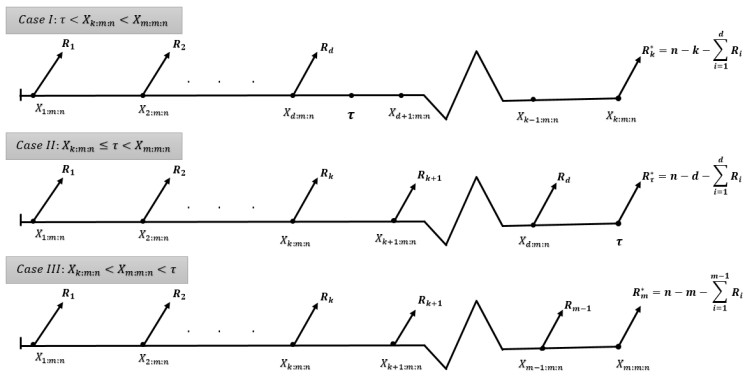
The competing risk (CR) model is crucial for studying various areas, such as biology, econometrics, and engineering. When multiple factors could cause a product to fail, these factors often work against each other, resulting in the product's failure. This scenario is known as the CR problem. This study focused on parameter estimation of the generalized Lomax distribution under a generalized progressive hybrid censoring scheme in the presence of CR when the cause of failure for each item was known and independent. Both maximum likelihood (ML) and Bayesian approaches were used to estimate the unknown parameters, reliability characteristics, and relative risks due to two causes. Bayesian estimators under gamma priors with different loss functions were generated using Markov chain Monte Carlo, and confidence intervals (CIs) were generated using the ML estimation method. Additionally, two bootstrap CIs for the unknown parameters were presented. According to the conditional posterior distribution, credible intervals and the highest posterior density intervals were further generated. The performance of different estimators was compared using Monte Carlo simulation, and real-data applications were used to verify the proposed estimates.
Citation: Amal Hassan, Sudhansu Maiti, Rana Mousa, Najwan Alsadat, Mahmoued Abu-Moussa. Analysis of competing risks model using the generalized progressive hybrid censored data from the generalized Lomax distribution[J]. AIMS Mathematics, 2024, 9(12): 33756-33799. doi: 10.3934/math.20241611
[1] | Hanan Haj Ahmad, Ehab M. Almetwally, Dina A. Ramadan . A comparative inference on reliability estimation for a multi-component stress-strength model under power Lomax distribution with applications. AIMS Mathematics, 2022, 7(10): 18050-18079. doi: 10.3934/math.2022994 |
[2] | Samah M. Ahmed, Abdelfattah Mustafa . Estimation of the coefficients of variation for inverse power Lomax distribution. AIMS Mathematics, 2024, 9(12): 33423-33441. doi: 10.3934/math.20241595 |
[3] | Abdulhakim A. Al-Babtain, Rehan A. K. Sherwani, Ahmed Z. Afify, Khaoula Aidi, M. Arslan Nasir, Farrukh Jamal, Abdus Saboor . The extended Burr-R class: properties, applications and modified test for censored data. AIMS Mathematics, 2021, 6(3): 2912-2931. doi: 10.3934/math.2021176 |
[4] | Mohamed S. Eliwa, Essam A. Ahmed . Reliability analysis of constant partially accelerated life tests under progressive first failure type-II censored data from Lomax model: EM and MCMC algorithms. AIMS Mathematics, 2023, 8(1): 29-60. doi: 10.3934/math.2023002 |
[5] | Magdy Nagy, Khalaf S. Sultan, Mahmoud H. Abu-Moussa . Analysis of the generalized progressive hybrid censoring from Burr Type-Ⅻ lifetime model. AIMS Mathematics, 2021, 6(9): 9675-9704. doi: 10.3934/math.2021564 |
[6] | Essam A. Ahmed, Laila A. Al-Essa . Inference of stress-strength reliability based on adaptive progressive type-Ⅱ censing from Chen distribution with application to carbon fiber data. AIMS Mathematics, 2024, 9(8): 20482-20515. doi: 10.3934/math.2024996 |
[7] | Tahani A. Abushal, Alaa H. Abdel-Hamid . Inference on a new distribution under progressive-stress accelerated life tests and progressive type-II censoring based on a series-parallel system. AIMS Mathematics, 2022, 7(1): 425-454. doi: 10.3934/math.2022028 |
[8] | Refah Alotaibi, Mazen Nassar, Zareen A. Khan, Ahmed Elshahhat . Analysis of Weibull progressively first-failure censored data with beta-binomial removals. AIMS Mathematics, 2024, 9(9): 24109-24142. doi: 10.3934/math.20241172 |
[9] | Ammar M. Sarhan, Rabab S. Gomaa, Alia M. Magar, Najwan Alsadat . Bivariate exponentiated generalized inverted exponential distribution with applications on dependent competing risks data. AIMS Mathematics, 2024, 9(10): 29439-29473. doi: 10.3934/math.20241427 |
[10] | Abdulhakim A. Al-Babtain, Ibrahim Elbatal, Christophe Chesneau, Mohammed Elgarhy . On a new modeling strategy: The logarithmically-exponential class of distributions. AIMS Mathematics, 2021, 6(7): 7845-7871. doi: 10.3934/math.2021456 |
The competing risk (CR) model is crucial for studying various areas, such as biology, econometrics, and engineering. When multiple factors could cause a product to fail, these factors often work against each other, resulting in the product's failure. This scenario is known as the CR problem. This study focused on parameter estimation of the generalized Lomax distribution under a generalized progressive hybrid censoring scheme in the presence of CR when the cause of failure for each item was known and independent. Both maximum likelihood (ML) and Bayesian approaches were used to estimate the unknown parameters, reliability characteristics, and relative risks due to two causes. Bayesian estimators under gamma priors with different loss functions were generated using Markov chain Monte Carlo, and confidence intervals (CIs) were generated using the ML estimation method. Additionally, two bootstrap CIs for the unknown parameters were presented. According to the conditional posterior distribution, credible intervals and the highest posterior density intervals were further generated. The performance of different estimators was compared using Monte Carlo simulation, and real-data applications were used to verify the proposed estimates.
Studies on lifetime testing focused on the specific causes of failure. However, recent lifetime measurement models have been built upon the concept of multiple competing factors leading to the failure of lifetime testing units. These contemporary models for estimating lifetimes consider various competing causes of failure that challenge the durability of lifetime testing units.
Competing risks (CR) analysis is commonly used in medical research, reliability engineering, and econometric sciences to investigate outcomes in which numerous alternative events may affect the path to the primary event. For example, in a lifetime experiment conducted by Boag [1], the cause of death was recorded as either breast cancer or another cancer type, demonstrating that multiple factors can contribute to a subject's death or failure. In the current context, individuals infected with COVID-19 may also face other health complications, potentially resulting in death due to one of these underlying conditions. These models are referred to as CR models. For a more comprehensive understanding of CR, refer to Pintilie[2] and Chen et al. [3].
In reliability engineering, the failure times of components with numerous possible failure modes are analyzed (for example, a machine that can fail owing to wear, electrical problems, or other factors). In another application, using the brake system, Liu et al.[4] where found the brake system of a racing car consists of a pedal, a balance lever, a main cylinder, and a brake which is considered as CR.
In econometrics or social sciences, let us say that you are researching how long people work in a certain industry. Some conflicting hazards in this situation could end the employment period, including voluntary resignation, such as when someone leaves to look for work elsewhere, retirement, illness, or disability, meaning that situation can be treated using CR.
CR models are statistical tools designed to analyze scenarios where multiple events can occur concurrently or sequentially, potentially influencing each other's probabilities. These models find widespread application in fields, like medicine, engineering, and economics, where various factors can contribute to an outcome. CR models and uncertainty are closely intertwined. While CR models focus on the simultaneous or sequential occurrence of multiple events, uncertainty refers to the lack of complete knowledge about future events. Uncertainty can significantly impact CR models, arising from factors such as insufficient or biased data, model assumptions that may not accurately reflect reality, and unforeseen events that disrupt expected patterns. For further details, please refer to [5,6,7].
In various industries and businesses, lifetime tests are commonly employed. Because these tests can be costly and time-consuming, statisticians devised alternative procedures known as censored samples, which allow experimenters to stop work before all units fail. As a result, things are often checked using various censoring systems, with types I and II being the most popular. In such cases, removing live units from the testing process is not an option, resulting in the design of a more adaptable and progressive type-II censoring scheme (PTIICS), which marks a significant advancement in the practice of censorship and practical application. To understand the PTIICS approach and implementation comprehensively, it has been examined by Balakrishnan [8], and Ng and Chan [9].
This subsection shows one type of censoring scheme called the generalized progressive hybrid censoring scheme (GPHCS), introduced by Cho et al. [10] to overcome the problems with type-I progressive hybrid censoring schemes. It has also been proposed by Kundu and Joarder[11], a mixture of type-I HCS and PTIICS since HCS combines type-I and type-II censoring schemes.
This technique would prefer to allow the experiment to continue after a predetermined time if very few failures have been noticed up to that time. So we assumed a predetermined minimum of k failures, and the experimenter would ideally like to observe m failures but is willing to accept a bare minimum of k(k<m) failures.
The GPHCS can be depicted as follows: If n∈N identical items X1,X2,...,Xn are placed on a test and τ∈(0,∞) is a predetermined time, with integers k and m being predetermined such that k is the minimum number of failing items allowed since k,m∈{1,2,...,n} and (R1,R2,...,Rm) are predetermined integers such that
m∑i=1Ri+m=n, |
then at the time of each failure Xi:m:n, Ri, i=1,2,…,m, the remaining units are randomly removed. This process continues until the termination time
T∗=max(Xk:m:n,min(Xm:m:n,τ)) |
when all remaining units are removed from the experiment. Under this setup, the experimenter would ideally like to observe m failures but would be willing to accept a minimum of k failures. The number of observed failures until time τ is denoted by d. We noted the observed data and survival items removed in the GPHCS categories, which have one of the three possible types:
X_′={{(X1:m:n,R1),(X2:m:n,R2),…,(Xk:m:n,R∗k)},ifτ<Xk:m:n<Xm:m:n,{(X1:m:n,R1),(X2:m:n,R2),…,(Xd:m:n,Rd)},ifXk:m:n<τ<Xm:m:n,{(X1:m:n,R1),(X2:m:n,R2),…,(Xm:m:n,R∗m)},ifXk:m:n<Xm:m:n<τ. | (1.1) |
From Eq (1.1), we make one of the following observations:
For Case I, Xd:m:n<τ<Xd+1:m:n<...<Xk:m:n, Rd+1=...=Rk−1=0 and
R∗k=n−k−d∑i=1Ri. |
For Case II, Xd:m:n<τ<Xd+1:m:n<...<Xm:m:n and Xd+1:m:n,...,Xm;m:n are not observed and are at time τ, which observed some survival items R∗τ since
R∗τ=n−d−d∑i=1Ri. |
For Case III,
R∗m=n−m−m−1∑i=1Ri |
is the number of remaining units left at time Xm:m:n.
Figure 1 shows a graphical description of the GPHCS.
A survey of the literature on lifetime distributions with CR under various censorship schemes is suggested in this subsection. As shown in Table 1, some researchers considered the estimation problem based on CR under censoring data.
Authors | Related works |
Liao and Gui [12] | Discussed the inference for Rayleigh distribution in the presence of CR under PTIICS. |
Wang et al. [13] | Examined the inference for the Weibull CR model with partially observed failure causes under GPHCS. |
Almarashi et al. [14] | Investigated the statistical analysis of Nadarajaha-Haghighi distribution's CR lifetime under type-II censoring. |
Mahmoud et al. [15] | Studied the progressive of the type-I censoring scheme under CR, focusing specifically on the case where the lifetimes of the subjects follow a generalized inverted exponential distribution. |
El-Raheem et al. [16] | Discussed the point and interval statistical inference of the extension of the exponential distribution's parameters under CR data with PTIICS. |
Abushal et al. [17] | Studied the inference of Lomax CR model with partially observed failure causes under the type-II |
generalized hybrid censoring scheme. | |
Qin and Gui [18] | Analyzed CR from Lomax distribution based on adaptive progressive type-II hybrid censored. |
Hassan et al. [19] | Evaluated CR from the analysis of the inverted Topp-Leone distribution under PTIICS. |
Ahmed and Nassar[20] | Examined electrode data analysis within the framework of the Weibull lifetime CR model in the context of improved adaptive progressive censoring. |
Hassen et al.[21] | Studied the Bayesian statistical inference of the generalized inverted exponential distribution through the CR model under GPHCS. |
Tian et al.[22] | Examined the implications of the inverted exponential Rayleigh distribution using the CR model when |
employing optimal PTIICS. | |
Lv et al.[23] | Explored the process of inferring statistics through the CR sample when subjects' lifetimes follow the |
Gompertz distribution in the context of general PTIICS. | |
Salem et al.[24] | Obtained the maximum likelihood estimates (MLEs) and Bayesian estimates (BEs) from the Weibull distribution based on the censored Generalized Progressive Hybrid type-II in the presence of the CR model. |
This study aims to analyze the generalized Lomax distribution (GLD) under the GPHCS scheme in the context of the CR model. The GPHCS method is increasingly popular due to its potential to reduce time and cost in life-testing experiments significantly. Moreover, the GLD's versatility has led to its wide application across various fields. Figure 2 presents a visual breakdown of the methodological framework employed in this study. The core objectives of this study are:
(1) Analyze the GLD's parameters through CR based on the GPHCS by using maximum likelihood (ML) and Bayesian approaches.
(2) Acquire the asymptotic two-sided confidence intervals (CIs) and two bootstrap CIs for GLD parameters.
(3) Explain the Bayesian estimation of the GLD's parameters using an independent gamma prior distribution under three loss functions.
(4) Use the Markov chain Monte Carlo (MCMC) algorithm, to generate the Bayesian credible intervals (BCIs) and the highest posterior density (HPD) intervals.
(5) Evaluate the performance of the suggested estimators with different GPHC schemes through a simulation study and real data.
The remaining sections of this article are arranged as follows: Section 2 formulates the model. In Section 3, the maximum likelihood estimates (MLEs) for the parameters, the reliability characteristics, and the relative risks are obtained. Additionally, the Bayesian estimates (BEs) based on the linear exponential (LINEX), generalized entropy (GE), and squared error loss functions are presented. The MCMC technique is used to create the BCI and HPD intervals. Asymptotic CIs (ACIs), Bootstrap CIs, and other significant functions of the model parameters are given in Section 4. Section 5 shows the performance of the suggested estimates by comparison through mean squared error (MSE). Section 6 describes a practical application. The study's conclusion and future research are offered in Section 7. Finally, two algorithms used and tables of numerical work results in the present article are included in Appendixes A and B, respectively.
Suppose that n∈N identical components X1,X2,...,Xn are put on a GPHC life test in the presence of CR. Each component is exposed to two risks. We have
Xi=min(X1i,X2i); ∀i=1,2,...,n, |
where X1i and X2i are the latent failure times and the pairs (X1i,X2i) are assumed to be distributed independently and identically (i.i.d). Now, the consequential data in the presence of CR under the GPHC experiment is shown:
X_={{(X1:m:n,δ1,R1),(X2:m:n,δ2,R2),...,(Xk:m:n,δk,R∗k)},ifτ<Xk:m:n<Xm:m:n,{(X1:m:n,δ1,R1),(X2:m:n,δ2,R2),...,(Xd:m:n,δd,Rd)},ifXk:m:n<τ<Xm:m:n,(X1:m:n,δ1,R1),(X2:m:n,δ2,R2),...,(Xm:m:n,δm,R∗m), ifXk:m:n<Xm:m:n<τ, | (2.1) |
where δi=1 when X1i<X2i (failure from Cause 1) or δi=2 when X1i>X2i (failure from Cause 2); ∀i=1,2,...,n.
The GLD, a widely used power-type heavy-tailed model, has been employed in numerous applied problems. Specifically, it was utilized to analyze business failure data. The GLD is a Lomax generalization proposed by Maurya et al. [25]. Researchers have employed various generalizations of the Lomax distribution in studies for modeling business records to reliability and lifetime testing, as shown in Hassan et al.[26] (for more details about the study of GLD, see Alghamdi[27]).
The GLD is derived by using the power transformation method of Box and Cox [28]. This distribution has three parameters: only one shape parameter αj;j=1,2, and the common other parameters β,γ>0 are scale and shape, respectively. The following are the probability density function (PDF) and the cumulative distribution function (CDF) for the GLD:
f(x;αj,β,γ)=αjβγxγ−1(1+βxγ)−(αj+1)andF(x;αj,β,γ)=1−(1+βxγ)−αj, | (2.2) |
respectively. The GLD's PDF is denoted by GLD(αj,β,γ), where j=1,2 and if γ is less than or more than 1, the PDFs may exhibit a decrease in value or a unimodal form. For β=1 and γ=1, it leads to Burr XII and Lomax distributions, respectively. In numerous cases, the GLD often proves to be more appropriate than the Burr XII, transmuted Burr III, and Burr III distributions, as shown by Maurya et al. [25].
Remark 1. If the latent failure times X1i and X2i are i.i.d random variables following GLD(α1,β,γ) and GLD(α2,β,γ), respectively, then the random variable
Xi=min(X1i,X2i) |
follows GLD(α1+α2,β,γ), where β,γ are the scale and shape parameters and (α1+α2) is the shape parameter.
Applying Remark 1, the reliability and hazard function of the random variable Xi, referred to as ℜ(t) and ℏ(t), respectively, are provided by
ℜ(t)=P(Xi>t)=P(X1i>t)P(X2i>t)=(1+βtγ)−(α1+α2), | (2.3) |
ℏ(t)=(α1+α2)γβtγ−1(1+βtγ)−1. | (2.4) |
Remark 2. The relative risks due to Causes 1 and 2 are determined using the independence of the latent failure times, and they are as follows:
πj=P(Xji<X(3−j)i)=∫∞0αjβγxγ−1(1+βxγ)−(αj+α(3−j)+1)dx=αjαj+α3−j, | (2.5) |
where j=1,2.
Now, based on the observed sample in Eq (2.1), the likelihood function formula for three different cases is defined in a unified expression:
L∝ΠW∗i=1[S(xi;α1,β,γ)S(xi;α2,β,γ)]Ri([S(τ;α1,β,γ)S(τ;α2,β,γ)]n−W∗−W∗∑i=1Ri)D∗×Πj1i=1[f(xi;α1,β,γ)S(xi;α2,β,γ)]Πj2i=1[f(xi;α2,β,γ)S(xi;α1,β,γ)], | (2.6) |
where
xi=xi:m:n |
for simplicity of notation,
S(xi;αj,β,γ)=1−F(xi;αj,β,γ); i=1,2,...,n, j=1,2 |
is the survival function and j1 and j2 are the numbers of the observed failure times due to reasons 1 and 2. The total number of failures observed at experiment terminated time is denoted by W∗; i.e.,
W∗=j1+j2. |
Also, W∗=k for Case I, or W∗=d for Case II, W∗=m for Case III. The indicator function is denoted D∗; i.e., D∗=1 when W∗=d; otherwise, D∗=0.
In this section, we propose the two methods of point estimation for the CR model using the GPHCS data from the GLD.
In the presence of CR data, the GPHC schemes are used to acquire the ML estimators of the unknown parameters α1, α2, and β, the reliability characteristics ℜ(t) and ℏ(t), and the relative risks πj,j=1,2. Assuming that γ is known, without any generality, γ=γ0.
From Eqs (2.2) and (2.6), the likelihood function can be written as follows:
L(x_|α1,α2,β)∝αj11αj22βW∗γW∗0(ΠW∗i=1xγ0−1i:m:n)ΠW∗i=1[1+βxγ0i:m:n]−(Ri+1)(α1+α2)−1×[1+βτγ0]−D∗(α1+α2)(n−W∗−∑W∗i=1Ri). | (3.1) |
Therefore, taking the logarithms of likelihood function (3.1), say, l∗, then
l∗∝j1ln(α1)+j2ln(α2)+W∗ln(β)+W∗ln(γ0)−W∗∑i=1ln(1+βxγ0i:m:n)+(γ0−1)W∗∑i=1ln(xi:m:n)−(α1+α2)[W∗∑i=1(Ri+1)ln(1+βxγ0i:m:n)+D∗(n−W∗−W∗∑i=1Ri)ln(1+βτγ0)]. | (3.2) |
Now, by differentiating l∗ with respect to α1, α2, and β, then
∂l∗∂α1=j1α1−[W∗∑i=1(Ri+1)ln(1+βxγ0i:m:n)+D∗(n−W∗−W∗∑i=1Ri)ln(1+βτγ0)], | (3.3) |
∂l∗∂α2=j2α2−[W∗∑i=1(Ri+1)ln(1+βxγ0i:m:n)+D∗(n−W∗−W∗∑i=1Ri)ln(1+βτγ0)] | (3.4) |
and
∂l∗∂β=−(α1+α2)[W∗∑i=1(Ri+1)(xγ0i:m:n1+βxγ0i:m:n)+D∗(n−W∗−W∗∑i=1Ri)τγ01+βτγ0]+W∗β−W∗∑i=1xγ0i:m:n1+βxγ0i:m:n. | (3.5) |
Equating (3.3) and (3.4) with zero, then
ˆα1ML=j1ϕ(x_,τ,ˆβML,γ0)andˆα2ML=j2ϕ(x_,τ,ˆβML,γ0), | (3.6) |
where
ϕ(x_,τ,ˆβML,γ0)=W∗∑i=1(Ri+1)ln(1+ˆβMLxγ0i:m:n)+D∗(n−W∗−W∗∑i=1Ri)ln(1+ˆβMLτγ0). |
To solve Eq (3.6), we need to get the ML estimator of β say ˆβML. There is no closed-form solution for β, so by setting Eq (3.5) with zero and solving numerically, the ˆβML is produced. Now, by substituting (3.6) into (3.5), we get the following:
g(ˆβML)=W∗[1ˆβML−ϕ′(x_,τ,ˆβML,γ0)ϕ(x_,τ,ˆβML,γ0)]−W∗∑i=1xγ0i:m:n1+βxγ0i:m:n=0. | (3.7) |
In this situation, one can utilize numerical methods such as the Newton-Raphson method to solve for β and then use this value to determine ˆα1ML and ˆα2ML, respectively. In the real data example, we used profile plots of the log-likelihood function of parameter β, as shown in Figure 3. These findings suggest that the required ML estimators may exist uniquely; see Section 7.
Using the invariance property, the ML estimator of the reliability characteristics ˆℜ(t) and ˆℏ(t), at the various time t, can be acquired from (2.3) and (2.4) by replacing the original values α1, α2, and β by their ML estimators ˆα1ML,ˆα2ML, and ˆβML, respectively.
ˆℜ(t)=(1+ˆβMLtγ0)−W∗/ϕ(t_,τ,ˆβML,γ0)andˆℏ(t)=W∗γ0ˆβMLtγ0−1ϕ(t_,τ,ˆβML,γ0)(1+ˆβMLtγ0). |
In the same way, the ML estimator of the relative risk resulting from Causes 1 and 2 may be determined from Eq (2.5) as follows:
ˆπ1ML=ˆα1MLˆα1ML+ˆα2ML=j1W∗andˆπ2ML=1−j1W∗=j2W∗. |
In this subsection, we discuss Bayesian estimators for the unknown parameters α1,α2, and β of the GLD based on the CR model under GPHCS. We assumed independent gamma with hyper-parameters al>0 and bl>0, l=1,2,3. The following forms can be acquired from the combined prior distribution of these parameters:
π(α1,α2,β)∝αa1−11αa2−12βa3−1e−(b1α1+b2α2+b3β);α1,α2 and β>0. | (3.8) |
The joint posterior distribution of unknown parameters α1, α2, and β is determined by utilizing the likelihood function (3.1) and the joint prior distributions (3.8) as follows:
π∗(α1,α2,β|x_)∝αa1+j1−11e−α1[b1+ϕ(x_,τ,β,γ0)]αa2+j2−12e−α2[b2+ϕ(x_,τ,β,γ0)]γw∗0×βa3−1+W∗e−[b3β+∑W∗i=1ln(1+βxγ0i:m:n)−(γ0−1)∑W∗i=1ln(xi:m:n))], | (3.9) |
where
ϕ(x_,τ,β,γ0)=W∗∑i=1(Ri+1)ln(1+βxγ0i:m:n)+D∗(n−W∗−W∗∑i=1Ri)ln(1+βτγ0). |
In Bayesian analysis, a loss function must be specified to estimate an unknown parameter. There are no hard-and-fast guidelines for choosing the best loss function; it depends on the particular issue. The two main categories of loss functions are symmetric and asymmetric. The Bayesian estimator for the function u(α1,α2,β) under symmetric (SE) and asymmetric (LINEX and GE) loss functions are driven. A common symmetric loss that penalizes overestimation and underestimating equally is the SE. Because of its convexity, which guarantees a unique minimum and makes optimization easier, it is frequently utilized in regression situations (Varde[29]). The posterior mean is the Bayesian estimator for a function u(α1,α2,β) under the SE loss function and is computed by
˜u(α1,α2,β)BS=E(u(α1,α2,β)|x_)=∫∞0∫∞0∫∞0u(α1,α2,β)π∗(α1,α2,β|x_)dα1dα2dβ. | (3.10) |
In machine learning, the LINEX is a commonly used statistic, especially for classification issues. It calculates how much the target variable's actual distribution differs from the predicated probability distribution (Varian[30]). Although it provides flexibility in estimating location parameters, the LINEX loss function might not be the best option for estimating scale parameters (Basu and Ibrahimi[31]. Based on the LINEX loss function, the Bayesian estimator for a function u(α1,α2,β) is given by
˜u(α1,α2,β)BL=−1hln[E(e−hu(α1,α2,β)|x_)]=−1hln[∫∞0∫∞0∫∞0e−hu(α1,α2,β)π∗(α1,α2,β|x_)dα1dα2dβ]. | (3.11) |
A good alternative for the modified LINEX loss function is the GE, which Calabria and Pulcini [32] suggested. The GE loss function is a useful tool in information theory and machine learning. The Bayesian estimator for function u(α1,α2,β) under the GE loss function is described below:
˜u(α1,α2,β)BG=[E((u(α1,α2,β))−c|x_)]−1c=[∫∞0∫∞0∫∞0(u(α1,α2,β))−cπ∗(α1,α2,β|x_)dα1dα2dβ]−1c. | (3.12) |
Moreover, from Eq (3.9), we can obtain the Bayesian estimators of the reliability characteristics at operation time t and relative risks, respectively, as follows:
˜ℜ(t)=∫β∫α2∫α1ℜ(t,α1,α2,β)π∗(α1,α2,β|x_)dα1dα2dβ, | (3.13) |
˜ℏ(t)=∫β∫α2∫α1ℏ(t,α1,α2,β)π∗(α1,α2,β|x_)dα1dα2dβ, | (3.14) |
˜πj=∫β∫α2∫α1πj(α1,α2)π∗(α1,α2,β|x_)dα1dα2dβ;j=1,2. | (3.15) |
Since it is extremely difficult to solve the integrals from (3.10) to (3.15) analytically, we shall use the MCMC approach. The lower and upper limits of the BCI and HPD intervals for the unknown parameters, reliability characteristics, and relative risks will also be calculated using the MCMC approach's sample data. Therefore, the conditional posterior probability for α1 given α2,β, and x_, and α2 given α1,β, and x_ are, respectively, expressed as follows:
π∗1(α1|α2,β,x_)∝αa1+j1−11e−α1[b1+ϕ(x_,τ,β,γ0)]∼Gamma(a1+j1,b1+ϕ(x_,τ,β,γ0)), | (3.16) |
π∗2(α2|α1,β,x_)∝αa1+j1−12e−α2[b2+ϕ(x_,τ,β,γ0)]∼Gamma(a2+j2,b2+ϕ(x_,τ,β,γ0)). | (3.17) |
Also, the conditional posterior probability for β given α1,α2, and x_ is given by:
π∗3(β|α1,α2,x_)∝βa3+W∗−1e−(α1+α2)ϕ(x_,τ,β,γ0)eb3β+∑W∗i=1ln(1+βxγ0i:m:n). | (3.18) |
Because the conditional probability distribution in Eq (3.18) is not well-known, we will apply the Metropolis-Hastings sampler to obtain the values of β that follow the distribution in (3.18). Using the Metropolis-Hastings technique, we can produce random samples from the normal proposal distribution; see Metropolis et al.[33]. The conditional posterior distributions α1, α2, and β are generated using Algorithm A.2, as shown in Appendix A.
The Bayesian estimators based on the three loss functions and the generated values φ(z)g; g=1,2,...,7; and
z=NB+1,NB+2,...,M, |
respectively, are obtained as follows:
˜φgBS=∑Mz=NB+1φ(z)M−NB, ˜φgBL=−1hln[∑Mz=NB+1e−hφ(z)M−NB]and˜φgBG=[∑Mz=NB+1[φ(z)]−cM−NB]−1/c. |
If the values of the hyper-parameters tend to be zero, then the Bayesian estimator for vector φg can be computed using the same approach as before. In this case, the prior is a uniform prior.
This section discusses two methods of constructing CIs for α1, α2, β, reliability characteristics and relative risks. The first method uses the asymptotic distributions of the estimators to obtain CIs of the different parameters of interest. The second method is the parametric bootstrap CIs, constructed using the percentile bootstrap (Boot-p) and the bootstrap-t (Boot-t) methods.
This subsection presents the ACIs of the unknown parameters, reliability characteristics and relative risks. The ACIs can be acquired by inverting the Fisher information matrix (FIM) with the negative elements of expected values of the second-order derivatives of logarithms of the likelihood functions. Cohen [34] replaced expected values with their ML estimators to obtain the approximation variance-covariance matrix. Let
Θ_=(α1,α2,β)T |
be the vector of unknown parameters, where Θ1=α1,Θ2=α2, and Θ3=β. Let I−1(Θ_) denote the inverse of FIM of the parameters,
I−1(ˆα1MLˆα2MLˆβML)=[−∂2l∗∂α21−∂2l∗∂α1∂α2−∂2l∗∂α1∂β−∂2l∗∂α2∂α1−∂2l∗∂α22−∂2l∗∂α2∂β−∂2l∗∂β∂α1−∂2l∗∂β∂α2−∂2l∗∂β2]−1(α1=ˆα1ML,α2=ˆα2ML,β=ˆβML). |
From Eqs (3.3)–(3.5), the second derivatives of l∗ with respect to α1,α2 and β are
∂2l∗∂α21=−j1α21,∂2l∗∂α22=−j2α22,∂2l∗∂β2=−W∗β2−(α1+α2)ϕ″(x_,τ,β,γ0)+W∗∑i=1(xγ0i:m:n1+βxi:m:n)2,∂2l∗∂α1∂β=∂2l∗∂α2∂β=∂2l∗∂β∂α1=∂2l∗∂β∂α2=−ϕ′(x_,τ,β,γ0), ∂2l∗∂α1∂α2=∂2l∗∂α2∂α1=0, |
where
ϕ(x_,τ,ˆβ,γ0)=W∗∑i=1(Ri+1)ln(1+ˆβxγ0i:m:n)+D∗(n−W∗−W∗∑i=1Ri)ln(1+ˆβτγ0),ϕ′(x_,τ,β,γ0)=[W∗∑i=1(Ri+1)(xγ0i:m:n1+βxγ0i:m:n)+D∗(n−W∗−W∗∑i=1Ri)τγ01+βτγ0],ϕ″(x_,τ,β,γ0)=−[W∗∑i=1(Ri+1)(xγ0i:m:n1+βxγ0i:m:n)2+D∗(n−W∗−W∗∑i=1Ri)(τγ01+βτγ0)2]. |
Therefore, we obtain the ACIs of the unknown parameters α1,α2 and β for j1,j2>0. Depending upon the asymptotic distribution of the ML estimators of the parameters, it is considered as the following:
ˆΘ_−Θ_⟶N3(0,I−1(ˆΘ_)). |
Now, the two-sided 100(1−δ)%, 0<δ<1, ACIs for the vector of unknown parameters can be obtained as follows:
ˆΘl±Zδ/2√Var(ˆΘl),l=1,2,3, |
where Zδ/2 is the percentile of the standard normal distribution with right-tail probability δ/2, and Var(ˆΘl) is the element of the main diagonal of I−1(ˆΘl) for l=1,2,3.
The obtained ACIs may occasionally have negative lower bounds. To overcome this issue, the logarithmic transformation and delta techniques can be used to construct the asymptotic normality distribution of lnˆΘl as
lnˆΘl−lnΘlVar(lnˆΘl)⟶N(0,1); l=1,2,3. |
As a result, the formula for a 100(1−δ)%, 0<δ<1, ACI of Θl obtained in this way is
(ˆΘlexp(Zδ/2√^Var(lnˆΘl)),ˆΘlexp(Zδ/2√^Var(lnˆΘl))), |
where
^Var(lnˆΘl)=^Var(ˆΘl)/ˆΘl. |
Furthermore, based on the ML estimators' asymptotic normality, it is recognized that ˆℜ(t)∼N(ℜ(t), ˆσ2ℜ),ˆℏ(t)∼N(ℏ(t),ˆσ2ℏ), and ˆπj∼N(πj,ˆσ2πj);j=1,2. It is possible to create the ACIs for ℜ(t),ℏ(t), and πj by employing the corresponding normality. Therefore, Greene's [35] delta approach must be used to approximate and represent the variances of the estimators of ℜ(t),ℏ(t), and πj. The three partial derivative vectors for ℜ(t),ℏ(t), and πj with respect to unknown parameters are known as △ℜ,△ℏ, and △πj;j=1,2, respectively, which are described as follows:
△ℜ=(∂ℜ(t)∂α1,∂ℜ(t)∂α2,∂ℜ(t)∂β),△ℏ=(∂ℏ(t)∂α1,∂ℏ(t)∂α2,∂ℏ(t)∂β),△πj=(∂πj∂α1,∂πj∂α2,∂πj∂β), |
where
∂ℜ(t)∂αj=−ln(1+βtγ0)(1+βtγ0)(α1+α2),∂ℜ(t)∂β=−(α1+α2)tγ0(1+βtγ0)(α1+α2+1),∂πj∂β=0,∂πj∂αj=α3−j(αj+α3−j)2,∂πj∂α3−j=−αj(αj+α3−j)2, ∂ℏ(t)∂αj=βγ0tγ0−11+βtγ0, ∂ℏ(t)∂β=(α1+α2)γ0tγ0−1(βtγ0+1)2;j=1,2. |
Consequently, it is possible to acquire the approximate variances of ℜ(t),ℏ(t), πj;j=1,2 as follows:
ˆσ2ℜ=[△ℜ I−1(Θ_) △Tℜ]|Θ_=ˆΘ_,ˆσ2ℏ=[△ℏ I−1(Θ_) △Tℏ]|Θ_=ˆΘ_, ˆσ2πj=[△πj I−1(Θ_) △Tπj]|Θ_=ˆΘ_. |
Now, the two-sided 100(1−δ)%, 0<δ<1, ACIs for ℜ(t),ℏ(t), and πj can be obtained as follows:
ˆℜ(t)±zδ/2√ˆσ2ℜ, ˆℏ(t)±zδ/2√ˆσ2ℏ,^πj±zδ/2√ˆσ2πj;j=1,2. |
This subsection constructs two parametric Boot-p CIs for unknown parameters, reliability characteristics, and relative risks of the GLD in the presence of two CR based on GPHC schemes, which are known as the Boot-p (Efron[36]) and the Boot-t (Hall[37]) methods.
Let
φ_=(α1,α2,β,ℜ(t),ℏ(t),π1,π2) |
be a vector of the unknown parameters, reliability characteristics, and relative risks, where
φ1=α1,φ2=α2,φ3=β,φ4=ℜ(t),φ5=ℏ(t),φ6=π1andφ7=π2. |
By following Kundu et al.[38] and Kundu and Joarder[11], we generate Boot-p CIs by using Algorithm 1, as shown in Appendix A.
A Monte Carlo analysis is used in this section to compare the performance of the MLEs and the BEs for gamma distribution under different GPHC schemes. In addition, the BEs using the gamma prior distribution are compared. We generated GPHC data for each failure sample. In addition, we identified the cause of failure as 1 or 2 with a probability of α1α1+α2 and α2α1+α2, according to an algorithm proposed by Balakrishnan and Sandhu[39]. With the following assumptions, one can generate 10,000 GPHC samples, 11,000 MCMC samples with 1000 nburn, and 1000 Boot-p samples in the presence of CR from the GLD.
(1) Under the prefixed time τ=1.2, assume the sample sizes for each cause of failure, and the number of failed units with the various minimum number of failure units are
(n,m,k)=(60,40,25),(60,40,30),(60,50,25),(60,50,30). |
(2) Assume that the following censoring schemes will be used to remove the remaining units:
– Scheme 1: R1=n−m; otherwise, Ri=0.
– Scheme 2: Ri=1 if i=1,2,...,n−m; otherwise, Ri=0.
– Scheme 3: Rm=n−m and Ri=0 if i otherwise.
(3) In this study, we assumed fixed parameters γ0=3 and γ0=1 to use for choosing the GLD parameters
Θtrue=(α1,α2,β)=(0.5,0.75,1) and(0.8,1.5,2). |
It is important to mention that in both states 1 and 2, Xi=min(X1i,X2i) follows the Burr type XII(β=1) and Lomax(γ0=1) distributions, as special states of GLD, respectively.
(4) From BE, the three loss functions, SE, LINEX (h=1.5 and −1.5), and GE (c=0.5 and −0.5), are used. The hyper-parameters of gamma prior to BE are obtained in these states:
(a) State 1: (al,bl)=(5.00,10.00),(11.25,15.00),(20.00,20.00);l=1,2,3, when γ0=3.
(b) State 2: (al,bl)=(12.8,16),(45.0,30.0),(80.0,40.0);l=1,2,3, when γ0=1.
Let the mean of the marginal prior distribution be Θtrue and its variance be 0.05 for each prior see El-Din et al.[40].
We used MSE, absolute bias (AB), and average estimate (AE) to compare each estimate. The values of AB and MSE of MLEs and BEs for the parameters α1, α2 and β depending on gamma priors are presented in Tables B.1–B.6. In addition, for the values of the AE of the MLE and BEs for the reliability characteristics ℜ(t) and ℏ(t) at different times see Tables B.7–B.10). From these tables, we noted that
● The BEs under the LINEX loss function (BL) with h=−1.5 have the highest MSE values among all BEs for the various estimates.
● The BEs under BL with h=1.5 for ˆα1BL and ˆα2BL exhibit the lowest MSE values, as shown in Figure 4a, b.
● The BEs under the SE loss function (BS) for ˆβBS had the lowest MSE values when γ0=1; see Figure 4c.
● When γ0=3, the MSE values of BEs exhibit similar behavior for different schemes, but the MSE values of MLEs decrease.
● For a fixed m, the MSE values of various estimates of α1 and β decrease for different schemes as k increases (see Figure 5a, c), whereas the MSE values of α2 increase and vice versa.
● The MSE values of α2 and β estimates increase and vice versa for α1, for fixed k and change m, as shown in Figure 5.
● Tables B.3–B.5 show that the MSE values of all estimates decrease for different schemes when m increases with constant k in most cases.
● The MSE values for different estimates of ℜ(t) increase as time t increases, and vice versa for ℏ(t), as shown in Figure 6 under γ0=1.
● When γ0=3, the MSE values of ℜ(t) and ℏ(t) increased with time t.
● The BEs of ℜ(t) under BL with h=−1.5 have the minimum MSE values at t=0.1, whereas the MSE values of BE under BL (with h=1.5) are the smallest at t=0.5, as shown in Figure 6a.
● The BEs exhibit the lowest MSE values for ℏ(t) at time t=0.1 when the loss function is the BS; see Figure 6b.
● The MSE values are the highest under BL (with h=−1.5) at various times t.
● Scheme 3 has the lowest MSE values under the ML method, as shown in Figures 4a, b and 7 concerning both ℜ(t) and ℏ(t).
● In most instances, the MSE values of ℜ(t) and ℏ(t) are approximately equal to those of the BEs; see Figure 7.
● At the time t=0.5 and γ0=3, the BEs for ℜ(t) under BL with h=−1.5 had the smallest MSE, as shown in Figure 7a, b.
● For γ0=3 and at time t=0.1, the BEs for ℏ(t) under the GE loss function (BG) with c=0.5 had the smallest MSE; however, at time t=0.5, the BEs for ℏ(t) under the BL with h=1.5 had the smallest MSE; see Figure 7c, d.
Furthermore, Tables B.11–B.14 illustrate the average width (AW) and coverage probability (CP) for the 95% ACI, BCIs, and HPDs, respectively, using the MCMC-generated values of the parameters and reliability characteristics. According to the tables, we noted that
(1) The ACIs of all parameters have the largest CP; therefore, the AWs of these parameters are also the highest.
(2) Boot-t has the smallest CP for α1 and α2, whereas the opposite is true for β. Furthermore, Boot-p has a smaller AW than Boot-t for all the parameters.
(3) The HPDs had smaller AWs than BCI, which had a higher CP than the HPDs.
(4) When k=30 and m increase, the CP for ℜ(t) increases over time in most cases and vice versa for k=25.
(5) For fixed k, the CP for ℏ(t) increases over time in most cases as m increases.
(6) At γ0=3 and for fixed k and m, the AW for ℜ(t) increases over time, similar to that for ℏ(t).
(7) For fixed k, m and at γ0=1, the AW for ℜ(t) increases over time in most cases and vice versa for ℏ(t), except in case ACI.
This section examines the adaptability and validity of GLD using real-time data analysis. It also demonstrated the practical use of the proposed CR model based on the GPHCS. We display the original dataset from the jute fiber breaking strength experiment conducted (see Xia et al. [41]). In this experiment, the breaking strength failure data of the jute fiber was affected by two different gauge lengths: 5 mm and 10 mm. The following data is displayed using the transformation Y=X/200:
Time of failure due to Cause 1: 0.6454, 0.83935, 0.841, 0.89125, 0.9271, 0.9384, 1.0943, 1.13265, 1.27145, 1.30485, 1.341, 1.35395, 1.5242, 1.53495, 1.57665, 1.804, 1.8385, 1.8501, 2.20935, 2.47755, 2.4814, 2.5824, 2.68725, 2.73055, 2.77305, 2.83155, 2.91985, 3.09285, 4.04615, 4.11515.
Time of failure due to Cause 2: 0.21965, 0.2508, 0.50575, 0.5447, 0.6153, 0.7069, 0.7574, 0.817, 0.88625, 0.9158, 1.06065, 1.2872, 1.3145, 1.45635, 1.5195, 1.61915, 1.7662, 1.8821, 1.91715, 2.11055, 2.533, 2.65275, 2.9524, 3.1883, 3.35745, 3.46865, 3.5037, 3.5233, 3.63615, 3.89085.
We conducted the Kolmogorov-Smirnov (KS) test to evaluate whether the data conformed to the GLD, and the P-values obtained from the KS test for Causes 1 and 2 were 0.560938 and 0.651419, respectively. The GLD provided a reasonable fit for the data, see Figure 8. Subsequently, we employ a censored dataset and various values that differ from the original data (both big and small).
In this subsection, we create a PTIIC sample of m=40 out of n=60. The PTIIC sample is presented in Table 2. This table displays the failure lifetime yi:m:n, cause of failure δi, and censoring scheme R used to remove the remaining units. Three alternative GPHCS were produced when the known parameter γ0=3 was selected, assuming a value of k=25 and various values of τ.
yi:m:n | 0.50575 | 0.5447 | 0.7069 | 0.7574 | 0.817 | 0.83935 | 0.841 | 0.88625 | 0.89125 | 0.9271 |
δi | 2 | 2 | 2 | 2 | 2 | 1 | 1 | 2 | 1 | 1 |
R | 2 | 0 | 0 | 2 | 0 | 0 | 2 | 0 | 0 | 1 |
yi:m:n | 0.9384 | 1.06065 | 1.0943 | 1.13265 | 1.27145 | 1.341 | 1.35395 | 1.45635 | 1.5242 | 1.53495 |
δi | 1 | 2 | 1 | 1 | 1 | 1 | 1 | 2 | 1 | 1 |
R | 0 | 0 | 0 | 1 | 0 | 2 | 0 | 1 | 0 | 1 |
yi:m:n | 1.57665 | 1.61915 | 1.7662 | 1.8821 | 1.91715 | 2.20935 | 2.47755 | 2.533 | 2.5824 | 2.65275 |
δi | 1 | 2 | 2 | 2 | 2 | 1 | 1 | 2 | 1 | 2 |
R | 0 | 0 | 0 | 0 | 0 | 1 | 0 | 1 | 0 | 1 |
yi:m:n | 2.77305 | 2.83155 | 2.9524 | 3.09285 | 3.1883 | 3.5037 | 3.5233 | 3.63615 | 4.04615 | 4.11515 |
δi | 1 | 1 | 2 | 1 | 2 | 2 | 2 | 2 | 1 | 1 |
R | 0 | 0 | 0 | 0 | 0 | 1 | 0 | 0 | 1 | 3 |
1) Case 1: Suppose τ=1.88259, since τ<Y25:40:60, then the experiment would have terminated at y25:40:60, with J1=10, J2=15, R∗=(2,0,0,2,0,0,2,0,0,1,0,0,0,1,0,2,0,1,0,1,0,0,0,0,23), R∗k=23; and we would have the following data: 0.50575, 0.5447, 0.7069, 0.7574, 0.817, 0.83935, 0.841, 0.88625, 0.89125, 0.9271, 0.9384, 1.06065, 1.0943, 1.13265, 1.27145, 1.341, 1.35395, 1.45635, 1.5242, 1.53495, 1.57665, 1.61915, 1.7662, 1.8821, 1.91715.
2) Case 2: Suppose τ=2.7, since Y25:40:60<τ<Y40:40:60, then the experiment would have terminated at τ=2.7, with J1=12,J2=18,R∗∗=(,0,0,2,0,0,2,0,0,1,0,0,0,1,0,2,0,1,0,1,0,0,0,0,0,1,0,1,0,1), R∗∗τ=15; and we would have the following data: 0.50575, 0.5447, 0.7069, 0.7574, 0.817, 0.83935, 0.841, 0.88625, 0.89125, 0.9271, 0.9384, 1.06065, 1.0943, 1.13265, 1.27145, 1.341, 1.35395, 1.45635, 1.5242, 1.53495, 1.57665, 1.61915, 1.7662, 1.8821, 1.91715, 2.20935, 2.47755, 2.533, 2.5824, 2.65275.
3) Case 3: Suppose τ=4.5, since Y40:40:60<τ, then the experiment would have terminated at Y40:40:60=4.11515, with J1=15, J2=25, R∗∗∗=R, R∗∗∗m=0; and we would have the following data: 0.50575, 0.5447, 0.7069, 0.7574, 0.817, 0.83935, 0.841, 0.88625, 0.89125, 0.9271, 0.9384, 1.06065, 1.0943, 1.13265, 1.27145, 1.341, 1.35395, 1.45635, 1.5242, 1.53495, 1.57665, 1.61915, 1.7662, 1.8821, 1.91715, 2.20935, 2.47755, 2.533, 2.5824, 2.65275, 2.77305, 2.83155, 2.9524, 3.09285, 3.1883, 3.5037, 3.5233, 3.63615, 4.04615, 4.11515.
Next, the estimate for parameter β can be obtained numerically by setting g(β)=0 and graphically by plotting the graph of g(β) in Eq (3.7) and finding the point of intersection with the β-axis. Figure 9 illustrates the behavior of g(β) for cases 1–3. Additionally, Figure 3 confirms that the MLE of β is unique since it is the only one that maximizes the log-likelihood for each scheme. As a result, the MLEs for the parameters α1,α2, and β are unique and exist. Consequently, we can calculate the reliability characteristics ℜ(t) and ℏ(t) at different times and relative risks due to Causes 1 and 2. Therefore, we utilized ML and Bayesian approaches to estimate parameter values. Since we have no prior knowledge of the unknown parameters, we compute BEs with a non-informative (uniform) prior, as seen in Kundu and Pradhan [42].
