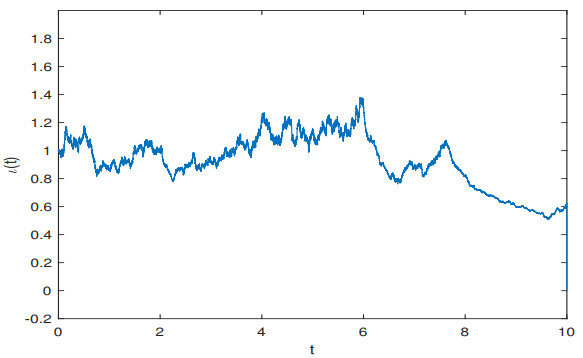
This paper aims to formulate a class of nonlinear hybrid stochastic time-delay neural networks (STDNNs) with Lévy noise. Specifically, the coefficients of networks grow polynomially instead of linearly, and the time delay of given neural networks is non-differentiable. In many practical situations, nonlinear hybrid STDNNs with Lévy noise are unstable. Hence, this paper uses feedback control based on discrete-time state and mode observations to stabilize the considered nonlinear hybrid STDNNs with Lévy noise. Then, we establish stabilization criteria of H∞ stability, asymptotic stability, and exponential stability for the controlled nonlinear hybrid STDNNs with Lévy noise. Finally, a numerical example illustrating the usefulness of theoretical results is provided.
Citation: Tian Xu, Ailong Wu. Stabilization of nonlinear hybrid stochastic time-delay neural networks with Lévy noise using discrete-time feedback control[J]. AIMS Mathematics, 2024, 9(10): 27080-27101. doi: 10.3934/math.20241317
[1] | Jingjing Yang, Jianqiu Lu . Stabilization in distribution of hybrid stochastic differential delay equations with Lévy noise by discrete-time state feedback controls. AIMS Mathematics, 2025, 10(2): 3457-3483. doi: 10.3934/math.2025160 |
[2] | Shuo Ma, Jiangman Li, Qiang Li, Ruonan Liu . Adaptive exponential synchronization of impulsive coupled neutral stochastic neural networks with Lévy noise and probabilistic delays under non-Lipschitz conditions. AIMS Mathematics, 2024, 9(9): 24912-24933. doi: 10.3934/math.20241214 |
[3] | Siru Li, Tian Xu, Ailong Wu . Existence and asymptotic properties of global solution for hybrid neutral stochastic differential delay equations with colored noise. AIMS Mathematics, 2025, 10(3): 6379-6405. doi: 10.3934/math.2025291 |
[4] | Yuanfu Shao . Dynamics and optimal harvesting of a stochastic predator-prey system with regime switching, S-type distributed time delays and Lévy jumps. AIMS Mathematics, 2022, 7(3): 4068-4093. doi: 10.3934/math.2022225 |
[5] | Yihang Kong, Xinghui Zhang, Yaxin Huang, Ancai Zhang, Jianlong Qiu . Prescribed-time adaptive stabilization of high-order stochastic nonlinear systems with unmodeled dynamics and time-varying powers. AIMS Mathematics, 2024, 9(10): 28447-28471. doi: 10.3934/math.20241380 |
[6] | Yijia Zhang, Tao Xie, Yunlong Ma . Robustness analysis of exponential stability of Cohen-Grossberg neural network with neutral terms. AIMS Mathematics, 2025, 10(3): 4938-4954. doi: 10.3934/math.2025226 |
[7] | Călin-Adrian Popa . Synchronization of Clifford-valued neural networks with leakage, time-varying, and infinite distributed delays on time scales. AIMS Mathematics, 2024, 9(7): 18796-18823. doi: 10.3934/math.2024915 |
[8] | Qinghua Zhou, Li Wan, Hongbo Fu, Qunjiao Zhang . Exponential stability of stochastic Hopfield neural network with mixed multiple delays. AIMS Mathematics, 2021, 6(4): 4142-4155. doi: 10.3934/math.2021245 |
[9] | Jenjira Thipcha, Presarin Tangsiridamrong, Thongchai Botmart, Boonyachat Meesuptong, M. Syed Ali, Pantiwa Srisilp, Kanit Mukdasai . Robust stability and passivity analysis for discrete-time neural networks with mixed time-varying delays via a new summation inequality. AIMS Mathematics, 2023, 8(2): 4973-5006. doi: 10.3934/math.2023249 |
[10] | Qingyi Cui, Changjin Xu, Wei Ou, Yicheng Pang, Zixin Liu, Jianwei Shen, Muhammad Farman, Shabir Ahmad . Further study on Hopf bifurcation and hybrid control strategy in BAM neural networks concerning time delay. AIMS Mathematics, 2024, 9(5): 13265-13290. doi: 10.3934/math.2024647 |
This paper aims to formulate a class of nonlinear hybrid stochastic time-delay neural networks (STDNNs) with Lévy noise. Specifically, the coefficients of networks grow polynomially instead of linearly, and the time delay of given neural networks is non-differentiable. In many practical situations, nonlinear hybrid STDNNs with Lévy noise are unstable. Hence, this paper uses feedback control based on discrete-time state and mode observations to stabilize the considered nonlinear hybrid STDNNs with Lévy noise. Then, we establish stabilization criteria of H∞ stability, asymptotic stability, and exponential stability for the controlled nonlinear hybrid STDNNs with Lévy noise. Finally, a numerical example illustrating the usefulness of theoretical results is provided.
Neural networks are successful tools for pattern recognition and machine learning, but traditional neural network models tend to ignore stochasticity, which may limit their performance and applicability. Compared to traditional deterministic neural networks, stochastic neural networks (SNNs) have greater robustness, generalization, and the ability to deal with uncertainty and noise, making them more suitable for use in areas such as financial forecasting, image processing, and natural language processing. During the evolution of many real neural networks, signal transmission between neurons inevitably generates time delay. The time delay has an important impact on the stability, oscillatory characteristics, and dynamic response of SNNs. Therefore, it is significant to analyze stochastic time-delay neural networks (STDNNs). There are numerous research results on STDNNs (see, e.g., [1,2,3,4,5]).
The classic enforcement condition is that the network coefficients satisfy the linear growth condition. However, in practice, such a restriction is too stringent for many STDNNs. Consequently, numerous scholars have shifted their focus to researching highly nonlinear STDNNs. Recently, the stability criteria for stochastic differential delay equations (SDDEs) driven by G-Brownian motion with highly nonlinear coefficients have been studied in [6], the finite-time stabilization criteria for highly nonlinear stochastic coupled systems of networks have been explored in [7], and a delayed feedback control function has been designed in [8] to stabilize nonlinear hybrid STDNNs with time-varying delays. Nevertheless, the above discriminant rules can only be applied in cases where the time delay of SNNs is constant or a differentiable function. In fact, these conditions may not be natural features of real-world SNNs. For instance, piecewise constant delays or sawtooth delays often appear in either sampled data control or network-based control (see, e.g., [9]), and yet these time delays are clearly not differentiable. Technically, it is crucial to ensure that the time delay is not limited to a constant or differentiable function to extend the applicability and expansiveness of dynamic evolution properties.
In many circumstances, neural networks are also subject to abrupt changes in parameters and structure caused by uncontrollable external factors, which can be efficiently modeled using Markov chains. As a result, STDNNs with Markov chains, namely hybrid STDNNs, are widely used to describe more complex dynamic phenomena in networks (see, e.g., [10,11,12,13]). However, Markov chains are unable to simulate random jumps of network states. In reality, many networks experience random state jumps due to unforeseen events such as earthquakes, storms, and floods. Lévy processes can describe such random jumps well, as these processes have significant tail and peak pulses. There are several related research achievements (see, e.g., [14,15,16,17]). Lately, the stability of hybrid stochastic delayed Cohen–Grossberg neural networks driven by Lévy noise has been investigated in [18], and the stabilization problem for a class of hybrid SDDEs with Lévy noise has been studied in [19]. Hence, it is worthwhile to consider Lévy noise in hybrid STDNNs.
It is already known that the stability of SNNs may decrease or eventually turn unstable under time delay, Markov chains, and so on. It is significant to consider how to make unstable SNNs stable. Various control strategies have been advanced to achieve the stabilization of a system. For example, Li and Mao used delayed feedback control to stabilize nonlinear hybrid SDDEs in [20]. Li and co-workers proposed feedback control based on discrete-time observations of state and mode to stabilize hybrid SDDEs with non-differential delays in [21].
