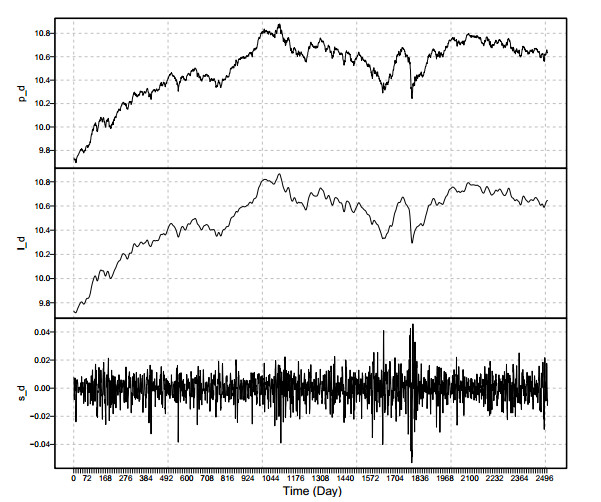
Traders and investors find predicting stock market values an intriguing subject to study in stock exchange markets. Accurate projections lead to high financial revenues and protect investors from market risks. This research proposes a unique filtering-combination approach to increase forecast accuracy. The first step is to filter the original series of stock market prices into two new series, consisting of a nonlinear trend series in the long run and a stochastic component of a series, using the Hodrick-Prescott filter. Next, all possible filtered combination models are considered to get the forecasts of each filtered series with linear and nonlinear time series forecasting models. Then, the forecast results of each filtered series are combined to extract the final forecasts. The proposed filtering-combination technique is applied to Pakistan's daily stock market price index data from January 2, 2013 to February 17, 2023. To assess the proposed forecasting methodology's performance in terms of model consistency, efficiency and accuracy, we analyze models in different data set ratios and calculate four mean errors, correlation coefficients and directional mean accuracy. Last, the authors recommend testing the proposed filtering-combination approach for additional complicated financial time series data in the future to achieve highly accurate, efficient and consistent forecasts.
Citation: Hasnain Iftikhar, Murad Khan, Josué E. Turpo-Chaparro, Paulo Canas Rodrigues, Javier Linkolk López-Gonzales. Forecasting stock prices using a novel filtering-combination technique: Application to the Pakistan stock exchange[J]. AIMS Mathematics, 2024, 9(2): 3264-3288. doi: 10.3934/math.2024159
[1] | Salvatore Mancha Gonzales, Hasnain Iftikhar, Javier Linkolk López-Gonzales . Analysis and forecasting of electricity prices using an improved time series ensemble approach: an application to the Peruvian electricity market. AIMS Mathematics, 2024, 9(8): 21952-21971. doi: 10.3934/math.20241067 |
[2] | Zhi Liu . Forecasting stock prices based on multivariable fuzzy time series. AIMS Mathematics, 2023, 8(6): 12778-12792. doi: 10.3934/math.2023643 |
[3] | Xiao Qi, Tianyao Duan, Lihua Wang, Huan Guo . CATL's stock price forecasting and its derived option pricing: a novel extended fNSDE-net method. AIMS Mathematics, 2025, 10(2): 2444-2465. doi: 10.3934/math.2025114 |
[4] | Pedro J. Gutiérrez-Diez, Jorge Alves-Antunes . Stock market uncertainty determination with news headlines: A digital twin approach. AIMS Mathematics, 2024, 9(1): 1683-1717. doi: 10.3934/math.2024083 |
[5] | Vladica S. Stojanović, Hassan S. Bakouch, Radica Bojičić, Gadir Alomair, Shuhrah A. Alghamdi . Poisson-Lindley minification INAR process with application to financial data. AIMS Mathematics, 2024, 9(8): 22627-22654. doi: 10.3934/math.20241102 |
[6] | Shuai Wang, Zhongyan Li, Jinyun Zhu, Zhicen Lin, Meiru Zhong . Stock selection strategy of A-share market based on rotation effect and random forest. AIMS Mathematics, 2020, 5(5): 4563-4580. doi: 10.3934/math.2020293 |
[7] | Hanjie Liu, Yuanguo Zhu, Yiyu Liu . European option pricing problem based on a class of Caputo-Hadamard uncertain fractional differential equation. AIMS Mathematics, 2023, 8(7): 15633-15650. doi: 10.3934/math.2023798 |
[8] | Javed Hussain, Saba Shahid, Tareq Saeed . Pricing forward-start style exotic options under uncertain stock models with periodic dividends. AIMS Mathematics, 2024, 9(9): 24934-24954. doi: 10.3934/math.20241215 |
[9] | Ming Yang, Yin Gao . Pricing formulas of binary options in uncertain financial markets. AIMS Mathematics, 2023, 8(10): 23336-23351. doi: 10.3934/math.20231186 |
[10] | Mohammed Alharbey, Turki Mohammed Alfahaid, Ousama Ben-Salha . Asymmetric volatility spillover between oil prices and regional renewable energy stock markets: A time-varying parameter vector autoregressive-based connectedness approach. AIMS Mathematics, 2023, 8(12): 30639-30667. doi: 10.3934/math.20231566 |
Traders and investors find predicting stock market values an intriguing subject to study in stock exchange markets. Accurate projections lead to high financial revenues and protect investors from market risks. This research proposes a unique filtering-combination approach to increase forecast accuracy. The first step is to filter the original series of stock market prices into two new series, consisting of a nonlinear trend series in the long run and a stochastic component of a series, using the Hodrick-Prescott filter. Next, all possible filtered combination models are considered to get the forecasts of each filtered series with linear and nonlinear time series forecasting models. Then, the forecast results of each filtered series are combined to extract the final forecasts. The proposed filtering-combination technique is applied to Pakistan's daily stock market price index data from January 2, 2013 to February 17, 2023. To assess the proposed forecasting methodology's performance in terms of model consistency, efficiency and accuracy, we analyze models in different data set ratios and calculate four mean errors, correlation coefficients and directional mean accuracy. Last, the authors recommend testing the proposed filtering-combination approach for additional complicated financial time series data in the future to achieve highly accurate, efficient and consistent forecasts.
Ancient civilizations used merchants to trade goods across the seas. In the late Middle Ages, merchants gathered in city centers to exchange goods worldwide. Antwerp, Belgium became a hub for global traders in the 1400s. Wealthy merchants lent money to small traders, who released interest-based bonds [1]. The Dutch East India Company established the first modern Amsterdam stock exchange in 1602. Stock markets are crucial for boosting economies, controlling inflation and driving global economic growth [2,3]. The primary objective of investors is to earn higher returns on their investment in the stock market and reduce the risk of losses as minimally as possible [4,5,6,7]. Moreover, the prediction of the stock price is a complex task due to the high volatility of stock market data, and investors are reluctant to invest due to this complexity.
To tackle the above mentioned problems, many researchers have used various time series, machine learning and hybrid models to forecast stock prices and returns to assess investors' state financial decisions to earn higher returns and reduce losses to the minimum extent possible [8,9,10,11]. For example, the researchers in reference [12] conducted a study on the effectiveness of an autoregressive integrated moving average (ARIMA) model for 56 Indian stocks in various sectors. The results reveal that the accuracy of the ARIMA model in predicting stock prices is greater than 85% across all sectors, indicating that ARIMA provides strong prediction accuracy on different ranges of data for historical periods. Single-time series models were limited to the conventional approach of index projections. Although the structure of stock market data is very complicated, it is nonlinear and nonstationary, which leads to the failure of conventional time series methods [13]. On the other hand, the authors of [14] employed the GARCH-MIDAS model to examine the impact of the economic policy uncertainty index and macroeconomic variables on Pakistan stock market volatility. The results reveal that the index has predictive power, that oil prices are the strongest predictor, and that all macroeconomic variables provide important information for anticipating stock market volatility. However, long-run interest rates are ineffectual. In contrast, machine learning models have caught the attention of researchers when forecasting stock market prices. These models can deal with nonlinear time series data and the handling capacity of complex economic data, which became helpful in solving these complexities in financial and stock data [15,16,17,18]. For example, the researchers in [19] used the ANN with learning algorithms including standard backward propagation, backward propagation with Bayesian regularization and scaled conjugate gradient as the prediction models for foreign currency and compared the results with the ARIMA model. It was found that ANN outperformed the ARIMA model. On the other hand, to improve predictive stock prices, the researchers have assembled features from two or more models to create novel models, commonly referred to as hybrid models [20,21,22,23,24]. For instance, the researchers in [25] selected technical indicators, including the opening, lowest, highest and closing prices of stock trading data by applying an integrated selection method, then analyzed these indicators on a hybrid LSTM-GRU and got highly accurate one-step-ahead forecasts of the closing stock price compared to other methods. In another study [26], the researchers proposed a labeling approach to label data at certain points in time based on N-Period Min-Max (NPMM) to overcome the sensitivity of short-term price changes. The proposed method also builds a trading system by implementing XGBoost to verify and automate the trading of the labeling method based on the evaluation of 92 listed companies on NASDAQ. Later on, they found the proposed NPMM efficient method of labeling in stock price prediction compared to other used models.
Some authors have studied the comparison between different filters for forecasting financial time series. For example, a study [46] examined whether the use of Hamilton's regression filter significantly alters the cyclical components of unemployment in Greece compared to the Hodrick-Prescott double filter (HPDF). However, we discovered that the trend and cycle components of Hamilton's filter regression resulted in much higher cycle volatilities than the HPDF when using quarterly data for unemployment in Greece in a macroeconomic model decomposition. The HPDF, combined with its limitations at the end of the time series, was utilized for dynamic forecasting in the sample and autoregressive forecasting, which gives steady forecasts for a wide variety of non-stationary operations. The findings revealed that the HPDF's dynamic forecasting outperforms Hamilton's in all assessment measures. On the other hand, the researchers in [47] proposed a boosted HP filter that uses machine learning for smarter trend estimation and elimination. It uses limit theory to recover common trends in macroeconomic data and current modeling methodology. The boosted filter provides a new mechanism for estimating multiple structural breaks and is data-determined for modern economic research environments. The methodology was illustrated using real data examples and showed the best results. Similarly, the researchers in [49] suggested iterating the HP filter to create an intelligent smoothing tool called the boosted HP filter. This filter is based on L2-boosting in machine learning. According to the limit theory, the bHP filter can asymptotically recover trend mechanisms involving integrated processes, deterministic drifts and structural breaks, covering the most common trends that appear in the proposed modeling methodology. The algorithm was automated with a stopping criterion, providing a data-determined method for data-rich environments. The methodology is demonstrated with simulations and three real data examples, highlighting the differences between simple HP filtering, the bHP filter and an alternative autoregressive approach.
Contrary to the above-stated techniques and models, another way to forecast time series data accurately and efficiently in the literature is through decomposition methods [27,28]. In these methods, the original time series data is divided into many subseries using different filtering or decomposition techniques [29,30]. For instance, the researchers [31] proposed a hybrid approach based on three components: Novel features, noise filtering and ML-based prediction, and applied a Hodrick-Prescott filter technique in its fully modified form for smoothing historical stock price data by decomposing and discarding the seasonal component of the time series data, achieving 70.88 % forecasting accuracy in comparison to other used techniques. Sometimes, stock prices follow a specific trend that leads to overfitting and erroneous forecasting. To do this, in [32] proposed a new version of empirical mode decomposition (EMD) to remove the trend component in stock price forecasting, and a complete ensemble empirical mode decomposition with adaptive noise (CEEMDAN) wavelet model was used in [33] to check the contagion effects among stock markets in America, Asia and Europe. In this approach, the variations are broken down into several intrinsic mode functions (IMFs) in time series data to get a monotone residue component. In another work, the authors in [34] proposed a novel improved hybrid method based on Akima-EMD-LSTM for forecasting the stock price of the KE-100 index complex time series of Pakistan stock exchange data. In the first phase, they decomposed the original data into sub-components, or IMFs, and modeled the LSTM network based on the highly correlated sub-components. The Akima spline interpolation technique was also used to tackle the noisy component of the data. Later, they found the proposed Akima-EMD-LSTM network outperformed other models, in the forecasting of stock prices.
In this paper, we proposes a new filtering-combination technique for forecasting the daily stock index prices. The technique is simple to implement and enhances the accuracy of predictions. It combines the Hodrick-Prescott filter with standard linear and nonlinear time series models, including two linear models, autoregressive and autoregressive moving averages and two nonlinear models, nonlinear autoregressive and autoregressive neural network models. Therefore, first, the original closing stock price time series data is filtered into two new time series using the Hodrick-Prescott filter. These represent a nonlinear trend and stochastic components. Then, two linear and nonlinear time series and all possible combination models are used to forecast the filtered time series, separately dealing with linear and nonlinear problems to achieve accurate and efficient forecasts. To this end, the main contribution of this work is the proposed filtering-combination methodology, which combines the Hodrick-Prescott filter with traditional time series models to improve the accuracy of the day-ahead daily closing stock price. Additionally, within the proposed forecasting technique, the paper compares the performance of sixteen different combinations of linear and nonlinear time series models using the Hodrick-Prescott filter. Furthermore, the proposed methodology is evaluated in terms of model consistency, efficiency and accuracy across different ratios of the dataset. Mean errors, correlation coefficients and directional mean accuracy are computed. Thus, the results of the final proposed best combination model obtained highly accurate, efficient and consistent gains in forecasts for the used data. In the end, the final combination model yields the highest directional mean accuracy and the lowest mean error, which is relatively better than the standard baseline models. In summary, the authors recommended that the proposed filtering-combination technique of forecasting could be considered for other complex economical time series datasets to extract highly accurate, efficient and consistent forecasts.
The rest of the paper is organized in the following sections: Section 2 explores the proposed filtering and combination technique for forecasting; Section 3 describes the case study results and provides a discussion of the proposed forecasting technique, the other benchmark models and the direction for the policy marker using the proposed final best model. Finally, Section 4 presents conclusions and future work directions.
This section explains the proposed filtering-combination technique of forecasting for daily closing stock price forecasts. In general, the time series of stock prices has nonlinear and complex structures. For this purpose, the daily closing stock price series (pd) is decomposed into two major subseries: The long-run trend component (ℓd) and a stochastic component (cd) using the Hodrick-Prescott filter. The mathematical representation of the decomposed components is given by:
pd=ℓd+cd. | (2.1) |
Hence, the Hodrick-Prescott Filter is described in the following section.
The Hodrick-Prescott filter (HPF) produces a smoothed-curve representation of a time series that is more complicated owing to long-term fluctuations than short-term fluctuations. The sensitivity of the trend to short-term changes is adjusted by altering a multiplier, β. Let the time series data be pd (d = 1, 2, ..., D). The series pd is composed of a trend component (ℓd) and an stochastic component (cd).
We are now put into a long-term trend component that may be calculated by minimizing the expression:
min(ℓd)ΣDd=1((pd−ℓd)2+βΣD−1d=2[(ℓd+1−ℓd)−(ℓd−ℓd−1)]2). |
The first term in the above formula is a loss function, while the second is a penalty term β multiplied by the sum of squares of the trend component's second difference, which penalizes variance in the trend component's growth rate. However, the lower values for the smoothing parameter β make the trend more volatile since it includes a large portion of the spectrum of high frequencies. The expression (2.3) is minimized with pd=ℓd if β = 0. On the other hand, the expression (2.3) is minimized for β→∞, and the predicted trend converges to the linear trend of the least squares, becoming a straight line. In our case, we set the β = 6.25 value provided by Ravn and Uhlig [52].
Figure 1 displays the filtered new subseries to visualize the performance of the previously applied HP filter. The figure comprises three planes. The first plane shows the daily time series of closing stock prices (pd), the second plane represents the nonlinear trend (ℓd) subseries and finally the third plane shows the stochastic subseries (cd). The figure demonstrates that the HPF has efficiently filtered the stock index prices (pd). The nonlinear trend series accurately captures the daily closing stock prices of the time series.
We forecast the filtered series using four different time series models, including two linear and two nonlinear time series models, after filtering the components from the daily closing prices using the HPF method. The two linear models, autoregressive and autoregressive moving averages, and two nonlinear models, nonlinear autoregressive and autoregressive neural network models, are included. Here is a brief description of the models under consideration.
