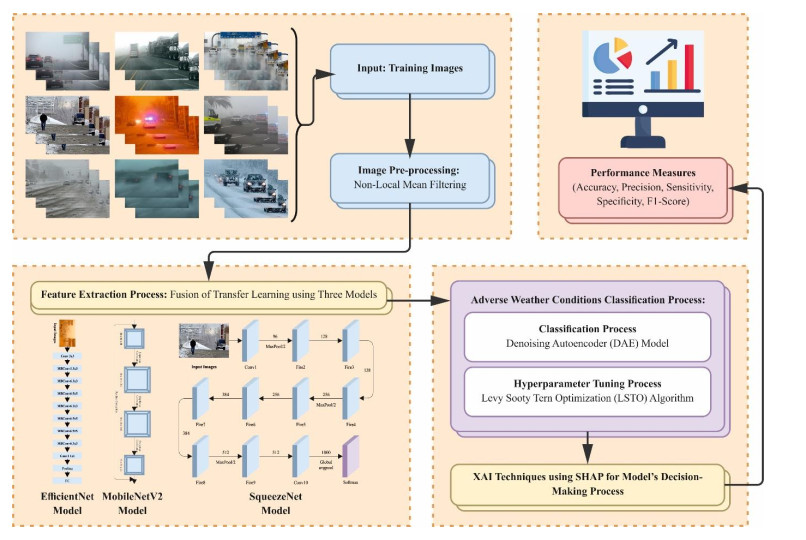
Autonomous vehicles (AVs), particularly self-driving cars, have produced a large amount of interest in artificial intelligence (AI), intelligent transportation, and computer vision. Tracing and detecting numerous targets in real-time, mainly in city arrangements in adversarial environmental conditions, has become a significant challenge for AVs. The effectiveness of vehicle detection has been measured as a crucial stage in intelligent visual surveillance or traffic monitoring. After developing driver assistance and AV methods, adversarial weather conditions have become an essential problem. Nowadays, deep learning (DL) and machine learning (ML) models are critical to enhancing object detection in AVs, particularly in adversarial weather conditions. However, according to statistical learning, conventional AI is fundamental, facing restrictions due to manual feature engineering and restricted flexibility in adaptive environments. This study presents the explainable artificial intelligence with fusion-based transfer learning on adverse weather conditions detection for autonomous vehicles (XAIFTL-AWCDAV) method. The XAIFTL-AWCDAV model's main aim is to detect and classify weather conditions for AVs in challenging scenarios. In the preprocessing stage, the XAIFTL-AWCDAV model utilizes a non-local mean filtering (NLM) method for noise reduction. Besides, the XAIFTL-AWCDAV model performs feature extraction by fusing three models: EfficientNet, SqueezeNet, and MobileNetv2. The denoising autoencoder (DAE) technique is employed to classify adverse weather conditions. Next, the DAE method's hyperparameter selection uses the Levy sooty tern optimization (LSTO) approach. Finally, to ensure the transparency of the model's predictions, XAIFTL-AWCDAV integrates explainable AI (XAI) techniques, utilizing SHAP to visualize and interpret each feature's impact on the model's decision-making process. The efficiency of the XAIFTL-AWCDAV method is validated by comprehensive studies using a benchmark dataset. Numerical results show that the XAIFTL-AWCDAV method obtained a superior value of 98.90% over recent techniques.
Citation: Khaled Tarmissi, Hanan Abdullah Mengash, Noha Negm, Yahia Said, Ali M. Al-Sharafi. Explainable artificial intelligence with fusion-based transfer learning on adverse weather conditions detection using complex data for autonomous vehicles[J]. AIMS Mathematics, 2024, 9(12): 35678-35701. doi: 10.3934/math.20241693
[1] | Thavavel Vaiyapuri, M. Sivakumar, Shridevi S, Velmurugan Subbiah Parvathy, Janjhyam Venkata Naga Ramesh, Khasim Syed, Sachi Nandan Mohanty . An intelligent water drop algorithm with deep learning driven vehicle detection and classification. AIMS Mathematics, 2024, 9(5): 11352-11371. doi: 10.3934/math.2024557 |
[2] | Jian Qi . Artificial intelligence-based intelligent computing using circular q-rung orthopair fuzzy information aggregation. AIMS Mathematics, 2025, 10(2): 3062-3094. doi: 10.3934/math.2025143 |
[3] | Maha M. Althobaiti, José Escorcia-Gutierrez . Weighted salp swarm algorithm with deep learning-powered cyber-threat detection for robust network security. AIMS Mathematics, 2024, 9(7): 17676-17695. doi: 10.3934/math.2024859 |
[4] | Iftikhar Ahmad, Hira Ilyas, Muhammad Asif Zahoor Raja, Tahir Nawaz Cheema, Hasnain Sajid, Kottakkaran Sooppy Nisar, Muhammad Shoaib, Mohammed S. Alqahtani, C Ahamed Saleel, Mohamed Abbas . Intelligent computing based supervised learning for solving nonlinear system of malaria endemic model. AIMS Mathematics, 2022, 7(11): 20341-20369. doi: 10.3934/math.20221114 |
[5] | Sultanah M. Alshammari, Nofe A. Alganmi, Mohammed H. Ba-Aoum, Sami Saeed Binyamin, Abdullah AL-Malaise AL-Ghamdi, Mahmoud Ragab . Hybrid arithmetic optimization algorithm with deep learning model for secure Unmanned Aerial Vehicle networks. AIMS Mathematics, 2024, 9(3): 7131-7151. doi: 10.3934/math.2024348 |
[6] | Muhammad Ali Khan, Saleem Abdullah, Alaa O. Almagrabi . Analysis of deep learning technique using a complex spherical fuzzy rough decision support model. AIMS Mathematics, 2023, 8(10): 23372-23402. doi: 10.3934/math.20231188 |
[7] | A. Presno Vélez, M. Z. Fernández Muñiz, J. L. Fernández Martínez . Enhancing structural health monitoring with machine learning for accurate prediction of retrofitting effects. AIMS Mathematics, 2024, 9(11): 30493-30514. doi: 10.3934/math.20241472 |
[8] | Manal Abdullah Alohali, Fuad Al-Mutiri, Kamal M. Othman, Ayman Yafoz, Raed Alsini, Ahmed S. Salama . An enhanced tunicate swarm algorithm with deep-learning based rice seedling classification for sustainable computing based smart agriculture. AIMS Mathematics, 2024, 9(4): 10185-10207. doi: 10.3934/math.2024498 |
[9] | Muhammad Danish Zia, Esmail Hassan Abdullatif Al-Sabri, Faisal Yousafzai, Murad-ul-Islam Khan, Rashad Ismail, Mohammed M. Khalaf . A study of quadratic Diophantine fuzzy sets with structural properties and their application in face mask detection during COVID-19. AIMS Mathematics, 2023, 8(6): 14449-14474. doi: 10.3934/math.2023738 |
[10] | Tamilvizhi Thanarajan, Youseef Alotaibi, Surendran Rajendran, Krishnaraj Nagappan . Improved wolf swarm optimization with deep-learning-based movement analysis and self-regulated human activity recognition. AIMS Mathematics, 2023, 8(5): 12520-12539. doi: 10.3934/math.2023629 |
Autonomous vehicles (AVs), particularly self-driving cars, have produced a large amount of interest in artificial intelligence (AI), intelligent transportation, and computer vision. Tracing and detecting numerous targets in real-time, mainly in city arrangements in adversarial environmental conditions, has become a significant challenge for AVs. The effectiveness of vehicle detection has been measured as a crucial stage in intelligent visual surveillance or traffic monitoring. After developing driver assistance and AV methods, adversarial weather conditions have become an essential problem. Nowadays, deep learning (DL) and machine learning (ML) models are critical to enhancing object detection in AVs, particularly in adversarial weather conditions. However, according to statistical learning, conventional AI is fundamental, facing restrictions due to manual feature engineering and restricted flexibility in adaptive environments. This study presents the explainable artificial intelligence with fusion-based transfer learning on adverse weather conditions detection for autonomous vehicles (XAIFTL-AWCDAV) method. The XAIFTL-AWCDAV model's main aim is to detect and classify weather conditions for AVs in challenging scenarios. In the preprocessing stage, the XAIFTL-AWCDAV model utilizes a non-local mean filtering (NLM) method for noise reduction. Besides, the XAIFTL-AWCDAV model performs feature extraction by fusing three models: EfficientNet, SqueezeNet, and MobileNetv2. The denoising autoencoder (DAE) technique is employed to classify adverse weather conditions. Next, the DAE method's hyperparameter selection uses the Levy sooty tern optimization (LSTO) approach. Finally, to ensure the transparency of the model's predictions, XAIFTL-AWCDAV integrates explainable AI (XAI) techniques, utilizing SHAP to visualize and interpret each feature's impact on the model's decision-making process. The efficiency of the XAIFTL-AWCDAV method is validated by comprehensive studies using a benchmark dataset. Numerical results show that the XAIFTL-AWCDAV method obtained a superior value of 98.90% over recent techniques.
