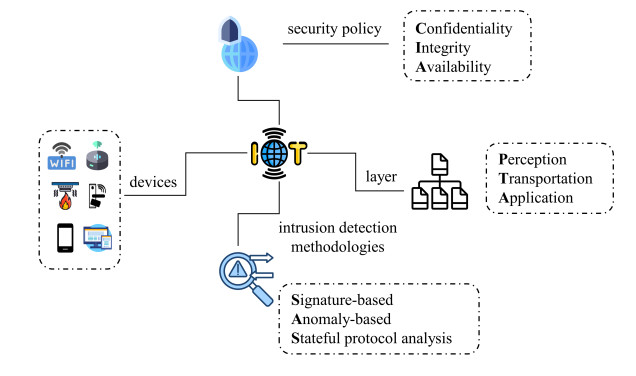
With the widespread application of smart devices, the security of internet of things (IoT) systems faces entirely new challenges. The IoT data stream operates in a non-stationary, dynamic environment, making it prone to concept drift. This paper focused on addressing the issue of concept drift in data streams, with a key emphasis on introducing an innovative drift detection method-ensemble multiple non-parametric concept localization detectors, abbreviated as EMNCD. EMNCD employs an ensemble of non-parametric statistical methods, including the Kolmogorov-Smirnov, Wilcoxon rank sum and Mann-Kendall tests. By comparing sample distributions within a sliding window, EMNCD accurately detects concept drift, achieving precise localization of drift points, and enhancing overall detection reliability. Experimental results demonstrated the superior performance of EMNCD compared to classical methods on artificial datasets. Simultaneously, to enhance the robustness of data stream processing, we presented an online anomaly detection method based on the isolation forest (iForest). Additionally, we proposedwhale optimization algorithm (WOA)-extreme gradient boosting (XGBoost), a drift adaptation model employing XGBoost as a base classifier. This model dynamically updates using drift points detected by EMNCD and fine-tunes parameters through the WOA. Real-world applications on the edge-industrial IoTset (IIoTset) intrusion dataset explore the impact of concept drift on intrusion detection, where IIoT is a subclass of IoT. In summary, this paper focused on EMNCD, introducing innovative approaches for drift detection, anomaly detection, and drift adaptation. The research provided practical and viable solutions to address concept drift in data streams, enhancing security in IoT systems.
Citation: Renjie Chu, Peiyuan Jin, Hanli Qiao, Quanxi Feng. Intrusion detection in the IoT data streams using concept drift localization[J]. AIMS Mathematics, 2024, 9(1): 1535-1561. doi: 10.3934/math.2024076
[1] | Mohammed Aljebreen, Hanan Abdullah Mengash, Khalid Mahmood, Asma A. Alhashmi, Ahmed S. Salama . Enhancing cybersecurity in cloud-assisted Internet of Things environments: A unified approach using evolutionary algorithms and ensemble learning. AIMS Mathematics, 2024, 9(6): 15796-15818. doi: 10.3934/math.2024763 |
[2] | Youseef Alotaibi, R Deepa, K Shankar, Surendran Rajendran . Inverse chi-square-based flamingo search optimization with machine learning-based security solution for Internet of Things edge devices. AIMS Mathematics, 2024, 9(1): 22-37. doi: 10.3934/math.2024002 |
[3] | Ibrahim R. Alzahrani, Randa Allafi . Integrating Ebola optimization search algorithm for enhanced deep learning-based ransomware detection in Internet of Things security. AIMS Mathematics, 2024, 9(3): 6784-6802. doi: 10.3934/math.2024331 |
[4] | Fahad F. Alruwaili . Ensuring data integrity in deep learning-assisted IoT-Cloud environments: Blockchain-assisted data edge verification with consensus algorithms. AIMS Mathematics, 2024, 9(4): 8868-8884. doi: 10.3934/math.2024432 |
[5] | Eatedal Alabdulkreem, Mesfer Alduhayyem, Mohammed Abdullah Al-Hagery, Abdelwahed Motwakel, Manar Ahmed Hamza, Radwa Marzouk . Artificial Rabbit Optimizer with deep learning for fall detection of disabled people in the IoT Environment. AIMS Mathematics, 2024, 9(6): 15486-15504. doi: 10.3934/math.2024749 |
[6] | E Laxmi Lydia, Chukka Santhaiah, Mohammed Altaf Ahmed, K. Vijaya Kumar, Gyanendra Prasad Joshi, Woong Cho . An equilibrium optimizer with deep recurrent neural networks enabled intrusion detection in secure cyber-physical systems. AIMS Mathematics, 2024, 9(5): 11718-11734. doi: 10.3934/math.2024574 |