Note that the stabilization problem of highly nonlinear STDNNs has been discussed in [8], but does not take into account non-differentiable time delay. The delayed feedback controls used in [19,20] have considered the time lag between the time of state observation and when the corresponding control reaches the system. Still, the control functions require continuous-time observations of system state and mode; in contrast, the controller based on discrete-time state and mode observations is easier to implement in practice and relatively less costly. Inspired by the preceding discussion, the primary objective of this paper is to apply discrete-time feedback control for stabilizing nonlinear hybrid STDNNs with Lévy noise, where the time delay is non-differentiable. The major advantages of this paper can be categorized as below:
(1) The coefficients of the considered STDNNs are highly nonlinear, and the time delay is non-differentiable, which makes the results we obtained more general.
(2) The introduction of Markov chains and Lévy noise to nonlinear STDNNs makes theoretical exploration in this paper more challenging. Additionally, the feedback control employed is more practical than continuous-time feedback control because it is based on discrete-time states and mode observations.
(3) This paper develops new stabilization criteria for H∞ stability, asymptotic stability, and exponential stability of global solutions for nonlinear hybrid STDNNs with Lévy noise.
Notations: We denote by ℜn the n-dimensional Euclidean space, ℜ=(−∞,+∞), and ℜ+=[0,+∞). For Φ∈ℜn, we define |Φ| as its Euclidean norm. Denote by BT the transpose of a vector or matrix B. With respect to a matrix B, |B|=√trace(BTB) denotes its trace norm. If B is a symmetric real matrix, its smallest and largest eigenvalues are expressed by λmin(B) as well as λmax(B), respectively. The family of cˊadlˊag functions ϱ:[−θ,0]→ℜn are denoted by D([−θ,0];ℜn) for θ>0, with its norm defined as ‖ϱ‖=sup−θ≤u≤0|ϱ(u)|. The family of all bounded, F0-measurable, D([−θ,0];ℜn)-valued random variables is denoted as DbF0([−θ,0];ℜn). For real numbers m and n, m∨n=max{m,n} as well as m∧n=min{m,n}. Let B be a subset of Ω, and IB represents its indicator function. Specifically, IB(ν)=1 if ν∈B and 0 else. Suppose that W(t)=(W1(t),⋯,Wm(t)) is an m-dimensional Brownian motion defined in the complete probability space (Ω,F,{Ft}t≥0,P) whose associated filtration {Ft}t≥0 satisfies the usual conditions. The Poisson random measure N(dt,dτ) is formed on ℜ+×Y, where Y=ℜn−{0}. The compensated Poisson random measure, denoted as ˜N(dt,dτ)=N(dt,dτ)−ϑ(dτ)dt, is introduced, with ϑ being a Lévy measure that satisfies
∫Y(1∧|τ|2)ϑ(dτ)<∞. | (2.1) |
In general, this pair (W,N) is known as Lévy noise.
Remark 1.1. (2.1) describes the properties of Lévy measure ϑ for noise, ensuring that the noise exhibits appropriate heavy-tailed behavior. Specifically, the term 1∧|τ|2 is used to regulate the noise tail, avoiding the excessive effects of tremendous noise values on the system. In physical systems, this type of noise is often used to describe phenomena with long memory or long-range dependence. In biological systems, noise sources that satisfy (2.1) are utilized to model processes with significant stochasticity.
Let π(t),t≥0 be a right-continuous Markov chain on the probability space with state space S={1,2,…,N} and generator Γ=[γij]N×N, where γij≥0 and γii=−∑Nj=1,j≠iγij≤0. Furthermore, we assume that π(⋅),W(⋅),˜N(⋅,⋅) are mutually independent. Generally speaking, the nonlinear hybrid STDNNs subject to Lévy noise have the following form:
dι(t)=[−A(π(t))ι(t−)+B(π(t))f(ι(t−),ι((t−θt)−),π(t),t)]dt+h(ι(t−),ι((t−θt)−),π(t),t)dW(t)+∫0<|τ|<eg(ι(t−),ι((t−θt)−),π(t),t,τ)˜N(dt,dτ)+∫|τ|≥eG(ι(t−),ι((t−θt)−),π(t),t,τ)N(dt,dτ), | (2.2) |
where
A(π(t))=diag{a1(π(t)),cdots,an(π(t))},B(π(t))=(bij(π(t)))n×n, |
and for any 1≤i,j≤n, ai(π(t))>0 and bij(π(t)) are real values. ι(t−)=limu→tι(u), f∈C(ℜn×ℜn×S×ℜ+;ℜn), h∈C(ℜn×ℜn×S×ℜ+;ℜn×m), g∈C(ℜn×ℜn ×S×ℜ+×Y;ℜn), G∈C(ℜn×ℜn×S×ℜ+×Y;ℜn). The constant e∈(0,∞) lets us clarify the meaning of 'large' and 'small' jumps in concrete applications. Additionally, θt is a time-varying function. Notice that the last integral term in (2.2) represents a compound Poisson process, which can be easily managed by employing techniques such as the interlacing method (see, e.g., [22]). Therefore, it is meaningful to focus on equations driven by continuous noise interspersed with small jumps by omitting the large jump term. Consequently, this study will focus on the reduced STDNNs with small jumps in the form
dι(t)=[−A(π(t))ι(t−)+B(π(t))f(ι(t−),ι((t−θt)−),π(t),t)]dt+h(ι(t−),ι((t−θt)−),π(t),t)dW(t)+∫0<|τ|<eg(ι(t−),ι((t−θt)−),π(t),t,τ)˜N(dt,dτ) | (2.3) |
with initial condition
{{ι(t):−θ≤t≤0}=ς∈DbF0([−θ,0];ℜn),π(0)=π0, | (2.4) |
where ι(t−)=limu→tι(u). Next, we will describe the required assumptions and lemmas.
Assumption 2.1. The time-varying delay θt is a Borel measurable function from ℜ+ to [θ1,θ] with the following properties
ˉθ:=lim supΔ→0+(supu≥−θμ(Mu,Δ)Δ)<∞, | (2.5) |
where θ1 and θ are constants with 0≤θ1<θ,Mu,Δ={t∈ℜ+:t−θt∈[u,u+Δ)} as well as μ(⋅) represents the Lebesgue measure on ℜ+.
Remark 2.1. It is important to note that Assumption 2.1 is indeed weaker than the condition that the time-varying delay θt is differentiable and its derivative is bounded by a positive constant less than 1. Furthermore, many time-varying delay functions actually satisfy Assumption 2.1. For instance, assume that θt is satisfying the Lipschitz condition, namely,
|θt−θu|≤θ2(t−u)for all0≤u<t<∞, |
where θ2∈(0,1). For any u≥−θ, let s=inf{t∈Mu,Δ}. It is clear that s∈Mu,Δ, that is, u≤s−θs<u+Δ. If t≥s+Δ1−θ2, then
t−θt−u≥t−θt−(s−θs)≥t−s−|θt−θs|≥(1−θ2)(t−s)≥Δ. |
Consequently, t−θt≤u+Δ, namely, t∉Mu,Δ. Put differently, we have Mu,Δ⊂[s,s+Δ1−θ2), which implies μ(Mu,Δ)Δ≤11−θ2. Since this holds for any u≥−θ and Δ∈(0,1), Assumption 2.1 must hold with ˉθ=11−θ2. This suggests, in particular, that many sawtooth delays, such as
θt=∞∑i=1[(0.15+0.05(t−2i))I[2i,2i+1)(t)+(0.25−0.05(t−2i))I[2i+1,2(i+1))(t)] |
satisfy Assumption 2.1.
Lemma 2.1. [19] Under Assumption 2.1 is satisfied, let T>0 and η is a cˊadlˊag function from [−θ,T−θ1] to ℜ+ with at most finite number of jumps in any finite time interval. Then
∫T0η(t−θt)dt≤ˉθ∫T−θ1−θη(t)dt. | (2.6) |
Remark 2.2. Lemma 2.2 in [21] demands that η is continuous, but here the solution is cˊadlˊag. Hence we need to come up with a new lemma, that is, Lemma 2.1.