This subsection details the creation of a PTIIC sample consisting of m=25 items from a total of n=60. Table 3 presents the PTIIC sample, showing the failure lifetime yi:m:n, failure cause δi, and the censoring scheme R employed to eliminate the remaining units. Using a known parameter value of γ0=3 and setting k=15, three different GPHCS were generated with varying values of τ.
yi:m:n | 0.21965 | 0.50575 | 0.6153 | 0.6454 | 0.817 | 0.83935 | 0.841 | 0.9271 | 1.13265 |
δi | 2 | 2 | 2 | 1 | 1 | 1 | 1 | 1 | 1 |
R | 3 | 0 | 3 | 0 | 3 | 0 | 3 | 0 | 3 |
yi:m:n | 1.30485 | 1.5242 | 1.57665 | 1.8385 | 1.8501 | 1.91715 | 2.20935 | 2.47755 | 2.68725 |
δi | 1 | 2 | 1 | 1 | 1 | 2 | 1 | 1 | 1 |
R | 0 | 3 | 0 | 3 | 0 | 3 | 0 | 3 | 0 |
yi:m:n | 2.77305 | 2.83155 | 2.9524 | 3.09285 | 3.5233 | 3.89085 | 4.11515 | ||
δi | 1 | 1 | 2 | 1 | 2 | 2 | 1 | ||
R | 3 | 0 | 3 | 0 | 2 | 0 | 0 |
1) Case 1*: Suppose τ=1.88432, since τ<Y15:25:60, then the experiment would have terminated at y15:25:60, with J1=6, J2=9, R∗=(,0,3,0,3,0,3,0,3,0,3,0,3,0,24), R∗k=24; and we would have the following data: 0.21965, 0.50575, 0.6153, 0.6454, 0.817, 0.83935, 0.841, 0.9271, 1.13265, 1.30485, 1.5242, 1.57665, 1.8385, 1.8501, 1.91715.
2) Case 2*: Suppose τ=2.5, since Y15:25:60<τ<Y25:25:60, then the experiment would have terminated at τ=2.7, with J1=8,J2=9,R∗∗=(,0,3,0,3,0,3,0,3,0,3,0,3,0,3,0,3), R∗∗τ=16; and we would have the following data: 0.21965, 0.50575, 0.6153, 0.6454, 0.817, 0.83935, 0.841, 0.9271, 1.13265, 1.30485, 1.5242, 1.57665, 1.8385, 1.8501, 1.91715, 2.20935, 2.47755.
3) Case 3*: Suppose τ=4.5, since Y25:25:60<τ, then the experiment would have terminated at Y25:25:60=4.11515, with J1=11, J2=14,R∗∗∗=R,R∗∗∗m=0; and we would have the following data: 0.21965, 0.50575, 0.6153, 0.6454, 0.817, 0.83935, 0.841, 0.9271, 1.13265, 1.30485, 1.5242, 1.57665, 1.8385, 1.8501, 1.91715, 2.20935, 2.47755, 2.68725, 2.77305, 2.83155, 2.9524, 3.09285, 3.5233, 3.89085, 4.11515.
The parameter β can be estimated numerically by solving g(β)=0 and visually by identifying where the graph of g(β) in Eq (3.7) crosses the β-axis. The behavior of g(β) for cases 1*–3* is depicted in Figure 10. Furthermore, Figure 11 demonstrates that the MLE of β is unique, as it is the sole value that optimizes the log-likelihood for each scenario.
Tables B.15 and B.18 display the standard error (SER), which is the root mean square error for the MLEs and BEs of the uniform prior distribution. Tables B.15 and B.18 show the result of real data when (n,m,k)=(60,40,25) and (n,m,k)=(60,25,15), respectively. From these tables, the SER for MLE was the smallest concerning most estimates. Moreover, the reliability characteristic ℜ(t) exhibits a decline from t=0.5 to t=1.5, whereas the opposite trend is observed for ℏ(t). Concurrently, the SER for both reliability characteristics exhibited an upward trend over time. Across all scenarios, the relative risk associated with Cause 1 was consistently lower than that of Cause 2.
Tables B.16 and B.17 show the lower and upper limits for the AWs of each parameter scheme for ACI, Boot-t, Boot-p, and BCI. It is evident from this table that the AWs of the HPD for all schemes were smaller than those of the BCI. The AWs for estimates of α1, α2, and β of Boot-t were found to be smaller than those of Boot-p, whereas the reliability characteristic ℜ(t) and ℏ(t) at different times t and relative risks due to Causes 1 and 2 showed the opposite trend. As time t increased, the AWs for ℜ(t) and ℏ(t) also increased. It is worth noting that BCI generally had the largest AWs in Table B.16, whereas in Table B.17, the AWs of ACI were the largest in most cases.
This study aimed to investigate the application of CR models for GLD in the context of GPHC. Within the CR model that incorporated the two failure mechanisms, it was postulated that the GLD independently determined the lifetime.
The GLD introduces a new parameter to the traditional Lomax distribution, making it more flexible for applications in diverse fields such as agriculture, finance, and actuarial sciences. This increased flexibility is particularly beneficial in lifetime studies and reliability analysis. For instance, the GLD can be effectively applied to analyze datasets like the failure times of Kevlar 373/epoxy under constant pressure. By incorporating CR and the GPHCS, we can extend this analysis to more complex scenarios.
In this paper, the point and interval estimators for the unknown parameters, reliability characteristics, and relative risks due to two causes were derived using both ML and Bayesian methods. Moreover, two Boot-p CIs based on MLEs were obtained. The BEs and their associated BCI and HPD intervals were calculated using the MCMC technique, which utilized three loss functions and gamma priors. A simulation analysis was conducted to assess the accuracy of the estimators. The results show the BEs of α1 and α2 under (h=1.5), whereas the BEs of β based on the BS loss function have the lowest MSE values when γ=1. BEs for the gamma prior outperformed the MLEs based on MSE values. The simulation study also revealed that as time t increased and γ0=1, the MSE values of the ℏ(t) estimates decreased, while the MSE values of the ℜ(t) estimates increased. When γ0=3, the MSE values of ℜ(t) and ℏ(t) increased with time t as shown in real data which the performance of the proposed estimators was evaluated using a real dataset, suggesting that the required MLEs may exist uniquely.
Future research directions include investigating lifetime distribution in the presence of CR and can be considered in the following scenarios as follows:
1) Exploring scenarios with dependent competing risks.
2) Employing alternative estimation techniques such as E-Bayesian method.
3) Examining various censoring schemes, including those with random removals.
Amal Hassan: conceptualization, validation and review; Sudhansu Maiti: visualization and validation; Rana Mousa: writing, editing and methodology.; Najwan Alsadat: conceptualization, methodology, funding acquisition and review; Mahmoued Abu-Moussa: methodology, software, and editing. All authors have read and agreed to the published version of the manuscript.
This research is supported by researchers supporting project number (RSPD2024R548), King Saud University, Riyadh, Saudi Arabia.
The authors declare no conflicts of interest.
First, Appendix A consists of two algorithms used.
Algorithm A.1 Boot-p method. |
Step 1: From the analysis in Section 3, we can estimate the vector φ_, say ˆφ_.
Step 2: Generate a Boot-p sample using ˆφ_, R1,R2,...,Rm. Obtain the Boot-p estimate of φl, say ^φl(b);l=1,2,...,7. Step 3: Compute the t-statistic Tl=(ˆφbl−ˆφl)/√Var(ˆφbl) where Var(ˆφbl) is the asymptotic variance of ˆφbl;l=1,2,3 and it can be obtained using the inverse of FIM, also Var(ˆφbl),l=4,5,6,7 can be acquired by the delta method. Step 4: Repeat Steps 1–3 BT times, and obtain { ˆφb(1)l,ˆφb(2)l,...,ˆφb(BT)l} and {T(1)l,T(2)l,...,T(BT)l} . Step 5: Arrange { ˆφb(1)l,ˆφb(2)l,...,ˆφb(BT)l} and { T(1)l,T(2)l,...,T(BT)l} in ascending order as { ˆφb[1]l,ˆφb[2]l,...,ˆφb[BT]l} and { T[1]l,T[2]l,...,T[BT]l} . Step 6: A two-sided 100(1−δ)%, 0<δ<1, boot-p and boot-t CIs for the vector φl are, respectively, expressed as follows: [ˆφb[BT(δ/2)]l,ˆφb[BT(1−δ/2)]l], [ˆφl+T[BT(δ/2)]l√Var(ˆφl),ˆφl+T[BT(1−δ/2)]l√Var(ˆφl)]. |
Algorithm A.2 MCMC method. |
Step 1: Start with (α01,α02,β0)=(ˆα1ML,ˆα2ML,ˆβML), M=MCMC with NB=n-burn. Step 2: Set z=1 Step 3: From (3.18), we can generate β∗ from normal distribution with mean β(z−1), and variance Var(β(z−1)) is the third element of the main diagonal of I−1(ˆΘ). Step 4: Calculate the acceptance probabilities ξβ=min[1,π∗3(β∗|α(z−1)1,α(z−1)2,x_)π∗3(β(z−1)|α(z−1)1,α(z−1)2,x_)]. Step 5: Generate U following a Uniform(0, 1) distribution. Step 6: If U≤ξβ, set β(z)=β∗, otherwise set β(z)=β(z−1). Step 7: Generate α1 and α2 from (3.16) and (3.17), respectively. Step 8: Acquire ℜ(z)(T) and ℏ(z)(T) at particular time T>0 and πj;j=1,2, by substituting α1,α2, and β with their α(z)1,α(z)2, and β(z), respectively. Step 9: Set z=z+1. Step 10: Repeat Steps 3–8 M times and obtain φ(z)g,g=1,2,...,7, and z=1,2,...,M; i.e., φ1=α1, φ2=α2, φ3=β, φ4=ℜ, φ5=ℏ, φ6=π1, and φ7=π2. Step 11: To compute the credible intervals for φg,g=1,2,...,7, based on the generated values, remove the first NB values for φ(z)g, which is the burn-in period, then sort the (M−NB) remaining values for φ(NB+1)g,φ(NB+2)g,...,φ(M)g ascending to be φ[1]g,φ[2]g,...,φ[M−NB]g;g=1,2,...,7. Step 12: A two-sided 100(1−δ)%, 0<δ<1 BCI for the unknown parameter φg is obtained as follows: [˜φ[(M−NB)δ/2]g,˜φ[(M−NB)(1−δ/2)]g];g=1,2,...,7, and the lengths of the credible intervals are the absolute difference between the lower and the upper bounds. Step 13: Furthermore, the HPD interval can be formed using the quantile of corresponding random samples, which means that the number of samples covered by the interval is (1−δ)(M−NB) and the interval length is the minimum of all the 100(1−δ)% intervals, i.e., ˜φ[z∗+(M−NB)(1−δ)]g−˜φ[z∗]g=min(M−NB)δz=1[˜φ[z+(M−NB)(1−δ)]g−˜φ[z]g], where g=1,2,...,7 and z∗ is set in this previous way (see Chen and Shao[43]). |
Next, all tables of numerical work results and real data outcomes are included in Appendix B.
n | m | k | Scheme | ˆα1ML | ˆα1BS | ˆα1BL | ˆα1BL | ˆα1BG | ˆα1BG | |
(h=1.5) | (h=−1.5) | (c=0.5) | (c=−0.5) | |||||||
60 | 40 | 25 | 1 | AB | 0.0061 | 0.0027 | 0.0102 | 0.0167 | 0.0234 | 0.0060 |
MSE | 0.0136 | 0.0093 | 0.0088 | 0.0102 | 0.0096 | 0.0092 | ||||
2 | AB | 0.0093 | 0.0045 | 0.0083 | 0.0184 | 0.0211 | 0.0040 | |||
MSE | 0.0123 | 0.0090 | 0.0085 | 0.0099 | 0.0092 | 0.0089 | ||||
3 | AB | 0.0079 | 0.0058 | 0.0054 | 0.0178 | 0.0163 | 0.0016 | |||
MSE | 0.0094 | 0.0084 | 0.0079 | 0.0092 | 0.0083 | 0.0083 | ||||
30 | 1 | AB | 0.0077 | 0.0056 | 0.0070 | 0.0192 | 0.0196 | 0.0028 | ||
MSE | 0.0124 | 0.0092 | 0.0087 | 0.0102 | 0.0093 | 0.0091 | ||||
2 | AB | 0.0067 | 0.0060 | 0.0067 | 0.0197 | 0.0192 | 0.0024 | |||
MSE | 0.0123 | 0.0092 | 0.0086 | 0.0102 | 0.0093 | 0.0091 | ||||
3 | AB | 0.0062 | 0.0054 | 0.0057 | 0.0174 | 0.0167 | 0.0020 | |||
MSE | 0.0094 | 0.0084 | 0.0079 | 0.0093 | 0.0084 | 0.0083 | ||||
50 | 25 | 1 | AB | 0.0095 | 0.0034 | 0.0082 | 0.0158 | 0.0198 | 0.0044 | |
MSE | 0.0106 | 0.0085 | 0.0081 | 0.0093 | 0.0087 | 0.0085 | ||||
2 | AB | 0.0094 | 0.0027 | 0.0088 | 0.0151 | 0.0203 | 0.0050 | |||
MSE | 0.0108 | 0.0086 | 0.0082 | 0.0094 | 0.0089 | 0.0086 | ||||
3 | AB | 0.0082 | 0.0043 | 0.0062 | 0.0155 | 0.0166 | 0.0027 | |||
MSE | 0.0090 | 0.0078 | 0.0074 | 0.0085 | 0.0079 | 0.0078 | ||||
30 | 1 | AB | 0.0069 | 0.0033 | 0.0082 | 0.0157 | 0.0198 | 0.0044 | ||
MSE | 0.0106 | 0.0087 | 0.0083 | 0.0094 | 0.0089 | 0.0086 | ||||
2 | AB | 0.0082 | 0.0041 | 0.0074 | 0.0165 | 0.0189 | 0.0036 | |||
MSE | 0.0106 | 0.0085 | 0.0080 | 0.0093 | 0.0086 | 0.0084 | ||||
3 | AB | 0.0070 | 0.0026 | 0.0079 | 0.0137 | 0.0183 | 0.0044 | |||
MSE | 0.0090 | 0.0077 | 0.0074 | 0.0083 | 0.0079 | 0.0077 |
n | m | k | Scheme | ˆα2ML | ˆα2BS | ˆα2BL | ˆα2BL | ˆα2BG | ˆα2BG | |
(h=1.5) | (h=−1.5) | (c=0.5) | (c=−0.5) | |||||||
60 | 40 | 25 | 1 | AB | 0.0151 | 0.0016 | 0.0157 | 0.0203 | 0.0219 | 0.0062 |
MSE | 0.0142 | 0.0091 | 0.0088 | 0.0100 | 0.0094 | 0.0091 | ||||
2 | AB | 0.0121 | 0.0023 | 0.0150 | 0.0208 | 0.0211 | 0.0055 | |||
MSE | 0.0129 | 0.0086 | 0.0084 | 0.0096 | 0.0089 | 0.0086 | ||||
3 | AB | 0.0097 | 0.0061 | 0.0096 | 0.0229 | 0.0149 | 0.0009 | |||
MSE | 0.0098 | 0.0080 | 0.0076 | 0.0090 | 0.0080 | 0.0079 | ||||
30 | 1 | AB | 0.0088 | 0.0046 | 0.0124 | 0.0227 | 0.0182 | 0.0031 | ||
MSE | 0.0131 | 0.0088 | 0.0084 | 0.0099 | 0.0089 | 0.0087 | ||||
2 | AB | 0.0120 | 0.0044 | 0.0127 | 0.0228 | 0.0186 | 0.0033 | |||
MSE | 0.0127 | 0.0087 | 0.0083 | 0.0097 | 0.0088 | 0.0086 | ||||
3 | AB | 0.0107 | 0.0058 | 0.0098 | 0.0226 | 0.0151 | 0.0012 | |||
MSE | 0.0099 | 0.0084 | 0.0080 | 0.0095 | 0.0084 | 0.0083 | ||||
50 | 25 | 1 | AB | 0.0110 | 0.0025 | 0.0135 | 0.0196 | 0.0190 | 0.0047 | |
MSE | 0.0112 | 0.0085 | 0.0082 | 0.0093 | 0.0087 | 0.0084 | ||||
2 | AB | 0.0110 | 0.0022 | 0.0137 | 0.0193 | 0.0193 | 0.0050 | |||
MSE | 0.0112 | 0.0085 | 0.0082 | 0.0093 | 0.0087 | 0.0085 | ||||
3 | AB | 0.0113 | 0.0031 | 0.0117 | 0.0190 | 0.0168 | 0.0035 | |||
MSE | 0.0094 | 0.0080 | 0.0077 | 0.0088 | 0.0082 | 0.0079 | ||||
30 | 1 | AB | 0.0126 | 0.0034 | 0.0126 | 0.0205 | 0.0181 | 0.0038 | ||
MSE | 0.0111 | 0.0087 | 0.0084 | 0.0096 | 0.0089 | 0.0087 | ||||
2 | AB | 0.0121 | 0.0041 | 0.0074 | 0.0165 | 0.0189 | 0.0036 | |||
MSE | 0.0112 | 0.0085 | 0.0080 | 0.0092 | 0.0086 | 0.0084 | ||||
3 | AB | 0.0132 | 0.0032 | 0.0115 | 0.0192 | 0.0167 | 0.0034 | |||
MSE | 0.0095 | 0.0079 | 0.0077 | 0.0087 | 0.0081 | 0.0079 |
n | m | k | Scheme | ˆα1ML | ˆα1BS | ˆα1BL | ˆα1BL | ˆα1BG | ˆα1BG | |
(h=1.5) | (h=−1.5) | (c=0.5) | (c=−0.5) | |||||||
60 | 40 | 25 | 1 | AB | 0.0063 | 0.0029 | 0.0160 | 0.0231 | 0.0212 | 0.0051 |
MSE | 0.0316 | 0.0116 | 0.0112 | 0.0129 | 0.0119 | 0.0116 | ||||
2 | AB | 0.0093 | 0.0026 | 0.0163 | 0.0227 | 0.0215 | 0.0054 | |||
MSE | 0.0315 | 0.0117 | 0.0113 | 0.0130 | 0.0120 | 0.0117 | ||||
3 | AB | 0.0065 | 0.0029 | 0.0157 | 0.0227 | 0.0207 | 0.0050 | |||
MSE | 0.0295 | 0.0117 | 0.0113 | 0.0130 | 0.0120 | 0.0117 | ||||
30 | 1 | AB | 0.0063 | 0.0023 | 0.0165 | 0.0225 | 0.0218 | 0.0057 | ||
MSE | 0.0322 | 0.0114 | 0.0110 | 0.0126 | 0.0117 | 0.0114 | ||||
2 | AB | 0.0077 | 0.0011 | 0.0177 | 0.0212 | 0.0230 | 0.0069 | |||
MSE | 0.0321 | 0.0118 | 0.0114 | 0.0130 | 0.0121 | 0.0117 | ||||
3 | AB | 0.0051 | 0.0033 | 0.0152 | 0.0232 | 0.0203 | 0.0045 | |||
MSE | 0.0303 | 0.0117 | 0.0113 | 0.0130 | 0.0119 | 0.0117 | ||||
50 | 25 | 1 | AB | 0.0062 | 0.0043 | 0.0127 | 0.0225 | 0.0174 | 0.0029 | |
MSE | 0.0262 | 0.0114 | 0.0109 | 0.0125 | 0.0115 | 0.0113 | ||||
2 | AB | 0.0069 | 0.0028 | 0.0142 | 0.0209 | 0.0188 | 0.0044 | |||
MSE | 0.0247 | 0.0113 | 0.01090 | 0.0124 | 0.0115 | 0.0113 | ||||
3 | AB | 0.0033 | 0.0044 | 0.0123 | 0.0221 | 0.0167 | 0.0027 | |||
MSE | 0.0247 | 0.0114 | 0.0109 | 0.0125 | 0.0114 | 0.0113 | ||||
30 | 1 | AB | 0.0061 | 0.0011 | 0.0159 | 0.0192 | 0.0205 | 0.0061 | ||
MSE | 0.0266 | 0.0112 | 0.0109 | 0.0122 | 0.0115 | 0.0112 | ||||
2 | AB | 0.0083 | 0.0052 | 0.0119 | 0.0234 | 0.0164 | 0.0020 | |||
MSE | 0.0258 | 0.0115 | 0.0110 | 0.0127 | 0.0116 | 0.0114 | ||||
3 | AB | 0.0041 | 0.0045 | 0.0122 | 0.0222 | 0.0166 | 0.0026 | |||
MSE | 0.0247 | 0.0112 | 0.0108 | 0.0124 | 0.0113 | 0.0112 |
n | m | k | Scheme | ˆα2ML | ˆα2BS | ˆα2BL | ˆα2BL | ˆα2BG | ˆα2BG | |
(h=1.5) | (h=−1.5) | (c=0.5) | (c=−0.5) | |||||||
60 | 40 | 25 | 1 | AB | 0.0130 | 0.0025 | 0.0225 | 0.0287 | 0.0145 | 0.0032 |
MSE | 0.0337 | 0.0092 | 0.0093 | 0.0105 | 0.0093 | 0.0092 | ||||
2 | AB | 0.0120 | 0.0005 | 0.0245 | 0.0267 | 0.0165 | 0.0052 | |||
MSE | 0.0335 | 0.0090 | 0.0092 | 0.0102 | 0.0092 | 0.0090 | ||||
3 | AB | 0.0105 | 0.0038 | 0.0212 | 0.0299 | 0.0131 | 0.0019 | |||
MSE | 0.0300 | 0.0089 | 0.0089 | 0.0102 | 0.0089 | 0.0088 | ||||
30 | 1 | AB | 0.0137 | 0.0013 | 0.0237 | 0.0275 | 0.0156 | 0.0043 | ||
MSE | 0.0342 | 0.0093 | 0.0095 | 0.0106 | 0.0095 | 0.0093 | ||||
2 | AB | 0.0131 | 0.0031 | 0.0220 | 0.0293 | 0.0139 | 0.0026 | |||
MSE | 0.0345 | 0.0092 | 0.0092 | 0.0105 | 0.0093 | 0.0092 | ||||
3 | AB | 0.0115 | 0.0042 | 0.0207 | 0.0304 | 0.0127 | 0.0014 | |||
MSE | 0.0310 | 0.0090 | 0.0090 | 0.0104 | 0.0091 | 0.0090 | ||||
50 | 25 | 1 | AB | 0.0115 | 0.0037 | 0.0198 | 0.0283 | 0.0122 | 0.0016 | |
MSE | 0.0281 | 0.0099 | 0.0098 | 0.0111 | 0.0099 | 0.0098 | ||||
2 | AB | 0.0100 | 0.0005 | 0.0230 | 0.0250 | 0.0154 | 0.0048 | |||
MSE | 0.0269 | 0.0096 | 0.0097 | 0.0107 | 0.0098 | 0.0096 | ||||
3 | AB | 0.0118 | 0.0034 | 0.0198 | 0.0277 | 0.0123 | 0.0019 | |||
MSE | 0.0258 | 0.0095 | 0.0094 | 0.0107 | 0.0095 | 0.0094 | ||||
30 | 1 | AB | 0.0114 | 0.0012 | 0.0223 | 0.0257 | 0.0147 | 0.0041 | ||
MSE | 0.0285 | 0.0097 | 0.0097 | 0.0108 | 0.0098 | 0.0096 | ||||
2 | AB | 0.0096 | 0.0009 | 0.0225 | 0.0254 | 0.0150 | 0.0044 | |||
MSE | 0.0277 | 0.0093 | 0.0094 | 0.0104 | 0.0094 | 0.0093 | ||||
3 | AB | 0.0106 | 0.0029 | 0.0203 | 0.0272 | 0.0128 | 0.0024 | |||
MSE | 0.0257 | 0.0095 | 0.0095 | 0.0107 | 0.0096 | 0.0095 |
n | m | k | Scheme | ˆβML | ˆβBS | ˆβBL | ˆβBL | ˆβBG | ˆβBG | |
(h=1.5) | (h=−1.5) | (c=0.5) | (c=−0.5) | |||||||
60 | 40 | 25 | 1 | AB | 0.0790 | 0.0116 | 0.0200 | 0.0447 | 0.0045 | 0.0062 |
MSE | 0.2168 | 0.0041 | 0.0042 | 0.0061 | 0.0040 | 0.0040 | ||||
2 | AB | 0.0750 | 0.0108 | 0.0205 | 0.0435 | 0.0051 | 0.0055 | |||
MSE | 0.2000 | 0.0042 | 0.0044 | 0.0062 | 0.0042 | 0.0042 | ||||
3 | AB | 0.0774 | 0.0106 | 0.0201 | 0.0427 | 0.0050 | 0.0054 | |||
MSE | 0.1685 | 0.0043 | 0.0045 | 0.0062 | 0.0042 | 0.0042 | ||||
30 | 1 | AB | 0.0851 | 0.0106 | 0.0210 | 0.0437 | 0.0054 | 0.0053 | ||
MSE | 0.2232 | 0.0040 | 0.0042 | 0.0059 | 0.0039 | 0.0039 | ||||
2 | AB | 0.0781 | 0.0116 | 0.0197 | 0.0444 | 0.0042 | 0.0063 | |||
MSE | 0.1966 | 0.0043 | 0.0044 | 0.0063 | 0.0041 | 0.0042 | ||||
3 | AB | 0.0716 | 0.0110 | 0.0196 | 0.0430 | 0.0045 | 0.0058 | |||
MSE | 0.1690 | 0.0044 | 0.0045 | 0.0063 | 0.0043 | 0.0043 | ||||
50 | 25 | 1 | AB | 0.0729 | 0.0115 | 0.0194 | 0.0439 | 0.0042 | 0.0063 | |
MSE | 0.1736 | 0.0043 | 0.0044 | 0.0063 | 0.0042 | 0.0042 | ||||
2 | AB | 0.0735 | 0.0089 | 0.0219 | 0.0412 | 0.0068 | 0.0037 | |||
MSE | 0.1701 | 0.0042 | 0.0045 | 0.0059 | 0.0041 | 0.0041 | ||||
3 | AB | 0.0669 | 0.0106 | 0.0198 | 0.0424 | 0.0049 | 0.0054 | |||
MSE | 0.1502 | 0.0043 | 0.0044 | 0.0061 | 0.0042 | 0.0042 | ||||
30 | 1 | AB | 0.0765 | 0.0093 | 0.0217 | 0.0417 | 0.0064 | 0.0040 | ||
MSE | 0.1721 | 0.0042 | 0.0044 | 0.0060 | 0.0041 | 0.0041 | ||||
2 | AB | 0.0697 | 0.0101 | 0.0208 | 0.0424 | 0.0056 | 0.0049 | |||
MSE | 0.1724 | 00042 | 0.0044 | 0.0060 | 0.0041 | 0.0041 | ||||
3 | AB | 0.0670 | 0.0103 | 0.0202 | 0.0421 | 0.0052 | 0.0051 | |||
MSE | 0.1477 | 0.0042 | 0.0044 | 0.0060 | 0.0041 | 0.0041 |
n | m | k | Scheme | ˆβML | ˆβBS | ˆβBL | ˆβ1BL | ˆβBG | ˆβBG | |
(h=1.5) | (h=−1.5) | (c=0.5) | (c=−0.5) | |||||||
60 | 40 | 25 | 1 | AB | 0.0551 | 0.0221 | 0.0064 | 0.0533 | 0.0066 | 0.0125 |
MSE | 0.0799 | 0.0045 | 0.0039 | 0.0072 | 0.0041 | 0.0042 | ||||
2 | AB | 0.0592 | 0.0232 | 0.0047 | 0.0537 | 0.0049 | 0.0139 | |||
MSE | 0.0729 | 0.0046 | 0.0039 | 0.0073 | 0.0041 | 0.0043 | ||||
3 | AB | 0.0468 | 0.0212 | 0.0061 | 0.0511 | 0.0063 | 0.0120 | |||
MSE | 0.0556 | 0.0043 | 0.0037 | 0.0067 | 0.0039 | 0.0040 | ||||
30 | 1 | AB | 0.0635 | 0.0219 | 0.0065 | 0.0529 | 0.0068 | 0.0123 | ||
MSE | 0.0792 | 0.0046 | 0.0039 | 0.0072 | 0.0041 | 0.0042 | ||||
2 | AB | 0.0647 | 0.0232 | 0.0046 | 0.0537 | 0.0048 | 0.0139 | |||
MSE | 0.0708 | 0.0047 | 0.0040 | 0.0074 | 0.0042 | 0.0044 | ||||
3 | AB | 0.0487 | 0.0219 | 0.0054 | 0.0517 | 0.0056 | 0.0127 | |||
MSE | 0.0546 | 0.0042 | 0.0036 | 0.0067 | 0.0038 | 0.0039 | ||||
50 | 25 | 1 | AB | 0.0521 | 0.0230 | 0.0048 | 0.0534 | 0.0050 | 0.0137 | |
MSE | 0.0644 | 0.0045 | 0.0037 | 0.0071 | 0.0040 | 0.0042 | ||||
2 | AB | 0.0511 | 0.0223 | 0.0054 | 0.0525 | 0.0056 | 0.0130 | |||
MSE | 0.0637 | 0.0046 | 0.0039 | 0.0071 | 0.0041 | 0.0042 | ||||
3 | AB | 0.0475 | 0.0208 | 0.0064 | 0.0506 | 0.0066 | 0.0117 | |||
MSE | 0.0543 | 0.0044 | 0.0038 | 0.0068 | 0.0040 | 0.0042 | ||||
30 | 1 | AB | 0.0565 | 0.0229 | 0.0050 | 0.0533 | 0.0052 | 0.0135 | ||
MSE | 0.0646 | 0.0045 | 0.0038 | 0.0071 | 0.0040 | 0.0042 | ||||
2 | AB | 0.0536 | 0.0230 | 0.0047 | 0.0533 | 0.0049 | 0.0137 | |||
MSE | 0.0638 | 0.0046 | 0.0038 | 0.0072 | 0.0040 | 0.0042 | ||||
3 | AB | 0.0462 | 0.0216 | 0.0057 | 0.0513 | 0.0059 | 0.0124 | |||
MSE | 0.0535 | 0.0044 | 0.0037 | 0.0068 | 0.0039 | 0.0040 |
n | t | m | k | Scheme | ˆℜML | ˆℜBS | ˆℜBL | ˆℜBL | ˆℜBG | ˆℜBG | |
(h=1.5) | (h=−1.5) | (c=0.5) | (c=−0.5) | ||||||||
60 | 0.1 | 40 | 25 | 1 | AE | 0.9987 | 0.9987 | 0.9987 | 0.9987 | 0.9987 | 0.9987 |
MSE | 1.2437*10−7 | 3.6402*10−8 | 3.6408*10−8 | 3.6396*10−8 | 3.6408*10−8 | 3.6404*10−8 | |||||
2 | AE | 0.99870 | 0.9987 | 0.9987 | 0.9987 | 0.9987 | 0.9987 | ||||
MSE | 1.0923*10−7 | 3.5807*10−8 | 3.5812*10−8 | 3.5818*10−8 | 3.5812*10−8 | 3.5809*10−8 | |||||
3 | AE | 0.9987 | 0.9987 | 0.9987 | 0.9987 | 0.9987 | 0.9987 | ||||
MSE | 8.5362*10−8 | 3.2401*10−8 | 3.2406*10−8 | 3.2397*10−8 | 3.2406*10−8 | 3.2403*10−8 | |||||
30 | 1 | AE | 0.9988 | 0.9987 | 0.9987 | 0.9987 | 0.9987 | 0.9987 | |||
MSE | 1.226*10−7 | 3.567*10−8 | 3.567*10−8 | 3.566*10−8 | 3.567*10−8 | 3.566*10−8 | |||||
2 | AE | 0.9987 | 0.9987 | 0.9987 | 0.9987 | 0.9987 | 0.9987 | ||||
MSE | 10.36*10−8 | 34.04*10−9 | 34.04*10−9 | 34.06*10−9 | 34.04*10−9 | 34.04*10−9 | |||||
3 | AE | 0.9987 | 0.9987 | 0.9987 | 0.9987 | 0.9987 | 0.9987 | ||||
MSE | 8.526*10−8 | 3.2184*10−8 | 3.2188*10−8 | 3.2180*10−8 | 3.2188*10−8 | 3.2185*10−8 | |||||
50 | 25 | 1 | AE | 0.9987 | 0.9987 | 0.9987 | 0.9987 | 0.9987 | 0.9987 | ||
MSE | 9.770*10−8 | 3.3138*10−8 | 3.3134*10−8 | 3.3134*10−8 | 3.3143*10−8 | 3.3140*10−8 | |||||
2 | AE | 0.9987 | 0.9987 | 0.9987 | 0.9987 | 0.9987 | 0.9987 | ||||
MSE | 9.3246*10−8 | 3.2173*10−8 | 3.2178*10−8 | 3.2169*10−8 | 3.2178*10−8 | 3.2175*10−8 | |||||
3 | AE | 0.9987 | 0.9987 | 0.9987 | 0.9987 | 0.9987 | 0.9987 | ||||
MSE | 8.0102*10−8 | 2.9671*10−8 | 2.9674*10−8 | 2.9668*10−8 | 2.9674*10−8 | 2.9672*10−8 | |||||
30 | 1 | AE | 0.9987 | 0.9987 | 0.9987 | 0.9987 | 0.9987 | 0.9987 | |||
MSE | 9.900*10−8 | 3.3295*10−8 | 3.3300*10−8 | 3.3291*10−8 | 3.330*10−8 | 3.3297*10−8 | |||||
2 | AE | 0.9987 | 0.9987 | 0.9987 | 0.9987 | 0.9987 | 0.9987 | ||||
MSE | 9.445*10−8 | 3.2535*10−8 | 3.2539*10−8 | 3.2530*10−8 | 3.2539*10−8 | 3.2536*10−8 | |||||
3 | AE | 0.9987 | 0.9987 | 0.9987 | 0.9987 | 0.9987 | 0.9987 | ||||
MSE | 7.702*10−8 | 2.8550*10−8 | 2.8554*10−8 | 2.8547*10−8 | 2.8554*10−8 | 2.8552*10−8 | |||||
0.5 | 40 | 25 | 1 | AE | 0.8587 | 0.8616 | 0.8613 | 0.8621 | 0.8612 | 0.8615 | |
MSE | 0.0011 | 34.93*10−5 | 35.34*10−5 | 34.53*10−5 | 35.46*10−5 | 35.10*10−5 | |||||
2 | AE | 0.8597 | 0.8621 | 0.8617 | 0.8624 | 0.8616 | 0.8619 | ||||
MSE | 9.393*10−4 | 34.41*10−5 | 34.78*10−5 | 34.07*10−5 | 34.88*10−5 | 34.56*10−5 | |||||
3 | AE | 0.8600 | 0.8619 | 0.8616 | 0.8622 | 0.8616 | 0.8618 | ||||
MSE | 7.479*10−4 | 31.24*10−5 | 31.55*10−5 | 30.96*10−5 | 31.63*10−5 | 31.37*10−5 | |||||
30 | 1 | AE | 0.8583 | 0.8615 | 0.8611 | 0.8618 | 0.8610 | 0.8613 | |||
MSE | 10.44*10−4 | 34.12*10−5 | 34.57*10−5 | 33.71*10−5 | 34.68*10−5 | 34.30*10−5 | |||||
2 | AE | 0.8587 | 0.8615 | 0.8611 | 0.8618 | 0.6810 | 0.8613 | ||||
MSE | 8.931*10−4 | 32.58*10−5 | 32.99*10−5 | 32.21*10−5 | 33.10*10−5 | 32.75*10−5 | |||||
3 | AE | 0.8598 | 0.8618 | 0.8615 | 0.8621 | 0.8614 | 0.8617 | ||||
MSE | 7.448*10−4 | 30.98*10−5 | 31.30*10−5 | 30.69*10−5 | 31.38*10−5 | 31.11*10−5 | |||||
50 | 25 | 1 | AE | 0.8600 | 0.8621 | 0.8618 | 0.8624 | 0.8617 | 0.8620 | ||
MSE | 8.528*10−4 | 32.01*10−5 | 32.30*10−5 | 31.73*10−5 | 32.39*10−5 | 32.13*10−5 | |||||
2 | AE | 0.8601 | 0.8622 | 0.8618 | 0.8625 | 0.8618 | 0.8620 | ||||
MSE | 8.140*10−4 | 31.06*10−5 | 31.35*10−5 | 30.80*10−5 | 31.43*10−5 | 31.18*10−5 | |||||
3 | AE | 0.8609 | 0.8626 | 0.8623 | 0.8629 | 0.8622 | 0.8624 | ||||
MSE | 7.049*10−4 | 28.76*10−5 | 28.97*10−5 | 28.56*10−5 | 29.03*10−5 | 28.85*10−5 | |||||
30 | 1 | AE | 0.8597 | 0.8620 | 0.8617 | 0.8623 | 0.8616 | 0.8619 | |||
MSE | 8.597*10−4 | 32.09*10−5 | 32.40*10−5 | 31.81*10−5 | 32.49*10−5 | 32.22*10−5 | |||||
2 | AE | 0.8600 | 0.8622 | 0.8619 | 0.8625 | 0.8618 | 0.8620 | ||||
MSE | 8.218*10−4 | 31.37*10−5 | 31.67*10−5 | 31.11*10−5 | 31.75*10−5 | 31.50*10−5 | |||||
3 | AE | 0.8605 | 0.8623 | 0.8620 | 0.8626 | 0.8619 | 0.8622 | ||||
MSE | 6.793*10−4 | 27.68*10−5 | 27.89*10−5 | 27.47*10−5 | 27.96*10−5 | 27.76*10−5 |
n | t | m | k | Scheme | ˆℏML | ˆℏBS | ˆℏBL | ˆℏBL | ˆℏBG | ˆℏBG | |
(h=1.5) | (h=−1.5) | (c=0.5) | (c=−0.5) | ||||||||
60 | 0.1 | 40 | 25 | 1 | AE | 0.03922 | 0.03814 | 0.038102 | 0.03818 | 0.03718 | 0.03782 |
MSE | 1.11*10−4 | 3.28*10−5 | 3.26*10−5 | 3.30*10−5 | 3.133*10−5 | 3.21*10−5 | |||||
2 | AE | 0.0389 | 0.03801 | 0.03797 | 0.03804 | 0.03710 | 0.03771 | ||||
MSE | 9.836*10−5 | 3.227*10−5 | 3.213*10−5 | 3.241*10−5 | 3.097*10−5 | 3.165*10−5 | |||||
3 | AE | 0.03870 | 0.03802 | 0.03799 | 0.3805 | 0.03727 | 0.03777 | ||||
MSE | 7.687*10−5 | 2.920*10−5 | 2.908*10−5 | 2.931*10−5 | 2.799*10−5 | 2.867*10−5 | |||||
30 | 1 | AE | 0.0393 | 0.03820 | 0.03816 | 0.03823 | 0.03725 | 0.03788 | |||
MSE | 1.104*10−4 | 3.214*10−5 | 3.198*10−5 | 3.231*10−5 | 3.041*10−5 | 3.136*10−5 | |||||
2 | AE | 0.03916 | 0.03818 | 0.03184 | 0.03821 | 0.03728 | 0.03788 | ||||
MSE | 9.333*10−5 | 3.067*10−5 | 3.052*10−5 | 3.083*10−5 | 2.905*10−5 | 2.995*10−5 | |||||
3 | AE | 0.03876 | 0.03806 | 0.03803 | 0.03809 | 0.03705 | 0.037809 | ||||
MSE | 7.677*10−5 | 2.900*10−5 | 2.889*10−5 | 2.912*10−5 | 2.77*10−5 | 2.845*10−5 | |||||
50 | 25 | 1 | AE | 0.03875 | 0.03797 | 0.037938 | 0.03800 | 0.03714 | 0.03769 | ||
MSE | 8.798*10−5 | 2.986*10−5 | 2.975*10−5 | 2.998*10−5 | 2.887*10−5 | 2.938*10−5 | |||||
2 | AE | 0.03872 | 0.03496 | 0.03793 | 0.03799 | 0.03714 | 0.03769 | ||||
MSE | 8.397*10−5 | 2.899*10−5 | 2.888*10−5 | 2.911*10−5 | 2.802*10−5 | 2.852*10−5 | |||||
3 | AE | 0.03841 | 0.03782 | 0.03779 | 0.03785 | 0.03709 | 0.03758 | ||||
MSE | 7.213*10−5 | 2.674*10−5 | 2.665*10−5 | 2.682*10−5 | 2.609*10−5 | 2.640*10−5 | |||||
30 | 1 | AE | 0.03883 | 0.03802 | 0.03798 | 0.03805 | 0.03719 | 0.03774 | |||
MSE | 8.915*10−5 | 3.00*10−5 | 2.988*10−5 | 3.012*10−5 | 2.893*10−5 | 2.949*10−5 | |||||
2 | AE | 0.03873 | 0.03796 | 0.03793 | 0.03799 | 0.03714 | 0.03377 | ||||
MSE | 8.505*10−5 | 2.932*10−5 | 2.920*10−5 | 2.943*10−5 | 2.833*10−5 | 2.884*10−5 | |||||
3 | AE | 0.03853 | 0.03790 | 0.037874 | 0.037931 | 0.03717 | 0.03766 | ||||
MSE | 6.936*10−5 | 2.573*10−5 | 2.564*10−5 | 2.582*10−5 | 2.499*10−5 | 2.536*10−5 | |||||
0.5 | 40 | 25 | 1 | AE | 0.8603 | 0.8440 | 0.8284 | 0.8606 | 0.8253 | 0.8378 | |
MSE | 0.0408 | 0.0146 | 0.0137 | 0.0162 | 0.0142 | 0.0144 | |||||
2 | AE | 0.8541 | 0.8413 | 0.8266 | 0.8568 | 0.8237 | 0.8354 | ||||
MSE | 0.0361 | 0.0144 | 0.0135 | 0.0158 | 0.0140 | 0.0142 | |||||
3 | AE | 0.8522 | 0.8421 | 0.8298 | 0.8548 | 0.8275 | 0.8372 | ||||
MSE | 0.0287 | 0.0131 | 0.0123 | 0.0142 | 0.0127 | 0.0129 | |||||
30 | 1 | AE | 0.8628 | 0.8454 | 0.8300 | 0.8616 | 0.8271 | 0.8393 | |||
MSE | 0.0401 | 0.0143 | 0 0133 | 0.0160 | 0.0137 | 0.0140 | |||||
2 | AE | 0.8604 | 0.8451 | 0.8305 | 0.8605 | 0.8277 | 0.8393 | ||||
MSE | 0.0343 | 0.0136 | 0.0127 | 0.0151 | 0.0131 | 0.0134 | |||||
3 | AE | 0.8534 | 0.8428 | 0.8306 | 0.8557 | 0.8283 | 0.8380 | ||||
MSE | 0.0286 | 0.0130 | 0.0122 | 0.0141 | 0.0125 | 0.0128 | |||||
50 | 25 | 1 | AE | 0.8524 | 0.8406 | 0.8273 | 0.8546 | 0.8247 | 0.8353 | ||
MSE | 0.0328 | 0.0133 | 0.0127 | 0.0145 | 0.0131 | 0.0132 | |||||
2 | AE | 0.8519 | 0.8405 | 0.8273 | 0.8543 | 0.8247 | 0.8352 | ||||
MSE | 0.0313 | 0.0130 | 0.0123 | 0.0141 | 0.0127 | 0.0128 | |||||
3 | AE | 0.8466 | 0.8377 | 0.8261 | 0.8499 | 0.8568 | 0.8331 | ||||
MSE | 0.0271 | 0.0120 | 0.0115 | 0.0128 | 0.0118 | 0.0119 | |||||
30 | 1 | AE | 0.8539 | 0.8417 | 0.8283 | 0.8557 | 0.8257 | 0.8363 | |||
MSE | 0.0330 | 0.0134 | 0.0127 | 0.0146 | 0.0131 | 0.0133 | |||||
2 | AE | 0.8522 | 0.8405 | 0.8274 | 0.8544 | 0.8247 | 0.8353 | ||||
MSE | 0.0316 | 0.0131 | 0.0124 | 0.0142 | 0.0128 | 0.0129 | |||||
3 | AE | 0.8491 | 0.8350 | 0.8278 | 0.8516 | 0.8255 | 0.8348 | ||||
MSE | 0.0261 | 0.0115 | 0.0110 | 0.0124 | 0.0113 | 0.0114 |
t | (n,m,k) | Scheme | ˆℜML | ˆℜBS | ˆℜBL | ˆℜBL | ˆℜBG | ˆℜBG | |
(h=1.5) | (h=−1.5) | (c=0.5) | (c=−0.5) | ||||||
0.1 | (60, 40, 25) | 1 | AE | 0.6471 | 0.6573 | 0.6565 | 0.6581 | 0.6560 | 0.6587 |
MSE | 0.0032 | 536*10−6 | 541*10−6 | 533*10−6 | 546*10−6 | 539*10−6 | |||
