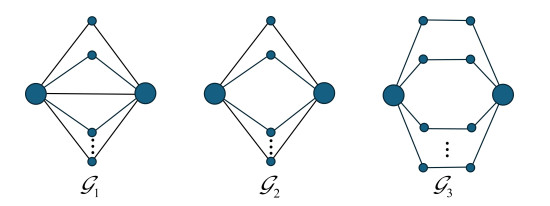
Diffusion is a ubiquitous process in real-world syetems. In many complex systems, ranging from neuronal networks to traffic in cities, diffusion is nonconservative (NC) in the sense that diffusive particles can be created/annihilated at the entities of the system. Here, we consider the important problem of identifying potential navigational bottlenecks in NC diffusion occurring in the networks representing skeletons of complex systems. We develop a first-principles approach based on an NC diffusion using the Lerman-Ghosh Laplacian on graphs. By solving analytically this NC diffusion equation at two different times, we get an index which characterizes the capacity of every vertex in a network to spread the diffusive particles across the network in a short time. Vertices having such capacity diminished are potential navigational bottlenecks in this kind of dynamics. We solve analytically the situations in which the vertices with the highest degree (hubs) are at different distances in the network, allowing us to understand the structural significance of the index. Using algebraic methods, we derive a Euclidean distance between vertices in the context of NC diffusion with potential navigational bottlenecks. We then apply these indices to study several real-world networks. First, we confronted our theoretical results with experimental data about traffic congestion in a city. Then, we illustrated the application of the new methodologies to the study of a neuronal system, an air transportation network and two urban street networks.
Citation: Giovanni G. Soares, Ernesto Estrada. Navigational bottlenecks in nonconservative diffusion dynamics on networks[J]. AIMS Mathematics, 2024, 9(9): 24297-24325. doi: 10.3934/math.20241182
[1] | Jesús Gómez-Gardeñes, Ernesto Estrada . Network bipartitioning in the anti-communicability Euclidean space. AIMS Mathematics, 2021, 6(2): 1153-1174. doi: 10.3934/math.2021070 |
[2] | Ali Raza, Mobeen Munir, Tasawar Abbas, Sayed M Eldin, Ilyas Khan . Spectrum of prism graph and relation with network related quantities. AIMS Mathematics, 2023, 8(2): 2634-2647. doi: 10.3934/math.2023137 |
[3] | Pablo Fernández-López, Patricio García Báez, Ylermi Cabrera-León, Aleš Procházka, Carmen Paz Suárez-Araujo . Modeling the implications of nitric oxide dynamics on information transmission: An automata networks approach. AIMS Mathematics, 2023, 8(12): 30142-30181. doi: 10.3934/math.20231541 |
[4] | Shumin Zhang, Tianxia Jia, Minhui Li . Partial domination of network modelling. AIMS Mathematics, 2023, 8(10): 24225-24232. doi: 10.3934/math.20231235 |
[5] | Yanxin Li, Shangkun Liu, Jia Li, Weimin Zheng . Congestion tracking control of multi-bottleneck TCP networks with input-saturation and dead-zone. AIMS Mathematics, 2024, 9(5): 10935-10954. doi: 10.3934/math.2024535 |
[6] | Ling Zhou, Zuhan Liu . Blow-up in a $ p $-Laplacian mutualistic model based on graphs. AIMS Mathematics, 2023, 8(12): 28210-28218. doi: 10.3934/math.20231444 |
[7] | Muhammad Ahmad, Muhammad Faheem, Sanaa A. Bajri, Zohaib Zahid, Muhammad Javaid, Hamiden Abd El-Wahed Khalifa . Optimizing SNARK networks via double metric dimension. AIMS Mathematics, 2024, 9(8): 22091-22111. doi: 10.3934/math.20241074 |
[8] | Yike Wang, Gaoxia Wang, Ximei Hou, Fan Yang . Motif adjacency matrix and spectral clustering of directed weighted networks. AIMS Mathematics, 2023, 8(6): 13797-13814. doi: 10.3934/math.2023706 |
[9] | Tengda Wei, Xiang Xie, Xiaodi Li . Input-to-state stability of delayed reaction-diffusion neural networks with multiple impulses. AIMS Mathematics, 2021, 6(6): 5786-5800. doi: 10.3934/math.2021342 |
[10] | Fan Yang, Xiaohui Ai . Exponential stability of stochastic complex networks with multi-weights driven by second-order process based on graph theory. AIMS Mathematics, 2024, 9(4): 9847-9866. doi: 10.3934/math.2024482 |
Diffusion is a ubiquitous process in real-world syetems. In many complex systems, ranging from neuronal networks to traffic in cities, diffusion is nonconservative (NC) in the sense that diffusive particles can be created/annihilated at the entities of the system. Here, we consider the important problem of identifying potential navigational bottlenecks in NC diffusion occurring in the networks representing skeletons of complex systems. We develop a first-principles approach based on an NC diffusion using the Lerman-Ghosh Laplacian on graphs. By solving analytically this NC diffusion equation at two different times, we get an index which characterizes the capacity of every vertex in a network to spread the diffusive particles across the network in a short time. Vertices having such capacity diminished are potential navigational bottlenecks in this kind of dynamics. We solve analytically the situations in which the vertices with the highest degree (hubs) are at different distances in the network, allowing us to understand the structural significance of the index. Using algebraic methods, we derive a Euclidean distance between vertices in the context of NC diffusion with potential navigational bottlenecks. We then apply these indices to study several real-world networks. First, we confronted our theoretical results with experimental data about traffic congestion in a city. Then, we illustrated the application of the new methodologies to the study of a neuronal system, an air transportation network and two urban street networks.
Networks [1] represent the skeletons of complex systems [2], which facilitate the spread of "information" between the entities of the systems (vertices of the network) using their pairwise connections (edges) [3]. Here, "information" is used generically to account for any item-from electrons in a molecule to people in a city-moving across a network [4]. Therefore, one of the most fundamental problems in the investigation of complex networks is to understand the mechanisms behind the transmission of information across them. In a connected network, there is always a shortest path between any pair of vertices. These are paths of the minimum lengths in terms of the number of edges connecting the pairs of vertices [5,6,7]. There is a misunderstanding in considering that information flows in a network via shortest paths, even in systems with billions of entities like the human brain [8]. As clearly remarked by Goñi et al. [9] "a routing/navigation process implies that communication flows from a specific source to a specific target along the fastest or most direct route, which implies a global knowledge about the network topology". For most of complex systems, the sender of information is unaware of the global structure of the network used to transmit the information, which excludes the possibility that it uses the shortest path [10,11,12,13,14,15,16]. Diffusion is a navigational process which seems to be ubiquitous in nature, implying that [9] "communication occurs in the absence of specific targets, or that, even if targets are specified, a lack of knowledge about global network topology prevents particles or messages from taking the shortest paths".
Diffusion has been widely studied in the context of complex networks [17,18,19] as well as in engineering under the term "consensus dynamics" [20,21,22]. A characteristic signature of diffusion in graphs is its conservative nature. A dynamical process on a graph is mediated by the interactions between nodes [1,3]. It is said to be conservative if the total amount of diffusive material is constant on the graph at any time [23]. This type of process excludes those in which (ⅰ) some information is dissipated to or taken from outside the graph, (ⅱ) part of the information is annihilated/created at the vertices of the graph. In these cases, the amount of diffusive material on the graph does not remain constant in time, and these are known as nonconservative (NC) processes. There are several important examples of NC diffusive processes in complex systems [24,25,26,27,28,29,30]. Traffic in a city is frequently studied by assuming that it flows via the shortest paths between origin-destination pairs [31,32,33,34], or by considering a conservative diffusion model [35,36,37]. However, the number of cars flowing through the streets (edges) of an urban street network is not necessarily the same at slightly different times, making the process NC in its nature. The reason is that some cars may "disappear"/"appear" in the street leg between two intersections due to the fact that they may park or emerge from parking in such street leg, known as "mid-link sink and sources" in traffic literature [38,39]. Another example frequently considered from the perspective of shortest paths and conservative diffusion is the flow of matter and energy in a food web. In this casethe nodes represent species and the directed edges represent their trophic relations (who eats who), which are the pathways over which energy and matter can flow. When one species A (predator) predates another species B (prey), A utilizes only a portion of the material and free energy originally in B, which is then retained in the predator, giving rise to a non-conservative process. Such mass and energy can then be diffused across the food web in an NC diffusive way [40]. Another non-conservative scenario is the chemical synapses in neuronal systems where apart fromthe wiring intercellular communication, which is expected to be conservative, there is also communication in the form of a volume transmission (VT), which uses the extracellular fluid filling channels of the extracellular space and the cerebrospinal fluid filling ventricular space and sub-arachnoidal space (Figure 1(e)) [26,41,42,43,44,45,46]. More examples of NC diffusion include communication in social media like X (formerly known as Twitter) [27,28,29], where a user can post a message that can be read by her followers, but also (if not constrained by the user) by non-followers, all of whom can retweet such information to others. Even the transmission of packages of information via the Internet may be an NC diffusion process due to the existence of long queues at given servers, which may result in some packages being discarded, making the transmission process NC on the graph.*
*We remark that the process is always conservative in the physical universe, but we are referring to the case of being conservative or not on the discrete space defined by the graph.