Furthermore, the coefficients of (2.3) considered are polynomially rather than linearly increasing, namely highly nonlinear. Therefore, we give the assumption as follows:
Assumption 2.2. Suppose that for any constants h>0, k,ˉk,l,ˉl∈ℜn and |k|∨|ˉk|∨|l|∨|ˉl|≤h, there exists a constant Hh satisfying
|f(k,l,i,t)−f(ˉk,ˉl,i,t)|∨|h(k,l,i,t)−h(ˉk,ˉl,i,t)|≤Hh(|k−ˉk|+|l−ˉl|), | (2.7) |
where (i,t)∈S×ℜ+. In addition, there are constants H>0,β1>1, as well as βi≥1(2≤i≤4) satisfying
|f(k,l,i,t)|≤H(|k|+|l|+|k|β1+|l|β2),|h(k,l,i,t)|≤H(|k|+|l|+|k|β3+|l|β4). | (2.8) |
Under Assumption 2.2, the existence and uniqueness of the maximal local solution for (2.3) can only be ensured. However, it has the potential to blow up to infinity in finite time. To escape this potential explosion, the following assumptions become necessary:
Assumption 2.3. Suppose there exist some positive constants α,β,ω1,ω2,ω3 which make
β>(α+β1−1)∨[2(β1∨β2∨β3∨β4)],α≥2(β1∨β2∨β3∨β4)−β1+1 | (2.9) |
and
ιT[−A(i)ι+B(i)f(ι,κ,i,t)]+β−12|h(ι,κ,i,t)|2≤ω1(|ι|2+|κ|2)−ω2|ι|α+ω3|κ|α | (2.10) |
hold. Furthermore, let us assume that ω2>ω3ˉθ. Assuming that
q1=βω2−ω3β(β−2)α+β−2,q2=ω3αβα+β−2, |
we obtain q1>q2ˉθ.
Assumption 2.4. [19] For any L∈ℜ+, there exists a constant ϕL so that for any k,ˉk,l,ˉl∈ℜn and |k|∨|ˉk|∨|l|∨|ˉl|≤L, we have
∫0<|τ|<e|g(l,k,i,t,τ)−g(ˉl,ˉk,i,t,τ)|ϑ(dτ)≤ϕL(|l−ˉl|+|k−ˉk|), | (2.11) |
where (i,t)∈S×ℜ+. There are constants χ>0 and ω≥1 so that for 0<|τ|<e, satisfying
|g(ι,κ,i,t,τ)|≤χ|τ|ω(|ι|+|κ|). | (2.12) |
Under Assumptions 2.1–2.4, it is known that (2.3) possesses a unique global solution and sup−θ≤u<∞E |ι(u)|β<∞, but the stability of (2.3) is not guaranteed (see, e.g., [19]). Our goal is to devise a feedback control function u(⋅,⋅,⋅) to enable the controlled nonlinear hybrid STDNNs with Lévy noise
dι(t)=[−A(π(t))ι(t−)+B(π(t))f(ι(t−),ι((t−θt)−),π(t),t)+u(ι(σt−),π(σt),t))]dt+h(ι(t−),ι((t−θt)−),π(t),t)dW(t)+∫0<|τ|<eg(ι(t−),ι((t−θt)−),π(t),t,τ)˜N(dt,dτ) | (2.13) |
become stable, where u:ℜn×S×ℜ+→ℜn is Borel measurable, ι(σt−)=limu→tι(σu), as well as σt=[t/λ]λ, where [t/λ] is the integer part of t/λ. λ>0 denotes the duration between two consecutive observations. The assumption about the function u(⋅,⋅,⋅) will be made as below.
Assumption 2.5. Consider all ι,κ∈ℜn, suppose that there is a constant ρ satisfying
|u(ι,i,t)−u(κ,i,t)|≤ρ|ι−κ|, | (2.14) |
where (i,t)∈S×ℜ+. Also suppose that u(0,i,t)=0.
To deal with the discrete-time Markov chain, we introduce the lemma as follows:
Lemma 2.2. [21] For all t≥0,m>0, as well as i∈S, one has
P(π(u)≠ifor someu∈[t,t+m]|π(t)=i)≤1−e−ˉγm, |
where ˉγ=maxi∈S(−γii).
Within this section, we will present a theorem establishing the existence and uniqueness of a global solution for (2.13), with the property that the solution is Lβ-bounded.
Theorem 3.1. According to Assumptions 2.1–2.5, for any initial condition (2.4), the hybrid STDNNs (2.13) yield a unique global solution ι(t) on [−θ,∞) satisfying
sup−θ≤t<∞E|ι(t)|β<∞. | (3.1) |
Proof. Define a bounded function φ:ℜ+→[0,λ] with the form
φ(t)=t−mλformλ≤t<(m+1)λ,m=0,1,2,⋯, |
then (2.13) can be represented as
dι(t)=[−A(π(t))ι(t−)+B(π(t))f(ι(t−),ι((t−θt)−),π(t),t)+u(ι((t−φ(t))−),π(t−φ(t)),t))]dt+h(ι(t−),ι((t−θt)−),π(t),t)dW(t)+∫0<|τ|<eg(ι(t−),ι((t−θt)−),π(t),t,τ)˜N(dt,dτ). | (3.2) |
Given F(ι)=|ι|β, from the generalized Itˆo formula, one has
dF(ι(t))=LF(ι(t−),ι((t−θt)−),ι((t−φ(t))−),π(t),π(t−φ(t)),t)dt+M(t), | (3.3) |
where the specific form of M(t) is not used and will not be described in detail here. The operator LF from ℜn×ℜn×ℜn×S×S×ℜ+ to ℜ is defined as
LF(ι,κ,z,i,ˆi,t)=β|ι|β−2ιT[−A(i)ι+B(i)f(ι,κ,i,t)+u(z,ˆi,t)]+β2|ι|β−2|h(ι,κ,i,t)|2+β(β−2)2|ι|β−4|ιTh(ι,κ,i,t)|2+∫0<|τ|<e{|ι+g(ι,κ,τ,i,t)|β−|ι|β−β|ι|β−2ιTg(ι,κ,τ,i,t)}ϑ(dτ)≤β|ι|β−2(ιT[−A(i)ι+B(i)f(ι,κ,i,t)+u(z,ˆi,t)]+β−12|h(ι,κ,i,t)|2)+∫0<|τ|<e{|ι+g(ι,κ,τ,i,t)|β−|ι|β−β|ι|β−2ιTg(ι,κ,τ,i,t)}ϑ(dτ). |
In accordance with the proof of [19, Lemma 2.7], we can find two constants b1,b2>0 that satisfy
∫0<|τ|<e[|ι+g(ι,κ,τ,i,t)|β−|ι|β−β|ι|β−2ιTg(ι,κ,τ,i,t)]ϑ(dτ)≤b1|ι|β+b2|κ|β. | (3.4) |
Using Assumptions 2.3, 2.5, and (3.4), we can conclude that
LF(ι,κ,z,i,ˆi,t)≤βρ|ι|β−1|z|+ω1β|ι|β+ω1β|ι|β−2|κ|2−ω2β|ι|α+β−2+ω3β|ι|β−2|κ|α+b1|ι|β+b2|κ|β. | (3.5) |
Based on Assumption 2.3, we can pick a constant δ0 to ensure that
0<δ0<q1−q2ˉθ,2−1θlnq1−δ0q2ˉθ>0 |
and further, another constant δ to satisfy
0<δ<min{2ˉθ,2−1θlnq1−δ0q2ˉθ1+q1−δ0q2}. |
According to Young inequality, we have
βρ|ι|β−1|z|≤ˆu|ι|β+δ|z|β,ω1β|ι|β−2|κ|2≤ˆu|ι|β+δ|κ|β,β|ι|β−2|κ|α≤β−2α+β−2|ι|α+β−2+αα+β−2|κ|α+β−2, |
where ˆu denotes a positive constant. Therefore, we can obtain
LF(ι,κ,z,i,ˆi,t)≤U−2|ι|β+δ|κ|β+δ|z|β−(q1−δ0)|ι|α+β−2+q2|κ|α+β−2, | (3.6) |
where U=sups≥0[(2ˆu+2+ω1β+b1+b2)|s|β−δ0|s|α+β−2]. Since the proof closely resembles that of [21, Theorem 3.1], it is omitted here.
Remark 3.1. ˆu changes may occur from line to line, but their particular form is not used.
This section discusses the stabilization characteristic of (2.13). Next, we introduce some necessary assumptions.