2 | AE | 0.6493 | 0.6577 | 0.6569 | 0.6585 | 0.6564 | 0.6573 | ||
MSE | 0.0029 | 538*10−6 | 542*10−6 | 535*10−6 | 547*10−6 | 540*10−6 | |||
3 | AE | 0.6510 | 0.6573 | 0.6565 | 0.6581 | 0.6561 | 0.6569 | ||
MSE | 0.0023 | 530*10−6 | 535*10−6 | 527*10−6 | 540*10−6 | 533*10−6 | |||
(60, 40, 30) | 1 | AE | 0.6479 | 0.6576 | 0.6568 | 0.6585 | 0.6563 | 0.6572 | |
MSE | 0.0031 | 524*10−6 | 528*10−6 | 521*10−6 | 532*10−6 | 526*10−6 | |||
2 | AE | 0.6485 | 0.6575 | 0.6566 | 0.6583 | 0.6562 | 0.6570 | ||
MSE | 0.0029 | 531*10−6 | 535*10−6 | 528*10−6 | 541*10−6 | 534*10−6 | |||
3 | AE | 0.6507 | 0.6571 | 0.6563 | 0.6579 | 0.6559 | 0.6567 | ||
MSE | 0.0023 | 538*10−6 | 543*10−6 | 534*10−6 | 548*10−6 | 541*10−6 | |||
(60, 50, 25) | 1 | AE | 0.6473 | 0.6570 | 0.6562 | 0.6577 | 0.6558 | 0.6566 | |
MSE | 0.0027 | 544*10−6 | 549*10−6 | 541*10−6 | 554*10−6 | 547*10−6 | |||
2 | AE | 0.6495 | 0.6579 | 0.6571 | 0.6586 | 0.6567 | 0.6575 | ||
MSE | 0.0025 | 532*10−6 | 535*10−6 | 530*10−6 | 539*10−6 | 534*10−6 | |||
3 | AE | 0.6496 | 0.6571 | 0.6564 | 0.6579 | 0.6560 | 0.6568 | ||
MSE | 0.0022 | 533*10−6 | 537*10−6 | 530*10−6 | 542*10−6 | 536*10−6 | |||
(60, 50, 30) | 1 | AE | 0.6494 | 0.6580 | 0.6572 | 0.6587 | 0.6568 | 0.6576 | |
MSE | 0.0026 | 532*10−6 | 535*10−6 | 530*10−6 | 539*10−6 | 534*10−6 | |||
2 | AE | 0.6485 | 0.6574 | 0.6566 | 0.6581 | 0.6562 | 0.6570 | ||
MSE | 0.0025 | 524*10−6 | 528*10−6 | 522*10−6 | 533*10−6 | 527*10−6 | |||
3 | AE | 0.6498 | 0.6572 | 0.6565 | 0.6580 | 0.6561 | 0.6569 | ||
MSE | 0.0022 | 527*10−6 | 531*10−6 | 523*10−6 | 535*10−6 | 529*10−6 | |||
0.5 | (60, 40, 25) | 1 | ES | 0.2057 | 0.2069 | 0.2059 | 0.2079 | 0.2020 | 0.2052 |
MSE | 0.0026 | 658*10−6 | 647*10−6 | 671*10−6 | 651*10−6 | 650*10−6 | |||
2 | AE | 0.2072 | 0.2073 | 0.2063 | 0.2082 | 0.2024 | 0.2056 | ||
MSE | 0.0024 | 662*10−6 | 651*10−6 | 676*10−6 | 652*10−6 | 654*10−6 | |||
3 | AE | 0.2077 | 0.2066 | 0.2057 | 0.2076 | 0.2020 | 0.2051 | ||
MSE | 0.0019 | 642*10−6 | 632*10−6 | 654*10−6 | 639*10−6 | 636*10−6 | |||
(60, 40, 30) | 1 | AE | 0.2063 | 0.2072 | 0.2062 | 0.2082 | 0.2022 | 0.2056 | |
MSE | 0.0025 | 648*10−6 | 636*10−6 | 662*10−6 | 638*10−6 | 639*10−6 | |||
2 | AE | 0.2065 | 0.2070 | 0.2060 | 0.2080 | 0.2021 | 0.2054 | ||
MSE | 0.0023 | 650*10−6 | 639*10−6 | 663*10−6 | 642*10−6 | 642*10−6 | |||
3 | AE | 0.2074 | 0.2065 | 0.2055 | 0.2074 | 0.2019 | 0.2050 | ||
MSE | 0.0019 | 645*10−6 | 636*10−6 | 657*10−6 | 644*10−6 | 640*10−6 | |||
(60, 50, 25) | 1 | AE | 0.2047 | 0.2062 | 0.2053 | 0.2071 | 0.2019 | 0.2048 | |
MSE | 0.0021 | 659*10−6 | 651*10−6 | 671*10−6 | 657*10−6 | 654*10−6 | |||
2 | AE | 0.2065 | 0.2072 | 0.2063 | 0.2081 | 0.2028 | 0.2057 | ||
MSE | 0.0021 | 656*10−6 | 645*10−6 | 668*10−6 | 645*10−6 | 648*10−6 | |||
3 | AE | 0.2059 | 0.2062 | 0.2054 | 0.2071 | 0.2021 | 0.2049 | ||
MSE | 0.0017 | 641*10−6 | 632*10−6 | 651*10−6 | 638*10−6 | 636*10−6 | |||
(60, 50, 30) | 1 | AE | 0.2065 | 0.2073 | 0.2064 | 0.2082 | 0.2029 | 0.2058 | |
MSE | 0.0021 | 655*10−6 | 644*10−6 | 667*10−6 | 642*10−6 | 646*10−6 | |||
2 | AE | 0.2055 | 0.2066 | 0.2057 | 0.2075 | 0.2023 | 0.2052 | ||
MSE | 0.0020 | 640*10−6 | 631*10−6 | 652*10−6 | 634*10−6 | 634*10−6 | |||
3 | AE | 0.2061 | 0.2063 | 0.2055 | 0.2072 | 0.2022 | 0.2049 | ||
MSE | 0.0017 | 632*10−6 | 624*10−6 | 642*10−6 | 629*10−6 | 627*10−6 |
t | (n,m,k) | Scheme | ˆℏML | ˆℏBS | ˆℏBL | ˆℏBL | ˆℏBG | ˆℏBG | |
(h=1.5) | (h=−1.5) | (c=0.5) | (c=−0.5) | ||||||
0.1 | (60, 40, 25) | 1 | AE | 3.9493 | 3.8496 | 3.7028 | 4.0153 | 3.8095 | 3.8362 |
MSE | 0.5200 | 0.0999 | 0.1028 | 0.1507 | 0.09823 | 0.09896 | |||
2 | AE | 3.9209 | 3.8441 | 3.6997 | 4.0068 | 3.8048 | 3.8310 | ||
MSE | 0.4718 | 0.0998 | 0.1036 | 0.1477 | 0.0985 | 0.0990 | |||
3 | AE | 3.894 | 3.8490 | 3.7112 | 4.0029 | 3.8116 | 3.8365 | ||
MSE | 0.3638 | 0.0985 | 0.0994 | 0.1446 | 0.0966 | 0.0975 | |||
(60, 40, 30) | 1 | AE | 3.9388 | 3.8450 | 3.6985 | 4.0103 | 3.8050 | 3.8316 | |
MSE | 0.5054 | 0.0972 | 0.1018 | 0.1458 | 0.0960 | 0.0964 | |||
2 | AE | 3.9294 | 3.8472 | 3.7026 | 4.0101 | 3.8078 | 3.8341 | ||
MSE | 0.4694 | 0.0987 | 0.1019 | 0.1475 | 0.0972 | 0.0978 | |||
3 | AE | 3.8991 | 3.8513 | 3.7134 | 4.0054 | 3.8139 | 3.8388 | ||
MSE | 0.3717 | 0.1001 | 0.1001 | 0.1473 | 0.0981 | 0.0991 | |||
(60, 50, 25) | 1 | AE | 3.9448 | 3.8529 | 3.7210 | 3.9997 | 3.8172 | 3.8410 | |
MSE | 0.4297 | 0.1014 | 0.01011 | 0.1444 | 0.0996 | 0.1005 | |||
2 | AE | 3.9165 | 3.8407 | 3.7099 | 3.9861 | 3.8051 | 3.8288 | ||
MSE | 0.4039 | 0.0985 | 0.1015 | 0.1370 | 0.0976 | 0.0978 | |||
3 | AE | 3.9131 | 3.8502 | 3.7248 | 3.9889 | 3.8166 | 3.8389 | ||
MSE | 0.3468 | 0.0993 | 0.0984 | 0.1387 | 0.0975 | 0.0984 | |||
(60, 50, 30) | 1 | AE | 3.9179 | 3.8396 | 3.7085 | 3.9855 | 3.8040 | 3.8277 | |
MSE | 0.4118 | 0.0985 | 0.1019 | 0.1370 | 0.0977 | 0.0980 | |||
2 | AE | 3.9284 | 3.8472 | 3.7161 | 3.9931 | 3.8117 | 3.8354 | ||
MSE | 0.4006 | 0.0974 | 0.0989 | 0.1379 | 0.0961 | 0.0967 | |||
3 | AE | 3.9102 | 3.8490 | 3.7237 | 3.9875 | 3.8151 | 3.8377 | ||
MSE | 0.3435 | 0.0980 | 0.0976 | 0.1369 | 0.0964 | 0.0972 | |||
0.5 | (60, 40, 25) | 1 | AE | 2.4150 | 2.3035 | 2.2597 | 2.3499 | 2.2841 | 2.2971 |
MSE | 0.0613 | 0.02778 | 0.0274 | 0.0325 | 0.0276 | 0.0276 | |||
2 | AE | 2.2319 | 2.3007 | 2.2573 | 2.3465 | 2.2814 | 2.2943 | ||
MSE | 0.0579 | 0.0276 | 0.0275 | 0.0320 | 0.0275 | 0.0275 | |||
3 | AE | 2.2257 | 2.3039 | 2.2619 | 2.3482 | 2.2853 | 2.2977 | ||
MSE | 0.0452 | 0.0270 | 0.0264 | 0.0315 | 0.0267 | 0.0268 | |||
(60, 40, 30) | 1 | AE | 2.2385 | 2.3012 | 2.2574 | 2.3474 | 2.2818 | 2.2947 | |
MSE | 0.0609 | 0.0271 | 0.0270 | 0.0315 | 0.0270 | 0.0270 | |||
2 | AE | 2.2353 | 2.3022 | 2.2588 | 2.3481 | 2.2830 | 2.2958 | ||
MSE | 0.0569 | 0.0273 | 0.0270 | 0.03178 | 0.0271 | 0.0272 | |||
3 | AE | 2.2273 | 2.3051 | 2.2630 | 2.3494 | 2.2865 | 2.2989 | ||
MSE | 0.0454 | 0.0273 | 0.0267 | 0.0320 | 0.0270 | 0.0271 | |||
(60, 50, 25) | 1 | AE | 2.2445 | 2.3059 | 2.2664 | 2.3472 | 2.2884 | 2.3001 | |
MSE | 0.0514 | 0.0281 | 0.0275 | 0.0323 | 0.0279 | 0.0280 | |||
2 | AE | 2.2357 | 2.2996 | 2.2604 | 2.3407 | 2.2822 | 2.2938 | ||
MSE | 0.0508 | 0.0274 | 0.0273 | 0.0310 | 0.02736 | 0.2733 | |||
3 | AE | 2.2353 | 2.3048 | 2.2669 | 2.3446 | 2.2881 | 2.2993 | ||
MSE | 0.0424 | 0.0273 | 0.0266 | 0.0313 | 0.0270 | 0.0272 | |||
(60, 50, 30) | 1 | AE | 2.2352 | 2.2988 | 2.2596 | 2.3399 | 2.2814 | 2.2930 | |
MSE | 0.0511 | 0.0274 | 0.0273 | 0.0391 | 0.0274 | 0.0273 | |||
2 | AE | 2.240 | 2.3031 | 2.2638 | 2.3443 | 2.2857 | 2.293 | ||
MSE | 0.0490 | 0.0271 | 0.0267 | 0.0309 | 0.0269 | 0.0270 | |||
3 | AE | 2.2344 | 2.3042 | 2.2663 | 2.3439 | 2.2875 | 2.2987 | ||
MSE | 0.0422 | 0.0270 | 0.0263 | 0.0309 | 0.0267 | 0.0269 |
m | k | Scheme | ACI | Boot-p | Gamma prior | |||
Boot-p | Boot-t | BCI | HPD | |||||
40 | 25 | 1 | α1 | 1.397(0.9959) | 0.429(0.9300) | 0.747(0.8784) | 0.518(0.9912) | 0.506(0.9858) |
α2 | 2.873(0.9995) | 0.440(0.9322) | 0.732(0.8796) | 0.6022(0.9983) | 0.593(0.9971) | |||
β | 5.322(1.0000) | 1.146(0.9446) | 1.473(0.9846) | 0.776(1.0000) | 0.759(1.0000) | |||
2 | α1 | 1.846(0.9980) | 0.421(0.9371) | 0.668(0.8726) | 0.515(0.9930) | 0.504(0.9885) | ||
α2 | 2.717(0.9999) | 0.430(0.9375) | 0.650(0.8786) | 0.601(0.9990) | 0.592(0.9978) | |||
β | 4.891(0.9999) | 1.081(0.9407) | 1.270(0.9654) | 0.768(1.0000) | 0.752(1.0000) | |||
3 | α1 | 1.857(0.9987) | 0.369(0.9393) | 0.579(0.8798) | 0.480(0.9913) | 0.470(0.9870) | ||
α2 | 2.760(0.9999) | 0.376(0.9413) | 0.570(0.8879) | 0.572(0.9986) | 0.563(0.9972) | |||
β | 5.143(1.0000) | 0.939(0.9389) | 1.202(0.9511) | 0.760(1.0000) | 0.743(1.0000) | |||
30 | 1 | α1 | 1.818(0.9962) | 0.414(0.9328) | 0.774(0.8922) | 0.510(0.9914) | 0.499(0.9860) | |
α2 | 2.695(0.9996) | 0.427(0.9365) | 0.789(0.9088) | 0.594(0.9978) | 0.586(0.9963) | |||
β | 5.145(0.9997) | 1.1435(0.9446) | 1.700(0.9626) | 0.775(1.0000) | 0.758(1.0000) | |||
2 | α1 | 1.839(0.9968) | 0.410(0.9291) | 0.726(0.8944) | 0.512(0.992) | 0.500(0.9882) | ||
α2 | 2.688(0.9999) | 0.421(0.9318) | 0.729(0.9079) | 0.597(0.9987) | 0.588(0.9974) | |||
β | 4.926(0.9999) | 1.078(0.9460) | 1.466(0.9733) | 0.768(1.0000) | 0.751(1.000) | |||
3 | α1 | 1.892(0.9992) | 0.370(0.9386) | 0.588(0.8815) | 0.480(0.9910) | 0.470(0.9870) | ||
α2 | 2.793(1.0000) | 0.376(0.9376) | 0.578(0.8830) | 0.571(0.9985) | 0.563(0.9975) | |||
β | 5.221(1.0000) | 0.941(0.9409) | 1.221(0.9536) | 0.760(1.0000) | 0.743(1.0000) | |||
50 | 25 | 1 | α1 | 1.619(0.9981) | 0.391(0.9363) | 0.581(0.8675) | 0.489(0.9890) | 0.479(0.9856) |
α2 | 2.394(0.9995) | 0.400(0.9385) | 0.571(0.8754) | 0.577(0.9983) | 0.569(0.9973) | |||
β | 4.464(1.0000) | 1.020(0.9438) | 1.185(0.9541) | 0.767(1.0000) | 0.751(1.0000) | |||
2 | α1 | 1.628(0.9976) | 0.389(0.9340) | 0.582(0.8676) | 0.488(0.9894) | 0.478(0.856) | ||
α2 | 2.395(1.0000) | 0.398(0.9361) | 0.572(0.8740) | 0.577(0.9978) | 0.568(0.9955) | |||
β | 4.424(1.0000) | 1.007(0.9430) | 1.171(0.9534) | 0.765(1.0000) | 0.749(1.0000) | |||
3 | α1 | 1.449(0.9979) | 0.358(0.9359) | 0.505(0.8711) | 0.466(0.9902) | 0.456(0.9868) | ||
α2 | 2.144(1.0000) | 0.367(0.9360) | 0.501(0.8799) | 0.557(0.9986) | 0.549(0.9971) | |||
β | 4.009(1.0000) | 0.923(0.9399) | 1.051(0.9533) | 0.759(1.0000) | 0.742(1.0000) | |||
30 | 1 | α1 | 1.644(0.9982) | 0.389(0.9387) | 0.594(0.8773) | 0.489(0.9920) | 0.478(0.9866) | |
α2 | 2.400(1.0000) | 0.398(0.9385) | 0.587(0.8849) | 0.578(0.9977) | 0.569(0.9967) | |||
β | 4.544(1.0000) | 1.020(0.9456) | 1.220(0.9776) | 0.768(1.0000) | 0.751(1.0000) | |||
2 | α1 | 1.612(0.9979) | 0.387(0.9337) | 0.583(0.8728) | 0.489(0.9921) | 0.479(0.9876) | ||
α2 | 2.384(0.9998) | 0.397(0.9356) | 0.576(0.8803) | 0.577(0.9982) | 0.569(0.9967) | |||
β | 4.411(1.0000) | 1.01(0.9437) | 1.177(0.9727) | 0.766(1.0000) | 0.749(1.0000) | |||
3 | α1 | 1.451(0.9984) | 0.358(0.9365) | 0.503(0.8755) | 0.465(0.9908) | 0.455(0.9860) | ||
α2 | 2.124(0.9998) | 0.367(0.9390) | 0.499(0.8738) | 0.557(0.9986) | 0.549(0.9970) | |||
β | 3.983(1.0000) | 0.922(0.9409) | 1.042(0.9564) | 0.759(1.0000) | 0.743(1.0000) |
m | k | Scheme | ACI | Boot-p | Gamma prior | |||
Boot-p | Boot-t | BCI | HPD | |||||
40 | 20 | 1 | ℜ(0.1) | 0.0022(0.9850) | 0.0014(0.9532) | 0.2626(1.0000) | 0.00092(0.986) | 0.00090(0.9846) |
ℜ(0.5) | 0.1847(0.9822) | 0.1266(0.9531) | 0.2838(0.9999) | 0.08888(0.9842) | 0.08808(0.9824) | |||
ℏ(0.1) | 0.0654(0.9850) | 0.0419(0.9532) | 0.0510(0.9274) | 0.0275(0.9860) | 0.0271(0.9846) | |||
ℏ(0.5) | 1.0230(0.9758) | 0.7854(0.9537) | 0.9281(0.9389) | 0.5683(0.9832) | 0.5620(0.9804) | |||
2 | ℜ(0.1) | 0.00194(0.9815) | 0.0013(0.9494) | 0.2622(1.0000) | 0.00088(0.9827) | 0.00087(0.9808) | ||
ℜ(0.5) | 0.1635(0.9760) | 0.1198(0.9497) | 0.2862(0.9999) | 0.0861(0.9803) | 0.0854(0.9787) | |||
ℏ(0.1) | 0.0583(0.9815) | 0.0395(0.9494) | 0.0444(0.9246) | 0.0266(0.9827) | 0.0262(0.9806) | |||
ℏ(0.5) | 0.8977(0.9641) | 0.7428(0.9502) | 0.8107(0.9355) | 0.5504(0.9795) | 0.5547(0.9774) | |||
3 | ℜ(0.1) | 0.0020(0.9836) | 0.0012(0.9441) | 0.2108(1.0000) | 0.00081(0.9786) | 0.00080(0.9758) | ||
ℜ(0.5) | 0.1651(0.9810) | 0.1058(0..9944) | 0.2329(0.9998) | 0.0786(0.9766) | 0.07803(0.9727) | |||
ℏ(0.1) | 0.0599(0.6836) | 0.0345(0.9441) | 0.0413(0.9086) | 0.0243(0.9786) | 0.0240(0.9757) | |||
ℏ(0.5) | 0.8805(0.9692) | 0.6555(0.9442) | 0.7536(0.9231) | 0.5012(0.9750) | 0.4966(0.9700) | |||
30 | 1 | ℜ(0.1) | 0.00226(0.9776) | 0.0014(0.9522) | 0.2685(1.0000) | 0.00091(0.9842) | 0.00090(0.9813) | |
ℜ(0.5) | 0.1911(0.9745) | 0.1273(0.9526) | 0.2858(1.0000) | 0.0881(0.9829) | 0.0873(0.9793) | |||
ℏ(0.1) | 0.0676(0.9776) | 0.0422(0.9522) | 0.0534(0.9334) | 0.0272(0.9843) | 0.0269(0.9814) | |||
ℏ(0.5) | 1.0587(0.9674) | 0.7911(0.9531) | 0.9533(0.9390) | 0.5629(0.9803) | 0.5568(0.9770) | |||
2 | ℜ(0.1) | 0.0202(0.9816) | 0.0013(0.9534) | 0.2659(1.0000) | 0.00088(0.9839) | 0.00087(0.9825) | ||
ℜ(0.5) | 0.1694(0.9787) | 0.1200(0.9538) | 0.2864(0.9999) | 0.0859(0.9823) | 0.0852(0.9807) | |||
ℏ(0.1) | 0.0606(0.9816) | 0.0396(0.9534) | 0.0462(0.9452) | 0.0265(0.9839) | 0.0262(0.9824) | |||
ℏ(0.5) | 0.9274(0.9711) | 0.7448(0.9541) | 0.8259(0.9475) | 0.5494(0.9811) | 0.5438(0.9784) | |||
3 | ℜ(0.1) | 0.0020(0.9821) | 0.0012(0.9457) | 0.2105(1.0000) | 0.00081(0.9782) | 0.00080(0.9762) | ||
ℜ(0.5) | 0.1655(0.9783) | 0.1060(0.9458) | 0.2325(0.9998) | 0.0787(0.9767) | 0.0781(0.9749) | |||
ℏ(0.1) | 0.0599(0.9821) | 0.0346(0.9457) | 0.0418(0.9099) | 0.0243(0.9782) | 0.0240(0.9760) | |||
ℏ(0.5) | 0.8836(0.9688) | 0.6567(0.9469) | 0.7617(0.9259) | 0.5015(0.9750) | 0.4970(0.9728) | |||
50 | 20 | 1 | ℜ(0.1) | 0.00212(0.9834) | 0.0012(0.9475) | 0.2359(1.0000) | 0.00085(0.9810) | 0.00084(0.9802) |
ℜ(0.5) | 0.1800(0.9786) | 0.1145(0.9478) | 0.2558(0.9999) | 0.0822(0.9794) | 0.0816(0.9773) | |||
ℏ(0.1) | 0.00634(0.9833) | 0.0374(0.9475) | 0.0431(0.9198) | 0.0254(0.9809) | 0.0251(0.9802) | |||
ℏ(0.5) | 0.9915(0.9713) | 0.7097(0.9475) | 0.8001(0.9300) | 0.5242(0.9782) | 0.5191(0.9764) | |||
2 | ℜ(0.1) | 0.00191(0.9841) | 0.0012(0.9478) | 0.2373(1.0000) | 0.00084(0.9846) | 0.00083(0.9810) | ||
ℜ(0.5) | 0.1624(0.9807) | 0.1130(0.9477) | 0.2576(0.9998) | 0.0818(0.9830) | 0.0811(0.9797) | |||
ℏ(0.1) | 0.0057(0.9841) | 0.0369(0.9478) | 0.0425(0.9176) | 0.0253(0.9845) | 0.0250(0.9809) | |||
ℏ(0.5) | 0.8979(0.9705) | 0.7006(0.9476) | 0.7885(0.9296) | 0.5213(0.9802) | 0.5162(0.9770) | |||
3 | ℜ(0.1) | 0.00174(0.9833) | 0.0011(0.9438) | 0.2133(1.0000) | 0.00079(0.9799) | 0.00078(0.9770) | ||
ℜ(0.5) | 0.1491(0.9792) | 0.1046(0.9437) | 0.2315(0.9996) | 0.0769(0.9772) | 0.0764(0.9743) | |||
ℏ(0.1) | 0.00522(0.9833) | 0.03 40(0.9437) | 0.0383(0.9219) | 0.0238(0.9800) | 0.0235(0.9770) | |||
ℏ(0.5) | 0.8271(0.9704) | 0.6479(0.9442) | 0.7176(0.9333) | 0.4891(0.9748) | 0.4847(0.9727) | |||
30 | 1 | ℜ(0.1) | 0.00194(0.9871) | 0.0012(0.9500) | 0.2326(1.0000) | 0.00085(0.9824) | 0.0008(0.9810) | |
ℜ(0.5) | 0.1647(0.9836) | 0.1143(0.9503) | 0.2523(1.0000) | 0.0823(0.9809) | 0.0816(0.9783) | |||
ℏ(0.1) | 0.0058(0.9871) | 0.0375(0.9500) | 0.0435(0.9255) | 0.0254(0.9825) | 0.0251(0.9806) | |||
ℏ(0.5) | 0.9127(0.9751) | 0.7085(0.9504) | 0.8013(0.9346) | 0.5247(0.9793) | 0.5195(0.9764) | |||
2 | ℜ(0.1) | 0.00188(0.9874) | 0.0012(0.9488) | 0.2342(1.0000) | 0.00084(0.9823) | 0.00083(0.9786) | ||
ℜ(0.5) | 0.1598(0.9838) | 0.1128(0.9490) | 0.2543(0.9997) | 0.0818(0.9799) | 0.0811(0.9779) | |||
ℏ(0.1) | 0.0056(0.9874) | 0.0369(0.9488) | 0.0419(0.9219) | 0.0253(0.9822) | 0.0250(0.9786) | |||
ℏ(0.5) | 0.8855(0.9737) | 0.6994(0.9490) | 0.7768(0.9334) | 0.5213(0.9789) | 0.5161(0.9764) | |||
3 | ℜ(0.1) | 0.00176(0.9851) | 0.0113(0.9472) | 0.2129(1.0000) | 0.00079(0.9820) | 0.00078(0.9806) | ||
ℜ(0.5) | 0.1506(0.9824) | 0.1044(0.9473) | 0.2312(0.9999) | 0.0771(0.9800) | 0.0765(0.9783) | |||
ℏ(0.1) | 0.0528(0.9851) | 0.0340(0.9472) | 0.0380(0.9230) | 0.0239(0.9820) | 0.0236(0.9806) | |||
ℏ(0.5) | 0.8350(0.9735) | 0.6468(0.9472) | 0.7132(0.9331) | 0.4903(0.9785) | 0.4857(0.9758) |
τ=1.2 | ACI | Boot-p | Gamma prior | ||||||||||||||
Scheme | Boot-p | Boot-t | BCI | HPD | |||||||||||||
(m,k) | α1 | α2 | β | α1 | α2 | β | α1 | α2 | β | α1 | α2 | β | α1 | α2 | β | ||
(40, 25) | 1 | AW | 2.686 | 4.883 | 8.725 | 0.684 | 0.709 | 1.861 | 1.050 | 1.032 | 2.323 | 0.627 | 0.722 | 0.812 | 0.620 | 0.718 | 0.799 |
CP | 0.9962 | 0.9998 | 1.0000 | 0.9374 | 0.9388 | 0.9452 | 0.8704 | 0.8798 | 0.9502 | 0.9968 | 0.9995 | 1.0000 | 0.9954 | 0.9995 | 1.0000 | ||
2 | AW | 2.835 | 5.179 | 8.895 | 0.680 | 0.704 | 1.785 | 1.088 | 1.072 | 2.316 | 0.627 | 0.722 | 0.808 | 0.619 | 0.717 | 0.795 | |
CP | 0.9968 | 1.0000 | 1.0000 | 0.9418 | 0.9438 | 0.9444 | 0.8802 | 0.8896 | 0.9488 | 0.9958 | 0.9998 | 1.0000 | 0.9938 | 0.9995 | 1.0000 | ||
3 | AW | 5.360 | 10.057 | 16.591 | 0.658 | 0.663 | 1.618 | 1.111 | 1.026 | 2.192 | 0.622 | 0.721 | 0.800 | 0.614 | 0.716 | 0.783 | |
CP | 0.9998 | 1.0000 | 1.0000 | 0.9416 | 0.9412 | 0.9422 | 0.8930 | 0.9104 | 0.9506 | 0.9951 | 0.9998 | 1.0000 | 0.9944 | 0.9998 | 1.0000 | ||
(40, 30) | 1 | AW | 2.657 | 4.871 | 8.665 | 0.684 | 0.708 | 1.860 | 1.046 | 1.026 | 2.307 | 0.627 | 0.722 | 0.812 | 0.619 | 0.717 | 0.799 |
CP | 0.9964 | 1.0000 | 1.0000 | 0.9330 | 0.9402 | 0.9438 | 0.8742 | 0.8860 | 0.9492 | 0.99665 | 0.9998 | 1.0000 | 0.9956 | 0.9995 | 1.0000 | ||
2 | AW | 2.827 | 5.199 | 8.986 | 0.679 | 0.703 | 1.796 | 1.093 | 1.077 | 2.345 | 0.626 | 0.723 | 0.808 | 0.618 | 0.718 | 0.795 | |
CP | 0.9976 | 1.0000 | 1.0000 | 0.9346 | 0.9406 | 0.9450 | 0.8732 | 0.8924 | 0.9588 | 0.9948 | 0.9999 | 1.0000 | 0.9947 | 0.9998 | 1.0000 | ||
3 | AW | 5.408 | 10.001 | 16.559 | 0.659 | 0.664 | 1.621 | 1.113 | 1.031 | 2.190 | 0.622 | 0.721 | 0.799 | 0.614 | 0.716 | 0.783 | |
CP | 0.9994 | 1.0000 | 1.0000 | 0.9388 | 0.9426 | 0.9400 | 0.8946 | 0.9132 | 0.9458 | 0.9956 | 0.9999 | 1.0000 | 0.9935 | 0.9999 | 1.0000 | ||
(50, 25) | 1 | AW | 2.182 | 3.971 | 8.703 | 0.616 | 0.639 | 1.642 | 0.933 | 0.932 | 2.101 | 0.596 | 0.700 | 0.804 | 0.589 | 0.695 | 0.791 |
CP | 0.9958 | 1.0000 | 1.000 | 0.9416 | 0.9374 | 0.9528 | 0.8904 | 0.8946 | 0.9564 | 0.9947 | 0.9994 | 1.0000 | 0.9929 | 0.9989 | 1.0000 | ||
2 | AW | 2.209 | 4.016 | 8.640 | 0.615 | 0.638 | 1.627 | 0.870 | 0.866 | 1.934 | 0.595 | 0.698 | 0.802 | 0.588 | 0.694 | 0.790 | |
CP | 0.9952 | 1.0000 | 1.0000 | 0.9404 | 0.9410 | 0.9488 | 0.8830 | 0.8932 | 0.9542 | 0.9953 | 0.9998 | 1.0000 | 0.9931 | 0.9996 | 1.0000 | ||
3 | AW | 2.809 | 5.189 | 10.893 | 0.595 | 0.608 | 1.512 | 0.922 | 0.900 | 1.983 | 0.589 | 0.695 | 0.796 | 0.582 | 0.691 | 0.783 | |
CP | 0.9976 | 1.0000 | 1.0000 | 0.9490 | 0.9468 | 0.9518 | 0.9042 | 0.9094 | 0.9538 | 0.9941 | 0.9996 | 1.0000 | 0.9919 | 0.9995 | 1.0000 | ||
(50, 30) | 1 | AW | 2.195 | 4.008 | 7.847 | 0.616 | 0.639 | 1.640 | 0.909 | 0.905 | 2.041 | 0.594 | 0.699 | 0.804 | 0.588 | 0.694 | 0.791 |
CP | 0.9957 | 1.000 | 1.000 | 0.938 | 0.939 | 0.956 | 0.884 | 0.888 | 0.952 | 0.9942 | 0.9994 | 1.0000 | 0.9923 | 0.9991 | 1.0000 | ||
2 | AW | 2.196 | 3.957 | 8.589 | 0.616 | 0.638 | 1.633 | 0.894 | 0.891 | 2.009 | 0.596 | 0.699 | 0.803 | 0.589 | 0.694 | 0.790 | |
CP | 0.9957 | 1.0000 | 1.0000 | 0.9478 | 0.9458 | 0.9440 | 0.8900 | 0.8928 | 0.9486 | 0.9952 | 1.0000 | 1.0000 | 0.9935 | 1.0000 | 1.0000 | ||
3 | AW | 2.812 | 5.191 | 10.943 | 0.595 | 0.607 | 1.527 | 0.913 | 0.891 | 1.992 | 0.589 | 0.695 | 0.797 | 0.582 | 0.691 | 0.783 | |
CP | 0.9979 | 1.0000 | 1.0000 | 0.9420 | 0.9446 | 0.9440 | 0.8946 | 0.9096 | 0.9546 | 0.9939 | 0.9996 | 1.0000 | 0.9909 | 0.9996 | 1.0000 |
n | m | k | Scheme | ACI | Boot-p | Gamma prior | |||
Boot-p | Boot-t | BCI | HPD | ||||||
60 | 40 | 25 | 1 | ℜ(0.1) | 0.296(0.9735) | 0.216(0.9516) | 0.268(0.9806) | 0.131(0.9956) | 0.131(0.9945) |
ℜ(0.5) | 0.215(0.9642) | 0.198(0.9506) | 0.284(0.9958) | 0.143(0.9953) | 0.142(0.9950) | ||||
ℏ(0.1) | 3.049(0.9569) | 2.807(0.9518) | 2.923(0.9356) | 1.777(0.9954) | 1.765(0.9936) | ||||
ℏ(0.5) | 3.083(0.9956) | 0.994(0.9498) | 1.304(0.8706) | 0.957(0.9959) | 0.952(0.9949) | ||||
2 | ℜ(0.1) | 0.259(0.9682) | 0.208(0.9498) | 0.276(0.9840) | 0.130(0.9929) | 0.130(0.9916) | |||
ℜ(0.5) | 0.223(0.9737) | 0.190(0.9498) | 0.267(0.9920) | 0.142(0.9925) | 0.141(0.9921) | ||||
ℏ(0.1) | 2.758(0.9559) | 2.702(0.9494) | 2.723(0.9384) | 1.761(0.9925) | 1.750(0.9912) | ||||
ℏ(0.5) | 3.141(0.9962) | 0.959(0.9484) | 1.325(0.8786) | 0.952(0.9943) | 0.947(0.9922) | ||||
3 | ℜ(0.1) | 0.252(0.9771) | 0.188(0.9456) | 0.251(0.9838) | 0.127(0.9941) | 0.126(0.9932) | |||
ℜ(0.5) | 0.356(0.9941) | 0.169(0.947) | 0.177(0.9446) | 0.138(0.9942) | 0.137(0.9934) | ||||
ℏ(0.1) | 2.618(0.9717) | 2.436(0.9454) | 2.267(0.9562) | 1.716(0.9941) | 1.706(0.9925) | ||||
ℏ(0.5) | 6.124(0.9997) | 0.840(0.9470) | 1.330(0.8970) | 0.936(0.9955) | 0.931(0.9943) | ||||
30 | 1 | ℜ(0.1) | 0.286(0.9755) | 0.216(0.9460) | 0.267(0.9798) | 0.131(0.9951) | 0.131(0.9945) | ||
ℜ(0.5) | 0.212(0.9683) | 0.198(0.9468) | 0.283(0.9932) | 0.143(0.9947) | 0.142(0.9946) | ||||
ℏ(0.1) | 3.008(0.9610) | 2.806(0.9456) | 2.913(0.9374) | 1.775(0.9947) | 1.763(0.9940) | ||||
ℏ(0.5) | 2.913(0.9956) | 0.994(0.9480) | 1.295(0.8764) | 0.956(0.9956) | 0.951(0.9942) | ||||
2 | ℜ(0.1) | 0.260(0.9686) | 0.208(0.9464) | 0.275(0.9864) | 0.130(0.9961) | 0.130(0.9953) | |||
ℜ(0.5) | 0.224(0.9770) | 0.190(0.9470) | 0.266(0.9936) | 0.142(0.9959) | 0.141(0.9955) | ||||
ℏ(0.1) | 2.764(0.9578) | 2.713(0.9462) | 2.732(0.9470) | 1.762(0.9961) | 1.751(0.9944) | ||||
ℏ(0.5) | 3.176(0.9975) | 0.959(0.9480) | 1.334(0.8810) | 0.953(0.9966) | 0.947(0.9955) | ||||
3 | ℜ(0.1) | 0.252(0.9734) | 0.188(0.9442) | 0.252(0.9834) | 0.127(0.9928) | 0.126(0.9912) | |||
ℜ(0.5) | 0.358(0.9935) | 0.169(0.9478) | 0.177(0.9432) | 0.138(0.9928) | 0.137(0.9918) | ||||
ℏ(0.1) | 2.629(0.9714) | 2.440(0.9462) | 2.263(0.9564) | 1.717(0.9929) | 1.706(0.9913) | ||||
ℏ(0.5) | 6.180(0.9999) | 0.841(0.9500) | 1.332(0.8970) | 0.936(0.9946) | 0.931(0.9929) | ||||
50 | 25 | 1 | ℜ(0.1) | 0.258(0.9718) | 0.194(0.9558) | 0.238(0.9852) | 0.124(0.9920) | 0.124(0.9905) | |
ℜ(0.5) | 0.189(0.9611) | 0.178(0.9568) | 0.257(0.9962) | 0.135(0.9909) | 0.134(0.9897) | ||||
ℏ(0.1) | 2.705(0.9553) | 2.515(0.9548) | 2.661(0.9480) | 1.679(0.9915) | 1.669(0.9903) | ||||
ℏ(0.5) | 2.590(0.9947) | 0.899(0.9580) | 1.181(0.8898) | 0.906(0.9924) | 0.9015(0.9915) | ||||
2 | ℜ(0.1) | 0.253(0.9745) | 0.192(0.9532) | 0.239(0.9826) | 0.124(0.9930) | 0.123(0.992) | |||
ℜ(0.5) | 0.191(0.9660) | 0.177(0.9522) | 0.254(0.9962) | 0.135(0.9923) | 0.134(0.9915) | ||||
ℏ(0.1) | 2.661(0.9574) | 2.494(0.9526) | 2.556(0.9434) | 1.671(0.9923) | 1.661(0.9914) | ||||
ℏ(0.5) | 2.613(0.9965) | 0.892(0.9520) | 1.102(0.887) | 0.903(0.9935) | 0.898(0.9925) | ||||
3 | ℜ(0.1) | 0.246(0.9780) | 0.179(0.9532) | 0.222(0.9814) | 0.121(0.9897) | 0.120(0.9891) | |||
ℜ(0.5) | 0.212(0.9803) | 0.163(0.9532) | 0.213(0.9910) | 0.131(0.9896) | 0.130(0.9881) | ||||
ℏ(0.1) | 2.417(0.9634) | 2.315(0.9532) | 2.283(0.9572) | 1.634(0.9896) | 1.624(0.9892) | ||||
ℏ(0.5) | 3.486(0.9974) | 0.817(0.9520) | 1.206(0.8836) | 0.888(0.9918) | 0.884(0.9909) | ||||
30 | 1 | ℜ(0.1) | 0.259(0.9744) | 0.194(0.9530) | 0.238(0.9836) | 0.124(0.9925) | 0.123(0.9913) | ||
ℜ(0.5) | 0.190(0.9643) | 0.178(0.9532) | 0.257(0.9964) | 0.135(0.9917) | 0.134(0.9922) | ||||
ℏ(0.1) | 2.704(0.9590) | 2.512(0.9526) | 2.637(0.9448) | 1.674(0.9918) | 1.664(0.9898) | ||||
ℏ(0.5) | 2.593(0.9965) | 0.899(0.9572) | 1.154(0.8874) | 0.904(0.9930) | 0.899(0.9924) | ||||
2 | ℜ(0.1) | 0.252(0.9729) | 0.193(0.9462) | 0.240(0.9790) | 0.124(0.9929) | 0.123(0.9918) | |||
ℜ(0.5) | 0.190(0.9651) | .176(0.9472) | 0.254(0.9948) | 0.134(0.9919) | 0.134(0.9922) | ||||
ℏ(0.1) | 2.655(0.9590) | 2.501(0.9464) | 2.587(0.9402) | 1.674(0.9919) | 1.664(0.9908) | ||||
ℏ(0.5) | 2.600(0.9969) | 0.893(0.9472) | 1.134(0.8878) | 0.904(0.9931) | 0.900(0.9922) | ||||
3 | ℜ(0.1) | 0.246(0.9771) | 0.180(0.9474) | 0.222(0.9790) | 0.121(0.9924) | 0.120(0.9917) | |||
ℜ(0.5) | 0.213(0.9840) | 0.162(0.9478) | 0.213(0.9890) | 0.131(0.9915) | 0.130(0.9909) | ||||
ℏ(0.1) | 2.422(0.9651) | 2.328(0.9478) | 2.292(0.9580) | 1.633(0.9918) | 1.624(0.9909) | ||||
ℏ(0.5) | 3.503(0.9985) | 0.817(0.9494) | 1.198(0.8872) | 0.888(0.9933) | 0.883(0.9922) |
Cases | ML | Bayesian(uniform prior) | |||||
BS | BL | BL | BG | BG | |||
(h=1.5) | (h=−1.5) | (c=0.5) | (c=−0.5) | ||||
1 | ˆα1 | 0.1795(0.1053) | 0.2292(0.1774) | 0.2106(0.1784) | 0.2632(0.1806) | 0.1718(0.1864) | 0.2064(0.1788) |
ˆα2 | 0.2692(0.1500) | 0.3459(0.2598) | 0.3099(0.2623) | 0.4364(0.2751) | 0.2656(0.2719) | 0.3138(0.2617) | |
ˆβ | 0.4985(0.4210) | 0.6279(0.5469) | 0.4872(0.5647) | 1.2542(0.8314) | 0.3796(0.6006) | 0.5372(0.5543) | |
ˆℜ(0.5) | 0.9734(0.0102) | 0.9721(0.0106) | 0.9720(0.0106) | 0.9722(0.0106) | 0.9720(0.0106) | 0.9721(0.0106) | |
ˆℜ(1.5) | 0.6436(0.0583) | 0.6493(0.0566) | 0.6469(0.0567) | 0.6517(0.0567) | 0.6455(0.0568) | 0.6481(0.0567) | |
ˆℏ(0.5) | 0.1568(0.0575) | 0.1627(0.0576) | 0.1603(0.0577) | 0.1653(0.0577) | 0.1491(0.0592) | 0.1581(0.0578) | |
ˆℏ(1.5) | 0.5613(0.1492) | 0.5601(0.4213) | 0.5458(0.1407) | 0.5753(0.1409) | 0.5339(0.1425) | 0.5514(0.1403) | |
ˆπ1 | 0.4(0.0980) | 0.3990(0.0961) | 0.3921(0.0964) | 0.4060(0.0964) | 0.3806(0.0979) | 0.3931(0.0963) | |
ˆπ2 | 0.6(0.0980) | 0.6010(0.0961) | 0.5940(0.0964) | 0.6079(0.0964) | 0.5887(0.0970) | 0.5970(0.0962) | |
2 | ˆα1 | 0.1383(0.0553) | 0.1445(0.0624) | 0.1417(0.0625) | 0.1476(0.0625) | 0.1274(0.0647) | 0.1385(0.0627) |
ˆα2 | 0.2075(0.0754) | 0.2172(0.0856) | 0.2120(0.0857) | 0.2231(0.0858) | 0.1960(0.0881) | 0.2098(0.0859) | |
ˆβ | 0.7013(0.4397) | 0.8856(0.6072) | 0.7113(0.6317) | 1.6297(0.9603) | 0.6585(0.6482) | 0.8034(0.6127) | |
ˆℜ(0.5) | 0.9714(0.0105) | 0.9690(0.0117) | 0.9689(0.0117) | 0.9691(0.0117) | 0.9689(0.0117) | 0.9690(0.0117) | |
ˆℜ(1.5) | 0.6571(0.0558) | 0.6602(0.0548) | .6580(0.0548) | 0.6625(0.0548) | 0.6567(0.0549) | 0.6591(0.0548) | |
ˆℏ(0.5) | 0.1673(0.0587) | 0.1783(0.0625) | 0.1755(0.0626) | 0.1813(0.0626) | 0.1632(0.0643) | 0.1732(0.0627) | |
ˆℏ(1.5) | 0.4863(0.0990) | 0.4757(0.3124) | 0.4690(0.0959) | 0.4827(0.0959) | 0.4612(0.0967) | 0.4709(0.0958) | |
ˆπ1 | 0.4(0.0894) | 0.3993(0.0879) | 0.3935(0.0881) | 0.4051(0.0881) | 0.3841(0.0892) | 0.3943(0.0881) | |
ˆπ2 | 0.6(0.0894) | 0.6007(0.0879) | 0.5949(0.0881) | 0.6065(0.0881) | 0.5905(0.0885) | 0.5974(0.0880) | |
3 | ˆα1 | 0.2099(0.0795) | 0.2217(0.0914) | 0.2158(0.0916) | 0.2285(0.0917) | 0.1982(0.0944) | 0.2134(0.0918) |
ˆα2 | 0.3498(0.1196) | 0.3699(0.1404) | 0.3565(0.1411) | 0.3866(0.1414) | 0.3366(0.1443) | 0.3582(0.1409) | |
ˆβ | 0.3291(0.2020) | 0.4031(0.2896) | 0.3579(0.2931) | 0.5407(0.3206) | 0.3001(0.3073) | 0.3654(0.2920) | |
ˆℜ(0.5) | 0.9777(0.0079) | 0.9762(0.0091) | 0.9761(0.0091) | 0.9762(0.0091) | 0.9761(0.0091) | =0.9761(0.0091) | |
ˆℜ(1.5) | 0.658262(0.0609) | 0.6616(0.0591) | 0.6589(0.0591) | 0.6641(0.0591) | 0.6575(0.0592) | 0.6602(0.0591) | |
ˆℏ(0.5) | 0.1327(0.0464) | 0.1408(0.0513) | 0.1389(0.0514) | 0.1429(0.05138) | 0.1285(0.0528) | 0.1366(0.05151) | |
ˆℏ(1.5) | 0.5891(0.0935) | 0.5715(0.4406) | 0.5651(0.0933) | 0.5781(0.0934) | 0.5601(0.0938) | 0.5677(0.0932) | |
ˆπ1 | 0.375(0.0765) | 0.3751(0.0751) | 0.3709(0.0752) | 0.3794(0.0752) | 0.3634(0.0760) | 0.3713(0.0752) | |
ˆπ2 | 0.625(0.0765) | 0.6249(0.0751) | 0.6206(0.0752) | 0.6291(0.0752) | 0.6178(0.0754) | 0.6226(0.0751) |
Cases | ACI | Boot-p | Uniform prior | |||
Boot-p | Boot-t | BCI | HPD | |||
α1 | 1 | (0.1103, 0.2920) | (0.1876, 0.3355) | (0.1880, 0.2771) | (0.0641, 0.6904) | (0.0419, 0.5590) |
0.1817 | 0.1479 | 0.0891 | 0.6263 | 0.5171 | ||
2 | (0.0300, 0.2467) | (0.1396, 0.2392) | (0.1396, 0.2045) | (0.0595, 0.2999) | (0.0446, 0.2651) | |
0.2167 | 0.0996 | 0.0649 | 0.2405 | 0.2205 | ||
3 | (0.0540, 0.3658) | (0.2108, 0.3104) | (0.2108, 0.2815) | (0.0978, 0.4485) | (0.0794, 0.3995) | |
0.3112 | 0.0996 | 0.0707 | 0.3507 | 0.3201 | ||
α2 | 1 | (0.1528, 0.4744) | (0.2745, 0.3190) | (0.2749, 0.3165) | (0.1071, 1.0452) | (0.0689, 0.8282) |
0.3216 | 0.0445 | 0.0416 | 0.9381 | 0.7593 | ||
2 | (0.0598, 0.3552) | (0.2080, 0.3312) | (0.2080, 0.2932) | (0.0999, 0.4382) | (0.0798, 0.3859) | |
0.2955 | 0.1232 | 0.0852 | 0.3383 | 0.3061 | ||
3 | (0.1154, 0.5843) | (0.3508, 0.4483) | (0.3509, 0.4240) | (0.1181, 0.7192) | (0.1545, 0.6508) | |
0.4689 | 0.0975 | 0.0731 | 0.5383 | 0.4963 | ||
β | 1 | (0.1531, 1.5992) | (0.5314, 0.6447) | (0.5340, 0.6197) | (0.0794, 2.1151) | (0.0382, 1.6710) |
1.4460 | 0.1133 | 0.0857 | 2.0357 | 1.6327 | ||
2 | (0.2506, 1.9626) | (0.7107, 1.1594) | (0.7111, 1.0276) | (0.1973, 2.4641) | (0.1180, 2.0447) | |
1.7120 | 0.4487 | 0.3165 | 2.2668 | 1.9267 | ||
3 | (0.1651, 0.6563) | (0.3317, 0.5494) | (0.3317, 0.4834) | (0.0912, 1.0850) | (0.0535, 0.8972) | |
0.7918 | 0.2177 | 0.1517 | 0.9937 | 0.8437 | ||
ℜ(0.5) | 1 | (0.9535, 0.9933) | (0.9564, 0.9675) | (0.9560, 0.9648) | (0.9458, 0.9866) | (0.9515, 0.9892) |