Here, we consider navigational processes occurring in complex systems that can be modeled by NC diffusive dynamics. We then focus on understanding which are the network characteristics that may burden the navigability in this framework. Let us consider an NC diffusion dynamics reaching a steady state at a given time tc. Then, if we start the diffusive process by concentrating all the diffusive particles at a vertex i, we can obtain tc,i. Similarly, we can initialize the process at vertex j and obtain tc,j. Obviously, if tc,j>tc,i it means that it is more difficult to reach the steady state of the diffusion when starting at vertex j than when starting at vertex i. If we do this for all vertices in the network, we can obtain those which are the ones producing the biggest delay in reaching the steady state. We will call these vertices "navigational bottlenecks" for obvious reasons. The identification of network bottlenecks is a foundational work for improving network traffic conditions and preventing traffic congestion [47,48,49,50,51,52,53,54,55]. However, we should stress an important problem with dealing with this matter before we can proceed. In a real-world situation, the existence of a bottleneck does not only depend on the topology of the network supporting the dynamics but also depends on the traffic allocated to the given vertices. For instance, a vertex that is identified as a navigational bottleneck in a network does not necessarily behave as a bottleneck if the traffic allocated to it is relatively small. Therefore, what we propose in this work is the identification of those vertices that have a large propensity to become navigational bottlenecks if the amount of traffic allocated to them is significantly large. Developing the previously mentioned program for identifying navigational bottlenecks implies the realization of n simulations of the NC diffusion on a network of n vertices, i.e., one simulation starting the process at each vertex. Therefore, what we propose here is to solve the problem analytically, such that we can define an index characterizing the propensity of every vertex to become a navigational bottleneck without the necessity of performing any simulation of the dynamics.
Let G=(V,E) be a simple, connected graph and let A be its adjacency matrix. The degree of the vertex i, ki, is the number of edges connected to that vertex in the graph. Let A=UΛUT be the spectral decomposition of the adjacency matrix, such that Λ is the diagonal matrix of eigenvalues of A and U is the orthogonal matrix of eigenvectors. In general, we designate the spectrum of a matrix M, Spec(M) to be the set of eigenvalues of this matrix together with their multiplicities, which are represented as superscripts in parentheses. In the case of the matrix A of a connected undirected graph: Spec(M)={λ1,λ(m2)2,⋯,λ(m2)n}, such that λ1>λ2≥⋯≥λn are the eigenvalues of A. Let ψj be the eigenvector associated with the eigenvalue λj. We will designate by ψj,i the ith entry of ψj. The entry ψ1,i is known as the eigenvector centrality of the vertex i, ECi [56,57,58]. The standard, conservative diffusion process on a graph represents the change of the "concentration" Ci of items at the vertices of the graph with the pass of time, which is defined by [17]:
˙C(t)=−LC(t), | (2.1) |
with initial condition C(0)=C0. In this equation, ˙C(t)=[˙C1(t),…,˙Cn(t)]Twhere ˙C(t)=dC(t)dt, C(t)=[C1(t),…,Cn(t)]T and L=K−A is the graph Laplacian, with K being the diagonal matrix of vertex degrees. The solution of the Cauchy problem (2.1) is given by C(t)=e−tLC0, where e−tL is the matrix exponential function of the graph Laplacian. Matrix functions of the adjacency matrix, which will frequently appear in this work, are defined by: G:=exp(A). The term Gi,j for i≠j is known as the communicability between the two vertices, while the term Gii is known as the subgraph centrality of the vertex i. The term EE(G):=Trexp(A) is known as the Estrada index of the graph, where Tr is the trace of the corresponding matrix.
The squared communicability distance between two vertices in a graph is defined as [59] (see also [60,61,62]):
ξ2vw:=Gvv+Gww−2Gvw, | (2.2) |
which has been proved to be a Euclidean and spherical distance between the corresponding vertices in the graph.
The nonconservative (NC) diffusion process on a graph has been previously studied. In this case, the change of concentration of items at the vertices of the graph with time is described by the equation:
˙C(t)=−LχC(t), | (2.3) |
where Lχ:=χI−A is the Lerman-Ghosh Laplacian of the graph [64] (see also [65]), and the process has initial condition C(0)=C0.
We start by proving the following result.
Theorem 1. Let G be a graph in which the NC diffusion (2.3) takes place. Then,
limt→∞C(t)={(ψT1C0)ψ1et(λ1−χ)=∞forχ<λ1(∑jC0jψ1(j))ψ1forχ=λ1(ψT1C0)ψ1e−t(χ−λ1)=0forχ>λ1. | (3.1) |
Proof. The solution of the diffusion equation is given by
C(t)=e−t(χI−A)C0, | (3.2) |
which can be written as
C(t)=et(λ1−χ)(ψT1C0)ψ1+et(λ2−χ)(ψT2C0)ψ2+⋯+et(λn−χ)(ψTnC0)ψn. | (3.3) |
Then, when χ<λ1 we have
limt→∞C(t)=et(λ1−χ)(ψT1C0)ψ1, | (3.4) |
which diverges as t→∞.
If χ=λ1 we have that the first term of Eq (3.3) is zero, and the rest are negative, such that
limt→∞C(t)=(ψT1C0)ψ1, | (3.5) |
which indicates that the solution is proportional to the entries of the eigenvector ψ1 associated with the spectral radius λ1 of A. This eigenvector was introduced by Bonacich [56,57,58] as a centrality index of the vertices in a graph and it is nowadays known as the eigenvector centrality. Therefore, the current framework provides a dynamics interpretation of this centrality index in terms of the concentration reached by a vertex at the steady state of a non-conservative diffusion controlled by the Lerman-Ghosh Laplacian matrix [64] when χ=λ1. Because the second smallest eigenvalue of (λ1I−A) is λ1−λ2, it determines the rate of convergence of the diffusive process. Notice that if C0=ψ1, then the diffusion process is conservative because:
1TC(t)=[1Tψ1]=1TC0. | (3.6) |
Finally, if χ>λ1 then,
limt→∞C(t)=e−t(χ−λ1)(ψT1C0)ψ1, | (3.7) |
which goes to zero as t→∞.
Therefore, the only NC diffusion process that does not diverge is when χ=λ1. Let us then consider such a process on G. Let us then consider the concentration of items at a given vertex i∈V when C0i=1 and C0j=0 for all j≠i, when t≪∞, which is given by
Ci(t)=(e−t(λ1I−A)C0)i=e−tλ1(etA)ii. | (3.8) |
Let us now consider such concentration at an infinitely large time. That is the following:
limt→∞Ci(t)=limt→∞e−tλ1(etA)ii=e−tλ1((ψ1etλ1ψT1)C0)i=((∑jC0jψ1,i)ψ1)i=ψ21,i, | (3.9) |
where ψ21,i is the square of ith entry of the eigenvector associated with λ1. Notice that ψ1,i is the eigenvector centrality of i, ECi, such that limt→∞Ci(t)=EC2i when C0i=1 and C0j=0 for all j≠i.
Remark 2. Notice that when C0i=1 and C0j=0 for all j≠i, the concentration at any node j≠i when the steady state is reached is given by
limt→∞Cj(t)=((∑jC0jψ1,i)ψ1)j=ψ1,iψ1,j. | (3.10) |
Let us then consider the following.
Definition 3. Let G be a graph in which the NC diffusion (2.3) takes place with initial conditions given by C0i=1 and C0j=0 for all j≠i. Let χ=λ1. Then, the difference Ci(t=1)−limt→∞Ci(t) accounts for how fast or slow a vertex spreads the concentration of items across the graph when all the initial concentration is at this vertex:
Ci(t=1)−limt→∞Ci(t)=e−λ1(eA)ii−EC2i=(eA)ii−EC2ieλ1eλ1=SCi−EC2ieλ1eλ1, | (3.11) |
where SCi is the subgraph centrality of the vertex i [66]. Then, because the denominator is just a normalization factor we will consider here the index
Xi:=SCi−EC2ieλ1. | (3.12) |
A small value of Xi indicates that the vertex i has a large capacity to deliver items from it to reach the steady-state concentration corresponding to an NC process taking place on the graph. On the other hand, a large value of this index indicates that it takes a long time for this vertex to reach such steady-state concentration. Therefore, the last vertex is a potential bottleneck in the diffusive navigational processes taking place on the graph. Therefore, we will call Xi the navigational bottleneck index (NBI) of the vertex i. Notice that if comparisons between vertices in different graphs are intended, then the normalization by exp(λ1) is necessary.
