 0.20186 ^{\left\{2 \right\}} | 0.26830 ^{\left\{7 \right\}} | 0.21385 ^{\left\{4 \right\}} | 0.26575 ^{\left\{6 \right\}} | 0.16575 ^{\left\{1 \right\}} | 0.27408 ^{\left\{8 \right\}} | 0.20558 ^{\left\{3 \right\}} | 0.23166 ^{\left\{5 \right\}} | ||
\hat{\lambda} | 0.06056 ^{\left\{8 \right\}} | 0.05260 ^{\left\{3 \right\}} | 0.05573 ^{\left\{7 \right\}} | 0.05571 ^{\left\{6 \right\}} | 0.02038 ^{\left\{1 \right\}} | 0.04542 ^{\left\{2 \right\}} | 0.05309 ^{\left\{4 \right\}} | 0.05470 ^{\left\{5 \right\}} | ||
\hat{\theta} | 0.54563 ^{\left\{8 \right\}} | 0.42847 ^{\left\{3 \right\}} | 0.47838 ^{\left\{7 \right\}} | 0.45810 ^{\left\{5 \right\}} | 0.09746 ^{\left\{1 \right\}} | 0.36816 ^{\left\{2 \right\}} | 0.44966 ^{\left\{4 \right\}} | 0.46232 ^{\left\{6 \right\}} | ||
MSEs | \hat{\vartheta} | 2.63270 ^{\left\{8 \right\}} | 2.08923 ^{\left\{3 \right\}} | 2.37558 ^{\left\{7 \right\}} | 2.20468 ^{\left\{5 \right\}} | 0.02535 ^{\left\{1 \right\}} | 1.86391 ^{\left\{2 \right\}} | 2.18628 ^{\left\{4 \right\}} | 2.32684 ^{\left\{6 \right\}} | |
\hat{\varphi} | 0.06343 ^{\left\{2 \right\}} | 0.11259 ^{\left\{7 \right\}} | 0.07126 ^{\left\{4 \right\}} | 0.11064 ^{\left\{6 \right\}} | 0.04499 ^{\left\{1 \right\}} | 0.12626 ^{\left\{8 \right\}} | 0.06649 ^{\left\{3 \right\}} | 0.08397 ^{\left\{5 \right\}} | ||
\hat{\lambda} | 0.00531 ^{\left\{8 \right\}} | 0.00437 ^{\left\{3 \right\}} | 0.00472 ^{\left\{6 \right\}} | 0.00486 ^{\left\{7 \right\}} | 0.00066 ^{\left\{1 \right\}} | 0.00347 ^{\left\{2 \right\}} | 0.00441 ^{\left\{4 \right\}} | 0.00470 ^{\left\{5 \right\}} | ||
\hat{\theta} | 0.44122 ^{\left\{8 \right\}} | 0.33416 ^{\left\{3 \right\}} | 0.37078 ^{\left\{5 \right\}} | 0.37139 ^{\left\{6 \right\}} | 0.01519 ^{\left\{1 \right\}} | 0.25838 ^{\left\{2 \right\}} | 0.33754 ^{\left\{4 \right\}} | 0.37704 ^{\left\{7 \right\}} | ||
MREs | \hat{\vartheta} | 0.32920 ^{\left\{8 \right\}} | 0.26063 ^{\left\{2 \right\}} | 0.31198 ^{\left\{7 \right\}} | 0.26954 ^{\left\{4 \right\}} | 0.00957 ^{\left\{1 \right\}} | 0.26211 ^{\left\{3 \right\}} | 0.29645 ^{\left\{6 \right\}} | 0.28705 ^{\left\{5 \right\}} | |
\hat{\varphi} | 0.26915 ^{\left\{2 \right\}} | 0.35774 ^{\left\{7 \right\}} | 0.28513 ^{\left\{4 \right\}} | 0.35434 ^{\left\{6 \right\}} | 0.22100 ^{\left\{1 \right\}} | 0.36544 ^{\left\{8 \right\}} | 0.27411 ^{\left\{3 \right\}} | 0.30888 ^{\left\{5 \right\}} | ||
\hat{\lambda} | 0.12113 ^{\left\{8 \right\}} | 0.10520 ^{\left\{3 \right\}} | 0.11146 ^{\left\{7 \right\}} | 0.11142 ^{\left\{6 \right\}} | 0.04075 ^{\left\{1 \right\}} | 0.09084 ^{\left\{2 \right\}} | 0.10618 ^{\left\{4 \right\}} | 0.10941 ^{\left\{5 \right\}} | ||
\hat{\theta} | 0.43650 ^{\left\{8 \right\}} | 0.34278 ^{\left\{3 \right\}} | 0.38270 ^{\left\{7 \right\}} | 0.36648 ^{\left\{5 \right\}} | 0.07797 ^{\left\{1 \right\}} | 0.29453 ^{\left\{2 \right\}} | 0.35973 ^{\left\{4 \right\}} | 0.36986 ^{\left\{6 \right\}} | ||
\sum{Ranks} | {\textbf{78}} ^{\left\{8 \right\}} | {\textbf{46}} ^{\left\{3 \right\}} | {\textbf{72}} ^{\left\{7 \right\}} | {\textbf{66}} ^{\left\{6 \right\}} | {\textbf{12}} ^{\left\{1 \right\}} | {\textbf{44}} ^{\left\{2 \right\}} | {\textbf{49}} ^{\left\{4 \right\}} | {\textbf{65}} ^{\left\{5 \right\}} | ||
500 | |BIAS| | \hat{\vartheta} | 1.29672 ^{\left\{5 \right\}} | 1.27235 ^{\left\{3 \right\}} | 1.31237 ^{\left\{6 \right\}} | 1.32513 ^{\left\{7 \right\}} | 0.01519 ^{\left\{1 \right\}} | 1.15120 ^{\left\{2 \right\}} | 1.28994 ^{\left\{4 \right\}} | 1.34189 ^{\left\{8 \right\}} |
\hat{\varphi} | 0.13744 ^{\left\{2 \right\}} | 0.17802 ^{\left\{6 \right\}} | 0.14815 ^{\left\{4 \right\}} | 0.18664 ^{\left\{7 \right\}} | 0.10227 ^{\left\{1 \right\}} | 0.19392 ^{\left\{8 \right\}} | 0.14640 ^{\left\{3 \right\}} | 0.15865 ^{\left\{5 \right\}} | ||
\hat{\lambda} | 0.04970 ^{\left\{5 \right\}} | 0.05107 ^{\left\{6 \right\}} | 0.04913 ^{\left\{4 \right\}} | 0.05400 ^{\left\{8 \right\}} | 0.01264 ^{\left\{1 \right\}} | 0.03665 ^{\left\{2 \right\}} | 0.04825 ^{\left\{3 \right\}} | 0.05335 ^{\left\{7 \right\}} | ||
\hat{\theta} | 0.44102 ^{\left\{5 \right\}} | 0.44509 ^{\left\{6 \right\}} | 0.43050 ^{\left\{4 \right\}} | 0.47518 ^{\left\{8 \right\}} | 0.05962 ^{\left\{1 \right\}} | 0.31796 ^{\left\{2 \right\}} | 0.42160 ^{\left\{3 \right\}} | 0.46945 ^{\left\{7 \right\}} | ||
MSEs | \hat{\vartheta} | 2.07266 ^{\left\{4 \right\}} | 2.19148 ^{\left\{6 \right\}} | 2.07839 ^{\left\{5 \right\}} | 2.34226 ^{\left\{8 \right\}} | 0.00344 ^{\left\{1 \right\}} | 1.67247 ^{\left\{2 \right\}} | 2.02330 ^{\left\{3 \right\}} | 2.31957 ^{\left\{7 \right\}} | |
\hat{\varphi} | 0.02955 ^{\left\{2 \right\}} | 0.05030 ^{\left\{6 \right\}} | 0.03417 ^{\left\{4 \right\}} | 0.05288 ^{\left\{7 \right\}} | 0.01679 ^{\left\{1 \right\}} | 0.06276 ^{\left\{8 \right\}} | 0.03295 ^{\left\{3 \right\}} | 0.03874 ^{\left\{5 \right\}} | ||
\hat{\lambda} | 0.00377 ^{\left\{4 \right\}} | 0.00418 ^{\left\{6 \right\}} | 0.00378 ^{\left\{5 \right\}} | 0.00452 ^{\left\{7 \right\}} | 0.00025 ^{\left\{1 \right\}} | 0.00242 ^{\left\{2 \right\}} | 0.00363 ^{\left\{3 \right\}} | 0.00454 ^{\left\{8 \right\}} | ||
\hat{\theta} | 0.30987 ^{\left\{5 \right\}} | 0.34423 ^{\left\{6 \right\}} | 0.30505 ^{\left\{4 \right\}} | 0.37795 ^{\left\{8 \right\}} | 0.00564 ^{\left\{1 \right\}} | 0.19875 ^{\left\{2 \right\}} | 0.29242 ^{\left\{3 \right\}} | 0.37470 ^{\left\{7 \right\}} | ||
MREs | \hat{\vartheta} | 0.28816 ^{\left\{5 \right\}} | 0.28275 ^{\left\{3 \right\}} | 0.29164 ^{\left\{6 \right\}} | 0.29447 ^{\left\{7 \right\}} | 0.00337 ^{\left\{1 \right\}} | 0.25582 ^{\left\{2 \right\}} | 0.28665 ^{\left\{4 \right\}} | 0.29820 ^{\left\{8 \right\}} | |
\hat{\varphi} | 0.18326 ^{\left\{2 \right\}} | 0.23736 ^{\left\{6 \right\}} | 0.19754 ^{\left\{4 \right\}} | 0.24885 ^{\left\{7 \right\}} | 0.13636 ^{\left\{1 \right\}} | 0.25856 ^{\left\{8 \right\}} | 0.19520 ^{\left\{3 \right\}} | 0.21153 ^{\left\{5 \right\}} | ||
\hat{\lambda} | 0.09940 ^{\left\{5 \right\}} | 0.10214 ^{\left\{6 \right\}} | 0.09825 ^{\left\{4 \right\}} | 0.10801 ^{\left\{8 \right\}} | 0.02528 ^{\left\{1 \right\}} | 0.07330 ^{\left\{2 \right\}} | 0.09651 ^{\left\{3 \right\}} | 0.10671 ^{\left\{7 \right\}} | ||
\hat{\theta} | 0.35281 ^{\left\{5 \right\}} | 0.35607 ^{\left\{6 \right\}} | 0.34440 ^{\left\{4 \right\}} | 0.38014 ^{\left\{8 \right\}} | 0.04770 ^{\left\{1 \right\}} | 0.25437 ^{\left\{2 \right\}} | 0.33728 ^{\left\{3 \right\}} | 0.37556 ^{\left\{7 \right\}} | ||
\sum{Ranks} | {\textbf{49}} ^{\left\{4 \right\}} | {\textbf{66}} ^{\left\{6 \right\}} | {\textbf{54}} ^{\left\{5 \right\}} | {\textbf{90}} ^{\left\{8 \right\}} | {\textbf{12}} ^{\left\{1 \right\}} | {\textbf{42}} ^{\left\{3 \right\}} | {\textbf{38}} ^{\left\{2 \right\}} | {\textbf{81}} ^{\left\{7 \right\}} |
\mathit{\boldsymbol{\eta}}^{\intercal} | n | MLEs | LSEs | WLSEs | CRVMEs | MPSEs | PCEs | ADEs | RADEs |
(\hat{\vartheta}=1.75, \hat{\varphi}=0.5, \hat{\lambda}=0.25, \hat{\theta}=1.25) | 20 | 2 | 7 | 1 | 5 | 4 | 8 | 3 | 6 |
80 | 3 | 7 | 2 | 6 | 1 | 5 | 4 | 8 | |
200 | 1.5 | 7 | 4 | 5 | 1.5 | 6 | 3 | 8 | |
500 | 2 | 5.5 | 4 | 5.5 | 1 | 7 | 3 | 8 | |
(\hat{\vartheta}=1.75, \hat{\varphi}=2, \hat{\lambda}=1.5, \hat{\theta}=2.5) | 20 | 1 | 3 | 2 | 7 | 4 | 8 | 5.5 | 5.5 |
80 | 1 | 3 | 4 | 6 | 2 | 8 | 5 | 7 | |
200 | 2 | 3 | 4.5 | 6.5 | 1 | 8 | 4.5 | 6.5 | |
500 | 3 | 4 | 5 | 6 | 1 | 8 | 2 | 7 | |
(\hat{\vartheta}=3.5, \hat{\varphi}=0.75, \hat{\lambda}=0.5, \hat{\theta}=1.25) | 20 | 7 | 4 | 3 | 6 | 1 | 8 | 2 | 5 |
80 | 8 | 3 | 4 | 7 | 1 | 5 | 2 | 6 | |
200 | 6 | 5 | 4 | 8 | 1 | 3 | 2 | 7 | |
500 | 2 | 6 | 5 | 7 | 1 | 4 | 3 | 8 | |
(\hat{\vartheta}=3.5, \hat{\varphi}=2, \hat{\lambda}=1.5, \hat{\theta}=2.5) | 20 | 8 | 2 | 6 | 3 | 1 | 7 | 5 | 4 |
80 | 8 | 2 | 7 | 3 | 1 | 6 | 4 | 5 | |
200 | 6.5 | 2 | 6.5 | 3 | 1 | 8 | 4 | 5 | |
500 | 5.5 | 3 | 7 | 5.5 | 1 | 8 | 2 | 4 | |
(\hat{\vartheta}=4.5, \hat{\varphi}=0.75, \hat{\lambda}=0.5, \hat{\theta}=1.25) | 20 | 8 | 2 | 7 | 3 | 1 | 6 | 5 | 4 |
80 | 8 | 2 | 7 | 6 | 1 | 3 | 5 | 4 | |
200 | 8 | 3 | 7 | 6 | 1 | 2 | 4 | 5 | |
500 | 4 | 6 | 5 | 8 | 1 | 3 | 2 | 7 | |
(\hat{\vartheta}=4.5, \hat{\varphi}=0.75, \hat{\lambda}=0.5, \hat{\theta}=1.25) | 20 | 8 | 2 | 5 | 3 | 1 | 7 | 6 | 4 |
80 | 6 | 2 | 4 | 3 | 1 | 8 | 7 | 5 | |
200 | 6 | 2 | 4 | 3 | 1 | 7 | 8 | 5 | |
500 | 6 | 2 | 5 | 3 | 1 | 8 | 7 | 4 | |
(\hat{\vartheta}=0.25, \hat{\varphi}=3.5, \hat{\lambda}=3, \hat{\theta}=0.25) | 20 | 1 | 6 | 8 | 2 | 7 | 5 | 4 | 3 |
80 | 1.5 | 5 | 8 | 1.5 | 6 | 4 | 3 | 7 | |
200 | 1 | 7 | 4 | 6 | 2 | 3 | 5 | 8 | |
500 | 2 | 8 | 6 | 7 | 1 | 3 | 4.5 | 4.5 | |
(\hat{\vartheta}=0.75, \hat{\varphi}=2.5, \hat{\lambda}=4.25, \hat{\theta}=0.25) | 20 | 2 | 8 | 6 | 5 | 3 | 1 | 4 | 7 |
80 | 1.5 | 7 | 5 | 6 | 1.5 | 3 | 4 | 8 | |
200 | 2 | 7 | 5 | 6 | 1 | 3 | 4 | 8 | |
500 | 2 | 8 | 5 | 6 | 1 | 3 | 4 | 7 | |
\sum Ranks | \textbf{133.5} | \textbf{143.5} | \textbf{160} | \textbf{164} | \textbf{54} | \textbf{176} | \textbf{130.5} | \textbf{190.5} | |
{\textbf{Overall Rank}} | \textbf{3} | \textbf{4} | \textbf{5} | \textbf{6} | \textbf{1} | \textbf{7} | \textbf{2} | \textbf{8} |
Model | Par.. | Estimates | (SEs) | AIC | CAIC | BIC | HQIC | W^{*} | A^{*} | KS | (PV) |
EWFr | \hat{\vartheta} | 56.93662 | (38.42845) | 827.52150 | 827.84670 | 838.92960 | 832.15660 | 0.01991 | 0.13402 | 0.03757 | 0.99713 |
\hat{\varphi} | 0.46697 | (0.22382) | |||||||||
\hat{\lambda} | 0.71825 | (0.01713) | |||||||||
\hat{\theta} | 0.01794 | (0.01207) | |||||||||
OLxFr | \hat{\vartheta} | 2.18260 | (1.04763) | 827.70270 | 828.02790 | 839.11080 | 832.33780 | 0.02380 | 0.16520 | 0.04060 | 0.98433 |
\hat{\varphi} | 625.38000 | (622.71234) | |||||||||
\hat{a} | 0.12210 | (0.08023) | |||||||||
\hat{b} | 1.38560 | (0.17273) | |||||||||
KMOFr | \hat{\vartheta} | 43.87750 | (128.52731) | 831.05330 | 831.54510 | 845.31340 | 836.84730 | 0.26278 | 0.34950 | 0.04457 | 0.96120 |
\hat{\varphi} | 0.52028 | (0.41388) | |||||||||
\hat{\delta} | 0.01314 | (0.00808) | |||||||||
\hat{a} | 3.27359 | (2.90929) | |||||||||
\hat{b} | 19.93782 | (47.58906) | |||||||||
EFr | \hat{a} | 1426.40000 | (1440.19245) | 830.85880 | 831.05240 | 839.41490 | 834.33520 | 0.06720 | 0.46640 | 0.04910 | 0.91754 |
\hat{b} | 0.26240 | (0.02850) | |||||||||
\hat{\theta} | 46.24800 | (22.87321) | |||||||||
BFr | \hat{\vartheta} | 0.60970 | (0.32261) | 833.09830 | 833.42350 | 844.50640 | 837.73350 | 0.06900 | 0.47650 | 0.05540 | 0.82683 |
\hat{\varphi} | 36.60200 | (19.48341) | |||||||||
\hat{a} | 739.38700 | (629.24021) | |||||||||
\hat{b} | 0.32240 | (0.06044) | |||||||||
GExFr | \hat{\vartheta} | 226.67000 | (267.96324) | 840.21630 | 840.54150 | 851.62450 | 844.85150 | 0.14320 | 0.95970 | 0.06640 | 0.62593 |
\hat{\varphi} | 91.93900 | (133.94324) | |||||||||
\hat{a} | 22.27100 | (119.19139) | |||||||||
\hat{b} | 0.07090 | (0.04132) | |||||||||
TEFr | \hat{\vartheta} | 3.25820 | (0.40714) | 892.00150 | 892.09750 | 897.70560 | 894.31910 | 0.74430 | 4.54640 | 0.14080 | 0.01254 |
\hat{\varphi} | 0.75210 | (0.04224) | |||||||||
\hat{a} | 39.38721 | (40.24321) | |||||||||
\hat{b} | 0.25243 | (0.76041) | |||||||||
Fr | \hat{\theta} | 0.75208 | (0.04242) | 892.00150 | 892.09750 | 897.70560 | 894.31910 | 0.74432 | 4.54642 | 0.14079 | 0.01250 |
\hat{\lambda} | 2.43109 | (0.21928) |