[7] | Sultanah M. Alshammari, Nofe A. Alganmi, Mohammed H. Ba-Aoum, Sami Saeed Binyamin, Abdullah AL-Malaise AL-Ghamdi, Mahmoud Ragab . Hybrid arithmetic optimization algorithm with deep learning model for secure Unmanned Aerial Vehicle networks. AIMS Mathematics, 2024, 9(3): 7131-7151. doi: 10.3934/math.2024348 |
[8] | Maha M. Althobaiti, José Escorcia-Gutierrez . Weighted salp swarm algorithm with deep learning-powered cyber-threat detection for robust network security. AIMS Mathematics, 2024, 9(7): 17676-17695. doi: 10.3934/math.2024859 |
[9] | Liu Yang, Lijun Yin, Zuicha Deng . Drift coefficient inversion problem of Kolmogorov-type equation. AIMS Mathematics, 2021, 6(4): 3432-3454. doi: 10.3934/math.2021205 |
[10] | Zengming Feng, Tingwen Cao . FOA-BDNet: A behavior detection algorithm for elevator maintenance personnel based on first-order deep network architecture. AIMS Mathematics, 2024, 9(11): 31295-31316. doi: 10.3934/math.20241509 |
With the widespread application of smart devices, the security of internet of things (IoT) systems faces entirely new challenges. The IoT data stream operates in a non-stationary, dynamic environment, making it prone to concept drift. This paper focused on addressing the issue of concept drift in data streams, with a key emphasis on introducing an innovative drift detection method-ensemble multiple non-parametric concept localization detectors, abbreviated as EMNCD. EMNCD employs an ensemble of non-parametric statistical methods, including the Kolmogorov-Smirnov, Wilcoxon rank sum and Mann-Kendall tests. By comparing sample distributions within a sliding window, EMNCD accurately detects concept drift, achieving precise localization of drift points, and enhancing overall detection reliability. Experimental results demonstrated the superior performance of EMNCD compared to classical methods on artificial datasets. Simultaneously, to enhance the robustness of data stream processing, we presented an online anomaly detection method based on the isolation forest (iForest). Additionally, we proposedwhale optimization algorithm (WOA)-extreme gradient boosting (XGBoost), a drift adaptation model employing XGBoost as a base classifier. This model dynamically updates using drift points detected by EMNCD and fine-tunes parameters through the WOA. Real-world applications on the edge-industrial IoTset (IIoTset) intrusion dataset explore the impact of concept drift on intrusion detection, where IIoT is a subclass of IoT. In summary, this paper focused on EMNCD, introducing innovative approaches for drift detection, anomaly detection, and drift adaptation. The research provided practical and viable solutions to address concept drift in data streams, enhancing security in IoT systems.
The IoT connects devices and communication networks globally, allowing things to interconnect and cooperate with each other, greatly facilitating our daily lives. The most common IoT applications are in smart homes [1], smart cities [2,3], intelligent transportation [4], security monitoring [5,6], and more. It is expected that by 2025, the global annual increase of IoT devices will reach trillions [7,8]. However, while cyberspace brings prosperity and convenience, it also presents various security risks and challenges.
The IoT system can be divided into three layers: perception, transportation, and application. At perception layer, the devices are deployed in the external zone, while the IoT application layer spans a multitude of devices, making the IoT system highly vulnerable to attacks [9]. Therefore, network security on the IoT has significant research significance. Traditional network security mechanisms often focus on static environments, effectively detecting specific network attacks such as wormhole attacks [10,11]. However, in the complex and destructive attack types present in the real IoT environment, such models struggle to adapt to environmental changes and their effectiveness is weakened.