The World Health Organization (WHO) has been frightened by the number of fatalities and deadly injuries owing to carelessness, driving too fast, and alcoholism. The Department of U.S. Transportation has stated that about 94% of crashes happen in the U.S. because of human carelessness [1]. Automation in transportation presents several exciting possibilities, such as road facility development, decrease in petrol depletion, decrease in emissions, and decrease in travel time. Hence, AVs have recently attracted considerable attention as a novel transportation structure [2]. To achieve autonomy in vehicles, numerous sensors are employed to perceive the surrounding environment, like cameras and photonic and classical radars. AVs, particularly in autonomous cars, have created significant interest in smart transportation, AI, and computer vision [3]. The AVs mainly rely on sensors and cameras, such as radar, ultrasonic sensors, and LiDAR (light detection and ranging). These technologies enable a range of self-driving features, including decreased fuel usage, reduced traffic crowding, reduced road crashes, and more efficient road use, contributing to an enhanced transport system. AVs could help study and manage traffic by collecting effectual and valid traffic data [4]. The domain of object detection (OD) has developed from the perception of advanced methods to becoming an essential portion of applications within these industries [5]. The implementation of OD in infinite real-world implications followed the development of recognition methods and the rising numerical abilities of processors. From monitoring processes to image analysis and face recognition, OD can be optimized to help humans with smart analysis and automating complex tasks [6].
Currently, the application of OD to AVs is gaining interest owing to the necessity for precise and fast indicators for navigation in urban and traffic environments. OD can be crucial for AVs as it assures protection by recognizing and evading stirring complications in their route. Various experiments have examined several techniques for identifying objects in AVs, namely fully automated, semi-automated, and manual methods [7]. The development of GPU and sensors besides DL methods has focused on self-driving or autonomous applications based on AI. AVs can accurately identify traffic objects to make precise control decisions and assure essential safety [8]. Various sensors like light recognition and cameras are generally used in AVs to identify these objects. However, unfavorable weather conditions can affect image quality of the camera. Conventional and DL methods are essential for improving OD in AVs, particularly under adverse weather [9]. However, conventional AI, based on statistical learning faces limitations owing to its restricted adaptability to dynamic environments and reliance on manual feature engineering, which require extensive retraining and hinder its efficiency in real-world applications [10]. DL provides a robust replacement to conventional ML techniques for OD in AVs, tackling the limits of adaptability in dynamic environments and manual feature engineering.
This study presents the explainable artificial intelligence with fusion-based transfer learning on adverse weather conditions detection for autonomous vehicles (XAIFTL-AWCDAV) method. The XAIFTL-AWCDAV model's main aim is to detect and classify weather conditions for AVs in challenging scenarios. In the preprocessing stage, the XAIFTL-AWCDAV model utilizes a non-local mean filtering (NLM) method for noise reduction. Besides, the XAIFTL-AWCDAV model performs feature extraction by fusing three models: EfficientNet, SqueezeNet, and MobileNetv2. The denoising autoencoder (DAE) technique is employed to classify adverse weather conditions. Next, the DAE method's hyperparameter selection uses the Levy sooty tern optimization (LSTO) approach. Finally, to ensure the transparency of the model's predictions, the XAIFTL-AWCDAV approach integrates explainable AI (XAI) techniques, utilizing SHAP to visualize and interpret each feature's impact on the model's decision-making process. The efficiency of the XAIFTL-AWCDAV method is validated by comprehensive studies using a benchmark dataset.
Appiah and Mensah [11] proposed the incorporation of two DL techniques, namely ESRGAN and YOLOv7. First, ESRGAN analyzes training data and adjusts for adverse weather conditions, followed by YOLOv7 performing OD. The use of ESRGAN allowed for the adaptive enhancement of each image, improving the recognition performance of YOLOv7. Tahir et al. [12] provided a comprehensive overview of OD under unfavorable weather conditions while also examining the constantly developing domain of Avs. This work addressed the challenges for AVs in adverse meteorological conditions and the fundamental architecture of OD and examined conventional and DL techniques for OD in the AVs domain. This work proved vital for enhancing AV abilities in identifying and acknowledging objects in environments. Vellaidurai and Rathinam [13] presented a new DL method called Optimized You Look Only Once Version 5 (OYOLOV5) for AV detection (AVD) in adverse weather conditions. This approach contains three stages: data gathering, preprocessing of data, and feature classification/extraction. Data used to implement VD was gathered from the COCO and DAWN datasets, which are freely accessible. Data augmentation was executed on the collected input data by adding noise, blur saturation, hue, and intensity to an improved observation of vehicles. Carvalho et al. [14] presented a structure for analyzing snow accretion on vehicles. Existing snow and icing accumulation methods were reviewed, and alterations for automobile applications were suggested. Based on novel abilities from the Weather on Wheels (WoW) program, the researchers designed a framework to predict snow accumulation for AV under operating conditions; initial outcomes were provided.
Wen et al. [15] designed a multimodal contrastive learning (CL) approach called DHT-CL to increase point cloud 3DSS in challenging weather conditions to recognize rail obstacles. DHT-CL employs a fusion tactic combining LiDAR and camera sensors for recognition tasks without requiring image input in the interpretation phase. The authors initially determined how the sensor fusing technique could be robust under snow and rain conditions and then devised a dual-helix transformer (DHT) to extract cross-modal data over an surroundings attention mechanism. Zhu et al. [16] presented a polarimetric OD benchmark (PODB) dataset. The PODB offers a combined quality assessment structure for DL-based OD methods in difficult road areas by integrating polarimetric imaging. Also, wide OD experimentations were performed using the PODB, enabling complete authentication and assessment of effectual benchmark methods. Kondapally et al. [17] developed a technique to outline and comprehend six transitional weather conditions, such as rainy to sunny or sunny to rainy. A VAE was used to incorporate the transitional weather change data, and VGG was used to remove its spatial features. Additionally, the authors designed a gated recurrent unit (GRU) to analyze the temporal distribution of this spatial feature, identifying the equivalent transitional conditions. Hou [18] presented a widespread assessment of mixed traffic comprehensive data for decision-making on upcoming CAV execution tactics. This research suggested a combined framework to assess the efficacy and security of combined traffic with CAV under unfavorable weather conditions using a multi-vehicle collision method, solitary-vehicle collision method, traffic method, and performance evaluation.
This work develops a new XAIFTL-AWCDAV method. The major goal is to detect and classify weather conditions for AVs in challenging scenarios. To achieve this, the XAIFTL-AWCDAV technique involves various stages such as noise reduction, fusion of feature extraction, hyperparameter tuning for the classification process, and XAI-based SHAP technique. Figure 1 presents the entire workflow of the XAIFTL-AWCDAV method.