Model | Par.. | Estimates | (SEs) | AIC | CAIC | BIC | HQIC | W^{*} | A^{*} | KS | (PV) |
EWFr | \hat{\vartheta} | 0.76563 | (0.3765631) | 119.68850 | 120.37820 | 128.26110 | 123.06020 | 0.04181 | 0.23988 | 0.06847 | 0.92920 |
\hat{\varphi} | 0.04349 | (0.3074545) | |||||||||
\hat{\lambda} | 145.67068 | (208.851634) | |||||||||
\hat{\theta} | 4.60846 | (1.2033681) | |||||||||
OLxFr | \hat{\vartheta} | 5.14301 | (9.9790719) | 119.94170 | 120.63140 | 128.51430 | 123.31340 | 0.04741 | 0.26530 | 0.07631 | 0.85664 |
\hat{\varphi} | 5.82568 | (7.6204045) | |||||||||
\hat{a} | 4.17374 | (2.671917) | |||||||||
\hat{b} | 2.82338 | (0.9308868) | |||||||||
KMOFr | \hat{\vartheta} | 31946.70000 | (837.7074) | 121.90480 | 122.95750 | 132.62050 | 126.11940 | 0.04616 | 0.26108 | 0.07450 | 0.87551 |
\hat{\varphi} | 3.40405 | (1.493079) | |||||||||
\hat{\delta} | 3.35291 | (0.9682264) | |||||||||
\hat{a} | 0.85381 | (0.1934628) | |||||||||
\hat{b} | 13724.50000 | (32837.29) | |||||||||
EFr | \hat{a} | 2.58672 | (2.2847576) | 122.19730 | 122.88700 | 130.76990 | 125.56900 | 0.07082 | 0.40707 | 0.08894 | 0.70136 |
\hat{b} | 0.50304 | (0.4780356) | |||||||||
\hat{\theta} | 6.25000 | (10.0300775) | |||||||||
BFr | \hat{\vartheta} | 2.38748 | (5.65075) | 120.58420 | 121.27380 | 129.15670 | 123.95580 | 0.06008 | 0.32203 | 0.07953 | 0.82045 |
\hat{\varphi} | 1.00168 | (5.322252) | |||||||||
\hat{a} | 22.38231 | (162.860747) | |||||||||
\hat{b} | 27.37096 | (295.790324) | |||||||||
GExFr | \hat{\vartheta} | 2.41716 | (8.509608) | 120.57980 | 121.26950 | 129.15240 | 123.95150 | 0.06064 | 0.32329 | 0.07962 | 0.81934 |
\hat{\varphi} | 1.20859 | (2.106142) | |||||||||
\hat{a} | 24.77784 | (82.649275) | |||||||||
\hat{b} | 15.60163 | (85.339807) | |||||||||
TEFr | \hat{\vartheta} | 4.04638 | (1.0119058) | 121.04420 | 121.73390 | 129.61670 | 124.41580 | 0.06547 | 0.34378 | 0.07500 | 0.87047 |
\hat{\varphi} | 2.50000 | (0.5973595) | |||||||||
\hat{a} | 5.25000 | (2.6300897) | |||||||||
\hat{b} | 0.09255 | (1.2750272) | |||||||||
Fr | \hat{\theta} | 5.43392 | (0.50788) | 121.80430 | 122.00430 | 126.09060 | 123.49010 | 0.11497 | 0.64202 | 0.10013 | 0.55274 |
\hat{\lambda} | 230.48644 | (110.91778) |
Methods | \hat{\vartheta} | \hat{\varphi} | \hat{\lambda} | \hat{\theta} | W^{*} | A^{*} | KS | PV |
MLEs | 56.93661 | 0.46697 | 0.71825 | 0.01794 | 0.01991 | 0.13402 | 0.03757 | 0.99713 |
LSEs | 56.93660 | 0.55502 | 0.71932 | 0.01871 | 0.01850 | 0.12986 | 0.03334 | 0.99918 |
MPSEs | 56.93660 | 0.46698 | 0.71750 | 0.01717 | 0.01916 | 0.13069 | 0.03617 | 0.99708 |
WLSEs | 56.94615 | 0.51962 | 0.71884 | 0.01825 | 0.01815 | 0.12600 | 0.03251 | 0.99947 |
CRVMEs | 56.93659 | 0.55064 | 0.71962 | 0.01891 | 0.01795 | 0.12584 | 0.03352 | 0.99910 |
ADEs | 56.93668 | 0.47772 | 0.71887 | 0.01815 | 0.01763 | 0.12016 | 0.03167 | 0.99968 |
PCEs | 57.47389 | 0.81500 | 0.72219 | 0.02155 | 0.03320 | 0.23254 | 0.03830 | 0.99367 |
RADEs | 56.93805 | 0.56319 | 0.71967 | 0.01899 | 0.01830 | 0.12878 | 0.03385 | 0.99895 |
\textbf{Methods} | \hat{\vartheta} | \hat{\varphi} | \hat{\lambda} | \hat{\theta} | W^{*} | A^{*} | \textbf{KS} | \textbf{PV} |
\textbf{MLEs} | 0.76563 | 0.04349 | 145.67068 | 4.60846 | 0.04181 | 0.23988 | 0.06847 | 0.92920 |
\textbf{LSEs} | 0.69421 | 0.08110 | 145.67010 | 4.56661 | 0.04850 | 0.26973 | 0.06486 | 0.94154 |
\textbf{MPSEs} | 0.75534 | 0.13947 | 145.66920 | 4.65631 | 0.05531 | 0.29551 | 0.07780 | 0.81897 |
\textbf{WLSEs} | 0.71596 | 0.01530 | 145.66740 | 4.57637 | 0.04747 | 0.26675 | 0.06895 | 0.90999 |
\textbf{CRVMEs} | 0.71398 | 0.00100 | 145.67030 | 4.57259 | 0.04761 | 0.26713 | 0.06733 | 0.92336 |
\textbf{ADEs} | 0.72963 | 0.00010 | 145.67980 | 4.57988 | 0.04696 | 0.26562 | 0.07047 | 0.89642 |
\textbf{PCEs} | 0.75075 | 0.02596 | 145.67020 | 4.59649 | 0.04775 | 0.26737 | 0.07311 | 0.87060 |
\textbf{RADEs} | 0.74062 | 0.00010 | 145.66970 | 4.57722 | 0.04668 | 0.26516 | 0.07058 | 0.89538 |
\mathit{\boldsymbol{\eta}}^{\intercal} | \mu | \sigma^{2} | \xi_{1}(X) | \xi_{2}(X) |
(\vartheta=1.50, \; \varphi=0.50, \; \theta=1.50) | 1.3511 | 0.4752 | 3.1421 | 56.2021 |
(\vartheta=2.50, \; \varphi=0.75, \; \theta=1.75) | 1.2701 | 0.1329 | 1.9395 | 16.1410 |
(\vartheta=2.25, \; \varphi=1.00, \; \theta=2.75) | 1.1904 | 0.0780 | 2.2648 | 19.2907 |
(\vartheta=2.25, \; \varphi=0.25, \; \theta=0.75) | 1.6154 | 0.7600 | 1.6132 | 9.0569 |
(\vartheta=2.25, \; \varphi=1.25, \; \theta=3.25) | 1.1831 | 0.0747 | 2.8068 | 27.8064 |
(\vartheta=5.50, \; \varphi=1.50, \; \theta=2.50) | 1.1927 | 0.0223 | 1.7507 | 11.7806 |
n | Est. | Est. Par. | MLEs | LSEs | WLSEs | CRVMEs | MPSEs | PCEs | ADEs | RADEs |
20 | |BIAS| | \hat{\vartheta} | 0.58150 ^{\{3\}} | 0.59477 ^{\{4\}} | 0.53422 ^{\{1\}} | 0.54541 ^{\{2\}} | 0.60555 ^{\{5\}} | 0.71685 ^{\{8\}} | 0.67445 ^{\{7\}} | 0.61466 ^{\{6\}} |
\hat{\varphi} | 0.47550 ^{\{4\}} | 0.54812 ^{\{7\}} | 0.46820 ^{\{2\}} | 0.53093 ^{\{6\}} | 0.19120 ^{\{1\}} | 0.55104 ^{\{8\}} | 0.46906 ^{\{3\}} | 0.51894 ^{\{5\}} | ||
\hat{\lambda} | 0.08930 ^{\{2\}} | 0.10351 ^{\{7\}} | 0.08402 ^{\{1\}} | 0.10057 ^{\{5\}} | 0.10077 ^{\{6\}} | 0.11204 ^{\{8\}} | 0.09758 ^{\{3\}} | 0.09866 ^{\{4\}} | ||
\hat{\theta} | 0.49749 ^{\{7\}} | 0.47497 ^{\{4\}} | 0.46884 ^{\{3\}} | 0.48364 ^{\{5\}} | 0.45238 ^{\{1\}} | 0.53285 ^{\{8\}} | 0.46505 ^{\{2\}} | 0.48608 ^{\{6\}} | ||
MSEs | \hat{\vartheta} | 0.47425 ^{\{2\}} | 0.52401 ^{\{4\}} | 0.52337 ^{\{3\}} | 0.46463 ^{\{1\}} | 0.54588 ^{\{6\}} | 0.64689 ^{\{8\}} | 0.59726 ^{\{7\}} | 0.53428 ^{\{5\}} | |
\hat{\varphi} | 0.27624 ^{\{1\}} | 0.38734 ^{\{7\}} | 0.29294 ^{\{2\}} | 0.36212 ^{\{6\}} | 0.31849 ^{\{4\}} | 0.39577 ^{\{8\}} | 0.30160 ^{\{3\}} | 0.35513 ^{\{5\}} | ||
\hat{\lambda} | 0.01107 ^{\{2\}} | 0.01423 ^{\{7\}} | 0.00983 ^{\{1\}} | 0.01356 ^{\{5\}} | 0.01357 ^{\{6\}} | 0.01651 ^{\{8\}} | 0.01289 ^{\{3\}} | 0.01296 ^{\{4\}} | ||
\hat{\theta} | 0.37764 ^{\{7\}} | 0.33320 ^{\{3\}} | 0.35778 ^{\{4\}} | 0.36101 ^{\{5\}} | 0.29381 ^{\{1\}} | 0.38887 ^{\{8\}} | 0.32193 ^{\{2\}} | 0.36311 ^{\{6\}} | ||
MREs | \hat{\vartheta} | 0.33229 ^{\{3\}} | 0.33987 ^{\{4\}} | 0.17807 ^{\{1\}} | 0.31166 ^{\{2\}} | 0.34603 ^{\{5\}} | 0.40963 ^{\{8\}} | 0.38540 ^{\{7\}} | 0.35123 ^{\{6\}} | |
\hat{\varphi} | 0.95101 ^{\{3\}} | 1.09624 ^{\{7\}} | 0.93641 ^{\{1\}} | 1.06186 ^{\{6\}} | 0.97231 ^{\{4\}} | 1.10208 ^{\{8\}} | 0.93811 ^{\{2\}} | 1.03788 ^{\{5\}} | ||
\hat{\lambda} | 0.35719 ^{\{2\}} | 0.41405 ^{\{7\}} | 0.33607 ^{\{1\}} | 0.40230 ^{\{5\}} | 0.40307 ^{\{6\}} | 0.44817 ^{\{8\}} | 0.39034 ^{\{3\}} | 0.39464 ^{\{4\}} | ||
\hat{\theta} | 0.39799 ^{\{7\}} | 0.37998 ^{\{4\}} | 0.37507 ^{\{3\}} | 0.38691 ^{\{5\}} | 0.36190 ^{\{1\}} | 0.42628 ^{\{8\}} | 0.37204 ^{\{2\}} | 0.38886 ^{\{6\}} | ||
\sum{Ranks} | 43 ^{\{2\}} | 65 ^{\{7\}} | 23 ^{\{1\}} | 53 ^{\{5\}} | 46 ^{\{4\}} | 96 ^{\{8\}} | 44 ^{\{3\}} | 62 ^{\{6\}} | ||
80 | |BIAS| | \hat{\vartheta} | 0.65720 ^{\{2\}} | 0.73260 ^{\{6\}} | 0.64523 ^{\{1\}} | 0.71780 ^{\{4\}} | 0.67014 ^{\{3\}} | 0.78730 ^{\{8\}} | 0.72979 ^{\{5\}} | 0.78285 ^{\{7\}} |
\hat{\varphi} | 0.29918 ^{\{4\}} | 0.36951 ^{\{7\}} | 0.27270 ^{\{1\}} | 0.36572 ^{\{6\}} | 0.28461 ^{\{2\}} | 0.43485 ^{\{8\}} | 0.29440 ^{\{3\}} | 0.31911 ^{\{5\}} | ||
\hat{\lambda} | 0.06946 ^{\{2\}} | 0.08089 ^{\{7\}} | 0.06755 ^{\{1\}} | 0.07955 ^{\{6\}} | 0.07162 ^{\{4\}} | 0.07099 ^{\{3\}} | 0.07301 ^{\{5\}} | 0.08347 ^{\{8\}} | ||
\hat{\theta} | 0.41705 ^{\{6\}} | 0.41605 ^{\{5\}} | 0.41277 ^{\{4\}} | 0.43216 ^{\{7\}} | 0.34179 ^{\{1\}} | 0.38656 ^{\{3\}} | 0.37952 ^{\{2\}} | 0.45183 ^{\{8\}} | ||
MSEs | \hat{\vartheta} | 0.53699 ^{\{1\}} | 0.65792 ^{\{5\}} | 0.71214 ^{\{7\}} | 0.63722 ^{\{3\}} | 0.62257 ^{\{2\}} | 0.71659 ^{\{8\}} | 0.64185 ^{\{4\}} | 0.70948 ^{\{6\}} | |
\hat{\varphi} | 0.12478 ^{\{3\}} | 0.19502 ^{\{7\}} | 0.11097 ^{\{1\}} | 0.18881 ^{\{6\}} | 0.12320 ^{\{2\}} | 0.28084 ^{\{8\}} | 0.13150 ^{\{4\}} | 0.15268 ^{\{5\}} | ||
\hat{\lambda} | 0.00672 ^{\{1\}} | 0.00884 ^{\{7\}} | 0.00693 ^{\{2\}} | 0.00853 ^{\{6\}} | 0.00712 ^{\{4\}} | 0.00711 ^{\{3\}} | 0.00740 ^{\{5\}} | 0.00932 ^{\{8\}} | ||
\hat{\theta} | 0.26049 ^{\{5\}} | 0.25434 ^{\{4\}} | 0.30456 ^{\{8\}} | 0.27845 ^{\{6\}} | 0.16695 ^{\{1\}} | 0.24032 ^{\{3\}} | 0.20942 ^{\{2\}} | 0.29980 ^{\{7\}} | ||
MREs | \hat{\vartheta} | 0.37554 ^{\{2\}} | 0.41863 ^{\{6\}} | 0.21508 ^{\{1\}} | 0.41017 ^{\{4\}} | 0.38294 ^{\{3\}} | 0.44989 ^{\{8\}} | 0.41702 ^{\{5\}} | 0.44734 ^{\{7\}} | |
\hat{\varphi} | 0.59835 ^{\{4\}} | 0.73902 ^{\{7\}} | 0.54540 ^{\{1\}} | 0.73144 ^{\{6\}} | 0.56922 ^{\{2\}} | 0.86970 ^{\{8\}} | 0.58881 ^{\{3\}} | 0.63822 ^{\{5\}} | ||
\hat{\lambda} | 0.27786 ^{\{2\}} | 0.32356 ^{\{7\}} | 0.27019 ^{\{1\}} | 0.31820 ^{\{6\}} | 0.28647 ^{\{4\}} | 0.28396 ^{\{3\}} | 0.29205 ^{\{5\}} | 0.33388 ^{\{8\}} | ||
\hat{\theta} | 0.33364 ^{\{6\}} | 0.33284 ^{\{5\}} | 0.33022 ^{\{4\}} | 0.34573 ^{\{7\}} | 0.27343 ^{\{1\}} | 0.30924 ^{\{3\}} | 0.30361 ^{\{2\}} | 0.36147 ^{\{8\}} | ||
\sum{Ranks} | 38 ^{\{3\}} | 73 ^{\{7\}} | 32 ^{\{2\}} | 67 ^{\{6\}} | 29 ^{\{1\}} | 66 ^{\{5\}} | 45 ^{\{4\}} | 82 ^{\{8\}} | ||
200 | |BIAS| | \hat{\vartheta} | 0.54244 ^{\{1\}} | 0.74060 ^{\{6\}} | 0.66029 ^{\{4\}} | 0.72065 ^{\{5\}} | 0.56860 ^{\{2\}} | 0.78528 ^{\{8\}} | 0.64417 ^{\{3\}} | 0.74318 ^{\{7\}} |
\hat{\varphi} | 0.20343 ^{\{3\}} | 0.27714 ^{\{7\}} | 0.18758 ^{\{1\}} | 0.27628 ^{\{6\}} | 0.19120 ^{\{2\}} | 0.33648 ^{\{8\}} | 0.21264 ^{\{4\}} | 0.21990 ^{\{5\}} | ||
\hat{\lambda} | 0.05329 ^{\{1\}} | 0.07033 ^{\{7\}} | 0.05985 ^{\{3\}} | 0.06898 ^{\{6\}} | 0.05669 ^{\{2\}} | 0.05992 ^{\{4\}} | 0.06059 ^{\{5\}} | 0.07617 ^{\{8\}} | ||
\hat{\theta} | 0.30910 ^{\{2\}} | 0.38666 ^{\{6\}} | 0.37834 ^{\{5\}} | 0.39240 ^{\{7\}} | 0.26754 ^{\{1\}} | 0.34697 ^{\{4\}} | 0.32167 ^{\{3\}} | 0.42492 ^{\{8\}} | ||
MSEs | \hat{\vartheta} | 0.39682 ^{\{1\}} | 0.65105 ^{\{6\}} | 0.72704 ^{\{8\}} | 0.62292 ^{\{4\}} | 0.51529 ^{\{2\}} | 0.70871 ^{\{7\}} | 0.53035 ^{\{3\}} | 0.65051 ^{\{5\}} | |
\hat{\varphi} | 0.06226 ^{\{3\}} | 0.11401 ^{\{7\}} | 0.05424 ^{\{1\}} | 0.11214 ^{\{6\}} | 0.05643 ^{\{2\}} | 0.18593 ^{\{8\}} | 0.06927 ^{\{4\}} | 0.07447 ^{\{5\}} | ||
\hat{\lambda} | 0.00405 ^{\{1\}} | 0.00665 ^{\{7\}} | 0.00571 ^{\{5\}} | 0.00644 ^{\{6\}} | 0.00452 ^{\{2\}} | 0.00513 ^{\{4\}} | 0.00507 ^{\{3\}} | 0.00753 ^{\{8\}} | ||
\hat{\theta} | 0.14395 ^{\{2\}} | 0.20851 ^{\{5\}} | 0.26568 ^{\{8\}} | 0.22075 ^{\{6\}} | 0.10322 ^{\{1\}} | 0.19203 ^{\{4\}} | 0.14560 ^{\{3\}} | 0.25309 ^{\{7\}} | ||
MREs | \hat{\vartheta} | 0.30997 ^{\{2\}} | 0.42320 ^{\{6\}} | 0.22010 ^{\{1\}} | 0.41180 ^{\{5\}} | 0.32491 ^{\{3\}} | 0.44873 ^{\{8\}} | 0.36810 ^{\{4\}} | 0.42468 ^{\{7\}} | |
\hat{\varphi} | 0.40685 ^{\{3\}} | 0.55429 ^{\{7\}} | 0.37516 ^{\{1\}} | 0.55256 ^{\{6\}} | 0.38240 ^{\{2\}} | 0.67295 ^{\{8\}} | 0.42528 ^{\{4\}} | 0.43980 ^{\{5\}} | ||
\hat{\lambda} | 0.21316 ^{\{1\}} | 0.28134 ^{\{7\}} | 0.23940 ^{\{3\}} | 0.27593 ^{\{6\}} | 0.22675 ^{\{2\}} | 0.23969 ^{\{4\}} | 0.24238 ^{\{5\}} | 0.30470 ^{\{8\}} | ||
\hat{\theta} | 0.24728 ^{\{2\}} | 0.30933 ^{\{6\}} | 0.30267 ^{\{5\}} | 0.31392 ^{\{7\}} | 0.21403 ^{\{1\}} | 0.27758 ^{\{4\}} | 0.25734 ^{\{3\}} | 0.33994 ^{\{8\}} | ||
\sum{Ranks} | 22 ^{\{1.5\}} | 77 ^{\{7\}} | 45 ^{\{4\}} | 70 ^{\{5\}} | 22 ^{\{1.5\}} | 71 ^{\{6\}} | 44 ^{\{3\}} | 81 ^{\{8\}} | ||