To gain insights about the role of graph structure in the values of Xi, we have designed the three toy graphs illustrated in Figure 3.1. The first graph, also known as an agave graph, represents two large degree vertices (hubs) that are connected to each other as well as through a series of vertices of degree two. The multiple dots indicate that the number of these vertices of degree two is arbitrary. The second graph is the same as the previous one, but in this case, the two hubs are not interconnected. The communication between the two hubs in this graph occurs only via the vertices of degree two. The third graph consists of two hubs that are not directly connected to each other but connected through paths of length three. In closing, this example has a pair of hubs communicated by a peripheral set of vertices of degree two. The difference among them resides in the fact that in the three graphs the "effective separation" between the two hubs increases from G1 to G3.
We now find the values of Xi for the two types of vertices in the graphs in Figure 3.1 analytically.
Lemma 4. Let G1 be the graph illustrated in Figure 3.1 on n vertices where v is any of the two vertices of degree n−1 (hubs) and w is any of the vertices of degree two. Let α=√8n−15. Then,
Xv(G1)=14e(1/2)(1−α)(1−α−1)+12e, | (3.13) |
and
Xw(G1)=n−3n−2+(α+1)e1/2(1−α)2α(n−2). | (3.14) |
Proof. The eigenvalues of the adjacency matrix of the agave graph G1 are:
Spec(A(G1))={12(1+α),0n−3,−1,12(1−α)}, | (3.15) |
and the entries of the eigenvector associated with λj for the vertex v are: ψ21,v=14(1+α−1), ψ22≤j≤n−2,v=0, ψ2n−1,v=12, ψ2n,v=α−14α. Therefore,
(eA(G1))vv=e3/2(1αsinh(α2))+cosh(α2)+12e. | (3.16) |
Because, Xv(G1)=(eA(G1))vv−14(1+α−1)e1/2(1+α) we have
Xv(G1)=12e+α−14αe1/2(1−α), | (3.17) |
which proves the first part of the result.
Then,
(eA(G1))ww=EE(G1)−2(eA(G1))vvn−2, | (3.18) |
where the Estrada index of an agave graph on n vertices EE(G1) is:
EE(G1)=Tr[eA(G1)]=e1/2(1+α)+e1/2(1−α)+e−1+n−3=2√ecosh(α2)+1e+n−3. | (3.19) |
Then, after substitution and arrangements, we have
(eA(G1))ww=n−3n−2+(α−1)e1/2(1+α)2α(n−2)+(α+1)e1/2(1−α)2α(n−2). | (3.20) |
Finally, we have that Xw(G1)=(eA(G1))ww−ψ21,weλ1=(eA(G1))ww−4α(α+1)e1/2(1+α), so that we obtain the final result.
Lemma 5. Let G2 be the graph illustrated in Figure 3.1 on n≥5 vertices where v is any of the two vertices of degree n−1 (hubs) and w is any of the vertices of degree two. Let β=√2(n−2). Then,
Xv(G2)=14e−β+12, | (3.21) |
and
Xw(G2)=e−ββ2+n−3n−2. | (3.22) |
Proof. The graph G2 is a complete bipartite graph K2,n−2, so the eigenvalues of its adjacency matrix are:
Spec(A(G2))={β,0n−2,−β}, | (3.23) |
and ψ1,v=12 such that
(eA(G2))vv=14eβ+14e−β+∑2≤j≤n−1ψ2j. | (3.24) |
Because ∑nj=1ψ21,j=1 we get ∑2≤j≤n−1ψ2j=12, such that
(eA(G2))vv=14(eβ+e−β)+12 | (3.25) |
and
Xvv(G2)=14e−β+12. | (3.26) |
For obtaining (eA)ww we use again the trace of exp(A), such that
Tr(eA(G2))=eβ+e−β+n−2 | (3.27) |
and
(eA(G2))ww=Tr(eA(G2))−2(eA(G2))vvn−2=cosh(β)+n−3n−2, | (3.28) |
such that
Xww(G2)=e−ββ2+n−3n−2. | (3.29) |
Lemma 6. Let G3 be the graph illustrated in Figure 3.1 on n≥8 vertices where v is any of the two vertices of degree n−1 (hubs) and w is any of the vertices of degree two. Let γ=√2(n−3). Then,
Xv(G3)=eγ(γ+1)+e(γ+1)+τ−14γe−1/2(γ+1), | (3.30) |
and
Xw(G3)=eγ(γ−1)+e(γ−1)+γ+12γ(n−2)e−1/2(γ+1)+1+e22e(n−4)(n−2). | (3.31) |
Proof. The eigenvalues of the adjacency matrix of the agave graph G3 are (notice that n is always even in this graph):
Spec(A(G3))={γ+12,γ−12,1n2−2,−1n2−2,1−γ2,−γ−12}, | (3.32) |
and ψ21,v=14(1−1γ), ψ21,w=γ+12(n−2)γ, ψ2n−1,v=14(1+1γ), ψ2n−1,w=γ−12(n−2)τ. Therefore,
(eA(G3))vv=cosh(12)cosh(γ2)−sinh(12)γsinh(γ2). | (3.33) |
We can also write
(eA(G3))ww=Tr(eA(G3))−2(eA)vvn−2, | (3.34) |
such that
(eA(G3))ww=2cosh(12)cosh(γ2)n−2+2sinh(12)sinh(γ2)(n−2)γ+1+e22e(n−4)(n−2). | (3.35) |
We can now obtain the values of Xv(G3) and Xw(G3) by subtracting ψ21,veλ1 and ψ21,weλ1 from the expressions of (eA(G3))vv and (eA(G3))ww, respectively, which proves the result.
To illustrate the results obtained in the previous three Lemmas we calculate here the values of the X index for the two non-equivalent vertices in the three classes of graphs illustrated in Figure 3.1. We select n=10 in this example. In the case of the graph G1 it is easy to see that limn→∞Xv(G1)=12e and limn→∞Xw(G1)=1. The results given in Table 1 give Xv(G1)≈0.19 and Xw(G1)≈0.88, which are close to the limit values. However, the most interesting thing here is that, as predicted, the vertex w, which is the one of degree two, is the one having the largest capacity to create a navigational bottleneck in the graph. This is corroborated by the fact that time tc(w) needed to reach the steady state, i.e., (ui(t)−uj(t))≤10−4 for all i,j∈V, when the initial concentration is located at the vertex w is bigger than that when the concentration is located at the vertex v, tc(v). That is, reaching the steady state of a nonconservative diffusion in G1 is harder if the initial concentration is located at the vertex w than at the vertex v, exactly as predicted by the index X. The situation is similar for the graph G2 where limn→∞Xv(G2)=12 and limn→∞Xw(G2)=1. Here again tc(w)>tc(v) indicating that the vertices of degree two are potential navigational bottlenecks relative to vertex w (see Table 1). The graph G3 is, however, an example where the hubs are the potential navigational bottlenecks for a nonconservative diffusion. In this case Xv(G3)>Xw(G3), indicating that the vertex with the largest degree inhibits the spread of concentration across the vertices of the graph more than the vertex w. In fact, we can observe that indeed tc(v)>tc(w), confirming that this hub as the navigational bottleneck of the graph.
Xv | Xw | tc(v) | tc(w) | |
G1 | 0.190 | 0.877 | 172 | 286 |
G2 | 0.505 | 0.877 | 232 | 246 |
G3 | 1.560 | 1.399 | 988 | 884 |
The previous example teaches us that such generalizations as "the hubs facilitate/inhibit" the dynamical spread of "information" across a network are not necessarily correct and they depend on the topological position of the hubs in the graph. For instance, when the hubs are directly connected or very close to each other, like in the cases of graphs G1 and G2, they have the capacity of spreading a lot of information between each other, avoiding the creation of a potential navigational bottleneck at them. However, if the hubs are not close together but separated by relatively large paths, like in the case of graph G3, they lose this capacity of alleviating the traffic by sending information among them. In this case they become navigational bottlenecks, inhibiting the spread of information between vertices in the graph.
Definition 7. Let ˜Λ be the (n−1)×(n−1) diagonal matrix obtained by removing the row and column of Λ corresponding to λ1 and let ˜U be the (n)×(n−1) matrix resulting from removing the column of U corresponding to ψ1. Let us define the matrix ˜G=˜Ue˜Λ˜UT.
Lemma 8. The matrix ˜G is positive semidefinite.
Proof. Let z be a nonzero column vector of length n. Then,
zT˜Gz=zT˜Ue˜Λ˜UTz=n∑j=2eλj(n∑u=1zuψju)2≥0, | (4.1) |
where λj is an eigenvalue of A and ψju is the uth entry of the eigenvector corresponding to λj. The term in parentheses is zero if and only if z=ψ1, where ψ1 is the eigenvector associated with the spectral radius of A. Because the eigenvectors are orthogonalized it happens that ψT1ψj=0 for all j≥2, which proves the result.