Initially, the XAIFTL-AWCDAV technique utilizes the NLM method for noise elimination [19]. This method is chosen for its ability to preserve image details while effectually mitigating noise. Unlike conventional filtering techniques that depend on local pixel neighborhoods, NLM computes the weighted average of all pixels in an image based on their similarity, making it highly effective in noise reduction without blurring essential image features. This methodology is specifically useful in medical imaging, where preserving fine details is crucial. The NLM method is less prone to over-smoothing, a common issue in other denoising methods such as Gaussian or median filters. Furthermore, its ability to handle diverse types of noise, such as Gaussian or salt-and-pepper, gives it an edge over simpler methods. NLM's computational efficiency and high-quality results make it a robust choice for noise elimination in sensitive imaging applications.
The primary stage represents NLM filtering to avoid noise. Assuming a separate noise image v={v(i)|i∈I} and a pixel i, their grayscale value within the denoised image is obtained by computing the weighted average of the values of grayscale of each pixel during searching by utilizing
ˆv(i)=∑jϵSw(i,j)v(j). | (1) |
Whereas {w(i,j)}j represents normalized weights agreeable 0≤w(i,j)≤1 and Σj∈Sw(i,j)=1, and S represents a predetermined searching area, usually a larger window positioned by the targeted ith pixel.
The similarities between dual pixels i and j are based on the weighted Euclidean distance among their corresponding neighborhoods of square windows v(Nj) and v(N). The mathematical equation for the weighted Euclidean distance is
d(i,j)=‖v(Ni)−v(Nj)‖22,a. | (2) |
Here, a>0 represents the Gaussian kernel normal deviation.
After computing the value of grayscale from the targeted ith pixel within the denoised image, the equivalent weight on the jth pixel is expressed as
w(i,j)=e−d(i,j)h2Z(i). | (3) |
Now, Z(i)=Σje−d(i,j)h2 symbolizes the normalization constant, and h represents the parameters that regulate the noise suppression degree.
The XAIFTL-AWCDAV method performs feature extraction by fusing three models: EfficientNet, SqueezeNet, and MobileNetv2. These methods present several advantages in the context of biomedical image analysis. EfficientNet is prevalent for its high accuracy and effectiveness, employing a compound scaling method to balance network depth, width, and resolution, making it appropriate for complex image data. SqueezeNet, on the contrary, gives a lightweight architecture with fewer parameters, confirming faster processing without sacrificing too much accuracy—ideal for applications requiring low computational resources. MobileNetv2 balances efficiency and accuracy using depthwise separable convolutions, mitigating computational costs while maintaining performance. Integrating these models improves the system's robustness, allowing for more accurate and scalable feature extraction from biomedical images. The fusion approach takes advantage of the strengths of each model, confirming high accuracy with reduced computational overhead, a key consideration for real-time medical applications.
EfficientNet belongs to the convolutional neural network (CNN) models, praised for their efficacy and higher performance in image classification tasks [20]. Every method in the EfficientNet sequence, from EfficientNet-B0 to EfficientNet-B7, signifies various scales of the model's framework, improving width, resolution, and depth. EfficientNet-B0 represents a standard model that attains a reasonable balance between computational efficiency and accuracy despite some source limitations. EfficientNet-B1 improves upon B0 by improving the width of the models, leading to superior performance at a marginally greater computational complexity. It offers an uncertain development in accuracy in comparison with B0. EfficientNet-B2 additionally improves the depth and width of the technique, providing enhanced performance at a somewhat advanced computational cost like Bl. EfficientNet-B3 enhances the model's depth and width, producing greater precision at a greater computational complexity. It is superior to B2 but maintains a moderate trade‐off between efficiency and accuracy. EfficientNet-B4 is a superior technique with enhanced width and depth compared to B3. It gains greater accuracy but needs more computational reserves. EfficientNet-B5 is greater than B4, offering additional developments in accuracy at an improved computational cost. EfficientNet-B6 and EfficientNet-B7 represent the main methods in the sequence, providing maximum accuracy. Nevertheless, they also need considerably advanced computational sources. EfficientNet-V2M and EfficientNet-V2L represent upgraded types of EfficientNet methods, combining developments in architectural plans. They intend to increase both efficiency and accuracy in comparison with their precursors. Figure 2 illustrates the working flow of the EfficientNet model.
The previous structure had the drawback of demanding larger parameter counts for training [21]. Hence, investigators from Deepscale produced a novel framework, SqueezeNet, that requires smaller training parameters and a smaller model size. The SqueezeNet structure contains a single convolutional layer and eight fire modules. The fire modules comprise a convolutional layer with a 1 x 1 filter dimension and n filters, denoted as the squeeze layer. The squeeze layer contains dual parallel layers, identified as expand layers. The first expand layer contains a 1x1 filter dimension and 4n filters. In the same way, the second expand layer includes 3x3 filter dimensions and 4n filters. A max pooling layer is applied in the initial convolutional layer and first, second, and fourth fire modules (stride = 2), which decreases the feature mapping dimension by half. When the final fire module, an FC layer (weight = 1000), transforms the feature mapping with a 1D vector, these feature vectors have been traversed as an average pooling layer. Each of the preceding convolutional layers follows an activation function of ReLU. The softmax layer finally transforms the feature vector within the binary classification. Figure 3 depicts SqueezeNet architecture.
DL is an advanced AI approach that uses neural networks (NNs), such as numerous layers, to gain information from wide-ranging data and identify complicated patterns [22]. On the other hand, MobileNet-V2 is an efficient NN design specially designed for mobiles. It uses a reversed residual framework to manage images with minimum delay and maximum accuracy. In the present analysis, MobileNet-V2 is referenced to solve the problem of colposcopy image collections. MobileNet-V2 is selected due to its architecture and efficiency. However, when adapting to a smaller dataset, optical recognition showed overfitting. This was mitigated by using a more compact and communicative network named MobileNet-V2, which enhances implementation speed and memory usage at a lower cost after an error occurs. Parameter experimentation and tuning result in enhanced performance at higher implementation speeds. To minimize memory usage, it is desirable to have a network architecture specially designed for this purpose. MobileNet-V2 incorporates two critical approaches, separable depth‐wise convolution (SD‐WC) and inverted residual (IR). Figure 4 demonstrates the structure of the MobileNet-V2 technique.
The SD‐WC changes the standard problem using dual techniques. The first operator uses a convolution plan‐to-plan, which creates single complexities for each fit-in strategy. The property's strategies are loaded and arranged using a point‐to-point convolution with the second operator using a 1 x 1 kernel. Utilizing these operations, the complete property plans are controlled concurrently, resulting in an efficient and quick association of the image with the network's size, width, and height. However, the SD‐WC transfers the image vertically and horizontally throughout the first process; the second operator fine-tunes the image dimension from the network. The designed value for standard convolutions and the SD‐WC is defined by Eqs (4) and (5).
ZN=dj.hj.wj.K2, | (4) |
Zs=dj(k2+di).hj. | (5) |
The traditional convolutions and the related SD‐WC value are denoted by Zs and ZN, respectively. The layers of output and input are denoted by j and i, whereas djanddi symbolize the sum of input and output property strategies. The width and height of the input mapping are demonstrated by wj and hj. Finally, the dimension of the filter is defined by k. The advantages of SD‐WC across standard convolutions are calculated by Eq (6).
ZNZs=k2.dik2+di. | (6) |
Essential components are enclosed within the blocks that have been overturned. Three standard operators are used in addition to blocks, and additional links are used inside these blocks. The initial and final operators utilize 1 x 1 filters that move the input layer to the central layer and from the central layer to the output layer. 3x3 filters are applied to enable the study of the layers with the central layer. Several networks stay within the blocks mainly in the initial and final convolutions, as opposed to the interior convolution blocks.