500 | |BIAS| | \hat{\vartheta} | 0.40816 ^{\{2\}} | 0.63822 ^{\{6\}} | 0.59712 ^{\{4\}} | 0.62303 ^{\{5\}} | 0.38723 ^{\{1\}} | 0.72351 ^{\{8\}} | 0.50869 ^{\{3\}} | 0.64537 ^{\{7\}} |
\hat{\varphi} | 0.13392 ^{\{3\}} | 0.19775 ^{\{6\}} | 0.12778 ^{\{1\}} | 0.19966 ^{\{7\}} | 0.13208 ^{\{2\}} | 0.26446 ^{\{8\}} | 0.14927 ^{\{4\}} | 0.15660 ^{\{5\}} | ||
\hat{\lambda} | 0.03902 ^{\{1\}} | 0.05985 ^{\{7\}} | 0.04987 ^{\{4\}} | 0.05913 ^{\{6\}} | 0.04014 ^{\{2\}} | 0.05408 ^{\{5\}} | 0.04806 ^{\{3\}} | 0.06618 ^{\{8\}} | ||
\hat{\theta} | 0.21255 ^{\{2\}} | 0.32416 ^{\{6\}} | 0.31285 ^{\{5\}} | 0.33146 ^{\{7\}} | 0.18308 ^{\{1\}} | 0.30762 ^{\{4\}} | 0.24975 ^{\{3\}} | 0.35803 ^{\{8\}} | ||
MSEs | \hat{\vartheta} | 0.24842 ^{\{1\}} | 0.52025 ^{\{5\}} | 0.58302 ^{\{7\}} | 0.50041 ^{\{4\}} | 0.33081 ^{\{2\}} | 0.63280 ^{\{8\}} | 0.37071 ^{\{3\}} | 0.52827 ^{\{6\}} | |
\hat{\varphi} | 0.02762 ^{\{3\}} | 0.05937 ^{\{6\}} | 0.02573 ^{\{1\}} | 0.06052 ^{\{7\}} | 0.02727 ^{\{2\}} | 0.12045 ^{\{8\}} | 0.03399 ^{\{4\}} | 0.03724 ^{\{5\}} | ||
\hat{\lambda} | 0.00224 ^{\{1\}} | 0.00478 ^{\{7\}} | 0.00422 ^{\{5\}} | 0.00470 ^{\{6\}} | 0.00257 ^{\{2\}} | 0.00402 ^{\{4\}} | 0.00325 ^{\{3\}} | 0.00569 ^{\{8\}} | ||
\hat{\theta} | 0.06777 ^{\{2\}} | 0.14391 ^{\{5\}} | 0.19157 ^{\{8\}} | 0.15413 ^{\{6\}} | 0.05743 ^{\{1\}} | 0.14128 ^{\{4\}} | 0.08776 ^{\{3\}} | 0.17743 ^{\{7\}} | ||
MREs | \hat{\vartheta} | 0.23323 ^{\{3\}} | 0.36470 ^{\{6\}} | 0.19904 ^{\{1\}} | 0.35602 ^{\{5\}} | 0.22127 ^{\{2\}} | 0.41343 ^{\{8\}} | 0.29068 ^{\{4\}} | 0.36879 ^{\{7\}} | |
\hat{\varphi} | 0.26784 ^{\{3\}} | 0.39550 ^{\{6\}} | 0.25556 ^{\{1\}} | 0.39932 ^{\{7\}} | 0.26417 ^{\{2\}} | 0.52891 ^{\{8\}} | 0.29853 ^{\{4\}} | 0.31320 ^{\{5\}} | ||
\hat{\lambda} | 0.15609 ^{\{1\}} | 0.23939 ^{\{7\}} | 0.19949 ^{\{4\}} | 0.23651 ^{\{6\}} | 0.16056 ^{\{2\}} | 0.21632 ^{\{5\}} | 0.19222 ^{\{3\}} | 0.26474 ^{\{8\}} | ||
\hat{\theta} | 0.17004 ^{\{2\}} | 0.25933 ^{\{6\}} | 0.25028 ^{\{5\}} | 0.26517 ^{\{7\}} | 0.14646 ^{\{1\}} | 0.24610 ^{\{4\}} | 0.19980 ^{\{3\}} | 0.28642 ^{\{8\}} | ||
\sum{Ranks} | 24 ^{\{2\}} | 73 ^{\{5.5\}} | 46 ^{\{4\}} | 73 ^{\{5.5\}} | 20 ^{\{1\}} | 74 ^{\{7\}} | 40 ^{\{3\}} | 82 ^{\{8\}} |
n | Est. | Est. Par. | MLEs | LSEs | WLSEs | CRVMEs | MPSEs | PCEs | ADEs | RADEs |
20 | |BIAS| | \hat{\vartheta} | 0.58249 ^{\left\{3 \right\}} | 0.53932 ^{\left\{1 \right\}} | 0.56241 ^{\left\{2 \right\}} | 0.61397 ^{\left\{5 \right\}} | 0.60739 ^{\left\{4 \right\}} | 0.82341 ^{\left\{8 \right\}} | 0.68123 ^{\left\{7 \right\}} | 0.66592 ^{\left\{6 \right\}} |
\hat{\varphi} | 0.70201 ^{\left\{2 \right\}} | 0.74992 ^{\left\{4 \right\}} | 0.71005 ^{\left\{3 \right\}} | 0.99334 ^{\left\{7 \right\}} | 0.41044 ^{\left\{1 \right\}} | 1.11769 ^{\left\{8 \right\}} | 0.88784 ^{\left\{5 \right\}} | 0.97457 ^{\left\{6 \right\}} | ||
\hat{\lambda} | 0.26246 ^{\left\{1 \right\}} | 0.28717 ^{\left\{3 \right\}} | 0.28400 ^{\left\{2 \right\}} | 0.42881 ^{\left\{5 \right\}} | 0.40138 ^{\left\{4 \right\}} | 0.64545 ^{\left\{8 \right\}} | 0.43589 ^{\left\{7 \right\}} | 0.43050 ^{\left\{6 \right\}} | ||
\hat{\theta} | 0.83485 ^{\left\{6 \right\}} | 0.91523 ^{\left\{7 \right\}} | 0.92408 ^{\left\{8 \right\}} | 0.76222 ^{\left\{3 \right\}} | 0.77841 ^{\left\{4 \right\}} | 0.78092 ^{\left\{5 \right\}} | 0.74535 ^{\left\{1 \right\}} | 0.74828 ^{\left\{2 \right\}} | ||
MSEs | \hat{\vartheta} | 0.45340 ^{\left\{3 \right\}} | 0.42087 ^{\left\{1 \right\}} | 0.44427 ^{\left\{2 \right\}} | 0.51220 ^{\left\{4 \right\}} | 0.51720 ^{\left\{5 \right\}} | 0.77654 ^{\left\{8 \right\}} | 0.58720 ^{\left\{7 \right\}} | 0.56968 ^{\left\{6 \right\}} | |
\hat{\varphi} | 0.65182 ^{\left\{1 \right\}} | 0.75540 ^{\left\{3 \right\}} | 0.66110 ^{\left\{2 \right\}} | 1.25282 ^{\left\{7 \right\}} | 0.95641 ^{\left\{4 \right\}} | 1.49737 ^{\left\{8 \right\}} | 1.01553 ^{\left\{5 \right\}} | 1.19476 ^{\left\{6 \right\}} | ||
\hat{\lambda} | 0.07059 ^{\left\{1 \right\}} | 0.08751 ^{\left\{3 \right\}} | 0.08543 ^{\left\{2 \right\}} | 0.29909 ^{\left\{7 \right\}} | 0.24742 ^{\left\{4 \right\}} | 0.52940 ^{\left\{8 \right\}} | 0.29252 ^{\left\{6 \right\}} | 0.29154 ^{\left\{5 \right\}} | ||
\hat{\theta} | 0.84612 ^{\left\{6 \right\}} | 1.00860 ^{\left\{8 \right\}} | 1.00532 ^{\left\{7 \right\}} | 0.71906 ^{\left\{3 \right\}} | 0.75325 ^{\left\{4 \right\}} | 0.82231 ^{\left\{5 \right\}} | 0.69565 ^{\left\{1 \right\}} | 0.70768 ^{\left\{2 \right\}} | ||
MREs | \hat{\vartheta} | 0.33285 ^{\left\{3 \right\}} | 0.30818 ^{\left\{1 \right\}} | 0.32138 ^{\left\{2 \right\}} | 0.35084 ^{\left\{5 \right\}} | 0.34708 ^{\left\{4 \right\}} | 0.47052 ^{\left\{8 \right\}} | 0.38927 ^{\left\{7 \right\}} | 0.38052 ^{\left\{6 \right\}} | |
\hat{\varphi} | 0.35100 ^{\left\{1 \right\}} | 0.37496 ^{\left\{3 \right\}} | 0.35503 ^{\left\{2 \right\}} | 0.49667 ^{\left\{7 \right\}} | 0.42863 ^{\left\{4 \right\}} | 0.55884 ^{\left\{8 \right\}} | 0.44392 ^{\left\{5 \right\}} | 0.48728 ^{\left\{6 \right\}} | ||
\hat{\lambda} | 0.17497 ^{\left\{1 \right\}} | 0.19145 ^{\left\{3 \right\}} | 0.18933 ^{\left\{2 \right\}} | 0.28587 ^{\left\{5 \right\}} | 0.26758 ^{\left\{4 \right\}} | 0.43030 ^{\left\{8 \right\}} | 0.29059 ^{\left\{7 \right\}} | 0.28700 ^{\left\{6 \right\}} | ||
\hat{\theta} | 0.33394 ^{\left\{6 \right\}} | 0.36609 ^{\left\{7 \right\}} | 0.36963 ^{\left\{8 \right\}} | 0.30489 ^{\left\{3 \right\}} | 0.31136 ^{\left\{4 \right\}} | 0.31237 ^{\left\{5 \right\}} | 0.29814 ^{\left\{1 \right\}} | 0.29931 ^{\left\{2 \right\}} | ||
\sum{Ranks} | {\textbf{34}} ^{\left\{1 \right\}} | {\textbf{44}} ^{\left\{3 \right\}} | {\textbf{42}} ^{\left\{2 \right\}} | {\textbf{61}} ^{\left\{7 \right\}} | {\textbf{46}} ^{\left\{4 \right\}} | {\textbf{87}} ^{\left\{8 \right\}} | {\textbf{59}} ^{\left\{5.5 \right\}} | {\textbf{59}} ^{\left\{5.5 \right\}} | ||
80 | |BIAS| | \hat{\vartheta} | 0.49925 ^{\left\{2 \right\}} | 0.47135 ^{\left\{1 \right\}} | 0.52766 ^{\left\{3 \right\}} | 0.66329 ^{\left\{5 \right\}} | 0.55966 ^{\left\{4 \right\}} | 0.89224 ^{\left\{8 \right\}} | 0.67228 ^{\left\{6 \right\}} | 0.69447 ^{\left\{7 \right\}} |
\hat{\varphi} | 0.52063 ^{\left\{1 \right\}} | 0.55025 ^{\left\{3 \right\}} | 0.52909 ^{\left\{2 \right\}} | 0.70683 ^{\left\{7 \right\}} | 0.56354 ^{\left\{4 \right\}} | 0.93582 ^{\left\{8 \right\}} | 0.63998 ^{\left\{5 \right\}} | 0.69954 ^{\left\{6 \right\}} | ||
\hat{\lambda} | 0.25198 ^{\left\{1 \right\}} | 0.25883 ^{\left\{3 \right\}} | 0.25641 ^{\left\{2 \right\}} | 0.43311 ^{\left\{7 \right\}} | 0.30034 ^{\left\{4 \right\}} | 0.66563 ^{\left\{8 \right\}} | 0.40607 ^{\left\{5 \right\}} | 0.43206 ^{\left\{6 \right\}} | ||
\hat{\theta} | 0.78480 ^{\left\{6 \right\}} | 0.82532 ^{\left\{7 \right\}} | 0.83697 ^{\left\{8 \right\}} | 0.69063 ^{\left\{3 \right\}} | 0.58559 ^{\left\{1 \right\}} | 0.72122 ^{\left\{5 \right\}} | 0.63842 ^{\left\{2 \right\}} | 0.69164 ^{\left\{4 \right\}} | ||
MSEs | \hat{\vartheta} | 0.31719 ^{\left\{2 \right\}} | 0.30026 ^{\left\{1 \right\}} | 0.34946 ^{\left\{3 \right\}} | 0.55815 ^{\left\{5 \right\}} | 0.49857 ^{\left\{4 \right\}} | 0.86629 ^{\left\{8 \right\}} | 0.56483 ^{\left\{6 \right\}} | 0.59417 ^{\left\{7 \right\}} | |
\hat{\varphi} | 0.27881 ^{\left\{1 \right\}} | 0.32553 ^{\left\{3 \right\}} | 0.29109 ^{\left\{2 \right\}} | 0.66883 ^{\left\{7 \right\}} | 0.44978 ^{\left\{4 \right\}} | 1.03760 ^{\left\{8 \right\}} | 0.55511 ^{\left\{5 \right\}} | 0.64550 ^{\left\{6 \right\}} | ||
\hat{\lambda} | 0.06369 ^{\left\{1 \right\}} | 0.06795 ^{\left\{3 \right\}} | 0.06642 ^{\left\{2 \right\}} | 0.29117 ^{\left\{7 \right\}} | 0.15725 ^{\left\{4 \right\}} | 0.54984 ^{\left\{8 \right\}} | 0.25250 ^{\left\{5 \right\}} | 0.28331 ^{\left\{6 \right\}} | ||
\hat{\theta} | 0.66344 ^{\left\{5 \right\}} | 0.73666 ^{\left\{7 \right\}} | 0.74889 ^{\left\{8 \right\}} | 0.58730 ^{\left\{3 \right\}} | 0.45144 ^{\left\{1 \right\}} | 0.66717 ^{\left\{6 \right\}} | 0.51377 ^{\left\{2 \right\}} | 0.59201 ^{\left\{4 \right\}} | ||
MREs | \hat{\vartheta} | 0.28529 ^{\left\{2 \right\}} | 0.26934 ^{\left\{1 \right\}} | 0.30152 ^{\left\{3 \right\}} | 0.37902 ^{\left\{5 \right\}} | 0.31981 ^{\left\{4 \right\}} | 0.50985 ^{\left\{8 \right\}} | 0.38416 ^{\left\{6 \right\}} | 0.39684 ^{\left\{7 \right\}} | |
\hat{\varphi} | 0.26032 ^{\left\{1 \right\}} | 0.27512 ^{\left\{3 \right\}} | 0.26454 ^{\left\{2 \right\}} | 0.35341 ^{\left\{7 \right\}} | 0.28177 ^{\left\{4 \right\}} | 0.46791 ^{\left\{8 \right\}} | 0.31999 ^{\left\{5 \right\}} | 0.34977 ^{\left\{6 \right\}} | ||
\hat{\lambda} | 0.16798 ^{\left\{1 \right\}} | 0.17255 ^{\left\{3 \right\}} | 0.17094 ^{\left\{2 \right\}} | 0.28874 ^{\left\{7 \right\}} | 0.20023 ^{\left\{4 \right\}} | 0.44375 ^{\left\{8 \right\}} | 0.27071 ^{\left\{5 \right\}} | 0.28804 ^{\left\{6 \right\}} | ||
\hat{\theta} | 0.31392 ^{\left\{6 \right\}} | 0.33013 ^{\left\{7 \right\}} | 0.33479 ^{\left\{8 \right\}} | 0.27625 ^{\left\{3 \right\}} | 0.23423 ^{\left\{1 \right\}} | 0.28849 ^{\left\{5 \right\}} | 0.25537 ^{\left\{2 \right\}} | 0.27666 ^{\left\{4 \right\}} | ||
\sum{Ranks} | {\textbf{29}} ^{\left\{1 \right\}} | {\textbf{42}} ^{\left\{3 \right\}} | {\textbf{45}} ^{\left\{4 \right\}} | {\textbf{66}} ^{\left\{6 \right\}} | {\textbf{39}} ^{\left\{2 \right\}} | {\textbf{88}} ^{\left\{8 \right\}} | {\textbf{54}} ^{\left\{5 \right\}} | {\textbf{69}} ^{\left\{7 \right\}} | ||
200 | |BIAS| | \hat{\vartheta} | 0.47555 ^{\left\{3 \right\}} | 0.44985 ^{\left\{2 \right\}} | 0.51341 ^{\left\{4 \right\}} | 0.67707 ^{\left\{6 \right\}} | 0.42436 ^{\left\{1 \right\}} | 0.89805 ^{\left\{8 \right\}} | 0.66134 ^{\left\{5 \right\}} | 0.69895 ^{\left\{7 \right\}} |
\hat{\varphi} | 0.50115 ^{\left\{2 \right\}} | 0.50837 ^{\left\{4 \right\}} | 0.50279 ^{\left\{3 \right\}} | 0.56296 ^{\left\{7 \right\}} | 0.41044 ^{\left\{1 \right\}} | 0.81679 ^{\left\{8 \right\}} | 0.51451 ^{\left\{5 \right\}} | 0.55936 ^{\left\{6 \right\}} | ||
\hat{\lambda} | 0.25019 ^{\left\{2 \right\}} | 0.25187 ^{\left\{4 \right\}} | 0.25076 ^{\left\{3 \right\}} | 0.41917 ^{\left\{7 \right\}} | 0.21499 ^{\left\{1 \right\}} | 0.65544 ^{\left\{8 \right\}} | 0.37297 ^{\left\{5 \right\}} | 0.41435 ^{\left\{6 \right\}} | ||
\hat{\theta} | 0.79159 ^{\left\{6 \right\}} | 0.80107 ^{\left\{7 \right\}} | 0.82123 ^{\left\{8 \right\}} | 0.64647 ^{\left\{3 \right\}} | 0.43028 ^{\left\{1 \right\}} | 0.75308 ^{\left\{5 \right\}} | 0.57181 ^{\left\{2 \right\}} | 0.66234 ^{\left\{4 \right\}} | ||
MSEs | \hat{\vartheta} | 0.25888 ^{\left\{2 \right\}} | 0.23847 ^{\left\{1 \right\}} | 0.29413 ^{\left\{3 \right\}} | 0.56097 ^{\left\{6 \right\}} | 0.37531 ^{\left\{4 \right\}} | 0.86783 ^{\left\{8 \right\}} | 0.54545 ^{\left\{5 \right\}} | 0.59107 ^{\left\{7 \right\}} | |
\hat{\varphi} | 0.25134 ^{\left\{1 \right\}} | 0.26082 ^{\left\{4 \right\}} | 0.25349 ^{\left\{2 \right\}} | 0.43952 ^{\left\{7 \right\}} | 0.25968 ^{\left\{3 \right\}} | 0.80523 ^{\left\{8 \right\}} | 0.37160 ^{\left\{5 \right\}} | 0.43084 ^{\left\{6 \right\}} | ||
\hat{\lambda} | 0.06261 ^{\left\{1 \right\}} | 0.06361 ^{\left\{3 \right\}} | 0.06295 ^{\left\{2 \right\}} | 0.25891 ^{\left\{7 \right\}} | 0.09970 ^{\left\{4 \right\}} | 0.52619 ^{\left\{8 \right\}} | 0.20829 ^{\left\{5 \right\}} | 0.25203 ^{\left\{6 \right\}} | ||