A linear and parametric autoregressive (AR) model, which uses a linear combination of the previous observations of pd time series and represents the short-term dynamics of this series, is referred to as:
pd=α+ϑ1pt-1+ϑ2pt-2+....+ϑnpd−n+εd. | (2.2) |
In the above formula, α is an intercept term, and ϑj(j=1,2,⋯,n) n) is the slope parameter of the underlying AR process, and εd is the disturbance term. However, the present study uses the maximum likelihood method to estimate the parameters of the AR model. The model includes lags 1–3, based on their significant results in the correlogram (autocorrelation and partial autocorrelation functions) for the series.
The autoregressive moving average (ARMA) model integrates not only the target variable's previous values but also vital information in the form of moving averages (the error lags). In our scenario, the study variable pd is explained by the prior terms, as are the delayed residual values. Mathematically,
pd=α+ϑ1pd-1+ϑ2pd-2+⋯+ϑnpd−n+εd+ζ1εd−1+ζ2εd−2+....+ζmεd−m. | (2.3) |
In the last equation, α denotes intercept, ϑj(j=1,2,⋯,n) and ζk(k=1,2,⋅,m) are the parameters of AR and MA process, respectively, and εd is a Gaussian white noise series with mean zero and variance σ2ε. Hence, the unknown population parameters are estimated through the maximum likelihood method. The ARMA model order selection, which is the number of past values and the past error term value, is established by examining the correlograms. In the MA part, the first two lags are significant, while in the AR part, only lags 1–4 are significant for closing prices time series (pd).
The additive nonparametric counterpart of the AR process leads to the additive model (NPAR), where the association between pd and its previous terms do not have any specific parametric form, letting, probably, for any sort of nonlinearity which is stated as:
pd=q1(pd-1)+q2(pd-2)+…+qn(p d- n)+εd, | (2.4) |
where qj(j=1,2,⋯,n) are showing smoothing functions and describe the association between pd and its previous values. Hence, in this work, the functions qi are represented by cubic regression splines and lags 1–3 are used for NPAR modeling.
An autoregressive neural network (ANN) is a type of machine learning model that predicts future values of a time series by analyzing its past observations. The model uses a mathematical function that factors in the previous values of the time series, represented by pd−1,pd−2,...,pd−n, where n is the time delay parameter. The ANN model is trained using the backpropagation method and the steepest descent approach to minimize the difference between the predicted and actual values.
The forecasting process in an ANN model involves two steps. First, the order of autoregression is determined, which refers to the number of previous values needed to predict the current value of the time series. Second, the NN is trained using a training set that considers the order of autoregression. The number of input nodes is determined by the order of autoregression and the inputs are the previous, lagged observations in univariate time series forecasting. The predicted values are the output of the NN model.
The number of hidden nodes is often determined by trial and error or experimentation since there is no theoretical basis for selecting them. It is important to select the number of iterations carefully to avoid overfitting. In this work, an ANN (4, 2) design is used, which can be represented as pd=f(pd-1). Here, pd-1=(pd-1,pd-2,pd-3,pd-4) is a vector containing past values of the time series of the closing stock prices (pd), and f is a neural network with 4 hidden nodes in a signal layer.
The proposed approach is evaluated using the metrics listed in Table 1. This table contains the formula used to calculate each metric. The metrics presented in the table are Root Mean Square Percentage Error (RMSPE), Root Mean Square Error (RMSE), Mean Absolute Error (MAE), Mean Absolute Percentage Error (MAPE) and Coefficient Correlation (CC) [35,36,37]. Here, D is the number of observations in the data set, (pd), and (ˆpd), is the nth estimated and observed data point.
S.No | Error | Formula |
1 | RMSPE | √1D∑Dd=1(|pd−^pd||pd|)2×100 |
2 | RMSE | √1D∑Dd=1(pd−ˆpd)2 |
3 | MAE | 1D∑Dd=1(|pd−^pd|) |
4 | MAPE | 1D∑Dd=1(|pd−^pd||pd|)×100 |
5 | CC | Corr(pd,ˆpd) |
The MDA is used to evaluate the ability of direction prediction, which is defined as follows:
MDA=1DD∑d=1αd, | (2.5) |
αd={1,(pd−pd−1)×(ˆpd−pd−1)≥0,0, otherwise. | (2.6) |
Normally, the smaller the RMSPE, MAPE, MAE and RMSE, the greater the CC and MDA, which represents a higher prediction accuracy and a better performance of direction prediction.
In addition to the performance indicators listed above, Diebold and Mariano's (DM) test was used to determine the significance of changes in the model's prediction performance [38]. The DM test is a popular statistical method for comparing predictions from two models in the literature [39,40,41]. For instance, consider the two forecasts obtained from the two different time series models, such as ˆp1d (model 1) and ˆp2d (model 2). However, ξ1d=pd−ˆp1d and ξ2d=pd−ˆp2d are the corresponding forecast errors. The loss associated with forecast error {ξid}2i=1 by ℓ(ξid). For example, time d absolute loss would be ℓ(ξid)=|ξid|. The loss differential between Forecasts 1 and 2 for time d is then ωd=ℓ(ξ1d)−ℓ(ξ2d). The null hypothesis of equal forecast accuracy for two forecasts is E[ωd]=0. The DM test requires that the loss differential be covariance stationary, that is,
E[ωd]=α,∀ d, | (2.7) |
cov(ωd−ωd−d)=ρ(d),∀ d, | (2.8) |
var(ωd)=σω,0<σω<∞, | (2.9) |
with these assumptions, the DM test of the same forecast accuracy is
DM=ˉωˆσˉωd→N(0,1). |
In the above equation, ˉω=1D∑Dd=1ωd is the sample mean loss differential and ˆσˉω is a consistent standard error estimate of ωd.
In this study, we denote each combined model with Hodrick-Prescott filter method by HPFcdℓd, where the ℓd at the top right is associated with a nonlinear trend subseries, and the cd at bottom right is associated to the residual subseries. In the forecasting models, we assign a code to each model: "1" for the AR, "2" for the ARIMA, "3" for the NPAR and "4" for the ANN. For example, HPF12 represents the estimate of the long-term trend (ℓd) with the AR and the residual series (cd) estimated using ARIMA. Hence, the individual forecast models are combined to obtain the final one-day-ahead closing stock price forecast. The final equation is as follows:
ˆpd+1=(^ℓd+1+ˆcd+1). | (2.10) |
To finish this part, Figure 2 depicts the design of the proposed filtering-combination forecasting technique.
The Pakistan Stock Exchange (PSX) is one of the largest stock markets in Pakistan for trading stocks, making investments, and earning a profit. It is highly preferred by investors due to its fame. PSX was established in 2016 by merging three prominent stock exchanges in Pakistan: Karachi, Lahore and Islamabad. The Financial Times Stock Exchange classified PSX as a secondary merging market, which was later reclassified as an emerging market by Morgan Stanley Capital International. Both local and foreign investors actively participate in the daily trading of stocks. PSX exhibits similar behavior to other stock markets in terms of bullish and bearish trends, depending on the situation. As of April 5, 2023, PSX had 545 listed companies with a market capitalization of Rs. 6,142.331 billion. The Securities Exchange Commission of Pakistan and Pakistan Stock Exchange Limited regulate these listed companies under strict rules and regulations. In the PSX, 37 listed sectors contribute to the capitalization of the market, including indexes, future bonds, etc. Some sectors are based on non-contributory funds.
PSX generates complex data due to political instability, terrorism, hoarding of dollars and money laundering. This creates confusion for investors when making decisions about holding and selling shares. To tackle this issue and help investors and policymakers with short-term strategy, a research study proposed a filtering-combination technique for accurately forecasting stock prices. Hence, to validate the consistency of the proposed filtering-combination technique, historical data was obtained from the Yahoo Finance website (https://finance.yahoo.com), which was visited on February 17, 2023. The data ranges from January 2, 2013, to February 17, 2023, covering the daily closing stock price for 2538 days, excluding off-working days. The whole dataset was divided into three different training and testing ratios: 70% training, 30% testing; 80% training, 20% testing; and 90% training, 10% testing. In every subset, the first part was considered for the training set to train the model and optimize parameters, and the second part consisted of a testing dataset used to evaluate the performance of the established forecasting approach. The details about these subset datasets are listed in Table 2.
Data | Scenario | Number of observation | Date |
Training | 70% | 1269 | 2 January 2013 to 21 December 2017 |
Testing | 30% | 1269 | 22 December 2017 to 17 February 2023 |
Training | 80% | 1904 | 2 January 2013 to 25 June 2020 |
Testing | 20% | 635 | 26 June 2020 to 17 February 2023 |
Training | 90% | 2285 | 2 January 2013 to 22 December 2021 |
Testing | 10% | 254 | 23 December 2021 to 17 February 2023 |
To confirm the nonstationarity and nonlinearity of the time series of closing stock prices, we performed the Augmented Dickey-Fuller and the Teraesvirta tests [42,43]. The results are listed in Table 3. Before discussing this table, it's important to note that the series should be stationary before modeling and forecasting decomposed subseries. A stationary process does not change over time in terms of its mean, variance and autocorrelation structure. If the underlying series is temporal, it should be transformed into a stationary series. Various techniques are used to achieve stationarity, such as natural logarithms, series derivatives and Box-Cox transformations [44,45]. In this work, the daily closing stock price time series is divided into two new subseries, namely a nonlinear trend series and a stochastic series, and is plotted in Figure 3. It can be observed from Figure 3 that the nonlinear long-run trend series has a curved trend, which shows that the series is nonstationary, hence the need to make it stationary. In contrast, the stochastic series has no trend component. To check the unit root issue of the filtered subseries statistically, we used the Augmented Dickey-Fuller test. The results (statistic values) are listed in Table 3, which suggests that the long-run trend series is nonstationary at the level. As a result, when the first-order difference was taken, the nonlinear trend series converted to a stationary one. Figure 3 depicts the first-order differencing series of long-run trend series, which assures stationarity. On the other hand, the original time series of the closing stock prices and the long-run nonlinear trend subseries have nonlinearity in the mean, while based on the Teraesvirta test results, the stochastic (residual) subseries have no more nonlinearity. The first-order differencing series of long-run trend series ensures linearity in the mean. Once we address both issues of nonstationarity and nonlinearity, we can proceed further to modeling and forecasting one-day-ahead closing stock prices using different combinations of linear and nonlinear time series models.
Nonlinearity outcomes | Nonstationarity outcomes | ||||
Series | Statistic Value | P-Value | At level | At first difference | Conclusion |
Closing stock prices(pd) | 97.2750 | 0.0100 | -1.3240 | -9.8100 | I (1) |
long-run trend (ℓd) | 4.4045 | 0.1106 | -2.4324 | -10.6700 | I (1) |
Stochastic (cd) | 51.2380 | 0.0100 | -32.8480 | - |
In order to obtain the daily closing stock price for the Pakistan stock exchange for the day out of sample forecasts via the proposed filtering-combination forecasting method explained in Section 2, we need to follow these steps: In the first step, the HPF method was used to extract a nonlinear trend (ℓd) component and a stochastic (cd) component. In the second step, the well-known two linear and two nonlinear time series models, such as the AR, the ARMA, the NPAR and the ANN, were applied to each component extracted in the first step. In the third step, the results of every subseries forecast were combined to get the final results. Thus, overall, sixteen possible combination models fall within the proposed forecasting technique. However, to compare and evaluate the forecast accuracy of each combined model for day-ahead closing stock prices, the accuracy metrics, including RMSPE, MAPE, MAE, RMSE, CC and MDA, were computed. In addition, to verify the consistency of the best model within the proposed filtering-combination technique, we divided the whole data set into three different training and testing ratio samples, such as (70%, 30%), (80%, 20%) and (90%, 10%). Therefore, there are three training and testing data sets, for a total of 48 (3×16) combination models. The day-ahead performance metrics (RMSPE, MAPE, MAE, RMSE, CC and MDA) for these 48 models are listed in Table 4. In general, the lower the accuracy mean errors (RMSPE, MAPE, MAE and RMSE) and the higher the value of the coefficient of correlation and mean directional accuracy, the higher the forecasting efficiency and accuracy model. Thus, within all sixteen combination models, the HPF23 combination model produced better forecasting accuracy for one-day-ahead daily closing stock prices throughout all three subsets of training and testing datasets.
The first scenario 70%, 30% | |||||||
S.No | Models | RMSPE | MAPE | MAE | RMSE | CC | MDA |
1 | HPF11 | 156.2373 | 0.3778 | 0.0053 | 209.6080 | 0.9987 | 0.8966 |
2 | HPF12 | 156.8296 | 0.3789 | 0.0053 | 209.9340 | 0.9987 | 0.9047 |
3 | HPF13 | 127.5402 | 0.3081 | 0.0043 | 170.2864 | 0.9991 | 0.9195 |
4 | HPF14 | 161.2721 | 0.3901 | 0.0054 | 213.6699 | 0.9986 | 0.9141 |
5 | HPF21 | 148.8171 | 0.3602 | 0.0051 | 202.2458 | 0.9988 | 0.9168 |
6 | HPF22 | 149.9615 | 0.3626 | 0.0051 | 203.1089 | 0.9988 | 0.9128 |
7 | HPF23 | 120.8022 | 0.2924 | 0.0041 | 161.9550 | 0.9992 | 0.9302 |
8 | HPF24 | 168.5671 | 0.4076 | 0.0057 | 225.6628 | 0.9985 | 0.8993 |
9 | HPF31 | 156.5730 | 0.3786 | 0.0053 | 209.6822 | 0.9987 | 0.8966 |
10 | HPF32 | 157.1322 | 0.3796 | 0.0053 | 210.0071 | 0.9987 | 0.8980 |
11 | HPF33 | 127.3876 | 0.3077 | 0.0043 | 170.2426 | 0.9991 | 0.9195 |
12 | HPF34 | 162.1289 | 0.3923 | 0.0054 | 214.3426 | 0.9986 | 0.9128 |
13 | HPF41 | 241.5573 | 0.5869 | 0.0105 | 425.4353 | 0.9946 | 0.8523 |
14 | HPF42 | 242.3449 | 0.5885 | 0.0105 | 426.2372 | 0.9946 | 0.8497 |
15 | HPF43 | 187.4053 | 0.4543 | 0.0090 | 369.9058 | 0.9960 | 0.9020 |
16 | HPF44 | 261.4660 | 0.6346 | 0.0109 | 442.4052 | 0.9942 | 0.8309 |
The second scenario 80%, 20% | |||||||
1 | HPF11 | 151.5090 | 0.3437 | 0.0045 | 199.2405 | 0.9964 | 0.8788 |
2 | HPF12 | 151.6872 | 0.3439 | 0.0045 | 199.5213 | 0.9964 | 0.8828 |
3 | HPF13 | 122.9512 | 0.2792 | 0.0037 | 160.5389 | 0.9977 | 0.9091 |
4 | HPF14 | 154.9424 | 0.3515 | 0.0046 | 201.5488 | 0.9963 | 0.8909 |
5 | HPF21 | 141.9220 | 0.3219 | 0.0043 | 190.3518 | 0.9967 | 0.8929 |
6 | HPF22 | 143.0074 | 0.3242 | 0.0044 | 191.4878 | 0.9967 | 0.8909 |
7 | HPF23 | 115.5138 | 0.2622 | 0.0034 | 149.8806 | 0.9980 | 0.9253 |
8 | HPF24 | 159.7229 | 0.3621 | 0.0048 | 210.7949 | 0.9960 | 0.8848 |
9 | HPF31 | 151.9299 | 0.3447 | 0.0045 | 199.3006 | 0.9964 | 0.8747 |
10 | HPF32 | 152.0373 | 0.3448 | 0.0045 | 199.5532 | 0.9964 | 0.8788 |
11 | HPF33 | 122.9880 | 0.2793 | 0.0037 | 160.4473 | 0.9977 | 0.9071 |
12 | HPF34 | 155.6473 | 0.3531 | 0.0046 | 202.1268 | 0.9963 | 0.8929 |
13 | HPF41 | 232.6237 | 0.5272 | 0.0094 | 415.7154 | 0.9843 | 0.8364 |
14 | HPF42 | 232.8815 | 0.5278 | 0.0094 | 416.4237 | 0.9843 | 0.8323 |
15 | HPF43 | 178.2604 | 0.4032 | 0.0082 | 366.0674 | 0.9879 | 0.8929 |
16 | HPF44 | 252.2533 | 0.5718 | 0.0098 | 436.0666 | 0.9828 | 0.8242 |
The third scenario 90%, 10% | |||||||
1 | HPF11 | 156.0030 | 0.3672 | 0.0047 | 199.1678 | 0.9927 | 0.8776 |
2 | HPF12 | 155.1474 | 0.3651 | 0.0047 | 198.6827 | 0.9927 | 0.8816 |
3 | HPF13 | 130.0234 | 0.3060 | 0.0038 | 162.9916 | 0.9951 | 0.9143 |
4 | HPF14 | 158.6718 | 0.3734 | 0.0048 | 202.2724 | 0.9925 | 0.8898 |
5 | HPF21 | 144.9313 | 0.3412 | 0.0045 | 189.5869 | 0.9934 | 0.8980 |
6 | HPF22 | 145.2673 | 0.3419 | 0.0045 | 190.0539 | 0.9933 | 0.8939 |
7 | HPF23 | 121.8376 | 0.2866 | 0.0036 | 151.6476 | 0.9958 | 0.9429 |
8 | HPF24 | 161.9019 | 0.3813 | 0.0050 | 212.5678 | 0.9917 | 0.8776 |
9 | HPF31 | 156.4563 | 0.3683 | 0.0047 | 199.1440 | 0.9927 | 0.8735 |
10 | HPF32 | 155.4268 | 0.3658 | 0.0047 | 198.6606 | 0.9927 | 0.8735 |
11 | HPF33 | 130.1624 | 0.3064 | 0.0038 | 163.0377 | 0.9951 | 0.9143 |
12 | HPF34 | 159.5017 | 0.3754 | 0.0048 | 202.7243 | 0.9924 | 0.8857 |
13 | HPF41 | 251.2031 | 0.5910 | 0.0123 | 528.4650 | 0.9491 | 0.8204 |
14 | HPF42 | 251.0871 | 0.5907 | 0.0123 | 528.8418 | 0.9490 | 0.8245 |
15 | HPF43 | 194.0790 | 0.4555 | 0.0113 | 488.9315 | 0.9567 | 0.8776 |
16 | HPF44 | 271.9590 | 0.6396 | 0.0125 | 539.1469 | 0.9470 | 0.8449 |
In the first scenario of 70% training and 30% testing, the best forecasting model is HPF23, which obtained 120.8022, 0.2924, 0.4076, 0.0041,161.9550, 0.9992 and 0.9302 for RMSPE, MAPE, MAE, RMSE, CC and MDA, respectively. It can be observed that the RMSPE, MAPE, MAE and RMSE are the lowest values compared to the rest, while the CC and MDA are the highest values among all other combinations. In the same way, the model HPF33 produced the second-best result compared to all other used combination models with 127.3876, 0.3077, 0.4076, 0.0041,161.9550, 0.9992 and 0.9302 for RMSPE, MAPE, MAE, RMSE, CC and MDA, accordingly. On the other hand, in the second scenario of 80% training and 20% testing sample set, again, it is evident that the HPF23 combination model outperformed all combination models with forecasting metric errors of 115.5138, 0.2622, 0.0034,149.8806, 0.9980 and 0.9253, RMSPE, MAPE, MAE, RMSE, CC and MDA, respectively, while the HPF33 and HPF13 models are declared the second and third best models, respectively. Finally, once again, in the third scenario of 90% training and 10% testing, it is confirmed that from the results stated in Table 4, the HPF23 model is the best combination model compared to the rest of the models, with mean forecasting accuracy of 121.8376, 0.2866, 0.0036,151.6476, 0.9958, 0.9429 RMSPE, MAPE, MAE, RMSE, CC and MDA, respectively. Thus, within all possible sixteen combination models, the HPF23 combination model is declared the best model, and the consistency of this (HPF23) model from all three scenarios of training and testing sample data sets is also confirmed.