Remark 9. It is easy to realize that ˜Guu=Xu. That is,
(˜Ue˜Λ˜UT)uu=n∑j=2ψ2j,ueλj=n∑j=1ψ2j,ueλj−ψ21,ueλ1:=Xu. | (4.2) |
Also notice that ˜Ue˜Λ˜UT≠e˜U˜Λ˜UT=e˜A, where ˜A:=˜U˜Λ˜UT.
Let us now interpret the term ˜Guv. As we have seen in Remark 2, limt→∞Cv(t)=ψ1,uψ1,v, which is the amount of items diffused to vertex v when the initial concentration is completely localized at vertex u in the NC diffusion that we are considering in this work. If we obtain the concentration Cv(t=1) under the same conditions, we obtain:
Cv(t=1)=e−λ1eAC0=e−λ1(eA)uv=e−λ1Guv, | (4.3) |
where Guv is the communicability function [67,68] between the two vertices. Therefore,
Cv(t=1)−limt→∞Cv(t)=e−λ1Guv−ψ1,uψ1,v=Guv−eλ1ψ1,uψ1,veλ1=˜Guveλ1, | (4.4) |
which means that ˜Guv is proportional to the amount of items diffused from u to v at an early time of the process minus the amount that arrives at v at the steady state. In other words, it is a measure of the rate of transfer from u to v when the whole initial concentration is at the first vertex. The largest ˜Guv indicates a larger capacity for delivering items from the two vertices (due to the non-directionality of the graphs) as the NC diffusion evolves. We now have the following.
Definition 10. Let ˜G be defined as before, and let us define
Yuv:=˜Guu+˜Gvv−2˜Guv. | (4.5) |
Notice that while ˜Guu and ˜Gvv give the capacity of the vertices to create navigational bottlenecks, the term ˜Guv is a measure of how good transmission exists between the two vertices. Thus, a large value of Yuv indicates that items can easily get trapped at the vertices u and v because both vertices are potential navigational bottlenecks and they do not have a large capacity of diffusing the items among them. A relatively low value of this measure indicates a good capacity for delivering the items between the two vertices at an early time of the NC process, such that there would not be a bottleneck among them. We now prove the following result.
Lemma 11. Yuv is a squared Euclidean distance between the vertices u and v of G.
Proof. Let us write
Yuv=n∑j=2ψ2j,ueλj+n∑j=2ψ2j,veλj−2n∑j=2ψj,uψj,veλj=n∑j=2(ψj,u−ψj,v)2eλj, | (4.6) |
which proves that Yuv≥0 as required for a distance.
Let us now define ˜φu:=[ψ2,v,…,ψn,v]T, such that we can write
Yuv=(˜φu−˜φv)Te˜Λ(˜φu−˜φv). | (4.7) |
Then, because e˜Λ is positive definite we can find its square root and express:
Yuv=(e˜Λ/2˜φu−e˜Λ/2˜φv)T(e˜Λ/2˜φu−e˜Λ/2˜φv). | (4.8) |
Finally, by defining ˜xu:=e˜Λ/2˜φu we have
Yuv=(˜xu−˜xv)T(˜xu−˜xv)=‖˜xu−˜xv‖2. | (4.9) |
Theorem 12. Let G1 be the graph described in Figure 3.1 on n vertices in which the vertices v and v′ are the two ones with degree n−1 and the vertices u and u′ are any two of the vertices of degree two. Then,
Yv,v′(G1)=2e, | (4.10) |
Yu,u′(G1)=2, | (4.11) |
Yu,v(G1)=n−3n−2+12e+(α2+n(α−1)+3)e1/2(1−α)4α(n−2). | (4.12) |
Proof. We have proved before that the eigenvalues of A(G1) are:
Spec(A(G1))={12(1+α),0(n−3),−1,12(1−α)} | (4.13) |
with α:=√8n−15. Let φi=[ψ1,i,ψ2,i,…,ψn,i]T. Then,
φv=[12√α+1α0n−3±2−1/2−12√α−1α], φu=[2√1α(α+1)ϱn−30−2√1α(α+1)], | (4.14) |
where 0n−3 is a column of zeros of length n−3 and ϱn−3 is a vector of length n−3 giving a different value for the different u vertices of An. By using this information it is straightforward to obtain:
˜Gvv′(G1)=α−14αe1/2(1−α)−12e, | (4.15) |
˜Guu′(G1)=n−3n−2+(α+1)e1/2(1−α)2α(n−2), | (4.16) |
˜Guv(G1)=−e1/2(1−α)α. | (4.17) |
We then obtain Yvv′(G1)=2˜Gvv(G1)−2˜Gvv′(G1), Yuu′(G1)=2˜Guu(G1)−2˜Guu(G1), and Yuv(G1)=˜Guu(G1)+˜Gvv(G1)−2˜Guv(G1), by substitution and further algebraic simplification to prove the result.
Theorem 13. Let G2 be the graph described in Figure 3.1 on n vertices in which the vertices v and v′ are the two ones with degree n−1 and the vertices u and u′ are any two of the vertices of degree two. Then,
Yv,v′(G2)=2, | (4.18) |
Yw,w′(G2)=2, | (4.19) |
Yv,w(G2)=(β+2)24β2e−β+12+n−3n−2. | (4.20) |
Proof. We start by finding Yv,v′(G2)=2˜Gvv(G2)−2˜Gvv′(G2) for which we have to find the second term. That is,
˜Gvv′(G2)=n∑j=2ψj,vψj,v′eλj=14e−β−∑2≤j≤n−1ψ2j,v=14e−β−12. | (4.21) |
Therefore, Yv,v′(G2)=2(14e−β+12)−2(14e−β−12), which proves this part of the result.
Similarly, for Yw,w′(G2)=2˜Gww(G2)−2˜Gww′(G2) we find
˜Gww′(G2)=e−ββ2+∑2≤j≤n−1ψj,wψj,w′, | (4.22) |
which by considering that ∑2≤j≤n−1ψj,wψj,w′=−2ψ21,w give ˜Gww′(G2)=e−ββ2−1n−2. Therefore, Yw,w′(G2)=e−βn−2+2n−3n−2−e−βn−2+2n−2, which proves this part of the result.
Finally, we have Yv,w(G2)=˜Gvv(G2)+˜Gww(G2)−2˜Gvw(G2) for which we find first
˜Gvw(G2)=−e−β2β, | (4.23) |
such that Yv,w(G2)=e−β4+12+e−ββ2+n−3n−2+2e−β2β, which, after simplifications, reduces to the final result.
The most important consequence of the previous two results is the fact that the distance between the two hubs in G1 and G2 is constant and independent of the size of the graphs. That is, Yv,v′(G1)=2e≈0.7358 and Yv,v′(G2)=2, which indicates that in G1, where the hubs are connected, the communication is very good and the Y-distance is smaller than the shortest path between the two hubs. In the case of G2, where the two hubs are separated at distance two, the communication between them is still good, but in this case the Y-distance is identical to the shortest path. To further investigate this phenomenon, we prove the following result for Yv,v′(G3). In this graph, there are six different distances between pairs of vertices, so we will focus only on the Y-distance between the two hubs.
Theorem 14. Let G3 be the graph described in Figure 3.1 on n vertices in which the vertices v and v′ are the two ones with degree n−1 and the vertices u and u′ are any two of the vertices of degree two. Then,
Yv,v′(G3)=2√eγ(sinh(γ2)+τcosh(γ2)), | (4.24) |
where γ=√2n−3.
Proof. The Y-distance between the two hubs in G3 is Yv,v′(G3)=2Xv,v(G3)−2Xv,v′(G3). Thus, we have to obtain Xv,v′(G3) as:
Xv,v′(G3)=14(1+1γ)e−1/2(γ−1)−14(1+1γ)e1/2(γ−1)−14(1−1γ)e−1/2(γ+1). | (4.25) |
Then, by using Xv(G3)=eγ(γ+1)+e(γ+1)+τ−14γe−1/2(γ+1) from Lemma 6, after some algebraic work, we obtain the result.
Remark 15. The previous results help us to interpret the differences in the propensities to create navigational bottlenecks of vertices in the graphs G1, G2 and G3. Let us consider n=10 for an example. In this case Yv,v′(G1)≈0.7359, Yv,v′(G2)=2, and Yv,v′(G3)≈5.9807. Notice that the shortest path distances between these two vertices are one, two, and three, respectively. However, while the Y-distance between the two hubs remains constant in G1, and G2, it grows very quickly with size in G3. This indicates that if we allocate a diffusive particle at the vertex v in G1 or G2, in a situation where the graph is susceptible to jamming due to its capacity, this vertex still has a large capacity for spreading this particle to the other hub, v′, avoiding the creation of a navigational bottleneck at v. This is the reason why in G1, and G2, the propensity to be a navigational bottleneck is higher in vertex w than in vertex v. However, when we consider the graph G3, the large value of Yv,v′(G3) indicates that the capacity of vertex v to spread a diffusive particle to the other hub is very much diminished. Therefore, the hubs have a larger propensity to be navigational bottlenecks in G3 than in G1 or G2, and consequently, as we have proven analytically, it has a higher propensity to be a bottleneck than the vertex w.