In this work, the classification process is performed using DAE [23]. This process is highly effective for handling noisy or incomplete data, making it specifically appropriate for real-world applications where data can be corrupted. By learning to reconstruct clean input from noisy versions, the DAE improves the robustness and generalization capacity of the model. Unlike conventional classifiers, the ability of the DAE model to extract crucial features while discarding irrelevant noise enhances the performance of the model in challenging environments. Additionally, DAEs are unsupervised, meaning they can learn effectual feature representations without the requirement for labeled data, which is a significant advantage when working with large, unlabeled datasets. The combination of feature extraction and noise reduction in a single framework makes DAEs ideal for tasks requiring high accuracy and resilience, such as image and signal processing. Figure 5 represents the structure of DAE.
According to unsupervised learning, AEs are a different kind of artificial NN (ANN), whereas the networks detect features and patterns using unlabeled data. When the input dataset X is applied to train the AE method, the AE attempts to make an equivalent rebuilt image dataset R so that,
rk=xk, | (7) |
whereas k∈m and R can be characterized as
R=(r1,r2,….rm). | (8) |
Convolutional DAEs are a subdivision of AEs that work to detect noticeable image features while also overlooking any noisy occurrence. The convolution-induced process differs from the conventional AE, which helps carry out superior images. DAE is still assumed to be a stochastic addition to the conventional AE. The stochastic corruption process functions based on the standard of arbitrarily adjusting one of the input pixels to zero, thus allowing the method to predict the degraded value of the images.
The standard structure considering the initially introduced method of convolutional denoising AE contains three modules as follows:
1) Encoder: The image with noise is offered as input in the encoder. During encoding, the convolutional layers remove the prominent features of the input image. The Max Pooling layers at the encoding level reduce the image dimensions so that this image is provided in an encoding way. Technically, the classical encoder maps a nonlinear predetermined activation function g1 in the output of the encoder as specified by
lk=g1(Wx′k+b). | (9) |
Here, lk represents latent space, W denotes the 2D weighting matrix, b represents biased vector, and xˊk refers to input noise images.
For a convolutional AE, Eq (9) is transformed to
lk=g1(x′k∗W+b). | (10) |
Now, ∗ symbolizes an operation of 2 D‐image convolution.
2) Latent space: The input image is accessible in compressed format within the latent space between the decoder and encoder.
3) Decoder: The decoder introduces the latent space output as input to rebuild the clear image. Decoding uses convolution up‐sampling and transposed layers, as in Eq (11).
rk=g1(Vl′k+b). | (11) |
Here, rk represents a reconstructed clear image, V refers to a 2D weighting matrix, and b relates to the decoding biased vector. Restructuring Eq (12) for convolutional methods gives
rk=g1(l′k∗V+b). | (12) |
The reconstructed image rk refers to a clean image output of similar size to the images x and x′s. The estimation metrics PSNR and SSIM are calculated approximately on the rebuilt image for the xk ground truth. This decoding creates the recreated image, which is the work of the input image provided by the network, which is smudged using noise. These are likely applying AE methods. Similarly, when normalized images have been applied, the corresponding variables with subscripts of k are liberally substituted using thek ‐norm.
Next, the XAIFTL-AWCDAV method utilizes the LSTO method for hyperparameter tuning [24]. This model is chosen because it can effectively explore complex search spaces, making it highly appropriate for hyperparameter optimization. Unlike conventional optimization techniques, LSTO utilizes Levy flight-based search mechanisms, which help escape local optima and explore global solutions more effectively. This characteristic makes it advantageous when the vast parameter space contains multiple local minima. Furthermore, the LSTO method replicates the foraging behavior of sooty terns, presenting a balance between exploration and exploitation, improving ML models' performance. It is specifically beneficial for fine-tuning hyperparameters in models with nonlinear relationships and large datasets, confirming improved model accuracy and reduced computational cost. Figure 6 represents the working flow of the LSTO model.
The STOA is a naturally stimulated optimizer method, which imitates the immigration and attacking performances of real-world sooty terns. While handling inhibited optimizer difficulties, the STOA uses a statical penalty technique to deal with the restraints. In these techniques, a penalty value can be allocated to every unfeasible solution. This penalty function technique aids in transferring the inhibited optimizer difficulties into unrestrained ones; this facilitates the searching process to discover possible areas. The STOA key values are presented as follows:
Initially, the migrating activities signify the global search process, whereas the search agents in the STOA alter their attack and speed angles in immigration. They upsurge the height by utilizing their wings and pursue the next equation to evade conflicts:
→Cst=SA×→Pst(z). | (13) |
Pst denotes the outdated location, z signifies the number of iterations, and SA is upgraded as:
SA=Cf−(z×(CfMaxiterations)), | (14) |
where z=0,1,2,…,Maxiterations.
After upgrading the location, the present individual adjusts to the optimal individual to attain a superior location:
→Mst=CB×(→Pbst(z)−→Pbst(z)). | (15) |
→Mst represents the comparative distance between the present and the optimal individuals, →Pbst signifies the location of the present optimal individual, and CB is computed as follows:
CB=0.5×Rand. | (16) |
Whereas Rand denotes a randomly generated value in the interval of zero and one. Once the comparative distances and collision‐free locations have been identified, the following stage is to reach the optimum individuals:
→Dst=→Cst+→Mst. | (17) |
In the process of attack, sooty terns create a spiral movement, labeled as
x′=Radius×sin(i), | (18) |
y′=Radius×cos(i), | (19) |
z′=Radius×i, | (20) |
r=u×ekv. | (21) |
Whereas Radius denotes the spiral fight radius, u=v=1 describes the shape of the spiral, and i stands for the angle of the spiral in (zero and 2∗π). In the optimization method, the searching agent unites in the direction of the finest neighbors to upgrade the location.
→Pst(z)=(→Dst×(x′+y′+z′×→Pbst(z). | (22) |
Levy fight (LF) is an arbitrary walk, whereas the lengths of the steps pursue a heavy‐tail of likelihood distribution. This tactic has been utilized in search methods and optimization, especially when the search space can be complex and multidimensional. It assists the method in escaping local optima by infrequently acquiring huge steps that enable it to find novel search space areas. The heavy‐tail step distribution nature shows that although many steps are large, others are small, inducing an offset between exploitation and exploration.
In an LF, the length of step l can be drained from the Levy distribution that contains a strong‐law tail:
P(l)∼|l|−(1+β),0<β≤2. | (23) |
P(1) denotes the functions of probability density for the lth stage. It defines the possibility of incidence at various lengths of the step. l. The step length generally follows a heavy‐tail distribution, denoting that several steps are small, and infrequently elongated ones take place. β denotes the proponent, which manages the distribution and identifies how heavily tailed the Levy distribution is. Usually, the β value is located among [0,2] (0<β≤2). As β approaches one, the distribution turns more severely tailed. The possibility of a longer step increases.
The STOA displays excellent investigative capabilities on multimodal and fixed‐dimensional multimodal testing functions, helping evade the local ideals. Predicting the relocation and attacking performances of real-time sooty terns helps discover the greatest optimization solution. It determines the greater exploitation and exploration abilities while handling various testing functions, allowing it to attain optimum solutions in intricate optimizer issues. However, the STOA can unite to local optima impulsively. While it retains great exploitative and exploratory abilities, its performances can be influenced by random factors and early conditions, causing deficient constancy. Therefore, this study proposes the Levy fight tactic to exchange the global optimum upgrade tactic in the STOA, modifying the effect of random factors and early conditions on optimizer outcomes. The Levy fight strategy can be used in future STOA iterations for the operations of the Levy fight, intensifying the STOA search range and attaining a larger code set.