Furthermore, to verify the performance of the proposed best combination model (HPF23) stated in Table 4 we have conducted the DM test. The results (p-values) of the DM test for each and every scenario training and testing sample data set are listed in Table 5, it is evident in this table that the proposed best combination model (HPF23) is statistically significant at 5% compared to the other fifteen combination models in all training and testing scenarios. Although, the HPF33, HPF13 and HPF21 were found good competitors to the final best (HPF23) model.
The first scenario 70%, 30% | ||||||||||||||||
Models | HPF11 | HPF12 | HPF13 | HPF14 | HPF21 | HPF22 | HPF23 | HPF24 | HPF31 | HPF32 | HPF33 | HPF43 | HPF41 | HPF42 | HPF43 | HPF44 |
HPF11 | 0.000 | 0.700 | 0.000 | 0.872 | 0.023 | 0.042 | 0.000 | 0.993 | 0.571 | 0.701 | 0.000 | 0.904 | 0.992 | 0.992 | 0.950 | 0.996 |
HPF12 | 0.300 | 0.000 | 0.000 | 0.852 | 0.019 | 0.031 | 0.000 | 0.992 | 0.366 | 0.570 | 0.000 | 0.888 | 0.992 | 0.992 | 0.950 | 0.996 |
HPF13 | 1.000 | 1.000 | 0.000 | 1.000 | 1.000 | 1.000 | 0.013 | 1.000 | 1.000 | 1.000 | 0.456 | 1.000 | 0.996 | 0.996 | 0.972 | 0.998 |
HPF14 | 0.128 | 0.148 | 0.000 | 0.000 | 0.000 | 0.000 | 0.000 | 1.000 | 0.131 | 0.152 | 0.000 | 0.949 | 0.991 | 0.991 | 0.947 | 0.996 |
HPF21 | 0.977 | 0.981 | 0.000 | 1.000 | 0.000 | 0.893 | 0.000 | 1.000 | 0.979 | 0.983 | 0.000 | 1.000 | 0.993 | 0.993 | 0.956 | 0.997 |
HPF22 | 0.959 | 0.969 | 0.000 | 1.000 | 0.107 | 0.000 | 0.000 | 1.000 | 0.962 | 0.971 | 0.000 | 1.000 | 0.993 | 0.993 | 0.955 | 0.997 |
HPF23 | 1.000 | 1.000 | 0.987 | 1.000 | 1.000 | 1.000 | 0.000 | 1.000 | 1.000 | 1.000 | 0.987 | 1.000 | 0.997 | 0.997 | 0.975 | 0.999 |
HPF24 | 0.007 | 0.008 | 0.000 | 0.000 | 0.000 | 0.000 | 0.000 | 0.000 | 0.007 | 0.008 | 0.000 | 0.001 | 0.989 | 0.989 | 0.936 | 0.995 |
HPF31 | 0.429 | 0.634 | 0.000 | 0.869 | 0.021 | 0.039 | 0.000 | 0.993 | 0.000 | 0.698 | 0.000 | 0.904 | 0.992 | 0.992 | 0.950 | 0.996 |
HPF32 | 0.299 | 0.430 | 0.000 | 0.849 | 0.017 | 0.029 | 0.000 | 0.992 | 0.302 | 0.000 | 0.000 | 0.888 | 0.992 | 0.992 | 0.950 | 0.996 |
HPF33 | 1.000 | 1.000 | 0.544 | 1.000 | 1.000 | 1.000 | 0.013 | 1.000 | 1.000 | 1.000 | 0.000 | 1.000 | 0.996 | 0.996 | 0.972 | 0.998 |
HPF34 | 0.096 | 0.112 | 0.000 | 0.051 | 0.000 | 0.000 | 0.000 | 0.999 | 0.096 | 0.112 | 0.000 | 0.000 | 0.991 | 0.991 | 0.946 | 0.996 |
HPF41 | 0.008 | 0.008 | 0.004 | 0.009 | 0.007 | 0.007 | 0.004 | 0.011 | 0.008 | 0.008 | 0.004 | 0.009 | 0.000 | 0.937 | 0.000 | 1.000 |
HPF42 | 0.008 | 0.008 | 0.004 | 0.009 | 0.007 | 0.007 | 0.003 | 0.011 | 0.008 | 0.008 | 0.004 | 0.009 | 0.063 | 0.000 | 0.000 | 1.000 |
HPF43 | 0.050 | 0.050 | 0.028 | 0.053 | 0.045 | 0.045 | 0.025 | 0.064 | 0.050 | 0.050 | 0.028 | 0.054 | 1.000 | 1.000 | 0.000 | 1.000 |
HPF44 | 0.004 | 0.004 | 0.002 | 0.004 | 0.003 | 0.003 | 0.002 | 0.006 | 0.004 | 0.004 | 0.002 | 0.004 | 0.000 | 0.000 | 0.000 | 0.000 |
The second scenario 80%, 20% | ||||||||||||||||
HPF11 | 0.000 | 0.665 | 0.000 | 0.740 | 0.012 | 0.029 | 0.000 | 0.959 | 0.562 | 0.655 | 0.000 | 0.781 | 0.950 | 0.950 | 0.881 | 0.961 |
HPF12 | 0.335 | 0.000 | 0.000 | 0.717 | 0.009 | 0.020 | 0.000 | 0.957 | 0.384 | 0.533 | 0.000 | 0.762 | 0.950 | 0.950 | 0.880 | 0.960 |
HPF13 | 1.000 | 1.000 | 0.000 | 1.000 | 1.000 | 1.000 | 0.002 | 1.000 | 1.000 | 1.000 | 0.406 | 1.000 | 0.965 | 0.965 | 0.912 | 0.972 |
HPF14 | 0.260 | 0.283 | 0.000 | 0.000 | 0.000 | 0.000 | 0.000 | 0.992 | 0.260 | 0.280 | 0.000 | 0.939 | 0.949 | 0.949 | 0.879 | 0.960 |
HPF21 | 0.988 | 0.991 | 0.000 | 1.000 | 0.000 | 0.943 | 0.000 | 1.000 | 0.990 | 0.992 | 0.000 | 1.000 | 0.954 | 0.955 | 0.889 | 0.964 |
HPF22 | 0.972 | 0.980 | 0.000 | 1.000 | 0.057 | 0.000 | 0.000 | 1.000 | 0.975 | 0.982 | 0.000 | 1.000 | 0.954 | 0.954 | 0.888 | 0.964 |
HPF23 | 1.000 | 1.000 | 0.998 | 1.000 | 1.000 | 1.000 | 0.000 | 1.000 | 1.000 | 1.000 | 0.998 | 1.000 | 0.968 | 0.969 | 0.918 | 0.975 |
HPF24 | 0.041 | 0.043 | 0.000 | 0.008 | 0.000 | 0.000 | 0.000 | 0.000 | 0.040 | 0.041 | 0.000 | 0.011 | 0.944 | 0.945 | 0.869 | 0.956 |
HPF31 | 0.438 | 0.616 | 0.000 | 0.740 | 0.010 | 0.025 | 0.000 | 0.960 | 0.000 | 0.649 | 0.000 | 0.785 | 0.950 | 0.950 | 0.881 | 0.961 |
HPF32 | 0.345 | 0.467 | 0.000 | 0.720 | 0.008 | 0.018 | 0.000 | 0.959 | 0.351 | 0.000 | 0.000 | 0.767 | 0.950 | 0.950 | 0.880 | 0.960 |
HPF33 | 1.000 | 1.000 | 0.594 | 1.000 | 1.000 | 1.000 | 0.002 | 1.000 | 1.000 | 1.000 | 0.000 | 1.000 | 0.965 | 0.966 | 0.912 | 0.972 |
HPF34 | 0.219 | 0.238 | 0.000 | 0.062 | 0.000 | 0.000 | 0.000 | 0.989 | 0.215 | 0.233 | 0.000 | 0.000 | 0.949 | 0.949 | 0.878 | 0.960 |
HPF41 | 0.050 | 0.050 | 0.035 | 0.051 | 0.046 | 0.046 | 0.032 | 0.056 | 0.050 | 0.050 | 0.035 | 0.051 | 0.000 | 0.900 | 0.000 | 1.000 |
HPF42 | 0.050 | 0.050 | 0.035 | 0.051 | 0.045 | 0.046 | 0.032 | 0.055 | 0.050 | 0.050 | 0.035 | 0.051 | 0.100 | 0.000 | 0.000 | 1.000 |
HPF43 | 0.120 | 0.120 | 0.088 | 0.122 | 0.111 | 0.112 | 0.082 | 0.131 | 0.120 | 0.120 | 0.088 | 0.122 | 1.000 | 1.000 | 0.000 | 1.000 |
HPF44 | 0.040 | 0.040 | 0.028 | 0.040 | 0.036 | 0.036 | 0.025 | 0.044 | 0.040 | 0.040 | 0.028 | 0.040 | 0.001 | 0.001 | 0.000 | 0.000 |
The third scenario 90%, 10% | ||||||||||||||||
HPF11 | 0.000 | 0.244 | 0.000 | 0.724 | 0.035 | 0.044 | 0.000 | 0.923 | 0.481 | 0.279 | 0.000 | 0.749 | 0.927 | 0.927 | 0.886 | 0.940 |
HPF12 | 0.756 | 0.000 | 0.000 | 0.754 | 0.043 | 0.050 | 0.000 | 0.930 | 0.709 | 0.482 | 0.000 | 0.777 | 0.927 | 0.927 | 0.886 | 0.940 |
HPF13 | 1.000 | 1.000 | 0.000 | 1.000 | 0.994 | 0.995 | 0.010 | 1.000 | 1.000 | 1.000 | 0.536 | 1.000 | 0.937 | 0.937 | 0.901 | 0.949 |
HPF14 | 0.277 | 0.246 | 0.000 | 0.000 | 0.000 | 0.001 | 0.000 | 0.976 | 0.275 | 0.245 | 0.000 | 0.813 | 0.926 | 0.925 | 0.884 | 0.939 |
HPF21 | 0.965 | 0.957 | 0.006 | 1.000 | 0.000 | 0.731 | 0.000 | 1.000 | 0.965 | 0.958 | 0.006 | 1.000 | 0.930 | 0.930 | 0.890 | 0.942 |
HPF22 | 0.956 | 0.950 | 0.005 | 0.999 | 0.269 | 0.000 | 0.000 | 1.000 | 0.956 | 0.951 | 0.005 | 1.000 | 0.930 | 0.929 | 0.890 | 0.942 |
HPF23 | 1.000 | 1.000 | 0.990 | 1.000 | 1.000 | 1.000 | 0.000 | 1.000 | 1.000 | 1.000 | 0.991 | 1.000 | 0.940 | 0.939 | 0.904 | 0.951 |
HPF24 | 0.077 | 0.070 | 0.000 | 0.024 | 0.000 | 0.000 | 0.000 | 0.000 | 0.076 | 0.069 | 0.000 | 0.029 | 0.922 | 0.922 | 0.879 | 0.935 |
HPF31 | 0.520 | 0.291 | 0.000 | 0.725 | 0.035 | 0.044 | 0.000 | 0.924 | 0.000 | 0.245 | 0.000 | 0.752 | 0.927 | 0.927 | 0.886 | 0.940 |
HPF32 | 0.721 | 0.518 | 0.000 | 0.755 | 0.043 | 0.049 | 0.000 | 0.931 | 0.755 | 0.000 | 0.000 | 0.781 | 0.927 | 0.927 | 0.886 | 0.940 |
HPF33 | 1.000 | 1.000 | 0.464 | 1.000 | 0.994 | 0.995 | 0.009 | 1.000 | 1.000 | 1.000 | 0.000 | 1.000 | 0.937 | 0.937 | 0.901 | 0.949 |
HPF34 | 0.251 | 0.223 | 0.000 | 0.187 | 0.000 | 0.001 | 0.000 | 0.971 | 0.248 | 0.219 | 0.000 | 0.000 | 0.925 | 0.925 | 0.884 | 0.939 |
HPF41 | 0.073 | 0.073 | 0.063 | 0.075 | 0.070 | 0.071 | 0.061 | 0.078 | 0.073 | 0.073 | 0.063 | 0.075 | 0.000 | 0.742 | 0.000 | 0.989 |
HPF42 | 0.074 | 0.073 | 0.063 | 0.075 | 0.071 | 0.071 | 0.061 | 0.078 | 0.074 | 0.073 | 0.063 | 0.075 | 0.258 | 0.000 | 0.000 | 0.981 |
HPF43 | 0.114 | 0.114 | 0.099 | 0.116 | 0.110 | 0.110 | 0.096 | 0.121 | 0.114 | 0.114 | 0.099 | 0.116 | 1.000 | 1.000 | 0.000 | 1.000 |
HPF44 | 0.060 | 0.060 | 0.051 | 0.061 | 0.058 | 0.058 | 0.049 | 0.065 | 0.060 | 0.060 | 0.051 | 0.062 | 0.012 | 0.019 | 0.000 | 0.000 |
After evaluating model performance using accuracy metric errors and the DM test, the next step is to determine the dominance of these findings. Figures 4 and 5 show a graphical depiction of the performance measures (MAE, MAPE, MSPE, RMSE) using bar plots for this purpose. The following is how these figures are arranged: (a, d, g, j) the first scenario results (70%, 30%), (b, e, h, k) the second scenario results (80%, 20%) and (c, f, i, l) the third scenario results (90%, 10%). It is clear from the numbers that the HPF23 model outperformed all other combination models across all three training and testing sample data sets. Additionally, The correlation plot of the best model (HPF23) out of all sixteen models in each training and testing scenario is shown in Figure 6. This includes the first scenario results (70%, 30%), the second scenario results (80%, 20%) and the third scenario results (90%, 10%). The figures indicate that the optimal model has the highest correlation coefficient values and a significant correlation between actual and projected values. Based on the accuracy metric errors, statistical test (DM test) and graphical results (bar plots and correlation plots), it can be inferred that the proposed filtering-combination approach is highly efficient and accurate for forecasting stock market daily closing prices. On the other hand, it is concluded that the HPF23 combination model is more precise in generating forecasts compared to other combination models within the proposed filtering-combination technique.