\hat{\theta} | 0.64670 ^{\left\{5 \right\}} | 0.66161 ^{\left\{6 \right\}} | 0.69240 ^{\left\{8 \right\}} | 0.51309 ^{\left\{3 \right\}} | 0.27527 ^{\left\{1 \right\}} | 0.68347 ^{\left\{7 \right\}} | 0.41304 ^{\left\{2 \right\}} | 0.53268 ^{\left\{4 \right\}} | ||
MREs | \hat{\vartheta} | 0.27175 ^{\left\{3 \right\}} | 0.25705 ^{\left\{2 \right\}} | 0.29338 ^{\left\{4 \right\}} | 0.38690 ^{\left\{6 \right\}} | 0.24249 ^{\left\{1 \right\}} | 0.51317 ^{\left\{8 \right\}} | 0.37791 ^{\left\{5 \right\}} | 0.39940 ^{\left\{7 \right\}} | |
\hat{\varphi} | 0.25057 ^{\left\{2 \right\}} | 0.25419 ^{\left\{4 \right\}} | 0.25139 ^{\left\{3 \right\}} | 0.28148 ^{\left\{7 \right\}} | 0.20522 ^{\left\{1 \right\}} | 0.40839 ^{\left\{8 \right\}} | 0.25725 ^{\left\{5 \right\}} | 0.27968 ^{\left\{6 \right\}} | ||
\hat{\lambda} | 0.16679 ^{\left\{2 \right\}} | 0.16791 ^{\left\{4 \right\}} | 0.16717 ^{\left\{3 \right\}} | 0.27945 ^{\left\{7 \right\}} | 0.14333 ^{\left\{1 \right\}} | 0.43696 ^{\left\{8 \right\}} | 0.24865 ^{\left\{5 \right\}} | 0.27623 ^{\left\{6 \right\}} | ||
\hat{\theta} | 0.31664 ^{\left\{6 \right\}} | 0.32043 ^{\left\{7 \right\}} | 0.32849 ^{\left\{8 \right\}} | 0.25859 ^{\left\{3 \right\}} | 0.17211 ^{\left\{1 \right\}} | 0.30123 ^{\left\{5 \right\}} | 0.22872 ^{\left\{2 \right\}} | 0.26494 ^{\left\{4 \right\}} | ||
\sum{Ranks} | {\textbf{35}} ^{\left\{2 \right\}} | {\textbf{48}} ^{\left\{3 \right\}} | {\textbf{51}} ^{\left\{4.5 \right\}} | {\textbf{69}} ^{\left\{6.5 \right\}} | {\textbf{20}} ^{\left\{1 \right\}} | {\textbf{89}} ^{\left\{8 \right\}} | {\textbf{51}} ^{\left\{4.5 \right\}} | {\textbf{69}} ^{\left\{6.5 \right\}} | ||
500 | |BIAS| | \hat{\vartheta} | 0.47314 ^{\left\{3 \right\}} | 0.44590 ^{\left\{2 \right\}} | 0.51215 ^{\left\{4 \right\}} | 0.64549 ^{\left\{6 \right\}} | 0.23589 ^{\left\{1 \right\}} | 0.89642 ^{\left\{8 \right\}} | 0.57765 ^{\left\{5 \right\}} | 0.66728 ^{\left\{7 \right\}} |
\hat{\varphi} | 0.50000 ^{\left\{5 \right\}} | 0.50031 ^{\left\{7 \right\}} | 0.50001 ^{\left\{6 \right\}} | 0.44580 ^{\left\{4 \right\}} | 0.25497 ^{\left\{1 \right\}} | 0.66140 ^{\left\{8 \right\}} | 0.39329 ^{\left\{2 \right\}} | 0.42269 ^{\left\{3 \right\}} | ||
\hat{\lambda} | 0.25000 ^{\left\{2 \right\}} | 0.25003 ^{\left\{4 \right\}} | 0.25001 ^{\left\{3 \right\}} | 0.38068 ^{\left\{6 \right\}} | 0.11500 ^{\left\{1 \right\}} | 0.63857 ^{\left\{8 \right\}} | 0.31593 ^{\left\{5 \right\}} | 0.38454 ^{\left\{7 \right\}} | ||
\hat{\theta} | 0.79445 ^{\left\{5 \right\}} | 0.80134 ^{\left\{6 \right\}} | 0.82604 ^{\left\{8 \right\}} | 0.59243 ^{\left\{3 \right\}} | 0.25280 ^{\left\{1 \right\}} | 0.80505 ^{\left\{7 \right\}} | 0.49356 ^{\left\{2 \right\}} | 0.61821 ^{\left\{4 \right\}} | ||
MSEs | \hat{\vartheta} | 0.23703 ^{\left\{3 \right\}} | 0.21187 ^{\left\{2 \right\}} | 0.27432 ^{\left\{4 \right\}} | 0.51809 ^{\left\{6 \right\}} | 0.19898 ^{\left\{1 \right\}} | 0.86166 ^{\left\{8 \right\}} | 0.44538 ^{\left\{5 \right\}} | 0.54549 ^{\left\{7 \right\}} | |
\hat{\varphi} | 0.25000 ^{\left\{3 \right\}} | 0.25034 ^{\left\{5 \right\}} | 0.25001 ^{\left\{4 \right\}} | 0.28548 ^{\left\{7 \right\}} | 0.11705 ^{\left\{1 \right\}} | 0.56366 ^{\left\{8 \right\}} | 0.22462 ^{\left\{2 \right\}} | 0.25796 ^{\left\{6 \right\}} | ||
\hat{\lambda} | 0.06250 ^{\left\{2.5 \right\}} | 0.06252 ^{\left\{4 \right\}} | 0.06250 ^{\left\{2.5 \right\}} | 0.20888 ^{\left\{6 \right\}} | 0.03935 ^{\left\{1 \right\}} | 0.50179 ^{\left\{8 \right\}} | 0.14873 ^{\left\{5 \right\}} | 0.20922 ^{\left\{7 \right\}} | ||
\hat{\theta} | 0.63903 ^{\left\{5 \right\}} | 0.64949 ^{\left\{6 \right\}} | 0.68943 ^{\left\{7 \right\}} | 0.43597 ^{\left\{3 \right\}} | 0.12546 ^{\left\{1 \right\}} | 0.74499 ^{\left\{8 \right\}} | 0.31912 ^{\left\{2 \right\}} | 0.46804 ^{\left\{4 \right\}} | ||
MREs | \hat{\vartheta} | 0.27037 ^{\left\{3 \right\}} | 0.25480 ^{\left\{2 \right\}} | 0.29266 ^{\left\{4 \right\}} | 0.36885 ^{\left\{6 \right\}} | 0.13479 ^{\left\{1 \right\}} | 0.51224 ^{\left\{8 \right\}} | 0.33008 ^{\left\{5 \right\}} | 0.38130 ^{\left\{7 \right\}} | |
\hat{\varphi} | 0.25000 ^{\left\{5.5 \right\}} | 0.25015 ^{\left\{7 \right\}} | 0.25000 ^{\left\{5.5 \right\}} | 0.22290 ^{\left\{4 \right\}} | 0.12748 ^{\left\{1 \right\}} | 0.33070 ^{\left\{8 \right\}} | 0.19665 ^{\left\{2 \right\}} | 0.21134 ^{\left\{3 \right\}} | ||
\hat{\lambda} | 0.16667 ^{\left\{2.5 \right\}} | 0.16669 ^{\left\{4 \right\}} | 0.16667 ^{\left\{2.5 \right\}} | 0.25379 ^{\left\{6 \right\}} | 0.07667 ^{\left\{1 \right\}} | 0.42571 ^{\left\{8 \right\}} | 0.21062 ^{\left\{5 \right\}} | 0.25636 ^{\left\{7 \right\}} | ||
\hat{\theta} | 0.31778 ^{\left\{5 \right\}} | 0.32053 ^{\left\{6 \right\}} | 0.33042 ^{\left\{8 \right\}} | 0.23697 ^{\left\{3 \right\}} | 0.10112 ^{\left\{1 \right\}} | 0.32202 ^{\left\{7 \right\}} | 0.19742 ^{\left\{2 \right\}} | 0.24728 ^{\left\{4 \right\}} | ||
\sum{Ranks} | {\textbf{44.5}} ^{\left\{3 \right\}} | {\textbf{55}} ^{\left\{4 \right\}} | {\textbf{58.5}} ^{\left\{5 \right\}} | {\textbf{60}} ^{\left\{6 \right\}} | {\textbf{12}} ^{\left\{1 \right\}} | {\textbf{94}} ^{\left\{8 \right\}} | {\textbf{42}} ^{\left\{2 \right\}} | {\textbf{66}} ^{\left\{7 \right\}} |
n | Est. | Est. Par. | MLEs | LSEs | WLSEs | CRVMEs | MPSEs | PCEs | ADEs | RADEs |
20 | |BIAS| | \hat{\vartheta} | 1.05821 ^{\left\{8 \right\}} | 0.62985 ^{\left\{3 \right\}} | 0.96882 ^{\left\{6 \right\}} | 0.61879 ^{\left\{2 \right\}} | 0.39728 ^{\left\{1 \right\}} | 1.00062 ^{\left\{7 \right\}} | 0.93184 ^{\left\{5 \right\}} | 0.74851 ^{\left\{4 \right\}} |
\hat{\varphi} | 0.58239 ^{\left\{4 \right\}} | 0.61540 ^{\left\{8 \right\}} | 0.57588 ^{\left\{3 \right\}} | 0.61442 ^{\left\{7 \right\}} | 0.16810 ^{\left\{1 \right\}} | 0.59648 ^{\left\{6 \right\}} | 0.54321 ^{\left\{2 \right\}} | 0.58344 ^{\left\{5 \right\}} | ||
\hat{\lambda} | 0.09205 ^{\left\{8 \right\}} | 0.08984 ^{\left\{5 \right\}} | 0.08749 ^{\left\{3 \right\}} | 0.09161 ^{\left\{6 \right\}} | 0.07490 ^{\left\{1 \right\}} | 0.09181 ^{\left\{7 \right\}} | 0.08360 ^{\left\{2 \right\}} | 0.08890 ^{\left\{4 \right\}} | ||
\hat{\theta} | 0.65193 ^{\left\{8 \right\}} | 0.48317 ^{\left\{2 \right\}} | 0.52978 ^{\left\{5 \right\}} | 0.51841 ^{\left\{4 \right\}} | 0.37874 ^{\left\{1 \right\}} | 0.57738 ^{\left\{7 \right\}} | 0.51808 ^{\left\{3 \right\}} | 0.53685 ^{\left\{6 \right\}} | ||
MSEs | \hat{\vartheta} | 1.62863 ^{\left\{8 \right\}} | 0.80009 ^{\left\{2 \right\}} | 1.26747 ^{\left\{6 \right\}} | 0.83422 ^{\left\{3 \right\}} | 0.44242 ^{\left\{1 \right\}} | 1.52407 ^{\left\{7 \right\}} | 1.26323 ^{\left\{5 \right\}} | 1.02485 ^{\left\{4 \right\}} | |
\hat{\varphi} | 0.42299 ^{\left\{3 \right\}} | 0.47351 ^{\left\{8 \right\}} | 0.43072 ^{\left\{4 \right\}} | 0.47310 ^{\left\{7 \right\}} | 0.39842 ^{\left\{2 \right\}} | 0.45399 ^{\left\{6 \right\}} | 0.39008 ^{\left\{1 \right\}} | 0.43912 ^{\left\{5 \right\}} | ||
\hat{\lambda} | 0.01191 ^{\left\{6 \right\}} | 0.01157 ^{\left\{5 \right\}} | 0.01104 ^{\left\{3 \right\}} | 0.01199 ^{\left\{7 \right\}} | 0.00839 ^{\left\{1 \right\}} | 0.01226 ^{\left\{8 \right\}} | 0.01037 ^{\left\{2 \right\}} | 0.01130 ^{\left\{4 \right\}} | ||
\hat{\theta} | 0.56633 ^{\left\{8 \right\}} | 0.35145 ^{\left\{2 \right\}} | 0.39993 ^{\left\{3 \right\}} | 0.40530 ^{\left\{5 \right\}} | 0.22597 ^{\left\{1 \right\}} | 0.46238 ^{\left\{7 \right\}} | 0.39998 ^{\left\{4 \right\}} | 0.42492 ^{\left\{6 \right\}} | ||
MREs | \hat{\vartheta} | 0.30235 ^{\left\{8 \right\}} | 0.17996 ^{\left\{3 \right\}} | 0.27681 ^{\left\{6 \right\}} | 0.17680 ^{\left\{2 \right\}} | 0.11351 ^{\left\{1 \right\}} | 0.28589 ^{\left\{7 \right\}} | 0.26624 ^{\left\{5 \right\}} | 0.21386 ^{\left\{4 \right\}} | |
\hat{\varphi} | 0.77652 ^{\left\{4 \right\}} | 0.82054 ^{\left\{8 \right\}} | 0.76784 ^{\left\{3 \right\}} | 0.81923 ^{\left\{7 \right\}} | 0.73001 ^{\left\{2 \right\}} | 0.79531 ^{\left\{6 \right\}} | 0.72428 ^{\left\{1 \right\}} | 0.77792 ^{\left\{5 \right\}} | ||
\hat{\lambda} | 0.18410 ^{\left\{8 \right\}} | 0.17968 ^{\left\{5 \right\}} | 0.17498 ^{\left\{3 \right\}} | 0.18321 ^{\left\{6 \right\}} | 0.14981 ^{\left\{1 \right\}} | 0.18363 ^{\left\{7 \right\}} | 0.16720 ^{\left\{2 \right\}} | 0.17780 ^{\left\{4 \right\}} | ||
\hat{\theta} | 0.52155 ^{\left\{8 \right\}} | 0.38654 ^{\left\{2 \right\}} | 0.42382 ^{\left\{5 \right\}} | 0.41473 ^{\left\{4 \right\}} | 0.30299 ^{\left\{1 \right\}} | 0.46190 ^{\left\{7 \right\}} | 0.41446 ^{\left\{3 \right\}} | 0.42948 ^{\left\{6 \right\}} | ||
\sum{Ranks} | {\textbf{81}} ^{\left\{7 \right\}} | {\textbf{53}} ^{\left\{4 \right\}} | {\textbf{50}} ^{\left\{3 \right\}} | {\textbf{60}} ^{\left\{6 \right\}} | {\textbf{14}} ^{\left\{1 \right\}} | {\textbf{82}} ^{\left\{8 \right\}} | {\textbf{35}} ^{\left\{2 \right\}} | {\textbf{57}} ^{\left\{5 \right\}} | ||
80 | |BIAS| | \hat{\vartheta} | 1.30458 ^{\left\{8 \right\}} | 0.99944 ^{\left\{2 \right\}} | 1.19014 ^{\left\{7 \right\}} | 1.02885 ^{\left\{3 \right\}} | 0.28553 ^{\left\{1 \right\}} | 1.10670 ^{\left\{4 \right\}} | 1.14568 ^{\left\{6 \right\}} | 1.11457 ^{\left\{5 \right\}} |
\hat{\varphi} | 0.32983 ^{\left\{3 \right\}} | 0.40620 ^{\left\{6 \right\}} | 0.34267 ^{\left\{4 \right\}} | 0.40746 ^{\left\{7 \right\}} | 0.27616 ^{\left\{1 \right\}} | 0.42471 ^{\left\{8 \right\}} | 0.32604 ^{\left\{2 \right\}} | 0.35934 ^{\left\{5 \right\}} | ||
\hat{\lambda} | 0.06496 ^{\left\{7 \right\}} | 0.06255 ^{\left\{5 \right\}} | 0.05976 ^{\left\{3 \right\}} | 0.06558 ^{\left\{8 \right\}} | 0.03810 ^{\left\{1 \right\}} | 0.06030 ^{\left\{4 \right\}} | 0.05724 ^{\left\{2 \right\}} | 0.06303 ^{\left\{6 \right\}} | ||
\hat{\theta} | 0.60157 ^{\left\{8 \right\}} | 0.45112 ^{\left\{2 \right\}} | 0.47825 ^{\left\{5 \right\}} | 0.49541 ^{\left\{6 \right\}} | 0.19099 ^{\left\{1 \right\}} | 0.45370 ^{\left\{3 \right\}} | 0.45453 ^{\left\{4 \right\}} | 0.50033 ^{\left\{7 \right\}} | ||
MSEs | \hat{\vartheta} | 1.98067 ^{\left\{8 \right\}} | 1.42246 ^{\left\{2 \right\}} | 1.62721 ^{\left\{7 \right\}} | 1.51028 ^{\left\{3 \right\}} | 0.28600 ^{\left\{1 \right\}} | 1.54773 ^{\left\{5 \right\}} | 1.54350 ^{\left\{4 \right\}} | 1.62460 ^{\left\{6 \right\}} | |
\hat{\varphi} | 0.16347 ^{\left\{2 \right\}} | 0.24145 ^{\left\{7 \right\}} | 0.17842 ^{\left\{4 \right\}} | 0.24070 ^{\left\{6 \right\}} | 0.12275 ^{\left\{1 \right\}} | 0.27309 ^{\left\{8 \right\}} | 0.16427 ^{\left\{3 \right\}} | 0.19753 ^{\left\{5 \right\}} | ||
\hat{\lambda} | 0.00614 ^{\left\{7 \right\}} | 0.00590 ^{\left\{5 \right\}} | 0.00549 ^{\left\{3 \right\}} | 0.00646 ^{\left\{8 \right\}} | 0.00224 ^{\left\{1 \right\}} | 0.00582 ^{\left\{4 \right\}} | 0.00513 ^{\left\{2 \right\}} | 0.00600 ^{\left\{6 \right\}} | ||
\hat{\theta} | 0.50150 ^{\left\{8 \right\}} | 0.33442 ^{\left\{3 \right\}} | 0.35764 ^{\left\{5 \right\}} | 0.39078 ^{\left\{6 \right\}} | 0.06108 ^{\left\{1 \right\}} | 0.33595 ^{\left\{4 \right\}} | 0.33240 ^{\left\{2 \right\}} | 0.39877 ^{\left\{7 \right\}} | ||
MREs | \hat{\vartheta} | 0.37274 ^{\left\{8 \right\}} | 0.28555 ^{\left\{2 \right\}} | 0.34004 ^{\left\{7 \right\}} | 0.29396 ^{\left\{3 \right\}} | 0.08158 ^{\left\{1 \right\}} | 0.31620 ^{\left\{4 \right\}} | 0.32734 ^{\left\{6 \right\}} | 0.31845 ^{\left\{5 \right\}} | |