0.0398 | 0.0111 | 0.0088 | 0.0408 | 0.0377 | ||
2 | (0.9507, 0.9920) | (0.9457, 0.9686) | (0.9438, 0.9683) | (0.9406, 0.9857) | (0.9454, 0.9879) | |
0.4125 | 0.0229 | 0.0245 | 0.0451 | 0.0425 | ||
3 | (0.9621, 0.9933) | (0.9601, 0.9766) | (0.9542, 0.9765) | (0.9550, 0.9888) | (0.9588, 0.9903) | |
0.0312 | 0.0165 | 0.0223 | 0.0339 | 0.0316 | ||
ℜ(1.5) | 1 | (0.5293, 0.7580) | (0.4986, 0.5916) | (0.4986, 0.5899) | (0.5355, 0.7552) | (0.5384, 0.7575) |
0.2287 | 0.0930 | 0.0913 | 0.2197 | 0.2191 | ||
2 | (0.5478, 0.7664) | (0.4970, 0.6376) | (0.4959, 0.6393) | (0.5481, 0.7634) | (0.5538, 0.7676) | |
0.2186 | 0.1406 | 0.1434 | 0.2154 | 0.2138 | ||
3 | (0.5388, 0.7777) | (0.5183, 0.6458) | (0.4863, 0.6464) | (0.54438, 0.7733) | (0.5465, 0.7752) | |
0.2388 | 0.1275 | 0.1601 | 0.2290 | 0.2287 | ||
ℏ(0.5) | 1 | (0.0441, 0.2696) | (0.1917, 0.2586) | (0.1872, 0.2171) | (0.8009, 0.3026) | (0.0655, 0.2749) |
0.2255 | 0.0669 | 0.0299 | 0.2225 | 0.2095 | ||
2 | (0.0521, 0.2824) | (0.1829, 0.3173) | (0.1826, 0.3055) | (0.0850, 0.3272) | (0.0727, 0.3033) | |
0.2303 | 0.1344 | 0.1229 | 0.2422 | 0.2306 | ||
3 | (0.0417, 0.2237) | (0.1392, 0.2381) | (0.1389, 0.2498) | (0.0669, 0.2616) | (0.0580, 0.2401) | |
0.1820 | 0.0989 | 0.1109 | 0.1947 | 0.1821 | ||
ℏ(1.5) | 1 | (0.2689, 0.6740) | (0.6344, 0.8545) | (0.6088, 1.0570) | (0.3206, 0.8656) | (0.0762, 0.2904) |
0.4051 | 0.2201 | 0.4482 | 0.5450 | 0.2142 | ||
2 | (0.2922, 0.6014) | (0.5138, 0.7627) | (0.5268, 0.7798) | (0.3047, 0.6761) | (0.0806, 0.3160) | |
0.3092 | 0.2489 | 0.2530 | 0.3714 | 0.2354 | ||
3 | (0.4059, 0.6801) | (0.6104, 0.8599) | (0.6074, 0.7769) | (0.4019, 0.7659) | (0.0658, 0.2558) | |
0.2742 | 0.2495 | 0.1695 | 0.3640 | 0.1900 | ||
π1 | 1 | (0.2080, 0.5920) | (0.3704, 0.5161) | (0.3670, 0.5423) | (0.2205, 0.5942) | (0.2154, 0.5879) |
0.3841 | 0.1457 | 0.1753 | 0.2908 | 0.3725 | ||
2 | (0.2247, 0.5753) | (0.3143, 0.5000) | (0.2898, 0.5398) | (0.2361, 0.5777) | (0.2273, 0.5677) | |
0.3506 | 0.1857 | 0.2500 | 0.3416 | 0.3404 | ||
3 | (0.2250, 0.5250) | (0.3500, 0.4500) | (0.3450, 0.4626) | (0.2353, 0.5261) | (0.2317, 0.5202) | |
0.3001 | 0.1000 | 0.1176 | 0.3736 | 0.2884 | ||
π2 | 1 | (0.4080, 0.7920) | (0.4839, 0.6296) | (0.4577, 0.6330) | (0.4058, 0.8794) | (0.4121, 0.7846) |
0.3841 | 0.1457 | 0.1753 | 0.2908 | 0.3725 | ||
2 | (0.4247, 0.7754) | (0.5000, 0.6857) | (0.4602, 0.7102) | (0.4219, 0.7639) | (0.4323, 0.7727) | |
0.3506 | 0.1857 | 0.2500 | 0.3420 | 0.3404 | ||
3 | (0.4750, 0.7750) | (0.55, 0.65) | (0.5374, 0.6550) | (0.4739, 0.7647) | (0.4798, 0.7683) | |
0.3001 | 0.1000 | 0.1176 | 0.3736 | 0.2884 |
Cases | ACI | Boot-p | Uniform prior | |||
Boot-p | Boot-t | BCI | HPD | |||
α1 | 1∗ | (0.0456, 0.0820) | (0.0619, 0.1506) | (0.0619, 0.1058) | (0.0163, 0.3172) | (0.0069, 0.2179) |
0.0364 | 0.0887 | 0.0439 | 0.3009 | 0.2110 | ||
2∗ | (0.0025, 0.1348) | (0.0697, 0.1277) | (0.0697, 0.1053) | (0.0245, 0.1804) | (0.0167, 0.1520) | |
0.1323 | 0.058 | 0.0356 | 0.1560 | 0.1353 | ||
3∗ | (0.1101, 0.6418) | (0.2677, 0.5379) | (0.2681, 0.4074) | (0.0755, 2.1628) | (0.0336, 1.6337) | |
0.5316 | 0.2702 | 0.1393 | 2.0873 | 1.6001 | ||
α2 | 1∗ | (0.0659, 0.1276) | (0.0923, 0.1731) | (0.0925, 0.1458) | (0.0292, 0.4916) | (0.0155, 0.3231) |
0.0617 | 0.0808 | 0.0533 | 0.4624 | 0.3077 | ||
2∗ | (0.0050, 0.1495) | (0.0775, 0.1713) | (0.0775, 0.1255) | (0.0285, 0.2011) | (0.0184, 0.1684) | |
0.1445 | 0.0956 | 0.048 | 0.1726 | 0.1500 | ||
3∗ | (0.1268, 0.9028) | (0.3407, 0.6385) | (0.3412, 0.4848) | (0.1029, 2.7150) | (0.0489, 2.0678) | |
0.776 | 0.2978 | 0.1436 | 2.6120 | 2.0189 | ||
β | 1∗ | (0.1191, 20.52) | (1.5804, 4.5806) | (1.5783, 2.9936) | (0.1183, 18.94) | (0.0257, 12.82) |
20.401 | 3.0002 | 1.4153 | 18.8205 | 12.80 | ||
2∗ | (0.1602, 17.6309) | (1.7368, 4.4798) | (1.7507, 2.8638) | (0.2671, 10.846) | (0.0912, 8.287) | |
17.4707 | 2.743 | 1.1131 | 10.579 | 8.1962 | ||
3∗ | (0.0488, 0.3839) | (0.1373, 0.3387) | (0.1376, 0.2714) | (0.0087, 1.0988) | (0.0051, 0.8157) | |
0.3351 | 0.2014 | 0.1338 | 1.0900 | 0.8105 | ||
ℜ(0.5) | 1∗ | (0.9431, 1.003) | (0.9276, 0.9647) | (0.9116, 0.9650) | (0.9159, 0.9914) | (0.9273, 0.9943) |
0.0599 | 0.0371 | 0.0534 | 0.0755 | 0.0670 | ||
2∗ | (0.9431, 1.0021) | (0.9231, 0.9673) | (0.9171, 0.9671) | (0.9264, 0.9904) | (0.9341, 0.9932) | |
0.059 | 0.0442 | 0.05 | 0.0640 | 0.0591 | ||
3∗ | (0.9774, 1.0022) | (0.9708, 0.9887) | (0.9519, 0.9883) | (0.9690, 09957) | (0.9739, 0.9967) | |
0.0249 | 0.0179 | 0.0364 | 0.0267 | 0.0228 | ||
ℜ(1.5) | 1∗ | (0.6470, 0.8635) | (0.5546, 0.7127) | (0.3798, 0.6750) | (0.6459, 0.8577) | (0.6562, 0.8638) |
0.2165 | 0.1581 | 0.2952 | 0.2118 | 0.2076 | ||
2∗ | (0.6540, 0.8623) | (0.5590, 0.7299) | (0.5688, 0.7302) | (0.6537, 0.8580) | (0.6631, 0.8660) | |
0.2083 | 0.1709 | 0.1614 | 0.2043 | 0.2028 | ||
3∗ | (0.6460, 0.9440) | (0.5743, 0.7764) | (0.2904, 0.7625) | (0.6607, 0.8934) | (0.6749, 0.9015) | |
0.2980 | 0.1065 | 0.2633 | 0.2327 | 0.2266 | ||
ℏ(0.5) | 1∗ | (0.0018, 0.2980) | (0.1973, 0.3822) | (0.1859, 0.3759) | (0.0508, 0.3388) | (0.0394, 0.3133) |
0.2962 | 0.1849 | 0.19 | 0.2880 | 0.2739 | ||
2∗ | (0.0068, 0.2972) | (0.1798, 0.3877) | (0.1791, 0.3872) | (0.0563, 0.3306) | (0.0447, 0.3066) | |
0.2904 | 0.2079 | 0.2081 | 0.2742 | 0.2618 | ||
3∗ | (0.0124, 0.1343) | (0.0675, 0.1739) | (0.2904, 0.7625) | (0.0258, 0.1785) | (0.0196, 0.1515) | |
0.1219 | 0.1064 | 0.4721 | 0.1527 | 0.1318 | ||
ℏ(1.5) | 1∗ | (0.0657, 0.4051) | (0.2891, 0.5050) | (0.2828, 0.5181) | (0.1072, 0.4887) | (0.0414, 0.3160) |
0.3394 | 0.2159 | 0.2353 | 0.3815 | 0.2746 | ||
2∗ | (0.0942, 0.3932) | (0.2733, 0.4883) | (0.2721, 0.5078) | (0.1216, 0.4052) | (0.0515, 0.3160) | |
0.299 | 0.215 | 0.2357 | 0.2836 | 0.2646 | ||
3∗ | (0.1924, 0.4551) | (0.0675, 0.1740) | (0.4148, 0.6781) | (0.2144.0.5378) | (0.0249, 0.1690) | |
0.2628 | 0.1065 | 0.2633 | 0.3234 | 0.1441 | ||
π1 | 1∗ | (0.1521, 0.6479) | (0.3333, 0.6) | (0.2953, 0.6505) | (0.1760, 0.6498) | (0.1721, 0.6445) |
0.4958 | 0.2667 | 0.3552 | 0.4738 | 0.4724 | ||
2∗ | (0.2333, 0.7079) | (0.3333, 0.6) | (0.2581, 0.6371) | (0.2463, 0.7006) | (0.2381, 0.6915) | |
0.4746 | 0.2667 | 0.379 | 0.4543 | 0.4533 | ||
3∗ | (0.2454, 0.6346) | (0.36, 0.56) | (0.3450, 0.5533) | (0.2555, 0.6323) | (0.2552, 0.6317) | |
0.3892 | 0.2 | 0.2083 | 0.3768 | 0.3765 | ||
π2 | 1∗ | (0.3521, 0.8479) | (0.4, 0.6667) | (0.3495, 0.7047) | (0.3501, 0.8239) | (0.3555, 0.8279) |
0.4958 | 0.2667 | 0.3552 | 0.4737 | 0.4724 | ||
2∗ | (0.2921, 0.7667) | (0.4, 0.6667) | (0.3629, 0.7419) | (0.2994, 0.7536) | (0.3086, 0.7618) | |
0.4746 | 0.2667 | 0.379 | 0.4542 | 0.4533 | ||
3∗ | (0.3654, 0.7545) | (0.44, 0.64) | (0.4467, 0.6550) | (0.3677, 0.7445) | (0.3683, 0.7448) | |
0.3892 | 0.2 | 0.2083 | 0.3768 | 0.3765 |
Cases | ML | Bayesian (uniform prior) | |||||
BS | BL | BL | BG | BG | |||
(h=1.5) | (h=−1.5) | (c=0.5) | (c=−0.5) | ||||
1∗ | ˆα1 | 0.0611(0.0370) | 0.0836(0.1177) | 0.07612(0.1180) | 0.1010(0.1190) | 0.0520(0.1220) | 0.0687(0.1187) |
ˆα2 | 0.0917(0.0511) | 0.1261(0.1702) | 0.1121(0.1708) | 0.1744(0.1769) | 0.0820(0.1758) | 0.1051(0.1715) | |
ˆβ | 1.5631(1.6424) | 3.4600(5.2310) | 1.1057(5.7364) | 49.410(46.25) | 1.0826(5.7459) | 2.4572(5.3263) | |
ˆℜ(0.5) | 0.9731(0.0153) | 0.9673(0.0198) | 0.9670(0.0198) | 0.9676(0.0199) | 0.9670(0.0198) | 0.9672(0.0198) | |
ˆℜ(1.5) | 0.7552(0.0552) | 0.7610(0.0541) | 0.7588(0.0542) | 0.7631(0.0542) | 0.7580(0.0542) | 0.7600(0.0542) | |
ˆℏ(0.5) | 0.1499(0.0756) | 0.1639(0.0775) | 0.1596(0.0777) | 0.1686(0.0777) | 0.1368(0.0822) | 0.1550(0.0781) | |
ˆℏ(1.5) | 0.2570(0.0976) | 0.2547(0.1338) | 0.2478(0.0985) | 0.2623(0.0986) | 0.2279(0.1019) | 0.2456(0.0987) | |
ˆπ1 | 0.4(0.1265) | 0.399(0.1226) | 0.3879(0.1231) | 0.4104(0.1232) | 0.3675(0.1266) | 0.3891(0.1230) | |
ˆπ2 | 0.6(0.1265) | 0.601(0.1226) | 0.5896(0.1232) | 0.6121(0.1231) | 0.5800(0.1244) | 0.5944(0.1228) | |
2∗ | ˆα1 | 0.0687(0.0338) | 0.0730(0.0422) | 0.0717(0.0422) | 0.0744(0.0422) | 0.0600(0.0441) | 0.0682(0.0424) |
ˆα2 | 0.0772(0.0369) | 0.0824(0.0465) | 0.0808(0.0465) | 0.0841(0.0465) | 0.0684(0.0485) | 0.0773(0.0467) | |
ˆβ | 1.6807(1.5547) | 2.7852(2.717) | 1.3028(3.0955) | 17.69(15.147) | 1.4983(3.0068) | 2.2997(2.7605) | |
ˆℜ(0.5) | 0.9726(0.0150) | 0.9679(0.0168) | 0.9677(0.0168) | 0.9681(0.0168) | 0.9677(0.0168) | 0.9679(0.0168) | |
ˆℜ(1.5) | 0.7581(0.0531) | 0.7624(0.0524) | 0.7603(0.0524) | 0.7644(0.0524) | 0.7596(0.0524) | 0.7615(0.0524) | |
ˆℏ(0.5) | 0.1520(0.0741) | 0.1656(0.0722) | 0.1618(0.0723) | 0.1697(0.0723) | 0.1422(0.0759) | 0.1579(0.0726) | |
ˆℏ(1.5) | 0.2480(0.0785) | 0.2408(0.1047) | 0.2370(0.0730) | 0.2449(0.0730) | 0.2246(0.0747) | 0.2354(0.0731) | |
ˆπ1 | 0.4706(0.1211) | 0.4699(0.1165) | 0.4598(0.1170) | 0.4802(0.1170) | 0.4463(0.1189) | 0.4624(0.1168) | |
ˆπ2 | 0.5294(0.1211) | 0.5301(0.1165) | 0.5198(0.1170) | 0.5402(0.1170) | 0.5089(0.1184) | 0.5233(0.1167) | |
3∗ | ˆα1 | 0.2659(0.2318) | 0.4510(0.5585) | 0.3281(0.5720) | 1.3421(1.0517) | 0.2458(0.5950) | 0.3570(0.5664) |
ˆα2 | 0.3384(0.2913) | 0.5750(0.7032) | 0.3992(0.7248) | 2.0700(1.6521) | 0.3186(0.7485) | 0.4571(0.7130) | |
ˆβ | 0.1368(0.1947) | 0.2622(0.4002) | 0.2007(0.4049) | 1.0029(0.8419) | 0.0825(0.4388) | 0.1880(0.4071) | |
ˆℜ(0.5) | 0.9898(0.0063) | 0.9877(0.0076) | 0.9876(0.0076) | 0.9877(0.0076) | 0.9876(0.0076) | 0.9877(0.0076) | |
ˆℜ(1.5) | 0.7975(0.0760) | 0.7922(0.0617) | 0.7893(0.0618) | 0.7951(0.0618) | 0.7885(0.0619) | 0.7910(0.0617) | |
ˆℏ(0.5) | 0.0610(0.0374) | 0.0725(0.0424) | 0.0712(0.0424) | 0.0739(0.0424) | 0.0592(0.0444) | 0.0676(0.0427) | |
ˆℏ(1.5) | 0.3818(0.0966) | 0.3613(0.007) | 0.3561(0.0840) | 0.666(0.08401) | 0.3465(0.0851) | 0.3564(0.0840) | |
ˆπ1 | 0.44(0.0993) | 0.4397(0.0975) | 0.4326(0.0977) | 0.4468(0.0977) | 0.4224(0.0990) | 0.4341(0.0976) | |
ˆπ2 | 0.56(0.0993) | 0.5603(0.0975) | 0.5532(0.0977) | 0.5674(0.0977) | 0.5468(0.0984) | 0.5560(0.0976) |
[1] |
J. Boag, Maximum likelihood estimates of the proportion of patients cured by cancer therapy, J. R. Stat. Soc., 11 (1949), 15–44. https://doi.org/10.1111/j.2517-6161.1949.tb00020.x doi: 10.1111/j.2517-6161.1949.tb00020.x
![]() |
[2] | M. Pintilie, Competing risks: a practical perspective, John Wiley & Sons, Inc., 2006. |
[3] |
T. Chen, S. Zheng, H. Luo, X. Liu, J. Feng, Reliability analysis of multiple causes of failure in presence of independent competing risks, Qual. Reliab. Eng. Int., 32 (2016), 363–372. https://doi.org/10.1002/qre.1755 doi: 10.1002/qre.1755
![]() |
[4] | L. Liu, X. Liu, X. Wang, Y. Wang, C. Li, Reliability analysis and evaluation of a brake system based on competing risk, J. Eng. Res., 5 (2017), 150–161. |
[5] | H. Li, M. Yazdi, Stochastic game theory approach to solve system safety and reliability decision-making problem under uncertainty, In: H. Li, M. Yazdi, Advanced decision-making methods and applications in system safety and reliability problems, Springer, 2022. https://doi.org/10.1007/978-3-031-07430-1-8 |
[6] |
M. Yazdi, E. Zarei, S. Adumene, R. Abbassi, P. Rahnamayiezekavat, Uncertainty modeling in risk assessment of digitalized process systems, Methods Chem. Process Saf., 6 (2022), 389–416. https://doi.org/10.1016/bs.mcps.2022.04.005 doi: 10.1016/bs.mcps.2022.04.005
![]() |
[7] | E. Zarei, M. Yazdi, R. Moradi, A. B. Toroody, Expert judgment and uncertainty in sociotechnical systems analysis, In: E. Zarei, Safety causation analysis in sociotechnical systems: advanced models and techniques, Springer, 2024. https://doi.org/10.1007/978-3-031-62470-4_18 |
[8] |
N. Balakrishnan, Progressive censoring methodology: an appraisal, Test, 16 (2007), 211–259. https://doi.org/10.1007/s11749-007-0061-y doi: 10.1007/s11749-007-0061-y
![]() |
[9] |
H. K. Ng, P. S. Chan, Comments on: progressive censoring methodology: an appraisal, Test, 16 (2007), 287–289. https://doi.org/10.1007/s11749-007-0071-9 doi: 10.1007/s11749-007-0071-9
![]() |
[10] |
Y. Cho, H. Sun, K. Lee, Exact likelihood inference for an exponential parameter under generalized progressive hybrid censoring scheme, Stat. Methodol., 23 (2015), 18–34. https://doi.org/10.1016/j.stamet.2014.09.002 doi: 10.1016/j.stamet.2014.09.002
![]() |
[11] |
D. Kundu, A. Joarder, Analysis of type-II progressively hybrid censored competing risks data, J. Mod. Appl. Stat. Methods, 5 (2006), 152–170. https://doi.org/10.22237/JMASM/1146456780 doi: 10.22237/JMASM/1146456780
![]() |
[12] |
H. Liao, W. Gui, Statistical inference of the Rayleigh distribution based on progressively type II censored competing risks data, Symmetry, 11 (2019), 898. https://doi.org/10.3390/sym11070898 doi: 10.3390/sym11070898
![]() |
[13] |
L. Wang, Y. M. Tripathi, C. Lodhi, Inference for Weibull competing risks model with partially observed failure causes under generalized progressive hybrid censoring, J. Comput. Appl. Math., 368 (2020), 112537. https://doi.org/10.1016/j.cam.2019.112537 doi: 10.1016/j.cam.2019.112537
![]() |
[14] |
A. M. Almarashi, A. Algarni, G. A. Abd-Elmougod, Statistical analysis of competing risks lifetime data from Nadarajaha and Haghighi distribution under type-II censoring, J. Intell. Fuzzy Syst., 38 (2020), 2591–2601. https://doi.org/10.3233/JIFS-179546 doi: 10.3233/JIFS-179546
![]() |
[15] |
M. R. Mahmoud, H. Z. Muhammed, A. R. El-Saeed, Analysis of progressively type-I censored data in competing risks models with generalized inverted exponential distribution, J. Stat. Appl. Probab., 9 (2020), 109–117. https://doi.org/10.18576/jsap/090110 doi: 10.18576/jsap/090110
![]() |
[16] |
A. M. Abd El-Raheem, M. Hosny, M. H. Abu-Moussa, On progressive censored competing risks data: real data application and simulation study, Mathematics, 9 (2021), 1805. https://doi.org/10.3390/math9151805 doi: 10.3390/math9151805
![]() |
[17] |
T. A. Abushal, A. A. Soliman, G. A. Abd-Elmougod, Inference of partially observed causes for failure of Lomax competing risks model under type-II generalized hybrid censoring scheme, Alex. Eng. J., 61 (2022), 5427–5439. https://doi.org/10.1016/j.aej.2021.10.058 doi: 10.1016/j.aej.2021.10.058
![]() |
[18] |
X. Qin, W. Gui, Statistical inference of Lomax distribution based on adaptive progressive type-II hybrid censored competing risks data, Commun. Stat., 52 (2023), 8114–8135. https://doi.org/10.1080/03610926.2022.2056750 doi: 10.1080/03610926.2022.2056750
![]() |
[19] |
A. S. Hassan, R. M. Mousa, M. H. Abu-Moussa, Analysis of progressive type-II competing risks data, with applications, Lobachevskii J. Math., 43 (2022), 2279–2292. https://doi.org/10.1134/S1995080222120149 doi: 10.1134/S1995080222120149
![]() |
[20] |
A. Elshahhat, M. Nassar, Inference of improved adaptive progressively censored competing risks data for Weibull lifetime models, Stat. Papers, 65 (2024), 1163–1196. https://doi.org/10.1007/s00362-023-01417-0 doi: 10.1007/s00362-023-01417-0
![]() |
[21] |
A. S. Hassan, R. M. Mousa, M. H. Abu-Moussa, Bayesian analysis of generalized inverted exponential distribution based on generalized progressive hybrid censoring competing risks data, Ann. Data Sci., 11 (2024), 1225–1264. https://doi.org/10.1007/s40745-023-00488-y doi: 10.1007/s40745-023-00488-y
![]() |
[22] |
Y. Tian, Y. Liang, W. Gui, Inference and optimal censoring scheme for a competing-risks model with type-II progressive censoring, Math. Popul. Stud., 31 (2024), 1–39. https://doi.org/10.1080/08898480.2023.2225349 doi: 10.1080/08898480.2023.2225349
![]() |
[23] |
Q. Lv, R. Hua, W. Gui, Statistical inference of Gompertz distribution under general progressive type II censored competing risks sample, Commun. Stat., 53 (2024), 682–701. https://doi.org/10.1080/03610918.2022.2028834 doi: 10.1080/03610918.2022.2028834
![]() |
[24] |
S. A. Salem, O. Abo-Kasem, A. A. Khairy, Inference for generalized progressive hybrid type-II censored Weibull lifetimes under competing risk data, Comput. J. Math. Stat. Sci., 3 (2024), 177–202. https://doi.org/10.21608/cjmss.2024.256760.1035 doi: 10.21608/cjmss.2024.256760.1035
![]() |
[25] |
R. Maurya, Y. Tripathi, C. Lodhi, M. Rastogi, On a generalized Lomax distribution, Int. J. Syst. Assur. Eng. Manag., 10 (2019), 1091–1104. https://doi.org/10.1007/s13198-019-00839-0 doi: 10.1007/s13198-019-00839-0
![]() |
[26] |
A. Hassan, S. M. Assar, A. Shelbaia, Optimum step stress accelerated life test plan for Lomax distribution with an adaptive type-II progressive hybrid censoring, Br. J. Math. Comput. Sci., 13 (2016), 1–9. https://doi.org/10.9734/BJMCS/2016/21964 doi: 10.9734/BJMCS/2016/21964
![]() |
[27] | A. Alghamdi, Study of generalized Lomax distribution and change point problem, Ph. D. thesis, Bowling Green State University, 2018. |
[28] |
G. Box, D. Cox, An analysis of transformations, J. R. Stat. Soc., 26 (1964), 211–243. https://doi.org/10.1111/j.2517-6161.1964.tb00553.x doi: 10.1111/j.2517-6161.1964.tb00553.x
![]() |
[29] |
S. Varde, Life testing and reliability estimtation for the two parameter exponential distribution, J. Amer. Statist. Assoc., 64 (1969), 621–631. https://doi.org/10.1080/01621459.1969.10501000 doi: 10.1080/01621459.1969.10501000
![]() |
[30] | H. Varian, A Bayesian approach to real estate assessment, In: L. J.Savage, S. E. Feinberg, A. Zellner, Studies in Bayesian econometric and statistics, North-Holland Publication, 1975. |
[31] |
A. Basu, N. Ibrahimi, Bayesian approach to life testing and reliability estimating using asymmetric loss function, J. Stat. Plan. Inference, 29 (1991), 21–31. https://doi.org/10.1016/0378-3758(92)90118-C doi: 10.1016/0378-3758(92)90118-C
![]() |
[32] |
R. Calabria, G. Pulcini, Point estimation under asymmetric loss functions for left-truncated exponential samples, Commun. Stat., 25 (1996), 585–600. https://doi.org/10.1080/0361092960883171583 doi: 10.1080/0361092960883171583
![]() |
[33] |
N. Metropolis, A. Rosenbluth, M. Rosenbluth, A. Teller, E. Teller, Equation of state calculations by fast computing machine, J. Chem. Phys., 21 (1953), 1087–1092. https://doi.org/10.1063/1.1699114 doi: 10.1063/1.1699114
![]() |
[34] |
A. Cohen, Maximum likelihood estimation in the Weibull distribution based on complete and on censored samples, Technometrics, 7 (1965), 579–588. https://doi.org/10.1080/00401706.1965.10490300 doi: 10.1080/00401706.1965.10490300
![]() |
[35] | W. Greene, Econometric analysis, 5 Eds., Pearson Education, Inc., 2003. |
[36] | B. Efron, The Jackknife, the Bootstrap and other resampling plans, CBMS-NSF Regional Conference Series in Applied Mathematics, 1982. |
[37] |
P. Hall, Theoretical comparison of bootstrap confidence intervals, Ann. Stat., 16 (1988), 927–953. https://doi.org/10.1214/aos/1176350933 doi: 10.1214/aos/1176350933
![]() |
[38] |
D. Kundu, N. Kannan, N. Balakrishnan, Analysis of progressively censored competing risks data, Handb. Stat., 23 (2003), 331–348. https://doi.org/10.1016/S0169-7161(03)23018-2 doi: 10.1016/S0169-7161(03)23018-2
![]() |
[39] |
N. Balakrishnan, R. Sandhu, A simple simulational algorithm for generating progressive type-II censored samples, Amer. Stat., 49 (1995), 229–230. https://doi.org/10.1080/00031305.1995.10476150 doi: 10.1080/00031305.1995.10476150
![]() |
[40] |
M. M. El-Din, M. Nagy, M. Abu-Moussa, Estimation and prediction for Gompertz distribution under the generalized progressive hybrid censored data, Ann. Data Sci., 6 (2019), 673–705. https://doi.org/10.1007/s40745-019-00199-3 doi: 10.1007/s40745-019-00199-3
![]() |
[41] |
Z. Xia, J. Yu, L. Cheng, L. Liu, W. Wang. Study on the breaking strength of jute fibers using modified Weibull distribution, Compos. Part A, 40 (2009), 54–59. https://doi.org/10.1016/j.compositesa.2008.10.001 doi: 10.1016/j.compositesa.2008.10.001
![]() |
[42] |
D. Kundu, B. Pradhan, Bayesian analysis of progressively censored competing risks data, Sankhya B, 73 (2011), 276–296. https://doi.org/10.1007/s13571-011-0024-x doi: 10.1007/s13571-011-0024-x
![]() |
[43] |
M. Chen, Q. Shao, Monte Carlo estimation of Bayesian credible and HPD intervals, J. Comput. Graph. Stat., 6 (1988), 66–92. https://doi.org/10.1080/10618600.1999.10474802 doi: 10.1080/10618600.1999.10474802
![]() |
Authors | Related works |
Liao and Gui [12] | Discussed the inference for Rayleigh distribution in the presence of CR under PTIICS. |
Wang et al. [13] | Examined the inference for the Weibull CR model with partially observed failure causes under GPHCS. |
Almarashi et al. [14] | Investigated the statistical analysis of Nadarajaha-Haghighi distribution's CR lifetime under type-II censoring. |
Mahmoud et al. [15] | Studied the progressive of the type-I censoring scheme under CR, focusing specifically on the case where the lifetimes of the subjects follow a generalized inverted exponential distribution. |
El-Raheem et al. [16] | Discussed the point and interval statistical inference of the extension of the exponential distribution's parameters under CR data with PTIICS. |
Abushal et al. [17] | Studied the inference of Lomax CR model with partially observed failure causes under the type-II |
generalized hybrid censoring scheme. | |
Qin and Gui [18] | Analyzed CR from Lomax distribution based on adaptive progressive type-II hybrid censored. |
Hassan et al. [19] | Evaluated CR from the analysis of the inverted Topp-Leone distribution under PTIICS. |
Ahmed and Nassar[20] | Examined electrode data analysis within the framework of the Weibull lifetime CR model in the context of improved adaptive progressive censoring. |
Hassen et al.[21] | Studied the Bayesian statistical inference of the generalized inverted exponential distribution through the CR model under GPHCS. |
Tian et al.[22] | Examined the implications of the inverted exponential Rayleigh distribution using the CR model when |
employing optimal PTIICS. | |
Lv et al.[23] | Explored the process of inferring statistics through the CR sample when subjects' lifetimes follow the |
Gompertz distribution in the context of general PTIICS. | |
Salem et al.[24] | Obtained the maximum likelihood estimates (MLEs) and Bayesian estimates (BEs) from the Weibull distribution based on the censored Generalized Progressive Hybrid type-II in the presence of the CR model. |
yi:m:n | 0.50575 | 0.5447 | 0.7069 | 0.7574 | 0.817 | 0.83935 | 0.841 | 0.88625 | 0.89125 | 0.9271 |
δi | 2 | 2 | 2 | 2 | 2 | 1 | 1 | 2 | 1 | 1 |
R | 2 | 0 | 0 | 2 | 0 | 0 | 2 | 0 | 0 | 1 |
yi:m:n | 0.9384 | 1.06065 | 1.0943 | 1.13265 | 1.27145 | 1.341 | 1.35395 | 1.45635 | 1.5242 | 1.53495 |
δi | 1 | 2 | 1 | 1 | 1 | 1 | 1 | 2 | 1 | 1 |
R | 0 | 0 | 0 | 1 | 0 | 2 | 0 | 1 | 0 | 1 |
yi:m:n | 1.57665 | 1.61915 | 1.7662 | 1.8821 | 1.91715 | 2.20935 | 2.47755 | 2.533 | 2.5824 | 2.65275 |
δi | 1 | 2 | 2 | 2 | 2 | 1 | 1 | 2 | 1 | 2 |
R | 0 | 0 | 0 | 0 | 0 | 1 | 0 | 1 | 0 | 1 |
yi:m:n | 2.77305 | 2.83155 | 2.9524 | 3.09285 | 3.1883 | 3.5037 | 3.5233 | 3.63615 | 4.04615 | 4.11515 |
δi | 1 | 1 | 2 | 1 | 2 | 2 | 2 | 2 | 1 | 1 |
R | 0 | 0 | 0 | 0 | 0 | 1 | 0 | 0 | 1 | 3 |
yi:m:n | 0.21965 | 0.50575 | 0.6153 | 0.6454 | 0.817 | 0.83935 | 0.841 | 0.9271 | 1.13265 |
δi | 2 | 2 | 2 | 1 | 1 | 1 | 1 | 1 | 1 |
R | 3 | 0 | 3 | 0 | 3 | 0 | 3 | 0 | 3 |
yi:m:n | 1.30485 | 1.5242 | 1.57665 | 1.8385 | 1.8501 | 1.91715 | 2.20935 | 2.47755 | 2.68725 |
δi | 1 | 2 | 1 | 1 | 1 | 2 | 1 | 1 | 1 |
R | 0 | 3 | 0 | 3 | 0 | 3 | 0 | 3 | 0 |
yi:m:n | 2.77305 | 2.83155 | 2.9524 | 3.09285 | 3.5233 | 3.89085 | 4.11515 | ||
δi | 1 | 1 | 2 | 1 | 2 | 2 | 1 | ||
R | 3 | 0 | 3 | 0 | 2 | 0 | 0 |
n | m | k | Scheme | ˆα1ML | ˆα1BS | ˆα1BL | ˆα1BL | ˆα1BG | ˆα1BG | |
(h=1.5) | (h=−1.5) | (c=0.5) | (c=−0.5) | |||||||
60 | 40 | 25 | 1 | AB | 0.0061 | 0.0027 | 0.0102 | 0.0167 | 0.0234 | 0.0060 |
MSE | 0.0136 | 0.0093 | 0.0088 | 0.0102 | 0.0096 | 0.0092 | ||||
2 | AB | 0.0093 | 0.0045 | 0.0083 | 0.0184 | 0.0211 | 0.0040 | |||
MSE | 0.0123 | 0.0090 | 0.0085 | 0.0099 | 0.0092 | 0.0089 | ||||
3 | AB | 0.0079 | 0.0058 | 0.0054 | 0.0178 | 0.0163 | 0.0016 | |||
MSE | 0.0094 | 0.0084 | 0.0079 | 0.0092 | 0.0083 | 0.0083 | ||||
30 | 1 | AB | 0.0077 | 0.0056 | 0.0070 | 0.0192 | 0.0196 | 0.0028 | ||
MSE | 0.0124 | 0.0092 | 0.0087 | 0.0102 | 0.0093 | 0.0091 | ||||
2 | AB | 0.0067 | 0.0060 | 0.0067 | 0.0197 | 0.0192 | 0.0024 | |||
MSE | 0.0123 | 0.0092 | 0.0086 | 0.0102 | 0.0093 | 0.0091 | ||||
3 | AB | 0.0062 | 0.0054 | 0.0057 | 0.0174 | 0.0167 | 0.0020 | |||
MSE | 0.0094 | 0.0084 | 0.0079 | 0.0093 | 0.0084 | 0.0083 | ||||
50 | 25 | 1 | AB | 0.0095 | 0.0034 | 0.0082 | 0.0158 | 0.0198 | 0.0044 | |
MSE | 0.0106 | 0.0085 | 0.0081 | 0.0093 | 0.0087 | 0.0085 | ||||
2 | AB | 0.0094 | 0.0027 | 0.0088 | 0.0151 | 0.0203 | 0.0050 | |||
MSE | 0.0108 | 0.0086 | 0.0082 | 0.0094 | 0.0089 | 0.0086 | ||||
3 | AB | 0.0082 | 0.0043 | 0.0062 | 0.0155 | 0.0166 | 0.0027 | |||
MSE | 0.0090 | 0.0078 | 0.0074 | 0.0085 | 0.0079 | 0.0078 | ||||
30 | 1 | AB | 0.0069 | 0.0033 | 0.0082 | 0.0157 | 0.0198 | 0.0044 | ||
MSE | 0.0106 | 0.0087 | 0.0083 | 0.0094 | 0.0089 | 0.0086 | ||||
2 | AB | 0.0082 | 0.0041 | 0.0074 | 0.0165 | 0.0189 | 0.0036 | |||
MSE | 0.0106 | 0.0085 | 0.0080 | 0.0093 | 0.0086 | 0.0084 | ||||
3 | AB | 0.0070 | 0.0026 | 0.0079 | 0.0137 | 0.0183 | 0.0044 | |||
MSE | 0.0090 | 0.0077 | 0.0074 | 0.0083 | 0.0079 | 0.0077 |
n | m | k | Scheme | ˆα2ML | ˆα2BS | ˆα2BL | ˆα2BL | ˆα2BG | ˆα2BG | |
(h=1.5) | (h=−1.5) | (c=0.5) | (c=−0.5) | |||||||
60 | 40 | 25 | 1 | AB | 0.0151 | 0.0016 | 0.0157 | 0.0203 | 0.0219 | 0.0062 |
MSE | 0.0142 | 0.0091 | 0.0088 | 0.0100 | 0.0094 | 0.0091 | ||||
2 | AB | 0.0121 | 0.0023 | 0.0150 | 0.0208 | 0.0211 | 0.0055 | |||
MSE | 0.0129 | 0.0086 | 0.0084 | 0.0096 | 0.0089 | 0.0086 | ||||
3 | AB | 0.0097 | 0.0061 | 0.0096 | 0.0229 | 0.0149 | 0.0009 | |||
MSE | 0.0098 | 0.0080 | 0.0076 | 0.0090 | 0.0080 | 0.0079 | ||||
30 | 1 | AB | 0.0088 | 0.0046 | 0.0124 | 0.0227 | 0.0182 | 0.0031 | ||
MSE | 0.0131 | 0.0088 | 0.0084 | 0.0099 | 0.0089 | 0.0087 | ||||
2 | AB | 0.0120 | 0.0044 | 0.0127 | 0.0228 | 0.0186 | 0.0033 | |||
MSE | 0.0127 | 0.0087 | 0.0083 | 0.0097 | 0.0088 | 0.0086 | ||||
3 | AB | 0.0107 | 0.0058 | 0.0098 | 0.0226 | 0.0151 | 0.0012 | |||
MSE | 0.0099 | 0.0084 | 0.0080 | 0.0095 | 0.0084 | 0.0083 | ||||
50 | 25 | 1 | AB | 0.0110 | 0.0025 | 0.0135 | 0.0196 | 0.0190 | 0.0047 | |
MSE | 0.0112 | 0.0085 | 0.0082 | 0.0093 | 0.0087 | 0.0084 | ||||
2 | AB | 0.0110 | 0.0022 | 0.0137 | 0.0193 | 0.0193 | 0.0050 | |||
MSE | 0.0112 | 0.0085 | 0.0082 | 0.0093 | 0.0087 | 0.0085 | ||||
3 | AB | 0.0113 | 0.0031 | 0.0117 | 0.0190 | 0.0168 | 0.0035 | |||
MSE | 0.0094 | 0.0080 | 0.0077 | 0.0088 | 0.0082 | 0.0079 | ||||
30 | 1 | AB | 0.0126 | 0.0034 | 0.0126 | 0.0205 | 0.0181 | 0.0038 | ||
MSE | 0.0111 | 0.0087 | 0.0084 | 0.0096 | 0.0089 | 0.0087 | ||||
2 | AB | 0.0121 | 0.0041 | 0.0074 | 0.0165 | 0.0189 | 0.0036 | |||
MSE | 0.0112 | 0.0085 | 0.0080 | 0.0092 | 0.0086 | 0.0084 | ||||
3 | AB | 0.0132 | 0.0032 | 0.0115 | 0.0192 | 0.0167 | 0.0034 | |||
MSE | 0.0095 | 0.0079 | 0.0077 | 0.0087 | 0.0081 | 0.0079 |
n | m | k | Scheme | ˆα1ML | ˆα1BS | ˆα1BL | ˆα1BL | ˆα1BG | ˆα1BG | |
(h=1.5) | (h=−1.5) | (c=0.5) | (c=−0.5) | |||||||
60 | 40 | 25 | 1 | AB | 0.0063 | 0.0029 | 0.0160 | 0.0231 | 0.0212 | 0.0051 |
MSE | 0.0316 | 0.0116 | 0.0112 | 0.0129 | 0.0119 | 0.0116 | ||||
2 | AB | 0.0093 | 0.0026 | 0.0163 | 0.0227 | 0.0215 | 0.0054 | |||
MSE | 0.0315 | 0.0117 | 0.0113 | 0.0130 | 0.0120 | 0.0117 | ||||
3 | AB | 0.0065 | 0.0029 | 0.0157 | 0.0227 | 0.0207 | 0.0050 | |||
MSE | 0.0295 | 0.0117 | 0.0113 | 0.0130 | 0.0120 | 0.0117 | ||||
30 | 1 | AB | 0.0063 | 0.0023 | 0.0165 | 0.0225 | 0.0218 | 0.0057 | ||
MSE | 0.0322 | 0.0114 | 0.0110 | 0.0126 | 0.0117 | 0.0114 | ||||
2 | AB | 0.0077 | 0.0011 | 0.0177 | 0.0212 | 0.0230 | 0.0069 | |||
MSE | 0.0321 | 0.0118 | 0.0114 | 0.0130 | 0.0121 | 0.0117 | ||||
3 | AB | 0.0051 | 0.0033 | 0.0152 | 0.0232 | 0.0203 | 0.0045 | |||
MSE | 0.0303 | 0.0117 | 0.0113 | 0.0130 | 0.0119 | 0.0117 | ||||