Every time a new index characterizing a topological property of networks or their parts is introduced, it is desirable to confront it with real-world situations that help us understand how to use it in an appropriate way. In this subsection, we confront the index X with the problem of traffic congestion in a real-world network. Unfortunately, there is no experimental data about congestion in networks apart from those related to the problem of urban traffic in cities. This particular problem is very complex, and many approaches exist in the literature to tackle it [47,48,49,50,51,52,53,54,55]. In one of these papers, Samani et al. [69] have combined data-driven approaches with mathematical modeling to estimate the non-congestion probability of every street in the city of Sioux Falls in South Dakota, USA. The network is formed by 24 street intersections which form the vertices of the graph and 38 streets forming its edges. The authors also provide the origin-destination travel demand Dij and calculated the non-congestion probability Pij for every street connecting intersections i and j. The representation of the network can be seen in Figure 5.1.
Our current approach is based on the propensity of intersections, more than streets, to get congested if we allocate a significant amount of diffusive particles to them. Therefore, we start by converting the data produced by Samani et al. [69] into information reliable to be compared with the X index. First, we compute the global demand Di at intersection i as: Di=∑jDij. We also obtained the average probability of observing congestion at the streets intersecting at i as Pi=1k∑kj=1Pij. We then consider that an intersection has a low probability of congestion if Pi<0.333 and it has a high probability of congestion otherwise. We found that nine intersections are classified as low congestion, with an average probability equal to 0.125, with only two values over 0.2. On the other hand, there are 15 intersections of high congestion in this city. The average probability of of these 15 intersections is 0.567, of which there are five intersections with a probability higher than 0.5.
In Figure 5.2, we plot the values of the global demand of every intersection versus their X indices. Every intersection is then drawn in the x,y-plane as a point with size and color proportional to its average congestion probability. A linear discriminant analysis (LDA) clearly separates the two groups of intersections, leaving only four ones incorrectly classified, i.e., a classification accuracy of 83.3%. As can be seen in Figure 5.2, the intersections with relatively low values of X display a low probability of congestion particularly when their traffic demand is also low. On the other hand, the intersections with relatively large values of X tend to have a larger congestion probability, particularly if they have a relatively large traffic demand.
The important message of this exercise is that the X index identifies the vertices in a graph that have a large propensity to get congested if (and this is a very important if) the amount of diffusive particles allocated at that vertex is significantly large. Therefore, in a real-world situation, like the study of urban traffic congestion, this index should be used accompanied by indicators of the demand of traffic at the corresponding vertices. The X itself teaches us about the topological propensity of a vertex to get congested, but such congestion will depend on the amount of traffic allocated to it. Let us illustrate this point with an example. The intersection number 3 in Sioux Falls has a large value of X, i.e., it has the third largest value of this index in this network. However, the traffic data indicates that this intersection has a congestion probability of 0.0033. The reason is not that the index X fails when identifying this intersection as one with large propensity of getting congested but that the traffic demand on it is extremely low, i.e., the lowest in the whole network. On the other extreme, we have the intersection 10, which has the highest demand in the whole network. However, it has only the fifth-highest congestion probability, mainly because, as identified by the X index, it has a large capacity to deliver traffic across the network, i.e., it has a relatively low value of the X index.
As a final proof of concept we ask whether the X index, which characterizes the propensity of a vertex to get congested, somehow reflects the different capacities of the edges to get congested, which is what is really measured by Pij in Samani et al. [69]. For this, we selected the vertex 8 which is the one having the largest value of X, i.e., X8≈3.2545. We then change the "length" of the edges which are incident with this vertex in the network. That is, instead of having A(8,i)=A(i,8)=1 in the adjacency matrix, we change this values to A(8,i)=A(i,8)=1/2. This is equivalent to reduce the length of the corresponding street, increasing its capacity to deliver traffic to its nearest intersection. Samani et al. [69] performed a similar exercise by increasing the width of streets, which is mathematically equivalent to what we are doing here. After dropping the value of the edge {8,6} to 0.5 we obtain X8(G−{8,6})=2.7989. Doing the same for the rest of edges incident with vertex 8 we get: X8(G−{8,7})=2.8483, X8(G−{8,9})=2.9412, and X8(G−{8,16})=3.0689. These results indicate that the edge contributing the most to the high value of X8 corresponds to the street {8,6} which is the one having the highest probability of congestion of all the streets in the city according to the results of Samani et al. [69]. It is followed by street {8,7} which is the one having the second highest congestion probability among all streets incident to intersection 8. Therefore, this experiment reveals that the topological indication about the potential congestion of vertices in a network as developed here matches some of the situations observed in the real-world, so it can be used in the subsequent analysis of complex systems.
We will start this section by studying three types of real-world networks (see [1] for more details about these networks). The first example is the representation of the airport transportation network of US, where the 332 vertices represent the airports in the continental USA, Alaska, and overseas places, and the 2,126 edges represent the existence of connecting flights between the airports. The hubs of the USA air transportation network are: Chicago O'Hare Int. (degree 139), Dallas/Fort Worth (degree 118), Hartsfield-Jackson Atlanta International Airport (degree 101), Pittsburg Int. (degree 94) and Lambert-St. Louis Int. (degree 94). All these airports are directly connected to each other forming a clique in the network. There are, of course, many other airports connected to pairs of these hubs, forming a structure similar to the one of the graph G1 in Figure 3.1. In the second class, we include the neuronal network of C. elegans, where 277 neurons of this worm are represented as vertices, and its 1,918 synaptic connections are accounted by means of edges. The top five hubs in this network represent the neurons AVAL (degree 76), AVAR (degree 74), AVER (degree 54), AVDR (degree 53), AVBR (degree 52), AVEL (degree 52) and PVCR (degree 52). The mean separation between these hubs is 1.28, with 6 pairs that are connected only via a path of length 2. We can consider these networks as examples of the class represented by the toy graph G2 in Figure 3.1. Finally, the third class is formed by two urban street networks representing the central area of São Paulo city and downtown Atlanta, which are illustrated in Figure 5.3. The nodes represent intersections and endpoints, while the edges represent the roads and streets. We obtain all data via the Python module OSMNx [63]. The obtained graph is simplified to undirected, unweighted, and without self-loops. The hubs in the urban street networks are intersections having connections to 6 or 5 other vertices. In the case of the city of São Paulo, the average separation between every pair of hubs is 19.3, and in the case of the city of Atlanta, it is 20.6. Every pair of hubs is separated by very long paths in these cities, which is similar to what happens in the case of the graph G3 in Figure 3.1.
We start our analysis by considering the network representing the US airport system. In Figure 5.4(a), we illustrate the network of US airports representing them with sizes proportional to their Xi values. The top ten airports in this ranking are (their degrees are in parenthesis): Salt Lake City Intl. (59); Portland Intl. (41); Stapleton Intl. (85); Raleigh-Durham Intl. (50); Seattle-Tacoma Intl. (57); Ontario Intl. (23); Sacramento Metropolitan (23); San Francisco Intl. (68); Metropolitan Oakland Intl. (20); Phoenix Sky Harbor Intl. (60). Therefore, the average degree of these airports is 48.6, and they do not include the main hubs of the network, although they are in the top 50 most connected airports. This confirms our previous hypothesis that this network behaves similarly to the one represented by the graph G1 in Figure 3.1. Let us consider the airport of Salt Lake City Int.; which is the one showing the highest X index. When we consider the distance Yuv which measures the quality of communication when there is a jam at the corresponding airport, the closest airports from Salt Lake City are Stapleton Intl.; Portland Intl.; Seattle-Tacoma Intl.; Ontario Intl.; San Francisco Intl.; Phoenix Sky Harbor Intl.; Sacramento Metropolitan.; Metropolitan Oakland Intl.; San Diego Intl.; Lindbergh Fld; San Jose Intl. All of which have direct flights to Salt Lake City and are also surrounding a relatively close area to that airport as can be seen in Figure 5.4(b). On the contrary, the most distant airports are: Raleigh-Durham Intl.; La Guardia; Washington National; Charlotte Douglas Intl.; Newark Intl; Bradley Intl.; Baltimore-Washington Intl.; Palm Beach Intl.; Fort Lauderdale Hollywood Intl.; Philadelphia Intl. All of them are on the east coast of the USA. Contrastingly, in normal conditions, as measured by the communicability distances ξuv, the airports which are better communication with the one of Salt Lake City are: General Mitchell Intl.; Louis Armstrong New Orleans International Airport; Fort Lauderdale-Hollywood Intl; Kansas City Intl; Raleigh-Durham Intl; Port Columbus Intl; Seattle-Tacoma Intl. Those in poor communication with it are mainly airports in Alaska or in the Pacific: Tuntutuliak; Kongiganak; Kwigillingok; Napaskiak; Napakiak; Eek; Quinhagak; West Tinian. Consequently, the current results indicate that in a situation of navigational vulnerability the airport of Salt Lake City represents a potential bottleneck, which will stay well communicated only with those nearby it and will dramatically diminish its communicability with those on the East Coast of the US.