As confirmed from the findings of the previous section, such as performance measures (MAE, MAPE, RMSPE, MDA and CC), a statistical test (the DM test) and graphical analysis (the bar-plot, correlation plot), the final best combination model was HPF23, which was highly accurate and efficient in forecasting the daily closing stock price of the PSX. Hence, it is important to mention that the obtained results from the considered benchmark models and the declared proposed best model are highly comparatively. The considered benchmark models include; three standard time series models, such as the autoregressive, autoregressive moving integrated average, nonparametric autoregressive and autoregressive neural network models. Hence, a comparative analysis of the proposed best model versed the considered benchmark models are numerically listed in Table 6 and graphically shown in Figure 7 for all three training and testing scenarios. From these presentations, one can observe that the final best combination model HPF23 in this work produced significantly lowest accuracy mean errors and higher accuracy values of CC and MDA as compared to the autoregressive, autoregressive moving integrated average, nonparametric autoregressive and autoregressive neural network models. Moreover, to confirm the superiority of the proposed best combination model HPF23 mentioned in Table 6, we performed the DM test on each pair of models. The outcomes (p-values) of the DM test are numerically listed in Table 7 for all three training and testing scenarios. It is confirmed from these results, that all considered bookmark models (time series and machine learning models) are outperformed by our proposed best combination model (HPF23) at the 5% significance level. To conclude, based on all of these findings, the accuracy of the proposed filtering-combination technique is comparatively high and efficient when compared with all considered competitor models.
The first scenario 70%, 30% | |||||||
S.No | Models | RMSPE | MAPE | MAE | RMSE | CC | MDA |
1 | HPF23 | 120.8022 | 0.2924 | 0.0041 | 161.9550 | 0.9992 | 0.9302 |
2 | AR | 156.5730 | 0.3786 | 0.0053 | 209.6822 | 0.9987 | 0.8966 |
3 | ARIMA | 157.1322 | 0.3796 | 0.0053 | 210.0071 | 0.9987 | 0.8980 |
4 | NPAR | 127.3876 | 0.3077 | 0.0043 | 170.2426 | 0.9991 | 0.9195 |
5 | ANN | 162.1289 | 0.3923 | 0.0054 | 214.3426 | 0.9986 | 0.9128 |
The second scenario 80%, 20% | |||||||
1 | HPF23 | 115.5138 | 0.2622 | 0.0034 | 149.8806 | 0.9980 | 0.9253 |
2 | AR | 151.9299 | 0.3447 | 0.0045 | 199.3006 | 0.9964 | 0.8747 |
3 | ARIMA | 152.0373 | 0.3448 | 0.0045 | 199.5532 | 0.9964 | 0.8788 |
4 | NPAR | 122.9880 | 0.2793 | 0.0037 | 160.4473 | 0.9977 | 0.9071 |
5 | ANN | 155.6473 | 0.3531 | 0.0046 | 202.1268 | 0.9963 | 0.8929 |
The third scenario 90%, 10% | |||||||
1 | HPF23 | 121.8376 | 0.2866 | 0.0036 | 151.6476 | 0.9958 | 0.9429 |
2 | AR | 156.4563 | 0.3683 | 0.0047 | 199.1440 | 0.9927 | 0.8735 |
3 | ARIMA | 155.4268 | 0.3658 | 0.0047 | 198.6606 | 0.9927 | 0.8735 |
4 | NPAR | 130.1624 | 0.3064 | 0.0038 | 163.0377 | 0.9951 | 0.9143 |
5 | ANN | 159.5017 | 0.3754 | 0.0048 | 202.7243 | 0.9924 | 0.8857 |
The first scenario 70%, 30% | |||||
models | HPF23 | AR | ARIMA | NPAR | ANN |
HPF23 | 0.000 | 1.000 | 1.000 | 0.987 | 1.000 |
AR | 0.000 | 0.000 | 0.698 | 0.000 | 0.904 |
ARIMA | 0.000 | 0.302 | 0.000 | 0.000 | 0.888 |
NPAR | 0.013 | 1.000 | 1.000 | 0.000 | 1.000 |
ANN | 0.000 | 0.096 | 0.112 | 0.000 | 0.000 |
The second scenario 80%, 20% | |||||
HPF23 | 0.000 | 1.000 | 1.000 | 0.998 | 1.000 |
AR | 0.000 | 0.000 | 0.649 | 0.000 | 0.785 |
ARIMA | 0.000 | 0.351 | 0.000 | 0.000 | 0.767 |
NPAR | 0.002 | 1.000 | 1.000 | 0.000 | 1.000 |
ANN | 0.000 | 0.215 | 0.233 | 0.000 | 0.000 |
The third scenario 90%, 10% | |||||
HPF23 | 0.000 | 1.000 | 1.000 | 0.991 | 1.000 |
AR | 0.000 | 0.000 | 0.245 | 0.000 | 0.752 |
ARIMA | 0.000 | 0.755 | 0.000 | 0.000 | 0.781 |
NPAR | 0.009 | 1.000 | 1.000 | 0.000 | 1.000 |
ANN | 0.000 | 0.248 | 0.219 | 0.000 | 0.000 |
It is worth noting the literature's arguments about the pros and cons of using HP filters vary [46,48,49,50,51]. Some researchers say that when applied to statistical data, the HP filter creates illusorycycles [48], while others claim that it is better treated using a random walk and therefore a distinct stationary process [46]. However, in the current study, the HP filter is utilized to extract a nonlinear trend component from daily data rather than a quarterly, monthly or annual dataset. In this regard, the authors argue that the complexity of diverse datasets varies and that no single technique, method or model is ideal in all circumstances. What performs best for one type of dataset may not work for another. As a result, each method or model has advantages and disadvantages. Furthermore, we are not entirely reliant on the HP filter; the HP filter was utilized only for decomposition. After obtaining the deconstructed series, we processed them for further modeling and forecasting applications. In this regard, this work obtained a stationary series by employing the first difference approach and then processing it with standard linear and nonlinear time series models. In summary, we can observe that the final best ensemble model within the proposed forecasting methodology was the combination of the nonlinear autoregressive model and the autoregressive moving average model. It was evidence for capturing the nonlinearity and complexity of the data well within the proposed methodology.
Day-ahead or short-term forecasting is a valuable tool for companies in today's modern world. It helps with operational planning by allowing companies to efficiently adjust production schedules, improve logistics and allocate resources. Additionally, short-term forecasting is useful for risk management as it enables traders to measure market volatility and control portfolio risk. Accurate and efficient day-ahead forecasts also assist traders in making informed decisions regarding the purchase and sale of commodities. Furthermore, these models can be beneficial to traders in developing short-term trading strategies for profitable trading. Moreover, this research shows that forecasting price indices can be useful for traders and investors who are interested in index-linked funds and index derivatives traded in the derivatives market. The proposed forecasting method can also be useful for policymakers, mutual funds, investment bankers, FIIs, arbitrage traders and other traders when forecasting economic or financial time series data. These research findings will be of particular interest to investors, traders, regulators and anyone who deals with the stock market. By making use of day-ahead forecasts and knowledge of stock index trends, traders can create more profitable business and trading plans and make useful asset allocation decisions. Additionally, based on our forecasts, we can take measures to mitigate possible exchange rate risks. By choosing the best-proposed model, traders can develop a more robust trading plan and select the model with the best risk-reward combination.
The main objective of our research was to forecast the daily closing stock price of the Pakistan Stock Exchange. To achieve this goal, we proposed a unique filtering-combination forecasting approach that involved using the HPF filter to divide the original time series of the daily closing price into two new subseries: A nonlinear long-term trend series and a stochastic series. The filtered series were predicted by four standard time-series models-two linear and two nonlinear-including the autoregressive moving average model, the nonparametric autoregressive model and the autoregressive neural network, as well as all possible combinations of these models. The proposed forecasting technique was applied and evaluated with daily close stock prices in Pakistan from January 1, 2013, to February 13, 2023. Six different accuracy measures, pictorial analysis and a statistical test were performed across three different scenarios of training and testing data, i.e., (70%, 30%), (80%, 20%) and (90%, 10%). For each of these three scenarios, the HPF23 model was found to be the best-proposed combination model because it resulted in lower accuracy measures and larger values for CC and MDA. However, the HPF13 and HPF33 models were found to be good competitors. After that, we compared the best-proposed combination model to the benchmark models (the autoregressive model, the autoregressive moving integrated average model, the nonparametric autoregressive model and the autoregressive neural network) by using different accuracy measures and the DM test, showing that the proposed model outperformed the other models.
Hence, we focused solely on closing prices. Moreover, the filtering-combination forecasting technique can be extended to other variables such as high and low prices, daily open prices, daily volume and more. Additionally, while we utilized only univariate linear and nonlinear time-series models, plan to expand this approach in the future to include machine learning and deep learning models such as random forests, decision trees, support vector machines, long short-term memory networks, convolutional neural networks and recurrent neural networks. Furthermore, we employed the HP filter in the current proposal. Furthermore, we intend to incorporate other filters and evaluate the performance of different filters within the proposed filtering-combination time series models, such as Hamilton's filter, robust regression filters, exponential moving filters, smoothing spline regression filters, etc. Finally, we believe that this filtering-combination forecasting method can obtain highly efficient and accurate forecasts for other complex financial time-series data such as inflation, unemployment and cryptocurrencies. Likewise, in other scenarios and with different data, for example, energy [53,54], air pollution [55,56,57,58,59], solid waste [60], academic performance [61] and digital marketing [62].
The authors declare that they have not used Artificial Intelligence (AI) tools in the creation of this article.
P. C. Rodrigues acknowledges financial support from the CNPq grant "bolsa de produtividade PQ-2" 309359/2022-8, Federal University of Bahia and CAPES-PRINT-UFBA, under the topic "Modelos Matemáticos, Estatísticos e Computacionais Aplicados às Ciências da Natureza".
The authors declare no conflicts of interest.