The LSTO method obtains a fitness function (FF) to achieve classification performances. It illustrates a positive integer to indicate the higher performance of the candidate solutions. This work determined the diminution of the classification error rate, like the FF, as specified in Eq (24).
fitness(xi)=ClassifierErrorRate(xi)=noofmisclassifiedsamplesTotalnoofsamples×100. | (24) |
Finally, to ensure the clearness of the model's predictions, the XAIFTL-AWCDAV method employs XAI techniques using SHAP to interpret and visualize the input of every feature to the model's decision-making method [25,26,27]. XAI methods, such as SHAP, are utilized to interpret and visualize each feature's influence on the model's decision-making process. In this context, XAI improves the transparency of ML models by giving clear explanations for individual predictions, assisting users in understanding how specific features affect results, and offering insights into model behavior. SHAP is a technique applied in explainable AI (XAI) to explain the ML output. It intends to present explainable clarifications for individual predictions produced by composite techniques. The SHAP structure depends on the cooperative game principle, specifically the Shapley values theory. One of the primary benefits of SHAP is its capability to offer local descriptions for individual predictions, allowing users to know why a particular sample was predicted or classified in a specific manner. Moreover, values of SHAP are joined to present global perceptions into feature position and model behavior through the complete dataset.
Regarding SHAP, Shapley values characterize the average marginal input of a feature value to the prediction through each promising feature coalition. These values gain significance for every feature in defining the output of the models. The SHAP values are used in various ML methods like linear, NNs, and tree‐based models. The Shapley value is calculated as:
ϕi(v)=1n!∑R[v(PRi∪{i})−v(PRi)]. | (25) |
Whereas some range across each n! orders R of the players, PRi represents a group of players in N who lead i within order R.
The XAIFTL-AWCDAV model's experimental validation is analyzed using the Detection in Adverse Weather Nature (DAWN) dataset [28]. The dataset consists of 1000 real-world traffic images accumulated under various adverse weather conditions, including fog, snow, rain, and sandstorms. It comprises annotated object bounding boxes for vehicle detection in urban, highway, and freeway environments, aimed at advancing research in AVs and intelligent traffic surveillance systems. Table 1 describes the dataset. Figure 7 portrays the sample images.
Weather | No. of samples |
Fog | 250 |
Snow | 180 |
Rain | 180 |
Sandstorms | 300 |
Total Samples | 910 |
Figure 8 comprises the confusion matrices formed by the XAIFTL-AWCDAV model under different epoch counts. The findings state that the XAIFTL-AWCDAV technique effectively achieves recognition and classification of all four labels.
Table 2 determines the weather condition recognition of the XAIFTL-AWCDAV method under dissimilar epochs, showing that it appropriately identified all four samples. On 500 epoch counts, the XAIFTL-AWCDAV model presents an average accuy of 97.25%, precn of 94.94%, sensy of 93.67%, specy of 98.08%, and F1score of 94.21%. For 1000 epoch counts, it presents an average accuy of 98.90%, precn of 97.77%, sensy of 97.51%, specy of 99.26%, and F1score of 97.63%. For 2000 epochs, it achieves an average accuy of 96.76%, precn of 93.65%, sensy of 92.62%, specy of 97.77%, and F1score of 93.07%. For 3000 epochs, it provides an average accuy of 98.19%, precn of 96.50%, sensy of 95.89%, specy of 98.75%, and F1score of 96.17%.
Class | Accuy | Precn | Sensy | Specy | F1score |
Epoch - 500 | |||||
Fog | 97.25 | 94.12 | 96.00 | 97.73 | 95.05 |
Snow | 98.02 | 96.55 | 93.33 | 99.18 | 94.92 |
Rain | 96.70 | 96.30 | 86.67 | 99.18 | 91.23 |
Sandstorms | 97.03 | 92.79 | 98.67 | 96.23 | 95.64 |
Average | 97.25 | 94.94 | 93.67 | 98.08 | 94.21 |
Epoch - 1000 | |||||
Fog | 98.90 | 97.24 | 98.80 | 98.94 | 98.02 |
Snow | 98.79 | 97.21 | 96.67 | 99.32 | 96.94 |
Rain | 98.79 | 98.29 | 95.56 | 99.59 | 96.90 |
Sandstorms | 99.12 | 98.34 | 99.00 | 99.18 | 98.67 |
Average | 98.90 | 97.77 | 97.51 | 99.26 | 97.63 |
Epoch - 1500 | |||||
Fog | 95.93 | 91.76 | 93.60 | 96.82 | 92.67 |
Snow | 96.92 | 95.24 | 88.89 | 98.90 | 91.95 |
Rain | 94.29 | 88.10 | 82.22 | 97.26 | 85.06 |
Sandstorms | 95.93 | 91.22 | 97.00 | 95.41 | 94.02 |
Average | 95.77 | 91.58 | 90.43 | 97.10 | 90.93 |
Epoch - 2000 | |||||
Fog | 96.59 | 92.28 | 95.60 | 96.97 | 93.91 |
Snow | 97.69 | 96.49 | 91.67 | 99.18 | 94.02 |
Rain | 95.71 | 92.22 | 85.56 | 98.22 | 88.76 |
Sandstorms | 97.03 | 93.61 | 97.67 | 96.72 | 95.60 |
Average | 96.76 | 93.65 | 92.62 | 97.77 | 93.07 |
Epoch - 2500 | |||||
Fog | 97.14 | 93.08 | 96.80 | 97.27 | 94.90 |
Snow | 98.24 | 97.13 | 93.89 | 99.32 | 95.48 |
Rain | 96.48 | 95.68 | 86.11 | 99.04 | 90.64 |
Sandstorms | 97.80 | 94.59 | 99.00 | 97.21 | 96.74 |
Average | 97.42 | 95.12 | 93.95 | 98.21 | 94.44 |
Epoch - 3000 | |||||
Fog | 98.13 | 95.33 | 98.00 | 98.18 | 96.65 |
Snow | 98.35 | 96.61 | 95.00 | 99.18 | 95.80 |
Rain | 98.02 | 97.65 | 92.22 | 99.45 | 94.86 |
Sandstorms | 98.24 | 96.41 | 98.33 | 98.20 | 97.36 |
Average | 98.19 | 96.50 | 95.89 | 98.75 | 96.17 |
Figure 9 shows the training accuy (TRAAC) and validation accuy (VLAAC) values of the XAIFTL-AWCDAV method under different epochs. The accuy rate is estimated for 0–3000 epoch counts. The TRAAC and VLAAC values display an increasing trend related to the capability of the XAIFTL-AWCDAV method to enhance execution over various iterations. Also, TRAAC and VLAAC accuy stay close over the epochs, which shows lower minimum overfitting and displays improved execution of the XAIFTL-AWCDAV approach, promising constant prediction on hidden samples.
Figure 10 presents the TRA loss (TRALS) and VLA loss (VLALS) for the XAIFTL-AWCDAV technique under different epochs. The loss rate is estimated for 0–3000 epoch counts. TRALS and VLALS values demonstrate a decreasing trend, informing the XAIFTL-AWCDAV method's ability to balance a trade-off between generalization and data fitting. Furthermore, the constant reduction in loss rate relates to improved execution of the XAIFTL-AWCDAV method and fine-tuning prediction values over time.
In Figure 11, the precision-recall (PR) curve study of the XAIFTL-AWCDAV model under different epochs shows its execution by plotting precision against recall for all four class labels. The XAIFTL-AWCDAV approach constantly achieves an enhanced PR rate across various classes, representing its capability to preserve a substantial portion of true positive predictions among every positive prediction (precision) while taking a great proportion of actual positives (recall). The continuous upsurge in PR values between all four class labels shows the efficiency of the XAIFTL-AWCDAV approach in the classification process.