Assumption 4.1. Designing the control function u:ℜn×S×ℜ+→ℜn, for any ι,κ∈ℜn, we can find constants pi,ˉpi∈ℜ, positive constants ˆpi,ˆci,mi,ˉmi, as well as ci,ˉci,ni,ˉni∈ℜ+(i∈S) satisfying
2[ιT[−A(i)ι+B(i)f(ι,κ,i,t)+u(ι,i,t)]+12|h(ι,κ,i,t)|2]+∫0<|τ|<e[|ι+g(ι,κ,i,t,τ)|2−|ι|2−2ιTg(ι,κ,i,t,τ)]ϑ(dτ)≤pi|ι|2+ci|κ|2−mi|ι|α+ni|κ|α, | (4.1) |
ιT[−A(i)ι+B(i)f(ι,κ,i,t)+u(ι,i,t)]+β12|h(ι,κ,i,t)|2≤ˉpi|ι|2+ˉci|κ|2−ˉmi|ι|α+ˉni|κ|α, | (4.2) |
and
∫0<|τ|<e[|ι+g(ι,κ,i,t,τ)|β1+1−|ι|β1+1−(β1+1)|ι|β1−1ιTg(ι,κ,i,t,τ)]ϑ(dτ)≤ˆpi|ι|β1+1+ˆci|κ|β1+1, | (4.3) |
where (i,t)∈S×ℜ+, while
P1:=−diag(p1,p2,⋯,pN)−ΓandP2:=−diag((β1+1)ˉp1+ˆp1,⋯,(β1+1)ˉpN+ˆpN)−Γ |
are nonsingular M-matrices. In addition,
ξ1<1,ξ3ˉθ<ξ2,ξ4(β1−1+2ˉθ)β1+1<1andξ6(β1−1+αˉθ)α+β1−1<ξ5, | (4.4) |
where
ξ1=maxi∈Sϱici,ξ2=mini∈Sϱimi,ξ3=maxi∈Sϱini,ξ4=maxi∈S[(β1+1)ˉci+ˆci]ˉϱi,ξ5=mini∈S(β1+1)ˉϱiˉmi,ξ6=maxi∈S(β1+1)ˉϱiˉni, | (4.5) |
in which
(ϱ1,⋯,ϱN)T=P−11(1,⋯,1)T,(ˉϱ1,⋯,ˉϱN)T=P−12(1,⋯,1)T. | (4.6) |
Based on the principles of M-matrix theory, we can conclude that the nonsingularity of the M-matrices P1 and P2 ensures that all ϱi and ˉϱi defined in (4.6) are positive.
Define the function
V(ι,i)=ϱi|ι|2+ˉϱi|ι|β1+1,(ι,i)∈ℜn×S, | (4.7) |
and the function LV by
LV(ι,κ,i,t)=2ϱi(ιT[−A(i)ι+B(i)f(ι,κ,i,t)+u(ι,i,t)]+12|h(ι,κ,i,t)|2)+(β1+1)ˉϱi(|ι|β1−1ιT[−A(i)ι+B(i)f(ι,κ,i,t)+u(ι,i,t)]+12|ι|β1−1|h(ι,κ,i,t)|2+β1−12|ι|β1−3|ιTh(ι,κ,i,t)|2)+N∑k=1γik(ϱk|ι|2+ˉϱk|ι|β1+1)+∫0<|τ|<eϱi[|ι+g(ι,κ,i,t,τ)|2−|ι|2−2ιTg(ι,κ,i,t,τ)]ϑ(dτ)+∫0<|τ|<eˉϱi[|ι+g(ι,κ,i,t,τ)|β1+1−|ι|β1+1−(β1+1)|ι|β1−1ιTg(ι,κ,i,t,τ)]ϑ(dτ). | (4.8) |
According to (4.1)–(4.3), (4.5), (4.6), and Young inequality, we can deduce that
LV(ι,κ,i,t)≤−|ι|2+ξ1|κ|2−ξ2|ι|α+ξ3|κ|α−(1−ξ4(β1−1)β1+1)|ι|β1+1+2ξ4β1+1|κ|β1+1−(ξ5−ξ6(β1−1)α+β1−1)|ι|α+β1−1+ξ6αα+β1−1|κ|α+β1−1. | (4.9) |
Assumption 4.2. Suppose that there are constants ψi>0(1≤i≤9) to make
LV(ι,κ,i,t)+ψ1(2ϱi|ι|+(β1+1)ˉϱi|ι|β1)2+ψ2|−A(i)ι+B(i)f(ι,κ,i,t)|2+ψ3|h(ι,κ,i,t)|2+ψ4∫0<|τ|<e|g(ι,κ,i,t,τ)|2ϑ(dτ)≤−ψ5|ι|2+ψ6|κ|2−Φ(ι)+ψ7Φ(κ) | (4.10) |
and
ψ8|ι|α+β1−1≤Φ(ι)≤ψ9(1+|ι|α+β1−1) | (4.11) |
hold, where ψ5>ψ6ˉθ,ψ7∈(0,1/ˉθ) and Φ∈C(ℜn;ℜ+).
Next, we describe our stabilization rules.
Theorem 4.1. Assume that Assumptions 2.1–2.5, 4.1, and 4.2 are satisfied, and let
ζ=9ρ24ψ1(1+8(1−e−ˉγ6ρ)). | (4.12) |
If λ is a sufficiently small positive constant such that
λ≤√ψ22ζ∧ψ3ζ∧ψ4ζ∧16ρ | (4.13) |
and
ψ5−ψ6ˉθ−4ζλ2ρ2−4ρ2ψ1(1−e−ˉγλ)>0 | (4.14) |
hold, then the solution of (2.13) satisfies
∫∞0E|ι(t)|ˉβdt<∞,ˉβ∈[2,α+β1−1] | (4.15) |
for any initial condition (2.4).
Proof. For convenience, the proof is divided into two steps.
Step 1. For t∈ℜ+, we define ˆιt={ι(t+s):−2θ≤s≤0} and ˆπt={π(t+s):−2θ≤s≤0}. For ˆιt and ˆπt to be well defined for t∈[0,2θ], define ι(s)=ς(−θ) for s∈[−2θ,−θ) and π(s)=π0 for s∈[−2θ,0). Next, we use the Lyapunov function stated below
U(ˆιt,ˆπt,t)=V(ι(t),π(t))+ζ∫0−λ∫tt+sQ(u)duds | (4.16) |
for t≥0, where V has been determined by (4.7) and
Q(u)=λ|−A(π(u))ι(u−)+B(π(u))f(ι(u−),ι((u−θu)−),π(u),u)+u(ι(σu−),π(σu),u)|2+|h(ι(u−),ι((u−θu)−),π(u),u)|2+∫0<|τ|<e|g(ι(u−),ι((u−θu)−),τ,π(u),u)|2ϑ(dτ). | (4.17) |
For u∈[−2θ,0), we set f(ι,κ,i,u)=f(ι,κ,i,0),g(ι,κ,i,u)=g(ι,κ,i,0),u(ι,i,u)=u(ι,i,0). Based on Itˆo formula, it follows that
dV(ι(t−),π(t))=LV(ι(t−),ι((t−θt)−),ι(σt−),π(t),π(σt),t)dt+dM(t), | (4.18) |
where M(t) denotes the continuous local martingale with M(0)=0 and LV is defined by
LV(ι,κ,z,i,ˆi,t)=2ϱi(ιT[−A(i)ι+B(i)f(ι,κ,i,t)+u(z,ˆi,t)]+12|h(ι,κ,i,t)|2)+(β1+1)ˉϱi|ι|β1−1(ιT[−A(i)ι+B(i)f(ι,κ,i,t)+u(z,ˆi,t)]+12|h(ι,κ,i,t)|2)+(β1+1)(β1−1)2¯ϱi|ι|β1−3|ιTh(ι,κ,i,t)|2+N∑k=1γik(ϱk|ι|2+ˉϱk|ι|β1+1)+∫0<|τ|<eϱi[|ι+g(ι,κ,i,t,τ)|2−|ι|2−2ιTg(ι,κ,i,t,τ)]ϑ(dτ)+∫0<|τ|<eˉϱi[|ι+g(ι,κ,i,t,τ)|β1+1−|ι|β1+1−(β1+1)|ι|β1−1ιTg(ι,κ,i,t,τ)]ϑ(dτ)=LV(ι,κ,i,t)−[2ϱi+(β1+1)ˉϱi|ι|β1−1]ιT[u(ι,i,t)−u(z,ˆi,t)]. |
In addition, we can obtain
d(ζ∫0−λ∫tt+sQ(u)duds)=ζλQ(t)−ζ∫tt−λQ(u)du. | (4.19) |
Together with (4.18) and (4.19), we obtain
dU(ˆιt,ˆπt,t)≤LU(ˆιt−,ˆπt,t)dt+dM(t), | (4.20) |
where
LU(ˆιt−,ˆπt,t)=LV(ι(t−),ι((t−θt)−),π(t),t)+ψ1(2ϱπ(t)|ι(t−)|+(β1+1)ˉϱπ(t)|ι(t−)|β1)2+14ψ1|u(ι(t−),π(t),t)−u(ι(σt−),π(σt),t)|2+ζλQ(t)−ζ∫tt−λQ(u)du. | (4.21) |