\hat{\varphi} | 0.43977 ^{\left\{3 \right\}} | 0.54161 ^{\left\{6 \right\}} | 0.45690 ^{\left\{4 \right\}} | 0.54328 ^{\left\{7 \right\}} | 0.36821 ^{\left\{1 \right\}} | 0.56628 ^{\left\{8 \right\}} | 0.43472 ^{\left\{2 \right\}} | 0.47912 ^{\left\{5 \right\}} | ||
\hat{\lambda} | 0.12992 ^{\left\{7 \right\}} | 0.12509 ^{\left\{5 \right\}} | 0.11952 ^{\left\{3 \right\}} | 0.13116 ^{\left\{8 \right\}} | 0.07620 ^{\left\{1 \right\}} | 0.12061 ^{\left\{4 \right\}} | 0.11448 ^{\left\{2 \right\}} | 0.12607 ^{\left\{6 \right\}} | ||
\hat{\theta} | 0.48126 ^{\left\{8 \right\}} | 0.36089 ^{\left\{2 \right\}} | 0.38260 ^{\left\{5 \right\}} | 0.39633 ^{\left\{6 \right\}} | 0.15279 ^{\left\{1 \right\}} | 0.36296 ^{\left\{3 \right\}} | 0.36362 ^{\left\{4 \right\}} | 0.40027 ^{\left\{7 \right\}} | ||
\sum{Ranks} | {\textbf{77}} ^{\left\{8 \right\}} | {\textbf{47}} ^{\left\{3 \right\}} | {\textbf{57}} ^{\left\{4 \right\}} | {\textbf{71}} ^{\left\{7 \right\}} | {\textbf{12}} ^{\left\{1 \right\}} | {\textbf{59}} ^{\left\{5 \right\}} | {\textbf{39}} ^{\left\{2 \right\}} | {\textbf{70}} ^{\left\{6 \right\}} | ||
200 | |BIAS| | \hat{\vartheta} | 1.18071 ^{\left\{8 \right\}} | 1.13329 ^{\left\{4 \right\}} | 1.15680 ^{\left\{6 \right\}} | 1.15101 ^{\left\{5 \right\}} | 0.12881 ^{\left\{1 \right\}} | 1.09310 ^{\left\{2 \right\}} | 1.11947 ^{\left\{3 \right\}} | 1.17399 ^{\left\{7 \right\}} |
\hat{\varphi} | 0.21288 ^{\left\{2 \right\}} | 0.27282 ^{\left\{6 \right\}} | 0.22827 ^{\left\{4 \right\}} | 0.28207 ^{\left\{7 \right\}} | 0.16810 ^{\left\{1 \right\}} | 0.31936 ^{\left\{8 \right\}} | 0.21687 ^{\left\{3 \right\}} | 0.23691 ^{\left\{5 \right\}} | ||
\hat{\lambda} | 0.05455 ^{\left\{5 \right\}} | 0.05483 ^{\left\{6 \right\}} | 0.05139 ^{\left\{4 \right\}} | 0.05648 ^{\left\{8 \right\}} | 0.02373 ^{\left\{1 \right\}} | 0.04586 ^{\left\{2 \right\}} | 0.05000 ^{\left\{3 \right\}} | 0.05607 ^{\left\{7 \right\}} | ||
\hat{\theta} | 0.50995 ^{\left\{8 \right\}} | 0.46810 ^{\left\{5 \right\}} | 0.45161 ^{\left\{4 \right\}} | 0.48892 ^{\left\{7 \right\}} | 0.11088 ^{\left\{1 \right\}} | 0.36948 ^{\left\{2 \right\}} | 0.42847 ^{\left\{3 \right\}} | 0.48519 ^{\left\{6 \right\}} | ||
MSEs | \hat{\vartheta} | 1.65210 ^{\left\{6 \right\}} | 1.59933 ^{\left\{5 \right\}} | 1.54359 ^{\left\{4 \right\}} | 1.65457 ^{\left\{7 \right\}} | 0.11230 ^{\left\{1 \right\}} | 1.41356 ^{\left\{2 \right\}} | 1.45126 ^{\left\{3 \right\}} | 1.65710 ^{\left\{8 \right\}} | |
\hat{\varphi} | 0.06995 ^{\left\{2 \right\}} | 0.11564 ^{\left\{6 \right\}} | 0.08167 ^{\left\{4 \right\}} | 0.12209 ^{\left\{7 \right\}} | 0.04607 ^{\left\{1 \right\}} | 0.16683 ^{\left\{8 \right\}} | 0.07397 ^{\left\{3 \right\}} | 0.08772 ^{\left\{5 \right\}} | ||
\hat{\lambda} | 0.00441 ^{\left\{5 \right\}} | 0.00457 ^{\left\{6 \right\}} | 0.00403 ^{\left\{4 \right\}} | 0.00483 ^{\left\{8 \right\}} | 0.00088 ^{\left\{1 \right\}} | 0.00341 ^{\left\{2 \right\}} | 0.00386 ^{\left\{3 \right\}} | 0.00477 ^{\left\{7 \right\}} | ||
\hat{\theta} | 0.38578 ^{\left\{8 \right\}} | 0.35447 ^{\left\{5 \right\}} | 0.32071 ^{\left\{4 \right\}} | 0.38130 ^{\left\{7 \right\}} | 0.02076 ^{\left\{1 \right\}} | 0.24604 ^{\left\{2 \right\}} | 0.29346 ^{\left\{3 \right\}} | 0.38069 ^{\left\{6 \right\}} | ||
MREs | \hat{\vartheta} | 0.33734 ^{\left\{8 \right\}} | 0.32380 ^{\left\{4 \right\}} | 0.33052 ^{\left\{6 \right\}} | 0.32886 ^{\left\{5 \right\}} | 0.03680 ^{\left\{1 \right\}} | 0.31232 ^{\left\{2 \right\}} | 0.31985 ^{\left\{3 \right\}} | 0.33543 ^{\left\{7 \right\}} | |
\hat{\varphi} | 0.28384 ^{\left\{2 \right\}} | 0.36376 ^{\left\{6 \right\}} | 0.30437 ^{\left\{4 \right\}} | 0.37610 ^{\left\{7 \right\}} | 0.22413 ^{\left\{1 \right\}} | 0.42582 ^{\left\{8 \right\}} | 0.28917 ^{\left\{3 \right\}} | 0.31587 ^{\left\{5 \right\}} | ||
\hat{\lambda} | 0.10910 ^{\left\{5 \right\}} | 0.10967 ^{\left\{6 \right\}} | 0.10278 ^{\left\{4 \right\}} | 0.11295 ^{\left\{8 \right\}} | 0.04745 ^{\left\{1 \right\}} | 0.09173 ^{\left\{2 \right\}} | 0.10000 ^{\left\{3 \right\}} | 0.11214 ^{\left\{7 \right\}} | ||
\hat{\theta} | 0.40796 ^{\left\{8 \right\}} | 0.37448 ^{\left\{5 \right\}} | 0.36129 ^{\left\{4 \right\}} | 0.39114 ^{\left\{7 \right\}} | 0.08871 ^{\left\{1 \right\}} | 0.29559 ^{\left\{2 \right\}} | 0.34277 ^{\left\{3 \right\}} | 0.38815 ^{\left\{6 \right\}} | ||
\sum{Ranks} | {\textbf{67}} ^{\left\{6 \right\}} | {\textbf{64}} ^{\left\{5 \right\}} | {\textbf{52}} ^{\left\{4 \right\}} | {\textbf{83}} ^{\left\{8 \right\}} | {\textbf{12}} ^{\left\{1 \right\}} | {\textbf{42}} ^{\left\{3 \right\}} | {\textbf{36}} ^{\left\{2 \right\}} | {\textbf{76}} ^{\left\{7 \right\}} | ||
500 | |BIAS| | \hat{\vartheta} | 0.99609 ^{\left\{2 \right\}} | 1.12481 ^{\left\{6 \right\}} | 1.05940 ^{\left\{5 \right\}} | 1.12860 ^{\left\{7 \right\}} | 0.04256 ^{\left\{1 \right\}} | 1.04542 ^{\left\{4 \right\}} | 1.03887 ^{\left\{3 \right\}} | 1.14079 ^{\left\{8 \right\}} |
\hat{\varphi} | 0.14496 ^{\left\{2 \right\}} | 0.18941 ^{\left\{6 \right\}} | 0.15844 ^{\left\{4 \right\}} | 0.19306 ^{\left\{7 \right\}} | 0.10411 ^{\left\{1 \right\}} | 0.23525 ^{\left\{8 \right\}} | 0.15349 ^{\left\{3 \right\}} | 0.16453 ^{\left\{5 \right\}} | ||
\hat{\lambda} | 0.04309 ^{\left\{3 \right\}} | 0.05010 ^{\left\{7 \right\}} | 0.04425 ^{\left\{5 \right\}} | 0.04998 ^{\left\{6 \right\}} | 0.01474 ^{\left\{1 \right\}} | 0.03603 ^{\left\{2 \right\}} | 0.04334 ^{\left\{4 \right\}} | 0.05109 ^{\left\{8 \right\}} | ||
\hat{\theta} | 0.38900 ^{\left\{4 \right\}} | 0.44670 ^{\left\{6 \right\}} | 0.39875 ^{\left\{5 \right\}} | 0.45266 ^{\left\{7 \right\}} | 0.06374 ^{\left\{1 \right\}} | 0.31824 ^{\left\{2 \right\}} | 0.38560 ^{\left\{3 \right\}} | 0.45867 ^{\left\{8 \right\}} | ||
MSEs | \hat{\vartheta} | 1.21294 ^{\left\{2 \right\}} | 1.50352 ^{\left\{6 \right\}} | 1.31440 ^{\left\{5 \right\}} | 1.52047 ^{\left\{8 \right\}} | 0.02893 ^{\left\{1 \right\}} | 1.25338 ^{\left\{3 \right\}} | 1.26686 ^{\left\{4 \right\}} | 1.51668 ^{\left\{7 \right\}} | |
\hat{\varphi} | 0.03263 ^{\left\{2 \right\}} | 0.05612 ^{\left\{6 \right\}} | 0.03902 ^{\left\{4 \right\}} | 0.05818 ^{\left\{7 \right\}} | 0.01736 ^{\left\{1 \right\}} | 0.09221 ^{\left\{8 \right\}} | 0.03639 ^{\left\{3 \right\}} | 0.04120 ^{\left\{5 \right\}} | ||
\hat{\lambda} | 0.00278 ^{\left\{3 \right\}} | 0.00378 ^{\left\{6.5 \right\}} | 0.00295 ^{\left\{5 \right\}} | 0.00378 ^{\left\{6.5 \right\}} | 0.00034 ^{\left\{1 \right\}} | 0.00220 ^{\left\{2 \right\}} | 0.00283 ^{\left\{4 \right\}} | 0.00400 ^{\left\{8 \right\}} | ||
\hat{\theta} | 0.23678 ^{\left\{4 \right\}} | 0.31580 ^{\left\{6 \right\}} | 0.25000 ^{\left\{5 \right\}} | 0.32430 ^{\left\{7 \right\}} | 0.00703 ^{\left\{1 \right\}} | 0.18536 ^{\left\{2 \right\}} | 0.23280 ^{\left\{3 \right\}} | 0.33477 ^{\left\{8 \right\}} | ||
MREs | \hat{\vartheta} | 0.28460 ^{\left\{2 \right\}} | 0.32137 ^{\left\{6 \right\}} | 0.30269 ^{\left\{5 \right\}} | 0.32246 ^{\left\{7 \right\}} | 0.01216 ^{\left\{1 \right\}} | 0.29869 ^{\left\{4 \right\}} | 0.29682 ^{\left\{3 \right\}} | 0.32594 ^{\left\{8 \right\}} | |
\hat{\varphi} | 0.19328 ^{\left\{2 \right\}} | 0.25255 ^{\left\{6 \right\}} | 0.21126 ^{\left\{4 \right\}} | 0.25741 ^{\left\{7 \right\}} | 0.13881 ^{\left\{1 \right\}} | 0.31367 ^{\left\{8 \right\}} | 0.20466 ^{\left\{3 \right\}} | 0.21938 ^{\left\{5 \right\}} | ||
\hat{\lambda} | 0.08617 ^{\left\{3 \right\}} | 0.10020 ^{\left\{7 \right\}} | 0.08850 ^{\left\{5 \right\}} | 0.09997 ^{\left\{6 \right\}} | 0.02948 ^{\left\{1 \right\}} | 0.07207 ^{\left\{2 \right\}} | 0.08669 ^{\left\{4 \right\}} | 0.10217 ^{\left\{8 \right\}} | ||
\hat{\theta} | 0.31120 ^{\left\{4 \right\}} | 0.35736 ^{\left\{6 \right\}} | 0.31900 ^{\left\{5 \right\}} | 0.36213 ^{\left\{7 \right\}} | 0.05100 ^{\left\{1 \right\}} | 0.25459 ^{\left\{2 \right\}} | 0.30848 ^{\left\{3 \right\}} | 0.36694 ^{\left\{8 \right\}} | ||
\sum{Ranks} | {\textbf{33}} ^{\left\{2 \right\}} | {\textbf{74.5}} ^{\left\{6 \right\}} | {\textbf{57}} ^{\left\{5 \right\}} | {\textbf{82.5}} ^{\left\{7 \right\}} | {\textbf{12}} ^{\left\{1 \right\}} | {\textbf{47}} ^{\left\{4 \right\}} | {\textbf{40}} ^{\left\{3 \right\}} | {\textbf{86}} ^{\left\{8 \right\}} |
n | Est. | Est. Par. | MLEs | LSEs | WLSEs | CRVMEs | MPSEs | PCEs | ADEs | RADEs |
20 | |BIAS| | \hat{\vartheta} | 0.98923 ^{\left\{7 \right\}} | 0.64331 ^{\left\{3 \right\}} | 0.95672 ^{\left\{6 \right\}} | 0.63413 ^{\left\{2 \right\}} | 0.44818 ^{\left\{1 \right\}} | 1.04755 ^{\left\{8 \right\}} | 0.90857 ^{\left\{5 \right\}} | 0.78916 ^{\left\{4 \right\}} |
\hat{\varphi} | 0.95367 ^{\left\{6 \right\}} | 0.90318 ^{\left\{4 \right\}} | 0.88553 ^{\left\{3 \right\}} | 0.95450 ^{\left\{7 \right\}} | 0.31253 ^{\left\{1 \right\}} | 0.97463 ^{\left\{8 \right\}} | 0.85825 ^{\left\{2 \right\}} | 0.93652 ^{\left\{5 \right\}} | ||
\hat{\lambda} | 0.50387 ^{\left\{8 \right\}} | 0.29370 ^{\left\{2 \right\}} | 0.38814 ^{\left\{5 \right\}} | 0.31558 ^{\left\{3 \right\}} | 0.23477 ^{\left\{1 \right\}} | 0.41787 ^{\left\{7 \right\}} | 0.38863 ^{\left\{6 \right\}} | 0.36144 ^{\left\{4 \right\}} | ||
\hat{\theta} | 0.83605 ^{\left\{8 \right\}} | 0.71444 ^{\left\{3 \right\}} | 0.81238 ^{\left\{7 \right\}} | 0.68991 ^{\left\{2 \right\}} | 0.62151 ^{\left\{1 \right\}} | 0.74404 ^{\left\{5 \right\}} | 0.75604 ^{\left\{6 \right\}} | 0.71561 ^{\left\{4 \right\}} | ||
MSEs | \hat{\vartheta} | 1.35323 ^{\left\{7 \right\}} | 0.71389 ^{\left\{2 \right\}} | 1.18225 ^{\left\{6 \right\}} | 0.73718 ^{\left\{3 \right\}} | 0.37586 ^{\left\{1 \right\}} | 1.57224 ^{\left\{8 \right\}} | 1.13154 ^{\left\{5 \right\}} | 1.02701 ^{\left\{4 \right\}} | |
\hat{\varphi} | 1.18857 ^{\left\{8 \right\}} | 1.06788 ^{\left\{4 \right\}} | 1.02750 ^{\left\{3 \right\}} | 1.18599 ^{\left\{7 \right\}} | 0.87512 ^{\left\{1 \right\}} | 1.15778 ^{\left\{6 \right\}} | 0.96462 ^{\left\{2 \right\}} | 1.13400 ^{\left\{5 \right\}} | ||
\hat{\lambda} | 0.36432 ^{\left\{8 \right\}} | 0.14714 ^{\left\{2 \right\}} | 0.22539 ^{\left\{5 \right\}} | 0.17712 ^{\left\{3 \right\}} | 0.09172 ^{\left\{1 \right\}} | 0.28638 ^{\left\{7 \right\}} | 0.23923 ^{\left\{6 \right\}} | 0.22267 ^{\left\{4 \right\}} | ||
\hat{\theta} | 0.77865 ^{\left\{8 \right\}} | 0.64926 ^{\left\{4 \right\}} | 0.77561 ^{\left\{7 \right\}} | 0.60519 ^{\left\{2 \right\}} | 0.52925 ^{\left\{1 \right\}} | 0.67762 ^{\left\{5 \right\}} | 0.68866 ^{\left\{6 \right\}} | 0.64046 ^{\left\{3 \right\}} | ||
MREs | \hat{\vartheta} | 0.28264 ^{\left\{7 \right\}} | 0.18380 ^{\left\{3 \right\}} | 0.27335 ^{\left\{6 \right\}} | 0.18118 ^{\left\{2 \right\}} | 0.12805 ^{\left\{1 \right\}} | 0.29930 ^{\left\{8 \right\}} | 0.25959 ^{\left\{5 \right\}} | 0.22547 ^{\left\{4 \right\}} | |
\hat{\varphi} | 0.47683 ^{\left\{6 \right\}} | 0.45159 ^{\left\{4 \right\}} | 0.44276 ^{\left\{3 \right\}} | 0.47725 ^{\left\{7 \right\}} | 0.40539 ^{\left\{1 \right\}} | 0.48731 ^{\left\{8 \right\}} | 0.42912 ^{\left\{2 \right\}} | 0.46826 ^{\left\{5 \right\}} | ||
\hat{\lambda} | 0.33591 ^{\left\{8 \right\}} | 0.19580 ^{\left\{2 \right\}} | 0.25876 ^{\left\{5 \right\}} | 0.21038 ^{\left\{3 \right\}} | 0.15651 ^{\left\{1 \right\}} | 0.27858 ^{\left\{7 \right\}} | 0.25908 ^{\left\{6 \right\}} | 0.24096 ^{\left\{4 \right\}} | ||