50 | 25 | 1 | AB | 0.0062 | 0.0043 | 0.0127 | 0.0225 | 0.0174 | 0.0029 | |
MSE | 0.0262 | 0.0114 | 0.0109 | 0.0125 | 0.0115 | 0.0113 | ||||
2 | AB | 0.0069 | 0.0028 | 0.0142 | 0.0209 | 0.0188 | 0.0044 | |||
MSE | 0.0247 | 0.0113 | 0.01090 | 0.0124 | 0.0115 | 0.0113 | ||||
3 | AB | 0.0033 | 0.0044 | 0.0123 | 0.0221 | 0.0167 | 0.0027 | |||
MSE | 0.0247 | 0.0114 | 0.0109 | 0.0125 | 0.0114 | 0.0113 | ||||
30 | 1 | AB | 0.0061 | 0.0011 | 0.0159 | 0.0192 | 0.0205 | 0.0061 | ||
MSE | 0.0266 | 0.0112 | 0.0109 | 0.0122 | 0.0115 | 0.0112 | ||||
2 | AB | 0.0083 | 0.0052 | 0.0119 | 0.0234 | 0.0164 | 0.0020 | |||
MSE | 0.0258 | 0.0115 | 0.0110 | 0.0127 | 0.0116 | 0.0114 | ||||
3 | AB | 0.0041 | 0.0045 | 0.0122 | 0.0222 | 0.0166 | 0.0026 | |||
MSE | 0.0247 | 0.0112 | 0.0108 | 0.0124 | 0.0113 | 0.0112 |
n | m | k | Scheme | ˆα2ML | ˆα2BS | ˆα2BL | ˆα2BL | ˆα2BG | ˆα2BG | |
(h=1.5) | (h=−1.5) | (c=0.5) | (c=−0.5) | |||||||
60 | 40 | 25 | 1 | AB | 0.0130 | 0.0025 | 0.0225 | 0.0287 | 0.0145 | 0.0032 |
MSE | 0.0337 | 0.0092 | 0.0093 | 0.0105 | 0.0093 | 0.0092 | ||||
2 | AB | 0.0120 | 0.0005 | 0.0245 | 0.0267 | 0.0165 | 0.0052 | |||
MSE | 0.0335 | 0.0090 | 0.0092 | 0.0102 | 0.0092 | 0.0090 | ||||
3 | AB | 0.0105 | 0.0038 | 0.0212 | 0.0299 | 0.0131 | 0.0019 | |||
MSE | 0.0300 | 0.0089 | 0.0089 | 0.0102 | 0.0089 | 0.0088 | ||||
30 | 1 | AB | 0.0137 | 0.0013 | 0.0237 | 0.0275 | 0.0156 | 0.0043 | ||
MSE | 0.0342 | 0.0093 | 0.0095 | 0.0106 | 0.0095 | 0.0093 | ||||
2 | AB | 0.0131 | 0.0031 | 0.0220 | 0.0293 | 0.0139 | 0.0026 | |||
MSE | 0.0345 | 0.0092 | 0.0092 | 0.0105 | 0.0093 | 0.0092 | ||||
3 | AB | 0.0115 | 0.0042 | 0.0207 | 0.0304 | 0.0127 | 0.0014 | |||
MSE | 0.0310 | 0.0090 | 0.0090 | 0.0104 | 0.0091 | 0.0090 | ||||
50 | 25 | 1 | AB | 0.0115 | 0.0037 | 0.0198 | 0.0283 | 0.0122 | 0.0016 | |
MSE | 0.0281 | 0.0099 | 0.0098 | 0.0111 | 0.0099 | 0.0098 | ||||
2 | AB | 0.0100 | 0.0005 | 0.0230 | 0.0250 | 0.0154 | 0.0048 | |||
MSE | 0.0269 | 0.0096 | 0.0097 | 0.0107 | 0.0098 | 0.0096 | ||||
3 | AB | 0.0118 | 0.0034 | 0.0198 | 0.0277 | 0.0123 | 0.0019 | |||
MSE | 0.0258 | 0.0095 | 0.0094 | 0.0107 | 0.0095 | 0.0094 | ||||
30 | 1 | AB | 0.0114 | 0.0012 | 0.0223 | 0.0257 | 0.0147 | 0.0041 | ||
MSE | 0.0285 | 0.0097 | 0.0097 | 0.0108 | 0.0098 | 0.0096 | ||||
2 | AB | 0.0096 | 0.0009 | 0.0225 | 0.0254 | 0.0150 | 0.0044 | |||
MSE | 0.0277 | 0.0093 | 0.0094 | 0.0104 | 0.0094 | 0.0093 | ||||
3 | AB | 0.0106 | 0.0029 | 0.0203 | 0.0272 | 0.0128 | 0.0024 | |||
MSE | 0.0257 | 0.0095 | 0.0095 | 0.0107 | 0.0096 | 0.0095 |
n | m | k | Scheme | ˆβML | ˆβBS | ˆβBL | ˆβBL | ˆβBG | ˆβBG | |
(h=1.5) | (h=−1.5) | (c=0.5) | (c=−0.5) | |||||||
60 | 40 | 25 | 1 | AB | 0.0790 | 0.0116 | 0.0200 | 0.0447 | 0.0045 | 0.0062 |
MSE | 0.2168 | 0.0041 | 0.0042 | 0.0061 | 0.0040 | 0.0040 | ||||
2 | AB | 0.0750 | 0.0108 | 0.0205 | 0.0435 | 0.0051 | 0.0055 | |||
MSE | 0.2000 | 0.0042 | 0.0044 | 0.0062 | 0.0042 | 0.0042 | ||||
3 | AB | 0.0774 | 0.0106 | 0.0201 | 0.0427 | 0.0050 | 0.0054 | |||
MSE | 0.1685 | 0.0043 | 0.0045 | 0.0062 | 0.0042 | 0.0042 | ||||
30 | 1 | AB | 0.0851 | 0.0106 | 0.0210 | 0.0437 | 0.0054 | 0.0053 | ||
MSE | 0.2232 | 0.0040 | 0.0042 | 0.0059 | 0.0039 | 0.0039 | ||||
2 | AB | 0.0781 | 0.0116 | 0.0197 | 0.0444 | 0.0042 | 0.0063 | |||
MSE | 0.1966 | 0.0043 | 0.0044 | 0.0063 | 0.0041 | 0.0042 | ||||
3 | AB | 0.0716 | 0.0110 | 0.0196 | 0.0430 | 0.0045 | 0.0058 | |||
MSE | 0.1690 | 0.0044 | 0.0045 | 0.0063 | 0.0043 | 0.0043 | ||||
50 | 25 | 1 | AB | 0.0729 | 0.0115 | 0.0194 | 0.0439 | 0.0042 | 0.0063 | |
MSE | 0.1736 | 0.0043 | 0.0044 | 0.0063 | 0.0042 | 0.0042 | ||||
2 | AB | 0.0735 | 0.0089 | 0.0219 | 0.0412 | 0.0068 | 0.0037 | |||
MSE | 0.1701 | 0.0042 | 0.0045 | 0.0059 | 0.0041 | 0.0041 | ||||
3 | AB | 0.0669 | 0.0106 | 0.0198 | 0.0424 | 0.0049 | 0.0054 | |||
MSE | 0.1502 | 0.0043 | 0.0044 | 0.0061 | 0.0042 | 0.0042 | ||||
30 | 1 | AB | 0.0765 | 0.0093 | 0.0217 | 0.0417 | 0.0064 | 0.0040 | ||
MSE | 0.1721 | 0.0042 | 0.0044 | 0.0060 | 0.0041 | 0.0041 | ||||
2 | AB | 0.0697 | 0.0101 | 0.0208 | 0.0424 | 0.0056 | 0.0049 | |||
MSE | 0.1724 | 00042 | 0.0044 | 0.0060 | 0.0041 | 0.0041 | ||||
3 | AB | 0.0670 | 0.0103 | 0.0202 | 0.0421 | 0.0052 | 0.0051 | |||
MSE | 0.1477 | 0.0042 | 0.0044 | 0.0060 | 0.0041 | 0.0041 |
n | m | k | Scheme | ˆβML | ˆβBS | ˆβBL | ˆβ1BL | ˆβBG | ˆβBG | |
(h=1.5) | (h=−1.5) | (c=0.5) | (c=−0.5) | |||||||
60 | 40 | 25 | 1 | AB | 0.0551 | 0.0221 | 0.0064 | 0.0533 | 0.0066 | 0.0125 |
MSE | 0.0799 | 0.0045 | 0.0039 | 0.0072 | 0.0041 | 0.0042 | ||||
2 | AB | 0.0592 | 0.0232 | 0.0047 | 0.0537 | 0.0049 | 0.0139 | |||
MSE | 0.0729 | 0.0046 | 0.0039 | 0.0073 | 0.0041 | 0.0043 | ||||
3 | AB | 0.0468 | 0.0212 | 0.0061 | 0.0511 | 0.0063 | 0.0120 | |||
MSE | 0.0556 | 0.0043 | 0.0037 | 0.0067 | 0.0039 | 0.0040 | ||||
30 | 1 | AB | 0.0635 | 0.0219 | 0.0065 | 0.0529 | 0.0068 | 0.0123 | ||
MSE | 0.0792 | 0.0046 | 0.0039 | 0.0072 | 0.0041 | 0.0042 | ||||
2 | AB | 0.0647 | 0.0232 | 0.0046 | 0.0537 | 0.0048 | 0.0139 | |||
MSE | 0.0708 | 0.0047 | 0.0040 | 0.0074 | 0.0042 | 0.0044 | ||||
3 | AB | 0.0487 | 0.0219 | 0.0054 | 0.0517 | 0.0056 | 0.0127 | |||
MSE | 0.0546 | 0.0042 | 0.0036 | 0.0067 | 0.0038 | 0.0039 | ||||
50 | 25 | 1 | AB | 0.0521 | 0.0230 | 0.0048 | 0.0534 | 0.0050 | 0.0137 | |
MSE | 0.0644 | 0.0045 | 0.0037 | 0.0071 | 0.0040 | 0.0042 | ||||
2 | AB | 0.0511 | 0.0223 | 0.0054 | 0.0525 | 0.0056 | 0.0130 | |||
MSE | 0.0637 | 0.0046 | 0.0039 | 0.0071 | 0.0041 | 0.0042 | ||||
3 | AB | 0.0475 | 0.0208 | 0.0064 | 0.0506 | 0.0066 | 0.0117 | |||
MSE | 0.0543 | 0.0044 | 0.0038 | 0.0068 | 0.0040 | 0.0042 | ||||
30 | 1 | AB | 0.0565 | 0.0229 | 0.0050 | 0.0533 | 0.0052 | 0.0135 | ||
MSE | 0.0646 | 0.0045 | 0.0038 | 0.0071 | 0.0040 | 0.0042 | ||||
2 | AB | 0.0536 | 0.0230 | 0.0047 | 0.0533 | 0.0049 | 0.0137 | |||
MSE | 0.0638 | 0.0046 | 0.0038 | 0.0072 | 0.0040 | 0.0042 | ||||
3 | AB | 0.0462 | 0.0216 | 0.0057 | 0.0513 | 0.0059 | 0.0124 | |||
MSE | 0.0535 | 0.0044 | 0.0037 | 0.0068 | 0.0039 | 0.0040 |
n | t | m | k | Scheme | ˆℜML | ˆℜBS | ˆℜBL | ˆℜBL | ˆℜBG | ˆℜBG | |
(h=1.5) | (h=−1.5) | (c=0.5) | (c=−0.5) | ||||||||
60 | 0.1 | 40 | 25 | 1 | AE | 0.9987 | 0.9987 | 0.9987 | 0.9987 | 0.9987 | 0.9987 |
MSE | 1.2437*10−7 | 3.6402*10−8 | 3.6408*10−8 | 3.6396*10−8 | 3.6408*10−8 | 3.6404*10−8 | |||||
2 | AE | 0.99870 | 0.9987 | 0.9987 | 0.9987 | 0.9987 | 0.9987 | ||||
MSE | 1.0923*10−7 | 3.5807*10−8 | 3.5812*10−8 | 3.5818*10−8 | 3.5812*10−8 | 3.5809*10−8 | |||||
3 | AE | 0.9987 | 0.9987 | 0.9987 | 0.9987 | 0.9987 | 0.9987 | ||||
MSE | 8.5362*10−8 | 3.2401*10−8 | 3.2406*10−8 | 3.2397*10−8 | 3.2406*10−8 | 3.2403*10−8 | |||||
30 | 1 | AE | 0.9988 | 0.9987 | 0.9987 | 0.9987 | 0.9987 | 0.9987 | |||
MSE | 1.226*10−7 | 3.567*10−8 | 3.567*10−8 | 3.566*10−8 | 3.567*10−8 | 3.566*10−8 | |||||
2 | AE | 0.9987 | 0.9987 | 0.9987 | 0.9987 | 0.9987 | 0.9987 | ||||
MSE | 10.36*10−8 | 34.04*10−9 | 34.04*10−9 | 34.06*10−9 | 34.04*10−9 | 34.04*10−9 | |||||
3 | AE | 0.9987 | 0.9987 | 0.9987 | 0.9987 | 0.9987 | 0.9987 | ||||
MSE | 8.526*10−8 | 3.2184*10−8 | 3.2188*10−8 | 3.2180*10−8 | 3.2188*10−8 | 3.2185*10−8 | |||||
50 | 25 | 1 | AE | 0.9987 | 0.9987 | 0.9987 | 0.9987 | 0.9987 | 0.9987 | ||
MSE | 9.770*10−8 | 3.3138*10−8 | 3.3134*10−8 | 3.3134*10−8 | 3.3143*10−8 | 3.3140*10−8 | |||||
2 | AE | 0.9987 | 0.9987 | 0.9987 | 0.9987 | 0.9987 | 0.9987 | ||||
MSE | 9.3246*10−8 | 3.2173*10−8 | 3.2178*10−8 | 3.2169*10−8 | 3.2178*10−8 | 3.2175*10−8 | |||||
3 | AE | 0.9987 | 0.9987 | 0.9987 | 0.9987 | 0.9987 | 0.9987 | ||||
MSE | 8.0102*10−8 | 2.9671*10−8 | 2.9674*10−8 | 2.9668*10−8 | 2.9674*10−8 | 2.9672*10−8 | |||||
30 | 1 | AE | 0.9987 | 0.9987 | 0.9987 | 0.9987 | 0.9987 | 0.9987 | |||
MSE | 9.900*10−8 | 3.3295*10−8 | 3.3300*10−8 | 3.3291*10−8 | 3.330*10−8 | 3.3297*10−8 | |||||
2 | AE | 0.9987 | 0.9987 | 0.9987 | 0.9987 | 0.9987 | 0.9987 | ||||
MSE | 9.445*10−8 | 3.2535*10−8 | 3.2539*10−8 | 3.2530*10−8 | 3.2539*10−8 | 3.2536*10−8 | |||||
3 | AE | 0.9987 | 0.9987 | 0.9987 | 0.9987 | 0.9987 | 0.9987 | ||||
MSE | 7.702*10−8 | 2.8550*10−8 | 2.8554*10−8 | 2.8547*10−8 | 2.8554*10−8 | 2.8552*10−8 | |||||
0.5 | 40 | 25 | 1 | AE | 0.8587 | 0.8616 | 0.8613 | 0.8621 | 0.8612 | 0.8615 | |
MSE | 0.0011 | 34.93*10−5 | 35.34*10−5 | 34.53*10−5 | 35.46*10−5 | 35.10*10−5 | |||||
2 | AE | 0.8597 | 0.8621 | 0.8617 | 0.8624 | 0.8616 | 0.8619 | ||||
MSE | 9.393*10−4 | 34.41*10−5 | 34.78*10−5 | 34.07*10−5 | 34.88*10−5 | 34.56*10−5 | |||||
3 | AE | 0.8600 | 0.8619 | 0.8616 | 0.8622 | 0.8616 | 0.8618 | ||||
MSE | 7.479*10−4 | 31.24*10−5 | 31.55*10−5 | 30.96*10−5 | 31.63*10−5 | 31.37*10−5 | |||||
30 | 1 | AE | 0.8583 | 0.8615 | 0.8611 | 0.8618 | 0.8610 | 0.8613 | |||
MSE | 10.44*10−4 | 34.12*10−5 | 34.57*10−5 | 33.71*10−5 | 34.68*10−5 | 34.30*10−5 | |||||
2 | AE | 0.8587 | 0.8615 | 0.8611 | 0.8618 | 0.6810 | 0.8613 | ||||
MSE | 8.931*10−4 | 32.58*10−5 | 32.99*10−5 | 32.21*10−5 | 33.10*10−5 | 32.75*10−5 | |||||
3 | AE | 0.8598 | 0.8618 | 0.8615 | 0.8621 | 0.8614 | 0.8617 | ||||
MSE | 7.448*10−4 | 30.98*10−5 | 31.30*10−5 | 30.69*10−5 | 31.38*10−5 | 31.11*10−5 | |||||
50 | 25 | 1 | AE | 0.8600 | 0.8621 | 0.8618 | 0.8624 | 0.8617 | 0.8620 | ||
MSE | 8.528*10−4 | 32.01*10−5 | 32.30*10−5 | 31.73*10−5 | 32.39*10−5 | 32.13*10−5 | |||||
2 | AE | 0.8601 | 0.8622 | 0.8618 | 0.8625 | 0.8618 | 0.8620 | ||||
MSE | 8.140*10−4 | 31.06*10−5 | 31.35*10−5 | 30.80*10−5 | 31.43*10−5 | 31.18*10−5 | |||||
3 | AE | 0.8609 | 0.8626 | 0.8623 | 0.8629 | 0.8622 | 0.8624 | ||||
MSE | 7.049*10−4 | 28.76*10−5 | 28.97*10−5 | 28.56*10−5 | 29.03*10−5 | 28.85*10−5 | |||||
30 | 1 | AE | 0.8597 | 0.8620 | 0.8617 | 0.8623 | 0.8616 | 0.8619 | |||
MSE | 8.597*10−4 | 32.09*10−5 | 32.40*10−5 | 31.81*10−5 | 32.49*10−5 | 32.22*10−5 | |||||
2 | AE | 0.8600 | 0.8622 | 0.8619 | 0.8625 | 0.8618 | 0.8620 | ||||
MSE | 8.218*10−4 | 31.37*10−5 | 31.67*10−5 | 31.11*10−5 | 31.75*10−5 | 31.50*10−5 | |||||
3 | AE | 0.8605 | 0.8623 | 0.8620 | 0.8626 | 0.8619 | 0.8622 | ||||
MSE | 6.793*10−4 | 27.68*10−5 | 27.89*10−5 | 27.47*10−5 | 27.96*10−5 | 27.76*10−5 |
n | t | m | k | Scheme | ˆℏML | ˆℏBS | ˆℏBL | ˆℏBL | ˆℏBG | ˆℏBG | |
(h=1.5) | (h=−1.5) | (c=0.5) | (c=−0.5) | ||||||||
60 | 0.1 | 40 | 25 | 1 | AE | 0.03922 | 0.03814 | 0.038102 | 0.03818 | 0.03718 | 0.03782 |
MSE | 1.11*10−4 | 3.28*10−5 | 3.26*10−5 | 3.30*10−5 | 3.133*10−5 | 3.21*10−5 | |||||
2 | AE | 0.0389 | 0.03801 | 0.03797 | 0.03804 | 0.03710 | 0.03771 | ||||
MSE | 9.836*10−5 | 3.227*10−5 | 3.213*10−5 | 3.241*10−5 | 3.097*10−5 | 3.165*10−5 | |||||
3 | AE | 0.03870 | 0.03802 | 0.03799 | 0.3805 | 0.03727 | 0.03777 | ||||
MSE | 7.687*10−5 | 2.920*10−5 | 2.908*10−5 | 2.931*10−5 | 2.799*10−5 | 2.867*10−5 | |||||
30 | 1 | AE | 0.0393 | 0.03820 | 0.03816 | 0.03823 | 0.03725 | 0.03788 | |||
MSE | 1.104*10−4 | 3.214*10−5 | 3.198*10−5 | 3.231*10−5 | 3.041*10−5 | 3.136*10−5 | |||||
2 | AE | 0.03916 | 0.03818 | 0.03184 | 0.03821 | 0.03728 | 0.03788 | ||||
MSE | 9.333*10−5 | 3.067*10−5 | 3.052*10−5 | 3.083*10−5 | 2.905*10−5 | 2.995*10−5 | |||||
3 | AE | 0.03876 | 0.03806 | 0.03803 | 0.03809 | 0.03705 | 0.037809 | ||||
MSE | 7.677*10−5 | 2.900*10−5 | 2.889*10−5 | 2.912*10−5 | 2.77*10−5 | 2.845*10−5 | |||||
50 | 25 | 1 | AE | 0.03875 | 0.03797 | 0.037938 | 0.03800 | 0.03714 | 0.03769 | ||
MSE | 8.798*10−5 | 2.986*10−5 | 2.975*10−5 | 2.998*10−5 | 2.887*10−5 | 2.938*10−5 | |||||
2 | AE | 0.03872 | 0.03496 | 0.03793 | 0.03799 | 0.03714 | 0.03769 | ||||
MSE | 8.397*10−5 | 2.899*10−5 | 2.888*10−5 | 2.911*10−5 | 2.802*10−5 | 2.852*10−5 | |||||
3 | AE | 0.03841 | 0.03782 | 0.03779 | 0.03785 | 0.03709 | 0.03758 | ||||
MSE | 7.213*10−5 | 2.674*10−5 | 2.665*10−5 | 2.682*10−5 | 2.609*10−5 | 2.640*10−5 | |||||
30 | 1 | AE | 0.03883 | 0.03802 | 0.03798 | 0.03805 | 0.03719 | 0.03774 | |||
MSE | 8.915*10−5 | 3.00*10−5 | 2.988*10−5 | 3.012*10−5 | 2.893*10−5 | 2.949*10−5 | |||||
2 | AE | 0.03873 | 0.03796 | 0.03793 | 0.03799 | 0.03714 | 0.03377 | ||||
MSE | 8.505*10−5 | 2.932*10−5 | 2.920*10−5 | 2.943*10−5 | 2.833*10−5 | 2.884*10−5 | |||||
3 | AE | 0.03853 | 0.03790 | 0.037874 | 0.037931 | 0.03717 | 0.03766 | ||||
MSE | 6.936*10−5 | 2.573*10−5 | 2.564*10−5 | 2.582*10−5 | 2.499*10−5 | 2.536*10−5 | |||||
0.5 | 40 | 25 | 1 | AE | 0.8603 | 0.8440 | 0.8284 | 0.8606 | 0.8253 | 0.8378 | |
MSE | 0.0408 | 0.0146 | 0.0137 | 0.0162 | 0.0142 | 0.0144 | |||||
2 | AE | 0.8541 | 0.8413 | 0.8266 | 0.8568 | 0.8237 | 0.8354 | ||||
MSE | 0.0361 | 0.0144 | 0.0135 | 0.0158 | 0.0140 | 0.0142 | |||||
3 | AE | 0.8522 | 0.8421 | 0.8298 | 0.8548 | 0.8275 | 0.8372 | ||||
MSE | 0.0287 | 0.0131 | 0.0123 | 0.0142 | 0.0127 | 0.0129 | |||||
30 | 1 | AE | 0.8628 | 0.8454 | 0.8300 | 0.8616 | 0.8271 | 0.8393 | |||
MSE | 0.0401 | 0.0143 | 0 0133 | 0.0160 | 0.0137 | 0.0140 | |||||
2 | AE | 0.8604 | 0.8451 | 0.8305 | 0.8605 | 0.8277 | 0.8393 | ||||
MSE | 0.0343 | 0.0136 | 0.0127 | 0.0151 | 0.0131 | 0.0134 | |||||
3 | AE | 0.8534 | 0.8428 | 0.8306 | 0.8557 | 0.8283 | 0.8380 | ||||
MSE | 0.0286 | 0.0130 | 0.0122 | 0.0141 | 0.0125 | 0.0128 | |||||
50 | 25 | 1 | AE | 0.8524 | 0.8406 | 0.8273 | 0.8546 | 0.8247 | 0.8353 | ||
MSE | 0.0328 | 0.0133 | 0.0127 | 0.0145 | 0.0131 | 0.0132 | |||||
2 | AE | 0.8519 | 0.8405 | 0.8273 | 0.8543 | 0.8247 | 0.8352 | ||||
MSE | 0.0313 | 0.0130 | 0.0123 | 0.0141 | 0.0127 | 0.0128 | |||||
3 | AE | 0.8466 | 0.8377 | 0.8261 | 0.8499 | 0.8568 | 0.8331 | ||||
MSE | 0.0271 | 0.0120 | 0.0115 | 0.0128 | 0.0118 | 0.0119 | |||||
30 | 1 | AE | 0.8539 | 0.8417 | 0.8283 | 0.8557 | 0.8257 | 0.8363 | |||
MSE | 0.0330 | 0.0134 | 0.0127 | 0.0146 | 0.0131 | 0.0133 | |||||
2 | AE | 0.8522 | 0.8405 | 0.8274 | 0.8544 | 0.8247 | 0.8353 | ||||
MSE | 0.0316 | 0.0131 | 0.0124 | 0.0142 | 0.0128 | 0.0129 | |||||
3 | AE | 0.8491 | 0.8350 | 0.8278 | 0.8516 | 0.8255 | 0.8348 | ||||
MSE | 0.0261 | 0.0115 | 0.0110 | 0.0124 | 0.0113 | 0.0114 |
t | (n,m,k) | Scheme | ˆℜML | ˆℜBS | ˆℜBL | ˆℜBL | ˆℜBG | ˆℜBG | |
(h=1.5) | (h=−1.5) | (c=0.5) | (c=−0.5) | ||||||
0.1 | (60, 40, 25) | 1 | AE | 0.6471 | 0.6573 | 0.6565 | 0.6581 | 0.6560 | 0.6587 |
MSE | 0.0032 | 536*10−6 | 541*10−6 | 533*10−6 | 546*10−6 | 539*10−6 | |||
2 | AE | 0.6493 | 0.6577 | 0.6569 | 0.6585 | 0.6564 | 0.6573 | ||
MSE | 0.0029 | 538*10−6 | 542*10−6 | 535*10−6 | 547*10−6 | 540*10−6 | |||
3 | AE | 0.6510 | 0.6573 | 0.6565 | 0.6581 | 0.6561 | 0.6569 | ||
MSE | 0.0023 | 530*10−6 | 535*10−6 | 527*10−6 | 540*10−6 | 533*10−6 | |||
(60, 40, 30) | 1 | AE | 0.6479 | 0.6576 | 0.6568 | 0.6585 | 0.6563 | 0.6572 | |
MSE | 0.0031 | 524*10−6 | 528*10−6 | 521*10−6 | 532*10−6 | 526*10−6 | |||
2 | AE | 0.6485 | 0.6575 | 0.6566 | 0.6583 | 0.6562 | 0.6570 | ||
MSE | 0.0029 | 531*10−6 | 535*10−6 | 528*10−6 | 541*10−6 | 534*10−6 | |||
3 | AE | 0.6507 | 0.6571 | 0.6563 | 0.6579 | 0.6559 | 0.6567 | ||
MSE | 0.0023 | 538*10−6 | 543*10−6 | 534*10−6 | 548*10−6 | 541*10−6 | |||
(60, 50, 25) | 1 | AE | 0.6473 | 0.6570 | 0.6562 | 0.6577 | 0.6558 | 0.6566 | |
MSE | 0.0027 | 544*10−6 | 549*10−6 | 541*10−6 | 554*10−6 | 547*10−6 | |||
2 | AE | 0.6495 | 0.6579 | 0.6571 | 0.6586 | 0.6567 | 0.6575 | ||
MSE | 0.0025 | 532*10−6 | 535*10−6 | 530*10−6 | 539*10−6 | 534*10−6 | |||
3 | AE | 0.6496 | 0.6571 | 0.6564 | 0.6579 | 0.6560 | 0.6568 | ||
MSE | 0.0022 | 533*10−6 | 537*10−6 | 530*10−6 | 542*10−6 | 536*10−6 | |||
(60, 50, 30) | 1 | AE | 0.6494 | 0.6580 | 0.6572 | 0.6587 | 0.6568 | 0.6576 | |
MSE | 0.0026 | 532*10−6 | 535*10−6 | 530*10−6 | 539*10−6 | 534*10−6 | |||
2 | AE | 0.6485 | 0.6574 | 0.6566 | 0.6581 | 0.6562 | 0.6570 | ||
MSE | 0.0025 | 524*10−6 | 528*10−6 | 522*10−6 | 533*10−6 | 527*10−6 | |||
3 | AE | 0.6498 | 0.6572 | 0.6565 | 0.6580 | 0.6561 | 0.6569 | ||
MSE | 0.0022 | 527*10−6 | 531*10−6 | 523*10−6 | 535*10−6 | 529*10−6 | |||
0.5 | (60, 40, 25) | 1 | ES | 0.2057 | 0.2069 | 0.2059 | 0.2079 | 0.2020 | 0.2052 |
MSE | 0.0026 | 658*10−6 | 647*10−6 | 671*10−6 | 651*10−6 | 650*10−6 | |||
2 | AE | 0.2072 | 0.2073 | 0.2063 | 0.2082 | 0.2024 | 0.2056 | ||
MSE | 0.0024 | 662*10−6 | 651*10−6 | 676*10−6 | 652*10−6 | 654*10−6 | |||
3 | AE | 0.2077 | 0.2066 | 0.2057 | 0.2076 | 0.2020 | 0.2051 | ||
MSE | 0.0019 | 642*10−6 | 632*10−6 | 654*10−6 | 639*10−6 | 636*10−6 | |||
(60, 40, 30) | 1 | AE | 0.2063 | 0.2072 | 0.2062 | 0.2082 | 0.2022 | 0.2056 | |
MSE | 0.0025 | 648*10−6 | 636*10−6 | 662*10−6 | 638*10−6 | 639*10−6 | |||
2 | AE | 0.2065 | 0.2070 | 0.2060 | 0.2080 | 0.2021 | 0.2054 | ||
MSE | 0.0023 | 650*10−6 | 639*10−6 | 663*10−6 | 642*10−6 | 642*10−6 | |||
3 | AE | 0.2074 | 0.2065 | 0.2055 | 0.2074 | 0.2019 | 0.2050 | ||
MSE | 0.0019 | 645*10−6 | 636*10−6 | 657*10−6 | 644*10−6 | 640*10−6 | |||
(60, 50, 25) | 1 | AE | 0.2047 | 0.2062 | 0.2053 | 0.2071 | 0.2019 | 0.2048 | |
MSE | 0.0021 | 659*10−6 | 651*10−6 | 671*10−6 | 657*10−6 | 654*10−6 | |||
2 | AE | 0.2065 | 0.2072 | 0.2063 | 0.2081 | 0.2028 | 0.2057 | ||
MSE | 0.0021 | 656*10−6 | 645*10−6 | 668*10−6 | 645*10−6 | 648*10−6 | |||
3 | AE | 0.2059 | 0.2062 | 0.2054 | 0.2071 | 0.2021 | 0.2049 | ||
MSE | 0.0017 | 641*10−6 | 632*10−6 | 651*10−6 | 638*10−6 | 636*10−6 | |||
(60, 50, 30) | 1 | AE | 0.2065 | 0.2073 | 0.2064 | 0.2082 | 0.2029 | 0.2058 | |
MSE | 0.0021 | 655*10−6 | 644*10−6 | 667*10−6 | 642*10−6 | 646*10−6 | |||
2 | AE | 0.2055 | 0.2066 | 0.2057 | 0.2075 | 0.2023 | 0.2052 | ||
MSE | 0.0020 | 640*10−6 | 631*10−6 | 652*10−6 | 634*10−6 | 634*10−6 | |||
3 | AE | 0.2061 | 0.2063 | 0.2055 | 0.2072 | 0.2022 | 0.2049 | ||
MSE | 0.0017 | 632*10−6 | 624*10−6 | 642*10−6 | 629*10−6 | 627*10−6 |
t | (n,m,k) | Scheme | ˆℏML | ˆℏBS | ˆℏBL | ˆℏBL | ˆℏBG | ˆℏBG | |
(h=1.5) | (h=−1.5) | (c=0.5) | (c=−0.5) | ||||||
0.1 | (60, 40, 25) | 1 | AE | 3.9493 | 3.8496 | 3.7028 | 4.0153 | 3.8095 | 3.8362 |
MSE | 0.5200 | 0.0999 | 0.1028 | 0.1507 | 0.09823 | 0.09896 | |||
2 | AE | 3.9209 | 3.8441 | 3.6997 | 4.0068 | 3.8048 | 3.8310 | ||
MSE | 0.4718 | 0.0998 | 0.1036 | 0.1477 | 0.0985 | 0.0990 | |||
3 | AE | 3.894 | 3.8490 | 3.7112 | 4.0029 | 3.8116 | 3.8365 | ||
MSE | 0.3638 | 0.0985 | 0.0994 | 0.1446 | 0.0966 | 0.0975 | |||
(60, 40, 30) | 1 | AE | 3.9388 | 3.8450 | 3.6985 | 4.0103 | 3.8050 | 3.8316 | |
MSE | 0.5054 | 0.0972 | 0.1018 | 0.1458 | 0.0960 | 0.0964 | |||
2 | AE | 3.9294 | 3.8472 | 3.7026 | 4.0101 | 3.8078 | 3.8341 | ||
MSE | 0.4694 | 0.0987 | 0.1019 | 0.1475 | 0.0972 | 0.0978 | |||
3 | AE | 3.8991 | 3.8513 | 3.7134 | 4.0054 | 3.8139 | 3.8388 | ||
MSE | 0.3717 | 0.1001 | 0.1001 | 0.1473 | 0.0981 | 0.0991 | |||
(60, 50, 25) | 1 | AE | 3.9448 | 3.8529 | 3.7210 | 3.9997 | 3.8172 | 3.8410 | |
MSE | 0.4297 | 0.1014 | 0.01011 | 0.1444 | 0.0996 | 0.1005 | |||
2 | AE | 3.9165 | 3.8407 | 3.7099 | 3.9861 | 3.8051 | 3.8288 | ||
MSE | 0.4039 | 0.0985 | 0.1015 | 0.1370 | 0.0976 | 0.0978 | |||
3 | AE | 3.9131 | 3.8502 | 3.7248 | 3.9889 | 3.8166 | 3.8389 | ||
MSE | 0.3468 | 0.0993 | 0.0984 | 0.1387 | 0.0975 | 0.0984 | |||
(60, 50, 30) | 1 | AE | 3.9179 | 3.8396 | 3.7085 | 3.9855 | 3.8040 | 3.8277 | |
MSE | 0.4118 | 0.0985 | 0.1019 | 0.1370 | 0.0977 | 0.0980 | |||
2 | AE | 3.9284 | 3.8472 | 3.7161 | 3.9931 | 3.8117 | 3.8354 | ||
MSE | 0.4006 | 0.0974 | 0.0989 | 0.1379 | 0.0961 | 0.0967 | |||
3 | AE | 3.9102 | 3.8490 | 3.7237 | 3.9875 | 3.8151 | 3.8377 | ||
MSE | 0.3435 | 0.0980 | 0.0976 | 0.1369 | 0.0964 | 0.0972 | |||
0.5 | (60, 40, 25) | 1 | AE | 2.4150 | 2.3035 | 2.2597 | 2.3499 | 2.2841 | 2.2971 |
MSE | 0.0613 | 0.02778 | 0.0274 | 0.0325 | 0.0276 | 0.0276 | |||
2 | AE | 2.2319 | 2.3007 | 2.2573 | 2.3465 | 2.2814 | 2.2943 | ||
MSE | 0.0579 | 0.0276 | 0.0275 | 0.0320 | 0.0275 | 0.0275 | |||
3 | AE | 2.2257 | 2.3039 | 2.2619 | 2.3482 | 2.2853 | 2.2977 | ||
MSE | 0.0452 | 0.0270 | 0.0264 | 0.0315 | 0.0267 | 0.0268 | |||
(60, 40, 30) | 1 | AE | 2.2385 | 2.3012 | 2.2574 | 2.3474 | 2.2818 | 2.2947 | |
MSE | 0.0609 | 0.0271 | 0.0270 | 0.0315 | 0.0270 | 0.0270 | |||
2 | AE | 2.2353 | 2.3022 | 2.2588 | 2.3481 | 2.2830 | 2.2958 | ||
MSE | 0.0569 | 0.0273 | 0.0270 | 0.03178 | 0.0271 | 0.0272 | |||
3 | AE | 2.2273 | 2.3051 | 2.2630 | 2.3494 | 2.2865 | 2.2989 | ||
MSE | 0.0454 | 0.0273 | 0.0267 | 0.0320 | 0.0270 | 0.0271 | |||
(60, 50, 25) | 1 | AE | 2.2445 | 2.3059 | 2.2664 | 2.3472 | 2.2884 | 2.3001 | |
MSE | 0.0514 | 0.0281 | 0.0275 | 0.0323 | 0.0279 | 0.0280 | |||
2 | AE | 2.2357 | 2.2996 | 2.2604 | 2.3407 | 2.2822 | 2.2938 | ||
MSE | 0.0508 | 0.0274 | 0.0273 | 0.0310 | 0.02736 | 0.2733 | |||
3 | AE | 2.2353 | 2.3048 | 2.2669 | 2.3446 | 2.2881 | 2.2993 | ||
MSE | 0.0424 | 0.0273 | 0.0266 | 0.0313 | 0.0270 | 0.0272 | |||
(60, 50, 30) | 1 | AE | 2.2352 | 2.2988 | 2.2596 | 2.3399 | 2.2814 | 2.2930 | |
MSE | 0.0511 | 0.0274 | 0.0273 | 0.0391 | 0.0274 | 0.0273 | |||
2 | AE | 2.240 | 2.3031 | 2.2638 | 2.3443 | 2.2857 | 2.293 | ||
MSE | 0.0490 | 0.0271 | 0.0267 | 0.0309 | 0.0269 | 0.0270 | |||
3 | AE | 2.2344 | 2.3042 | 2.2663 | 2.3439 | 2.2875 | 2.2987 | ||
MSE | 0.0422 | 0.0270 | 0.0263 | 0.0309 | 0.0267 | 0.0269 |
m | k | Scheme | ACI | Boot-p | Gamma prior | |||
Boot-p | Boot-t | BCI | HPD | |||||
40 | 25 | 1 | α1 | 1.397(0.9959) | 0.429(0.9300) | 0.747(0.8784) | 0.518(0.9912) | 0.506(0.9858) |
α2 | 2.873(0.9995) | 0.440(0.9322) | 0.732(0.8796) | 0.6022(0.9983) | 0.593(0.9971) | |||
β | 5.322(1.0000) | 1.146(0.9446) | 1.473(0.9846) | 0.776(1.0000) | 0.759(1.0000) | |||
2 | α1 | 1.846(0.9980) | 0.421(0.9371) | 0.668(0.8726) | 0.515(0.9930) | 0.504(0.9885) | ||
α2 | 2.717(0.9999) | 0.430(0.9375) | 0.650(0.8786) | 0.601(0.9990) | 0.592(0.9978) | |||
β | 4.891(0.9999) | 1.081(0.9407) | 1.270(0.9654) | 0.768(1.0000) | 0.752(1.0000) | |||
3 | α1 | 1.857(0.9987) | 0.369(0.9393) | 0.579(0.8798) | 0.480(0.9913) | 0.470(0.9870) | ||
α2 | 2.760(0.9999) | 0.376(0.9413) | 0.570(0.8879) | 0.572(0.9986) | 0.563(0.9972) | |||
β | 5.143(1.0000) | 0.939(0.9389) | 1.202(0.9511) | 0.760(1.0000) | 0.743(1.0000) | |||
30 | 1 | α1 | 1.818(0.9962) | 0.414(0.9328) | 0.774(0.8922) | 0.510(0.9914) | 0.499(0.9860) | |
α2 | 2.695(0.9996) | 0.427(0.9365) | 0.789(0.9088) | 0.594(0.9978) | 0.586(0.9963) | |||
β | 5.145(0.9997) | 1.1435(0.9446) | 1.700(0.9626) | 0.775(1.0000) | 0.758(1.0000) | |||
2 | α1 | 1.839(0.9968) | 0.410(0.9291) | 0.726(0.8944) | 0.512(0.992) | 0.500(0.9882) | ||
α2 | 2.688(0.9999) | 0.421(0.9318) | 0.729(0.9079) | 0.597(0.9987) | 0.588(0.9974) | |||
β | 4.926(0.9999) | 1.078(0.9460) | 1.466(0.9733) | 0.768(1.0000) | 0.751(1.000) | |||
3 | α1 | 1.892(0.9992) | 0.370(0.9386) | 0.588(0.8815) | 0.480(0.9910) | 0.470(0.9870) | ||
α2 | 2.793(1.0000) | 0.376(0.9376) | 0.578(0.8830) | 0.571(0.9985) | 0.563(0.9975) | |||
β | 5.221(1.0000) | 0.941(0.9409) | 1.221(0.9536) | 0.760(1.0000) | 0.743(1.0000) | |||
50 | 25 | 1 | α1 | 1.619(0.9981) | 0.391(0.9363) | 0.581(0.8675) | 0.489(0.9890) | 0.479(0.9856) |
α2 | 2.394(0.9995) | 0.400(0.9385) | 0.571(0.8754) | 0.577(0.9983) | 0.569(0.9973) | |||
β | 4.464(1.0000) | 1.020(0.9438) | 1.185(0.9541) | 0.767(1.0000) | 0.751(1.0000) | |||
2 | α1 | 1.628(0.9976) | 0.389(0.9340) | 0.582(0.8676) | 0.488(0.9894) | 0.478(0.856) | ||
α2 | 2.395(1.0000) | 0.398(0.9361) | 0.572(0.8740) | 0.577(0.9978) | 0.568(0.9955) | |||
β | 4.424(1.0000) | 1.007(0.9430) | 1.171(0.9534) | 0.765(1.0000) | 0.749(1.0000) | |||
3 | α1 | 1.449(0.9979) | 0.358(0.9359) | 0.505(0.8711) | 0.466(0.9902) | 0.456(0.9868) | ||
α2 | 2.144(1.0000) | 0.367(0.9360) | 0.501(0.8799) | 0.557(0.9986) | 0.549(0.9971) | |||
β | 4.009(1.0000) | 0.923(0.9399) | 1.051(0.9533) | 0.759(1.0000) | 0.742(1.0000) | |||
30 | 1 | α1 | 1.644(0.9982) | 0.389(0.9387) | 0.594(0.8773) | 0.489(0.9920) | 0.478(0.9866) | |
α2 | 2.400(1.0000) | 0.398(0.9385) | 0.587(0.8849) | 0.578(0.9977) | 0.569(0.9967) | |||
β | 4.544(1.0000) | 1.020(0.9456) | 1.220(0.9776) | 0.768(1.0000) | 0.751(1.0000) | |||
2 | α1 | 1.612(0.9979) | 0.387(0.9337) | 0.583(0.8728) | 0.489(0.9921) | 0.479(0.9876) | ||
α2 | 2.384(0.9998) | 0.397(0.9356) | 0.576(0.8803) | 0.577(0.9982) | 0.569(0.9967) | |||
β | 4.411(1.0000) | 1.01(0.9437) | 1.177(0.9727) | 0.766(1.0000) | 0.749(1.0000) | |||
3 | α1 | 1.451(0.9984) | 0.358(0.9365) | 0.503(0.8755) | 0.465(0.9908) | 0.455(0.9860) | ||
α2 | 2.124(0.9998) | 0.367(0.9390) | 0.499(0.8738) | 0.557(0.9986) | 0.549(0.9970) | |||
β | 3.983(1.0000) | 0.922(0.9409) | 1.042(0.9564) | 0.759(1.0000) | 0.743(1.0000) |
m | k | Scheme | ACI | Boot-p | Gamma prior | |||
Boot-p | Boot-t | BCI | HPD | |||||
40 | 20 | 1 | ℜ(0.1) | 0.0022(0.9850) | 0.0014(0.9532) | 0.2626(1.0000) | 0.00092(0.986) | 0.00090(0.9846) |
ℜ(0.5) | 0.1847(0.9822) | 0.1266(0.9531) | 0.2838(0.9999) | 0.08888(0.9842) | 0.08808(0.9824) | |||
ℏ(0.1) | 0.0654(0.9850) | 0.0419(0.9532) | 0.0510(0.9274) | 0.0275(0.9860) | 0.0271(0.9846) | |||
ℏ(0.5) | 1.0230(0.9758) | 0.7854(0.9537) | 0.9281(0.9389) | 0.5683(0.9832) | 0.5620(0.9804) | |||
2 | ℜ(0.1) | 0.00194(0.9815) | 0.0013(0.9494) | 0.2622(1.0000) | 0.00088(0.9827) | 0.00087(0.9808) | ||
ℜ(0.5) | 0.1635(0.9760) | 0.1198(0.9497) | 0.2862(0.9999) | 0.0861(0.9803) | 0.0854(0.9787) | |||
ℏ(0.1) | 0.0583(0.9815) | 0.0395(0.9494) | 0.0444(0.9246) | 0.0266(0.9827) | 0.0262(0.9806) | |||
ℏ(0.5) | 0.8977(0.9641) | 0.7428(0.9502) | 0.8107(0.9355) | 0.5504(0.9795) | 0.5547(0.9774) | |||
3 | ℜ(0.1) | 0.0020(0.9836) | 0.0012(0.9441) | 0.2108(1.0000) | 0.00081(0.9786) | 0.00080(0.9758) | ||
ℜ(0.5) | 0.1651(0.9810) | 0.1058(0..9944) | 0.2329(0.9998) | 0.0786(0.9766) | 0.07803(0.9727) | |||
ℏ(0.1) | 0.0599(0.6836) | 0.0345(0.9441) | 0.0413(0.9086) | 0.0243(0.9786) | 0.0240(0.9757) | |||
ℏ(0.5) | 0.8805(0.9692) | 0.6555(0.9442) | 0.7536(0.9231) | 0.5012(0.9750) | 0.4966(0.9700) | |||
30 | 1 | ℜ(0.1) | 0.00226(0.9776) | 0.0014(0.9522) | 0.2685(1.0000) | 0.00091(0.9842) | 0.00090(0.9813) | |
ℜ(0.5) | 0.1911(0.9745) | 0.1273(0.9526) | 0.2858(1.0000) | 0.0881(0.9829) | 0.0873(0.9793) | |||
ℏ(0.1) | 0.0676(0.9776) | 0.0422(0.9522) | 0.0534(0.9334) | 0.0272(0.9843) | 0.0269(0.9814) | |||
ℏ(0.5) | 1.0587(0.9674) | 0.7911(0.9531) | 0.9533(0.9390) | 0.5629(0.9803) | 0.5568(0.9770) | |||
2 | ℜ(0.1) | 0.0202(0.9816) | 0.0013(0.9534) | 0.2659(1.0000) | 0.00088(0.9839) | 0.00087(0.9825) | ||
ℜ(0.5) | 0.1694(0.9787) | 0.1200(0.9538) | 0.2864(0.9999) | 0.0859(0.9823) | 0.0852(0.9807) | |||
ℏ(0.1) | 0.0606(0.9816) | 0.0396(0.9534) | 0.0462(0.9452) | 0.0265(0.9839) | 0.0262(0.9824) | |||
ℏ(0.5) | 0.9274(0.9711) | 0.7448(0.9541) | 0.8259(0.9475) | 0.5494(0.9811) | 0.5438(0.9784) | |||
3 | ℜ(0.1) | 0.0020(0.9821) | 0.0012(0.9457) | 0.2105(1.0000) | 0.00081(0.9782) | 0.00080(0.9762) | ||
ℜ(0.5) | 0.1655(0.9783) | 0.1060(0.9458) | 0.2325(0.9998) | 0.0787(0.9767) | 0.0781(0.9749) | |||
ℏ(0.1) | 0.0599(0.9821) | 0.0346(0.9457) | 0.0418(0.9099) | 0.0243(0.9782) | 0.0240(0.9760) | |||
ℏ(0.5) | 0.8836(0.9688) | 0.6567(0.9469) | 0.7617(0.9259) | 0.5015(0.9750) | 0.4970(0.9728) | |||
50 | 20 | 1 | ℜ(0.1) | 0.00212(0.9834) | 0.0012(0.9475) | 0.2359(1.0000) | 0.00085(0.9810) | 0.00084(0.9802) |
ℜ(0.5) | 0.1800(0.9786) | 0.1145(0.9478) | 0.2558(0.9999) | 0.0822(0.9794) | 0.0816(0.9773) | |||
ℏ(0.1) | 0.00634(0.9833) | 0.0374(0.9475) | 0.0431(0.9198) | 0.0254(0.9809) | 0.0251(0.9802) | |||
ℏ(0.5) | 0.9915(0.9713) | 0.7097(0.9475) | 0.8001(0.9300) | 0.5242(0.9782) | 0.5191(0.9764) | |||
2 | ℜ(0.1) | 0.00191(0.9841) | 0.0012(0.9478) | 0.2373(1.0000) | 0.00084(0.9846) | 0.00083(0.9810) | ||
ℜ(0.5) | 0.1624(0.9807) | 0.1130(0.9477) | 0.2576(0.9998) | 0.0818(0.9830) | 0.0811(0.9797) | |||
ℏ(0.1) | 0.0057(0.9841) | 0.0369(0.9478) | 0.0425(0.9176) | 0.0253(0.9845) | 0.0250(0.9809) | |||
ℏ(0.5) | 0.8979(0.9705) | 0.7006(0.9476) | 0.7885(0.9296) | 0.5213(0.9802) | 0.5162(0.9770) | |||
3 | ℜ(0.1) | 0.00174(0.9833) | 0.0011(0.9438) | 0.2133(1.0000) | 0.00079(0.9799) | 0.00078(0.9770) | ||
ℜ(0.5) | 0.1491(0.9792) | 0.1046(0.9437) | 0.2315(0.9996) | 0.0769(0.9772) | 0.0764(0.9743) | |||
ℏ(0.1) | 0.00522(0.9833) | 0.03 40(0.9437) | 0.0383(0.9219) | 0.0238(0.9800) | 0.0235(0.9770) | |||
ℏ(0.5) | 0.8271(0.9704) | 0.6479(0.9442) | 0.7176(0.9333) | 0.4891(0.9748) | 0.4847(0.9727) | |||