We now investigate the network which resembles the graph G2 Figure 3.1. In Figure 5.5(a), we illustrate the values of X for every neuron in C. elegans representing them proportionally to the color and size of the vertices of the neuronal network. The average degree of the neurons in the top ten of the X ranking is 24.1. The two neurons identified with the highest propensity to become navigability bottlenecks are Ring Interneuron A Right (RIAR) (degree 35) and Ring Interneuron A Left (RIAL) (degree 30), which are located on the head of the worm and form part of the "second layer" interneurons in the process of integration of information from the outside world and the inner state of the animal, which then leads to a behavioral response. None of the neurons with the largest values of the X index is among the main hubs of this network, as we would expect from its resemblance to the toy model provided by the graph G2 in Figure 3.1. In panel (b), we illustrate again the communicability distance from the RIAR neuron (very similar results for RIAL) to the rest of the neurons. The shortest communicability distances between RIAL and RIAR are with the neurons located in the lateral ganglia of the head (ASHL, AIZD), the head (RMGL), left lumbar ganglia (PQR) and retrovesicular ganglion of head (AVF). They have a variety of functions, like the main nociceptor being a center of hub-and-spoke circuit, and integration of information, among others. The longest communicability distance is with command interneurons AVAR, AVAL, AVBR and AVBL, all located in the lateral ganglia of the head. When considering the Y-distance (see panel (c) of Figure 5.5) the largest proximity is to neurons located in the head, such as RIH, RMDR, and RMDL, as well as among themselves, i.e., a short distance between RIAL and RIAR. The neurons of the group RMD regulate the spontaneous foraging movements in the worm. On the other hand, the longest Y-distances are with neurons located in the tail or lumbar ganglion, such as PVCR, PVCL, PVNR, PVNL, or in the lateral ganglia of the head (AVDR and AVDL), which are all command interneurons. These results point out the possibility that certain navigational bottlenecks at the RIA neurons, which are involved in processing information from outside, limit their diffusive communication only to neurons located in the head and affect more significantly the communication with farthest away neurons which are involved in locomotion.
We now consider the two urban street networks described before. The plots in Figure 5.6 illustrate the X indices associated with each node in the urban street networks in both cities. We point to the vertices with the two highest X, which are marked as A and B, respectively. As we have mentioned before, the urban street networks have the largest degrees of 6 or 5, which are separated by about 20 streets as average from each other. This is a characteristic feature of the graphs of the class of G3 in Figure 3.1. Therefore, it is expected that the intersections with the largest values of X are those with the largest degree. Indeed, in the city of Atlanta, the vertices marked as A and B both have degree 6, and in São Paulo they have degrees 5 and 6.
The separation between the two vertices with the largest X values, which coincide with the hubs in both urban street networks, is of 24 street legs in Atlanta and 20 in São Paulo. In Atlanta there are 31 different paths of 25 street legs which connect both hubs (see Figure 5.7(a)) and in São Paulo there are 37 paths of length 21 between the vertices A and B as illustrated in Figure 5.7(b). Therefore, the two urban street networks studied here, which can be thought of as representatives of their class of networks, correspond to the class of networks in which the hubs are separated by a relatively large shortest path distance, for which the graph G3 in Figure 3.1 serves as a toy model.
Finally, to understand the reasons why the intersections A and B are the ones with the highest risk of becoming navigational bottlenecks in the cities of Atlanta and São Paulo we investigate the Y-distances from these intersections to the rest of the vertices in their respective urban street networks. The results are illustrated in Figure 5.8. As can be seen in the figure, the Y-distance from the intersections A and B in both cities is significant only to a close neighborhood of these vertices and not to any intersection relatively distant from them. If we remember what we observed for the toy graph G3 in Figure 3.1, the Y-distance between the two hubs in this graph was significantly larger than the shortest path distance connecting them. That is, the two hubs were in poor communication. This resulted in the fact that if one of the hubs is jammed, it loses its capacity to deliver traffic to the other one, increasing the probability of remaining jammed. This is exactly what happens in the intersections A and B, whose Y-distance is extremely large in these two cities, as can be seen in the plots of Figure 5.8. It is remarkable to realize that in Atlanta, the intersection A connects six roads categorized as two avenues, three boulevards, and one residential, and the intersection B has a traffic light and connects six roads, categorized as an avenue link, three boulevards, and a road that connects neighborhoods. In the case of São Paulo the intersection A has six roads connecting it, one of which is a link to one of the most critical avenues in the city, while the other five interconnect neighborhoods. The intersection B connects five roads, two of them are avenues; one links to an avenue, and two others connect neighborhoods.
The networked structure of complex systems facilitates the spread of information between the entities that compose the system. Therefore, detecting potential bottlenecks inhibiting the information spreading in these networks is of great practical relevance for the study of complex systems. Additionally, the problem is far from trivial if approached from a structure-dynamics perspective. That is, we are interested in finding vertices and edges that potentially inhibit information spreading by analyzing the network structure without the necessity of performing intensive simulations of the dynamics of interest. For that purpose we have obtained a first principles connection between the NC diffusion on a network and functions of the adjacency matrix representing their structure. In this way we measure the capacity of every vertex in a network to spread the diffusive particles across the network in a short time. This approach allows one to identify those vertices which are potential navigational bottlenecks due to their diminished capacity to spread information through the network. We have shown here how this approach can be used to study analytically the problem using algebraic graph-theoretic approaches gaining many insights about the different situations in which a vertex can be a potential navigational bottleneck. More interestingly, our approach connects this topic with that of Euclidean distance matrix analysis creating a mathematical framework for the analysis of navigational bottlenecks in networks. Our results are confirmed by studying several real-world networks, such as a neuronal system, an air transportation network and two urban street networks. All in all, the current approach represents a step-forward in our understanding of the structure-dynamics relations in complex networks, which is one of the foundational goals of this discipline.
Ernesto Estrada: Conceptualization, formal analysis, proofs and theorems, writing, methodology, applications, review, and validation; Giovanni Soares: Conceptualization, writing, methodology, simulations, cities applications, review, editing, and validation. All authors have read and approved the final version of the manuscript for publication.
The authors declare they have not used Artificial Intelligence (AI) tools in the creation of this article.
EE acknowledges funding from Ministerio de Ciencia e Innovacion, Agencia Estatal de Investigacion Program for Units of Excellences Maria de Maeztu (CEX2021−001164-M/10.13039/501100011033).
GGS acknowledges the funding of projects 446053/2023-6 and 140196/2022-6 CNPq (Brazil) and CAPES-PRINT project 88887.900891/2023-00.
All authors declare no conflicts of interest in this paper.