\hat{\theta} | 0.33442 ^{\left\{8 \right\}} | 0.28578 ^{\left\{3 \right\}} | 0.32495 ^{\left\{7 \right\}} | 0.27596 ^{\left\{2 \right\}} | 0.24860 ^{\left\{1 \right\}} | 0.29762 ^{\left\{5 \right\}} | 0.30242 ^{\left\{6 \right\}} | 0.28624 ^{\left\{4 \right\}} | ||
\sum{Ranks} | {\textbf{89}} ^{\left\{8 \right\}} | {\textbf{36}} ^{\left\{2 \right\}} | {\textbf{63}} ^{\left\{6 \right\}} | {\textbf{43}} ^{\left\{3 \right\}} | {\textbf{12}} ^{\left\{1 \right\}} | {\textbf{82}} ^{\left\{7 \right\}} | {\textbf{57}} ^{\left\{5 \right\}} | {\textbf{50}} ^{\left\{4 \right\}} | ||
80 | |BIAS| | \hat{\vartheta} | 1.00512 ^{\left\{8 \right\}} | 0.76116 ^{\left\{3 \right\}} | 0.98814 ^{\left\{7 \right\}} | 0.76068 ^{\left\{2 \right\}} | 0.22490 ^{\left\{1 \right\}} | 0.94177 ^{\left\{5 \right\}} | 0.95845 ^{\left\{6 \right\}} | 0.83406 ^{\left\{4 \right\}} |
\hat{\varphi} | 0.56247 ^{\left\{3 \right\}} | 0.64453 ^{\left\{6 \right\}} | 0.57353 ^{\left\{4 \right\}} | 0.64522 ^{\left\{7 \right\}} | 0.46925 ^{\left\{1 \right\}} | 0.76277 ^{\left\{8 \right\}} | 0.55915 ^{\left\{2 \right\}} | 0.63949 ^{\left\{5 \right\}} | ||
\hat{\lambda} | 0.45485 ^{\left\{8 \right\}} | 0.31115 ^{\left\{2 \right\}} | 0.36895 ^{\left\{6 \right\}} | 0.33000 ^{\left\{3 \right\}} | 0.11306 ^{\left\{1 \right\}} | 0.39112 ^{\left\{7 \right\}} | 0.36093 ^{\left\{5 \right\}} | 0.34405 ^{\left\{4 \right\}} | ||
\hat{\theta} | 0.76702 ^{\left\{8 \right\}} | 0.62063 ^{\left\{3 \right\}} | 0.68635 ^{\left\{7 \right\}} | 0.62598 ^{\left\{4 \right\}} | 0.33066 ^{\left\{1 \right\}} | 0.60384 ^{\left\{2 \right\}} | 0.66063 ^{\left\{6 \right\}} | 0.63130 ^{\left\{5 \right\}} | ||
MSEs | \hat{\vartheta} | 1.24539 ^{\left\{7 \right\}} | 0.92842 ^{\left\{2 \right\}} | 1.16588 ^{\left\{6 \right\}} | 0.94580 ^{\left\{3 \right\}} | 0.13919 ^{\left\{1 \right\}} | 1.25646 ^{\left\{8 \right\}} | 1.12647 ^{\left\{5 \right\}} | 1.05555 ^{\left\{4 \right\}} | |
\hat{\varphi} | 0.45689 ^{\left\{3 \right\}} | 0.57098 ^{\left\{6 \right\}} | 0.46410 ^{\left\{4 \right\}} | 0.57244 ^{\left\{7 \right\}} | 0.32482 ^{\left\{1 \right\}} | 0.71835 ^{\left\{8 \right\}} | 0.44181 ^{\left\{2 \right\}} | 0.55971 ^{\left\{5 \right\}} | ||
\hat{\lambda} | 0.28629 ^{\left\{8 \right\}} | 0.17260 ^{\left\{2 \right\}} | 0.20504 ^{\left\{5 \right\}} | 0.19582 ^{\left\{3 \right\}} | 0.02220 ^{\left\{1 \right\}} | 0.25122 ^{\left\{7 \right\}} | 0.20232 ^{\left\{4 \right\}} | 0.20735 ^{\left\{6 \right\}} | ||
\hat{\theta} | 0.68706 ^{\left\{8 \right\}} | 0.51170 ^{\left\{3 \right\}} | 0.58072 ^{\left\{7 \right\}} | 0.52071 ^{\left\{4 \right\}} | 0.16747 ^{\left\{1 \right\}} | 0.49400 ^{\left\{2 \right\}} | 0.55280 ^{\left\{6 \right\}} | 0.52733 ^{\left\{5 \right\}} | ||
MREs | \hat{\vartheta} | 0.28718 ^{\left\{8 \right\}} | 0.21747 ^{\left\{3 \right\}} | 0.28233 ^{\left\{7 \right\}} | 0.21734 ^{\left\{2 \right\}} | 0.06426 ^{\left\{1 \right\}} | 0.26908 ^{\left\{5 \right\}} | 0.27384 ^{\left\{6 \right\}} | 0.23830 ^{\left\{4 \right\}} | |
\hat{\varphi} | 0.28123 ^{\left\{3 \right\}} | 0.32226 ^{\left\{6 \right\}} | 0.28677 ^{\left\{4 \right\}} | 0.32261 ^{\left\{7 \right\}} | 0.23462 ^{\left\{1 \right\}} | 0.38138 ^{\left\{8 \right\}} | 0.27957 ^{\left\{2 \right\}} | 0.31974 ^{\left\{5 \right\}} | ||
\hat{\lambda} | 0.30323 ^{\left\{8 \right\}} | 0.20744 ^{\left\{2 \right\}} | 0.24597 ^{\left\{6 \right\}} | 0.22000 ^{\left\{3 \right\}} | 0.07538 ^{\left\{1 \right\}} | 0.26075 ^{\left\{7 \right\}} | 0.24062 ^{\left\{5 \right\}} | 0.22937 ^{\left\{4 \right\}} | ||
\hat{\theta} | 0.30681 ^{\left\{8 \right\}} | 0.24825 ^{\left\{3 \right\}} | 0.27454 ^{\left\{7 \right\}} | 0.25039 ^{\left\{4 \right\}} | 0.13226 ^{\left\{1 \right\}} | 0.24153 ^{\left\{2 \right\}} | 0.26425 ^{\left\{6 \right\}} | 0.25252 ^{\left\{5 \right\}} | ||
\sum{Ranks} | {\textbf{80}} ^{\left\{8 \right\}} | {\textbf{41}} ^{\left\{2 \right\}} | {\textbf{70}} ^{\left\{7 \right\}} | {\textbf{49}} ^{\left\{3 \right\}} | {\textbf{12}} ^{\left\{1 \right\}} | {\textbf{69}} ^{\left\{6 \right\}} | {\textbf{55}} ^{\left\{4 \right\}} | {\textbf{56}} ^{\left\{5 \right\}} | ||
200 | |BIAS| | \hat{\vartheta} | 0.98460 ^{\left\{6 \right\}} | 0.86500 ^{\left\{3 \right\}} | 1.00674 ^{\left\{7 \right\}} | 0.86298 ^{\left\{2 \right\}} | 0.12154 ^{\left\{1 \right\}} | 1.05269 ^{\left\{8 \right\}} | 0.98185 ^{\left\{5 \right\}} | 0.89235 ^{\left\{4 \right\}} |
\hat{\varphi} | 0.38859 ^{\left\{2 \right\}} | 0.45911 ^{\left\{5 \right\}} | 0.41129 ^{\left\{4 \right\}} | 0.46722 ^{\left\{6 \right\}} | 0.31253 ^{\left\{1 \right\}} | 0.67732 ^{\left\{8 \right\}} | 0.40329 ^{\left\{3 \right\}} | 0.46920 ^{\left\{7 \right\}} | ||
\hat{\lambda} | 0.39873 ^{\left\{8 \right\}} | 0.31023 ^{\left\{2 \right\}} | 0.35370 ^{\left\{6 \right\}} | 0.32546 ^{\left\{3 \right\}} | 0.06881 ^{\left\{1 \right\}} | 0.37938 ^{\left\{7 \right\}} | 0.34126 ^{\left\{5 \right\}} | 0.32900 ^{\left\{4 \right\}} | ||
\hat{\theta} | 0.68985 ^{\left\{8 \right\}} | 0.57483 ^{\left\{3 \right\}} | 0.64129 ^{\left\{7 \right\}} | 0.59868 ^{\left\{5 \right\}} | 0.21029 ^{\left\{1 \right\}} | 0.55195 ^{\left\{2 \right\}} | 0.61763 ^{\left\{6 \right\}} | 0.59808 ^{\left\{4 \right\}} | ||
MSEs | \hat{\vartheta} | 1.11626 ^{\left\{6 \right\}} | 1.01791 ^{\left\{2 \right\}} | 1.13048 ^{\left\{7 \right\}} | 1.02064 ^{\left\{3 \right\}} | 0.05498 ^{\left\{1 \right\}} | 1.30905 ^{\left\{8 \right\}} | 1.09868 ^{\left\{5 \right\}} | 1.05550 ^{\left\{4 \right\}} | |
\hat{\varphi} | 0.23053 ^{\left\{2 \right\}} | 0.31234 ^{\left\{5 \right\}} | 0.25573 ^{\left\{4 \right\}} | 0.32276 ^{\left\{6 \right\}} | 0.15678 ^{\left\{1 \right\}} | 0.58268 ^{\left\{8 \right\}} | 0.24649 ^{\left\{3 \right\}} | 0.32421 ^{\left\{7 \right\}} | ||
\hat{\lambda} | 0.22103 ^{\left\{7 \right\}} | 0.16583 ^{\left\{2 \right\}} | 0.18036 ^{\left\{5 \right\}} | 0.17985 ^{\left\{4 \right\}} | 0.00837 ^{\left\{1 \right\}} | 0.22479 ^{\left\{8 \right\}} | 0.17270 ^{\left\{3 \right\}} | 0.18259 ^{\left\{6 \right\}} | ||
\hat{\theta} | 0.58585 ^{\left\{8 \right\}} | 0.45832 ^{\left\{3 \right\}} | 0.51809 ^{\left\{7 \right\}} | 0.48976 ^{\left\{5 \right\}} | 0.07171 ^{\left\{1 \right\}} | 0.44322 ^{\left\{2 \right\}} | 0.49209 ^{\left\{6 \right\}} | 0.48968 ^{\left\{4 \right\}} | ||
MREs | \hat{\vartheta} | 0.28132 ^{\left\{6 \right\}} | 0.24714 ^{\left\{3 \right\}} | 0.28764 ^{\left\{7 \right\}} | 0.24657 ^{\left\{2 \right\}} | 0.03472 ^{\left\{1 \right\}} | 0.30077 ^{\left\{8 \right\}} | 0.28053 ^{\left\{5 \right\}} | 0.25496 ^{\left\{4 \right\}} | |
\hat{\varphi} | 0.19429 ^{\left\{2 \right\}} | 0.22956 ^{\left\{5 \right\}} | 0.20564 ^{\left\{4 \right\}} | 0.23361 ^{\left\{6 \right\}} | 0.15627 ^{\left\{1 \right\}} | 0.33866 ^{\left\{8 \right\}} | 0.20165 ^{\left\{3 \right\}} | 0.23460 ^{\left\{7 \right\}} | ||
\hat{\lambda} | 0.26582 ^{\left\{8 \right\}} | 0.20682 ^{\left\{2 \right\}} | 0.23580 ^{\left\{6 \right\}} | 0.21697 ^{\left\{3 \right\}} | 0.04587 ^{\left\{1 \right\}} | 0.25292 ^{\left\{7 \right\}} | 0.22751 ^{\left\{5 \right\}} | 0.21933 ^{\left\{4 \right\}} | ||
\hat{\theta} | 0.27594 ^{\left\{8 \right\}} | 0.22993 ^{\left\{3 \right\}} | 0.25652 ^{\left\{7 \right\}} | 0.23947 ^{\left\{5 \right\}} | 0.08412 ^{\left\{1 \right\}} | 0.22078 ^{\left\{2 \right\}} | 0.24705 ^{\left\{6 \right\}} | 0.23923 ^{\left\{4 \right\}} | ||
\sum{Ranks} | {\textbf{71}} ^{\left\{6.5 \right\}} | {\textbf{38}} ^{\left\{2 \right\}} | {\textbf{71}} ^{\left\{6.5 \right\}} | {\textbf{50}} ^{\left\{3 \right\}} | {\textbf{12}} ^{\left\{1 \right\}} | {\textbf{76}} ^{\left\{8 \right\}} | {\textbf{55}} ^{\left\{4 \right\}} | {\textbf{59}} ^{\left\{5 \right\}} | ||
500 | |BIAS| | \hat{\vartheta} | 0.93304 ^{\left\{5 \right\}} | 0.93263 ^{\left\{4 \right\}} | 0.97539 ^{\left\{7 \right\}} | 0.92961 ^{\left\{3 \right\}} | 0.05348 ^{\left\{1 \right\}} | 1.08240 ^{\left\{8 \right\}} | 0.95522 ^{\left\{6 \right\}} | 0.92527 ^{\left\{2 \right\}} |
\hat{\varphi} | 0.27572 ^{\left\{2 \right\}} | 0.32936 ^{\left\{5 \right\}} | 0.29210 ^{\left\{4 \right\}} | 0.33160 ^{\left\{6 \right\}} | 0.19113 ^{\left\{1 \right\}} | 0.58412 ^{\left\{8 \right\}} | 0.28766 ^{\left\{3 \right\}} | 0.33820 ^{\left\{7 \right\}} | ||
\hat{\lambda} | 0.34882 ^{\left\{7 \right\}} | 0.31976 ^{\left\{2 \right\}} | 0.33838 ^{\left\{6 \right\}} | 0.33117 ^{\left\{5 \right\}} | 0.03908 ^{\left\{1 \right\}} | 0.35124 ^{\left\{8 \right\}} | 0.32736 ^{\left\{3 \right\}} | 0.32969 ^{\left\{4 \right\}} | ||
\hat{\theta} | 0.61892 ^{\left\{8 \right\}} | 0.57580 ^{\left\{3 \right\}} | 0.60923 ^{\left\{7 \right\}} | 0.59341 ^{\left\{6 \right\}} | 0.12272 ^{\left\{1 \right\}} | 0.51040 ^{\left\{2 \right\}} | 0.59246 ^{\left\{5 \right\}} | 0.58416 ^{\left\{4 \right\}} | ||
MSEs | \hat{\vartheta} | 0.99386 ^{\left\{2 \right\}} | 1.04383 ^{\left\{6 \right\}} | 1.04849 ^{\left\{7 \right\}} | 1.04352 ^{\left\{5 \right\}} | 0.00732 ^{\left\{1 \right\}} | 1.28318 ^{\left\{8 \right\}} | 1.01861 ^{\left\{3 \right\}} | 1.03056 ^{\left\{4 \right\}} | |
\hat{\varphi} | 0.11591 ^{\left\{2 \right\}} | 0.16651 ^{\left\{5 \right\}} | 0.13079 ^{\left\{4 \right\}} | 0.16807 ^{\left\{6 \right\}} | 0.06394 ^{\left\{1 \right\}} | 0.45248 ^{\left\{8 \right\}} | 0.12739 ^{\left\{3 \right\}} | 0.17250 ^{\left\{7 \right\}} | ||
\hat{\lambda} | 0.17000 ^{\left\{7 \right\}} | 0.15789 ^{\left\{3 \right\}} | 0.15993 ^{\left\{4 \right\}} | 0.16663 ^{\left\{5 \right\}} | 0.00250 ^{\left\{1 \right\}} | 0.18698 ^{\left\{8 \right\}} | 0.15186 ^{\left\{2 \right\}} | 0.16915 ^{\left\{6 \right\}} | ||
\hat{\theta} | 0.48714 ^{\left\{8 \right\}} | 0.45146 ^{\left\{4 \right\}} | 0.47250 ^{\left\{6 \right\}} | 0.47497 ^{\left\{7 \right\}} | 0.02504 ^{\left\{1 \right\}} | 0.39915 ^{\left\{2 \right\}} | 0.45105 ^{\left\{3 \right\}} | 0.47078 ^{\left\{5 \right\}} | ||
MREs | \hat{\vartheta} | 0.26658 ^{\left\{5 \right\}} | 0.26647 ^{\left\{4 \right\}} | 0.27868 ^{\left\{7 \right\}} | 0.26560 ^{\left\{3 \right\}} | 0.01528 ^{\left\{1 \right\}} | 0.30926 ^{\left\{8 \right\}} | 0.27292 ^{\left\{6 \right\}} | 0.26436 ^{\left\{2 \right\}} | |
\hat{\varphi} | 0.13786 ^{\left\{2 \right\}} | 0.16468 ^{\left\{5 \right\}} | 0.14605 ^{\left\{4 \right\}} | 0.16580 ^{\left\{6 \right\}} | 0.09557 ^{\left\{1 \right\}} | 0.29206 ^{\left\{8 \right\}} | 0.14383 ^{\left\{3 \right\}} | 0.16910 ^{\left\{7 \right\}} | ||
\hat{\lambda} | 0.23255 ^{\left\{7 \right\}} | 0.21318 ^{\left\{2 \right\}} | 0.22559 ^{\left\{6 \right\}} | 0.22078 ^{\left\{5 \right\}} | 0.02606 ^{\left\{1 \right\}} | 0.23416 ^{\left\{8 \right\}} | 0.21824 ^{\left\{3 \right\}} | 0.21980 ^{\left\{4 \right\}} | ||
\hat{\theta} | 0.24757 ^{\left\{8 \right\}} | 0.23032 ^{\left\{3 \right\}} | 0.24369 ^{\left\{7 \right\}} | 0.23736 ^{\left\{6 \right\}} | 0.04909 ^{\left\{1 \right\}} | 0.20416 ^{\left\{2 \right\}} | 0.23698 ^{\left\{5 \right\}} | 0.23367 ^{\left\{4 \right\}} | ||
\sum{Ranks} | {\textbf{63}} ^{\left\{5.5 \right\}} | {\textbf{46}} ^{\left\{3 \right\}} | {\textbf{69}} ^{\left\{7 \right\}} | {\textbf{63}} ^{\left\{5.5 \right\}} | {\textbf{12}} ^{\left\{1 \right\}} | {\textbf{78}} ^{\left\{8 \right\}} | {\textbf{45}} ^{\left\{2 \right\}} | {\textbf{56}} ^{\left\{4 \right\}} |
n | Est. | Est. Par. | MLEs | LSEs | WLSEs | CRVMEs | MPSEs | PCEs | ADEs | RADEs |