Intrusion detection system (IDS) is an effective technique to identify attacks. IDS detects various network attacks by monitoring network traffic or system running status [9]. The IoT IDS architecture is shown in Figure 1.
In [12], the authors introduced an intelligent IDS based on deep learning algorithms. By integrating recursive neural networks and gated recurrent units, the system comprehensively detects attacks across different layers, offering robust support for the overall security of the IoT system. An IDS based on transformer neural networks (TNN) was proposed in [13]. By leveraging the parallel processing capabilities of TNN, this approach accelerates the learning process, enhancing the effectiveness of detecting network attacks. Experimental results indicate that TNN-IDS outperforms other machine learning and deep learning-based IDS in detecting malicious activities. In [14], the authors aim to enhance performance and reduce computational power by leveraging deep convolutional neural networks and feature engineering. Through extensive experiments on the IoT network intrusion dataset 2020 (IoTID20), the model demonstrates outstanding performance across multiple evaluation metrics, providing new insights into the field of intrusion detection in IoT networks.
The above studies have introduced new possibilities to the field of IDS by incorporating deep learning and novel neural network architectures. These innovative approaches not only provide us with more accurate intrusion detection tools but also enable us to better adapt to the complexity of the IoT environment. However, in this rapidly evolving field, the data streams [15] in the IoT operate non-stationarily, exhibiting dynamism in a constantly changing environment. This poses new challenges for data processing and analysis. In this context, concept drift [16] emerges as a significant concern within the IoT domain, as the continuous shifts in data distribution can impact the stability and accuracy of models. Researchers typically classify concept drift into four types based on the duration of the change in data distribution: sudden drift (changes in distribution over a short period), gradual drift (distribution changes over an extended period, with new concepts replacing old ones), incremental drift (gradual transformation of old concepts into new ones, with many intermediate concepts appearing during the transition), and recurring drift (a repeat of a concept over time).
We can break down the concept drift detection framework into four key stages: data retrieval, data modeling, test statistic computation, and hypothesis testing [16]. The selection of crucial features and the configuration of statistical metrics and hypothesis testing methods during the test statistic computation and hypothesis testing stages significantly impact the accuracy of drift detection. Regarding the choice of statistical metrics, various drift detection methods have been proposed from different perspectives, including those based on means value [17], density [18], information entropy [19], and more. These existing methods lay the foundation for addressing concept drift challenges in the field of the IoT [20,21]. Utilizing methods such as mean, density, and information entropy for concept drift detection offers significant intuitive and interpretive advantages, assisting researchers in quantitatively analyzing concept drift phenomena. These methods rely on the overall distribution characteristics of the data, enabling them to be highly sensitive in certain scenarios and effectively capturing pronounced changes in distribution. However, despite their numerous strengths, these methods also come with certain limitations. For instance, they often depend on specific data distribution assumptions, like normality, which might limit their accuracy in complex data distributions. Furthermore, they are susceptible to the influence of outliers and noise, potentially leading to false positive or false negative concept drift reports. Of special note is the challenge that these methods might face in capturing complex concept drift patterns, especially in the presence of nonlinear or higher-order shifts. It is precisely this realization that drives us to acknowledge the necessity of exploring more robust methodologies to comprehensively address these issues.
In this context, our research uses an ensemble learning approach to improve the accuracy and robustness of concept drift localization. This method overcomes the limitations of a single method by integrating the results of multiple detectors and taking full advantage of the complementarity between different methods. This paper presents a concept drift localization method based on multiple nonparametric statistical analysis. The advantage of this approach is that it does not require assumptions about a particular distribution of data, especially when dealing with complex and changing IoT environments. Beyond the concept drift method, we introduce an online data anomaly detection technique based on iForest to enhance the robustness of data stream processing. Additionally, we present a drift adaptation method specifically tailored to confront the challenges of concept drift in IoT data streams, thereby furnishing practical and viable solutions to bolster the security of IoT systems.
The main contributions of this paper are as follows:
(1) We propose an ensemble concept drift localization algorithm that integrates multiple nonparametric statistical methods that are well-suited for practical applications.
(2) We design an online outlier detection method for data streams that considers both global and local outliers.