[1] | L. O. Petram, The world's first stock exchange: How the Amsterdam market for Dutch East India Company shares became a modern securities market, Doctoral dissertation, Universiteit van Amsterdam, 2011, 1602–1700. |
[2] |
L. A. Castillo, M. J. Orraca, G. S. Molina, The global component of headline and core inflation in emerging market economies and its ability to improve forecasting performance, Econ. Model., 120 (2023), 106121. https://doi.org/10.1016/j.econmod.2022.106121 doi: 10.1016/j.econmod.2022.106121
![]() |
[3] |
C. He, K. Huang, J. Lin, T. Wang, Z. Zhang, Explain systemic risk of commodity futures market by dynamic network, Int. Rev. Financ. Anal., 88 (2023), 102658. https://doi.org/10.1016/j.irfa.2023.102658 doi: 10.1016/j.irfa.2023.102658
![]() |
[4] |
I. K. Nti, A. F. Adekoya, B. A. Weyori, A systematic review of fundamental and technical analysis of stock market predictions, Artif. Intell. Rev., 53 (2020), 3007–3057. https://doi.org/10.1007/s10462-019-09754-z doi: 10.1007/s10462-019-09754-z
![]() |
[5] |
Z. Li, X. Zhou, S. Huang, Managing skill certification in online outsourcing platforms: A perspective of buyer-determined reverse auctions, Int. J. Prod. Econ., 238 (2021), 108166. https://doi.org/10.1016/j.ijpe.2021.108166 doi: 10.1016/j.ijpe.2021.108166
![]() |
[6] |
E. Catullo, M. Gallegati, A. Russo, Forecasting in a complex environment: Machine learning sales expectations in a stock flow consistent agent-based simulation model, J. Econ. Dyn. Control, 139 (2022), 104405. https://doi.org/10.1016/j.jedc.2022.104405 doi: 10.1016/j.jedc.2022.104405
![]() |
[7] |
A. Bucci, G. Palomba, E. Rossi, The role of uncertainty in forecasting volatility comovements across stock markets, Econ. Modell., 125 (2023), 106309. https://doi.org/10.1016/j.econmod.2023.106309 doi: 10.1016/j.econmod.2023.106309
![]() |
[8] |
M. M. Kumbure, C. Lohrmann, P. Luukka, J. Porras, Machine learning techniques and data for stock market forecasting: A literature review, Expert Syst. Appl., 2022, 116659. https://doi.org/10.1016/j.eswa.2022.116659 doi: 10.1016/j.eswa.2022.116659
![]() |
[9] |
H. Iftikhar, J. E. T. Chaparro, P. C. Rodrigues, J. L. López-Gonzales, Forecasting Day-Ahead electricity prices for the Italian electricity market using a new decomposition-combination technique, Energies, 16 (2023), 6669. https://doi.org/10.3390/en16186669 doi: 10.3390/en16186669
![]() |
[10] |
N. C. Bustinza, H. Iftikhar, M. Belmonte, R. J. C. Torres, A. R. H. De La Cruz, J. L. López-Gonzales, Short-term forecasting of Ozone concentration in metropolitan Lima using hybrid combinations of time series models, Appl. Sci., 13 (2023), 10514. https://doi.org/10.3390/app131810514 doi: 10.3390/app131810514
![]() |
[11] |
X. Li, Y. Sun, Stock intelligent investment strategy based on support vector machine parameter optimization algorithm, Neural Comput. Appl., 32 (2020), 1765–1775. https://doi.org/10.1007/s00521-019-04566-2 doi: 10.1007/s00521-019-04566-2
![]() |
[12] |
P. Mondal, L. Shit, S. Goswami, Study of effectiveness of time series modeling (ARIMA) in forecasting stock prices, Int. J. Comput. Sci. Eng. Appl., 4 (2014), 13. https://doi.org/10.5121/ijcsea.2014.4202 doi: 10.5121/ijcsea.2014.4202
![]() |
[13] |
B. Wang, H. Huang, X. Wang, A novel text mining approach to financial time series forecasting, Neurocomputing, 83 (2012), 136–145. https://doi.org/10.1016/j.neucom.2011.12.013 doi: 10.1016/j.neucom.2011.12.013
![]() |
[14] |
M. Ghani, Q. Guo, F. Ma, T. Li, Forecasting Pakistan stock market volatility: Evidence from economic variables and the uncertainty index, Int. Rev. Econ. Financ., 80 (2022), 1180–1189. https://doi.org/10.1016/j.iref.2022.04.003 doi: 10.1016/j.iref.2022.04.003
![]() |
[15] |
D. Kumar, P. K. Sarangi, R. Verma, A systematic review of stock market prediction using machine learning and statistical techniques, Materials Today Proc., 49 (2022), 3187–3191. https://doi.org/10.1016/j.matpr.2020.11.399 doi: 10.1016/j.matpr.2020.11.399
![]() |
[16] |
S. Raubitzek, T. Neubauer, An exploratory study on the complexity and machine learning predictability of stock market data, Entropy, 24 (2022), 332. https://doi.org/10.3390/e24030332 doi: 10.3390/e24030332
![]() |
[17] |
X. Li, Y. Sun, Application of RBF neural network optimal segmentation algorithm in credit rating, Neural Comput. Appl., 33 (2021), 8227–8235. https://doi.org/10.1007/s00521-020-04958-9 doi: 10.1007/s00521-020-04958-9
![]() |
[18] |
H. Dichtl, W. Drobetz, T. Otto, Forecasting stock market crashes via machine learning, J. Financ. Stabil., 65 (2023), 101099. https://doi.org/10.1016/j.jfs.2022.101099 doi: 10.1016/j.jfs.2022.101099
![]() |
[19] | J. Kamruzzaman, R. A. Sarker, ANN-based forecasting of foreign currency exchange rates, Neural Inform. Process.-Lett. Rev., 3 (2004), 49–58. |
[20] |
H. Iftikhar, A. Zafar, J. E. T. Chaparro, P. C. Rodrigues, J. L. López-Gonzales, Forecasting Day-Ahead Brent crude oil prices using hybrid combinations of time series models, Mathematics, 11 (2023), 3548. https://doi.org/10.3390/math11163548 doi: 10.3390/math11163548
![]() |
[21] |
C. Ma, D. Wen, G. J. Wang, Y. Jiang, Further mining the predictability of moving averages: Evidence from the US stock market, Int. Rev. Financ., 19 (2019), 413–433. https://doi.org/10.1111/irfi.12166 doi: 10.1111/irfi.12166
![]() |
[22] |
Z. Jiang, C. Xu, Disrupting the technology innovation efficiency of manufacturing enterprises through digital technology promotion: An evidence of 5G technology construction in China, IEEE T. Eng. Manage., 2023. https://doi.org/10.1109/TEM.2023.3261940 doi: 10.1109/TEM.2023.3261940
![]() |
[23] |
L. Lin, Y. Jiang, H. Xiao, Z. Zhou, Crude oil price forecasting based on a novel hybrid long memory GARCH-M and wavelet analysis model, Phys. A, 543 (2020), 123532. https://doi.org/10.1016/j.physa.2019.123532 doi: 10.1016/j.physa.2019.123532
![]() |
[24] |
Z. Zhou, Y. Jiang, Y. Liu, L. Lin, Q. Liu, Does international oil volatility have directional predictability for stock returns? Evidence from BRICS countries based on cross-quantilogram analysis, Econ. Modell., 80 (2019), 352–382. https://doi.org/10.1016/j.econmod.2018.11.021 doi: 10.1016/j.econmod.2018.11.021
![]() |
[25] |
C. H. Cheng, M. C. Tsai, C. Chang, A time series model based on deep learning and integrated indicator selection method for forecasting stock prices and evaluating trading profits, Systems, 10 (2022), 243. https://doi.org/10.3390/systems10060243 doi: 10.3390/systems10060243
![]() |
[26] |
Y. Han, J. Kim, D. Enke, A machine learning trading system for the stock market based on N-period Min-Max labeling using XGBoost, Expert Syst. Appl., 211 (2023), 118581. https://doi.org/10.1016/j.eswa.2022.118581 doi: 10.1016/j.eswa.2022.118581
![]() |
[27] |
H. Iftikhar, N. Bibi, P. C. Rodrigues, J. L. López-Gonzales, Multiple novel decomposition techniques for time series forecasting: Application to monthly forecasting of electricity consumption in Pakistan, Energies, 2023 (2023), 2579. https://doi.org/10.3390/en16062579 doi: 10.3390/en16062579
![]() |
[28] |
H. Iftikhar, M. Daniyal, M. Qureshi, K. Tawaiah, R. K. Ansah, J. K. Afriyie, A hybrid forecasting technique for infection and death from the mpox virus, Digit. Health, 9 (2023). https://doi.org/10.1177/20552076231204748 doi: 10.1177/20552076231204748
![]() |
[29] |
H. Iftikhar, J. E. T. Chaparro, P. C. Rodrigues, J. L. López-Gonzales, Day-Ahead electricity demand forecasting using a novel decomposition combination method, Energies, 16 (2023), 6675. https://doi.org/10.3390/en16186675 doi: 10.3390/en16186675
![]() |
[30] |
H. Iftikhar, M. Khan, M. S. Khan, M. Khan, Short-term forecasting of Monkeypox cases using a novel filtering and combining technique, Diagnostics, 13 (2023), 1923. https://doi.org/10.3390/diagnostics13111923 doi: 10.3390/diagnostics13111923
![]() |
[31] |
Q. M. Ilyas, K. Iqbal, S. Ijaz, A. Mehmood, S. Bhatia, A hybrid model to predict stock closing price using novel features and a fully modified Hodrick-Prescott filter, Electronics, 11 (2022), 3588. https://doi.org/10.3390/electronics11213588 doi: 10.3390/electronics11213588
![]() |
[32] |
I. Daubechies, J. Lu, H. T. Wu, Synchrosqueezed wavelet transforms: An empirical mode decomposition-like tool, Appl. Comput. Harmon. A., 30 (2011), 243–261. https://doi.org/10.1016/j.acha.2010.08.002 doi: 10.1016/j.acha.2010.08.002
![]() |
[33] |
Z. Zhou, L. Lin, S. Li, International stock market contagion: A CEEMDAN wavelet analysis, Econ. Model., 72 (2018), 333–352. https://doi.org/10.1016/j.econmod.2018.02.010 doi: 10.1016/j.econmod.2018.02.010
![]() |
[34] |
M. Ali, D. M. Khan, H. M. Alshanbari, A. A. A. H. El-Bagoury, Prediction of complex stock market data using an improved hybrid EMD-LSTM model, Appl. Sci., 13 (2023), 1429. https://doi.org/10.3390/app13031429 doi: 10.3390/app13031429
![]() |
[35] |
I. Shah, H. Iftikhar, S. Ali, D. Wang, Short-term electricity demand forecasting using components estimation technique, Energies, 12 (2019), 2532. https://doi.org/10.3390/en12132532 doi: 10.3390/en12132532
![]() |
[36] | H. Iftikhar, Modeling and forecasting complex time series: A case of electricity demand, M. Phil, thesis, Quaidi-Azam University, Islamabad, Pakistan, 2018, 1–94. |
[37] |
I. Shah, H. Iftikhar, S. Ali, Modeling and forecasting electricity demand and prices: A comparison of alternative approaches, J. Math., 2022 (2022), 3581037. https://doi.org/10.1155/2022/3581037 doi: 10.1155/2022/3581037
![]() |
[38] |
F. X. Diebold, R. S. Mariano, Comparing predictive accuracy, J. Bus. Econ. Stat., 20 (2002), 134–144. https://doi.org/10.1198/073500102753410444 doi: 10.1198/073500102753410444
![]() |
[39] |
I. Shah, H. Iftikhar, S. Ali, Modeling and forecasting medium-term electricity consumption using component estimation technique, Forecasting, 2 (2020), 163–179. https://doi.org/10.3390/forecast2020009 doi: 10.3390/forecast2020009
![]() |
[40] |
H. Iftikhar, M. Khan, Z. Khan, F. Khan, H. M. Alshanbari, Z. Ahmad, A comparative analysis of machine learning models: A case study in predicting chronic kidney disease, Sustainability, 15 (2023), 2754. https://doi.org/10.3390/su15032754 doi: 10.3390/su15032754
![]() |
[41] |
H. M. Alshanbari, H. Iftikhar, F. Khan, M. Rind, Z. Ahmad, A. A. A. H. El-Bagoury, On the implementation of the artificial neural network approach for forecasting different healthcare events, Diagnostics, 7 (2023), 1310. https://doi.org/10.3390/diagnostics13071310 doi: 10.3390/diagnostics13071310
![]() |
[42] |
D. A. Dickey, W. A. Fuller, Distribution of the estimators for autoregressive time series with a unit root, J. Am. Stat. Assoc., 74 (1979), 427–431. https://doi.org/10.1080/01621459.1979.10482531 doi: 10.1080/01621459.1979.10482531
![]() |
[43] |
T. Teraesvirta, C. F. Lin, C. W. J. Granger, Power of the neural network linearity test, J. Time Ser. Anal., 14 (1993), 209–220. https://doi.org/10.1111/j.1467-9892.1993.tb00139.x doi: 10.1111/j.1467-9892.1993.tb00139.x
![]() |
[44] | P. J. Brockwell, R. A. Davis, Introduction to time series and forecasting, Berlin/Heidelberg: Springer, 2016. |
[45] |