[1] | E. Estrada, The structure of complex networks: Theory and applications, New York: Oxford University Press, 2012. |
[2] | E. Estrada, What is a complex system, after all? Found. Sci., 2023, 1–28. https://doi.org/10.1007/s10699-023-09917-w |
[3] |
S. Boccaletti, V. Latora, Y. Moreno, M. Chavez, D. U. Hwang, Complex networks: Structure and dynamics, Phys. Rep., 424 (2006), 175–308. https://doi.org/10.1016/j.physrep.2005.10.009 doi: 10.1016/j.physrep.2005.10.009
![]() |
[4] |
L. D. Costa, O. N. Oliveira Jr, G. Travieso, F. A. Rodrigues, P. R. Villas Boas, L. Antiqueira, et al., Analyzing and modeling real-world phenomena with complex networks: A survey of applications, Adv. Phys., 60 (2011), 329–412. https://doi.org/10.1080/00018732.2011.572452 doi: 10.1080/00018732.2011.572452
![]() |
[5] |
L. Zhao, J. Zhao, Comparison study of three shortest path algorithm, Proc. Int. Conf. Comput. Technol. Eléctron. Commun. (ICCTEC), 3 (2017), 748–751. https://doi.org/10.1109/ICCTEC.2017.00165 doi: 10.1109/ICCTEC.2017.00165
![]() |
[6] | B. Golden, Shortest-path algorithms: A comparison, Op. Res., 24 (1976), 1164–1168. |
[7] |
X. Z. Wang, The comparison of three algorithms in shortest path issue, J. Phys. Conf. Ser., 1087 (2018). https://dx.doi.org/10.1088/1742-6596/1087/2/022011 doi: 10.1088/1742-6596/1087/2/022011
![]() |
[8] |
J. Liu, M. Li, Y. Pan, W. Lan, R. Zheng, F. X. Wu, et al., Complex brain network analysis and its applications to brain disorders: A survey, Complexity, 2017 (2017), 1–27. https://doi.org/10.1155/2017/8362741 doi: 10.1155/2017/8362741
![]() |
[9] | J. Goñi, A. Avena-Koenigsberger, N. Velez de Mendizabal, M. P. van den Heuvel, R. F. Betzel, O. Sporns, Exploring the morphospace of communication efficiency in complex networks, PLoS One, 8 (2013). https://doi.org/10.1371/journal.pone.0058070 |
[10] |
M. Boguna, D. Krioukov, K. C. Claffy, Navigability of complex networks, Nature Phys., 5 (2009), 74–80. https://doi.org/10.1038/nphys1130 doi: 10.1038/nphys1130
![]() |
[11] |
E. Estrada, Informational cost and networks navigability, App. Math. Comp., 397 (2021), 1–10. https://doi.org/10.1016/j.amc.2020.125914 doi: 10.1016/j.amc.2020.125914
![]() |
[12] |
C. Seguin, M. P. Van Den Heuvel, A. Zalesky, Navigation of brain networks, Proc. Natl. Acad. Sci., 115 (2018), 6297–6302. https://doi.org/10.1073/pnas.1801351115 doi: 10.1073/pnas.1801351115
![]() |
[13] |
M. Rosvall, A. Grönlund, P. Minnhagen, K. Sneppen, Searchability of networks, Phys. Rev. E, 72 (2005). https://doi.org/10.1103/PhysRevE.72.046117 doi: 10.1103/PhysRevE.72.046117
![]() |
[14] |
E. Estrada, J. Gómez-Gardeñes, L. Lacasa, Network bypasses sustain complexity, Proc. Natl. Acad. Sci., 120 (2023). https://doi.org/10.1073/pnas.2305001120 doi: 10.1073/pnas.2305001120
![]() |
[15] |
C. Seguin, O. Sporns, A. Zalesky, Brain network communication: concepts, models and applications, Nat. Rev. Neurosci., 24 (2023), 557–574. https://doi.org/10.1038/s41583-023-00718-5 doi: 10.1038/s41583-023-00718-5
![]() |
[16] | A. Avena-Koenigsberger, X. Yan, A. Kolchinsky, M. P. van den Heuvel, P. Hagmann, O. Sporns, A spectrum of routing strategies for brain networks, PLoS Comput. Biol., 15 (2019). https://doi.org/10.1371/journal.pcbi.1006833 |
[17] |
N. Masuda, M. A. Porter, R. Lambiotte, Random walks and diffusion on networks, Phys. Rep., 716-717 (2017), 1–58 https://doi.org/10.1016/j.physrep.2017.07.007 doi: 10.1016/j.physrep.2017.07.007
![]() |
[18] |
I. Simonsen, Diffusion and networks: A powerful combination! Physica A, 357 (2005), 317–330. https://doi.org/10.1016/j.physa.2005.06.032 doi: 10.1016/j.physa.2005.06.032
![]() |
[19] | R. Kasprzak, Diffusion in networks, J. Telecommun. Inf. Technol., 2012, 99–106. |
[20] |
W. Yu, G. Chen, M. Cao, Consensus in directed networks of agents with nonlinear dynamics, IEEE Trans. Automat. Contr., 56 (2011), 1436–1441. https://doi.org/10.1109/TAC.2011.2112477 doi: 10.1109/TAC.2011.2112477
![]() |
[21] | R. O. Saber, R. M. Murray, Consensus protocols for networks of dynamic agents, Proc. Am. Control. Conf., 2 (2003), 951–956. |
[22] | M. Mesbahi, Graph theoretic methods in multiagent networks, Princeton University Press, 2010. |
[23] | E. Estrada, Conservative vs. non-conservative diffusion towards a target in a networked environment, The target problem, Springer, Berlin, 2024 |
[24] |
J. Tønnesen, S. Hrabĕtová, F. N. Soria, Local diffusion in the extracellular space of the brain, Neurobiol. Dis., 177 (2023), 105981. https://doi.org/10.1016/j.nbd.2022.105981 doi: 10.1016/j.nbd.2022.105981
![]() |
[25] |
C. Nicholson, Diffusion and related transport mechanisms in brain tissue, Rep. Prog. Phys., 64 (2001), 815–885. https://iopscience.iop.org/article/10.1088/0034-4885/64/7/202 doi: 10.1088/0034-4885/64/7/202
![]() |
[26] |
L. F. Agnati, D. Guidolin, M. Guescini, S. Genedani, K. Fuxe, Understanding wiring and volume transmission, Brain Res. Rev., 64 (2010), 137–159. https://doi.org/10.1016/j.brainresrev.2010.03.003 doi: 10.1016/j.brainresrev.2010.03.003
![]() |
[27] |
L. Liu, J. Tang, J. Han, S. Yang, Learning influence from heterogeneous social networks, Data Min. Knowl. Discov., 25 (2012), 511–544. https://doi.org/10.1007/s10618-012-0252-3 doi: 10.1007/s10618-012-0252-3
![]() |
[28] |
A. Zeng, C. H. Yeung, Predicting the future trend of popularity by network diffusion, Chaos, 26 (2016), 063102. https://doi.org/10.1063/1.4953013 doi: 10.1063/1.4953013
![]() |
[29] |
L. A. Overbey, B. Greco, C. Paribello, T. Jackson, Structure and prominence in Twitter networks centered on contentious politics, Soc. Netw. Anal. Min., 3 (2013), 1351–1378. https://doi.org/10.1007/s13278-013-0134-8 doi: 10.1007/s13278-013-0134-8
![]() |
[30] | S. Goel, D. J. Watts, D. G. Goldstein, The structure of online diffusion networks, Proc. 13th ACM Conf. Elect. Comm., 2012,623–638. https://doi.org/10.1145/2229012.2229058 |
[31] |
J. Long, Z. Gao, H. Ren, A. Lian, Urban traffic congestion propagation and bottleneck identification, Sci. China. Ser. F-Inf. Sci., 51 (2008), 948–964. https://doi.org/10.1007/s11432-008-0038-9 doi: 10.1007/s11432-008-0038-9
![]() |
[32] |
J. Wang, Resilience of self-organised and top-down planned cities–-a case study on London and Beijing street networks, PloS One, 10 (2015), e0141736. https://doi.org/10.1371/journal.pone.0141736 doi: 10.1371/journal.pone.0141736
![]() |
[33] |
J. Buhl, J. Gautrais, N. Reeves, R. V. Solé, S. Valverde, P. Kuntz, et al., Topological patterns in street networks of self-organized urban settlements, Eur. Phys. J. B, 49 (2006), 513–522. https://doi.org/10.1140/epjb/e2006-00085-1 doi: 10.1140/epjb/e2006-00085-1
![]() |
[34] | A. Furno, N. E. El Faouzi, R. Sharma, V. Cammarota, E. Zimeo, A graph-based framework for real-time vulnerability assessment of road networks, Proc. Int. Conf. Smart. Comput. SMARTCOMP, 2018,234–241. |
[35] |
P. Medina, S. C. Carrasco, M. S. Jofré, J. Rogan, J. A. Valdivia, Characterizing diffusion processes in city traffic, Chaos Soliton. Fract., 165 (2022), 112846. https://doi.org/10.1016/j.chaos.2022.112846 doi: 10.1016/j.chaos.2022.112846
![]() |
[36] | S. S. Kim, M. Chung, Y. K. Kim, Urban traffic prediction using congestion diffusion model, Proc. IEEE Int. Conf. Consum. Electron-Asia (ICCE-Asia), 2020, 1–4. |
[37] | T. Anwar, C. Liu, L. V. Hai, M. S. Islam, Roadrank: Traffic diffusion and influence estimation in dynamic urban road networks, Proc. ACM Int. Conf. Inf. Knowl. Manag., 2015, 1671–1674. |
[38] |
A. Bhaskar, T. Tsubota, E. Chung, Urban traffic state estimation: Fusing point and zone based data, Transport. Res. C Emer., 48 (2014), 120–142. https://doi.org/10.1016/j.trc.2014.08.015 doi: 10.1016/j.trc.2014.08.015
![]() |
[39] |