30 | 1 | ℜ(0.1) | 0.00194(0.9871) | 0.0012(0.9500) | 0.2326(1.0000) | 0.00085(0.9824) | 0.0008(0.9810) | |
ℜ(0.5) | 0.1647(0.9836) | 0.1143(0.9503) | 0.2523(1.0000) | 0.0823(0.9809) | 0.0816(0.9783) | |||
ℏ(0.1) | 0.0058(0.9871) | 0.0375(0.9500) | 0.0435(0.9255) | 0.0254(0.9825) | 0.0251(0.9806) | |||
ℏ(0.5) | 0.9127(0.9751) | 0.7085(0.9504) | 0.8013(0.9346) | 0.5247(0.9793) | 0.5195(0.9764) | |||
2 | ℜ(0.1) | 0.00188(0.9874) | 0.0012(0.9488) | 0.2342(1.0000) | 0.00084(0.9823) | 0.00083(0.9786) | ||
ℜ(0.5) | 0.1598(0.9838) | 0.1128(0.9490) | 0.2543(0.9997) | 0.0818(0.9799) | 0.0811(0.9779) | |||
ℏ(0.1) | 0.0056(0.9874) | 0.0369(0.9488) | 0.0419(0.9219) | 0.0253(0.9822) | 0.0250(0.9786) | |||
ℏ(0.5) | 0.8855(0.9737) | 0.6994(0.9490) | 0.7768(0.9334) | 0.5213(0.9789) | 0.5161(0.9764) | |||
3 | ℜ(0.1) | 0.00176(0.9851) | 0.0113(0.9472) | 0.2129(1.0000) | 0.00079(0.9820) | 0.00078(0.9806) | ||
ℜ(0.5) | 0.1506(0.9824) | 0.1044(0.9473) | 0.2312(0.9999) | 0.0771(0.9800) | 0.0765(0.9783) | |||
ℏ(0.1) | 0.0528(0.9851) | 0.0340(0.9472) | 0.0380(0.9230) | 0.0239(0.9820) | 0.0236(0.9806) | |||
ℏ(0.5) | 0.8350(0.9735) | 0.6468(0.9472) | 0.7132(0.9331) | 0.4903(0.9785) | 0.4857(0.9758) |
τ=1.2 | ACI | Boot-p | Gamma prior | ||||||||||||||
Scheme | Boot-p | Boot-t | BCI | HPD | |||||||||||||
(m,k) | α1 | α2 | β | α1 | α2 | β | α1 | α2 | β | α1 | α2 | β | α1 | α2 | β | ||
(40, 25) | 1 | AW | 2.686 | 4.883 | 8.725 | 0.684 | 0.709 | 1.861 | 1.050 | 1.032 | 2.323 | 0.627 | 0.722 | 0.812 | 0.620 | 0.718 | 0.799 |
CP | 0.9962 | 0.9998 | 1.0000 | 0.9374 | 0.9388 | 0.9452 | 0.8704 | 0.8798 | 0.9502 | 0.9968 | 0.9995 | 1.0000 | 0.9954 | 0.9995 | 1.0000 | ||
2 | AW | 2.835 | 5.179 | 8.895 | 0.680 | 0.704 | 1.785 | 1.088 | 1.072 | 2.316 | 0.627 | 0.722 | 0.808 | 0.619 | 0.717 | 0.795 | |
CP | 0.9968 | 1.0000 | 1.0000 | 0.9418 | 0.9438 | 0.9444 | 0.8802 | 0.8896 | 0.9488 | 0.9958 | 0.9998 | 1.0000 | 0.9938 | 0.9995 | 1.0000 | ||
3 | AW | 5.360 | 10.057 | 16.591 | 0.658 | 0.663 | 1.618 | 1.111 | 1.026 | 2.192 | 0.622 | 0.721 | 0.800 | 0.614 | 0.716 | 0.783 | |
CP | 0.9998 | 1.0000 | 1.0000 | 0.9416 | 0.9412 | 0.9422 | 0.8930 | 0.9104 | 0.9506 | 0.9951 | 0.9998 | 1.0000 | 0.9944 | 0.9998 | 1.0000 | ||
(40, 30) | 1 | AW | 2.657 | 4.871 | 8.665 | 0.684 | 0.708 | 1.860 | 1.046 | 1.026 | 2.307 | 0.627 | 0.722 | 0.812 | 0.619 | 0.717 | 0.799 |
CP | 0.9964 | 1.0000 | 1.0000 | 0.9330 | 0.9402 | 0.9438 | 0.8742 | 0.8860 | 0.9492 | 0.99665 | 0.9998 | 1.0000 | 0.9956 | 0.9995 | 1.0000 | ||
2 | AW | 2.827 | 5.199 | 8.986 | 0.679 | 0.703 | 1.796 | 1.093 | 1.077 | 2.345 | 0.626 | 0.723 | 0.808 | 0.618 | 0.718 | 0.795 | |
CP | 0.9976 | 1.0000 | 1.0000 | 0.9346 | 0.9406 | 0.9450 | 0.8732 | 0.8924 | 0.9588 | 0.9948 | 0.9999 | 1.0000 | 0.9947 | 0.9998 | 1.0000 | ||
3 | AW | 5.408 | 10.001 | 16.559 | 0.659 | 0.664 | 1.621 | 1.113 | 1.031 | 2.190 | 0.622 | 0.721 | 0.799 | 0.614 | 0.716 | 0.783 | |
CP | 0.9994 | 1.0000 | 1.0000 | 0.9388 | 0.9426 | 0.9400 | 0.8946 | 0.9132 | 0.9458 | 0.9956 | 0.9999 | 1.0000 | 0.9935 | 0.9999 | 1.0000 | ||
(50, 25) | 1 | AW | 2.182 | 3.971 | 8.703 | 0.616 | 0.639 | 1.642 | 0.933 | 0.932 | 2.101 | 0.596 | 0.700 | 0.804 | 0.589 | 0.695 | 0.791 |
CP | 0.9958 | 1.0000 | 1.000 | 0.9416 | 0.9374 | 0.9528 | 0.8904 | 0.8946 | 0.9564 | 0.9947 | 0.9994 | 1.0000 | 0.9929 | 0.9989 | 1.0000 | ||
2 | AW | 2.209 | 4.016 | 8.640 | 0.615 | 0.638 | 1.627 | 0.870 | 0.866 | 1.934 | 0.595 | 0.698 | 0.802 | 0.588 | 0.694 | 0.790 | |
CP | 0.9952 | 1.0000 | 1.0000 | 0.9404 | 0.9410 | 0.9488 | 0.8830 | 0.8932 | 0.9542 | 0.9953 | 0.9998 | 1.0000 | 0.9931 | 0.9996 | 1.0000 | ||
3 | AW | 2.809 | 5.189 | 10.893 | 0.595 | 0.608 | 1.512 | 0.922 | 0.900 | 1.983 | 0.589 | 0.695 | 0.796 | 0.582 | 0.691 | 0.783 | |
CP | 0.9976 | 1.0000 | 1.0000 | 0.9490 | 0.9468 | 0.9518 | 0.9042 | 0.9094 | 0.9538 | 0.9941 | 0.9996 | 1.0000 | 0.9919 | 0.9995 | 1.0000 | ||
(50, 30) | 1 | AW | 2.195 | 4.008 | 7.847 | 0.616 | 0.639 | 1.640 | 0.909 | 0.905 | 2.041 | 0.594 | 0.699 | 0.804 | 0.588 | 0.694 | 0.791 |
CP | 0.9957 | 1.000 | 1.000 | 0.938 | 0.939 | 0.956 | 0.884 | 0.888 | 0.952 | 0.9942 | 0.9994 | 1.0000 | 0.9923 | 0.9991 | 1.0000 | ||
2 | AW | 2.196 | 3.957 | 8.589 | 0.616 | 0.638 | 1.633 | 0.894 | 0.891 | 2.009 | 0.596 | 0.699 | 0.803 | 0.589 | 0.694 | 0.790 | |
CP | 0.9957 | 1.0000 | 1.0000 | 0.9478 | 0.9458 | 0.9440 | 0.8900 | 0.8928 | 0.9486 | 0.9952 | 1.0000 | 1.0000 | 0.9935 | 1.0000 | 1.0000 | ||
3 | AW | 2.812 | 5.191 | 10.943 | 0.595 | 0.607 | 1.527 | 0.913 | 0.891 | 1.992 | 0.589 | 0.695 | 0.797 | 0.582 | 0.691 | 0.783 | |
CP | 0.9979 | 1.0000 | 1.0000 | 0.9420 | 0.9446 | 0.9440 | 0.8946 | 0.9096 | 0.9546 | 0.9939 | 0.9996 | 1.0000 | 0.9909 | 0.9996 | 1.0000 |
n | m | k | Scheme | ACI | Boot-p | Gamma prior | |||
Boot-p | Boot-t | BCI | HPD | ||||||
60 | 40 | 25 | 1 | ℜ(0.1) | 0.296(0.9735) | 0.216(0.9516) | 0.268(0.9806) | 0.131(0.9956) | 0.131(0.9945) |
ℜ(0.5) | 0.215(0.9642) | 0.198(0.9506) | 0.284(0.9958) | 0.143(0.9953) | 0.142(0.9950) | ||||
ℏ(0.1) | 3.049(0.9569) | 2.807(0.9518) | 2.923(0.9356) | 1.777(0.9954) | 1.765(0.9936) | ||||
ℏ(0.5) | 3.083(0.9956) | 0.994(0.9498) | 1.304(0.8706) | 0.957(0.9959) | 0.952(0.9949) | ||||
2 | ℜ(0.1) | 0.259(0.9682) | 0.208(0.9498) | 0.276(0.9840) | 0.130(0.9929) | 0.130(0.9916) | |||
ℜ(0.5) | 0.223(0.9737) | 0.190(0.9498) | 0.267(0.9920) | 0.142(0.9925) | 0.141(0.9921) | ||||
ℏ(0.1) | 2.758(0.9559) | 2.702(0.9494) | 2.723(0.9384) | 1.761(0.9925) | 1.750(0.9912) | ||||
ℏ(0.5) | 3.141(0.9962) | 0.959(0.9484) | 1.325(0.8786) | 0.952(0.9943) | 0.947(0.9922) | ||||
3 | ℜ(0.1) | 0.252(0.9771) | 0.188(0.9456) | 0.251(0.9838) | 0.127(0.9941) | 0.126(0.9932) | |||
ℜ(0.5) | 0.356(0.9941) | 0.169(0.947) | 0.177(0.9446) | 0.138(0.9942) | 0.137(0.9934) | ||||
ℏ(0.1) | 2.618(0.9717) | 2.436(0.9454) | 2.267(0.9562) | 1.716(0.9941) | 1.706(0.9925) | ||||
ℏ(0.5) | 6.124(0.9997) | 0.840(0.9470) | 1.330(0.8970) | 0.936(0.9955) | 0.931(0.9943) | ||||
30 | 1 | ℜ(0.1) | 0.286(0.9755) | 0.216(0.9460) | 0.267(0.9798) | 0.131(0.9951) | 0.131(0.9945) | ||
ℜ(0.5) | 0.212(0.9683) | 0.198(0.9468) | 0.283(0.9932) | 0.143(0.9947) | 0.142(0.9946) | ||||
ℏ(0.1) | 3.008(0.9610) | 2.806(0.9456) | 2.913(0.9374) | 1.775(0.9947) | 1.763(0.9940) | ||||
ℏ(0.5) | 2.913(0.9956) | 0.994(0.9480) | 1.295(0.8764) | 0.956(0.9956) | 0.951(0.9942) | ||||
2 | ℜ(0.1) | 0.260(0.9686) | 0.208(0.9464) | 0.275(0.9864) | 0.130(0.9961) | 0.130(0.9953) | |||
ℜ(0.5) | 0.224(0.9770) | 0.190(0.9470) | 0.266(0.9936) | 0.142(0.9959) | 0.141(0.9955) | ||||
ℏ(0.1) | 2.764(0.9578) | 2.713(0.9462) | 2.732(0.9470) | 1.762(0.9961) | 1.751(0.9944) | ||||
ℏ(0.5) | 3.176(0.9975) | 0.959(0.9480) | 1.334(0.8810) | 0.953(0.9966) | 0.947(0.9955) | ||||
3 | ℜ(0.1) | 0.252(0.9734) | 0.188(0.9442) | 0.252(0.9834) | 0.127(0.9928) | 0.126(0.9912) | |||
ℜ(0.5) | 0.358(0.9935) | 0.169(0.9478) | 0.177(0.9432) | 0.138(0.9928) | 0.137(0.9918) | ||||
ℏ(0.1) | 2.629(0.9714) | 2.440(0.9462) | 2.263(0.9564) | 1.717(0.9929) | 1.706(0.9913) | ||||
ℏ(0.5) | 6.180(0.9999) | 0.841(0.9500) | 1.332(0.8970) | 0.936(0.9946) | 0.931(0.9929) | ||||
50 | 25 | 1 | ℜ(0.1) | 0.258(0.9718) | 0.194(0.9558) | 0.238(0.9852) | 0.124(0.9920) | 0.124(0.9905) | |
ℜ(0.5) | 0.189(0.9611) | 0.178(0.9568) | 0.257(0.9962) | 0.135(0.9909) | 0.134(0.9897) | ||||
ℏ(0.1) | 2.705(0.9553) | 2.515(0.9548) | 2.661(0.9480) | 1.679(0.9915) | 1.669(0.9903) | ||||
ℏ(0.5) | 2.590(0.9947) | 0.899(0.9580) | 1.181(0.8898) | 0.906(0.9924) | 0.9015(0.9915) | ||||
2 | ℜ(0.1) | 0.253(0.9745) | 0.192(0.9532) | 0.239(0.9826) | 0.124(0.9930) | 0.123(0.992) | |||
ℜ(0.5) | 0.191(0.9660) | 0.177(0.9522) | 0.254(0.9962) | 0.135(0.9923) | 0.134(0.9915) | ||||
ℏ(0.1) | 2.661(0.9574) | 2.494(0.9526) | 2.556(0.9434) | 1.671(0.9923) | 1.661(0.9914) | ||||
ℏ(0.5) | 2.613(0.9965) | 0.892(0.9520) | 1.102(0.887) | 0.903(0.9935) | 0.898(0.9925) | ||||
3 | ℜ(0.1) | 0.246(0.9780) | 0.179(0.9532) | 0.222(0.9814) | 0.121(0.9897) | 0.120(0.9891) | |||
ℜ(0.5) | 0.212(0.9803) | 0.163(0.9532) | 0.213(0.9910) | 0.131(0.9896) | 0.130(0.9881) | ||||
ℏ(0.1) | 2.417(0.9634) | 2.315(0.9532) | 2.283(0.9572) | 1.634(0.9896) | 1.624(0.9892) | ||||
ℏ(0.5) | 3.486(0.9974) | 0.817(0.9520) | 1.206(0.8836) | 0.888(0.9918) | 0.884(0.9909) | ||||
30 | 1 | ℜ(0.1) | 0.259(0.9744) | 0.194(0.9530) | 0.238(0.9836) | 0.124(0.9925) | 0.123(0.9913) | ||
ℜ(0.5) | 0.190(0.9643) | 0.178(0.9532) | 0.257(0.9964) | 0.135(0.9917) | 0.134(0.9922) | ||||
ℏ(0.1) | 2.704(0.9590) | 2.512(0.9526) | 2.637(0.9448) | 1.674(0.9918) | 1.664(0.9898) | ||||
ℏ(0.5) | 2.593(0.9965) | 0.899(0.9572) | 1.154(0.8874) | 0.904(0.9930) | 0.899(0.9924) | ||||
2 | ℜ(0.1) | 0.252(0.9729) | 0.193(0.9462) | 0.240(0.9790) | 0.124(0.9929) | 0.123(0.9918) | |||
ℜ(0.5) | 0.190(0.9651) | .176(0.9472) | 0.254(0.9948) | 0.134(0.9919) | 0.134(0.9922) | ||||
ℏ(0.1) | 2.655(0.9590) | 2.501(0.9464) | 2.587(0.9402) | 1.674(0.9919) | 1.664(0.9908) | ||||
ℏ(0.5) | 2.600(0.9969) | 0.893(0.9472) | 1.134(0.8878) | 0.904(0.9931) | 0.900(0.9922) | ||||
3 | ℜ(0.1) | 0.246(0.9771) | 0.180(0.9474) | 0.222(0.9790) | 0.121(0.9924) | 0.120(0.9917) | |||
ℜ(0.5) | 0.213(0.9840) | 0.162(0.9478) | 0.213(0.9890) | 0.131(0.9915) | 0.130(0.9909) | ||||
ℏ(0.1) | 2.422(0.9651) | 2.328(0.9478) | 2.292(0.9580) | 1.633(0.9918) | 1.624(0.9909) | ||||
ℏ(0.5) | 3.503(0.9985) | 0.817(0.9494) | 1.198(0.8872) | 0.888(0.9933) | 0.883(0.9922) |
Cases | ML | Bayesian(uniform prior) | |||||
BS | BL | BL | BG | BG | |||
(h=1.5) | (h=−1.5) | (c=0.5) | (c=−0.5) | ||||
1 | ˆα1 | 0.1795(0.1053) | 0.2292(0.1774) | 0.2106(0.1784) | 0.2632(0.1806) | 0.1718(0.1864) | 0.2064(0.1788) |
ˆα2 | 0.2692(0.1500) | 0.3459(0.2598) | 0.3099(0.2623) | 0.4364(0.2751) | 0.2656(0.2719) | 0.3138(0.2617) | |
ˆβ | 0.4985(0.4210) | 0.6279(0.5469) | 0.4872(0.5647) | 1.2542(0.8314) | 0.3796(0.6006) | 0.5372(0.5543) | |
ˆℜ(0.5) | 0.9734(0.0102) | 0.9721(0.0106) | 0.9720(0.0106) | 0.9722(0.0106) | 0.9720(0.0106) | 0.9721(0.0106) | |
ˆℜ(1.5) | 0.6436(0.0583) | 0.6493(0.0566) | 0.6469(0.0567) | 0.6517(0.0567) | 0.6455(0.0568) | 0.6481(0.0567) | |
ˆℏ(0.5) | 0.1568(0.0575) | 0.1627(0.0576) | 0.1603(0.0577) | 0.1653(0.0577) | 0.1491(0.0592) | 0.1581(0.0578) | |
ˆℏ(1.5) | 0.5613(0.1492) | 0.5601(0.4213) | 0.5458(0.1407) | 0.5753(0.1409) | 0.5339(0.1425) | 0.5514(0.1403) | |
ˆπ1 | 0.4(0.0980) | 0.3990(0.0961) | 0.3921(0.0964) | 0.4060(0.0964) | 0.3806(0.0979) | 0.3931(0.0963) | |
ˆπ2 | 0.6(0.0980) | 0.6010(0.0961) | 0.5940(0.0964) | 0.6079(0.0964) | 0.5887(0.0970) | 0.5970(0.0962) | |
2 | ˆα1 | 0.1383(0.0553) | 0.1445(0.0624) | 0.1417(0.0625) | 0.1476(0.0625) | 0.1274(0.0647) | 0.1385(0.0627) |
ˆα2 | 0.2075(0.0754) | 0.2172(0.0856) | 0.2120(0.0857) | 0.2231(0.0858) | 0.1960(0.0881) | 0.2098(0.0859) | |
ˆβ | 0.7013(0.4397) | 0.8856(0.6072) | 0.7113(0.6317) | 1.6297(0.9603) | 0.6585(0.6482) | 0.8034(0.6127) | |
ˆℜ(0.5) | 0.9714(0.0105) | 0.9690(0.0117) | 0.9689(0.0117) | 0.9691(0.0117) | 0.9689(0.0117) | 0.9690(0.0117) | |
ˆℜ(1.5) | 0.6571(0.0558) | 0.6602(0.0548) | .6580(0.0548) | 0.6625(0.0548) | 0.6567(0.0549) | 0.6591(0.0548) | |
ˆℏ(0.5) | 0.1673(0.0587) | 0.1783(0.0625) | 0.1755(0.0626) | 0.1813(0.0626) | 0.1632(0.0643) | 0.1732(0.0627) | |
ˆℏ(1.5) | 0.4863(0.0990) | 0.4757(0.3124) | 0.4690(0.0959) | 0.4827(0.0959) | 0.4612(0.0967) | 0.4709(0.0958) | |
ˆπ1 | 0.4(0.0894) | 0.3993(0.0879) | 0.3935(0.0881) | 0.4051(0.0881) | 0.3841(0.0892) | 0.3943(0.0881) | |
ˆπ2 | 0.6(0.0894) | 0.6007(0.0879) | 0.5949(0.0881) | 0.6065(0.0881) | 0.5905(0.0885) | 0.5974(0.0880) | |
3 | ˆα1 | 0.2099(0.0795) | 0.2217(0.0914) | 0.2158(0.0916) | 0.2285(0.0917) | 0.1982(0.0944) | 0.2134(0.0918) |
ˆα2 | 0.3498(0.1196) | 0.3699(0.1404) | 0.3565(0.1411) | 0.3866(0.1414) | 0.3366(0.1443) | 0.3582(0.1409) | |
ˆβ | 0.3291(0.2020) | 0.4031(0.2896) | 0.3579(0.2931) | 0.5407(0.3206) | 0.3001(0.3073) | 0.3654(0.2920) | |
ˆℜ(0.5) | 0.9777(0.0079) | 0.9762(0.0091) | 0.9761(0.0091) | 0.9762(0.0091) | 0.9761(0.0091) | =0.9761(0.0091) | |
ˆℜ(1.5) | 0.658262(0.0609) | 0.6616(0.0591) | 0.6589(0.0591) | 0.6641(0.0591) | 0.6575(0.0592) | 0.6602(0.0591) | |
ˆℏ(0.5) | 0.1327(0.0464) | 0.1408(0.0513) | 0.1389(0.0514) | 0.1429(0.05138) | 0.1285(0.0528) | 0.1366(0.05151) | |
ˆℏ(1.5) | 0.5891(0.0935) | 0.5715(0.4406) | 0.5651(0.0933) | 0.5781(0.0934) | 0.5601(0.0938) | 0.5677(0.0932) | |
ˆπ1 | 0.375(0.0765) | 0.3751(0.0751) | 0.3709(0.0752) | 0.3794(0.0752) | 0.3634(0.0760) | 0.3713(0.0752) | |
ˆπ2 | 0.625(0.0765) | 0.6249(0.0751) | 0.6206(0.0752) | 0.6291(0.0752) | 0.6178(0.0754) | 0.6226(0.0751) |
Cases | ACI | Boot-p | Uniform prior | |||
Boot-p | Boot-t | BCI | HPD | |||
α1 | 1 | (0.1103, 0.2920) | (0.1876, 0.3355) | (0.1880, 0.2771) | (0.0641, 0.6904) | (0.0419, 0.5590) |
0.1817 | 0.1479 | 0.0891 | 0.6263 | 0.5171 | ||
2 | (0.0300, 0.2467) | (0.1396, 0.2392) | (0.1396, 0.2045) | (0.0595, 0.2999) | (0.0446, 0.2651) | |
0.2167 | 0.0996 | 0.0649 | 0.2405 | 0.2205 | ||
3 | (0.0540, 0.3658) | (0.2108, 0.3104) | (0.2108, 0.2815) | (0.0978, 0.4485) | (0.0794, 0.3995) | |
0.3112 | 0.0996 | 0.0707 | 0.3507 | 0.3201 | ||
α2 | 1 | (0.1528, 0.4744) | (0.2745, 0.3190) | (0.2749, 0.3165) | (0.1071, 1.0452) | (0.0689, 0.8282) |
0.3216 | 0.0445 | 0.0416 | 0.9381 | 0.7593 | ||
2 | (0.0598, 0.3552) | (0.2080, 0.3312) | (0.2080, 0.2932) | (0.0999, 0.4382) | (0.0798, 0.3859) | |
0.2955 | 0.1232 | 0.0852 | 0.3383 | 0.3061 | ||
3 | (0.1154, 0.5843) | (0.3508, 0.4483) | (0.3509, 0.4240) | (0.1181, 0.7192) | (0.1545, 0.6508) | |
0.4689 | 0.0975 | 0.0731 | 0.5383 | 0.4963 | ||
β | 1 | (0.1531, 1.5992) | (0.5314, 0.6447) | (0.5340, 0.6197) | (0.0794, 2.1151) | (0.0382, 1.6710) |
1.4460 | 0.1133 | 0.0857 | 2.0357 | 1.6327 | ||
2 | (0.2506, 1.9626) | (0.7107, 1.1594) | (0.7111, 1.0276) | (0.1973, 2.4641) | (0.1180, 2.0447) | |
1.7120 | 0.4487 | 0.3165 | 2.2668 | 1.9267 | ||
3 | (0.1651, 0.6563) | (0.3317, 0.5494) | (0.3317, 0.4834) | (0.0912, 1.0850) | (0.0535, 0.8972) | |
0.7918 | 0.2177 | 0.1517 | 0.9937 | 0.8437 | ||
ℜ(0.5) | 1 | (0.9535, 0.9933) | (0.9564, 0.9675) | (0.9560, 0.9648) | (0.9458, 0.9866) | (0.9515, 0.9892) |
0.0398 | 0.0111 | 0.0088 | 0.0408 | 0.0377 | ||
2 | (0.9507, 0.9920) | (0.9457, 0.9686) | (0.9438, 0.9683) | (0.9406, 0.9857) | (0.9454, 0.9879) | |
0.4125 | 0.0229 | 0.0245 | 0.0451 | 0.0425 | ||
3 | (0.9621, 0.9933) | (0.9601, 0.9766) | (0.9542, 0.9765) | (0.9550, 0.9888) | (0.9588, 0.9903) | |
0.0312 | 0.0165 | 0.0223 | 0.0339 | 0.0316 | ||
ℜ(1.5) | 1 | (0.5293, 0.7580) | (0.4986, 0.5916) | (0.4986, 0.5899) | (0.5355, 0.7552) | (0.5384, 0.7575) |
0.2287 | 0.0930 | 0.0913 | 0.2197 | 0.2191 | ||
2 | (0.5478, 0.7664) | (0.4970, 0.6376) | (0.4959, 0.6393) | (0.5481, 0.7634) | (0.5538, 0.7676) | |
0.2186 | 0.1406 | 0.1434 | 0.2154 | 0.2138 | ||
3 | (0.5388, 0.7777) | (0.5183, 0.6458) | (0.4863, 0.6464) | (0.54438, 0.7733) | (0.5465, 0.7752) | |
0.2388 | 0.1275 | 0.1601 | 0.2290 | 0.2287 | ||
ℏ(0.5) | 1 | (0.0441, 0.2696) | (0.1917, 0.2586) | (0.1872, 0.2171) | (0.8009, 0.3026) | (0.0655, 0.2749) |
0.2255 | 0.0669 | 0.0299 | 0.2225 | 0.2095 | ||
2 | (0.0521, 0.2824) | (0.1829, 0.3173) | (0.1826, 0.3055) | (0.0850, 0.3272) | (0.0727, 0.3033) | |
0.2303 | 0.1344 | 0.1229 | 0.2422 | 0.2306 | ||
3 | (0.0417, 0.2237) | (0.1392, 0.2381) | (0.1389, 0.2498) | (0.0669, 0.2616) | (0.0580, 0.2401) | |
0.1820 | 0.0989 | 0.1109 | 0.1947 | 0.1821 | ||
ℏ(1.5) | 1 | (0.2689, 0.6740) | (0.6344, 0.8545) | (0.6088, 1.0570) | (0.3206, 0.8656) | (0.0762, 0.2904) |
0.4051 | 0.2201 | 0.4482 | 0.5450 | 0.2142 | ||
2 | (0.2922, 0.6014) | (0.5138, 0.7627) | (0.5268, 0.7798) | (0.3047, 0.6761) | (0.0806, 0.3160) | |
0.3092 | 0.2489 | 0.2530 | 0.3714 | 0.2354 | ||
3 | (0.4059, 0.6801) | (0.6104, 0.8599) | (0.6074, 0.7769) | (0.4019, 0.7659) | (0.0658, 0.2558) | |
0.2742 | 0.2495 | 0.1695 | 0.3640 | 0.1900 | ||
π1 | 1 | (0.2080, 0.5920) | (0.3704, 0.5161) | (0.3670, 0.5423) | (0.2205, 0.5942) | (0.2154, 0.5879) |
0.3841 | 0.1457 | 0.1753 | 0.2908 | 0.3725 | ||
2 | (0.2247, 0.5753) | (0.3143, 0.5000) | (0.2898, 0.5398) | (0.2361, 0.5777) | (0.2273, 0.5677) | |
0.3506 | 0.1857 | 0.2500 | 0.3416 | 0.3404 | ||
3 | (0.2250, 0.5250) | (0.3500, 0.4500) | (0.3450, 0.4626) | (0.2353, 0.5261) | (0.2317, 0.5202) | |
0.3001 | 0.1000 | 0.1176 | 0.3736 | 0.2884 | ||
π2 | 1 | (0.4080, 0.7920) | (0.4839, 0.6296) | (0.4577, 0.6330) | (0.4058, 0.8794) | (0.4121, 0.7846) |
0.3841 | 0.1457 | 0.1753 | 0.2908 | 0.3725 | ||
2 | (0.4247, 0.7754) | (0.5000, 0.6857) | (0.4602, 0.7102) | (0.4219, 0.7639) | (0.4323, 0.7727) | |
0.3506 | 0.1857 | 0.2500 | 0.3420 | 0.3404 | ||
3 | (0.4750, 0.7750) | (0.55, 0.65) | (0.5374, 0.6550) | (0.4739, 0.7647) | (0.4798, 0.7683) | |
0.3001 | 0.1000 | 0.1176 | 0.3736 | 0.2884 |
Cases | ACI | Boot-p | Uniform prior | |||
Boot-p | Boot-t | BCI | HPD | |||
α1 | 1∗ | (0.0456, 0.0820) | (0.0619, 0.1506) | (0.0619, 0.1058) | (0.0163, 0.3172) | (0.0069, 0.2179) |
0.0364 | 0.0887 | 0.0439 | 0.3009 | 0.2110 | ||
2∗ | (0.0025, 0.1348) | (0.0697, 0.1277) | (0.0697, 0.1053) | (0.0245, 0.1804) | (0.0167, 0.1520) | |
0.1323 | 0.058 | 0.0356 | 0.1560 | 0.1353 | ||
3∗ | (0.1101, 0.6418) | (0.2677, 0.5379) | (0.2681, 0.4074) | (0.0755, 2.1628) | (0.0336, 1.6337) | |
0.5316 | 0.2702 | 0.1393 | 2.0873 | 1.6001 | ||
α2 | 1∗ | (0.0659, 0.1276) | (0.0923, 0.1731) | (0.0925, 0.1458) | (0.0292, 0.4916) | (0.0155, 0.3231) |
0.0617 | 0.0808 | 0.0533 | 0.4624 | 0.3077 | ||
2∗ | (0.0050, 0.1495) | (0.0775, 0.1713) | (0.0775, 0.1255) | (0.0285, 0.2011) | (0.0184, 0.1684) | |
0.1445 | 0.0956 | 0.048 | 0.1726 | 0.1500 | ||
3∗ | (0.1268, 0.9028) | (0.3407, 0.6385) | (0.3412, 0.4848) | (0.1029, 2.7150) | (0.0489, 2.0678) | |
0.776 | 0.2978 | 0.1436 | 2.6120 | 2.0189 | ||
β | 1∗ | (0.1191, 20.52) | (1.5804, 4.5806) | (1.5783, 2.9936) | (0.1183, 18.94) | (0.0257, 12.82) |
20.401 | 3.0002 | 1.4153 | 18.8205 | 12.80 | ||
2∗ | (0.1602, 17.6309) | (1.7368, 4.4798) | (1.7507, 2.8638) | (0.2671, 10.846) | (0.0912, 8.287) | |
17.4707 | 2.743 | 1.1131 | 10.579 | 8.1962 | ||
3∗ | (0.0488, 0.3839) | (0.1373, 0.3387) | (0.1376, 0.2714) | (0.0087, 1.0988) | (0.0051, 0.8157) | |
0.3351 | 0.2014 | 0.1338 | 1.0900 | 0.8105 | ||
ℜ(0.5) | 1∗ | (0.9431, 1.003) | (0.9276, 0.9647) | (0.9116, 0.9650) | (0.9159, 0.9914) | (0.9273, 0.9943) |
0.0599 | 0.0371 | 0.0534 | 0.0755 | 0.0670 | ||
2∗ | (0.9431, 1.0021) | (0.9231, 0.9673) | (0.9171, 0.9671) | (0.9264, 0.9904) | (0.9341, 0.9932) | |
0.059 | 0.0442 | 0.05 | 0.0640 | 0.0591 | ||
3∗ | (0.9774, 1.0022) | (0.9708, 0.9887) | (0.9519, 0.9883) | (0.9690, 09957) | (0.9739, 0.9967) | |
0.0249 | 0.0179 | 0.0364 | 0.0267 | 0.0228 | ||
ℜ(1.5) | 1∗ | (0.6470, 0.8635) | (0.5546, 0.7127) | (0.3798, 0.6750) | (0.6459, 0.8577) | (0.6562, 0.8638) |
0.2165 | 0.1581 | 0.2952 | 0.2118 | 0.2076 | ||
2∗ | (0.6540, 0.8623) | (0.5590, 0.7299) | (0.5688, 0.7302) | (0.6537, 0.8580) | (0.6631, 0.8660) | |
0.2083 | 0.1709 | 0.1614 | 0.2043 | 0.2028 | ||
3∗ | (0.6460, 0.9440) | (0.5743, 0.7764) | (0.2904, 0.7625) | (0.6607, 0.8934) | (0.6749, 0.9015) | |
0.2980 | 0.1065 | 0.2633 | 0.2327 | 0.2266 | ||
ℏ(0.5) | 1∗ | (0.0018, 0.2980) | (0.1973, 0.3822) | (0.1859, 0.3759) | (0.0508, 0.3388) | (0.0394, 0.3133) |
0.2962 | 0.1849 | 0.19 | 0.2880 | 0.2739 | ||
2∗ | (0.0068, 0.2972) | (0.1798, 0.3877) | (0.1791, 0.3872) | (0.0563, 0.3306) | (0.0447, 0.3066) | |
0.2904 | 0.2079 | 0.2081 | 0.2742 | 0.2618 | ||
3∗ | (0.0124, 0.1343) | (0.0675, 0.1739) | (0.2904, 0.7625) | (0.0258, 0.1785) | (0.0196, 0.1515) | |
0.1219 | 0.1064 | 0.4721 | 0.1527 | 0.1318 | ||
ℏ(1.5) | 1∗ | (0.0657, 0.4051) | (0.2891, 0.5050) | (0.2828, 0.5181) | (0.1072, 0.4887) | (0.0414, 0.3160) |
0.3394 | 0.2159 | 0.2353 | 0.3815 | 0.2746 | ||
2∗ | (0.0942, 0.3932) | (0.2733, 0.4883) | (0.2721, 0.5078) | (0.1216, 0.4052) | (0.0515, 0.3160) | |
0.299 | 0.215 | 0.2357 | 0.2836 | 0.2646 | ||
3∗ | (0.1924, 0.4551) | (0.0675, 0.1740) | (0.4148, 0.6781) | (0.2144.0.5378) | (0.0249, 0.1690) | |
0.2628 | 0.1065 | 0.2633 | 0.3234 | 0.1441 | ||
π1 | 1∗ | (0.1521, 0.6479) | (0.3333, 0.6) | (0.2953, 0.6505) | (0.1760, 0.6498) | (0.1721, 0.6445) |
0.4958 | 0.2667 | 0.3552 | 0.4738 | 0.4724 | ||
2∗ | (0.2333, 0.7079) | (0.3333, 0.6) | (0.2581, 0.6371) | (0.2463, 0.7006) | (0.2381, 0.6915) | |
0.4746 | 0.2667 | 0.379 | 0.4543 | 0.4533 | ||
3∗ | (0.2454, 0.6346) | (0.36, 0.56) | (0.3450, 0.5533) | (0.2555, 0.6323) | (0.2552, 0.6317) | |
0.3892 | 0.2 | 0.2083 | 0.3768 | 0.3765 | ||
π2 | 1∗ | (0.3521, 0.8479) | (0.4, 0.6667) | (0.3495, 0.7047) | (0.3501, 0.8239) | (0.3555, 0.8279) |
0.4958 | 0.2667 | 0.3552 | 0.4737 | 0.4724 | ||
2∗ | (0.2921, 0.7667) | (0.4, 0.6667) | (0.3629, 0.7419) | (0.2994, 0.7536) | (0.3086, 0.7618) | |
0.4746 | 0.2667 | 0.379 | 0.4542 | 0.4533 | ||
3∗ | (0.3654, 0.7545) | (0.44, 0.64) | (0.4467, 0.6550) | (0.3677, 0.7445) | (0.3683, 0.7448) | |
0.3892 | 0.2 | 0.2083 | 0.3768 | 0.3765 |
Cases | ML | Bayesian (uniform prior) | |||||
BS | BL | BL | BG | BG | |||
(h=1.5) | (h=−1.5) | (c=0.5) | (c=−0.5) | ||||
1∗ | ˆα1 | 0.0611(0.0370) | 0.0836(0.1177) | 0.07612(0.1180) | 0.1010(0.1190) | 0.0520(0.1220) | 0.0687(0.1187) |
ˆα2 | 0.0917(0.0511) | 0.1261(0.1702) | 0.1121(0.1708) | 0.1744(0.1769) | 0.0820(0.1758) | 0.1051(0.1715) | |
ˆβ | 1.5631(1.6424) | 3.4600(5.2310) | 1.1057(5.7364) | 49.410(46.25) | 1.0826(5.7459) | 2.4572(5.3263) | |
ˆℜ(0.5) | 0.9731(0.0153) | 0.9673(0.0198) | 0.9670(0.0198) | 0.9676(0.0199) | 0.9670(0.0198) | 0.9672(0.0198) | |
ˆℜ(1.5) | 0.7552(0.0552) | 0.7610(0.0541) | 0.7588(0.0542) | 0.7631(0.0542) | 0.7580(0.0542) | 0.7600(0.0542) | |
ˆℏ(0.5) | 0.1499(0.0756) | 0.1639(0.0775) | 0.1596(0.0777) | 0.1686(0.0777) | 0.1368(0.0822) | 0.1550(0.0781) | |
ˆℏ(1.5) | 0.2570(0.0976) | 0.2547(0.1338) | 0.2478(0.0985) | 0.2623(0.0986) | 0.2279(0.1019) | 0.2456(0.0987) | |
ˆπ1 | 0.4(0.1265) | 0.399(0.1226) | 0.3879(0.1231) | 0.4104(0.1232) | 0.3675(0.1266) | 0.3891(0.1230) | |
ˆπ2 | 0.6(0.1265) | 0.601(0.1226) | 0.5896(0.1232) | 0.6121(0.1231) | 0.5800(0.1244) | 0.5944(0.1228) | |
2∗ | ˆα1 | 0.0687(0.0338) | 0.0730(0.0422) | 0.0717(0.0422) | 0.0744(0.0422) | 0.0600(0.0441) | 0.0682(0.0424) |
ˆα2 | 0.0772(0.0369) | 0.0824(0.0465) | 0.0808(0.0465) | 0.0841(0.0465) | 0.0684(0.0485) | 0.0773(0.0467) | |
ˆβ | 1.6807(1.5547) | 2.7852(2.717) | 1.3028(3.0955) | 17.69(15.147) | 1.4983(3.0068) | 2.2997(2.7605) | |
ˆℜ(0.5) | 0.9726(0.0150) | 0.9679(0.0168) | 0.9677(0.0168) | 0.9681(0.0168) | 0.9677(0.0168) | 0.9679(0.0168) | |
ˆℜ(1.5) | 0.7581(0.0531) | 0.7624(0.0524) | 0.7603(0.0524) | 0.7644(0.0524) | 0.7596(0.0524) | 0.7615(0.0524) | |
ˆℏ(0.5) | 0.1520(0.0741) | 0.1656(0.0722) | 0.1618(0.0723) | 0.1697(0.0723) | 0.1422(0.0759) | 0.1579(0.0726) | |
ˆℏ(1.5) | 0.2480(0.0785) | 0.2408(0.1047) | 0.2370(0.0730) | 0.2449(0.0730) | 0.2246(0.0747) | 0.2354(0.0731) | |
ˆπ1 | 0.4706(0.1211) | 0.4699(0.1165) | 0.4598(0.1170) | 0.4802(0.1170) | 0.4463(0.1189) | 0.4624(0.1168) | |
ˆπ2 | 0.5294(0.1211) | 0.5301(0.1165) | 0.5198(0.1170) | 0.5402(0.1170) | 0.5089(0.1184) | 0.5233(0.1167) | |
3∗ | ˆα1 | 0.2659(0.2318) | 0.4510(0.5585) | 0.3281(0.5720) | 1.3421(1.0517) | 0.2458(0.5950) | 0.3570(0.5664) |
ˆα2 | 0.3384(0.2913) | 0.5750(0.7032) | 0.3992(0.7248) | 2.0700(1.6521) | 0.3186(0.7485) | 0.4571(0.7130) | |
ˆβ | 0.1368(0.1947) | 0.2622(0.4002) | 0.2007(0.4049) | 1.0029(0.8419) | 0.0825(0.4388) | 0.1880(0.4071) | |
ˆℜ(0.5) | 0.9898(0.0063) | 0.9877(0.0076) | 0.9876(0.0076) | 0.9877(0.0076) | 0.9876(0.0076) | 0.9877(0.0076) | |
ˆℜ(1.5) | 0.7975(0.0760) | 0.7922(0.0617) | 0.7893(0.0618) | 0.7951(0.0618) | 0.7885(0.0619) | 0.7910(0.0617) | |
ˆℏ(0.5) | 0.0610(0.0374) | 0.0725(0.0424) | 0.0712(0.0424) | 0.0739(0.0424) | 0.0592(0.0444) | 0.0676(0.0427) | |
ˆℏ(1.5) | 0.3818(0.0966) | 0.3613(0.007) | 0.3561(0.0840) | 0.666(0.08401) | 0.3465(0.0851) | 0.3564(0.0840) | |
ˆπ1 | 0.44(0.0993) | 0.4397(0.0975) | 0.4326(0.0977) | 0.4468(0.0977) | 0.4224(0.0990) | 0.4341(0.0976) | |
ˆπ2 | 0.56(0.0993) | 0.5603(0.0975) | 0.5532(0.0977) | 0.5674(0.0977) | 0.5468(0.0984) | 0.5560(0.0976) |
Authors | Related works |
Liao and Gui [12] | Discussed the inference for Rayleigh distribution in the presence of CR under PTIICS. |
Wang et al. [13] | Examined the inference for the Weibull CR model with partially observed failure causes under GPHCS. |
Almarashi et al. [14] | Investigated the statistical analysis of Nadarajaha-Haghighi distribution's CR lifetime under type-II censoring. |
Mahmoud et al. [15] | Studied the progressive of the type-I censoring scheme under CR, focusing specifically on the case where the lifetimes of the subjects follow a generalized inverted exponential distribution. |
El-Raheem et al. [16] | Discussed the point and interval statistical inference of the extension of the exponential distribution's parameters under CR data with PTIICS. |
Abushal et al. [17] | Studied the inference of Lomax CR model with partially observed failure causes under the type-II |
generalized hybrid censoring scheme. | |
Qin and Gui [18] | Analyzed CR from Lomax distribution based on adaptive progressive type-II hybrid censored. |
Hassan et al. [19] | Evaluated CR from the analysis of the inverted Topp-Leone distribution under PTIICS. |
Ahmed and Nassar[20] | Examined electrode data analysis within the framework of the Weibull lifetime CR model in the context of improved adaptive progressive censoring. |
Hassen et al.[21] | Studied the Bayesian statistical inference of the generalized inverted exponential distribution through the CR model under GPHCS. |
Tian et al.[22] | Examined the implications of the inverted exponential Rayleigh distribution using the CR model when |
employing optimal PTIICS. | |
Lv et al.[23] | Explored the process of inferring statistics through the CR sample when subjects' lifetimes follow the |
Gompertz distribution in the context of general PTIICS. | |
Salem et al.[24] | Obtained the maximum likelihood estimates (MLEs) and Bayesian estimates (BEs) from the Weibull distribution based on the censored Generalized Progressive Hybrid type-II in the presence of the CR model. |
yi:m:n | 0.50575 | 0.5447 | 0.7069 | 0.7574 | 0.817 | 0.83935 | 0.841 | 0.88625 | 0.89125 | 0.9271 |
δi | 2 | 2 | 2 | 2 | 2 | 1 | 1 | 2 | 1 | 1 |
R | 2 | 0 | 0 | 2 | 0 | 0 | 2 | 0 | 0 | 1 |
yi:m:n | 0.9384 | 1.06065 | 1.0943 | 1.13265 | 1.27145 | 1.341 | 1.35395 | 1.45635 | 1.5242 | 1.53495 |
δi | 1 | 2 | 1 | 1 | 1 | 1 | 1 | 2 | 1 | 1 |
R | 0 | 0 | 0 | 1 | 0 | 2 | 0 | 1 | 0 | 1 |
yi:m:n | 1.57665 | 1.61915 | 1.7662 | 1.8821 | 1.91715 | 2.20935 | 2.47755 | 2.533 | 2.5824 | 2.65275 |
δi | 1 | 2 | 2 | 2 | 2 | 1 | 1 | 2 | 1 | 2 |
R | 0 | 0 | 0 | 0 | 0 | 1 | 0 | 1 | 0 | 1 |
yi:m:n | 2.77305 | 2.83155 | 2.9524 | 3.09285 | 3.1883 | 3.5037 | 3.5233 | 3.63615 | 4.04615 | 4.11515 |
δi | 1 | 1 | 2 | 1 | 2 | 2 | 2 | 2 | 1 | 1 |
R | 0 | 0 | 0 | 0 | 0 | 1 | 0 | 0 | 1 | 3 |
yi:m:n | 0.21965 | 0.50575 | 0.6153 | 0.6454 | 0.817 | 0.83935 | 0.841 | 0.9271 | 1.13265 |
δi | 2 | 2 | 2 | 1 | 1 | 1 | 1 | 1 | 1 |
R | 3 | 0 | 3 | 0 | 3 | 0 | 3 | 0 | 3 |
yi:m:n | 1.30485 | 1.5242 | 1.57665 | 1.8385 | 1.8501 | 1.91715 | 2.20935 | 2.47755 | 2.68725 |
δi | 1 | 2 | 1 | 1 | 1 | 2 | 1 | 1 | 1 |
R | 0 | 3 | 0 | 3 | 0 | 3 | 0 | 3 | 0 |
yi:m:n | 2.77305 | 2.83155 | 2.9524 | 3.09285 | 3.5233 | 3.89085 | 4.11515 | ||
δi | 1 | 1 | 2 | 1 | 2 | 2 | 1 | ||
R | 3 | 0 | 3 | 0 | 2 | 0 | 0 |
n | m | k | Scheme | ˆα1ML | ˆα1BS | ˆα1BL | ˆα1BL | ˆα1BG | ˆα1BG | |
(h=1.5) | (h=−1.5) | (c=0.5) | (c=−0.5) | |||||||
60 | 40 | 25 | 1 | AB | 0.0061 | 0.0027 | 0.0102 | 0.0167 | 0.0234 | 0.0060 |
MSE | 0.0136 | 0.0093 | 0.0088 | 0.0102 | 0.0096 | 0.0092 | ||||
2 | AB | 0.0093 | 0.0045 | 0.0083 | 0.0184 | 0.0211 | 0.0040 | |||
MSE | 0.0123 | 0.0090 | 0.0085 | 0.0099 | 0.0092 | 0.0089 | ||||
3 | AB | 0.0079 | 0.0058 | 0.0054 | 0.0178 | 0.0163 | 0.0016 | |||
MSE | 0.0094 | 0.0084 | 0.0079 | 0.0092 | 0.0083 | 0.0083 | ||||