20 | |BIAS| | \hat{\vartheta} | 1.25197 ^{\left\{8 \right\}} | 0.45438 ^{\left\{3 \right\}} | 1.06521 ^{\left\{7 \right\}} | 0.44888 ^{\left\{2 \right\}} | 0.20680 ^{\left\{1 \right\}} | 0.83805 ^{\left\{5 \right\}} | 1.02528 ^{\left\{6 \right\}} | 0.62513 ^{\left\{4 \right\}} |
\hat{\varphi} | 0.58123 ^{\left\{5 \right\}} | 0.60203 ^{\left\{7 \right\}} | 0.57995 ^{\left\{4 \right\}} | 0.61278 ^{\left\{8 \right\}} | 0.16575 ^{\left\{1 \right\}} | 0.57749 ^{\left\{3 \right\}} | 0.53508 ^{\left\{2 \right\}} | 0.58165 ^{\left\{6 \right\}} | ||
\hat{\lambda} | 0.08854 ^{\left\{8 \right\}} | 0.07652 ^{\left\{2 \right\}} | 0.08227 ^{\left\{7 \right\}} | 0.07831 ^{\left\{3 \right\}} | 0.06474 ^{\left\{1 \right\}} | 0.08065 ^{\left\{6 \right\}} | 0.07939 ^{\left\{4 \right\}} | 0.07980 ^{\left\{5 \right\}} | ||
\hat{\theta} | 0.66759 ^{\left\{8 \right\}} | 0.42262 ^{\left\{2 \right\}} | 0.53041 ^{\left\{7 \right\}} | 0.44839 ^{\left\{3 \right\}} | 0.32466 ^{\left\{1 \right\}} | 0.51024 ^{\left\{5 \right\}} | 0.52797 ^{\left\{6 \right\}} | 0.48959 ^{\left\{4 \right\}} | ||
MSEs | \hat{\vartheta} | 2.41625 ^{\left\{8 \right\}} | 0.60512 ^{\left\{2 \right\}} | 1.67131 ^{\left\{6 \right\}} | 0.67908 ^{\left\{3 \right\}} | 0.18285 ^{\left\{1 \right\}} | 1.58289 ^{\left\{5 \right\}} | 1.74298 ^{\left\{7 \right\}} | 1.01407 ^{\left\{4 \right\}} | |
\hat{\varphi} | 0.42148 ^{\left\{3 \right\}} | 0.46192 ^{\left\{7 \right\}} | 0.43565 ^{\left\{5 \right\}} | 0.46874 ^{\left\{8 \right\}} | 0.38218 ^{\left\{1 \right\}} | 0.43432 ^{\left\{4 \right\}} | 0.38271 ^{\left\{2 \right\}} | 0.43679 ^{\left\{6 \right\}} | ||
\hat{\lambda} | 0.01072 ^{\left\{8 \right\}} | 0.00870 ^{\left\{2 \right\}} | 0.00963 ^{\left\{7 \right\}} | 0.00906 ^{\left\{3 \right\}} | 0.00620 ^{\left\{1 \right\}} | 0.00950 ^{\left\{6 \right\}} | 0.00923 ^{\left\{5 \right\}} | 0.00919 ^{\left\{4 \right\}} | ||
\hat{\theta} | 0.58894 ^{\left\{8 \right\}} | 0.28693 ^{\left\{2 \right\}} | 0.40885 ^{\left\{6 \right\}} | 0.32249 ^{\left\{3 \right\}} | 0.16767 ^{\left\{1 \right\}} | 0.38646 ^{\left\{5 \right\}} | 0.41599 ^{\left\{7 \right\}} | 0.36863 ^{\left\{4 \right\}} | ||
MREs | \hat{\vartheta} | 0.27821 ^{\left\{8 \right\}} | 0.10097 ^{\left\{3 \right\}} | 0.23671 ^{\left\{7 \right\}} | 0.09975 ^{\left\{2 \right\}} | 0.04596 ^{\left\{1 \right\}} | 0.18623 ^{\left\{5 \right\}} | 0.22784 ^{\left\{6 \right\}} | 0.13892 ^{\left\{4 \right\}} | |
\hat{\varphi} | 0.77497 ^{\left\{5 \right\}} | 0.80271 ^{\left\{7 \right\}} | 0.77327 ^{\left\{4 \right\}} | 0.81704 ^{\left\{8 \right\}} | 0.70903 ^{\left\{1 \right\}} | 0.76998 ^{\left\{3 \right\}} | 0.71344 ^{\left\{2 \right\}} | 0.77554 ^{\left\{6 \right\}} | ||
\hat{\lambda} | 0.17707 ^{\left\{8 \right\}} | 0.15303 ^{\left\{2 \right\}} | 0.16453 ^{\left\{7 \right\}} | 0.15662 ^{\left\{3 \right\}} | 0.12948 ^{\left\{1 \right\}} | 0.16130 ^{\left\{6 \right\}} | 0.15879 ^{\left\{4 \right\}} | 0.15960 ^{\left\{5 \right\}} | ||
\hat{\theta} | 0.53407 ^{\left\{8 \right\}} | 0.33809 ^{\left\{2 \right\}} | 0.42432 ^{\left\{7 \right\}} | 0.35871 ^{\left\{3 \right\}} | 0.25973 ^{\left\{1 \right\}} | 0.40819 ^{\left\{5 \right\}} | 0.42237 ^{\left\{6 \right\}} | 0.39167 ^{\left\{4 \right\}} | ||
\sum{Ranks} | {\textbf{85}} ^{\left\{8 \right\}} | {\textbf{41}} ^{\left\{2 \right\}} | {\textbf{74}} ^{\left\{7 \right\}} | {\textbf{49}} ^{\left\{3 \right\}} | {\textbf{12}} ^{\left\{1 \right\}} | {\textbf{58}} ^{\left\{6 \right\}} | {\textbf{57}} ^{\left\{5 \right\}} | {\textbf{56}} ^{\left\{4 \right\}} | ||
80 | |BIAS| | \hat{\vartheta} | 1.62748 ^{\left\{8 \right\}} | 0.91367 ^{\left\{2 \right\}} | 1.37823 ^{\left\{7 \right\}} | 0.98139 ^{\left\{3 \right\}} | 0.11224 ^{\left\{1 \right\}} | 1.13720 ^{\left\{5 \right\}} | 1.31803 ^{\left\{6 \right\}} | 1.11805 ^{\left\{4 \right\}} |
\hat{\varphi} | 0.31837 ^{\left\{3 \right\}} | 0.39968 ^{\left\{7 \right\}} | 0.34474 ^{\left\{4 \right\}} | 0.40380 ^{\left\{8 \right\}} | 0.27352 ^{\left\{1 \right\}} | 0.37466 ^{\left\{6 \right\}} | 0.31821 ^{\left\{2 \right\}} | 0.35079 ^{\left\{5 \right\}} | ||
\hat{\lambda} | 0.07169 ^{\left\{8 \right\}} | 0.05804 ^{\left\{3 \right\}} | 0.06253 ^{\left\{7 \right\}} | 0.06113 ^{\left\{6 \right\}} | 0.03286 ^{\left\{1 \right\}} | 0.05699 ^{\left\{2 \right\}} | 0.05980 ^{\left\{5 \right\}} | 0.05870 ^{\left\{4 \right\}} | ||
\hat{\theta} | 0.64853 ^{\left\{8 \right\}} | 0.39956 ^{\left\{2 \right\}} | 0.49396 ^{\left\{7 \right\}} | 0.44278 ^{\left\{4 \right\}} | 0.16292 ^{\left\{1 \right\}} | 0.43570 ^{\left\{3 \right\}} | 0.47512 ^{\left\{6 \right\}} | 0.45276 ^{\left\{5 \right\}} | ||
MSEs | \hat{\vartheta} | 3.14585 ^{\left\{8 \right\}} | 1.58782 ^{\left\{2 \right\}} | 2.33956 ^{\left\{7 \right\}} | 1.79431 ^{\left\{3 \right\}} | 0.08679 ^{\left\{1 \right\}} | 1.96177 ^{\left\{4 \right\}} | 2.22845 ^{\left\{6 \right\}} | 2.05141 ^{\left\{5 \right\}} | |
\hat{\varphi} | 0.15254 ^{\left\{2 \right\}} | 0.23761 ^{\left\{7 \right\}} | 0.18156 ^{\left\{4 \right\}} | 0.24031 ^{\left\{8 \right\}} | 0.12335 ^{\left\{1 \right\}} | 0.22013 ^{\left\{6 \right\}} | 0.15798 ^{\left\{3 \right\}} | 0.19064 ^{\left\{5 \right\}} | ||
\hat{\lambda} | 0.00707 ^{\left\{8 \right\}} | 0.00520 ^{\left\{4 \right\}} | 0.00579 ^{\left\{7 \right\}} | 0.00566 ^{\left\{6 \right\}} | 0.00171 ^{\left\{1 \right\}} | 0.00514 ^{\left\{2 \right\}} | 0.00541 ^{\left\{5 \right\}} | 0.00519 ^{\left\{3 \right\}} | ||
\hat{\theta} | 0.56658 ^{\left\{8 \right\}} | 0.28713 ^{\left\{2 \right\}} | 0.38674 ^{\left\{7 \right\}} | 0.34041 ^{\left\{4 \right\}} | 0.04197 ^{\left\{1 \right\}} | 0.32550 ^{\left\{3 \right\}} | 0.36722 ^{\left\{6 \right\}} | 0.35156 ^{\left\{5 \right\}} | ||
MREs | \hat{\vartheta} | 0.36166 ^{\left\{8 \right\}} | 0.20304 ^{\left\{2 \right\}} | 0.30627 ^{\left\{7 \right\}} | 0.21809 ^{\left\{3 \right\}} | 0.02494 ^{\left\{1 \right\}} | 0.25271 ^{\left\{5 \right\}} | 0.29289 ^{\left\{6 \right\}} | 0.24846 ^{\left\{4 \right\}} | |
\hat{\varphi} | 0.42449 ^{\left\{3 \right\}} | 0.53291 ^{\left\{7 \right\}} | 0.45966 ^{\left\{4 \right\}} | 0.53840 ^{\left\{8 \right\}} | 0.36469 ^{\left\{1 \right\}} | 0.49955 ^{\left\{6 \right\}} | 0.42429 ^{\left\{2 \right\}} | 0.46771 ^{\left\{5 \right\}} | ||
\hat{\lambda} | 0.14337 ^{\left\{8 \right\}} | 0.11609 ^{\left\{3 \right\}} | 0.12506 ^{\left\{7 \right\}} | 0.12226 ^{\left\{6 \right\}} | 0.06572 ^{\left\{1 \right\}} | 0.11399 ^{\left\{2 \right\}} | 0.11961 ^{\left\{5 \right\}} | 0.11740 ^{\left\{4 \right\}} | ||
\hat{\theta} | 0.51882 ^{\left\{8 \right\}} | 0.31965 ^{\left\{2 \right\}} | 0.39517 ^{\left\{7 \right\}} | 0.35422 ^{\left\{4 \right\}} | 0.13034 ^{\left\{1 \right\}} | 0.34856 ^{\left\{3 \right\}} | 0.38010 ^{\left\{6 \right\}} | 0.36221 ^{\left\{5 \right\}} | ||
\sum{Ranks} | {\textbf{80}} ^{\left\{8 \right\}} | {\textbf{43}} ^{\left\{2 \right\}} | {\textbf{75}} ^{\left\{7 \right\}} | {\textbf{63}} ^{\left\{6 \right\}} | {\textbf{12}} ^{\left\{1 \right\}} | {\textbf{47}} ^{\left\{3 \right\}} | {\textbf{58}} ^{\left\{5 \right\}} | {\textbf{54}} ^{\left\{4 \right\}} | ||
200 | |BIAS| | \hat{\vartheta} | 1.48139 ^{\left\{8 \right\}} | 1.17284 ^{\left\{2 \right\}} | 1.40391 ^{\left\{7 \right\}} | 1.21294 ^{\left\{4 \right\}} | 0.04307 ^{\left\{1 \right\}} | 1.17950 ^{\left\{3 \right\}} | 1.33404 ^{\left\{6 \right\}} | 1.29174 ^{\left\{5 \right\}} |
\hat{\varphi} | 0.20186 ^{\left\{2 \right\}} | 0.26830 ^{\left\{7 \right\}} | 0.21385 ^{\left\{4 \right\}} | 0.26575 ^{\left\{6 \right\}} | 0.16575 ^{\left\{1 \right\}} | 0.27408 ^{\left\{8 \right\}} | 0.20558 ^{\left\{3 \right\}} | 0.23166 ^{\left\{5 \right\}} | ||
\hat{\lambda} | 0.06056 ^{\left\{8 \right\}} | 0.05260 ^{\left\{3 \right\}} | 0.05573 ^{\left\{7 \right\}} | 0.05571 ^{\left\{6 \right\}} | 0.02038 ^{\left\{1 \right\}} | 0.04542 ^{\left\{2 \right\}} | 0.05309 ^{\left\{4 \right\}} | 0.05470 ^{\left\{5 \right\}} | ||
\hat{\theta} | 0.54563 ^{\left\{8 \right\}} | 0.42847 ^{\left\{3 \right\}} | 0.47838 ^{\left\{7 \right\}} | 0.45810 ^{\left\{5 \right\}} | 0.09746 ^{\left\{1 \right\}} | 0.36816 ^{\left\{2 \right\}} | 0.44966 ^{\left\{4 \right\}} | 0.46232 ^{\left\{6 \right\}} | ||
MSEs | \hat{\vartheta} | 2.63270 ^{\left\{8 \right\}} | 2.08923 ^{\left\{3 \right\}} | 2.37558 ^{\left\{7 \right\}} | 2.20468 ^{\left\{5 \right\}} | 0.02535 ^{\left\{1 \right\}} | 1.86391 ^{\left\{2 \right\}} | 2.18628 ^{\left\{4 \right\}} | 2.32684 ^{\left\{6 \right\}} | |
\hat{\varphi} | 0.06343 ^{\left\{2 \right\}} | 0.11259 ^{\left\{7 \right\}} | 0.07126 ^{\left\{4 \right\}} | 0.11064 ^{\left\{6 \right\}} | 0.04499 ^{\left\{1 \right\}} | 0.12626 ^{\left\{8 \right\}} | 0.06649 ^{\left\{3 \right\}} | 0.08397 ^{\left\{5 \right\}} | ||
\hat{\lambda} | 0.00531 ^{\left\{8 \right\}} | 0.00437 ^{\left\{3 \right\}} | 0.00472 ^{\left\{6 \right\}} | 0.00486 ^{\left\{7 \right\}} | 0.00066 ^{\left\{1 \right\}} | 0.00347 ^{\left\{2 \right\}} | 0.00441 ^{\left\{4 \right\}} | 0.00470 ^{\left\{5 \right\}} | ||
\hat{\theta} | 0.44122 ^{\left\{8 \right\}} | 0.33416 ^{\left\{3 \right\}} | 0.37078 ^{\left\{5 \right\}} | 0.37139 ^{\left\{6 \right\}} | 0.01519 ^{\left\{1 \right\}} | 0.25838 ^{\left\{2 \right\}} | 0.33754 ^{\left\{4 \right\}} | 0.37704 ^{\left\{7 \right\}} | ||
MREs | \hat{\vartheta} | 0.32920 ^{\left\{8 \right\}} | 0.26063 ^{\left\{2 \right\}} | 0.31198 ^{\left\{7 \right\}} | 0.26954 ^{\left\{4 \right\}} | 0.00957 ^{\left\{1 \right\}} | 0.26211 ^{\left\{3 \right\}} | 0.29645 ^{\left\{6 \right\}} | 0.28705 ^{\left\{5 \right\}} | |
\hat{\varphi} | 0.26915 ^{\left\{2 \right\}} | 0.35774 ^{\left\{7 \right\}} | 0.28513 ^{\left\{4 \right\}} | 0.35434 ^{\left\{6 \right\}} | 0.22100 ^{\left\{1 \right\}} | 0.36544 ^{\left\{8 \right\}} | 0.27411 ^{\left\{3 \right\}} | 0.30888 ^{\left\{5 \right\}} | ||
\hat{\lambda} | 0.12113 ^{\left\{8 \right\}} | 0.10520 ^{\left\{3 \right\}} | 0.11146 ^{\left\{7 \right\}} | 0.11142 ^{\left\{6 \right\}} | 0.04075 ^{\left\{1 \right\}} | 0.09084 ^{\left\{2 \right\}} | 0.10618 ^{\left\{4 \right\}} | 0.10941 ^{\left\{5 \right\}} | ||
\hat{\theta} | 0.43650 ^{\left\{8 \right\}} | 0.34278 ^{\left\{3 \right\}} | 0.38270 ^{\left\{7 \right\}} | 0.36648 ^{\left\{5 \right\}} | 0.07797 ^{\left\{1 \right\}} | 0.29453 ^{\left\{2 \right\}} | 0.35973 ^{\left\{4 \right\}} | 0.36986 ^{\left\{6 \right\}} | ||
\sum{Ranks} | {\textbf{78}} ^{\left\{8 \right\}} | {\textbf{46}} ^{\left\{3 \right\}} | {\textbf{72}} ^{\left\{7 \right\}} | {\textbf{66}} ^{\left\{6 \right\}} | {\textbf{12}} ^{\left\{1 \right\}} | {\textbf{44}} ^{\left\{2 \right\}} | {\textbf{49}} ^{\left\{4 \right\}} | {\textbf{65}} ^{\left\{5 \right\}} | ||
500 | |BIAS| | \hat{\vartheta} | 1.29672 ^{\left\{5 \right\}} | 1.27235 ^{\left\{3 \right\}} | 1.31237 ^{\left\{6 \right\}} | 1.32513 ^{\left\{7 \right\}} | 0.01519 ^{\left\{1 \right\}} | 1.15120 ^{\left\{2 \right\}} | 1.28994 ^{\left\{4 \right\}} | 1.34189 ^{\left\{8 \right\}} |
\hat{\varphi} | 0.13744 ^{\left\{2 \right\}} | 0.17802 ^{\left\{6 \right\}} | 0.14815 ^{\left\{4 \right\}} | 0.18664 ^{\left\{7 \right\}} | 0.10227 ^{\left\{1 \right\}} | 0.19392 ^{\left\{8 \right\}} | 0.14640 ^{\left\{3 \right\}} | 0.15865 ^{\left\{5 \right\}} | ||