Q. Gu, S. Li, Z. Liao, Solving nonlinear equation systems based on evolutionary multitasking with neighborhood-based speciation differential evolution, Expert Syst. Appl., 238 (2024), 122025. https://doi.org/10.1016/j.eswa.2023.122025 doi: 10.1016/j.eswa.2023.122025
![]() |
[46] |
M. Dritsaki, C. Dritsaki, Comparison of HP filter and the Hamilton's regression, Mathematics, 10 (2022), 1237. https://doi.org/10.3390/math10081237 doi: 10.3390/math10081237
![]() |
[47] | P. C. Phillips, Z. Shi, Boosting the Hodrick-Prescott filter, 2019. |
[48] |
J. D. Hamilton, Why you should never use the Hodrick-Prescott filter, Rev. Econ. Stat., 100 (2018), 831–843. https://doi.org/10.1162/rest_a_00706 doi: 10.1162/rest_a_00706
![]() |
[49] |
P. C. B. Phillips, Z. Shi, Boosting: Why you can use the HP filter, Int. Econ. Rev., 62 (2021), 521–570. https://doi.org/10.1111/iere.12495 doi: 10.1111/iere.12495
![]() |
[50] | E. Wolf, F. Mokinski, Y. Schüler, On adjusting the one-sided Hodrick-Prescott filter, Deutsche Bundesbank: Frankfurt, 2020. |
[51] | R. J. Hodrick, E. Prescott, U. S. Postwar, Business cycles: An empirical investigation, J. Money Credit Bank., 29 (1997), 1–16. |
[52] | M. Ravn, H. Uhlig, On adjusting the HP-filter for the frequency of observations, Rev. Econ. Stat., 84 (2002), 371–380. |
[53] |
J. L. L. Gonzales, R. F. Calili, R. C. Souza, F. L. Coelho da Silva, Simulation of the energy efficiency auction prices in Brazil, Renew. Energy Power Qual. J., 1 (2016), 574–579. https://doi.org/10.48550/arXiv.1811.04144 doi: 10.48550/arXiv.1811.04144
![]() |
[54] |
J. L. L. Gonzales, R. C. Souza, F. L. C. Da Silva, N. C. Bustinza, G. I. Pulgar, R. F. Calili, Simulation of the energy efficiency auction prices via the markov chain monte carlo method, Energies, 13 (2020), 4544. https://doi.org/10.3390/en13174544 doi: 10.3390/en13174544
![]() |
[55] |
N. C. Bustinza, M. Belmonte, V. Jimenez, P. Montalban, M. Rivera, F. G. Martínez, et al., A machine learning approach to analyse ozone concentration in metropolitan area of Lima, Peru, Sci. Rep.-UK, 12 (2022), 22084. https://doi.org/10.1038/s41598-022-26575-3 doi: 10.1038/s41598-022-26575-3
![]() |
[56] |
K. L. S. da Silva, J. L. L. Gonzales, J. E. T. Chaparro, E. T. Cano, P. C. Rodrigues, Spatio-temporal visualization and forecasting of PM10 in the Brazilian state of Minas Gerais, Sci. Rep.-UK, 13 (2023), 3269. https://doi.org/10.1038/s41598-023-30365-w doi: 10.1038/s41598-023-30365-w
![]() |
[57] |
N. Jeldes, G. I. Pulgar, C. Marchant, J. L. López-Gonzales, Modeling air pollution using partially varying coefficient models with heavy tails, Mathematics, 10 (2022), 3677. https://doi.org/10.3390/math10193677 doi: 10.3390/math10193677
![]() |
[58] |
R. J. C. Torres, M. A. P. Estela, O. S. Ccoyllo, E. A. R. Cabello, F. F. G. Ávila, C. A. C. Olivera, et al., Statistical modeling approach for PM10 prediction before and during confinement by COVID-19 in South Lima, Perú, Sci. Rep.-UK, 12 (2022). https://doi.org/10.1038/s41598-022-20904-2 doi: 10.1038/s41598-022-20904-2
![]() |
[59] |
A. R. H. de la Cruz, R. F. O. Ayuque, R. W. H. de la Cruz, J. L. López-Gonzales, A. Gioda, Air quality biomonitoring of trace elements in the metropolitan area of Huancayo, Peru using transplanted Tillandsia capillaris as a biomonitor, An. Acad. Bras. Cienc., 92 (2020). https://doi.org/10.1590/0001-3765202020180813 doi: 10.1590/0001-3765202020180813
![]() |
[60] |
K. Quispe, M. Martínez, K. da Costa, H. R. Giron, J. F. Via y Rada Vittes, L. D. M. Mincami, et al., Solid waste management in Peru's cities: A clustering approach for an Andean district, Appl. Sci., 13 (2023), 1646. https://doi.org/10.3390/app13031646 doi: 10.3390/app13031646
![]() |
[61] |
D. O. Granados, J. Ugalde, R. Salas, R. Torres, J. L. L. Gonzales, Visual-Predictive data analysis approach for the academic performance of students from a Peruvian University, Appl. Sci., 12 (2022), 11251. https://doi.org/10.3390/app122111251 doi: 10.3390/app122111251
![]() |
[62] |
J. S. Garcés, J. J. Soria, J. E. T. Chaparro, H. A. George, J. L. L. Gonzales, Implementing the reconac marketing strategy for the interaction and brand adoption of peruvian university students, Appl. Sci., 11 (2021), 1–11. https://doi.org/10.3390/app11052131 doi: 10.3390/app11052131
![]() |
1. | Flor Quispe, Eddy Salcedo, Hasnain Iftikhar, Aimel Zafar, Murad Khan, Josué E. Turpo-Chaparro, Paulo Canas Rodrigues, Javier Linkolk López-Gonzales, Multi-step ahead ozone level forecasting using a component-based technique: A case study in Lima, Peru, 2024, 11, 2372-0352, 401, 10.3934/environsci.2024020 | |
2. | Wilfredo Meza Cuba, Juan Carlos Huaman Alfaro, Hasnain Iftikhar, Javier Linkolk López-Gonzales, Modeling and Analysis of Monkeypox Outbreak Using a New Time Series Ensemble Technique, 2024, 13, 2075-1680, 554, 10.3390/axioms13080554 | |
3. | Hasnain Iftikhar, Moiz Qureshi, Justyna Zywiołek, Javier Linkolk López-Gonzales, Olayan Albalawi, Short-term PM2.5 forecasting using a unique ensemble technique for proactive environmental management initiatives, 2024, 12, 2296-665X, 10.3389/fenvs.2024.1442644 | |
4. | Hasnain Iftikhar, Murad Khan, Justyna Żywiołek, Mehak Khan, Javier Linkolk López-Gonzales, Modeling and forecasting carbon dioxide emission in Pakistan using a hybrid combination of regression and time series models, 2024, 10, 24058440, e33148, 10.1016/j.heliyon.2024.e33148 | |
5. | Hasnain Iftikhar, Salvatore Mancha Gonzales, Justyna Zywiołek, Javier Linkolk López-Gonzales, Electricity Demand Forecasting Using a Novel Time Series Ensemble Technique, 2024, 12, 2169-3536, 88963, 10.1109/ACCESS.2024.3419551 | |
6. | Ibrar Hussain, Moiz Qureshi, Muhammad Ismail, Hasnain Iftikhar, Justyna Zywiołek, Javier Linkolk López-Gonzales, Optimal features selection in the high dimensional data based on robust technique: Application to different health database, 2024, 10, 24058440, e37241, 10.1016/j.heliyon.2024.e37241 | |
7. | Salvatore Mancha Gonzales, Hasnain Iftikhar, Javier Linkolk López-Gonzales, Analysis and forecasting of electricity prices using an improved time series ensemble approach: an application to the Peruvian electricity market, 2024, 9, 2473-6988, 21952, 10.3934/math.20241067 | |
8. | Moiz Qureshi, Hasnain Iftikhar, Paulo Canas Rodrigues, Mohd Ziaur Rehman, S. A. Atif Salar, Statistical Modeling to Improve Time Series Forecasting Using Machine Learning, Time Series, and Hybrid Models: A Case Study of Bitcoin Price Forecasting, 2024, 12, 2227-7390, 3666, 10.3390/math12233666 | |
9. | Hasnain Iftikhar, Faridoon Khan, Elías A. Torres Armas, Paulo Canas Rodrigues, Javier Linkolk López-Gonzales, A novel hybrid framework for forecasting stock indices based on the nonlinear time series models, 2025, 0943-4062, 10.1007/s00180-025-01614-5 |
S.No | Error | Formula |
1 | RMSPE | √1D∑Dd=1(|pd−^pd||pd|)2×100 |
2 | RMSE | √1D∑Dd=1(pd−ˆpd)2 |
3 | MAE | 1D∑Dd=1(|pd−^pd|) |
4 | MAPE | 1D∑Dd=1(|pd−^pd||pd|)×100 |
5 | CC | Corr(pd,ˆpd) |
Data | Scenario | Number of observation | Date |
Training | 70% | 1269 | 2 January 2013 to 21 December 2017 |
Testing | 30% | 1269 | 22 December 2017 to 17 February 2023 |
Training | 80% | 1904 | 2 January 2013 to 25 June 2020 |
Testing | 20% | 635 | 26 June 2020 to 17 February 2023 |
Training | 90% | 2285 | 2 January 2013 to 22 December 2021 |
Testing | 10% | 254 | 23 December 2021 to 17 February 2023 |
Nonlinearity outcomes | Nonstationarity outcomes | ||||
Series | Statistic Value | P-Value | At level | At first difference | Conclusion |
Closing stock prices(pd) | 97.2750 | 0.0100 | -1.3240 | -9.8100 | I (1) |
long-run trend (ℓd) | 4.4045 | 0.1106 | -2.4324 | -10.6700 | I (1) |
Stochastic (cd) | 51.2380 | 0.0100 | -32.8480 | - |
The first scenario 70%, 30% | |||||||
S.No | Models | RMSPE | MAPE | MAE | RMSE | CC | MDA |
1 | HPF11 | 156.2373 | 0.3778 | 0.0053 | 209.6080 | 0.9987 | 0.8966 |
2 | HPF12 | 156.8296 | 0.3789 | 0.0053 | 209.9340 | 0.9987 | 0.9047 |
3 | HPF13 | 127.5402 | 0.3081 | 0.0043 | 170.2864 | 0.9991 | 0.9195 |
4 | HPF14 | 161.2721 | 0.3901 | 0.0054 | 213.6699 | 0.9986 | 0.9141 |
5 | HPF21 | 148.8171 | 0.3602 | 0.0051 | 202.2458 | 0.9988 | 0.9168 |
6 | HPF22 | 149.9615 | 0.3626 | 0.0051 | 203.1089 | 0.9988 | 0.9128 |
7 | HPF23 | 120.8022 | 0.2924 | 0.0041 | 161.9550 | 0.9992 | 0.9302 |
8 | HPF24 | 168.5671 | 0.4076 | 0.0057 | 225.6628 | 0.9985 | 0.8993 |
9 | HPF31 | 156.5730 | 0.3786 | 0.0053 | 209.6822 | 0.9987 | 0.8966 |
10 | HPF32 | 157.1322 | 0.3796 | 0.0053 | 210.0071 | 0.9987 | 0.8980 |
11 | HPF33 | 127.3876 | 0.3077 | 0.0043 | 170.2426 | 0.9991 | 0.9195 |
12 | HPF34 | 162.1289 | 0.3923 | 0.0054 | 214.3426 | 0.9986 | 0.9128 |
13 | HPF41 | 241.5573 | 0.5869 | 0.0105 | 425.4353 | 0.9946 | 0.8523 |
14 | HPF42 | 242.3449 | 0.5885 | 0.0105 | 426.2372 | 0.9946 | 0.8497 |
15 | HPF43 | 187.4053 | 0.4543 | 0.0090 | 369.9058 | 0.9960 | 0.9020 |
16 | HPF44 | 261.4660 | 0.6346 | 0.0109 | 442.4052 | 0.9942 | 0.8309 |
The second scenario 80%, 20% | |||||||
1 | HPF11 | 151.5090 | 0.3437 | 0.0045 | 199.2405 | 0.9964 | 0.8788 |
2 | HPF12 | 151.6872 | 0.3439 | 0.0045 | 199.5213 | 0.9964 | 0.8828 |
3 | HPF13 | 122.9512 | 0.2792 | 0.0037 | 160.5389 | 0.9977 | 0.9091 |
4 | HPF14 | 154.9424 | 0.3515 | 0.0046 | 201.5488 | 0.9963 | 0.8909 |
5 | HPF21 | 141.9220 | 0.3219 | 0.0043 | 190.3518 | 0.9967 | 0.8929 |
6 | HPF22 | 143.0074 | 0.3242 | 0.0044 | 191.4878 | 0.9967 | 0.8909 |
7 | HPF23 | 115.5138 | 0.2622 | 0.0034 | 149.8806 | 0.9980 | 0.9253 |
8 | HPF24 | 159.7229 | 0.3621 | 0.0048 | 210.7949 | 0.9960 | 0.8848 |
9 | HPF31 | 151.9299 | 0.3447 | 0.0045 | 199.3006 | 0.9964 | 0.8747 |
10 | HPF32 | 152.0373 | 0.3448 | 0.0045 | 199.5532 | 0.9964 | 0.8788 |
11 | HPF33 | 122.9880 | 0.2793 | 0.0037 | 160.4473 | 0.9977 | 0.9071 |
12 | HPF34 | 155.6473 | 0.3531 | 0.0046 | 202.1268 | 0.9963 | 0.8929 |
13 | HPF41 | 232.6237 | 0.5272 | 0.0094 | 415.7154 | 0.9843 | 0.8364 |
14 | HPF42 | 232.8815 | 0.5278 | 0.0094 | 416.4237 | 0.9843 | 0.8323 |
15 | HPF43 | 178.2604 | 0.4032 | 0.0082 | 366.0674 | 0.9879 | 0.8929 |
16 | HPF44 | 252.2533 | 0.5718 | 0.0098 | 436.0666 | 0.9828 | 0.8242 |
The third scenario 90%, 10% | |||||||
1 | HPF11 | 156.0030 | 0.3672 | 0.0047 | 199.1678 | 0.9927 | 0.8776 |
2 | HPF12 | 155.1474 | 0.3651 | 0.0047 | 198.6827 | 0.9927 | 0.8816 |
3 | HPF13 | 130.0234 | 0.3060 | 0.0038 | 162.9916 | 0.9951 | 0.9143 |
4 | HPF14 | 158.6718 | 0.3734 | 0.0048 | 202.2724 | 0.9925 | 0.8898 |
5 | HPF21 | 144.9313 | 0.3412 | 0.0045 | 189.5869 | 0.9934 | 0.8980 |
6 | HPF22 | 145.2673 | 0.3419 | 0.0045 | 190.0539 | 0.9933 | 0.8939 |
7 | HPF23 | 121.8376 | 0.2866 | 0.0036 | 151.6476 | 0.9958 | 0.9429 |
8 | HPF24 | 161.9019 | 0.3813 | 0.0050 | 212.5678 | 0.9917 | 0.8776 |
9 | HPF31 | 156.4563 | 0.3683 | 0.0047 | 199.1440 | 0.9927 | 0.8735 |
10 | HPF32 | 155.4268 | 0.3658 | 0.0047 | 198.6606 | 0.9927 | 0.8735 |
11 | HPF33 | 130.1624 | 0.3064 | 0.0038 | 163.0377 | 0.9951 | 0.9143 |
12 | HPF34 | 159.5017 | 0.3754 | 0.0048 | 202.7243 | 0.9924 | 0.8857 |
13 | HPF41 | 251.2031 | 0.5910 | 0.0123 | 528.4650 | 0.9491 | 0.8204 |
14 | HPF42 | 251.0871 | 0.5907 | 0.0123 | 528.8418 | 0.9490 | 0.8245 |
15 | HPF43 | 194.0790 | 0.4555 | 0.0113 | 488.9315 | 0.9567 | 0.8776 |