(3) We propose a drift adaptive framework based on a WOA to optimize hyperparameters that can effectively address the problem of network attack detection in IoT systems and consider the effects of concept drift on network attack identification.
(4) The proposed model improves the effectiveness and efficiency of the IDS and protects the IoT system from network attacks.
The rapid development of the IoT has led to an increase in network threats, which can have a negative impact on the operation of IoT systems. IDSs are effective means of protecting IoT systems, and researchers have conducted extensive research on them. For example, federated learning intrusion detection systems (FLIDS), a distributed IDS for IoT that uses federated learning and reinforcement mechanisms for privacy protection, have been proposed in [22] and verified on the NF-UNSW-NB15-v2 dataset to confirm its effectiveness. Similarly, in [23], a machine learning-based model was developed to identify common types of attacks on the network, and in [24] an intelligent two-layer IDS for IoT was proposed. Additionally, the data sampling technique with a two-stage convolutional neural network (DS-2CNN) model was proposed in [25] to accurately identify IoT devices with only a small number of samples, and a communication mechanism based on blockchain was proposed in [26] to monitor the evaluation trust of each smart device in real time to ensure IoT system security. While most of the methods for IoT network attack detection focus on applications, algorithms, feature selection, and other aspects, some scholars have begun to consider solving the concept drift problem in the IoT scenario in recent years due to the special characteristics of network data streams.
Reference [20] discusses the impact of concept drift on Botnet cyber-attack detection. The author proposes a dynamic residual projection method (DRPM) for network attack detection. The method first determines whether there is a drift in the data stream, and once the drift is detected, CNN and long short-term memory (LSTM) are used to learn the features of new concepts. The validation dataset is cyber-attack detection on a realistic botnet dataset in IoT (Bot-IoT) combined with the concept drift analysis method; the network attack detection performance is significantly improved. In [27], the principal component analysis (PCA) method is used to detect concept drift in IoT intrusion detection data streams and an outlier detection technology for data streams is proposed. The main contribution of this paper is to solve the drift problem in network data streams. The author proposes an online deep neural network method based on hedge weighting mechanism, which enables the model to learn and adapt stably when new intrusion data arrives; thus, effectively reducing the false positive and missing positive of the model. An adaptive light gradient boosting machine (light GBM) model for IoT data analysis is proposed in [21], which can detect IoT attacks with high accuracy and adapt to concept drift. Clearly, for the phenomenon of concept drift in IoT security problems, an effective solution is to detect the localization of concept drift and update the current model according to the data stream of drift.
Methods based on error rate and data distribution are two important algorithms in drift detection. The drift detection method (DDM) algorithm [17] takes the sample mean and standard deviation as detection statistics and detects drift through the change of statistics. The Page-Hinckley [28] algorithm is a simple and effective concept drift detection method, which is suitable for batch processing data streams. As the data stream is processed, each sample is gradually added to the sliding window and updates the cumulative sum. The algorithm determines concept drift by manually setting a threshold. At each time step, it compares the cumulative sum between the latest window and the previous window. If the threshold is exceeded, concept drift is considered to occur. Alippi et al. [29] proposed a concept drift detection called hierarchical change-detection tests (HCDTs), which includes a detection layer and a verification layer. The detection layer has two design strategies for rapidly detecting data changes. The first strategy involves using the maximum likelihood method to calculate the probability density function of the corresponding distribution of samples and comparing the differences. The second strategy is to directly test whether the two data distributions are consistent using hypothesis testing methods, such as the Kolmogorov-Smirnov test. When the detection layer identifies a change, it immediately activates the verification layer to recheck the data distribution change. This hierarchical drift test effectively reduces false positives.
A statistical test of equal proportions detection method (STEPD) [30] compares the classification error rate of local samples included in the latest time window and all samples included in the whole-time window, and detects the concept drift by judging whether the prediction error has changed significantly through the chi-square test. From the perspective of the source of concept drift, the drift detection algorithm based on data distribution uses the window mechanism to calculate the distance between window samples to measure the difference between the new and old data distributions. [31] proposed a Wilcoxon rank sum statistical test for concept drift detection (WSTD) algorithm, which used the Wilcoxon rank sum test to judge the difference between two window distributions so as to detect concept drift. [32] proposed a PCA-based change detection (PCA-CD) framework, which first projected data into a low-dimensional space, then detected changes in data distribution by estimating the density and divergence between Windows. The algorithm is friendly to high-dimensional data streams and reduces the computational cost.