30 | 1 | AB | 0.0077 | 0.0056 | 0.0070 | 0.0192 | 0.0196 | 0.0028 | ||
MSE | 0.0124 | 0.0092 | 0.0087 | 0.0102 | 0.0093 | 0.0091 | ||||
2 | AB | 0.0067 | 0.0060 | 0.0067 | 0.0197 | 0.0192 | 0.0024 | |||
MSE | 0.0123 | 0.0092 | 0.0086 | 0.0102 | 0.0093 | 0.0091 | ||||
3 | AB | 0.0062 | 0.0054 | 0.0057 | 0.0174 | 0.0167 | 0.0020 | |||
MSE | 0.0094 | 0.0084 | 0.0079 | 0.0093 | 0.0084 | 0.0083 | ||||
50 | 25 | 1 | AB | 0.0095 | 0.0034 | 0.0082 | 0.0158 | 0.0198 | 0.0044 | |
MSE | 0.0106 | 0.0085 | 0.0081 | 0.0093 | 0.0087 | 0.0085 | ||||
2 | AB | 0.0094 | 0.0027 | 0.0088 | 0.0151 | 0.0203 | 0.0050 | |||
MSE | 0.0108 | 0.0086 | 0.0082 | 0.0094 | 0.0089 | 0.0086 | ||||
3 | AB | 0.0082 | 0.0043 | 0.0062 | 0.0155 | 0.0166 | 0.0027 | |||
MSE | 0.0090 | 0.0078 | 0.0074 | 0.0085 | 0.0079 | 0.0078 | ||||
30 | 1 | AB | 0.0069 | 0.0033 | 0.0082 | 0.0157 | 0.0198 | 0.0044 | ||
MSE | 0.0106 | 0.0087 | 0.0083 | 0.0094 | 0.0089 | 0.0086 | ||||
2 | AB | 0.0082 | 0.0041 | 0.0074 | 0.0165 | 0.0189 | 0.0036 | |||
MSE | 0.0106 | 0.0085 | 0.0080 | 0.0093 | 0.0086 | 0.0084 | ||||
3 | AB | 0.0070 | 0.0026 | 0.0079 | 0.0137 | 0.0183 | 0.0044 | |||
MSE | 0.0090 | 0.0077 | 0.0074 | 0.0083 | 0.0079 | 0.0077 |
n | m | k | Scheme | ˆα2ML | ˆα2BS | ˆα2BL | ˆα2BL | ˆα2BG | ˆα2BG | |
(h=1.5) | (h=−1.5) | (c=0.5) | (c=−0.5) | |||||||
60 | 40 | 25 | 1 | AB | 0.0151 | 0.0016 | 0.0157 | 0.0203 | 0.0219 | 0.0062 |
MSE | 0.0142 | 0.0091 | 0.0088 | 0.0100 | 0.0094 | 0.0091 | ||||
2 | AB | 0.0121 | 0.0023 | 0.0150 | 0.0208 | 0.0211 | 0.0055 | |||
MSE | 0.0129 | 0.0086 | 0.0084 | 0.0096 | 0.0089 | 0.0086 | ||||
3 | AB | 0.0097 | 0.0061 | 0.0096 | 0.0229 | 0.0149 | 0.0009 | |||
MSE | 0.0098 | 0.0080 | 0.0076 | 0.0090 | 0.0080 | 0.0079 | ||||
30 | 1 | AB | 0.0088 | 0.0046 | 0.0124 | 0.0227 | 0.0182 | 0.0031 | ||
MSE | 0.0131 | 0.0088 | 0.0084 | 0.0099 | 0.0089 | 0.0087 | ||||
2 | AB | 0.0120 | 0.0044 | 0.0127 | 0.0228 | 0.0186 | 0.0033 | |||
MSE | 0.0127 | 0.0087 | 0.0083 | 0.0097 | 0.0088 | 0.0086 | ||||
3 | AB | 0.0107 | 0.0058 | 0.0098 | 0.0226 | 0.0151 | 0.0012 | |||
MSE | 0.0099 | 0.0084 | 0.0080 | 0.0095 | 0.0084 | 0.0083 | ||||
50 | 25 | 1 | AB | 0.0110 | 0.0025 | 0.0135 | 0.0196 | 0.0190 | 0.0047 | |
MSE | 0.0112 | 0.0085 | 0.0082 | 0.0093 | 0.0087 | 0.0084 | ||||
2 | AB | 0.0110 | 0.0022 | 0.0137 | 0.0193 | 0.0193 | 0.0050 | |||
MSE | 0.0112 | 0.0085 | 0.0082 | 0.0093 | 0.0087 | 0.0085 | ||||
3 | AB | 0.0113 | 0.0031 | 0.0117 | 0.0190 | 0.0168 | 0.0035 | |||
MSE | 0.0094 | 0.0080 | 0.0077 | 0.0088 | 0.0082 | 0.0079 | ||||
30 | 1 | AB | 0.0126 | 0.0034 | 0.0126 | 0.0205 | 0.0181 | 0.0038 | ||
MSE | 0.0111 | 0.0087 | 0.0084 | 0.0096 | 0.0089 | 0.0087 | ||||
2 | AB | 0.0121 | 0.0041 | 0.0074 | 0.0165 | 0.0189 | 0.0036 | |||
MSE | 0.0112 | 0.0085 | 0.0080 | 0.0092 | 0.0086 | 0.0084 | ||||
3 | AB | 0.0132 | 0.0032 | 0.0115 | 0.0192 | 0.0167 | 0.0034 | |||
MSE | 0.0095 | 0.0079 | 0.0077 | 0.0087 | 0.0081 | 0.0079 |
n | m | k | Scheme | ˆα1ML | ˆα1BS | ˆα1BL | ˆα1BL | ˆα1BG | ˆα1BG | |
(h=1.5) | (h=−1.5) | (c=0.5) | (c=−0.5) | |||||||
60 | 40 | 25 | 1 | AB | 0.0063 | 0.0029 | 0.0160 | 0.0231 | 0.0212 | 0.0051 |
MSE | 0.0316 | 0.0116 | 0.0112 | 0.0129 | 0.0119 | 0.0116 | ||||
2 | AB | 0.0093 | 0.0026 | 0.0163 | 0.0227 | 0.0215 | 0.0054 | |||
MSE | 0.0315 | 0.0117 | 0.0113 | 0.0130 | 0.0120 | 0.0117 | ||||
3 | AB | 0.0065 | 0.0029 | 0.0157 | 0.0227 | 0.0207 | 0.0050 | |||
MSE | 0.0295 | 0.0117 | 0.0113 | 0.0130 | 0.0120 | 0.0117 | ||||
30 | 1 | AB | 0.0063 | 0.0023 | 0.0165 | 0.0225 | 0.0218 | 0.0057 | ||
MSE | 0.0322 | 0.0114 | 0.0110 | 0.0126 | 0.0117 | 0.0114 | ||||
2 | AB | 0.0077 | 0.0011 | 0.0177 | 0.0212 | 0.0230 | 0.0069 | |||
MSE | 0.0321 | 0.0118 | 0.0114 | 0.0130 | 0.0121 | 0.0117 | ||||
3 | AB | 0.0051 | 0.0033 | 0.0152 | 0.0232 | 0.0203 | 0.0045 | |||
MSE | 0.0303 | 0.0117 | 0.0113 | 0.0130 | 0.0119 | 0.0117 | ||||
50 | 25 | 1 | AB | 0.0062 | 0.0043 | 0.0127 | 0.0225 | 0.0174 | 0.0029 | |
MSE | 0.0262 | 0.0114 | 0.0109 | 0.0125 | 0.0115 | 0.0113 | ||||
2 | AB | 0.0069 | 0.0028 | 0.0142 | 0.0209 | 0.0188 | 0.0044 | |||
MSE | 0.0247 | 0.0113 | 0.01090 | 0.0124 | 0.0115 | 0.0113 | ||||
3 | AB | 0.0033 | 0.0044 | 0.0123 | 0.0221 | 0.0167 | 0.0027 | |||
MSE | 0.0247 | 0.0114 | 0.0109 | 0.0125 | 0.0114 | 0.0113 | ||||
30 | 1 | AB | 0.0061 | 0.0011 | 0.0159 | 0.0192 | 0.0205 | 0.0061 | ||
MSE | 0.0266 | 0.0112 | 0.0109 | 0.0122 | 0.0115 | 0.0112 | ||||
2 | AB | 0.0083 | 0.0052 | 0.0119 | 0.0234 | 0.0164 | 0.0020 | |||
MSE | 0.0258 | 0.0115 | 0.0110 | 0.0127 | 0.0116 | 0.0114 | ||||
3 | AB | 0.0041 | 0.0045 | 0.0122 | 0.0222 | 0.0166 | 0.0026 | |||
MSE | 0.0247 | 0.0112 | 0.0108 | 0.0124 | 0.0113 | 0.0112 |
n | m | k | Scheme | ˆα2ML | ˆα2BS | ˆα2BL | ˆα2BL | ˆα2BG | ˆα2BG | |
(h=1.5) | (h=−1.5) | (c=0.5) | (c=−0.5) | |||||||
60 | 40 | 25 | 1 | AB | 0.0130 | 0.0025 | 0.0225 | 0.0287 | 0.0145 | 0.0032 |
MSE | 0.0337 | 0.0092 | 0.0093 | 0.0105 | 0.0093 | 0.0092 | ||||
2 | AB | 0.0120 | 0.0005 | 0.0245 | 0.0267 | 0.0165 | 0.0052 | |||
MSE | 0.0335 | 0.0090 | 0.0092 | 0.0102 | 0.0092 | 0.0090 | ||||
3 | AB | 0.0105 | 0.0038 | 0.0212 | 0.0299 | 0.0131 | 0.0019 | |||
MSE | 0.0300 | 0.0089 | 0.0089 | 0.0102 | 0.0089 | 0.0088 | ||||
30 | 1 | AB | 0.0137 | 0.0013 | 0.0237 | 0.0275 | 0.0156 | 0.0043 | ||
MSE | 0.0342 | 0.0093 | 0.0095 | 0.0106 | 0.0095 | 0.0093 | ||||
2 | AB | 0.0131 | 0.0031 | 0.0220 | 0.0293 | 0.0139 | 0.0026 | |||
MSE | 0.0345 | 0.0092 | 0.0092 | 0.0105 | 0.0093 | 0.0092 | ||||
3 | AB | 0.0115 | 0.0042 | 0.0207 | 0.0304 | 0.0127 | 0.0014 | |||
MSE | 0.0310 | 0.0090 | 0.0090 | 0.0104 | 0.0091 | 0.0090 | ||||
50 | 25 | 1 | AB | 0.0115 | 0.0037 | 0.0198 | 0.0283 | 0.0122 | 0.0016 | |
MSE | 0.0281 | 0.0099 | 0.0098 | 0.0111 | 0.0099 | 0.0098 | ||||
2 | AB | 0.0100 | 0.0005 | 0.0230 | 0.0250 | 0.0154 | 0.0048 | |||
MSE | 0.0269 | 0.0096 | 0.0097 | 0.0107 | 0.0098 | 0.0096 | ||||
3 | AB | 0.0118 | 0.0034 | 0.0198 | 0.0277 | 0.0123 | 0.0019 | |||
MSE | 0.0258 | 0.0095 | 0.0094 | 0.0107 | 0.0095 | 0.0094 | ||||
30 | 1 | AB | 0.0114 | 0.0012 | 0.0223 | 0.0257 | 0.0147 | 0.0041 | ||
MSE | 0.0285 | 0.0097 | 0.0097 | 0.0108 | 0.0098 | 0.0096 | ||||
2 | AB | 0.0096 | 0.0009 | 0.0225 | 0.0254 | 0.0150 | 0.0044 | |||
MSE | 0.0277 | 0.0093 | 0.0094 | 0.0104 | 0.0094 | 0.0093 | ||||
3 | AB | 0.0106 | 0.0029 | 0.0203 | 0.0272 | 0.0128 | 0.0024 | |||
MSE | 0.0257 | 0.0095 | 0.0095 | 0.0107 | 0.0096 | 0.0095 |
n | m | k | Scheme | ˆβML | ˆβBS | ˆβBL | ˆβBL | ˆβBG | ˆβBG | |
(h=1.5) | (h=−1.5) | (c=0.5) | (c=−0.5) | |||||||
60 | 40 | 25 | 1 | AB | 0.0790 | 0.0116 | 0.0200 | 0.0447 | 0.0045 | 0.0062 |
MSE | 0.2168 | 0.0041 | 0.0042 | 0.0061 | 0.0040 | 0.0040 | ||||
2 | AB | 0.0750 | 0.0108 | 0.0205 | 0.0435 | 0.0051 | 0.0055 | |||
MSE | 0.2000 | 0.0042 | 0.0044 | 0.0062 | 0.0042 | 0.0042 | ||||
3 | AB | 0.0774 | 0.0106 | 0.0201 | 0.0427 | 0.0050 | 0.0054 | |||
MSE | 0.1685 | 0.0043 | 0.0045 | 0.0062 | 0.0042 | 0.0042 | ||||
30 | 1 | AB | 0.0851 | 0.0106 | 0.0210 | 0.0437 | 0.0054 | 0.0053 | ||
MSE | 0.2232 | 0.0040 | 0.0042 | 0.0059 | 0.0039 | 0.0039 | ||||
2 | AB | 0.0781 | 0.0116 | 0.0197 | 0.0444 | 0.0042 | 0.0063 | |||
MSE | 0.1966 | 0.0043 | 0.0044 | 0.0063 | 0.0041 | 0.0042 | ||||
3 | AB | 0.0716 | 0.0110 | 0.0196 | 0.0430 | 0.0045 | 0.0058 | |||
MSE | 0.1690 | 0.0044 | 0.0045 | 0.0063 | 0.0043 | 0.0043 | ||||
50 | 25 | 1 | AB | 0.0729 | 0.0115 | 0.0194 | 0.0439 | 0.0042 | 0.0063 | |
MSE | 0.1736 | 0.0043 | 0.0044 | 0.0063 | 0.0042 | 0.0042 | ||||
2 | AB | 0.0735 | 0.0089 | 0.0219 | 0.0412 | 0.0068 | 0.0037 | |||
MSE | 0.1701 | 0.0042 | 0.0045 | 0.0059 | 0.0041 | 0.0041 | ||||
3 | AB | 0.0669 | 0.0106 | 0.0198 | 0.0424 | 0.0049 | 0.0054 | |||
MSE | 0.1502 | 0.0043 | 0.0044 | 0.0061 | 0.0042 | 0.0042 | ||||
30 | 1 | AB | 0.0765 | 0.0093 | 0.0217 | 0.0417 | 0.0064 | 0.0040 | ||
MSE | 0.1721 | 0.0042 | 0.0044 | 0.0060 | 0.0041 | 0.0041 | ||||
2 | AB | 0.0697 | 0.0101 | 0.0208 | 0.0424 | 0.0056 | 0.0049 | |||
MSE | 0.1724 | 00042 | 0.0044 | 0.0060 | 0.0041 | 0.0041 | ||||
3 | AB | 0.0670 | 0.0103 | 0.0202 | 0.0421 | 0.0052 | 0.0051 | |||
MSE | 0.1477 | 0.0042 | 0.0044 | 0.0060 | 0.0041 | 0.0041 |
n | m | k | Scheme | ˆβML | ˆβBS | ˆβBL | ˆβ1BL | ˆβBG | ˆβBG | |
(h=1.5) | (h=−1.5) | (c=0.5) | (c=−0.5) | |||||||
60 | 40 | 25 | 1 | AB | 0.0551 | 0.0221 | 0.0064 | 0.0533 | 0.0066 | 0.0125 |
MSE | 0.0799 | 0.0045 | 0.0039 | 0.0072 | 0.0041 | 0.0042 | ||||
2 | AB | 0.0592 | 0.0232 | 0.0047 | 0.0537 | 0.0049 | 0.0139 | |||
MSE | 0.0729 | 0.0046 | 0.0039 | 0.0073 | 0.0041 | 0.0043 | ||||
3 | AB | 0.0468 | 0.0212 | 0.0061 | 0.0511 | 0.0063 | 0.0120 | |||
MSE | 0.0556 | 0.0043 | 0.0037 | 0.0067 | 0.0039 | 0.0040 | ||||
30 | 1 | AB | 0.0635 | 0.0219 | 0.0065 | 0.0529 | 0.0068 | 0.0123 | ||
MSE | 0.0792 | 0.0046 | 0.0039 | 0.0072 | 0.0041 | 0.0042 | ||||
2 | AB | 0.0647 | 0.0232 | 0.0046 | 0.0537 | 0.0048 | 0.0139 | |||
MSE | 0.0708 | 0.0047 | 0.0040 | 0.0074 | 0.0042 | 0.0044 | ||||
3 | AB | 0.0487 | 0.0219 | 0.0054 | 0.0517 | 0.0056 | 0.0127 | |||
MSE | 0.0546 | 0.0042 | 0.0036 | 0.0067 | 0.0038 | 0.0039 | ||||
50 | 25 | 1 | AB | 0.0521 | 0.0230 | 0.0048 | 0.0534 | 0.0050 | 0.0137 | |
MSE | 0.0644 | 0.0045 | 0.0037 | 0.0071 | 0.0040 | 0.0042 | ||||
2 | AB | 0.0511 | 0.0223 | 0.0054 | 0.0525 | 0.0056 | 0.0130 | |||
MSE | 0.0637 | 0.0046 | 0.0039 | 0.0071 | 0.0041 | 0.0042 | ||||
3 | AB | 0.0475 | 0.0208 | 0.0064 | 0.0506 | 0.0066 | 0.0117 | |||
MSE | 0.0543 | 0.0044 | 0.0038 | 0.0068 | 0.0040 | 0.0042 | ||||
30 | 1 | AB | 0.0565 | 0.0229 | 0.0050 | 0.0533 | 0.0052 | 0.0135 | ||
MSE | 0.0646 | 0.0045 | 0.0038 | 0.0071 | 0.0040 | 0.0042 | ||||
2 | AB | 0.0536 | 0.0230 | 0.0047 | 0.0533 | 0.0049 | 0.0137 | |||
MSE | 0.0638 | 0.0046 | 0.0038 | 0.0072 | 0.0040 | 0.0042 | ||||
3 | AB | 0.0462 | 0.0216 | 0.0057 | 0.0513 | 0.0059 | 0.0124 | |||
MSE | 0.0535 | 0.0044 | 0.0037 | 0.0068 | 0.0039 | 0.0040 |
n | t | m | k | Scheme | ˆℜML | ˆℜBS | ˆℜBL | ˆℜBL | ˆℜBG | ˆℜBG | |
(h=1.5) | (h=−1.5) | (c=0.5) | (c=−0.5) | ||||||||
60 | 0.1 | 40 | 25 | 1 | AE | 0.9987 | 0.9987 | 0.9987 | 0.9987 | 0.9987 | 0.9987 |
MSE | 1.2437*10−7 | 3.6402*10−8 | 3.6408*10−8 | 3.6396*10−8 | 3.6408*10−8 | 3.6404*10−8 | |||||
2 | AE | 0.99870 | 0.9987 | 0.9987 | 0.9987 | 0.9987 | 0.9987 | ||||
MSE | 1.0923*10−7 | 3.5807*10−8 | 3.5812*10−8 | 3.5818*10−8 | 3.5812*10−8 | 3.5809*10−8 | |||||
3 | AE | 0.9987 | 0.9987 | 0.9987 | 0.9987 | 0.9987 | 0.9987 | ||||
MSE | 8.5362*10−8 | 3.2401*10−8 | 3.2406*10−8 | 3.2397*10−8 | 3.2406*10−8 | 3.2403*10−8 | |||||
30 | 1 | AE | 0.9988 | 0.9987 | 0.9987 | 0.9987 | 0.9987 | 0.9987 | |||
MSE | 1.226*10−7 | 3.567*10−8 | 3.567*10−8 | 3.566*10−8 | 3.567*10−8 | 3.566*10−8 | |||||
2 | AE | 0.9987 | 0.9987 | 0.9987 | 0.9987 | 0.9987 | 0.9987 | ||||
MSE | 10.36*10−8 | 34.04*10−9 | 34.04*10−9 | 34.06*10−9 | 34.04*10−9 | 34.04*10−9 | |||||
3 | AE | 0.9987 | 0.9987 | 0.9987 | 0.9987 | 0.9987 | 0.9987 | ||||
MSE | 8.526*10−8 | 3.2184*10−8 | 3.2188*10−8 | 3.2180*10−8 | 3.2188*10−8 | 3.2185*10−8 | |||||
50 | 25 | 1 | AE | 0.9987 | 0.9987 | 0.9987 | 0.9987 | 0.9987 | 0.9987 | ||
MSE | 9.770*10−8 | 3.3138*10−8 | 3.3134*10−8 | 3.3134*10−8 | 3.3143*10−8 | 3.3140*10−8 | |||||
2 | AE | 0.9987 | 0.9987 | 0.9987 | 0.9987 | 0.9987 | 0.9987 | ||||
MSE | 9.3246*10−8 | 3.2173*10−8 | 3.2178*10−8 | 3.2169*10−8 | 3.2178*10−8 | 3.2175*10−8 | |||||
3 | AE | 0.9987 | 0.9987 | 0.9987 | 0.9987 | 0.9987 | 0.9987 | ||||
MSE | 8.0102*10−8 | 2.9671*10−8 | 2.9674*10−8 | 2.9668*10−8 | 2.9674*10−8 | 2.9672*10−8 | |||||
30 | 1 | AE | 0.9987 | 0.9987 | 0.9987 | 0.9987 | 0.9987 | 0.9987 | |||
MSE | 9.900*10−8 | 3.3295*10−8 | 3.3300*10−8 | 3.3291*10−8 | 3.330*10−8 | 3.3297*10−8 | |||||
2 | AE | 0.9987 | 0.9987 | 0.9987 | 0.9987 | 0.9987 | 0.9987 | ||||
MSE | 9.445*10−8 | 3.2535*10−8 | 3.2539*10−8 | 3.2530*10−8 | 3.2539*10−8 | 3.2536*10−8 | |||||
3 | AE | 0.9987 | 0.9987 | 0.9987 | 0.9987 | 0.9987 | 0.9987 | ||||
MSE | 7.702*10−8 | 2.8550*10−8 | 2.8554*10−8 | 2.8547*10−8 | 2.8554*10−8 | 2.8552*10−8 | |||||
0.5 | 40 | 25 | 1 | AE | 0.8587 | 0.8616 | 0.8613 | 0.8621 | 0.8612 | 0.8615 | |
MSE | 0.0011 | 34.93*10−5 | 35.34*10−5 | 34.53*10−5 | 35.46*10−5 | 35.10*10−5 | |||||
2 | AE | 0.8597 | 0.8621 | 0.8617 | 0.8624 | 0.8616 | 0.8619 | ||||
MSE | 9.393*10−4 | 34.41*10−5 | 34.78*10−5 | 34.07*10−5 | 34.88*10−5 | 34.56*10−5 | |||||
3 | AE | 0.8600 | 0.8619 | 0.8616 | 0.8622 | 0.8616 | 0.8618 | ||||
MSE | 7.479*10−4 | 31.24*10−5 | 31.55*10−5 | 30.96*10−5 | 31.63*10−5 | 31.37*10−5 | |||||
30 | 1 | AE | 0.8583 | 0.8615 | 0.8611 | 0.8618 | 0.8610 | 0.8613 | |||
MSE | 10.44*10−4 | 34.12*10−5 | 34.57*10−5 | 33.71*10−5 | 34.68*10−5 | 34.30*10−5 | |||||
2 | AE | 0.8587 | 0.8615 | 0.8611 | 0.8618 | 0.6810 | 0.8613 | ||||
MSE | 8.931*10−4 | 32.58*10−5 | 32.99*10−5 | 32.21*10−5 | 33.10*10−5 | 32.75*10−5 | |||||
3 | AE | 0.8598 | 0.8618 | 0.8615 | 0.8621 | 0.8614 | 0.8617 | ||||
MSE | 7.448*10−4 | 30.98*10−5 | 31.30*10−5 | 30.69*10−5 | 31.38*10−5 | 31.11*10−5 | |||||
50 | 25 | 1 | AE | 0.8600 | 0.8621 | 0.8618 | 0.8624 | 0.8617 | 0.8620 | ||
MSE | 8.528*10−4 | 32.01*10−5 | 32.30*10−5 | 31.73*10−5 | 32.39*10−5 | 32.13*10−5 | |||||
2 | AE | 0.8601 | 0.8622 | 0.8618 | 0.8625 | 0.8618 | 0.8620 | ||||
MSE | 8.140*10−4 | 31.06*10−5 | 31.35*10−5 | 30.80*10−5 | 31.43*10−5 | 31.18*10−5 | |||||
3 | AE | 0.8609 | 0.8626 | 0.8623 | 0.8629 | 0.8622 | 0.8624 | ||||
MSE | 7.049*10−4 | 28.76*10−5 | 28.97*10−5 | 28.56*10−5 | 29.03*10−5 | 28.85*10−5 | |||||
30 | 1 | AE | 0.8597 | 0.8620 | 0.8617 | 0.8623 | 0.8616 | 0.8619 | |||
MSE | 8.597*10−4 | 32.09*10−5 | 32.40*10−5 | 31.81*10−5 | 32.49*10−5 | 32.22*10−5 | |||||
2 | AE | 0.8600 | 0.8622 | 0.8619 | 0.8625 | 0.8618 | 0.8620 | ||||
MSE | 8.218*10−4 | 31.37*10−5 | 31.67*10−5 | 31.11*10−5 | 31.75*10−5 | 31.50*10−5 | |||||
3 | AE | 0.8605 | 0.8623 | 0.8620 | 0.8626 | 0.8619 | 0.8622 | ||||
MSE | 6.793*10−4 | 27.68*10−5 | 27.89*10−5 | 27.47*10−5 | 27.96*10−5 | 27.76*10−5 |
n | t | m | k | Scheme | ˆℏML | ˆℏBS | ˆℏBL | ˆℏBL | ˆℏBG | ˆℏBG | |
(h=1.5) | (h=−1.5) | (c=0.5) | (c=−0.5) | ||||||||
60 | 0.1 | 40 | 25 | 1 | AE | 0.03922 | 0.03814 | 0.038102 | 0.03818 | 0.03718 | 0.03782 |
MSE | 1.11*10−4 | 3.28*10−5 | 3.26*10−5 | 3.30*10−5 | 3.133*10−5 | 3.21*10−5 | |||||
2 | AE | 0.0389 | 0.03801 | 0.03797 | 0.03804 | 0.03710 | 0.03771 | ||||
MSE | 9.836*10−5 | 3.227*10−5 | 3.213*10−5 | 3.241*10−5 | 3.097*10−5 | 3.165*10−5 | |||||
3 | AE | 0.03870 | 0.03802 | 0.03799 | 0.3805 | 0.03727 | 0.03777 | ||||
MSE | 7.687*10−5 | 2.920*10−5 | 2.908*10−5 | 2.931*10−5 | 2.799*10−5 | 2.867*10−5 | |||||
30 | 1 | AE | 0.0393 | 0.03820 | 0.03816 | 0.03823 | 0.03725 | 0.03788 | |||
MSE | 1.104*10−4 | 3.214*10−5 | 3.198*10−5 | 3.231*10−5 | 3.041*10−5 | 3.136*10−5 | |||||
2 | AE | 0.03916 | 0.03818 | 0.03184 | 0.03821 | 0.03728 | 0.03788 | ||||
MSE | 9.333*10−5 | 3.067*10−5 | 3.052*10−5 | 3.083*10−5 | 2.905*10−5 | 2.995*10−5 | |||||
3 | AE | 0.03876 | 0.03806 | 0.03803 | 0.03809 | 0.03705 | 0.037809 | ||||
MSE | 7.677*10−5 | 2.900*10−5 | 2.889*10−5 | 2.912*10−5 | 2.77*10−5 | 2.845*10−5 | |||||
50 | 25 | 1 | AE | 0.03875 | 0.03797 | 0.037938 | 0.03800 | 0.03714 | 0.03769 | ||
MSE | 8.798*10−5 | 2.986*10−5 | 2.975*10−5 | 2.998*10−5 | 2.887*10−5 | 2.938*10−5 | |||||
2 | AE | 0.03872 | 0.03496 | 0.03793 | 0.03799 | 0.03714 | 0.03769 | ||||
MSE | 8.397*10−5 | 2.899*10−5 | 2.888*10−5 | 2.911*10−5 | 2.802*10−5 | 2.852*10−5 | |||||
3 | AE | 0.03841 | 0.03782 | 0.03779 | 0.03785 | 0.03709 | 0.03758 | ||||
MSE | 7.213*10−5 | 2.674*10−5 | 2.665*10−5 | 2.682*10−5 | 2.609*10−5 | 2.640*10−5 | |||||
30 | 1 | AE | 0.03883 | 0.03802 | 0.03798 | 0.03805 | 0.03719 | 0.03774 | |||
MSE | 8.915*10−5 | 3.00*10−5 | 2.988*10−5 | 3.012*10−5 | 2.893*10−5 | 2.949*10−5 | |||||
2 | AE | 0.03873 | 0.03796 | 0.03793 | 0.03799 | 0.03714 | 0.03377 | ||||
MSE | 8.505*10−5 | 2.932*10−5 | 2.920*10−5 | 2.943*10−5 | 2.833*10−5 | 2.884*10−5 | |||||
3 | AE | 0.03853 | 0.03790 | 0.037874 | 0.037931 | 0.03717 | 0.03766 | ||||
MSE | 6.936*10−5 | 2.573*10−5 | 2.564*10−5 | 2.582*10−5 | 2.499*10−5 | 2.536*10−5 | |||||
0.5 | 40 | 25 | 1 | AE | 0.8603 | 0.8440 | 0.8284 | 0.8606 | 0.8253 | 0.8378 | |
MSE | 0.0408 | 0.0146 | 0.0137 | 0.0162 | 0.0142 | 0.0144 | |||||
2 | AE | 0.8541 | 0.8413 | 0.8266 | 0.8568 | 0.8237 | 0.8354 | ||||
MSE | 0.0361 | 0.0144 | 0.0135 | 0.0158 | 0.0140 | 0.0142 | |||||
3 | AE | 0.8522 | 0.8421 | 0.8298 | 0.8548 | 0.8275 | 0.8372 | ||||
MSE | 0.0287 | 0.0131 | 0.0123 | 0.0142 | 0.0127 | 0.0129 | |||||
30 | 1 | AE | 0.8628 | 0.8454 | 0.8300 | 0.8616 | 0.8271 | 0.8393 | |||
MSE | 0.0401 | 0.0143 | 0 0133 | 0.0160 | 0.0137 | 0.0140 | |||||
2 | AE | 0.8604 | 0.8451 | 0.8305 | 0.8605 | 0.8277 | 0.8393 | ||||
MSE | 0.0343 | 0.0136 | 0.0127 | 0.0151 | 0.0131 | 0.0134 | |||||
3 | AE | 0.8534 | 0.8428 | 0.8306 | 0.8557 | 0.8283 | 0.8380 | ||||
MSE | 0.0286 | 0.0130 | 0.0122 | 0.0141 | 0.0125 | 0.0128 | |||||
50 | 25 | 1 | AE | 0.8524 | 0.8406 | 0.8273 | 0.8546 | 0.8247 | 0.8353 | ||
MSE | 0.0328 | 0.0133 | 0.0127 | 0.0145 | 0.0131 | 0.0132 | |||||
2 | AE | 0.8519 | 0.8405 | 0.8273 | 0.8543 | 0.8247 | 0.8352 | ||||
MSE | 0.0313 | 0.0130 | 0.0123 | 0.0141 | 0.0127 | 0.0128 | |||||
3 | AE | 0.8466 | 0.8377 | 0.8261 | 0.8499 | 0.8568 | 0.8331 | ||||
MSE | 0.0271 | 0.0120 | 0.0115 | 0.0128 | 0.0118 | 0.0119 | |||||
30 | 1 | AE | 0.8539 | 0.8417 | 0.8283 | 0.8557 | 0.8257 | 0.8363 | |||
MSE | 0.0330 | 0.0134 | 0.0127 | 0.0146 | 0.0131 | 0.0133 | |||||
2 | AE | 0.8522 | 0.8405 | 0.8274 | 0.8544 | 0.8247 | 0.8353 | ||||
MSE | 0.0316 | 0.0131 | 0.0124 | 0.0142 | 0.0128 | 0.0129 | |||||
3 | AE | 0.8491 | 0.8350 | 0.8278 | 0.8516 | 0.8255 | 0.8348 | ||||
MSE | 0.0261 | 0.0115 | 0.0110 | 0.0124 | 0.0113 | 0.0114 |
t | (n,m,k) | Scheme | ˆℜML | ˆℜBS | ˆℜBL | ˆℜBL | ˆℜBG | ˆℜBG | |
(h=1.5) | (h=−1.5) | (c=0.5) | (c=−0.5) | ||||||
0.1 | (60, 40, 25) | 1 | AE | 0.6471 | 0.6573 | 0.6565 | 0.6581 | 0.6560 | 0.6587 |
MSE | 0.0032 | 536*10−6 | 541*10−6 | 533*10−6 | 546*10−6 | 539*10−6 | |||
2 | AE | 0.6493 | 0.6577 | 0.6569 | 0.6585 | 0.6564 | 0.6573 | ||
MSE | 0.0029 | 538*10−6 | 542*10−6 | 535*10−6 | 547*10−6 | 540*10−6 | |||
3 | AE | 0.6510 | 0.6573 | 0.6565 | 0.6581 | 0.6561 | 0.6569 | ||
MSE | 0.0023 | 530*10−6 | 535*10−6 | 527*10−6 | 540*10−6 | 533*10−6 | |||
(60, 40, 30) | 1 | AE | 0.6479 | 0.6576 | 0.6568 | 0.6585 | 0.6563 | 0.6572 | |
MSE | 0.0031 | 524*10−6 | 528*10−6 | 521*10−6 | 532*10−6 | 526*10−6 | |||
2 | AE | 0.6485 | 0.6575 | 0.6566 | 0.6583 | 0.6562 | 0.6570 | ||
MSE | 0.0029 | 531*10−6 | 535*10−6 | 528*10−6 | 541*10−6 | 534*10−6 | |||
3 | AE | 0.6507 | 0.6571 | 0.6563 | 0.6579 | 0.6559 | 0.6567 | ||
MSE | 0.0023 | 538*10−6 | 543*10−6 | 534*10−6 | 548*10−6 | 541*10−6 | |||
(60, 50, 25) | 1 | AE | 0.6473 | 0.6570 | 0.6562 | 0.6577 | 0.6558 | 0.6566 | |
MSE | 0.0027 | 544*10−6 | 549*10−6 | 541*10−6 | 554*10−6 | 547*10−6 | |||
2 | AE | 0.6495 | 0.6579 | 0.6571 | 0.6586 | 0.6567 | 0.6575 | ||
MSE | 0.0025 | 532*10−6 | 535*10−6 | 530*10−6 | 539*10−6 | 534*10−6 | |||
3 | AE | 0.6496 | 0.6571 | 0.6564 | 0.6579 | 0.6560 | 0.6568 | ||
MSE | 0.0022 | 533*10−6 | 537*10−6 | 530*10−6 | 542*10−6 | 536*10−6 | |||
(60, 50, 30) | 1 | AE | 0.6494 | 0.6580 | 0.6572 | 0.6587 | 0.6568 | 0.6576 | |
MSE | 0.0026 | 532*10−6 | 535*10−6 | 530*10−6 | 539*10−6 | 534*10−6 | |||
2 | AE | 0.6485 | 0.6574 | 0.6566 | 0.6581 | 0.6562 | 0.6570 | ||
MSE | 0.0025 | 524*10−6 | 528*10−6 | 522*10−6 | 533*10−6 | 527*10−6 | |||
3 | AE | 0.6498 | 0.6572 | 0.6565 | 0.6580 | 0.6561 | 0.6569 | ||
MSE | 0.0022 | 527*10−6 | 531*10−6 | 523*10−6 | 535*10−6 | 529*10−6 | |||
0.5 | (60, 40, 25) | 1 | ES | 0.2057 | 0.2069 | 0.2059 | 0.2079 | 0.2020 | 0.2052 |
MSE | 0.0026 | 658*10−6 | 647*10−6 | 671*10−6 | 651*10−6 | 650*10−6 | |||
2 | AE | 0.2072 | 0.2073 | 0.2063 | 0.2082 | 0.2024 | 0.2056 | ||
MSE | 0.0024 | 662*10−6 | 651*10−6 | 676*10−6 | 652*10−6 | 654*10−6 | |||
3 | AE | 0.2077 | 0.2066 | 0.2057 | 0.2076 | 0.2020 | 0.2051 | ||
MSE | 0.0019 | 642*10−6 | 632*10−6 | 654*10−6 | 639*10−6 | 636*10−6 | |||
(60, 40, 30) | 1 | AE | 0.2063 | 0.2072 | 0.2062 | 0.2082 | 0.2022 | 0.2056 | |
MSE | 0.0025 | 648*10−6 | 636*10−6 | 662*10−6 | 638*10−6 | 639*10−6 | |||
2 | AE | 0.2065 | 0.2070 | 0.2060 | 0.2080 | 0.2021 | 0.2054 | ||
MSE | 0.0023 | 650*10−6 | 639*10−6 | 663*10−6 | 642*10−6 | 642*10−6 | |||
3 | AE | 0.2074 | 0.2065 | 0.2055 | 0.2074 | 0.2019 | 0.2050 | ||
MSE | 0.0019 | 645*10−6 | 636*10−6 | 657*10−6 | 644*10−6 | 640*10−6 | |||
(60, 50, 25) | 1 | AE | 0.2047 | 0.2062 | 0.2053 | 0.2071 | 0.2019 | 0.2048 | |
MSE | 0.0021 | 659*10−6 | 651*10−6 | 671*10−6 | 657*10−6 | 654*10−6 | |||
2 | AE | 0.2065 | 0.2072 | 0.2063 | 0.2081 | 0.2028 | 0.2057 | ||
MSE | 0.0021 | 656*10−6 | 645*10−6 | 668*10−6 | 645*10−6 | 648*10−6 | |||
3 | AE | 0.2059 | 0.2062 | 0.2054 | 0.2071 | 0.2021 | 0.2049 | ||
MSE | 0.0017 | 641*10−6 | 632*10−6 | 651*10−6 | 638*10−6 | 636*10−6 | |||
(60, 50, 30) | 1 | AE | 0.2065 | 0.2073 | 0.2064 | 0.2082 | 0.2029 | 0.2058 | |
MSE | 0.0021 | 655*10−6 | 644*10−6 | 667*10−6 | 642*10−6 | 646*10−6 | |||
2 | AE | 0.2055 | 0.2066 | 0.2057 | 0.2075 | 0.2023 | 0.2052 | ||
MSE | 0.0020 | 640*10−6 | 631*10−6 | 652*10−6 | 634*10−6 | 634*10−6 | |||
3 | AE | 0.2061 | 0.2063 | 0.2055 | 0.2072 | 0.2022 | 0.2049 | ||
MSE | 0.0017 | 632*10−6 | 624*10−6 | 642*10−6 | 629*10−6 | 627*10−6 |
t | (n,m,k) | Scheme | ˆℏML | ˆℏBS | ˆℏBL | ˆℏBL | ˆℏBG | ˆℏBG | |
(h=1.5) | (h=−1.5) | (c=0.5) | (c=−0.5) | ||||||
0.1 | (60, 40, 25) | 1 | AE | 3.9493 | 3.8496 | 3.7028 | 4.0153 | 3.8095 | 3.8362 |
MSE | 0.5200 | 0.0999 | 0.1028 | 0.1507 | 0.09823 | 0.09896 | |||
2 | AE | 3.9209 | 3.8441 | 3.6997 | 4.0068 | 3.8048 | 3.8310 | ||
MSE | 0.4718 | 0.0998 | 0.1036 | 0.1477 | 0.0985 | 0.0990 | |||
3 | AE | 3.894 | 3.8490 | 3.7112 | 4.0029 | 3.8116 | 3.8365 | ||
MSE | 0.3638 | 0.0985 | 0.0994 | 0.1446 | 0.0966 | 0.0975 | |||
(60, 40, 30) | 1 | AE | 3.9388 | 3.8450 | 3.6985 | 4.0103 | 3.8050 | 3.8316 | |
MSE | 0.5054 | 0.0972 | 0.1018 | 0.1458 | 0.0960 | 0.0964 | |||
2 | AE | 3.9294 | 3.8472 | 3.7026 | 4.0101 | 3.8078 | 3.8341 | ||
MSE | 0.4694 | 0.0987 | 0.1019 | 0.1475 | 0.0972 | 0.0978 | |||
3 | AE | 3.8991 | 3.8513 | 3.7134 | 4.0054 | 3.8139 | 3.8388 | ||
MSE | 0.3717 | 0.1001 | 0.1001 | 0.1473 | 0.0981 | 0.0991 | |||
(60, 50, 25) | 1 | AE | 3.9448 | 3.8529 | 3.7210 | 3.9997 | 3.8172 | 3.8410 | |
MSE | 0.4297 | 0.1014 | 0.01011 | 0.1444 | 0.0996 | 0.1005 | |||
2 | AE | 3.9165 | 3.8407 | 3.7099 | 3.9861 | 3.8051 | 3.8288 | ||
MSE | 0.4039 | 0.0985 | 0.1015 | 0.1370 | 0.0976 | 0.0978 | |||
3 | AE | 3.9131 | 3.8502 | 3.7248 | 3.9889 | 3.8166 | 3.8389 | ||
MSE | 0.3468 | 0.0993 | 0.0984 | 0.1387 | 0.0975 | 0.0984 | |||
(60, 50, 30) | 1 | AE | 3.9179 | 3.8396 | 3.7085 | 3.9855 | 3.8040 | 3.8277 | |
MSE | 0.4118 | 0.0985 | 0.1019 | 0.1370 | 0.0977 | 0.0980 | |||
2 | AE | 3.9284 | 3.8472 | 3.7161 | 3.9931 | 3.8117 | 3.8354 | ||
MSE | 0.4006 | 0.0974 | 0.0989 | 0.1379 | 0.0961 | 0.0967 | |||
3 | AE | 3.9102 | 3.8490 | 3.7237 | 3.9875 | 3.8151 | 3.8377 | ||
MSE | 0.3435 | 0.0980 | 0.0976 | 0.1369 | 0.0964 | 0.0972 | |||
0.5 | (60, 40, 25) | 1 | AE | 2.4150 | 2.3035 | 2.2597 | 2.3499 | 2.2841 | 2.2971 |
MSE | 0.0613 | 0.02778 | 0.0274 | 0.0325 | 0.0276 | 0.0276 | |||
2 | AE | 2.2319 | 2.3007 | 2.2573 | 2.3465 | 2.2814 | 2.2943 | ||
MSE | 0.0579 | 0.0276 | 0.0275 | 0.0320 | 0.0275 | 0.0275 | |||
3 | AE | 2.2257 | 2.3039 | 2.2619 | 2.3482 | 2.2853 | 2.2977 | ||
MSE | 0.0452 | 0.0270 | 0.0264 | 0.0315 | 0.0267 | 0.0268 | |||
(60, 40, 30) | 1 | AE | 2.2385 | 2.3012 | 2.2574 | 2.3474 | 2.2818 | 2.2947 | |
MSE | 0.0609 | 0.0271 | 0.0270 | 0.0315 | 0.0270 | 0.0270 | |||
2 | AE | 2.2353 | 2.3022 | 2.2588 | 2.3481 | 2.2830 | 2.2958 | ||
MSE | 0.0569 | 0.0273 | 0.0270 | 0.03178 | 0.0271 | 0.0272 | |||
3 | AE | 2.2273 | 2.3051 | 2.2630 | 2.3494 | 2.2865 | 2.2989 | ||
MSE | 0.0454 | 0.0273 | 0.0267 | 0.0320 | 0.0270 | 0.0271 | |||
(60, 50, 25) | 1 | AE | 2.2445 | 2.3059 | 2.2664 | 2.3472 | 2.2884 | 2.3001 | |
MSE | 0.0514 | 0.0281 | 0.0275 | 0.0323 | 0.0279 | 0.0280 | |||
2 | AE | 2.2357 | 2.2996 | 2.2604 | 2.3407 | 2.2822 | 2.2938 | ||
MSE | 0.0508 | 0.0274 | 0.0273 | 0.0310 | 0.02736 | 0.2733 | |||
3 | AE | 2.2353 | 2.3048 | 2.2669 | 2.3446 | 2.2881 | 2.2993 | ||
MSE | 0.0424 | 0.0273 | 0.0266 | 0.0313 | 0.0270 | 0.0272 | |||
(60, 50, 30) | 1 | AE | 2.2352 | 2.2988 | 2.2596 | 2.3399 | 2.2814 | 2.2930 | |
MSE | 0.0511 | 0.0274 | 0.0273 | 0.0391 | 0.0274 | 0.0273 | |||
2 | AE | 2.240 | 2.3031 | 2.2638 | 2.3443 | 2.2857 | 2.293 | ||
MSE | 0.0490 | 0.0271 | 0.0267 | 0.0309 | 0.0269 | 0.0270 | |||
3 | AE | 2.2344 | 2.3042 | 2.2663 | 2.3439 | 2.2875 | 2.2987 | ||
MSE | 0.0422 | 0.0270 | 0.0263 | 0.0309 | 0.0267 | 0.0269 |
m | k | Scheme | ACI | Boot-p | Gamma prior | |||
Boot-p | Boot-t | BCI | HPD | |||||
40 | 25 | 1 | α1 | 1.397(0.9959) | 0.429(0.9300) | 0.747(0.8784) | 0.518(0.9912) | 0.506(0.9858) |
α2 | 2.873(0.9995) | 0.440(0.9322) | 0.732(0.8796) | 0.6022(0.9983) | 0.593(0.9971) | |||
β | 5.322(1.0000) | 1.146(0.9446) | 1.473(0.9846) | 0.776(1.0000) | 0.759(1.0000) | |||
2 | α1 | 1.846(0.9980) | 0.421(0.9371) | 0.668(0.8726) | 0.515(0.9930) | 0.504(0.9885) | ||
α2 | 2.717(0.9999) | 0.430(0.9375) | 0.650(0.8786) | 0.601(0.9990) | 0.592(0.9978) | |||
β | 4.891(0.9999) | 1.081(0.9407) | 1.270(0.9654) | 0.768(1.0000) | 0.752(1.0000) | |||
3 | α1 | 1.857(0.9987) | 0.369(0.9393) | 0.579(0.8798) | 0.480(0.9913) | 0.470(0.9870) | ||
α2 | 2.760(0.9999) | 0.376(0.9413) | 0.570(0.8879) | 0.572(0.9986) | 0.563(0.9972) | |||
β | 5.143(1.0000) | 0.939(0.9389) | 1.202(0.9511) | 0.760(1.0000) | 0.743(1.0000) | |||
30 | 1 | α1 | 1.818(0.9962) | 0.414(0.9328) | 0.774(0.8922) | 0.510(0.9914) | 0.499(0.9860) | |
α2 | 2.695(0.9996) | 0.427(0.9365) | 0.789(0.9088) | 0.594(0.9978) | 0.586(0.9963) | |||
β | 5.145(0.9997) | 1.1435(0.9446) | 1.700(0.9626) | 0.775(1.0000) | 0.758(1.0000) | |||
2 | α1 | 1.839(0.9968) | 0.410(0.9291) | 0.726(0.8944) | 0.512(0.992) | 0.500(0.9882) | ||
α2 | 2.688(0.9999) | 0.421(0.9318) | 0.729(0.9079) | 0.597(0.9987) | 0.588(0.9974) | |||
β | 4.926(0.9999) | 1.078(0.9460) | 1.466(0.9733) | 0.768(1.0000) | 0.751(1.000) | |||
3 | α1 | 1.892(0.9992) | 0.370(0.9386) | 0.588(0.8815) | 0.480(0.9910) | 0.470(0.9870) | ||
α2 | 2.793(1.0000) | 0.376(0.9376) | 0.578(0.8830) | 0.571(0.9985) | 0.563(0.9975) | |||
β | 5.221(1.0000) | 0.941(0.9409) | 1.221(0.9536) | 0.760(1.0000) | 0.743(1.0000) | |||
50 | 25 | 1 | α1 | 1.619(0.9981) | 0.391(0.9363) | 0.581(0.8675) | 0.489(0.9890) | 0.479(0.9856) |
α2 | 2.394(0.9995) | 0.400(0.9385) | 0.571(0.8754) | 0.577(0.9983) | 0.569(0.9973) | |||
β | 4.464(1.0000) | 1.020(0.9438) | 1.185(0.9541) | 0.767(1.0000) | 0.751(1.0000) | |||
2 | α1 | 1.628(0.9976) | 0.389(0.9340) | 0.582(0.8676) | 0.488(0.9894) | 0.478(0.856) | ||
α2 | 2.395(1.0000) | 0.398(0.9361) | 0.572(0.8740) | 0.577(0.9978) | 0.568(0.9955) | |||
β | 4.424(1.0000) | 1.007(0.9430) | 1.171(0.9534) | 0.765(1.0000) | 0.749(1.0000) | |||
3 | α1 | 1.449(0.9979) | 0.358(0.9359) | 0.505(0.8711) | 0.466(0.9902) | 0.456(0.9868) | ||
α2 | 2.144(1.0000) | 0.367(0.9360) | 0.501(0.8799) | 0.557(0.9986) | 0.549(0.9971) | |||
β | 4.009(1.0000) | 0.923(0.9399) | 1.051(0.9533) | 0.759(1.0000) | 0.742(1.0000) | |||
30 | 1 | α1 | 1.644(0.9982) | 0.389(0.9387) | 0.594(0.8773) | 0.489(0.9920) | 0.478(0.9866) | |
α2 | 2.400(1.0000) | 0.398(0.9385) | 0.587(0.8849) | 0.578(0.9977) | 0.569(0.9967) | |||
β | 4.544(1.0000) | 1.020(0.9456) | 1.220(0.9776) | 0.768(1.0000) | 0.751(1.0000) | |||
2 | α1 | 1.612(0.9979) | 0.387(0.9337) | 0.583(0.8728) | 0.489(0.9921) | 0.479(0.9876) | ||
α2 | 2.384(0.9998) | 0.397(0.9356) | 0.576(0.8803) | 0.577(0.9982) | 0.569(0.9967) | |||
β | 4.411(1.0000) | 1.01(0.9437) | 1.177(0.9727) | 0.766(1.0000) | 0.749(1.0000) | |||
3 | α1 | 1.451(0.9984) | 0.358(0.9365) | 0.503(0.8755) | 0.465(0.9908) | 0.455(0.9860) | ||