In Figure 12, the ROC curve of the XAIFTL-AWCDAV model under different epochs can be analyzed. The findings indicate that the XAIFTL-AWCDAV technique attains improved ROC values across every class label, representing a substantial ability to classify the class labels. This consistent tendency of enhanced ROC outcomes across several class labels indicates the efficient performance of the XAIFTL-AWCDAV technique in predicting class labels, emphasizing the robust nature of the classifier process.
Table 3 shows a comparison between the XAIFTL-AWCDAV technique with other current approaches [29,30]. The XAIFTL-AWCDAV technique outperformed other approaches: it has a greater accuy at 98.90%, whereas the DenseNet121, Stacked ensemble, AlexNet, GoogLeNet, Random Forest, ResNet-50, and ResNet-50-CNN techniques present 81.25%, 86.00%, 95.90%, 97.30%, 96.30%, 98.48%, and 98.50% accuy, respectively. Also, the XAIFTL-AWCDAV model has higher precn (97.77%) than the DenseNet121, Stacked ensemble, AlexNet, GoogLeNet, Random Forest, ResNet-50, and ResNet-50-CNN models (79.23%, 88.59%, 95.27%, 94.37%, 96.77%, 96.80%, and 94.70%, respectively). The XAIFTL-AWCDAV method also has a high F1score (92.65%), whereas the DenseNet121, Stacked ensemble, AlexNet, GoogLeNet, Random Forest, ResNet-50, and ResNet-50-CNN methods obtained F1scores of 83.99%, 88.90%, 95.68%, 94.10%, 95.43%, 94.80%, and 94.66%, respectively.
Model | Accuy | Precn | Sensy | F1score |
DenseNet121 Model | 81.25 | 79.23 | 88.66 | 83.99 |
Stacked ensemble | 86.00 | 88.59 | 80.05 | 88.90 |
AlexNet Classifier | 95.90 | 95.27 | 94.81 | 95.68 |
GoogLeNet Model | 97.30 | 94.37 | 95.29 | 94.10 |
Random Forest | 96.30 | 96.77 | 96.96 | 95.43 |
ResNet-50 Method | 98.48 | 96.80 | 96.84 | 94.80 |
ResNet-50-CNN | 98.50 | 94.70 | 94.41 | 94.66 |
XAIFTL-AWCDAV | 98.90 | 97.77 | 97.51 | 97.63 |
Table 4 shows a comparison between all methods based on their processing time (PT). The XAIFTL-AWCDAV technique shows superior execution, with a minimum PT of 2.37 s; the DenseNet121, Stacked ensemble, AlexNet, GoogLeNet, Random Forest, ResNet-50, and ResNet-50-CNN models achieved higher values of PT: 7.61, 9.51, 8.26, 9.87, 5.91, 15.58, and 10.15 s, respectively.
Model | Processing time (s) |
DenseNet121 Model | 7.61 |
Stacked ensemble | 9.51 |
AlexNet Classifier | 8.26 |
GoogLeNet Model | 9.87 |
Random Forest | 5.91 |
ResNet-50 Method | 15.58 |
ResNet-50-CNN | 10.15 |
XAIFTL-AWCDAV | 2.37 |
In this article, the XAIFTL-AWCDAV method was presented to detect and classify weather conditions for AVs in challenging scenarios. In the preprocessing stage, the XAIFTL-AWCDAV method utilized the NLM method to prevent and reduce noise. Three models, EfficientNet, SqueezeNet, and MobileNetv2, were employed for feature extraction. The DAE technique was utilized to classify adverse weather conditions. Next, the hyperparameter selection of the DAE model was performed using the LSTO method. Finally, to ensure the clarity of the model's predictions, the XAIFTL-AWCDAV method employed XAI techniques using SHAP to interpret and visualize the input of every feature to the model's decision-making process. The efficiency of the XAIFTL-AWCDAV method was validated by comprehensive studies using a benchmark dataset. The numerical results show that the XAIFTL-AWCDAV method obtained a superior value over recent techniques at 98.90%. The limitations of the XAIFTL-AWCDAV method lie on its reliance on a single dataset for model training and evaluation, which may affect the generalization of the results to other clinical settings. Additionally, the performance of the model could be further improved by integrating more diverse data from various institutions or geographical locations. The study also does not explore the impact of real-time data integration, which could enhance the robustness of the model in clinical environments. Future studies may concentrate on expanding the dataset to comprise a wide range of pathological images and integrating multimodal data sources such as patient demographics and genetic data. Furthermore, optimization of the computational efficiency of the model will be a priority to enable its practical deployment in clinical practice.
Khaled Tarmissi, Hanan Abdullah Mengash: Conceptualization; Noha Negm, Ali M. Al-Sharafi: Methodology; Noha Negm: Software, supervision, resources; Khaled Tarmissi, Yahia Said: Validation; Ali M. Al-Sharafi: Formal analysis, data curation; Hanan Abdullah Mengash: Investigation, visualization, project administration; Khaled Tarmissi, Hanan Abdullah Mengash and Yahia Said: Writing—original draft preparation; Yahia Said, Noha Negm, Ali M. Al-Sharafi, and Khaled Tarmissi: Writing—review and editing; Noha Negm, Hanan Abdullah Mengash, and Yahia Said: Funding acquisition. All authors have read and agreed to the published version of the manuscript.
The authors declare they have not used Artificial Intelligence (AI) tools in the creation of this article.
The authors extend their appreciation to the Deanship of Research and Graduate Studies at King Khalid University for funding this work through Large Research Project under grant number RGP2/86/45. Princess Nourah bint Abdulrahman University Researchers Supporting Project number (PNURSP2024R114), Princess Nourah bint Abdulrahman University, Riyadh, Saudi Arabia. The authors extend their appreciation to the Deanship of Scientific Research at Northern Border University, Arar, KSA for funding this research work through the project number "NBU-FPEJ-2024-3030-03. The authors are thankful to the Deanship of Graduate Studies and Scientific Research at University of Bisha for supporting this work through the Fast-Track Research Support Program.
The authors declare no conflict of interest.
The data supporting this study's findings are openly available: https://data.mendeley.com/datasets/766ygrbt8y/3 [28].