When the drift is detected, it needs to be properly processed so that the model can adapt to the new data stream later. [33] proposed a forgetting parameters extreme learning machine (FP-ELM) online incremental learning algorithm. FP-ELM will set forgetting parameters for the old concept training data according to the performance of the initial model, so as to adapt to the possible changes after the arrival of the new data stream. [34] investigates an ensemble learning approach that uses transfer learning to retrain the model to adapt to the current concept for newly arriving data streams. In this paper, an adaptive framework for IoT data streams is designed to address the impact of concept drift on cyberattack detection in IoT. In real life, because the acquisition of class labels of data streams is difficult and expensive, detection algorithms using class labels cannot be widely used. The proposed concept drift detection method no longer relies on labels and only utilizes data features for detection.
In this section, we will develop a drift localization algorithm using ensemble learning for multiple nonparametric statistical methods, which can accurately identify the location of drifts in a data stream. We will first review the fundamental concepts of nonparametric methods and then introduce the framework of our proposed drift localization technique, providing corresponding theoretical support. Finally, we will study an online outlier detection method for data streams, which can monitor abnormal situations in real time and provide timely alerts for anomalous data. Figure 2 illustrates the overall architecture of the research presented in this paper.
Based on the definition of drift, we consider that concept drift occurs when the distribution of the latter two concepts changes over time. However, in practical applications, the theoretical distribution of data streams is often unknown. In statistics, when the data cannot be assumed to meet the known distribution, nonparametric statistics can be used to solve problems, such as assuming the population has a certain distribution or whether the distribution of the population is the same.
The Kolmogorov-Smirnov test (K-S test) [35,36], Wilcoxon rank sum test (Wilcoxon test) [31], and Mann-Kendall test (M-K test) [37] are non-parametric tests used to compare the cumulative distribution functions of two independent samples.
Given two samples {X1,...,Xm} and {Y1,...,Yn}, where Fm(x) and Gn(x) are empirical distribution functions derived from two samples, ∀x∈R,
Fm(x)=1m{the number in {X1,…,Xm} that is less than x}, |
Gn(x)=1n{the number in {Y1,…,Yn} that is less than x}. |
The test statistic is defined as
Dm,n=sup−∞<x<+∞|Fm(x)−Gn(x)|. | (3.1) |
The K-S test reflects the difference between two samples through the degree of convergence of Dm,n.
The basic idea of the Wilcoxon rank sum test method is: m+n observations are mixed in order and T represents the rank sum of {X1,...,Xm} in the joint sample {X1,...,Xm}; {Y1,...,Yn}; that is,
T=m∑i=1Ri,i=1⋯m. | (3.2) |
If the statistic T does not fall into the rejection domain, the two samples are considered consistent.
The M-K test is to compare each number of data points (Xi,Yj) for a sequence containing n data points, and the M-K statistic S is defined as the sum of the number of increasing differences minus the number of decreasing differences:
S=n−1∑i=1n∑j=i+1sgn(Xj−Xi), | (3.3) |
sgn(θ)={1, if θ>0,0, if θ=0,−1, if θ<0. | (3.4) |
The significance level of statistic S is used to determine whether the trend of the data is caused by random or statistically significant.