Under Assumptions 2.2–2.5, 4.1, and Theorem 3.1, we can intuitively see that
E|LU(ˆιt−,ˆπt,t)|<∞,∀t≥0. | (4.22) |
Step 2. For a sufficiently large positive constant v0, consider the initial value ||ς||<v0. For any v0≤v, define the stopping time ϖv=inf{t≥0:|ι(t)|≥v}, for ϖv is increasing as v→∞ and limv→∞ϖv=∞. By generalized Itˆo formula, it is possible to obtain from (4.20) that
EU(ˆιt∧ϖv,ˆπt∧ϖv,t∧ϖv)≤U(ˆι0,ˆπ0,0)+E∫t∧ϖv0LU(ˆιs−,ˆπs,s)ds. |
Based on (4.22), let v→∞ and then apply the dominated convergence theorem and Fubini theorem to have
0≤EU(ˆιt,ˆπt,t)≤U(ˆι0,ˆπ0,0)+∫t0E(LU(ˆιs−,ˆπs,s))ds. | (4.23) |
According to (4.13), (4.21), Assumptions 2.5 and 4.2, we can conclude that
E(LU(ˆιt−,ˆπt,t))≤−ψ5E|ι(t−)|2+ψ6E|ι((t−θt)−)|2−EΦ(ι(t−))+ψ7EΦ(ι((t−θt))−)+2ζλ2ρ2E|ι(σt−)|2+14ψ1E|u(ι(t−),π(t),t)−u(ι(σt−),π(t),t)+u(ι(σt−),π(t),t)−u(ι(σt−),π(σt),t)|2−ζE∫tt−λQ(u)du≤−ψ5E|ι(t−)|2+ψ6E|ι((t−θt)−)|2−EΦ(ι(t−))+ψ7EΦ(ι((t−θt))−)+4ζλ2ρ2E|ι(σt−)−ι(t−)|2+4ζλ2ρ2E|ι(t−)|2+ρ22ψ1E|ι(t−)−ι(σt−)|2+12ψ1E|u(ι(σt−),π(t),t)−u(ι(σt−),π(σt),t)|2−ζE∫tt−λQ(u)du. | (4.24) |
Furthermore, under Assumption 2.5 and Lemma 2.2, it is concluded that
E|u(ι(σt−),π(t),t)−u(ι(σt−),π(σt),t)|2=E[E|u(ι(σt−),π(t),t)−u(ι(σt−),π(σt),t)|2|Fσt]≤E[4ρ2|ι(σt−)|2E(1{π(σt)≠π(t)}|Fσt)]=E[4ρ2|ι(σt−)|2E(∑i∈S1{π(σt)=i}1{π(t)≠i}|Fσt)]=E[4ρ2|ι(σt−)|2∑i∈S1{π(σt)=i}×P(π(t)≠i|π(σt)=i)]≤E[4ρ2|ι(σt−)|2(1−e−ˉγλ)]=4ρ2(1−e−ˉγλ)E|ι(σt−)|2≤8ρ2(1−e−ˉγλ)E|ι(t−)−ι(σt−)|2+8ρ2(1−e−ˉγλ)E|ι(t−)|2. | (4.25) |
Moreover, in view of the fact that t−σt≤λ holds for all t≥0, it can be proved on (2.13) that
E|ι(t)−ι(σt)|2≤3E∫tt−λ[λ|−A(π(u))ι(u−)+B(π(u))f(ι(u−),ι((u−θu)−),π(u),u)+u(ι(σu−,π(σu),u))|2+|h(ι(u−),ι((u−θu)−),π(u),u)|2+∫0≤|τ≤e|g(ι(u−),ι((u−θu)−),π(u),u,τ)|2ϑ(dτ)]du. | (4.26) |
Notice that ∫t0E|ι(u)−ι(σu)|2du is the equivalent of ∫t0E|ι(u−)−ι(σu−)|2du. Substituting (4.25) and (4.26) into (4.24), and using (4.13) and (4.14), it can be found that
EU(ˆιt,ˆπt,t)≤U(ˆι0,ˆπ0,0)−[ψ5−4ξλ2ρ2−4ρ2ψ1(1−e−ˉγλ)]E∫t0|ι(u−)|2du−E∫t0Φ(ι(u−))du+ψ6E∫t0|ι((u−θu)−)|2du+ψ7E∫t0Φ(ι((u−θu)−))du. |
Using Lemma 2.1, we obtain
E∫t0|ι((u−θu)−)|2du≤ˉθE∫t−θ1−θ|ι(u−)|2du≤ˉθ(∫0−θ|ι(u−)|2du+E∫t0|ι(u−)|2du) |
and
E∫t0Φ(ι((u−θu)−))du≤ˉθE∫t−θ1−θΦ(ι(u−))≤ˉθ(∫0−θΦ(ι(u−))du+E∫t0Φ(ι(u−))du). |
Therefore, we can further deduce
EU(ˆιt,ˆπt,t)≤H1−[ψ5−4ξλ2ρ2−4ρ2ψ1(1−e−ˉγλ)−ψ6ˉθ]∫t0E|ι(u−)|2du−(1−ψ7ˉθ)∫t0EΦ(ι(u−))du, |
where H1=U(ˆι0,ˆπ0,0)+ˉθθsup−θ≤u<0[ψ6E|ι(u)|2+ψ7EΦ(ι(u))]≤∞. From (4.14) and ψ7∈(0,1/ˉθ), we get
∫t0E|ι(u)|2du≤H1ψ5−4ξλ2ρ2−4ρ2ψ1(1−e−ˉγλ)−ψ6ˉθ,∫t0EΦ(ι(u))du≤H11−ψ7ˉθ. |
Let t→∞ and combine with (4.11) to obtain
∫∞0E|ι(u)|2du<∞,∫∞0E|ι(u)|α+β1−1du<∞, |
which means that the needed assertion (4.15) holds.
Theorem 4.2. Assuming that conditions of Theorem 4.1 hold, the solution of (2.13) satisfies
limt→∞E|ι(t)|ˉβ=0,ˉβ∈[2,β) | (4.27) |
for any initial condition (2.4).
Proof. From Theorem 3.1, let H2=sup−θ≤u<∞E|ι(t)|β<∞. We can prove, as (3.4) is to be shown, that there are two constants ˆb1,ˆb2>0 that make
∫0<|τ|<e[|ι+g(ι,κ,τ,i,t)|β−|ι|β−β|ι|β−2ιTg(ι,κ,τ,i,t)]ϑ(dτ)≤ˆb1|ι|β+ˆb2|κ|β. |
For any 0≤t1<t2<∞, under Assumptions 2.2, 2.3, and 2.5, with the use of Itˆo formula, we obtain
|E|ι(t2)|2−E|ι(t1)|2|≤|E∫t2t1[2|ι(t−)|(−A(π(t))ι(t−)+B(π(t))f(ι(t−),ι((t−θt)−),π(t),t)+u(ι(σt−),π(t−σt),t))+|h(ι(t−),ι((t−θt)−),π(t),t)|2+∫0<|τ|<e(|ι(t−)+g(ι(t−),ι((t−θt)−),π(t),t,τ)|2−|ι(t−)|2−2ιT(t−)g(ι(t−),ι((t−θt)−),π(t),t,τ))ϑ(dτ)]dt|≤E∫t2t1(2|ι(t−)|(˘a|ι(t−)|+H˘b(|ι(t−)|+|ι((t−θt)−)|+|ι(t−)|β1+|ι((t−θt)−)|β2)+2ρ|ι(t−)||ι(σt−)|+H2(|ι(t−)|+|ι((t−θt)−)|+|ι(t−)|β3+|ι((t−θt)−)|β4)2+ˆb1|ι(t−)|2+ˆb2|ι((t−θt)−)|2)dt≤∫t2t1H3(1+E|ι(t−)|β+E|ι((t−θt)−)|β+E|ι(σt−)|β)dt≤H3(1+3H2)(t2−t1), |
where ˘a=max1≤i≤n{ai},˘b=max1≤i,j≤n{bij} and H3 is a constant unrelated to t1 and t2. Hence, E|ι(t)|2 is uniformly continuous at t on ℜ+. This, combined with (4.15), means that
limt→∞E|ι(t)|2=0. | (4.28) |
Next, fix any ˉβ∈(2,β), it is obtained from the hölder inequality
E|ι(t)|ˉβ≤(E|ι(t)|2)(β−ˉβ)/(β−2)H(β−2)/(β−2)2. | (4.29) |
In combination with (4.28), it is implied that the required (4.27) holds.