A. Bhaskar, E. Chung, A. G. Dumont, Estimation of travel time on urban networks with midlink sources and sinks. Transp. Res. Rec., 2121 (2009), 41–54. https://doi.org/10.3141/2121-05 doi: 10.3141/2121-05
![]() |
[40] |
Å. Brännström, D. J. Sumpter, Coupled map lattice approximations for spatially explicit individual-based models of ecology, Bull. Math. Biol., 67 (2005), 663–682. https://doi.org/10.1016/j.bulm.2004.09.006 doi: 10.1016/j.bulm.2004.09.006
![]() |
[41] |
K. H. Taber, R. A. Hurley, Volume transmission in the brain: Beyond the synapse, J. Neuropsychiatry. Clin. Neurosci., 26 (2014), E01–104. https://doi.org/10.1176/appi.neuropsych.13110351 doi: 10.1176/appi.neuropsych.13110351
![]() |
[42] |
K. Fuxe, D. O. Borroto-Escuela, A. Tarakanov, W. R. Fernandez, P. Manger, A. Rivera, K. van Craenenbroeck, et al., Understanding the balance and integration of volume and synaptic transmission. Relevance for psychiatry, Neurol. Psychiat. B. R., 19 (2013), 141–158. https://doi.org/10.1016/j.npbr.2013.10.002 doi: 10.1016/j.npbr.2013.10.002
![]() |
[43] |
E. Sykova, Extrasynaptic volume transmission and diffusion parameters of the extracellular space, Neuroscience, 129 (2004), 861–876. https://doi.org/10.1016/j.neuroscience.2004.06.077 doi: 10.1016/j.neuroscience.2004.06.077
![]() |
[44] |
D. O. Borroto-Escuela, M. P. De La Mora, P. Manger, M. Narvaez, S. Beggiato, M. Crespo-Ramírez, et al., Brain dopamine transmission in health and parkinson's disease: Modulation of synaptic transmission and plasticity through volume transmission and dopamine heteroreceptors, Front. Synaptic Neurosci., 10 (2018), 20. https://doi.org/10.3389/fnsyn.2018.00020 doi: 10.3389/fnsyn.2018.00020
![]() |
[45] |
K. Wiencke, A. Horstmann, D. Mathar, A. Villringer, J. Neumann, Dopamine release, diffusion and uptake: A computational model for synaptic and volume transmission, PLoS Comput. Biol., 16 (2020), e1008410. https://doi.org/10.1371/journal.pcbi.1008410 doi: 10.1371/journal.pcbi.1008410
![]() |
[46] |
H. Xiong, E. Lacin, H. Ouyang, A. Naik, X. Q. Xu, C. Xie, et al., Probing neuropeptide volume transmission in vivo by simultaneous near-infrared light-triggered release and optical sensing, Angew. Chem. Int. Ed. Engl., 61 (2022), e202206122. https://doi.org/10.1101/2021.09.10.459853 doi: 10.1101/2021.09.10.459853
![]() |
[47] |
H. Hamedmoghadam, M. Jalili, H. L. Vu, L. Stone, Percolation of heterogeneous flows uncovers the bottlenecks of infrastructure networks, Nat. Commun., 12 (2021), 1254. https://doi.org/10.1038/s41467-021-21483-y doi: 10.1038/s41467-021-21483-y
![]() |
[48] |
P. A. Witte, B. W. Wiegmans, F. G. van Oort, T. J. Spit, Chokepoints in corridors: Perspectives on bottlenecks in the European transport network, Res. Transp. Bus. Manag., 5 (2012), 57–66. https://doi.org/10.1016/j.rtbm.2012.10.001 doi: 10.1016/j.rtbm.2012.10.001
![]() |
[49] |
W. H. Lee, S. S. Tseng, J. L. Shieh, H. H. Chen, Discovering traffic bottlenecks in an urban network by spatiotemporal data mining on location-based services, IEEE Trans. Intell. Transp. Syst., 12 (2011), 1047–1056. https://doi.org/10.1109/TITS.2011.2144586 doi: 10.1109/TITS.2011.2144586
![]() |
[50] |
C. Li, W. Yue, G. Mao, Z. Xu, Congestion propagation based bottleneck identification in urban road networks, IEEE Trans. Veh. Technol., 69 (2020), 4827–4841. https://doi.org/10.1109/TVT.2020.2973404 doi: 10.1109/TVT.2020.2973404
![]() |
[51] |
X. He, C. Papadopoulos, J. Heidemann, U. Mitra, U. Riaz, Remote detection of bottleneck links using spectral and statistical methods, Comput. Netw., 53 (2009), 279–298. https://doi.org/10.1016/j.comnet.2008.10.001 doi: 10.1016/j.comnet.2008.10.001
![]() |
[52] |
Q. K. Qu, F. J. Chen, X. J. Zhou, Road traffic bottleneck analysis for expressway for safety under disaster events using blockchain machine learning, Saf. Sci., 118 (2019), 925–932. https://doi.org/10.1016/j.ssci.2019.06.030 doi: 10.1016/j.ssci.2019.06.030
![]() |
[53] |
S. Sreenivasan, R. Cohen, E. López, Z. Toroczkai, H. E. Stanley, Structural bottlenecks for communication in networks, Phys. Rev. E, 75 (2007), 036105. https://doi.org/10.1103/PhysRevE.75.036105 doi: 10.1103/PhysRevE.75.036105
![]() |
[54] |
R. Banner, A. Orda, Bottleneck routing games in communication networks, IEEE J. Sel. Areas Commun., 25 (2007), 1173–1179. https://doi.org/10.1109/JSAC.2007.070811 doi: 10.1109/JSAC.2007.070811
![]() |
[55] |
N. Hu, L. Li, Z. M. Mao, P. Steenkiste, J. Wang, Locating internet bottlenecks: Algorithms, measurements, and implications, ACM Sigcomm. Comp. Com., 34 (2004), 41–54. https://doi.org/10.1145/1030194.1015474 doi: 10.1145/1030194.1015474
![]() |
[56] |
P. Bonacich, Factoring and weighting approaches to status scores and clique identification, J. Math. Sociol., 2 (1972), 113–120. https://doi.org/10.1080/0022250X.1972.9989806 doi: 10.1080/0022250X.1972.9989806
![]() |
[57] |
P. Bonacich, Power and centrality: A Family of measures, Am. J. Sociol., 92 (1987), 1170–1182. https://doi.org/10.1086/228631 doi: 10.1086/228631
![]() |
[58] |
P. Bonacich, Some unique properties of eigenvector centrality, Soc. Networks, 29 (2007), 555–564. https://doi.org/10.1016/j.socnet.2007.04.002 doi: 10.1016/j.socnet.2007.04.002
![]() |
[59] |
E. Estrada, The communicability distance in graphs, Linear Algebra Appl., 436 (2012), 4317–4328. https://doi.org/10.1016/j.laa.2012.01.017 doi: 10.1016/j.laa.2012.01.017
![]() |
[60] |
E. Estrada, M. G. Sanchez-Lirola, J. A. De La Peña, Hyperspherical embedding of graphs and networks in communicability spaces, Discrete Appl. Math., 176 (2014), 53–77. https://doi.org/10.1016/j.dam.2013.05.032 doi: 10.1016/j.dam.2013.05.032
![]() |
[61] |
E. Estrada, N. Hatano, Communicability angle and the spatial efficiency of networks, SIAM Rev. Soc. Ind. Appl. Math., 58 (2016), 692–715. https://doi.org/10.1137/141000555 doi: 10.1137/141000555
![]() |
[62] |
E. Estrada, Every nonsingular spherical Euclidean distance matrix is a resistance distance matrix, Linear Algebra Appl., 656 (2023), 198–209. https://doi.org/10.1016/j.laa.2022.09.025 doi: 10.1016/j.laa.2022.09.025
![]() |
[63] |
G. Boeing, OSMnx: New methods for acquiring, constructing, analyzing, and visualizing complex street networks, Comput. Environ. Urban. Syst., 65 (2017), 126–139. https://doi.org/10.1016/j.compenvurbsys.2017.05.004 doi: 10.1016/j.compenvurbsys.2017.05.004
![]() |
[64] |
R. Ghosh, K. Lerman, Parameterized centrality metric for network analysis, Phys. Rev. E, 83 (2011), 066118. https://link.aps.org/doi/10.1103/PhysRevE.83.066118 doi: 10.1103/PhysRevE.83.066118
![]() |
[65] |
R. Ghosh, K. Lerman, T. Surachawala, K. Voevodski, S. Teng, Non-conservative diffusion and its application to social network analysis, J. Complex. Netw., 12 (2024), cnae006. https://doi.org/10.48550/arXiv.1102.4639 doi: 10.48550/arXiv.1102.4639
![]() |
[66] |
E. Estrada, J. A. Rodriguez-Velazquez, Subgraph centrality in complex networks, Phys. Rev. E, 71 (2005), 056103. https://link.aps.org/doi/10.1103/PhysRevE.71.056103 doi: 10.1103/PhysRevE.71.056103
![]() |
[67] |
E. Estrada, N. Hatano, Communicability in complex networks, Phys. Rev. E, 77 (2008), 036111. https://link.aps.org/doi/10.1103/PhysRevE.77.036111 doi: 10.1103/PhysRevE.77.036111
![]() |
[68] |
E. Estrada, N. Hatano, M. Benzi, The physics of communicability in complex networks, Physics Rep., 514 (2012), 89–119. https://doi.org/10.1016/j.physrep.2012.01.006 doi: 10.1016/j.physrep.2012.01.006
![]() |
[69] |
A. R. Samani, S. N. Shetab-Boushehri, R. Mahmoudi, Reliable urban transportation network design problem considering recurrent traffic congestions, Adv. Ind. Eng., 55 (2021), 69–89. https://doi.org/10.22059/jieng.2021.326142.1784 doi: 10.22059/jieng.2021.326142.1784
![]() |
Xv | Xw | tc(v) | tc(w) | |
G1 | 0.190 | 0.877 | 172 | 286 |
G2 | 0.505 | 0.877 | 232 | 246 |
G3 | 1.560 | 1.399 | 988 | 884 |