[1] |
M. Hassaballah, M. A. Kenk, K. Muhammad, S. Minaee, Vehicle detection and tracking in adverse weather using a deep learning framework, IEEE Trans. Intell. Transp. Syst., 22 (2020), 4230–4242. https://doi.org/10.1109/TITS.2020.3014013 doi: 10.1109/TITS.2020.3014013
![]() |
[2] | M. A. Kenk, M. Hassaballah, DAWN: Vehicle detection in adverse weather nature dataset, arXiv preprint, 2020. |
[3] |
D. Wang, J. G. Wang, K. Xu, Deep learning for object detection, classification and tracking in industry applications, Sensors, 21 (2021), 7349. https://doi.org/10.3390/s21217349 doi: 10.3390/s21217349
![]() |
[4] |
J. Vargas, S. Alsweiss, O. Toker, R. Razdan, J. Santos, An overview of autonomous vehicles sensors and their vulnerability to weather conditions, Sensors, 21 (2021), 5397. https://doi.org/10.3390/s21165397 doi: 10.3390/s21165397
![]() |
[5] |
Y. Zhang, A. Carballo, H. Yang, K. Takeda, Perception and sensing for autonomous vehicles under adverse weather conditions: A survey, ISPRS J. Photogramm., 196 (2023), 146−177. https://doi.org/10.1016/j.isprsjprs.2022.12.021 doi: 10.1016/j.isprsjprs.2022.12.021
![]() |
[6] | A. Balasubramaniam, S. Pasricha, Object detection in autonomous vehicles: Status and open challenges, arXiv preprint, 2022. |
[7] | A. Z. Abualkishik, R. Almajed, W. Thompson, Multi-attribute decision-making method for prioritizing autonomous vehicles in real-time traffic management: Towards active sustainable transport, Int. J. Wirel. Ad Hoc Commun., 3 (2021), 91−101. https://doi.org/10.54216/IJWAC.030204 |
[8] |
A. S. Mohammed, A. Amamou, F. K. Ayevide, S. Kelouwani, K. Agbossou, N. Zioui, The perception system of intelligent ground vehicles in all weather conditions: A systematic literature review, Sensors, 20 (2020), 6532. https://doi.org/10.3390/s20226532 doi: 10.3390/s20226532
![]() |
[9] | J. Li, R. Xu, J. Ma, Q. Zou, J. Ma, H. Yu, Domain adaptive object detection for autonomous driving under foggy weather, In: Proceedings of the IEEE/CVF Winter Conference on Applications of Computer Vision, 2023,612−622. https://doi.org/10.1109/WACV56688.2023.00068 |
[10] |
M. Hasanujjaman, M. Z. Chowdhury, M. T. Hossan, Y. M. Jang, Autonomous vehicle driving in harsh weather: Adaptive fusion alignment modeling and analysis, Arab. J. Sci. Eng., 49 (2024), 6631−6640. https://doi.org/10.1007/s13369-023-08389-1 doi: 10.1007/s13369-023-08389-1
![]() |
[11] |
E. Q. Appiah, S. Mensah, Object detection in adverse weather conditions for autonomous vehicles, Multimed. Tools Appl., 83 (2024), 28235−28261. https://doi.org/10.1007/s11042-023-16453-z doi: 10.1007/s11042-023-16453-z
![]() |
[12] | N. U. A. Tahir, Z. Zhang, M. Asim, J. Chen, M. El Affendi, Object detection in autonomous vehicles under adverse weather: A review of traditional and deep learning approaches, Algorithms, 17 (2024), 103. https://doi.org/10.3390/a17030103 |
[13] |
A. Vellaidurai, M. Rathinam, A novel oyolov5 model for vehicle detection and classification in adverse weather conditions, Multimed. Tools Appl., 83 (2024), 25037−25054. https://doi.org/10.1007/s11042-023-16450-2 doi: 10.1007/s11042-023-16450-2
![]() |
[14] |
M. Carvalho, S. Moradi, F. Hosseinnouri, K. Keshavan, E. Villeneuve, I. Gultepe, et al., Towards a model of snow accretion for autonomous vehicles, Atmosphere, 15 (2024), 548. https://doi.org/10.3390/atmos15050548 doi: 10.3390/atmos15050548
![]() |
[15] |
L. Wen, Y. Peng, M. Lin, N. Gan, R. Tan, Multi-modal contrastive learning for LiDAR point cloud rail-obstacle detection in complex weather, Electronics, 13 (2024), 220. https://doi.org/10.3390/electronics13010220 doi: 10.3390/electronics13010220
![]() |
[16] |
Z. Zhu, X. Li, J. Zhai, H. Hu, PODB: A learning-based polarimetric object detection benchmark for road scenes in adverse weather conditions, Inform. Fusion, 108 (2024), 102385. https://doi.org/10.1016/j.inffus.2024.102385 doi: 10.1016/j.inffus.2024.102385
![]() |
[17] | M. Kondapally, K. N. Kumar, C. Vishnu, C. K. Mohan, Towards a transitional weather scene recognition approach for autonomous vehicles, IEEE T. Intell. Transp., 2023. https://doi.org/10.1109/TITS.2023.3331882 |
[18] |
G. Hou, Evaluating efficiency and safety of mixed traffic with connected and autonomous vehicles in adverse weather, Sustainability, 15 (2023), 3138. https://doi.org/10.3390/su15043138 doi: 10.3390/su15043138
![]() |
[19] |
Z. Chen, H. Zeng, Y. Wang, W. Yang, Y. Guan, W. Liu, A texture enhancement method for oceanic internal wave synthetic aperture radar images based on non-local mean filtering and texture layer enhancement, Remote Sens., 16 (2024), 1172. https://doi.org/10.3390/rs16071172 doi: 10.3390/rs16071172
![]() |
[20] |
G. M. S. Himel, M. M. Islam, M. Rahaman, Utilizing EfficientNet for sheep breed identification in low-resolution images, Syst. Soft Comput., 6 (2024), 200093. https://doi.org/10.1016/j.sasc.2024.200093 doi: 10.1016/j.sasc.2024.200093
![]() |
[21] |
S. Singh, P. K. Jain, N. Sharma, M. Pohit, S. Roy, Atherosclerotic plaque classification in carotid ultrasound images using machine learning and explainable deep learning, Intel. Med., 4 (2024), 83−95. https://doi.org/10.1016/j.imed.2023.05.003 doi: 10.1016/j.imed.2023.05.003
![]() |
[22] | G. Zhou, Q. He, X. Liu, X. Kai, W. Cao, J. Ding, et al., Optimizing MobileNetV2 for improved accuracy in early gastric cancer detection based on dynamic pelican optimizer, Heliyon, 10 (2024). https://doi.org/10.1016/j.heliyon.2024.e35854 |
[23] |
R. Ramakotti, S. Paneerselvam, An architecture-oriented analysis of stacked denoising autoencoders, Proc. Comput. Sci., 235 (2024), 2154−2166. https://doi.org/10.1016/j.procs.2024.04.204 doi: 10.1016/j.procs.2024.04.204
![]() |
[24] |
J. Zhang, Levy sooty tern optimization algorithm builds DNA storage coding sets for random access, Entropy, 26 (2024), 778. https://doi.org/10.3390/e26090778 doi: 10.3390/e26090778
![]() |
[25] |
A. M. Salih, Z. Raisi‐Estabragh, I. B. Galazzo, P. Radeva, S. E. Petersen, K. Lekadir, et al., A perspective on explainable artificial intelligence methods: SHAP and LIME, Adv. Intell. Syst., 2024. https://doi.org/10.1002/aisy.202400304 doi: 10.1002/aisy.202400304