α2 | 2.124(0.9998) | 0.367(0.9390) | 0.499(0.8738) | 0.557(0.9986) | 0.549(0.9970) | |||
β | 3.983(1.0000) | 0.922(0.9409) | 1.042(0.9564) | 0.759(1.0000) | 0.743(1.0000) |
m | k | Scheme | ACI | Boot-p | Gamma prior | |||
Boot-p | Boot-t | BCI | HPD | |||||
40 | 20 | 1 | ℜ(0.1) | 0.0022(0.9850) | 0.0014(0.9532) | 0.2626(1.0000) | 0.00092(0.986) | 0.00090(0.9846) |
ℜ(0.5) | 0.1847(0.9822) | 0.1266(0.9531) | 0.2838(0.9999) | 0.08888(0.9842) | 0.08808(0.9824) | |||
ℏ(0.1) | 0.0654(0.9850) | 0.0419(0.9532) | 0.0510(0.9274) | 0.0275(0.9860) | 0.0271(0.9846) | |||
ℏ(0.5) | 1.0230(0.9758) | 0.7854(0.9537) | 0.9281(0.9389) | 0.5683(0.9832) | 0.5620(0.9804) | |||
2 | ℜ(0.1) | 0.00194(0.9815) | 0.0013(0.9494) | 0.2622(1.0000) | 0.00088(0.9827) | 0.00087(0.9808) | ||
ℜ(0.5) | 0.1635(0.9760) | 0.1198(0.9497) | 0.2862(0.9999) | 0.0861(0.9803) | 0.0854(0.9787) | |||
ℏ(0.1) | 0.0583(0.9815) | 0.0395(0.9494) | 0.0444(0.9246) | 0.0266(0.9827) | 0.0262(0.9806) | |||
ℏ(0.5) | 0.8977(0.9641) | 0.7428(0.9502) | 0.8107(0.9355) | 0.5504(0.9795) | 0.5547(0.9774) | |||
3 | ℜ(0.1) | 0.0020(0.9836) | 0.0012(0.9441) | 0.2108(1.0000) | 0.00081(0.9786) | 0.00080(0.9758) | ||
ℜ(0.5) | 0.1651(0.9810) | 0.1058(0..9944) | 0.2329(0.9998) | 0.0786(0.9766) | 0.07803(0.9727) | |||
ℏ(0.1) | 0.0599(0.6836) | 0.0345(0.9441) | 0.0413(0.9086) | 0.0243(0.9786) | 0.0240(0.9757) | |||
ℏ(0.5) | 0.8805(0.9692) | 0.6555(0.9442) | 0.7536(0.9231) | 0.5012(0.9750) | 0.4966(0.9700) | |||
30 | 1 | ℜ(0.1) | 0.00226(0.9776) | 0.0014(0.9522) | 0.2685(1.0000) | 0.00091(0.9842) | 0.00090(0.9813) | |
ℜ(0.5) | 0.1911(0.9745) | 0.1273(0.9526) | 0.2858(1.0000) | 0.0881(0.9829) | 0.0873(0.9793) | |||
ℏ(0.1) | 0.0676(0.9776) | 0.0422(0.9522) | 0.0534(0.9334) | 0.0272(0.9843) | 0.0269(0.9814) | |||
ℏ(0.5) | 1.0587(0.9674) | 0.7911(0.9531) | 0.9533(0.9390) | 0.5629(0.9803) | 0.5568(0.9770) | |||
2 | ℜ(0.1) | 0.0202(0.9816) | 0.0013(0.9534) | 0.2659(1.0000) | 0.00088(0.9839) | 0.00087(0.9825) | ||
ℜ(0.5) | 0.1694(0.9787) | 0.1200(0.9538) | 0.2864(0.9999) | 0.0859(0.9823) | 0.0852(0.9807) | |||
ℏ(0.1) | 0.0606(0.9816) | 0.0396(0.9534) | 0.0462(0.9452) | 0.0265(0.9839) | 0.0262(0.9824) | |||
ℏ(0.5) | 0.9274(0.9711) | 0.7448(0.9541) | 0.8259(0.9475) | 0.5494(0.9811) | 0.5438(0.9784) | |||
3 | ℜ(0.1) | 0.0020(0.9821) | 0.0012(0.9457) | 0.2105(1.0000) | 0.00081(0.9782) | 0.00080(0.9762) | ||
ℜ(0.5) | 0.1655(0.9783) | 0.1060(0.9458) | 0.2325(0.9998) | 0.0787(0.9767) | 0.0781(0.9749) | |||
ℏ(0.1) | 0.0599(0.9821) | 0.0346(0.9457) | 0.0418(0.9099) | 0.0243(0.9782) | 0.0240(0.9760) | |||
ℏ(0.5) | 0.8836(0.9688) | 0.6567(0.9469) | 0.7617(0.9259) | 0.5015(0.9750) | 0.4970(0.9728) | |||
50 | 20 | 1 | ℜ(0.1) | 0.00212(0.9834) | 0.0012(0.9475) | 0.2359(1.0000) | 0.00085(0.9810) | 0.00084(0.9802) |
ℜ(0.5) | 0.1800(0.9786) | 0.1145(0.9478) | 0.2558(0.9999) | 0.0822(0.9794) | 0.0816(0.9773) | |||
ℏ(0.1) | 0.00634(0.9833) | 0.0374(0.9475) | 0.0431(0.9198) | 0.0254(0.9809) | 0.0251(0.9802) | |||
ℏ(0.5) | 0.9915(0.9713) | 0.7097(0.9475) | 0.8001(0.9300) | 0.5242(0.9782) | 0.5191(0.9764) | |||
2 | ℜ(0.1) | 0.00191(0.9841) | 0.0012(0.9478) | 0.2373(1.0000) | 0.00084(0.9846) | 0.00083(0.9810) | ||
ℜ(0.5) | 0.1624(0.9807) | 0.1130(0.9477) | 0.2576(0.9998) | 0.0818(0.9830) | 0.0811(0.9797) | |||
ℏ(0.1) | 0.0057(0.9841) | 0.0369(0.9478) | 0.0425(0.9176) | 0.0253(0.9845) | 0.0250(0.9809) | |||
ℏ(0.5) | 0.8979(0.9705) | 0.7006(0.9476) | 0.7885(0.9296) | 0.5213(0.9802) | 0.5162(0.9770) | |||
3 | ℜ(0.1) | 0.00174(0.9833) | 0.0011(0.9438) | 0.2133(1.0000) | 0.00079(0.9799) | 0.00078(0.9770) | ||
ℜ(0.5) | 0.1491(0.9792) | 0.1046(0.9437) | 0.2315(0.9996) | 0.0769(0.9772) | 0.0764(0.9743) | |||
ℏ(0.1) | 0.00522(0.9833) | 0.03 40(0.9437) | 0.0383(0.9219) | 0.0238(0.9800) | 0.0235(0.9770) | |||
ℏ(0.5) | 0.8271(0.9704) | 0.6479(0.9442) | 0.7176(0.9333) | 0.4891(0.9748) | 0.4847(0.9727) | |||
30 | 1 | ℜ(0.1) | 0.00194(0.9871) | 0.0012(0.9500) | 0.2326(1.0000) | 0.00085(0.9824) | 0.0008(0.9810) | |
ℜ(0.5) | 0.1647(0.9836) | 0.1143(0.9503) | 0.2523(1.0000) | 0.0823(0.9809) | 0.0816(0.9783) | |||
ℏ(0.1) | 0.0058(0.9871) | 0.0375(0.9500) | 0.0435(0.9255) | 0.0254(0.9825) | 0.0251(0.9806) | |||
ℏ(0.5) | 0.9127(0.9751) | 0.7085(0.9504) | 0.8013(0.9346) | 0.5247(0.9793) | 0.5195(0.9764) | |||
2 | ℜ(0.1) | 0.00188(0.9874) | 0.0012(0.9488) | 0.2342(1.0000) | 0.00084(0.9823) | 0.00083(0.9786) | ||
ℜ(0.5) | 0.1598(0.9838) | 0.1128(0.9490) | 0.2543(0.9997) | 0.0818(0.9799) | 0.0811(0.9779) | |||
ℏ(0.1) | 0.0056(0.9874) | 0.0369(0.9488) | 0.0419(0.9219) | 0.0253(0.9822) | 0.0250(0.9786) | |||
ℏ(0.5) | 0.8855(0.9737) | 0.6994(0.9490) | 0.7768(0.9334) | 0.5213(0.9789) | 0.5161(0.9764) | |||
3 | ℜ(0.1) | 0.00176(0.9851) | 0.0113(0.9472) | 0.2129(1.0000) | 0.00079(0.9820) | 0.00078(0.9806) | ||
ℜ(0.5) | 0.1506(0.9824) | 0.1044(0.9473) | 0.2312(0.9999) | 0.0771(0.9800) | 0.0765(0.9783) | |||
ℏ(0.1) | 0.0528(0.9851) | 0.0340(0.9472) | 0.0380(0.9230) | 0.0239(0.9820) | 0.0236(0.9806) | |||
ℏ(0.5) | 0.8350(0.9735) | 0.6468(0.9472) | 0.7132(0.9331) | 0.4903(0.9785) | 0.4857(0.9758) |
τ=1.2 | ACI | Boot-p | Gamma prior | ||||||||||||||
Scheme | Boot-p | Boot-t | BCI | HPD | |||||||||||||
(m,k) | α1 | α2 | β | α1 | α2 | β | α1 | α2 | β | α1 | α2 | β | α1 | α2 | β | ||
(40, 25) | 1 | AW | 2.686 | 4.883 | 8.725 | 0.684 | 0.709 | 1.861 | 1.050 | 1.032 | 2.323 | 0.627 | 0.722 | 0.812 | 0.620 | 0.718 | 0.799 |
CP | 0.9962 | 0.9998 | 1.0000 | 0.9374 | 0.9388 | 0.9452 | 0.8704 | 0.8798 | 0.9502 | 0.9968 | 0.9995 | 1.0000 | 0.9954 | 0.9995 | 1.0000 | ||
2 | AW | 2.835 | 5.179 | 8.895 | 0.680 | 0.704 | 1.785 | 1.088 | 1.072 | 2.316 | 0.627 | 0.722 | 0.808 | 0.619 | 0.717 | 0.795 | |
CP | 0.9968 | 1.0000 | 1.0000 | 0.9418 | 0.9438 | 0.9444 | 0.8802 | 0.8896 | 0.9488 | 0.9958 | 0.9998 | 1.0000 | 0.9938 | 0.9995 | 1.0000 | ||
3 | AW | 5.360 | 10.057 | 16.591 | 0.658 | 0.663 | 1.618 | 1.111 | 1.026 | 2.192 | 0.622 | 0.721 | 0.800 | 0.614 | 0.716 | 0.783 | |
CP | 0.9998 | 1.0000 | 1.0000 | 0.9416 | 0.9412 | 0.9422 | 0.8930 | 0.9104 | 0.9506 | 0.9951 | 0.9998 | 1.0000 | 0.9944 | 0.9998 | 1.0000 | ||
(40, 30) | 1 | AW | 2.657 | 4.871 | 8.665 | 0.684 | 0.708 | 1.860 | 1.046 | 1.026 | 2.307 | 0.627 | 0.722 | 0.812 | 0.619 | 0.717 | 0.799 |
CP | 0.9964 | 1.0000 | 1.0000 | 0.9330 | 0.9402 | 0.9438 | 0.8742 | 0.8860 | 0.9492 | 0.99665 | 0.9998 | 1.0000 | 0.9956 | 0.9995 | 1.0000 | ||
2 | AW | 2.827 | 5.199 | 8.986 | 0.679 | 0.703 | 1.796 | 1.093 | 1.077 | 2.345 | 0.626 | 0.723 | 0.808 | 0.618 | 0.718 | 0.795 | |
CP | 0.9976 | 1.0000 | 1.0000 | 0.9346 | 0.9406 | 0.9450 | 0.8732 | 0.8924 | 0.9588 | 0.9948 | 0.9999 | 1.0000 | 0.9947 | 0.9998 | 1.0000 | ||
3 | AW | 5.408 | 10.001 | 16.559 | 0.659 | 0.664 | 1.621 | 1.113 | 1.031 | 2.190 | 0.622 | 0.721 | 0.799 | 0.614 | 0.716 | 0.783 | |
CP | 0.9994 | 1.0000 | 1.0000 | 0.9388 | 0.9426 | 0.9400 | 0.8946 | 0.9132 | 0.9458 | 0.9956 | 0.9999 | 1.0000 | 0.9935 | 0.9999 | 1.0000 | ||
(50, 25) | 1 | AW | 2.182 | 3.971 | 8.703 | 0.616 | 0.639 | 1.642 | 0.933 | 0.932 | 2.101 | 0.596 | 0.700 | 0.804 | 0.589 | 0.695 | 0.791 |
CP | 0.9958 | 1.0000 | 1.000 | 0.9416 | 0.9374 | 0.9528 | 0.8904 | 0.8946 | 0.9564 | 0.9947 | 0.9994 | 1.0000 | 0.9929 | 0.9989 | 1.0000 | ||
2 | AW | 2.209 | 4.016 | 8.640 | 0.615 | 0.638 | 1.627 | 0.870 | 0.866 | 1.934 | 0.595 | 0.698 | 0.802 | 0.588 | 0.694 | 0.790 | |
CP | 0.9952 | 1.0000 | 1.0000 | 0.9404 | 0.9410 | 0.9488 | 0.8830 | 0.8932 | 0.9542 | 0.9953 | 0.9998 | 1.0000 | 0.9931 | 0.9996 | 1.0000 | ||
3 | AW | 2.809 | 5.189 | 10.893 | 0.595 | 0.608 | 1.512 | 0.922 | 0.900 | 1.983 | 0.589 | 0.695 | 0.796 | 0.582 | 0.691 | 0.783 | |
CP | 0.9976 | 1.0000 | 1.0000 | 0.9490 | 0.9468 | 0.9518 | 0.9042 | 0.9094 | 0.9538 | 0.9941 | 0.9996 | 1.0000 | 0.9919 | 0.9995 | 1.0000 | ||
(50, 30) | 1 | AW | 2.195 | 4.008 | 7.847 | 0.616 | 0.639 | 1.640 | 0.909 | 0.905 | 2.041 | 0.594 | 0.699 | 0.804 | 0.588 | 0.694 | 0.791 |
CP | 0.9957 | 1.000 | 1.000 | 0.938 | 0.939 | 0.956 | 0.884 | 0.888 | 0.952 | 0.9942 | 0.9994 | 1.0000 | 0.9923 | 0.9991 | 1.0000 | ||
2 | AW | 2.196 | 3.957 | 8.589 | 0.616 | 0.638 | 1.633 | 0.894 | 0.891 | 2.009 | 0.596 | 0.699 | 0.803 | 0.589 | 0.694 | 0.790 | |
CP | 0.9957 | 1.0000 | 1.0000 | 0.9478 | 0.9458 | 0.9440 | 0.8900 | 0.8928 | 0.9486 | 0.9952 | 1.0000 | 1.0000 | 0.9935 | 1.0000 | 1.0000 | ||
3 | AW | 2.812 | 5.191 | 10.943 | 0.595 | 0.607 | 1.527 | 0.913 | 0.891 | 1.992 | 0.589 | 0.695 | 0.797 | 0.582 | 0.691 | 0.783 | |
CP | 0.9979 | 1.0000 | 1.0000 | 0.9420 | 0.9446 | 0.9440 | 0.8946 | 0.9096 | 0.9546 | 0.9939 | 0.9996 | 1.0000 | 0.9909 | 0.9996 | 1.0000 |
n | m | k | Scheme | ACI | Boot-p | Gamma prior | |||
Boot-p | Boot-t | BCI | HPD | ||||||
60 | 40 | 25 | 1 | ℜ(0.1) | 0.296(0.9735) | 0.216(0.9516) | 0.268(0.9806) | 0.131(0.9956) | 0.131(0.9945) |
ℜ(0.5) | 0.215(0.9642) | 0.198(0.9506) | 0.284(0.9958) | 0.143(0.9953) | 0.142(0.9950) | ||||
ℏ(0.1) | 3.049(0.9569) | 2.807(0.9518) | 2.923(0.9356) | 1.777(0.9954) | 1.765(0.9936) | ||||
ℏ(0.5) | 3.083(0.9956) | 0.994(0.9498) | 1.304(0.8706) | 0.957(0.9959) | 0.952(0.9949) | ||||
2 | ℜ(0.1) | 0.259(0.9682) | 0.208(0.9498) | 0.276(0.9840) | 0.130(0.9929) | 0.130(0.9916) | |||
ℜ(0.5) | 0.223(0.9737) | 0.190(0.9498) | 0.267(0.9920) | 0.142(0.9925) | 0.141(0.9921) | ||||
ℏ(0.1) | 2.758(0.9559) | 2.702(0.9494) | 2.723(0.9384) | 1.761(0.9925) | 1.750(0.9912) | ||||
ℏ(0.5) | 3.141(0.9962) | 0.959(0.9484) | 1.325(0.8786) | 0.952(0.9943) | 0.947(0.9922) | ||||
3 | ℜ(0.1) | 0.252(0.9771) | 0.188(0.9456) | 0.251(0.9838) | 0.127(0.9941) | 0.126(0.9932) | |||
ℜ(0.5) | 0.356(0.9941) | 0.169(0.947) | 0.177(0.9446) | 0.138(0.9942) | 0.137(0.9934) | ||||
ℏ(0.1) | 2.618(0.9717) | 2.436(0.9454) | 2.267(0.9562) | 1.716(0.9941) | 1.706(0.9925) | ||||
ℏ(0.5) | 6.124(0.9997) | 0.840(0.9470) | 1.330(0.8970) | 0.936(0.9955) | 0.931(0.9943) | ||||
30 | 1 | ℜ(0.1) | 0.286(0.9755) | 0.216(0.9460) | 0.267(0.9798) | 0.131(0.9951) | 0.131(0.9945) | ||
ℜ(0.5) | 0.212(0.9683) | 0.198(0.9468) | 0.283(0.9932) | 0.143(0.9947) | 0.142(0.9946) | ||||
ℏ(0.1) | 3.008(0.9610) | 2.806(0.9456) | 2.913(0.9374) | 1.775(0.9947) | 1.763(0.9940) | ||||
ℏ(0.5) | 2.913(0.9956) | 0.994(0.9480) | 1.295(0.8764) | 0.956(0.9956) | 0.951(0.9942) | ||||
2 | ℜ(0.1) | 0.260(0.9686) | 0.208(0.9464) | 0.275(0.9864) | 0.130(0.9961) | 0.130(0.9953) | |||
ℜ(0.5) | 0.224(0.9770) | 0.190(0.9470) | 0.266(0.9936) | 0.142(0.9959) | 0.141(0.9955) | ||||
ℏ(0.1) | 2.764(0.9578) | 2.713(0.9462) | 2.732(0.9470) | 1.762(0.9961) | 1.751(0.9944) | ||||
ℏ(0.5) | 3.176(0.9975) | 0.959(0.9480) | 1.334(0.8810) | 0.953(0.9966) | 0.947(0.9955) | ||||
3 | ℜ(0.1) | 0.252(0.9734) | 0.188(0.9442) | 0.252(0.9834) | 0.127(0.9928) | 0.126(0.9912) | |||
ℜ(0.5) | 0.358(0.9935) | 0.169(0.9478) | 0.177(0.9432) | 0.138(0.9928) | 0.137(0.9918) | ||||
ℏ(0.1) | 2.629(0.9714) | 2.440(0.9462) | 2.263(0.9564) | 1.717(0.9929) | 1.706(0.9913) | ||||
ℏ(0.5) | 6.180(0.9999) | 0.841(0.9500) | 1.332(0.8970) | 0.936(0.9946) | 0.931(0.9929) | ||||
50 | 25 | 1 | ℜ(0.1) | 0.258(0.9718) | 0.194(0.9558) | 0.238(0.9852) | 0.124(0.9920) | 0.124(0.9905) | |
ℜ(0.5) | 0.189(0.9611) | 0.178(0.9568) | 0.257(0.9962) | 0.135(0.9909) | 0.134(0.9897) | ||||
ℏ(0.1) | 2.705(0.9553) | 2.515(0.9548) | 2.661(0.9480) | 1.679(0.9915) | 1.669(0.9903) | ||||
ℏ(0.5) | 2.590(0.9947) | 0.899(0.9580) | 1.181(0.8898) | 0.906(0.9924) | 0.9015(0.9915) | ||||
2 | ℜ(0.1) | 0.253(0.9745) | 0.192(0.9532) | 0.239(0.9826) | 0.124(0.9930) | 0.123(0.992) | |||
ℜ(0.5) | 0.191(0.9660) | 0.177(0.9522) | 0.254(0.9962) | 0.135(0.9923) | 0.134(0.9915) | ||||
ℏ(0.1) | 2.661(0.9574) | 2.494(0.9526) | 2.556(0.9434) | 1.671(0.9923) | 1.661(0.9914) | ||||
ℏ(0.5) | 2.613(0.9965) | 0.892(0.9520) | 1.102(0.887) | 0.903(0.9935) | 0.898(0.9925) | ||||
3 | ℜ(0.1) | 0.246(0.9780) | 0.179(0.9532) | 0.222(0.9814) | 0.121(0.9897) | 0.120(0.9891) | |||
ℜ(0.5) | 0.212(0.9803) | 0.163(0.9532) | 0.213(0.9910) | 0.131(0.9896) | 0.130(0.9881) | ||||
ℏ(0.1) | 2.417(0.9634) | 2.315(0.9532) | 2.283(0.9572) | 1.634(0.9896) | 1.624(0.9892) | ||||
ℏ(0.5) | 3.486(0.9974) | 0.817(0.9520) | 1.206(0.8836) | 0.888(0.9918) | 0.884(0.9909) | ||||
30 | 1 | ℜ(0.1) | 0.259(0.9744) | 0.194(0.9530) | 0.238(0.9836) | 0.124(0.9925) | 0.123(0.9913) | ||
ℜ(0.5) | 0.190(0.9643) | 0.178(0.9532) | 0.257(0.9964) | 0.135(0.9917) | 0.134(0.9922) | ||||
ℏ(0.1) | 2.704(0.9590) | 2.512(0.9526) | 2.637(0.9448) | 1.674(0.9918) | 1.664(0.9898) | ||||
ℏ(0.5) | 2.593(0.9965) | 0.899(0.9572) | 1.154(0.8874) | 0.904(0.9930) | 0.899(0.9924) | ||||
2 | ℜ(0.1) | 0.252(0.9729) | 0.193(0.9462) | 0.240(0.9790) | 0.124(0.9929) | 0.123(0.9918) | |||
ℜ(0.5) | 0.190(0.9651) | .176(0.9472) | 0.254(0.9948) | 0.134(0.9919) | 0.134(0.9922) | ||||
ℏ(0.1) | 2.655(0.9590) | 2.501(0.9464) | 2.587(0.9402) | 1.674(0.9919) | 1.664(0.9908) | ||||
ℏ(0.5) | 2.600(0.9969) | 0.893(0.9472) | 1.134(0.8878) | 0.904(0.9931) | 0.900(0.9922) | ||||
3 | ℜ(0.1) | 0.246(0.9771) | 0.180(0.9474) | 0.222(0.9790) | 0.121(0.9924) | 0.120(0.9917) | |||
ℜ(0.5) | 0.213(0.9840) | 0.162(0.9478) | 0.213(0.9890) | 0.131(0.9915) | 0.130(0.9909) | ||||
ℏ(0.1) | 2.422(0.9651) | 2.328(0.9478) | 2.292(0.9580) | 1.633(0.9918) | 1.624(0.9909) | ||||
ℏ(0.5) | 3.503(0.9985) | 0.817(0.9494) | 1.198(0.8872) | 0.888(0.9933) | 0.883(0.9922) |
Cases | ML | Bayesian(uniform prior) | |||||
BS | BL | BL | BG | BG | |||
(h=1.5) | (h=−1.5) | (c=0.5) | (c=−0.5) | ||||
1 | ˆα1 | 0.1795(0.1053) | 0.2292(0.1774) | 0.2106(0.1784) | 0.2632(0.1806) | 0.1718(0.1864) | 0.2064(0.1788) |
ˆα2 | 0.2692(0.1500) | 0.3459(0.2598) | 0.3099(0.2623) | 0.4364(0.2751) | 0.2656(0.2719) | 0.3138(0.2617) | |
ˆβ | 0.4985(0.4210) | 0.6279(0.5469) | 0.4872(0.5647) | 1.2542(0.8314) | 0.3796(0.6006) | 0.5372(0.5543) | |
ˆℜ(0.5) | 0.9734(0.0102) | 0.9721(0.0106) | 0.9720(0.0106) | 0.9722(0.0106) | 0.9720(0.0106) | 0.9721(0.0106) | |
ˆℜ(1.5) | 0.6436(0.0583) | 0.6493(0.0566) | 0.6469(0.0567) | 0.6517(0.0567) | 0.6455(0.0568) | 0.6481(0.0567) | |
ˆℏ(0.5) | 0.1568(0.0575) | 0.1627(0.0576) | 0.1603(0.0577) | 0.1653(0.0577) | 0.1491(0.0592) | 0.1581(0.0578) | |
ˆℏ(1.5) | 0.5613(0.1492) | 0.5601(0.4213) | 0.5458(0.1407) | 0.5753(0.1409) | 0.5339(0.1425) | 0.5514(0.1403) | |
ˆπ1 | 0.4(0.0980) | 0.3990(0.0961) | 0.3921(0.0964) | 0.4060(0.0964) | 0.3806(0.0979) | 0.3931(0.0963) | |
ˆπ2 | 0.6(0.0980) | 0.6010(0.0961) | 0.5940(0.0964) | 0.6079(0.0964) | 0.5887(0.0970) | 0.5970(0.0962) | |
2 | ˆα1 | 0.1383(0.0553) | 0.1445(0.0624) | 0.1417(0.0625) | 0.1476(0.0625) | 0.1274(0.0647) | 0.1385(0.0627) |
ˆα2 | 0.2075(0.0754) | 0.2172(0.0856) | 0.2120(0.0857) | 0.2231(0.0858) | 0.1960(0.0881) | 0.2098(0.0859) | |
ˆβ | 0.7013(0.4397) | 0.8856(0.6072) | 0.7113(0.6317) | 1.6297(0.9603) | 0.6585(0.6482) | 0.8034(0.6127) | |
ˆℜ(0.5) | 0.9714(0.0105) | 0.9690(0.0117) | 0.9689(0.0117) | 0.9691(0.0117) | 0.9689(0.0117) | 0.9690(0.0117) | |
ˆℜ(1.5) | 0.6571(0.0558) | 0.6602(0.0548) | .6580(0.0548) | 0.6625(0.0548) | 0.6567(0.0549) | 0.6591(0.0548) | |
ˆℏ(0.5) | 0.1673(0.0587) | 0.1783(0.0625) | 0.1755(0.0626) | 0.1813(0.0626) | 0.1632(0.0643) | 0.1732(0.0627) | |
ˆℏ(1.5) | 0.4863(0.0990) | 0.4757(0.3124) | 0.4690(0.0959) | 0.4827(0.0959) | 0.4612(0.0967) | 0.4709(0.0958) | |
ˆπ1 | 0.4(0.0894) | 0.3993(0.0879) | 0.3935(0.0881) | 0.4051(0.0881) | 0.3841(0.0892) | 0.3943(0.0881) | |
ˆπ2 | 0.6(0.0894) | 0.6007(0.0879) | 0.5949(0.0881) | 0.6065(0.0881) | 0.5905(0.0885) | 0.5974(0.0880) | |
3 | ˆα1 | 0.2099(0.0795) | 0.2217(0.0914) | 0.2158(0.0916) | 0.2285(0.0917) | 0.1982(0.0944) | 0.2134(0.0918) |
ˆα2 | 0.3498(0.1196) | 0.3699(0.1404) | 0.3565(0.1411) | 0.3866(0.1414) | 0.3366(0.1443) | 0.3582(0.1409) | |
ˆβ | 0.3291(0.2020) | 0.4031(0.2896) | 0.3579(0.2931) | 0.5407(0.3206) | 0.3001(0.3073) | 0.3654(0.2920) | |
ˆℜ(0.5) | 0.9777(0.0079) | 0.9762(0.0091) | 0.9761(0.0091) | 0.9762(0.0091) | 0.9761(0.0091) | =0.9761(0.0091) | |
ˆℜ(1.5) | 0.658262(0.0609) | 0.6616(0.0591) | 0.6589(0.0591) | 0.6641(0.0591) | 0.6575(0.0592) | 0.6602(0.0591) | |
ˆℏ(0.5) | 0.1327(0.0464) | 0.1408(0.0513) | 0.1389(0.0514) | 0.1429(0.05138) | 0.1285(0.0528) | 0.1366(0.05151) | |
ˆℏ(1.5) | 0.5891(0.0935) | 0.5715(0.4406) | 0.5651(0.0933) | 0.5781(0.0934) | 0.5601(0.0938) | 0.5677(0.0932) | |
ˆπ1 | 0.375(0.0765) | 0.3751(0.0751) | 0.3709(0.0752) | 0.3794(0.0752) | 0.3634(0.0760) | 0.3713(0.0752) | |
ˆπ2 | 0.625(0.0765) | 0.6249(0.0751) | 0.6206(0.0752) | 0.6291(0.0752) | 0.6178(0.0754) | 0.6226(0.0751) |
Cases | ACI | Boot-p | Uniform prior | |||
Boot-p | Boot-t | BCI | HPD | |||
α1 | 1 | (0.1103, 0.2920) | (0.1876, 0.3355) | (0.1880, 0.2771) | (0.0641, 0.6904) | (0.0419, 0.5590) |
0.1817 | 0.1479 | 0.0891 | 0.6263 | 0.5171 | ||
2 | (0.0300, 0.2467) | (0.1396, 0.2392) | (0.1396, 0.2045) | (0.0595, 0.2999) | (0.0446, 0.2651) | |
0.2167 | 0.0996 | 0.0649 | 0.2405 | 0.2205 | ||
3 | (0.0540, 0.3658) | (0.2108, 0.3104) | (0.2108, 0.2815) | (0.0978, 0.4485) | (0.0794, 0.3995) | |
0.3112 | 0.0996 | 0.0707 | 0.3507 | 0.3201 | ||
α2 | 1 | (0.1528, 0.4744) | (0.2745, 0.3190) | (0.2749, 0.3165) | (0.1071, 1.0452) | (0.0689, 0.8282) |
0.3216 | 0.0445 | 0.0416 | 0.9381 | 0.7593 | ||
2 | (0.0598, 0.3552) | (0.2080, 0.3312) | (0.2080, 0.2932) | (0.0999, 0.4382) | (0.0798, 0.3859) | |
0.2955 | 0.1232 | 0.0852 | 0.3383 | 0.3061 | ||
3 | (0.1154, 0.5843) | (0.3508, 0.4483) | (0.3509, 0.4240) | (0.1181, 0.7192) | (0.1545, 0.6508) | |
0.4689 | 0.0975 | 0.0731 | 0.5383 | 0.4963 | ||
β | 1 | (0.1531, 1.5992) | (0.5314, 0.6447) | (0.5340, 0.6197) | (0.0794, 2.1151) | (0.0382, 1.6710) |
1.4460 | 0.1133 | 0.0857 | 2.0357 | 1.6327 | ||
2 | (0.2506, 1.9626) | (0.7107, 1.1594) | (0.7111, 1.0276) | (0.1973, 2.4641) | (0.1180, 2.0447) | |
1.7120 | 0.4487 | 0.3165 | 2.2668 | 1.9267 | ||
3 | (0.1651, 0.6563) | (0.3317, 0.5494) | (0.3317, 0.4834) | (0.0912, 1.0850) | (0.0535, 0.8972) | |
0.7918 | 0.2177 | 0.1517 | 0.9937 | 0.8437 | ||
ℜ(0.5) | 1 | (0.9535, 0.9933) | (0.9564, 0.9675) | (0.9560, 0.9648) | (0.9458, 0.9866) | (0.9515, 0.9892) |
0.0398 | 0.0111 | 0.0088 | 0.0408 | 0.0377 | ||
2 | (0.9507, 0.9920) | (0.9457, 0.9686) | (0.9438, 0.9683) | (0.9406, 0.9857) | (0.9454, 0.9879) | |
0.4125 | 0.0229 | 0.0245 | 0.0451 | 0.0425 | ||
3 | (0.9621, 0.9933) | (0.9601, 0.9766) | (0.9542, 0.9765) | (0.9550, 0.9888) | (0.9588, 0.9903) | |
0.0312 | 0.0165 | 0.0223 | 0.0339 | 0.0316 | ||
ℜ(1.5) | 1 | (0.5293, 0.7580) | (0.4986, 0.5916) | (0.4986, 0.5899) | (0.5355, 0.7552) | (0.5384, 0.7575) |
0.2287 | 0.0930 | 0.0913 | 0.2197 | 0.2191 | ||
2 | (0.5478, 0.7664) | (0.4970, 0.6376) | (0.4959, 0.6393) | (0.5481, 0.7634) | (0.5538, 0.7676) | |
0.2186 | 0.1406 | 0.1434 | 0.2154 | 0.2138 | ||
3 | (0.5388, 0.7777) | (0.5183, 0.6458) | (0.4863, 0.6464) | (0.54438, 0.7733) | (0.5465, 0.7752) | |
0.2388 | 0.1275 | 0.1601 | 0.2290 | 0.2287 | ||
ℏ(0.5) | 1 | (0.0441, 0.2696) | (0.1917, 0.2586) | (0.1872, 0.2171) | (0.8009, 0.3026) | (0.0655, 0.2749) |
0.2255 | 0.0669 | 0.0299 | 0.2225 | 0.2095 | ||
2 | (0.0521, 0.2824) | (0.1829, 0.3173) | (0.1826, 0.3055) | (0.0850, 0.3272) | (0.0727, 0.3033) | |
0.2303 | 0.1344 | 0.1229 | 0.2422 | 0.2306 | ||
3 | (0.0417, 0.2237) | (0.1392, 0.2381) | (0.1389, 0.2498) | (0.0669, 0.2616) | (0.0580, 0.2401) | |
0.1820 | 0.0989 | 0.1109 | 0.1947 | 0.1821 | ||
ℏ(1.5) | 1 | (0.2689, 0.6740) | (0.6344, 0.8545) | (0.6088, 1.0570) | (0.3206, 0.8656) | (0.0762, 0.2904) |
0.4051 | 0.2201 | 0.4482 | 0.5450 | 0.2142 | ||
2 | (0.2922, 0.6014) | (0.5138, 0.7627) | (0.5268, 0.7798) | (0.3047, 0.6761) | (0.0806, 0.3160) | |
0.3092 | 0.2489 | 0.2530 | 0.3714 | 0.2354 | ||
3 | (0.4059, 0.6801) | (0.6104, 0.8599) | (0.6074, 0.7769) | (0.4019, 0.7659) | (0.0658, 0.2558) | |
0.2742 | 0.2495 | 0.1695 | 0.3640 | 0.1900 | ||
π1 | 1 | (0.2080, 0.5920) | (0.3704, 0.5161) | (0.3670, 0.5423) | (0.2205, 0.5942) | (0.2154, 0.5879) |
0.3841 | 0.1457 | 0.1753 | 0.2908 | 0.3725 | ||
2 | (0.2247, 0.5753) | (0.3143, 0.5000) | (0.2898, 0.5398) | (0.2361, 0.5777) | (0.2273, 0.5677) | |
0.3506 | 0.1857 | 0.2500 | 0.3416 | 0.3404 | ||
3 | (0.2250, 0.5250) | (0.3500, 0.4500) | (0.3450, 0.4626) | (0.2353, 0.5261) | (0.2317, 0.5202) | |
0.3001 | 0.1000 | 0.1176 | 0.3736 | 0.2884 | ||
π2 | 1 | (0.4080, 0.7920) | (0.4839, 0.6296) | (0.4577, 0.6330) | (0.4058, 0.8794) | (0.4121, 0.7846) |
0.3841 | 0.1457 | 0.1753 | 0.2908 | 0.3725 | ||
2 | (0.4247, 0.7754) | (0.5000, 0.6857) | (0.4602, 0.7102) | (0.4219, 0.7639) | (0.4323, 0.7727) | |
0.3506 | 0.1857 | 0.2500 | 0.3420 | 0.3404 | ||
3 | (0.4750, 0.7750) | (0.55, 0.65) | (0.5374, 0.6550) | (0.4739, 0.7647) | (0.4798, 0.7683) | |
0.3001 | 0.1000 | 0.1176 | 0.3736 | 0.2884 |
Cases | ACI | Boot-p | Uniform prior | |||
Boot-p | Boot-t | BCI | HPD | |||
α1 | 1∗ | (0.0456, 0.0820) | (0.0619, 0.1506) | (0.0619, 0.1058) | (0.0163, 0.3172) | (0.0069, 0.2179) |
0.0364 | 0.0887 | 0.0439 | 0.3009 | 0.2110 | ||
2∗ | (0.0025, 0.1348) | (0.0697, 0.1277) | (0.0697, 0.1053) | (0.0245, 0.1804) | (0.0167, 0.1520) | |
0.1323 | 0.058 | 0.0356 | 0.1560 | 0.1353 | ||
3∗ | (0.1101, 0.6418) | (0.2677, 0.5379) | (0.2681, 0.4074) | (0.0755, 2.1628) | (0.0336, 1.6337) | |
0.5316 | 0.2702 | 0.1393 | 2.0873 | 1.6001 | ||
α2 | 1∗ | (0.0659, 0.1276) | (0.0923, 0.1731) | (0.0925, 0.1458) | (0.0292, 0.4916) | (0.0155, 0.3231) |
0.0617 | 0.0808 | 0.0533 | 0.4624 | 0.3077 | ||
2∗ | (0.0050, 0.1495) | (0.0775, 0.1713) | (0.0775, 0.1255) | (0.0285, 0.2011) | (0.0184, 0.1684) | |
0.1445 | 0.0956 | 0.048 | 0.1726 | 0.1500 | ||
3∗ | (0.1268, 0.9028) | (0.3407, 0.6385) | (0.3412, 0.4848) | (0.1029, 2.7150) | (0.0489, 2.0678) | |
0.776 | 0.2978 | 0.1436 | 2.6120 | 2.0189 | ||
β | 1∗ | (0.1191, 20.52) | (1.5804, 4.5806) | (1.5783, 2.9936) | (0.1183, 18.94) | (0.0257, 12.82) |
20.401 | 3.0002 | 1.4153 | 18.8205 | 12.80 | ||
2∗ | (0.1602, 17.6309) | (1.7368, 4.4798) | (1.7507, 2.8638) | (0.2671, 10.846) | (0.0912, 8.287) | |
17.4707 | 2.743 | 1.1131 | 10.579 | 8.1962 | ||
3∗ | (0.0488, 0.3839) | (0.1373, 0.3387) | (0.1376, 0.2714) | (0.0087, 1.0988) | (0.0051, 0.8157) | |
0.3351 | 0.2014 | 0.1338 | 1.0900 | 0.8105 | ||
ℜ(0.5) | 1∗ | (0.9431, 1.003) | (0.9276, 0.9647) | (0.9116, 0.9650) | (0.9159, 0.9914) | (0.9273, 0.9943) |
0.0599 | 0.0371 | 0.0534 | 0.0755 | 0.0670 | ||
2∗ | (0.9431, 1.0021) | (0.9231, 0.9673) | (0.9171, 0.9671) | (0.9264, 0.9904) | (0.9341, 0.9932) | |
0.059 | 0.0442 | 0.05 | 0.0640 | 0.0591 | ||
3∗ | (0.9774, 1.0022) | (0.9708, 0.9887) | (0.9519, 0.9883) | (0.9690, 09957) | (0.9739, 0.9967) | |
0.0249 | 0.0179 | 0.0364 | 0.0267 | 0.0228 | ||
ℜ(1.5) | 1∗ | (0.6470, 0.8635) | (0.5546, 0.7127) | (0.3798, 0.6750) | (0.6459, 0.8577) | (0.6562, 0.8638) |
0.2165 | 0.1581 | 0.2952 | 0.2118 | 0.2076 | ||
2∗ | (0.6540, 0.8623) | (0.5590, 0.7299) | (0.5688, 0.7302) | (0.6537, 0.8580) | (0.6631, 0.8660) | |
0.2083 | 0.1709 | 0.1614 | 0.2043 | 0.2028 | ||
3∗ | (0.6460, 0.9440) | (0.5743, 0.7764) | (0.2904, 0.7625) | (0.6607, 0.8934) | (0.6749, 0.9015) | |
0.2980 | 0.1065 | 0.2633 | 0.2327 | 0.2266 | ||
ℏ(0.5) | 1∗ | (0.0018, 0.2980) | (0.1973, 0.3822) | (0.1859, 0.3759) | (0.0508, 0.3388) | (0.0394, 0.3133) |
0.2962 | 0.1849 | 0.19 | 0.2880 | 0.2739 | ||
2∗ | (0.0068, 0.2972) | (0.1798, 0.3877) | (0.1791, 0.3872) | (0.0563, 0.3306) | (0.0447, 0.3066) | |
0.2904 | 0.2079 | 0.2081 | 0.2742 | 0.2618 | ||
3∗ | (0.0124, 0.1343) | (0.0675, 0.1739) | (0.2904, 0.7625) | (0.0258, 0.1785) | (0.0196, 0.1515) | |
0.1219 | 0.1064 | 0.4721 | 0.1527 | 0.1318 | ||
ℏ(1.5) | 1∗ | (0.0657, 0.4051) | (0.2891, 0.5050) | (0.2828, 0.5181) | (0.1072, 0.4887) | (0.0414, 0.3160) |
0.3394 | 0.2159 | 0.2353 | 0.3815 | 0.2746 | ||
2∗ | (0.0942, 0.3932) | (0.2733, 0.4883) | (0.2721, 0.5078) | (0.1216, 0.4052) | (0.0515, 0.3160) | |
0.299 | 0.215 | 0.2357 | 0.2836 | 0.2646 | ||
3∗ | (0.1924, 0.4551) | (0.0675, 0.1740) | (0.4148, 0.6781) | (0.2144.0.5378) | (0.0249, 0.1690) | |
0.2628 | 0.1065 | 0.2633 | 0.3234 | 0.1441 | ||
π1 | 1∗ | (0.1521, 0.6479) | (0.3333, 0.6) | (0.2953, 0.6505) | (0.1760, 0.6498) | (0.1721, 0.6445) |
0.4958 | 0.2667 | 0.3552 | 0.4738 | 0.4724 | ||
2∗ | (0.2333, 0.7079) | (0.3333, 0.6) | (0.2581, 0.6371) | (0.2463, 0.7006) | (0.2381, 0.6915) | |
0.4746 | 0.2667 | 0.379 | 0.4543 | 0.4533 | ||
3∗ | (0.2454, 0.6346) | (0.36, 0.56) | (0.3450, 0.5533) | (0.2555, 0.6323) | (0.2552, 0.6317) | |
0.3892 | 0.2 | 0.2083 | 0.3768 | 0.3765 | ||
π2 | 1∗ | (0.3521, 0.8479) | (0.4, 0.6667) | (0.3495, 0.7047) | (0.3501, 0.8239) | (0.3555, 0.8279) |
0.4958 | 0.2667 | 0.3552 | 0.4737 | 0.4724 | ||
2∗ | (0.2921, 0.7667) | (0.4, 0.6667) | (0.3629, 0.7419) | (0.2994, 0.7536) | (0.3086, 0.7618) | |
0.4746 | 0.2667 | 0.379 | 0.4542 | 0.4533 | ||
3∗ | (0.3654, 0.7545) | (0.44, 0.64) | (0.4467, 0.6550) | (0.3677, 0.7445) | (0.3683, 0.7448) | |
0.3892 | 0.2 | 0.2083 | 0.3768 | 0.3765 |
Cases | ML | Bayesian (uniform prior) | |||||
BS | BL | BL | BG | BG | |||
(h=1.5) | (h=−1.5) | (c=0.5) | (c=−0.5) | ||||
1∗ | ˆα1 | 0.0611(0.0370) | 0.0836(0.1177) | 0.07612(0.1180) | 0.1010(0.1190) | 0.0520(0.1220) | 0.0687(0.1187) |
ˆα2 | 0.0917(0.0511) | 0.1261(0.1702) | 0.1121(0.1708) | 0.1744(0.1769) | 0.0820(0.1758) | 0.1051(0.1715) | |
ˆβ | 1.5631(1.6424) | 3.4600(5.2310) | 1.1057(5.7364) | 49.410(46.25) | 1.0826(5.7459) | 2.4572(5.3263) | |
ˆℜ(0.5) | 0.9731(0.0153) | 0.9673(0.0198) | 0.9670(0.0198) | 0.9676(0.0199) | 0.9670(0.0198) | 0.9672(0.0198) | |
ˆℜ(1.5) | 0.7552(0.0552) | 0.7610(0.0541) | 0.7588(0.0542) | 0.7631(0.0542) | 0.7580(0.0542) | 0.7600(0.0542) | |
ˆℏ(0.5) | 0.1499(0.0756) | 0.1639(0.0775) | 0.1596(0.0777) | 0.1686(0.0777) | 0.1368(0.0822) | 0.1550(0.0781) | |
ˆℏ(1.5) | 0.2570(0.0976) | 0.2547(0.1338) | 0.2478(0.0985) | 0.2623(0.0986) | 0.2279(0.1019) | 0.2456(0.0987) | |
ˆπ1 | 0.4(0.1265) | 0.399(0.1226) | 0.3879(0.1231) | 0.4104(0.1232) | 0.3675(0.1266) | 0.3891(0.1230) | |
ˆπ2 | 0.6(0.1265) | 0.601(0.1226) | 0.5896(0.1232) | 0.6121(0.1231) | 0.5800(0.1244) | 0.5944(0.1228) | |
2∗ | ˆα1 | 0.0687(0.0338) | 0.0730(0.0422) | 0.0717(0.0422) | 0.0744(0.0422) | 0.0600(0.0441) | 0.0682(0.0424) |
ˆα2 | 0.0772(0.0369) | 0.0824(0.0465) | 0.0808(0.0465) | 0.0841(0.0465) | 0.0684(0.0485) | 0.0773(0.0467) | |
ˆβ | 1.6807(1.5547) | 2.7852(2.717) | 1.3028(3.0955) | 17.69(15.147) | 1.4983(3.0068) | 2.2997(2.7605) | |
ˆℜ(0.5) | 0.9726(0.0150) | 0.9679(0.0168) | 0.9677(0.0168) | 0.9681(0.0168) | 0.9677(0.0168) | 0.9679(0.0168) | |
ˆℜ(1.5) | 0.7581(0.0531) | 0.7624(0.0524) | 0.7603(0.0524) | 0.7644(0.0524) | 0.7596(0.0524) | 0.7615(0.0524) | |
ˆℏ(0.5) | 0.1520(0.0741) | 0.1656(0.0722) | 0.1618(0.0723) | 0.1697(0.0723) | 0.1422(0.0759) | 0.1579(0.0726) | |
ˆℏ(1.5) | 0.2480(0.0785) | 0.2408(0.1047) | 0.2370(0.0730) | 0.2449(0.0730) | 0.2246(0.0747) | 0.2354(0.0731) | |
ˆπ1 | 0.4706(0.1211) | 0.4699(0.1165) | 0.4598(0.1170) | 0.4802(0.1170) | 0.4463(0.1189) | 0.4624(0.1168) | |
ˆπ2 | 0.5294(0.1211) | 0.5301(0.1165) | 0.5198(0.1170) | 0.5402(0.1170) | 0.5089(0.1184) | 0.5233(0.1167) | |
3∗ | ˆα1 | 0.2659(0.2318) | 0.4510(0.5585) | 0.3281(0.5720) | 1.3421(1.0517) | 0.2458(0.5950) | 0.3570(0.5664) |
ˆα2 | 0.3384(0.2913) | 0.5750(0.7032) | 0.3992(0.7248) | 2.0700(1.6521) | 0.3186(0.7485) | 0.4571(0.7130) | |
ˆβ | 0.1368(0.1947) | 0.2622(0.4002) | 0.2007(0.4049) | 1.0029(0.8419) | 0.0825(0.4388) | 0.1880(0.4071) | |
ˆℜ(0.5) | 0.9898(0.0063) | 0.9877(0.0076) | 0.9876(0.0076) | 0.9877(0.0076) | 0.9876(0.0076) | 0.9877(0.0076) | |
ˆℜ(1.5) | 0.7975(0.0760) | 0.7922(0.0617) | 0.7893(0.0618) | 0.7951(0.0618) | 0.7885(0.0619) | 0.7910(0.0617) | |
ˆℏ(0.5) | 0.0610(0.0374) | 0.0725(0.0424) | 0.0712(0.0424) | 0.0739(0.0424) | 0.0592(0.0444) | 0.0676(0.0427) | |
ˆℏ(1.5) | 0.3818(0.0966) | 0.3613(0.007) | 0.3561(0.0840) | 0.666(0.08401) | 0.3465(0.0851) | 0.3564(0.0840) | |
ˆπ1 | 0.44(0.0993) | 0.4397(0.0975) | 0.4326(0.0977) | 0.4468(0.0977) | 0.4224(0.0990) | 0.4341(0.0976) | |
ˆπ2 | 0.56(0.0993) | 0.5603(0.0975) | 0.5532(0.0977) | 0.5674(0.0977) | 0.5468(0.0984) | 0.5560(0.0976) |