Theorem 4.3. Assume that Assumptions 2.1–2.5, 4.1, and 4.2 are satisfied, and recall that
ζ=9ρ24ψ1(1+8(1−e−ˉγ6ρ)). | (4.30) |
If λ>0 is a small enough constant to make
λ≤√ψ22ζ∧ψ3ζ∧ψ4ζ∧16√2ρ | (4.31) |
and
ψ5−ψ6ˉθ−4ζλ2ρ2−4ρ2ψ1(1−e−ˉγλ)>0 | (4.32) |
hold, then the solution of (2.13) satisfies
lim supt→∞1tlog(E|ι(t)|ˉβ)<0 | (4.33) |
and
lim supt→∞1tlog(E|ι(t)|)<0a.s. | (4.34) |
for any initial condition (2.4) and ˉβ∈[2,β).
Proof. Similarly to the proof of Theorem 4.1, for t≥0, it is shown that
eγtEU(ˆιt∧ϖv,ˆπt∧ϖv,t∧ϖv)≤U(ˆι0,ˆπ0,0)+∫t0eγuE(γU(ˆιu−,ˆπu,u)+LU(ˆιu−,ˆπu,u))du, | (4.35) |
and γ is a sufficiently small positive constant. Setting ϵ1=mini∈Sϱi,ϵ2=maxi∈Sϱi,ϵ3=maxi∈Sˉϱi, we have
ϵ1eγtE|ι(t)|2≤U(ˆι0,ˆπ0,0)+∫t0eγu(γϵ2E|ι(u−)|2+γϵ3E|ι(u−)|β1+1)du+γζQ1(t)+∫t0eγuE(LU(ˆιu−,ˆπu,u))du, | (4.36) |
where
Q1(t)=E∫t0eγu(∫0−λ∫uu+vQ(s)dsdv)du. |
Analogous to the proof of Theorem 4.1,
E(LU(ˆιu−,ˆπu,u))≤−[ψ5−4ξλ2ρ2−4ρ2ψ1(1−e−ˉγλ)]E|ι(u−)|2−EΦ(ι(u−))du+ψ6E|ι((u−θu)−)|2+ψ7EΦ(ι((u−θu)−))−(ζ−12ζλ2ρ2−3ρ22ψ1+12ρ2ψ1(1−e−ˉγλ))E∫uu−λQ(v)dv. | (4.37) |
In addition, there is apparently
E|ι(u−)|β1+1≤E|ι(u−)|2+E|ι(u−)|α+β1−1≤E|ι(u−)|2+ψ−18EΦ(ι(u−)). | (4.38) |
From Lemma 2.1,
∫t0eγuE|ι((u−θu)−)|2du≤ˉθeγθ∫t−θ1−θeγuE|ι(u−)|2du≤ˉθeγθ(∫0−θeγuE|ι(u−)|2du+∫t0eγuE|ι(u−)|2du), |
∫t0eγuEΦ(ι((u−θu)−))du≤ˉθeγθ∫t−θ1−θeγuEΦ(ι(u−))du≤ˉθeγθ(∫0−θeγuEΦ(ι(u−))du+∫t0eγuEΦ(ι(u−))du). |
Substitute (4.37) and (4.38) into (4.36) to obtain
ϵ1eγtE|ι(t)|2≤H4−(ψ5−4ξλ2ρ2−4ρ2ψ1(1−e−ˉγλ)−γϵ2−γϵ3−ψ6ˉθeγθ)∫t0eγuE|ι(u−)|2du−(1−γϵ3ψ−18−ψ7ˉθeγθ)∫t0eγuEΦ(ι(u−))du+γζQ1(t)−(ζ−12ζλ2ρ2−3ρ22ψ1+12ρ2ψ1(1−e−ˉγλ))Q2(t), | (4.39) |
where
H4=U(ˆι0,ˆπ0,0)+ˉθeγθ(ψ6∫0−θeγuE|ι(u−)|2du+ψ7∫0−θeγuEΦ(ι(u−))du) |
as well as
Q2(t)=E∫t0eγu∫uu−λQ(v)dvdu. |
Clearly,
Q1(t)≤λQ2(t). |
Now, we may select a small enough constant γ>0 to satisfy
γλ≤16,1−γϵ3ψ−18−ψ7ˉθeγθ≥0,ψ5−4ξλ2ρ2−4ρ2ψ1(1−e−ˉγλ)−γϵ2−γϵ3−ψ6ˉθeγθ≥0. |
Again reviewing (4.30) and λ≤16√2ρ, from (4.39)
E|ι(t)|2≤H4ϵ1e−γt | (4.40) |
for any t∈ℜ+. Moreover, for any ˉβ∈(2,β), we have by (4.29) and (4.40) that
E|ι(t)|ˉβ≤H(β−2)/(β−2)2(E|ι(t)|2)(β−ˉβ)/(β−2)e−γt(β−ˉβ)/(β−2). | (4.41) |
Thus, the required assertion (4.33) is proved.
Put tk=kλ for k=0,1,2,⋯. By Hölder inequality and Doob martingale inequality, it is possible to show that
E(suptk≤t≤tk+1|ι(t)|2)≤4E|ι(tk)|2+4λE∫tk+1tk|−A(π(t))ι(t−)+B(π(t))f(ι(t−),ι((t−θt)−),π(t),t)+u(ι(σt−),π(σt),t)|2dt+16E∫tk+1tk|h(ι(t−),ι((t−θt)−),π(t),t)|2dt+16E∫tk+1tk∫0<|τ|<e|g(ι(t−),ι((t−θt)−),π(t),t,τ)|2ϑ(dτ)dt. |
Based on Assumptions 2.2, 2.4, and 2.5, it is possible to conclude that
E(suptk≤t≤tk+1|ι(t)|2)≤4E|ι(tk)|2+H5∫tk+1tkE(|ι(t−)|2+|ι((t−θt)−)|2+|ι(σt−)|2+|ι(t−)|ˉβ+|ι((t−θt)−)|ˉβ)dt, |
where ˉβ=2(β1∨β2∨β3∨β4) and H5>0 is a constant. From Assumption 2.3, we find ˉβ∈[2,β). We can obtain, by applying (4.40) and (4.41), that
E(suptk≤t≤tk+1|ι(t)|2)≤H6e−ˆεtk, |
where ˆε=γ(β−ˉβ)/(β−2) and H6>0 is also a constant. So
∞∑k=0P(suptk≤t≤tk+1|ι(t)|>e−0.25ˆεtk)≤∞∑k=0H6e−0.5ˆεtk<∞. |
It is shown by Borel–Cantelli Lemma that for almost all ˜ω∈Ω, there exists an integer k0=k0(˜ω)>0, which makes
suptk≤t≤tk+1|ι(t)|≤e−0.25ˆεtk,k≥k0. |
Thus, we have
1tlog(|ι(t)|)≤−0.25ˆεkλ(k+1)λ,t∈[tk,tk+1],k≥k0. |
This means
lim supt→∞1tlog(|ι(t)|)≤−0.25ˆε<0a.s. |
which is the desired assertion (4.34).
Remark 4.1. The asymptotic stability discussed in Theorem 4.2 states that the solution of system (2.13) will asymptotically converge to zero; however, its rate of decrease is not provided. In Theorem 4.3, we further show that the solution of system (2.13) converges to zero at an exponential rate.
Remark 4.2. In general, almost surely exponential stabilization cannot be obtained from exponential stabilization in Lˉβ, but it is possible in the content of this paper, as stated in Theorem 4.3.
Remark 4.3. Recently, Dong and collaborators [19] designed a feedback control function to stabilize highly nonlinear hybrid SDDEs with Lévy noise, which is based on continuous-time state and mode observations and difficult to implement in practice. Consequently, the feedback control function u(ι(σt−),π(σt),t) based on discrete-time state and mode observations used in this paper is more sensible and practical.