16 | HPF44 | 271.9590 | 0.6396 | 0.0125 | 539.1469 | 0.9470 | 0.8449 |
The first scenario 70%, 30% | ||||||||||||||||
Models | HPF11 | HPF12 | HPF13 | HPF14 | HPF21 | HPF22 | HPF23 | HPF24 | HPF31 | HPF32 | HPF33 | HPF43 | HPF41 | HPF42 | HPF43 | HPF44 |
HPF11 | 0.000 | 0.700 | 0.000 | 0.872 | 0.023 | 0.042 | 0.000 | 0.993 | 0.571 | 0.701 | 0.000 | 0.904 | 0.992 | 0.992 | 0.950 | 0.996 |
HPF12 | 0.300 | 0.000 | 0.000 | 0.852 | 0.019 | 0.031 | 0.000 | 0.992 | 0.366 | 0.570 | 0.000 | 0.888 | 0.992 | 0.992 | 0.950 | 0.996 |
HPF13 | 1.000 | 1.000 | 0.000 | 1.000 | 1.000 | 1.000 | 0.013 | 1.000 | 1.000 | 1.000 | 0.456 | 1.000 | 0.996 | 0.996 | 0.972 | 0.998 |
HPF14 | 0.128 | 0.148 | 0.000 | 0.000 | 0.000 | 0.000 | 0.000 | 1.000 | 0.131 | 0.152 | 0.000 | 0.949 | 0.991 | 0.991 | 0.947 | 0.996 |
HPF21 | 0.977 | 0.981 | 0.000 | 1.000 | 0.000 | 0.893 | 0.000 | 1.000 | 0.979 | 0.983 | 0.000 | 1.000 | 0.993 | 0.993 | 0.956 | 0.997 |
HPF22 | 0.959 | 0.969 | 0.000 | 1.000 | 0.107 | 0.000 | 0.000 | 1.000 | 0.962 | 0.971 | 0.000 | 1.000 | 0.993 | 0.993 | 0.955 | 0.997 |
HPF23 | 1.000 | 1.000 | 0.987 | 1.000 | 1.000 | 1.000 | 0.000 | 1.000 | 1.000 | 1.000 | 0.987 | 1.000 | 0.997 | 0.997 | 0.975 | 0.999 |
HPF24 | 0.007 | 0.008 | 0.000 | 0.000 | 0.000 | 0.000 | 0.000 | 0.000 | 0.007 | 0.008 | 0.000 | 0.001 | 0.989 | 0.989 | 0.936 | 0.995 |
HPF31 | 0.429 | 0.634 | 0.000 | 0.869 | 0.021 | 0.039 | 0.000 | 0.993 | 0.000 | 0.698 | 0.000 | 0.904 | 0.992 | 0.992 | 0.950 | 0.996 |
HPF32 | 0.299 | 0.430 | 0.000 | 0.849 | 0.017 | 0.029 | 0.000 | 0.992 | 0.302 | 0.000 | 0.000 | 0.888 | 0.992 | 0.992 | 0.950 | 0.996 |
HPF33 | 1.000 | 1.000 | 0.544 | 1.000 | 1.000 | 1.000 | 0.013 | 1.000 | 1.000 | 1.000 | 0.000 | 1.000 | 0.996 | 0.996 | 0.972 | 0.998 |
HPF34 | 0.096 | 0.112 | 0.000 | 0.051 | 0.000 | 0.000 | 0.000 | 0.999 | 0.096 | 0.112 | 0.000 | 0.000 | 0.991 | 0.991 | 0.946 | 0.996 |
HPF41 | 0.008 | 0.008 | 0.004 | 0.009 | 0.007 | 0.007 | 0.004 | 0.011 | 0.008 | 0.008 | 0.004 | 0.009 | 0.000 | 0.937 | 0.000 | 1.000 |
HPF42 | 0.008 | 0.008 | 0.004 | 0.009 | 0.007 | 0.007 | 0.003 | 0.011 | 0.008 | 0.008 | 0.004 | 0.009 | 0.063 | 0.000 | 0.000 | 1.000 |
HPF43 | 0.050 | 0.050 | 0.028 | 0.053 | 0.045 | 0.045 | 0.025 | 0.064 | 0.050 | 0.050 | 0.028 | 0.054 | 1.000 | 1.000 | 0.000 | 1.000 |
HPF44 | 0.004 | 0.004 | 0.002 | 0.004 | 0.003 | 0.003 | 0.002 | 0.006 | 0.004 | 0.004 | 0.002 | 0.004 | 0.000 | 0.000 | 0.000 | 0.000 |
The second scenario 80%, 20% | ||||||||||||||||
HPF11 | 0.000 | 0.665 | 0.000 | 0.740 | 0.012 | 0.029 | 0.000 | 0.959 | 0.562 | 0.655 | 0.000 | 0.781 | 0.950 | 0.950 | 0.881 | 0.961 |
HPF12 | 0.335 | 0.000 | 0.000 | 0.717 | 0.009 | 0.020 | 0.000 | 0.957 | 0.384 | 0.533 | 0.000 | 0.762 | 0.950 | 0.950 | 0.880 | 0.960 |
HPF13 | 1.000 | 1.000 | 0.000 | 1.000 | 1.000 | 1.000 | 0.002 | 1.000 | 1.000 | 1.000 | 0.406 | 1.000 | 0.965 | 0.965 | 0.912 | 0.972 |
HPF14 | 0.260 | 0.283 | 0.000 | 0.000 | 0.000 | 0.000 | 0.000 | 0.992 | 0.260 | 0.280 | 0.000 | 0.939 | 0.949 | 0.949 | 0.879 | 0.960 |
HPF21 | 0.988 | 0.991 | 0.000 | 1.000 | 0.000 | 0.943 | 0.000 | 1.000 | 0.990 | 0.992 | 0.000 | 1.000 | 0.954 | 0.955 | 0.889 | 0.964 |
HPF22 | 0.972 | 0.980 | 0.000 | 1.000 | 0.057 | 0.000 | 0.000 | 1.000 | 0.975 | 0.982 | 0.000 | 1.000 | 0.954 | 0.954 | 0.888 | 0.964 |
HPF23 | 1.000 | 1.000 | 0.998 | 1.000 | 1.000 | 1.000 | 0.000 | 1.000 | 1.000 | 1.000 | 0.998 | 1.000 | 0.968 | 0.969 | 0.918 | 0.975 |
HPF24 | 0.041 | 0.043 | 0.000 | 0.008 | 0.000 | 0.000 | 0.000 | 0.000 | 0.040 | 0.041 | 0.000 | 0.011 | 0.944 | 0.945 | 0.869 | 0.956 |
HPF31 | 0.438 | 0.616 | 0.000 | 0.740 | 0.010 | 0.025 | 0.000 | 0.960 | 0.000 | 0.649 | 0.000 | 0.785 | 0.950 | 0.950 | 0.881 | 0.961 |
HPF32 | 0.345 | 0.467 | 0.000 | 0.720 | 0.008 | 0.018 | 0.000 | 0.959 | 0.351 | 0.000 | 0.000 | 0.767 | 0.950 | 0.950 | 0.880 | 0.960 |
HPF33 | 1.000 | 1.000 | 0.594 | 1.000 | 1.000 | 1.000 | 0.002 | 1.000 | 1.000 | 1.000 | 0.000 | 1.000 | 0.965 | 0.966 | 0.912 | 0.972 |
HPF34 | 0.219 | 0.238 | 0.000 | 0.062 | 0.000 | 0.000 | 0.000 | 0.989 | 0.215 | 0.233 | 0.000 | 0.000 | 0.949 | 0.949 | 0.878 | 0.960 |
HPF41 | 0.050 | 0.050 | 0.035 | 0.051 | 0.046 | 0.046 | 0.032 | 0.056 | 0.050 | 0.050 | 0.035 | 0.051 | 0.000 | 0.900 | 0.000 | 1.000 |
HPF42 | 0.050 | 0.050 | 0.035 | 0.051 | 0.045 | 0.046 | 0.032 | 0.055 | 0.050 | 0.050 | 0.035 | 0.051 | 0.100 | 0.000 | 0.000 | 1.000 |
HPF43 | 0.120 | 0.120 | 0.088 | 0.122 | 0.111 | 0.112 | 0.082 | 0.131 | 0.120 | 0.120 | 0.088 | 0.122 | 1.000 | 1.000 | 0.000 | 1.000 |
HPF44 | 0.040 | 0.040 | 0.028 | 0.040 | 0.036 | 0.036 | 0.025 | 0.044 | 0.040 | 0.040 | 0.028 | 0.040 | 0.001 | 0.001 | 0.000 | 0.000 |
The third scenario 90%, 10% | ||||||||||||||||
HPF11 | 0.000 | 0.244 | 0.000 | 0.724 | 0.035 | 0.044 | 0.000 | 0.923 | 0.481 | 0.279 | 0.000 | 0.749 | 0.927 | 0.927 | 0.886 | 0.940 |
HPF12 | 0.756 | 0.000 | 0.000 | 0.754 | 0.043 | 0.050 | 0.000 | 0.930 | 0.709 | 0.482 | 0.000 | 0.777 | 0.927 | 0.927 | 0.886 | 0.940 |
HPF13 | 1.000 | 1.000 | 0.000 | 1.000 | 0.994 | 0.995 | 0.010 | 1.000 | 1.000 | 1.000 | 0.536 | 1.000 | 0.937 | 0.937 | 0.901 | 0.949 |
HPF14 | 0.277 | 0.246 | 0.000 | 0.000 | 0.000 | 0.001 | 0.000 | 0.976 | 0.275 | 0.245 | 0.000 | 0.813 | 0.926 | 0.925 | 0.884 | 0.939 |
HPF21 | 0.965 | 0.957 | 0.006 | 1.000 | 0.000 | 0.731 | 0.000 | 1.000 | 0.965 | 0.958 | 0.006 | 1.000 | 0.930 | 0.930 | 0.890 | 0.942 |
HPF22 | 0.956 | 0.950 | 0.005 | 0.999 | 0.269 | 0.000 | 0.000 | 1.000 | 0.956 | 0.951 | 0.005 | 1.000 | 0.930 | 0.929 | 0.890 | 0.942 |
HPF23 | 1.000 | 1.000 | 0.990 | 1.000 | 1.000 | 1.000 | 0.000 | 1.000 | 1.000 | 1.000 | 0.991 | 1.000 | 0.940 | 0.939 | 0.904 | 0.951 |
HPF24 | 0.077 | 0.070 | 0.000 | 0.024 | 0.000 | 0.000 | 0.000 | 0.000 | 0.076 | 0.069 | 0.000 | 0.029 | 0.922 | 0.922 | 0.879 | 0.935 |
HPF31 | 0.520 | 0.291 | 0.000 | 0.725 | 0.035 | 0.044 | 0.000 | 0.924 | 0.000 | 0.245 | 0.000 | 0.752 | 0.927 | 0.927 | 0.886 | 0.940 |
HPF32 | 0.721 | 0.518 | 0.000 | 0.755 | 0.043 | 0.049 | 0.000 | 0.931 | 0.755 | 0.000 | 0.000 | 0.781 | 0.927 | 0.927 | 0.886 | 0.940 |
HPF33 | 1.000 | 1.000 | 0.464 | 1.000 | 0.994 | 0.995 | 0.009 | 1.000 | 1.000 | 1.000 | 0.000 | 1.000 | 0.937 | 0.937 | 0.901 | 0.949 |
HPF34 | 0.251 | 0.223 | 0.000 | 0.187 | 0.000 | 0.001 | 0.000 | 0.971 | 0.248 | 0.219 | 0.000 | 0.000 | 0.925 | 0.925 | 0.884 | 0.939 |
HPF41 | 0.073 | 0.073 | 0.063 | 0.075 | 0.070 | 0.071 | 0.061 | 0.078 | 0.073 | 0.073 | 0.063 | 0.075 | 0.000 | 0.742 | 0.000 | 0.989 |
HPF42 | 0.074 | 0.073 | 0.063 | 0.075 | 0.071 | 0.071 | 0.061 | 0.078 | 0.074 | 0.073 | 0.063 | 0.075 | 0.258 | 0.000 | 0.000 | 0.981 |
HPF43 | 0.114 | 0.114 | 0.099 | 0.116 | 0.110 | 0.110 | 0.096 | 0.121 | 0.114 | 0.114 | 0.099 | 0.116 | 1.000 | 1.000 | 0.000 | 1.000 |
HPF44 | 0.060 | 0.060 | 0.051 | 0.061 | 0.058 | 0.058 | 0.049 | 0.065 | 0.060 | 0.060 | 0.051 | 0.062 | 0.012 | 0.019 | 0.000 | 0.000 |
The first scenario 70%, 30% | |||||||
S.No | Models | RMSPE | MAPE | MAE | RMSE | CC | MDA |
1 | HPF23 | 120.8022 | 0.2924 | 0.0041 | 161.9550 | 0.9992 | 0.9302 |
2 | AR | 156.5730 | 0.3786 | 0.0053 | 209.6822 | 0.9987 | 0.8966 |
3 | ARIMA | 157.1322 | 0.3796 | 0.0053 | 210.0071 | 0.9987 | 0.8980 |
4 | NPAR | 127.3876 | 0.3077 | 0.0043 | 170.2426 | 0.9991 | 0.9195 |
5 | ANN | 162.1289 | 0.3923 | 0.0054 | 214.3426 | 0.9986 | 0.9128 |
The second scenario 80%, 20% | |||||||
1 | HPF23 | 115.5138 | 0.2622 | 0.0034 | 149.8806 | 0.9980 | 0.9253 |
2 | AR | 151.9299 | 0.3447 | 0.0045 | 199.3006 | 0.9964 | 0.8747 |
3 | ARIMA | 152.0373 | 0.3448 | 0.0045 | 199.5532 | 0.9964 | 0.8788 |
4 | NPAR | 122.9880 | 0.2793 | 0.0037 | 160.4473 | 0.9977 | 0.9071 |
5 | ANN | 155.6473 | 0.3531 | 0.0046 | 202.1268 | 0.9963 | 0.8929 |
The third scenario 90%, 10% | |||||||
1 | HPF23 | 121.8376 | 0.2866 | 0.0036 | 151.6476 | 0.9958 | 0.9429 |
2 | AR | 156.4563 | 0.3683 | 0.0047 | 199.1440 | 0.9927 | 0.8735 |
3 | ARIMA | 155.4268 | 0.3658 | 0.0047 | 198.6606 | 0.9927 | 0.8735 |
4 | NPAR | 130.1624 | 0.3064 | 0.0038 | 163.0377 | 0.9951 | 0.9143 |
5 | ANN | 159.5017 | 0.3754 | 0.0048 | 202.7243 | 0.9924 | 0.8857 |
The first scenario 70%, 30% | |||||
models | HPF23 | AR | ARIMA | NPAR | ANN |
HPF23 | 0.000 | 1.000 | 1.000 | 0.987 | 1.000 |
AR | 0.000 | 0.000 | 0.698 | 0.000 | 0.904 |
ARIMA | 0.000 | 0.302 | 0.000 | 0.000 | 0.888 |
NPAR | 0.013 | 1.000 | 1.000 | 0.000 | 1.000 |
ANN | 0.000 | 0.096 | 0.112 | 0.000 | 0.000 |
The second scenario 80%, 20% | |||||
HPF23 | 0.000 | 1.000 | 1.000 | 0.998 | 1.000 |
AR | 0.000 | 0.000 | 0.649 | 0.000 | 0.785 |
ARIMA | 0.000 | 0.351 | 0.000 | 0.000 | 0.767 |
NPAR | 0.002 | 1.000 | 1.000 | 0.000 | 1.000 |
ANN | 0.000 | 0.215 | 0.233 | 0.000 | 0.000 |
The third scenario 90%, 10% | |||||
HPF23 | 0.000 | 1.000 | 1.000 | 0.991 | 1.000 |
AR | 0.000 | 0.000 | 0.245 | 0.000 | 0.752 |
ARIMA | 0.000 | 0.755 | 0.000 | 0.000 | 0.781 |
NPAR | 0.009 | 1.000 | 1.000 | 0.000 | 1.000 |
ANN | 0.000 | 0.248 | 0.219 | 0.000 | 0.000 |
S.No | Error | Formula |
1 | RMSPE | √1D∑Dd=1(|pd−^pd||pd|)2×100 |
2 | RMSE | √1D∑Dd=1(pd−ˆpd)2 |
3 | MAE | 1D∑Dd=1(|pd−^pd|) |
4 | MAPE | 1D∑Dd=1(|pd−^pd||pd|)×100 |
5 | CC | Corr(pd,ˆpd) |
Data | Scenario | Number of observation | Date |
Training | 70% | 1269 | 2 January 2013 to 21 December 2017 |
Testing | 30% | 1269 | 22 December 2017 to 17 February 2023 |
Training | 80% | 1904 | 2 January 2013 to 25 June 2020 |
Testing | 20% | 635 | 26 June 2020 to 17 February 2023 |
Training | 90% | 2285 | 2 January 2013 to 22 December 2021 |
Testing | 10% | 254 | 23 December 2021 to 17 February 2023 |
Nonlinearity outcomes | Nonstationarity outcomes | ||||
Series | Statistic Value | P-Value | At level | At first difference | Conclusion |
Closing stock prices(pd) | 97.2750 | 0.0100 | -1.3240 | -9.8100 | I (1) |
long-run trend (ℓd) | 4.4045 | 0.1106 | -2.4324 | -10.6700 | I (1) |
Stochastic (cd) | 51.2380 | 0.0100 | -32.8480 | - |
The first scenario 70%, 30% | |||||||
S.No | Models | RMSPE | MAPE | MAE | RMSE | CC | MDA |
1 | HPF11 | 156.2373 | 0.3778 | 0.0053 | 209.6080 | 0.9987 | 0.8966 |
2 | HPF12 | 156.8296 | 0.3789 | 0.0053 | 209.9340 | 0.9987 | 0.9047 |
3 | HPF13 | 127.5402 | 0.3081 | 0.0043 | 170.2864 | 0.9991 | 0.9195 |
4 | HPF14 | 161.2721 | 0.3901 | 0.0054 | 213.6699 | 0.9986 | 0.9141 |
5 | HPF21 | 148.8171 | 0.3602 | 0.0051 | 202.2458 | 0.9988 | 0.9168 |
6 | HPF22 | 149.9615 | 0.3626 | 0.0051 | 203.1089 | 0.9988 | 0.9128 |
7 | HPF23 | 120.8022 | 0.2924 | 0.0041 | 161.9550 | 0.9992 | 0.9302 |
8 | HPF24 | 168.5671 | 0.4076 | 0.0057 | 225.6628 | 0.9985 | 0.8993 |
9 | HPF31 | 156.5730 | 0.3786 | 0.0053 | 209.6822 | 0.9987 | 0.8966 |
10 | HPF32 | 157.1322 | 0.3796 | 0.0053 | 210.0071 | 0.9987 | 0.8980 |
11 | HPF33 | 127.3876 | 0.3077 | 0.0043 | 170.2426 | 0.9991 | 0.9195 |
12 | HPF34 | 162.1289 | 0.3923 | 0.0054 | 214.3426 | 0.9986 | 0.9128 |
13 | HPF41 | 241.5573 | 0.5869 | 0.0105 | 425.4353 | 0.9946 | 0.8523 |
14 | HPF42 | 242.3449 | 0.5885 | 0.0105 | 426.2372 | 0.9946 | 0.8497 |
15 | HPF43 | 187.4053 | 0.4543 | 0.0090 | 369.9058 | 0.9960 | 0.9020 |
16 | HPF44 | 261.4660 | 0.6346 | 0.0109 | 442.4052 | 0.9942 | 0.8309 |
The second scenario 80%, 20% | |||||||