\hat{\lambda} | 0.04970 ^{\left\{5 \right\}} | 0.05107 ^{\left\{6 \right\}} | 0.04913 ^{\left\{4 \right\}} | 0.05400 ^{\left\{8 \right\}} | 0.01264 ^{\left\{1 \right\}} | 0.03665 ^{\left\{2 \right\}} | 0.04825 ^{\left\{3 \right\}} | 0.05335 ^{\left\{7 \right\}} | ||
\hat{\theta} | 0.44102 ^{\left\{5 \right\}} | 0.44509 ^{\left\{6 \right\}} | 0.43050 ^{\left\{4 \right\}} | 0.47518 ^{\left\{8 \right\}} | 0.05962 ^{\left\{1 \right\}} | 0.31796 ^{\left\{2 \right\}} | 0.42160 ^{\left\{3 \right\}} | 0.46945 ^{\left\{7 \right\}} | ||
MSEs | \hat{\vartheta} | 2.07266 ^{\left\{4 \right\}} | 2.19148 ^{\left\{6 \right\}} | 2.07839 ^{\left\{5 \right\}} | 2.34226 ^{\left\{8 \right\}} | 0.00344 ^{\left\{1 \right\}} | 1.67247 ^{\left\{2 \right\}} | 2.02330 ^{\left\{3 \right\}} | 2.31957 ^{\left\{7 \right\}} | |
\hat{\varphi} | 0.02955 ^{\left\{2 \right\}} | 0.05030 ^{\left\{6 \right\}} | 0.03417 ^{\left\{4 \right\}} | 0.05288 ^{\left\{7 \right\}} | 0.01679 ^{\left\{1 \right\}} | 0.06276 ^{\left\{8 \right\}} | 0.03295 ^{\left\{3 \right\}} | 0.03874 ^{\left\{5 \right\}} | ||
\hat{\lambda} | 0.00377 ^{\left\{4 \right\}} | 0.00418 ^{\left\{6 \right\}} | 0.00378 ^{\left\{5 \right\}} | 0.00452 ^{\left\{7 \right\}} | 0.00025 ^{\left\{1 \right\}} | 0.00242 ^{\left\{2 \right\}} | 0.00363 ^{\left\{3 \right\}} | 0.00454 ^{\left\{8 \right\}} | ||
\hat{\theta} | 0.30987 ^{\left\{5 \right\}} | 0.34423 ^{\left\{6 \right\}} | 0.30505 ^{\left\{4 \right\}} | 0.37795 ^{\left\{8 \right\}} | 0.00564 ^{\left\{1 \right\}} | 0.19875 ^{\left\{2 \right\}} | 0.29242 ^{\left\{3 \right\}} | 0.37470 ^{\left\{7 \right\}} | ||
MREs | \hat{\vartheta} | 0.28816 ^{\left\{5 \right\}} | 0.28275 ^{\left\{3 \right\}} | 0.29164 ^{\left\{6 \right\}} | 0.29447 ^{\left\{7 \right\}} | 0.00337 ^{\left\{1 \right\}} | 0.25582 ^{\left\{2 \right\}} | 0.28665 ^{\left\{4 \right\}} | 0.29820 ^{\left\{8 \right\}} | |
\hat{\varphi} | 0.18326 ^{\left\{2 \right\}} | 0.23736 ^{\left\{6 \right\}} | 0.19754 ^{\left\{4 \right\}} | 0.24885 ^{\left\{7 \right\}} | 0.13636 ^{\left\{1 \right\}} | 0.25856 ^{\left\{8 \right\}} | 0.19520 ^{\left\{3 \right\}} | 0.21153 ^{\left\{5 \right\}} | ||
\hat{\lambda} | 0.09940 ^{\left\{5 \right\}} | 0.10214 ^{\left\{6 \right\}} | 0.09825 ^{\left\{4 \right\}} | 0.10801 ^{\left\{8 \right\}} | 0.02528 ^{\left\{1 \right\}} | 0.07330 ^{\left\{2 \right\}} | 0.09651 ^{\left\{3 \right\}} | 0.10671 ^{\left\{7 \right\}} | ||
\hat{\theta} | 0.35281 ^{\left\{5 \right\}} | 0.35607 ^{\left\{6 \right\}} | 0.34440 ^{\left\{4 \right\}} | 0.38014 ^{\left\{8 \right\}} | 0.04770 ^{\left\{1 \right\}} | 0.25437 ^{\left\{2 \right\}} | 0.33728 ^{\left\{3 \right\}} | 0.37556 ^{\left\{7 \right\}} | ||
\sum{Ranks} | {\textbf{49}} ^{\left\{4 \right\}} | {\textbf{66}} ^{\left\{6 \right\}} | {\textbf{54}} ^{\left\{5 \right\}} | {\textbf{90}} ^{\left\{8 \right\}} | {\textbf{12}} ^{\left\{1 \right\}} | {\textbf{42}} ^{\left\{3 \right\}} | {\textbf{38}} ^{\left\{2 \right\}} | {\textbf{81}} ^{\left\{7 \right\}} |
\mathit{\boldsymbol{\eta}}^{\intercal} | n | MLEs | LSEs | WLSEs | CRVMEs | MPSEs | PCEs | ADEs | RADEs |
(\hat{\vartheta}=1.75, \hat{\varphi}=0.5, \hat{\lambda}=0.25, \hat{\theta}=1.25) | 20 | 2 | 7 | 1 | 5 | 4 | 8 | 3 | 6 |
80 | 3 | 7 | 2 | 6 | 1 | 5 | 4 | 8 | |
200 | 1.5 | 7 | 4 | 5 | 1.5 | 6 | 3 | 8 | |
500 | 2 | 5.5 | 4 | 5.5 | 1 | 7 | 3 | 8 | |
(\hat{\vartheta}=1.75, \hat{\varphi}=2, \hat{\lambda}=1.5, \hat{\theta}=2.5) | 20 | 1 | 3 | 2 | 7 | 4 | 8 | 5.5 | 5.5 |
80 | 1 | 3 | 4 | 6 | 2 | 8 | 5 | 7 | |
200 | 2 | 3 | 4.5 | 6.5 | 1 | 8 | 4.5 | 6.5 | |
500 | 3 | 4 | 5 | 6 | 1 | 8 | 2 | 7 | |
(\hat{\vartheta}=3.5, \hat{\varphi}=0.75, \hat{\lambda}=0.5, \hat{\theta}=1.25) | 20 | 7 | 4 | 3 | 6 | 1 | 8 | 2 | 5 |
80 | 8 | 3 | 4 | 7 | 1 | 5 | 2 | 6 | |
200 | 6 | 5 | 4 | 8 | 1 | 3 | 2 | 7 | |
500 | 2 | 6 | 5 | 7 | 1 | 4 | 3 | 8 | |
(\hat{\vartheta}=3.5, \hat{\varphi}=2, \hat{\lambda}=1.5, \hat{\theta}=2.5) | 20 | 8 | 2 | 6 | 3 | 1 | 7 | 5 | 4 |
80 | 8 | 2 | 7 | 3 | 1 | 6 | 4 | 5 | |
200 | 6.5 | 2 | 6.5 | 3 | 1 | 8 | 4 | 5 | |
500 | 5.5 | 3 | 7 | 5.5 | 1 | 8 | 2 | 4 | |
(\hat{\vartheta}=4.5, \hat{\varphi}=0.75, \hat{\lambda}=0.5, \hat{\theta}=1.25) | 20 | 8 | 2 | 7 | 3 | 1 | 6 | 5 | 4 |
80 | 8 | 2 | 7 | 6 | 1 | 3 | 5 | 4 | |
200 | 8 | 3 | 7 | 6 | 1 | 2 | 4 | 5 | |
500 | 4 | 6 | 5 | 8 | 1 | 3 | 2 | 7 | |
(\hat{\vartheta}=4.5, \hat{\varphi}=0.75, \hat{\lambda}=0.5, \hat{\theta}=1.25) | 20 | 8 | 2 | 5 | 3 | 1 | 7 | 6 | 4 |
80 | 6 | 2 | 4 | 3 | 1 | 8 | 7 | 5 | |
200 | 6 | 2 | 4 | 3 | 1 | 7 | 8 | 5 | |
500 | 6 | 2 | 5 | 3 | 1 | 8 | 7 | 4 | |
(\hat{\vartheta}=0.25, \hat{\varphi}=3.5, \hat{\lambda}=3, \hat{\theta}=0.25) | 20 | 1 | 6 | 8 | 2 | 7 | 5 | 4 | 3 |
80 | 1.5 | 5 | 8 | 1.5 | 6 | 4 | 3 | 7 | |
200 | 1 | 7 | 4 | 6 | 2 | 3 | 5 | 8 | |
500 | 2 | 8 | 6 | 7 | 1 | 3 | 4.5 | 4.5 | |
(\hat{\vartheta}=0.75, \hat{\varphi}=2.5, \hat{\lambda}=4.25, \hat{\theta}=0.25) | 20 | 2 | 8 | 6 | 5 | 3 | 1 | 4 | 7 |
80 | 1.5 | 7 | 5 | 6 | 1.5 | 3 | 4 | 8 | |
200 | 2 | 7 | 5 | 6 | 1 | 3 | 4 | 8 | |
500 | 2 | 8 | 5 | 6 | 1 | 3 | 4 | 7 | |
\sum Ranks | \textbf{133.5} | \textbf{143.5} | \textbf{160} | \textbf{164} | \textbf{54} | \textbf{176} | \textbf{130.5} | \textbf{190.5} | |
{\textbf{Overall Rank}} | \textbf{3} | \textbf{4} | \textbf{5} | \textbf{6} | \textbf{1} | \textbf{7} | \textbf{2} | \textbf{8} |
Model | Par.. | Estimates | (SEs) | AIC | CAIC | BIC | HQIC | W^{*} | A^{*} | KS | (PV) |
EWFr | \hat{\vartheta} | 56.93662 | (38.42845) | 827.52150 | 827.84670 | 838.92960 | 832.15660 | 0.01991 | 0.13402 | 0.03757 | 0.99713 |
\hat{\varphi} | 0.46697 | (0.22382) | |||||||||
\hat{\lambda} | 0.71825 | (0.01713) | |||||||||
\hat{\theta} | 0.01794 | (0.01207) | |||||||||
OLxFr | \hat{\vartheta} | 2.18260 | (1.04763) | 827.70270 | 828.02790 | 839.11080 | 832.33780 | 0.02380 | 0.16520 | 0.04060 | 0.98433 |
\hat{\varphi} | 625.38000 | (622.71234) | |||||||||
\hat{a} | 0.12210 | (0.08023) | |||||||||
\hat{b} | 1.38560 | (0.17273) | |||||||||
KMOFr | \hat{\vartheta} | 43.87750 | (128.52731) | 831.05330 | 831.54510 | 845.31340 | 836.84730 | 0.26278 | 0.34950 | 0.04457 | 0.96120 |
\hat{\varphi} | 0.52028 | (0.41388) | |||||||||
\hat{\delta} | 0.01314 | (0.00808) | |||||||||
\hat{a} | 3.27359 | (2.90929) | |||||||||
\hat{b} | 19.93782 | (47.58906) | |||||||||
EFr | \hat{a} | 1426.40000 | (1440.19245) | 830.85880 | 831.05240 | 839.41490 | 834.33520 | 0.06720 | 0.46640 | 0.04910 | 0.91754 |
\hat{b} | 0.26240 | (0.02850) | |||||||||
\hat{\theta} | 46.24800 | (22.87321) | |||||||||
BFr | \hat{\vartheta} | 0.60970 | (0.32261) | 833.09830 | 833.42350 | 844.50640 | 837.73350 | 0.06900 | 0.47650 | 0.05540 | 0.82683 |
\hat{\varphi} | 36.60200 | (19.48341) | |||||||||
\hat{a} | 739.38700 | (629.24021) | |||||||||
\hat{b} | 0.32240 | (0.06044) | |||||||||
GExFr | \hat{\vartheta} | 226.67000 | (267.96324) | 840.21630 | 840.54150 | 851.62450 | 844.85150 | 0.14320 | 0.95970 | 0.06640 | 0.62593 |
\hat{\varphi} | 91.93900 | (133.94324) | |||||||||
\hat{a} | 22.27100 | (119.19139) | |||||||||
\hat{b} | 0.07090 | (0.04132) | |||||||||
TEFr | \hat{\vartheta} | 3.25820 | (0.40714) | 892.00150 | 892.09750 | 897.70560 | 894.31910 | 0.74430 | 4.54640 | 0.14080 | 0.01254 |
\hat{\varphi} | 0.75210 | (0.04224) | |||||||||
\hat{a} | 39.38721 | (40.24321) | |||||||||
\hat{b} | 0.25243 | (0.76041) | |||||||||
Fr | \hat{\theta} | 0.75208 | (0.04242) | 892.00150 | 892.09750 | 897.70560 | 894.31910 | 0.74432 | 4.54642 | 0.14079 | 0.01250 |
\hat{\lambda} | 2.43109 | (0.21928) |
Model | Par.. | Estimates | (SEs) | AIC | CAIC | BIC | HQIC | W^{*} | A^{*} | KS | (PV) |
EWFr | \hat{\vartheta} | 0.76563 | (0.3765631) | 119.68850 | 120.37820 | 128.26110 | 123.06020 | 0.04181 | 0.23988 | 0.06847 | 0.92920 |
\hat{\varphi} | 0.04349 | (0.3074545) | |||||||||
\hat{\lambda} | 145.67068 | (208.851634) | |||||||||
\hat{\theta} | 4.60846 | (1.2033681) | |||||||||
OLxFr | \hat{\vartheta} | 5.14301 | (9.9790719) | 119.94170 | 120.63140 | 128.51430 | 123.31340 | 0.04741 | 0.26530 | 0.07631 | 0.85664 |
\hat{\varphi} | 5.82568 | (7.6204045) | |||||||||
\hat{a} | 4.17374 | (2.671917) | |||||||||
\hat{b} | 2.82338 | (0.9308868) | |||||||||
KMOFr | \hat{\vartheta} | 31946.70000 | (837.7074) | 121.90480 | 122.95750 | 132.62050 | 126.11940 | 0.04616 | 0.26108 | 0.07450 | 0.87551 |
\hat{\varphi} | 3.40405 | (1.493079) | |||||||||
\hat{\delta} | 3.35291 | (0.9682264) | |||||||||
\hat{a} | 0.85381 | (0.1934628) | |||||||||
\hat{b} | 13724.50000 | (32837.29) | |||||||||
EFr | \hat{a} | 2.58672 | (2.2847576) | 122.19730 | 122.88700 | 130.76990 | 125.56900 | 0.07082 | 0.40707 | 0.08894 | 0.70136 |
\hat{b} | 0.50304 | (0.4780356) | |||||||||
\hat{\theta} | 6.25000 | (10.0300775) | |||||||||
BFr | \hat{\vartheta} | 2.38748 | (5.65075) | 120.58420 | 121.27380 | 129.15670 | 123.95580 | 0.06008 | 0.32203 | 0.07953 | 0.82045 |
\hat{\varphi} | 1.00168 | (5.322252) | |||||||||
\hat{a} | 22.38231 | (162.860747) | |||||||||
\hat{b} | 27.37096 | (295.790324) | |||||||||
GExFr | \hat{\vartheta} | 2.41716 | (8.509608) | 120.57980 | 121.26950 | 129.15240 | 123.95150 | 0.06064 | 0.32329 | 0.07962 | 0.81934 |
\hat{\varphi} | 1.20859 | (2.106142) | |||||||||
\hat{a} | 24.77784 | (82.649275) | |||||||||
\hat{b} | 15.60163 | (85.339807) | |||||||||
TEFr | \hat{\vartheta} | 4.04638 | (1.0119058) | 121.04420 | 121.73390 | 129.61670 | 124.41580 | 0.06547 | 0.34378 | 0.07500 | 0.87047 |
\hat{\varphi} | 2.50000 | (0.5973595) | |||||||||
\hat{a} | 5.25000 | (2.6300897) | |||||||||
\hat{b} | 0.09255 | (1.2750272) | |||||||||
Fr | \hat{\theta} | 5.43392 | (0.50788) | 121.80430 | 122.00430 | 126.09060 | 123.49010 | 0.11497 | 0.64202 | 0.10013 | 0.55274 |
\hat{\lambda} | 230.48644 | (110.91778) |
Methods | \hat{\vartheta} | \hat{\varphi} | \hat{\lambda} | \hat{\theta} | W^{*} | A^{*} | KS | PV |
MLEs | 56.93661 | 0.46697 | 0.71825 | 0.01794 | 0.01991 | 0.13402 | 0.03757 | 0.99713 |
LSEs | 56.93660 | 0.55502 | 0.71932 | 0.01871 | 0.01850 | 0.12986 | 0.03334 | 0.99918 |
MPSEs | 56.93660 | 0.46698 | 0.71750 | 0.01717 | 0.01916 | 0.13069 | 0.03617 | 0.99708 |
WLSEs | 56.94615 | 0.51962 | 0.71884 | 0.01825 | 0.01815 | 0.12600 | 0.03251 | 0.99947 |
CRVMEs | 56.93659 | 0.55064 | 0.71962 | 0.01891 | 0.01795 | 0.12584 | 0.03352 | 0.99910 |
ADEs | 56.93668 | 0.47772 | 0.71887 | 0.01815 | 0.01763 | 0.12016 | 0.03167 | 0.99968 |
PCEs | 57.47389 | 0.81500 | 0.72219 | 0.02155 | 0.03320 | 0.23254 | 0.03830 | 0.99367 |
RADEs | 56.93805 | 0.56319 | 0.71967 | 0.01899 | 0.01830 | 0.12878 | 0.03385 | 0.99895 |
\textbf{Methods} | \hat{\vartheta} | \hat{\varphi} | \hat{\lambda} | \hat{\theta} | W^{*} | A^{*} | \textbf{KS} | \textbf{PV} |
\textbf{MLEs} | 0.76563 | 0.04349 | 145.67068 | 4.60846 | 0.04181 | 0.23988 | 0.06847 | 0.92920 |
\textbf{LSEs} | 0.69421 | 0.08110 | 145.67010 | 4.56661 | 0.04850 | 0.26973 | 0.06486 | 0.94154 |
\textbf{MPSEs} | 0.75534 | 0.13947 | 145.66920 | 4.65631 | 0.05531 | 0.29551 | 0.07780 | 0.81897 |
\textbf{WLSEs} | 0.71596 | 0.01530 | 145.66740 | 4.57637 | 0.04747 | 0.26675 | 0.06895 | 0.90999 |
\textbf{CRVMEs} | 0.71398 | 0.00100 | 145.67030 | 4.57259 | 0.04761 | 0.26713 | 0.06733 | 0.92336 |
\textbf{ADEs} | 0.72963 | 0.00010 | 145.67980 | 4.57988 | 0.04696 | 0.26562 | 0.07047 | 0.89642 |
\textbf{PCEs} | 0.75075 | 0.02596 | 145.67020 | 4.59649 | 0.04775 | 0.26737 | 0.07311 | 0.87060 |
\textbf{RADEs} | 0.74062 | 0.00010 | 145.66970 | 4.57722 | 0.04668 | 0.26516 | 0.07058 | 0.89538 |