An example will be given throughout this section to demonstrate the validity of theoretical results.
Example 1. Consider the nonlinear hybrid STDNNs with Lévy noise
dι(t)=[−A(π(t))ι(t−)+B(π(t))f(ι(t−),ι((t−θt)−),π(t),t))]dt+h(ι(t−),ι((t−θt)−),π(t),t)dW(t)+∫0<|τ|<eg(ι(t−),ι((t−θt)−),π(t),t,τ)˜N(dt,dτ) | (5.1) |
on t≥0 and ι(t)=1+sin(t) for t∈[−0.2,0]. The coefficients are defined by A(1)=0.3,A(2)=0.2,B(1)=0.5,B(2)=0.6, and
f(ι,κ,i,t)={−ι3+ικ,i=1,−1.5ι3+1.2ικ,i=2,h(ι,κ,i,t)={0.2ικ,i=1,0.1ικ,i=2,g(ι,κ,τ,i,t)={0.5κτ−0.5ιτ,i=1,0.25κτ−0.5ιτ,i=2, |
where e=5, W(t) is a scalar Brownian motion, the Markov chain π(t) over the state space S={1,2}, possessing the generator matrix Γ=(−1,1;1,−1), and time delay θt=0.1|sin(t)|+0.1.
The Lévy measure ϑ is characterized by ϑ(dτ)=aϕ(dτ)=0.5×e−2|τ|dτ, where a=0.5 represents the jump rate, ϕ(⋅) is the jump distribution, and the probability density function of ϕ(⋅) is e−2|τ|. This ensures the fulfillment of (2.1).
We can check that Assumption 2.1 is true when θ1=0.1,θ=0.2,ˉθ=1.1111. Assumption 2.2 holds when β1=3,β2=β3=β4=2. Assumption 2.3 holds when α=4,ω1=0.72,ω2=0.19,ω3=0.06,q1=1.0967,q2=0.1867,β>6, we may then choose β=7 and satisfy the condition q1>q2ˉθ. Figure 1 indicates that (5.1) is unstable. To make it stable, we devise the control function
u(ι,1)=−2(|ι|∧1.8)ι/|ι|,u(ι,2)=−2.5(|ι|∧2)ι/|ι|, | (5.2) |
clearly, ρ=2.5 satisfies Assumption 2.5. The controlled nonlinear hybrid STDNNs with Lévy noise take the following form:
dι(t)=[−A(π(t))ι(t−)+B(π(t))f(ι(t−),ι((t−θt)−),π(t),t))+u(ι(σt−),π(σt),t)]dt+h(ι(t−),ι((t−θt)−),π(t),t)dW(t)+∫0<|τ|<eg(ι(t−),ι((t−θt)−),π(t),t,τ)˜N(dt,dτ). | (5.3) |
Due to
ιu(ι,i)≤0.095ι4−(2I1(i)+2.5I2(i))ι2, |
it follows that for (ι,κ,i,t,τ)∈ℜ×ℜ×S×ℜ+×Y, we obtain
2[ιT[−A(i)ι+B(i)f(ι,κ,i,t)+u(ι,i,t)]+12|h(ι,κ,i,t)|2]+∫0<|τ|<e[|ι+g(ι,κ,i,t,τ)|2−|ι|2−2ιTg(ι,κ,i,t,τ)]ϑ(dτ)≤{−3.413ι2+0.687κ2−0.29ι4+0.02κ4,i=1,−4.213ι2+0.7667κ2−0.885ι4+0.005κ4,i=2, |
ιT[−A(i)ι+B(i)f(ι,κ,i,t)+u(ι,i,t)]+β12|h(ι,κ,i,t)|2≤{−2.3ι2+0.25κ2−0.125ι4+0.03κ4,i=1,−2.7ι2+0.36κ2−0.4375ι4+0.0075κ4,i=2, |
and
∫0<|τ|<e[|ι+g(ι,κ,i,t,τ)|4−|ι|4−(4)|ι|2ιTg(ι,κ,i,t,τ)]ϑ(dτ)≤{1.4748ι4+0.7268κ4,i=1,0.5834ι4+0.2455κ4,i=2. |
Hence, (4.1)–(4.3) hold when
p1=−3.413,c1=0.687,m1=0.29,n1=0.02,p2=−4.213,c2=0.7667,m2=0.885,n2=0.005,ˉp1=−2.3,ˉc1=0.25,ˉm1=0.125,ˉn1=0.03,ˉp2=−2.7,ˉc2=0.36,ˉm2=0.4375,ˉn2=0.0075,ˆp1=1.4748,ˆc1=0.7268,ˆp2=0.8534,ˆc2=0.2455, |
as well as
P1=(4.413−1−15.213),P2=(8.7252−1−110.9466), |
which are both M-matrices. From (4.6), we derive
ϱ1=0.2823,ϱ2=0.2459,ˉϱ1=0.1264,ˉϱ2=0.1029. |
So
ξ1=0.1939,ξ2=0.0819,ξ3=0.0056,ξ4=0.2183,ξ5=0.1801,ξ6=0.0152, |
which satisfies (4.4). It is obvious that
V(ι,i)={0.2823ι2+0.1264ι4,i=1,0.2459ι2+0.1029ι4,i=2. |
As a result of (4.9), we obtain
LV(ι,κ,i,t)≤−ι2+0.1939κ2−0.9727ι4+0.1148κ4−0.175ι6+0.0101κ6. |
In addition, we have
(2ϱi|ι|+(β1+1)ˉϱi|ι|β1)2≤0.3188ι2+0.5709ι4+0.2556ι6,|−A(i)ι+B(i)f(ι,κ,i,t)|2≤0.27ι2+0.7776ι4+0.7776κ4+2.43ι6,|h(ι,κ,i,t)|2≤0.02ι4+0.02κ4,∫0<|τ|<e|g(ι,κ,i,t,τ)|2ϑ(dτ)≤0.0623ι2+0.0623κ2. |
Selecting ψ1=0.2,ψ2=0.01,ψ3=0.3, and ψ4=0.5, we obtain
LV(ι,κ,i,t)+ψ1(2ϱi|ι|+(β1+1)ˉϱi|ι|β1)2+ψ2|−A(i)ι+B(i)f(ι,κ,i,t)|2+ψ3|h(ι,κ,i,t)|2+ψ4∫0<|τ|<e|g(ι,κ,i,t,τ)|2ϑ(dτ)≤−0.9023ι2+0.2251κ2−0.8722ι4+0.1286κ4−0.0995ι6+0.0101κ6≤−0.9023ι2++0.2251κ2−Φ(ι)+0.1474Φ(κ), |
where Φ(ι)=0.8722ι4+0.0995ι6, ψ5=0.9023,ψ6=0.2251,ψ7=0.1474,ψ8=0.0995, and ψ9=0.9717. Based on Theorems 4.1 and 4.3, we have ζ=106.5937 and λ≤0.0028. Thus, in view of Theorems 4.1–4.3, it follows that the controlled nonlinear hybrid STDNNs with Lévy noise (5.3) are H∞-stable, asymptotically stable, and exponentially stable in Lˉβ for any ˉβ∈[2,7). We perform a computer simulation with initial data ι(t)=1+sin(t) for t∈[−0.2,0] and π(0)=1. Figure 2 shows sample paths of the Markov chain and the solution of controlled STDNNs (5.3). The simulation results apparently support our theoretical results.
In this paper, we probe into the stabilization problem of nonlinear hybrid STDNNs with Lévy noise, whose coefficients are highly nonlinear. Unlike the constant time delay considered in [20] and the time delay studied in [8], which is a continuous function, we focus on the case where the time delay of STDNNs is time-varying and non-differentiable. We employ feedback control based on discrete-time state and mode observations to make unstable nonlinear hybrid STDNNs with Lévy noise stable. In addition, utilizing M-matrix theory and Lyapunov functional techniques, we explore the H∞ stability, asymptotic stability, and exponential stability of the controlled nonlinear hybrid STDNNs with Lévy noise. In future work, we will explore introducing mixed delays into highly nonlinear hybrid SNNs with Lévy noise [23]. Additionally, incorporating Lévy noise into metapopulation models will be considered (see, e.g., [24,25]), as it could enhance the realism and complexity of the models.
Tian Xu: Writing-original draft; Ailong Wu: Supervision, writing-review & editing. All authors have read and approved the final version of the manuscript for publication.