![]() |
[26] |
J. Ukwaththa, S. Herath, D. P. P. Meddage, A review of machine learning (ML) and explainable artificial intelligence (XAI) methods in additive manufacturing (3D printing), Mater. Today Commun., 2024. https://doi.org/10.1016/j.mtcomm.2024.110294 doi: 10.1016/j.mtcomm.2024.110294
![]() |
[27] |
D. P. P. Meddage, I. Fonseka, D. Mohotti, K. Wijesooriya, C. K. Lee, An explainable machine learning approach to predict the compressive strength of graphene oxide-based concrete, Constr. Build. Mater., 449 (2024), 138346. https://doi.org/10.1016/j.conbuildmat.2024.138346 doi: 10.1016/j.conbuildmat.2024.138346
![]() |
[28] | https://data.mendeley.com/datasets/766ygrbt8y/3 |
[29] |
Q. A. Al-Haija, M. Gharaibeh, A. Odeh, Detection in adverse weather conditions for autonomous vehicles via deep learning, Ai, 3 (2022), 303−317. https://doi.org/10.3390/ai3020019 doi: 10.3390/ai3020019
![]() |
[30] |
M. N. Khan, M. M. Ahmed, Weather and surface condition detection based on road-side webcams: Application of pre-trained convolutional neural network, Int. J. Transp. Sci. Tec., 11 (2022), 468−483. https://doi.org/10.1016/j.ijtst.2021.06.003 doi: 10.1016/j.ijtst.2021.06.003
![]() |
Weather | No. of samples |
Fog | 250 |
Snow | 180 |
Rain | 180 |
Sandstorms | 300 |
Total Samples | 910 |
Class | Accuy | Precn | Sensy | Specy | F1score |
Epoch - 500 | |||||
Fog | 97.25 | 94.12 | 96.00 | 97.73 | 95.05 |
Snow | 98.02 | 96.55 | 93.33 | 99.18 | 94.92 |
Rain | 96.70 | 96.30 | 86.67 | 99.18 | 91.23 |
Sandstorms | 97.03 | 92.79 | 98.67 | 96.23 | 95.64 |
Average | 97.25 | 94.94 | 93.67 | 98.08 | 94.21 |
Epoch - 1000 | |||||
Fog | 98.90 | 97.24 | 98.80 | 98.94 | 98.02 |
Snow | 98.79 | 97.21 | 96.67 | 99.32 | 96.94 |
Rain | 98.79 | 98.29 | 95.56 | 99.59 | 96.90 |
Sandstorms | 99.12 | 98.34 | 99.00 | 99.18 | 98.67 |
Average | 98.90 | 97.77 | 97.51 | 99.26 | 97.63 |
Epoch - 1500 | |||||
Fog | 95.93 | 91.76 | 93.60 | 96.82 | 92.67 |
Snow | 96.92 | 95.24 | 88.89 | 98.90 | 91.95 |
Rain | 94.29 | 88.10 | 82.22 | 97.26 | 85.06 |
Sandstorms | 95.93 | 91.22 | 97.00 | 95.41 | 94.02 |
Average | 95.77 | 91.58 | 90.43 | 97.10 | 90.93 |
Epoch - 2000 | |||||
Fog | 96.59 | 92.28 | 95.60 | 96.97 | 93.91 |
Snow | 97.69 | 96.49 | 91.67 | 99.18 | 94.02 |
Rain | 95.71 | 92.22 | 85.56 | 98.22 | 88.76 |
Sandstorms | 97.03 | 93.61 | 97.67 | 96.72 | 95.60 |
Average | 96.76 | 93.65 | 92.62 | 97.77 | 93.07 |
Epoch - 2500 | |||||
Fog | 97.14 | 93.08 | 96.80 | 97.27 | 94.90 |
Snow | 98.24 | 97.13 | 93.89 | 99.32 | 95.48 |
Rain | 96.48 | 95.68 | 86.11 | 99.04 | 90.64 |
Sandstorms | 97.80 | 94.59 | 99.00 | 97.21 | 96.74 |
Average | 97.42 | 95.12 | 93.95 | 98.21 | 94.44 |
Epoch - 3000 | |||||
Fog | 98.13 | 95.33 | 98.00 | 98.18 | 96.65 |
Snow | 98.35 | 96.61 | 95.00 | 99.18 | 95.80 |
Rain | 98.02 | 97.65 | 92.22 | 99.45 | 94.86 |
Sandstorms | 98.24 | 96.41 | 98.33 | 98.20 | 97.36 |
Average | 98.19 | 96.50 | 95.89 | 98.75 | 96.17 |
Model | Accuy | Precn | Sensy | F1score |
DenseNet121 Model | 81.25 | 79.23 | 88.66 | 83.99 |
Stacked ensemble | 86.00 | 88.59 | 80.05 | 88.90 |
AlexNet Classifier | 95.90 | 95.27 | 94.81 | 95.68 |
GoogLeNet Model | 97.30 | 94.37 | 95.29 | 94.10 |
Random Forest | 96.30 | 96.77 | 96.96 | 95.43 |
ResNet-50 Method | 98.48 | 96.80 | 96.84 | 94.80 |
ResNet-50-CNN | 98.50 | 94.70 | 94.41 | 94.66 |
XAIFTL-AWCDAV | 98.90 | 97.77 | 97.51 | 97.63 |
Model | Processing time (s) |
DenseNet121 Model | 7.61 |
Stacked ensemble | 9.51 |
AlexNet Classifier | 8.26 |
GoogLeNet Model | 9.87 |
Random Forest | 5.91 |
ResNet-50 Method | 15.58 |
ResNet-50-CNN | 10.15 |
XAIFTL-AWCDAV | 2.37 |
Weather | No. of samples |
Fog | 250 |
Snow | 180 |
Rain | 180 |
Sandstorms | 300 |
Total Samples | 910 |
Class | Accuy | Precn | Sensy | Specy | F1score |
Epoch - 500 | |||||
Fog | 97.25 | 94.12 | 96.00 | 97.73 | 95.05 |
Snow | 98.02 | 96.55 | 93.33 | 99.18 | 94.92 |
Rain | 96.70 | 96.30 | 86.67 | 99.18 | 91.23 |
Sandstorms | 97.03 | 92.79 | 98.67 | 96.23 | 95.64 |
Average | 97.25 | 94.94 | 93.67 | 98.08 | 94.21 |
Epoch - 1000 | |||||
Fog | 98.90 | 97.24 | 98.80 | 98.94 | 98.02 |
Snow | 98.79 | 97.21 | 96.67 | 99.32 | 96.94 |
Rain | 98.79 | 98.29 | 95.56 | 99.59 | 96.90 |
Sandstorms | 99.12 | 98.34 | 99.00 | 99.18 | 98.67 |
Average | 98.90 | 97.77 | 97.51 | 99.26 | 97.63 |
Epoch - 1500 | |||||
Fog | 95.93 | 91.76 | 93.60 | 96.82 | 92.67 |
Snow | 96.92 | 95.24 | 88.89 | 98.90 | 91.95 |
Rain | 94.29 | 88.10 | 82.22 | 97.26 | 85.06 |
Sandstorms | 95.93 | 91.22 | 97.00 | 95.41 | 94.02 |
Average | 95.77 | 91.58 | 90.43 | 97.10 | 90.93 |
Epoch - 2000 | |||||
Fog | 96.59 | 92.28 | 95.60 | 96.97 | 93.91 |
Snow | 97.69 | 96.49 | 91.67 | 99.18 | 94.02 |
Rain | 95.71 | 92.22 | 85.56 | 98.22 | 88.76 |
Sandstorms | 97.03 | 93.61 | 97.67 | 96.72 | 95.60 |
Average | 96.76 | 93.65 | 92.62 | 97.77 | 93.07 |
Epoch - 2500 | |||||
Fog | 97.14 | 93.08 | 96.80 | 97.27 | 94.90 |
Snow | 98.24 | 97.13 | 93.89 | 99.32 | 95.48 |
Rain | 96.48 | 95.68 | 86.11 | 99.04 | 90.64 |
Sandstorms | 97.80 | 94.59 | 99.00 | 97.21 | 96.74 |
Average | 97.42 | 95.12 | 93.95 | 98.21 | 94.44 |
Epoch - 3000 | |||||
Fog | 98.13 | 95.33 | 98.00 | 98.18 | 96.65 |
Snow | 98.35 | 96.61 | 95.00 | 99.18 | 95.80 |
Rain | 98.02 | 97.65 | 92.22 | 99.45 | 94.86 |
Sandstorms | 98.24 | 96.41 | 98.33 | 98.20 | 97.36 |
Average | 98.19 | 96.50 | 95.89 | 98.75 | 96.17 |
Model | Accuy | Precn | Sensy | F1score |
DenseNet121 Model | 81.25 | 79.23 | 88.66 | 83.99 |
Stacked ensemble | 86.00 | 88.59 | 80.05 | 88.90 |
AlexNet Classifier | 95.90 | 95.27 | 94.81 | 95.68 |
GoogLeNet Model | 97.30 | 94.37 | 95.29 | 94.10 |
Random Forest | 96.30 | 96.77 | 96.96 | 95.43 |
ResNet-50 Method | 98.48 | 96.80 | 96.84 | 94.80 |
ResNet-50-CNN | 98.50 | 94.70 | 94.41 | 94.66 |
XAIFTL-AWCDAV | 98.90 | 97.77 | 97.51 | 97.63 |
Model | Processing time (s) |
DenseNet121 Model | 7.61 |
Stacked ensemble | 9.51 |
AlexNet Classifier | 8.26 |
GoogLeNet Model | 9.87 |
Random Forest | 5.91 |
ResNet-50 Method | 15.58 |
ResNet-50-CNN | 10.15 |
XAIFTL-AWCDAV | 2.37 |