1 | HPF11 | 151.5090 | 0.3437 | 0.0045 | 199.2405 | 0.9964 | 0.8788 |
2 | HPF12 | 151.6872 | 0.3439 | 0.0045 | 199.5213 | 0.9964 | 0.8828 |
3 | HPF13 | 122.9512 | 0.2792 | 0.0037 | 160.5389 | 0.9977 | 0.9091 |
4 | HPF14 | 154.9424 | 0.3515 | 0.0046 | 201.5488 | 0.9963 | 0.8909 |
5 | HPF21 | 141.9220 | 0.3219 | 0.0043 | 190.3518 | 0.9967 | 0.8929 |
6 | HPF22 | 143.0074 | 0.3242 | 0.0044 | 191.4878 | 0.9967 | 0.8909 |
7 | HPF23 | 115.5138 | 0.2622 | 0.0034 | 149.8806 | 0.9980 | 0.9253 |
8 | HPF24 | 159.7229 | 0.3621 | 0.0048 | 210.7949 | 0.9960 | 0.8848 |
9 | HPF31 | 151.9299 | 0.3447 | 0.0045 | 199.3006 | 0.9964 | 0.8747 |
10 | HPF32 | 152.0373 | 0.3448 | 0.0045 | 199.5532 | 0.9964 | 0.8788 |
11 | HPF33 | 122.9880 | 0.2793 | 0.0037 | 160.4473 | 0.9977 | 0.9071 |
12 | HPF34 | 155.6473 | 0.3531 | 0.0046 | 202.1268 | 0.9963 | 0.8929 |
13 | HPF41 | 232.6237 | 0.5272 | 0.0094 | 415.7154 | 0.9843 | 0.8364 |
14 | HPF42 | 232.8815 | 0.5278 | 0.0094 | 416.4237 | 0.9843 | 0.8323 |
15 | HPF43 | 178.2604 | 0.4032 | 0.0082 | 366.0674 | 0.9879 | 0.8929 |
16 | HPF44 | 252.2533 | 0.5718 | 0.0098 | 436.0666 | 0.9828 | 0.8242 |
The third scenario 90%, 10% | |||||||
1 | HPF11 | 156.0030 | 0.3672 | 0.0047 | 199.1678 | 0.9927 | 0.8776 |
2 | HPF12 | 155.1474 | 0.3651 | 0.0047 | 198.6827 | 0.9927 | 0.8816 |
3 | HPF13 | 130.0234 | 0.3060 | 0.0038 | 162.9916 | 0.9951 | 0.9143 |
4 | HPF14 | 158.6718 | 0.3734 | 0.0048 | 202.2724 | 0.9925 | 0.8898 |
5 | HPF21 | 144.9313 | 0.3412 | 0.0045 | 189.5869 | 0.9934 | 0.8980 |
6 | HPF22 | 145.2673 | 0.3419 | 0.0045 | 190.0539 | 0.9933 | 0.8939 |
7 | HPF23 | 121.8376 | 0.2866 | 0.0036 | 151.6476 | 0.9958 | 0.9429 |
8 | HPF24 | 161.9019 | 0.3813 | 0.0050 | 212.5678 | 0.9917 | 0.8776 |
9 | HPF31 | 156.4563 | 0.3683 | 0.0047 | 199.1440 | 0.9927 | 0.8735 |
10 | HPF32 | 155.4268 | 0.3658 | 0.0047 | 198.6606 | 0.9927 | 0.8735 |
11 | HPF33 | 130.1624 | 0.3064 | 0.0038 | 163.0377 | 0.9951 | 0.9143 |
12 | HPF34 | 159.5017 | 0.3754 | 0.0048 | 202.7243 | 0.9924 | 0.8857 |
13 | HPF41 | 251.2031 | 0.5910 | 0.0123 | 528.4650 | 0.9491 | 0.8204 |
14 | HPF42 | 251.0871 | 0.5907 | 0.0123 | 528.8418 | 0.9490 | 0.8245 |
15 | HPF43 | 194.0790 | 0.4555 | 0.0113 | 488.9315 | 0.9567 | 0.8776 |
16 | HPF44 | 271.9590 | 0.6396 | 0.0125 | 539.1469 | 0.9470 | 0.8449 |
The first scenario 70%, 30% | ||||||||||||||||
Models | HPF11 | HPF12 | HPF13 | HPF14 | HPF21 | HPF22 | HPF23 | HPF24 | HPF31 | HPF32 | HPF33 | HPF43 | HPF41 | HPF42 | HPF43 | HPF44 |
HPF11 | 0.000 | 0.700 | 0.000 | 0.872 | 0.023 | 0.042 | 0.000 | 0.993 | 0.571 | 0.701 | 0.000 | 0.904 | 0.992 | 0.992 | 0.950 | 0.996 |
HPF12 | 0.300 | 0.000 | 0.000 | 0.852 | 0.019 | 0.031 | 0.000 | 0.992 | 0.366 | 0.570 | 0.000 | 0.888 | 0.992 | 0.992 | 0.950 | 0.996 |
HPF13 | 1.000 | 1.000 | 0.000 | 1.000 | 1.000 | 1.000 | 0.013 | 1.000 | 1.000 | 1.000 | 0.456 | 1.000 | 0.996 | 0.996 | 0.972 | 0.998 |
HPF14 | 0.128 | 0.148 | 0.000 | 0.000 | 0.000 | 0.000 | 0.000 | 1.000 | 0.131 | 0.152 | 0.000 | 0.949 | 0.991 | 0.991 | 0.947 | 0.996 |
HPF21 | 0.977 | 0.981 | 0.000 | 1.000 | 0.000 | 0.893 | 0.000 | 1.000 | 0.979 | 0.983 | 0.000 | 1.000 | 0.993 | 0.993 | 0.956 | 0.997 |
HPF22 | 0.959 | 0.969 | 0.000 | 1.000 | 0.107 | 0.000 | 0.000 | 1.000 | 0.962 | 0.971 | 0.000 | 1.000 | 0.993 | 0.993 | 0.955 | 0.997 |
HPF23 | 1.000 | 1.000 | 0.987 | 1.000 | 1.000 | 1.000 | 0.000 | 1.000 | 1.000 | 1.000 | 0.987 | 1.000 | 0.997 | 0.997 | 0.975 | 0.999 |
HPF24 | 0.007 | 0.008 | 0.000 | 0.000 | 0.000 | 0.000 | 0.000 | 0.000 | 0.007 | 0.008 | 0.000 | 0.001 | 0.989 | 0.989 | 0.936 | 0.995 |
HPF31 | 0.429 | 0.634 | 0.000 | 0.869 | 0.021 | 0.039 | 0.000 | 0.993 | 0.000 | 0.698 | 0.000 | 0.904 | 0.992 | 0.992 | 0.950 | 0.996 |
HPF32 | 0.299 | 0.430 | 0.000 | 0.849 | 0.017 | 0.029 | 0.000 | 0.992 | 0.302 | 0.000 | 0.000 | 0.888 | 0.992 | 0.992 | 0.950 | 0.996 |
HPF33 | 1.000 | 1.000 | 0.544 | 1.000 | 1.000 | 1.000 | 0.013 | 1.000 | 1.000 | 1.000 | 0.000 | 1.000 | 0.996 | 0.996 | 0.972 | 0.998 |
HPF34 | 0.096 | 0.112 | 0.000 | 0.051 | 0.000 | 0.000 | 0.000 | 0.999 | 0.096 | 0.112 | 0.000 | 0.000 | 0.991 | 0.991 | 0.946 | 0.996 |
HPF41 | 0.008 | 0.008 | 0.004 | 0.009 | 0.007 | 0.007 | 0.004 | 0.011 | 0.008 | 0.008 | 0.004 | 0.009 | 0.000 | 0.937 | 0.000 | 1.000 |
HPF42 | 0.008 | 0.008 | 0.004 | 0.009 | 0.007 | 0.007 | 0.003 | 0.011 | 0.008 | 0.008 | 0.004 | 0.009 | 0.063 | 0.000 | 0.000 | 1.000 |
HPF43 | 0.050 | 0.050 | 0.028 | 0.053 | 0.045 | 0.045 | 0.025 | 0.064 | 0.050 | 0.050 | 0.028 | 0.054 | 1.000 | 1.000 | 0.000 | 1.000 |
HPF44 | 0.004 | 0.004 | 0.002 | 0.004 | 0.003 | 0.003 | 0.002 | 0.006 | 0.004 | 0.004 | 0.002 | 0.004 | 0.000 | 0.000 | 0.000 | 0.000 |
The second scenario 80%, 20% | ||||||||||||||||
HPF11 | 0.000 | 0.665 | 0.000 | 0.740 | 0.012 | 0.029 | 0.000 | 0.959 | 0.562 | 0.655 | 0.000 | 0.781 | 0.950 | 0.950 | 0.881 | 0.961 |
HPF12 | 0.335 | 0.000 | 0.000 | 0.717 | 0.009 | 0.020 | 0.000 | 0.957 | 0.384 | 0.533 | 0.000 | 0.762 | 0.950 | 0.950 | 0.880 | 0.960 |
HPF13 | 1.000 | 1.000 | 0.000 | 1.000 | 1.000 | 1.000 | 0.002 | 1.000 | 1.000 | 1.000 | 0.406 | 1.000 | 0.965 | 0.965 | 0.912 | 0.972 |
HPF14 | 0.260 | 0.283 | 0.000 | 0.000 | 0.000 | 0.000 | 0.000 | 0.992 | 0.260 | 0.280 | 0.000 | 0.939 | 0.949 | 0.949 | 0.879 | 0.960 |
HPF21 | 0.988 | 0.991 | 0.000 | 1.000 | 0.000 | 0.943 | 0.000 | 1.000 | 0.990 | 0.992 | 0.000 | 1.000 | 0.954 | 0.955 | 0.889 | 0.964 |
HPF22 | 0.972 | 0.980 | 0.000 | 1.000 | 0.057 | 0.000 | 0.000 | 1.000 | 0.975 | 0.982 | 0.000 | 1.000 | 0.954 | 0.954 | 0.888 | 0.964 |
HPF23 | 1.000 | 1.000 | 0.998 | 1.000 | 1.000 | 1.000 | 0.000 | 1.000 | 1.000 | 1.000 | 0.998 | 1.000 | 0.968 | 0.969 | 0.918 | 0.975 |
HPF24 | 0.041 | 0.043 | 0.000 | 0.008 | 0.000 | 0.000 | 0.000 | 0.000 | 0.040 | 0.041 | 0.000 | 0.011 | 0.944 | 0.945 | 0.869 | 0.956 |
HPF31 | 0.438 | 0.616 | 0.000 | 0.740 | 0.010 | 0.025 | 0.000 | 0.960 | 0.000 | 0.649 | 0.000 | 0.785 | 0.950 | 0.950 | 0.881 | 0.961 |
HPF32 | 0.345 | 0.467 | 0.000 | 0.720 | 0.008 | 0.018 | 0.000 | 0.959 | 0.351 | 0.000 | 0.000 | 0.767 | 0.950 | 0.950 | 0.880 | 0.960 |
HPF33 | 1.000 | 1.000 | 0.594 | 1.000 | 1.000 | 1.000 | 0.002 | 1.000 | 1.000 | 1.000 | 0.000 | 1.000 | 0.965 | 0.966 | 0.912 | 0.972 |
HPF34 | 0.219 | 0.238 | 0.000 | 0.062 | 0.000 | 0.000 | 0.000 | 0.989 | 0.215 | 0.233 | 0.000 | 0.000 | 0.949 | 0.949 | 0.878 | 0.960 |
HPF41 | 0.050 | 0.050 | 0.035 | 0.051 | 0.046 | 0.046 | 0.032 | 0.056 | 0.050 | 0.050 | 0.035 | 0.051 | 0.000 | 0.900 | 0.000 | 1.000 |
HPF42 | 0.050 | 0.050 | 0.035 | 0.051 | 0.045 | 0.046 | 0.032 | 0.055 | 0.050 | 0.050 | 0.035 | 0.051 | 0.100 | 0.000 | 0.000 | 1.000 |
HPF43 | 0.120 | 0.120 | 0.088 | 0.122 | 0.111 | 0.112 | 0.082 | 0.131 | 0.120 | 0.120 | 0.088 | 0.122 | 1.000 | 1.000 | 0.000 | 1.000 |
HPF44 | 0.040 | 0.040 | 0.028 | 0.040 | 0.036 | 0.036 | 0.025 | 0.044 | 0.040 | 0.040 | 0.028 | 0.040 | 0.001 | 0.001 | 0.000 | 0.000 |
The third scenario 90%, 10% | ||||||||||||||||
HPF11 | 0.000 | 0.244 | 0.000 | 0.724 | 0.035 | 0.044 | 0.000 | 0.923 | 0.481 | 0.279 | 0.000 | 0.749 | 0.927 | 0.927 | 0.886 | 0.940 |
HPF12 | 0.756 | 0.000 | 0.000 | 0.754 | 0.043 | 0.050 | 0.000 | 0.930 | 0.709 | 0.482 | 0.000 | 0.777 | 0.927 | 0.927 | 0.886 | 0.940 |
HPF13 | 1.000 | 1.000 | 0.000 | 1.000 | 0.994 | 0.995 | 0.010 | 1.000 | 1.000 | 1.000 | 0.536 | 1.000 | 0.937 | 0.937 | 0.901 | 0.949 |
HPF14 | 0.277 | 0.246 | 0.000 | 0.000 | 0.000 | 0.001 | 0.000 | 0.976 | 0.275 | 0.245 | 0.000 | 0.813 | 0.926 | 0.925 | 0.884 | 0.939 |
HPF21 | 0.965 | 0.957 | 0.006 | 1.000 | 0.000 | 0.731 | 0.000 | 1.000 | 0.965 | 0.958 | 0.006 | 1.000 | 0.930 | 0.930 | 0.890 | 0.942 |
HPF22 | 0.956 | 0.950 | 0.005 | 0.999 | 0.269 | 0.000 | 0.000 | 1.000 | 0.956 | 0.951 | 0.005 | 1.000 | 0.930 | 0.929 | 0.890 | 0.942 |
HPF23 | 1.000 | 1.000 | 0.990 | 1.000 | 1.000 | 1.000 | 0.000 | 1.000 | 1.000 | 1.000 | 0.991 | 1.000 | 0.940 | 0.939 | 0.904 | 0.951 |
HPF24 | 0.077 | 0.070 | 0.000 | 0.024 | 0.000 | 0.000 | 0.000 | 0.000 | 0.076 | 0.069 | 0.000 | 0.029 | 0.922 | 0.922 | 0.879 | 0.935 |
HPF31 | 0.520 | 0.291 | 0.000 | 0.725 | 0.035 | 0.044 | 0.000 | 0.924 | 0.000 | 0.245 | 0.000 | 0.752 | 0.927 | 0.927 | 0.886 | 0.940 |
HPF32 | 0.721 | 0.518 | 0.000 | 0.755 | 0.043 | 0.049 | 0.000 | 0.931 | 0.755 | 0.000 | 0.000 | 0.781 | 0.927 | 0.927 | 0.886 | 0.940 |
HPF33 | 1.000 | 1.000 | 0.464 | 1.000 | 0.994 | 0.995 | 0.009 | 1.000 | 1.000 | 1.000 | 0.000 | 1.000 | 0.937 | 0.937 | 0.901 | 0.949 |
HPF34 | 0.251 | 0.223 | 0.000 | 0.187 | 0.000 | 0.001 | 0.000 | 0.971 | 0.248 | 0.219 | 0.000 | 0.000 | 0.925 | 0.925 | 0.884 | 0.939 |
HPF41 | 0.073 | 0.073 | 0.063 | 0.075 | 0.070 | 0.071 | 0.061 | 0.078 | 0.073 | 0.073 | 0.063 | 0.075 | 0.000 | 0.742 | 0.000 | 0.989 |
HPF42 | 0.074 | 0.073 | 0.063 | 0.075 | 0.071 | 0.071 | 0.061 | 0.078 | 0.074 | 0.073 | 0.063 | 0.075 | 0.258 | 0.000 | 0.000 | 0.981 |
HPF43 | 0.114 | 0.114 | 0.099 | 0.116 | 0.110 | 0.110 | 0.096 | 0.121 | 0.114 | 0.114 | 0.099 | 0.116 | 1.000 | 1.000 | 0.000 | 1.000 |
HPF44 | 0.060 | 0.060 | 0.051 | 0.061 | 0.058 | 0.058 | 0.049 | 0.065 | 0.060 | 0.060 | 0.051 | 0.062 | 0.012 | 0.019 | 0.000 | 0.000 |
The first scenario 70%, 30% | |||||||
S.No | Models | RMSPE | MAPE | MAE | RMSE | CC | MDA |
1 | HPF23 | 120.8022 | 0.2924 | 0.0041 | 161.9550 | 0.9992 | 0.9302 |
2 | AR | 156.5730 | 0.3786 | 0.0053 | 209.6822 | 0.9987 | 0.8966 |
3 | ARIMA | 157.1322 | 0.3796 | 0.0053 | 210.0071 | 0.9987 | 0.8980 |
4 | NPAR | 127.3876 | 0.3077 | 0.0043 | 170.2426 | 0.9991 | 0.9195 |
5 | ANN | 162.1289 | 0.3923 | 0.0054 | 214.3426 | 0.9986 | 0.9128 |
The second scenario 80%, 20% | |||||||
1 | HPF23 | 115.5138 | 0.2622 | 0.0034 | 149.8806 | 0.9980 | 0.9253 |
2 | AR | 151.9299 | 0.3447 | 0.0045 | 199.3006 | 0.9964 | 0.8747 |
3 | ARIMA | 152.0373 | 0.3448 | 0.0045 | 199.5532 | 0.9964 | 0.8788 |
4 | NPAR | 122.9880 | 0.2793 | 0.0037 | 160.4473 | 0.9977 | 0.9071 |
5 | ANN | 155.6473 | 0.3531 | 0.0046 | 202.1268 | 0.9963 | 0.8929 |
The third scenario 90%, 10% | |||||||
1 | HPF23 | 121.8376 | 0.2866 | 0.0036 | 151.6476 | 0.9958 | 0.9429 |
2 | AR | 156.4563 | 0.3683 | 0.0047 | 199.1440 | 0.9927 | 0.8735 |
3 | ARIMA | 155.4268 | 0.3658 | 0.0047 | 198.6606 | 0.9927 | 0.8735 |
4 | NPAR | 130.1624 | 0.3064 | 0.0038 | 163.0377 | 0.9951 | 0.9143 |
5 | ANN | 159.5017 | 0.3754 | 0.0048 | 202.7243 | 0.9924 | 0.8857 |
The first scenario 70%, 30% | |||||
models | HPF23 | AR | ARIMA | NPAR | ANN |
HPF23 | 0.000 | 1.000 | 1.000 | 0.987 | 1.000 |
AR | 0.000 | 0.000 | 0.698 | 0.000 | 0.904 |
ARIMA | 0.000 | 0.302 | 0.000 | 0.000 | 0.888 |
NPAR | 0.013 | 1.000 | 1.000 | 0.000 | 1.000 |
ANN | 0.000 | 0.096 | 0.112 | 0.000 | 0.000 |
The second scenario 80%, 20% | |||||
HPF23 | 0.000 | 1.000 | 1.000 | 0.998 | 1.000 |
AR | 0.000 | 0.000 | 0.649 | 0.000 | 0.785 |
ARIMA | 0.000 | 0.351 | 0.000 | 0.000 | 0.767 |
NPAR | 0.002 | 1.000 | 1.000 | 0.000 | 1.000 |
ANN | 0.000 | 0.215 | 0.233 | 0.000 | 0.000 |
The third scenario 90%, 10% | |||||
HPF23 | 0.000 | 1.000 | 1.000 | 0.991 | 1.000 |
AR | 0.000 | 0.000 | 0.245 | 0.000 | 0.752 |
ARIMA | 0.000 | 0.755 | 0.000 | 0.000 | 0.781 |
NPAR | 0.009 | 1.000 | 1.000 | 0.000 | 1.000 |
ANN | 0.000 | 0.248 | 0.219 | 0.000 | 0.000 |