This work is supported by the National Natural Science Foundation of China under Grant 62476082 and the Natural Science Foundation of Hubei Province of China under Grant 2021CFA080.
The authors declare that there are no conflicts of interest.
[1] |
S. Blythe, X. R. Mao, X. X. Liao, Stability of stochastic delay neural networks, J Franklin Inst., 338 (2001), 481–495. https://doi.org/10.1016/S0016-0032(01)00016-3 doi: 10.1016/S0016-0032(01)00016-3
![]() |
[2] |
Y. Chen, W. X. Zheng, Stability analysis of time-delay neural networks subject to stochastic perturbations, IEEE Trans. Cybern., 43 (2013), 2122–2134. https://doi.org/10.1109/TCYB.2013.2240451 doi: 10.1109/TCYB.2013.2240451
![]() |
[3] |
Z. D. Wang, H. S. Shu, J. A. Fang, X. H. Liu, Robust stability for stochastic Hopfield neural networks with time delays, Nonlinear Anal.: Real World Appl., 7 (2006), 1119–1128. https://doi.org/10.1016/j.nonrwa.2005.10.004 doi: 10.1016/j.nonrwa.2005.10.004
![]() |
[4] |
P. F. Wang, Q. J. He, H. Su, Stabilization of discrete-time stochastic delayed neural networks by intermittent control, IEEE Trans. Cybern., 53 (2021), 2017–2027. https://doi.org/10.1109/TCYB.2021.3108574 doi: 10.1109/TCYB.2021.3108574
![]() |
[5] |
D. N. Lu, D. B. Tong, Q. Y. Chen, W. N. Zhou, J. Zhou, S. G. Shen, Exponential synchronization of stochastic neural networks with time-varying delays and Lévy noises via event-triggered control, Neural Process. Lett., 53 (2021), 2175–2196. https://doi.org/10.1007/s11063-021-10509-7 doi: 10.1007/s11063-021-10509-7
![]() |
[6] |
C. Fei, W. Y. Fei, X. R. Mao, L. T. Yan, Delay-dependent asymptotic stability of highly nonlinear stochastic differential delay equations driven by G-Brownian motion, J. Franklin Inst., 359 (2022), 4366–4392. https://doi.org/10.1016/j.jfranklin.2022.03.027 doi: 10.1016/j.jfranklin.2022.03.027
![]() |
[7] | Q. He, Y. Ren, Finite-time stabilisation issue for a class of highly nonlinear stochastic coupled systems, Int. J. Control, 2023. https://doi.org/10.1080/00207179.2023.2263580 |
[8] |
A. L. Wu, H. Yu, Z. G. Zeng, Variable-delay feedback control for stabilisation of highly nonlinear hybrid stochastic neural networks with time-varying delays, Int. J. Control, 97 (2024), 744–755. https://doi.org/10.1080/00207179.2023.2168878 doi: 10.1080/00207179.2023.2168878
![]() |
[9] | E. Fridman, Introduction to time-delay systems: analysis and control, Birkh¨auser: Cham, 2014. https://doi.org/10.1007/978-3-319-09393-2 |
[10] |
H. Huang, D. W. C. Ho, Y. Z. Qu, Robust stability of stochastic delayed additive neural networks with Markovian switching, Neural Netw., 20 (2007), 799–809. https://doi.org/10.1016/j.neunet.2007.07.003 doi: 10.1016/j.neunet.2007.07.003
![]() |
[11] |
L. C. Feng, J. D. Cao, L. Liu, Stability analysis in a class of Markov switched stochastic Hopfield neural networks, Neural Process. Lett., 50 (2019), 413–430. https://doi.org/10.1007/s11063-018-9912-7 doi: 10.1007/s11063-018-9912-7
![]() |
[12] |
C. D. Zheng, Q. H. Shan, H. G. Zhang, Z. S. Wang, On stabilization of stochastic Cohen-Grossberg neural networks with mode-dependent mixed time-delays and Markovian switching, IEEE Trans. Neural Networks Learn. Syst., 24 (2013), 800–811. https://doi.org/10.1109/TNNLS.2013.2244613 doi: 10.1109/TNNLS.2013.2244613
![]() |
[13] |
A. L. Wu, H. Yu, Z. G. Zeng, Stabilization of highly nonlinear hybrid neutral stochastic neural networks with time-varying delays by variable-delay feedback control, Syst. Control Lett., 172 (2023), 105434. https://doi.org/10.1016/j.sysconle.2022.105434 doi: 10.1016/j.sysconle.2022.105434
![]() |
[14] |
W. N. Zhou, J. Yang, X. Q. Yang, A. D. Dai, H. S. Liu, J. A. Fang, Almost surely exponential stability of neural networks with Lévy noise and Markovian switching, Neurocomputing, 145 (2014), 154–159. https://doi.org/10.1016/j.neucom.2014.05.048 doi: 10.1016/j.neucom.2014.05.048
![]() |
[15] |
Q. X. Zhu, Stability analysis of stochastic delay differential equations with Lévy noise, Syst. Control Lett., 118 (2018), 62–68. https://doi.org/10.1016/j.sysconle.2018.05.015 doi: 10.1016/j.sysconle.2018.05.015
![]() |
[16] |
J. Yang, W. N. Zhou, P. Shi, X. Q. Yang, X. H. Zhou, H. Y. Su, Adaptive synchronization of delayed Markovian switching neural networks with Lévy noise, Neurocomputing, 156 (2015), 231–238. https://doi.org/10.1016/j.neucom.2014.12.056 doi: 10.1016/j.neucom.2014.12.056
![]() |
[17] |
C. Imzegouan, Stability for Markovian switching stochastic neural networks with infinite delay driven by Lévy noise, Int. J. Dyn. Control, 7 (2019), 547–556. https://doi.org/10.1007/s40435-018-0451-x doi: 10.1007/s40435-018-0451-x
![]() |
[18] |
P. L. Yu, F. Q. Deng, P. Cheng, Stability analysis of hybrid stochastic delayed Cohen-Grossberg neural networks with Lévy noise and Markov switching, J. Franklin Inst., 359 (2022), 10831–10848. https://doi.org/10.1016/j.jfranklin.2022.05.025 doi: 10.1016/j.jfranklin.2022.05.025
![]() |
[19] |
H. L. Dong, J. Tang, X. R. Mao, Stabilization of highly nonlinear hybrid stochastic differential delay equations with Lévy noise by delay feedback control, SIAM J. Control Optim., 60 (2022), 3302–3325. https://doi.org/10.1137/22M1480392 doi: 10.1137/22M1480392
![]() |
[20] |
X. Y. Li, X. R. Mao, Stabilisation of highly nonlinear hybrid stochastic differential delay equations by delay feedback control, Automatica, 112 (2020), 108657. https://doi.org/10.1016/j.automatica.2019.108657 doi: 10.1016/j.automatica.2019.108657
![]() |
[21] |
Y. Y. Li, R. Dong, X. R. Mao, Discrete-time feedback control for highly nonlinear hybrid stochastic systems with non-differentiable delays, Syst. Control Lett., 175 (2023), 105507. https://doi.org/10.1016/j.sysconle.2023.105507 doi: 10.1016/j.sysconle.2023.105507
![]() |
[22] | D. Applebaum, Lévy processes and stochastic calculus, 2 Eds., Berlin: Cambridge University Press, 2009. Available from: http://www.cambridge.org/9780521738651. |
[23] | C. Zhao, Y. F. Song, Q. X. Zhu, K. B. Shi, Input-to-state stability analysis for stochastic mixed time-delayed neural networks with hybrid impulses, Math. Probl. Eng., 2022, 6135390. https://doi.org/10.1155/2022/6135390 |
[24] |
K. K. Wang, L. Ju, Y. J. Wang, S. H. Li, Impact of colored cross-correlated non-Gaussian and Gaussian noises on stochastic resonance and stochastic stability for a metapopulation system driven by a multiplicative signal, Chaos Soliton. Fract., 108 (2018), 166–181. https://doi.org/10.1016/j.chaos.2018.02.004 doi: 10.1016/j.chaos.2018.02.004
![]() |
[25] |
K. K. Wang, Y. J. Wang, S. H. Li, J. C. Wu, Double time-delays induced stochastic dynamical characteristics for a metapopulation system subjected to the associated noises and a multiplicative periodic signal, Chaos Soliton. Fract., 104 (2017), 400–417. https://doi.org/10.1016/j.chaos.2017.08.030 doi: 10.1016/j.chaos.2017.08.030
![]() |