Non-parametric testing techniques require no assumptions about population parameters and are applicable to various data types. The aforementioned methods can be employed to compare the data distribution between the current time t and the previous time t−1 in data streams. In this study, we introduce the EMNCD method. This approach integrates the K-S test, Wilcoxon test and M-K test, three non-parametric statistical methods to examine whether changes occur in data distribution. By configuring significance levels and sample sizes to determine critical values, these techniques assess the degree of association or disparity between the two datasets. We recommend utilizing these methods for executing drift localization. The detailed procedure is shown in Algorithm 1
Algorithm 1. EMNCD. |
Input: Data stream S; n; window_size; step; threshold |
Output: concept |
1: Initialize concept = [] |
2: for i in 1 : int((n−window_size)/step) do |
3: WS1 = S[(i−1)∗step:(i−1)∗step+window_size,:] |
4: WS2 = S[i∗step:i∗step+window_size,:] |
5: for i in 1 : int((window_size)/step) do |
6: Perform K-S test for (WS1[:, j], WS2[:, j]), obtain p value ks_p |
7: Perform Wilcoxon test for (WS1[:, j], WS2[:, j]), obtain p value wilcoxon_p |
8: Perform M-K test for (WS1[:, j], WS2[:, j]), obtain p value mk_p |
9: Ensemble the three p values: |
10: ensemble_result = ensemble (ks_p, wilcoxon_p, mk_p) |
11: if ensemble_result < threshold then |
12: concept[i, 1] = i |
13: concept[i, 2] = (i−1)∗step |
14: end if |
15: end for |
16: end for |
The algorithm is designed to detect drift in an online data stream S. It first sets the size of the sliding window and the sliding step size, which are used to divide the data stream into two windows WS1 and WS2. The window WS1 contains the initial window_size data samples, then the window WS1 is slid backward step by step to obtain the current window WS2. The algorithm then compares the degree of difference between the two datasets within the two windows using three non-parametric statistical methods to detect the occurrence of drift. If the p values of the test result are less than the threshold, the threshold is significance level, meaning that the difference between the two datasets is significant, thus, it is considered that there is drift, and the algorithm outputs the window and sample position where the drift occurs. If there is no significant difference, then no drift warning is given. The algorithm repeats these steps until all samples in the data stream have been tested.
Outliers [38] refer to observation values that significantly deviate from the majority of samples in the dataset where they are located. In data preprocessing, how to deal with outliers depends on the situation. Some outliers may affect the efficiency of modeling and even lead to the deviation of results, while some outliers may contain useful information. Most traditional outlier detection techniques are analyzed on offline datasets, including statistics-based methods, distance-based methods, and density-based methods. However, for dynamically changing data streams, the direct use of these methods cannot obtain the desired effect. [39] proposed a powerful dimensionality reduction approach to visualize IoT network traffic. This method has been proven effective in detecting anomalies in IoT traffic data, bringing valuable insights into the domain of anomaly detection within the IoT.
iForest algorithm [40,41] is a fast and efficient unsupervised anomaly detection method. It is particularly suitable for detecting anomalies in continuous structured data due to its low computational cost. The time complexity of constructing isolation trees is approximately O(N⋅log(N)) and the space complexity is O(N), where N is the number of samples. iForest is utilized for detecting anomalies in a dataset X, involving training and testing stages. During training, isolation trees are constructed for the samples, forming isolation forests. The path length of each sample is recorded. In the testing stage, iForest determines if a sample is abnormal based on its path length.
The anomaly score s(x,n) for an instance x is calculated using the formula:
s(x,n)=2−E(h(x))/c(n), | (3.5) |
where E(h(x)) is the average of h(x) from a collection of isolation trees.
c(n)=2H(n−1)−(2(n−1)/n), |
where H(i) is the harmonic number and it can be estimated by ln(i)+0.5772156649. The anomaly score signifies the likelihood of an instance being an outlier, with values closer to one indicating anomalies.
The iForest algorithm is suitable for detecting anomalies in continuous structured data. Due to the characteristics of large data volume, fast dynamic change speed, and strong continuity of data streams, real-time monitoring is required to ensure the accuracy and precision of outlier detection for data streams [42]. Therefore, this paper proposes an online outlier detection method based on iForest. Compared with other anomaly detection algorithms, iForest does not need to calculate density, such as the local outlier factor (LOF) algorithm or distance (such as k-nearest neighbor algorithm), but to divide features and preferentially find outliers. Therefore, the calculation cost of the iForest algorithm is lower and the speed is faster. At the same time, the iForest algorithm can quantify the anomaly degree of each sample, while the outlier detection method based on clustering can only judge whether it is an anomaly, such as density-based spatial clustering of applications with noise (DBSCAN). By using the historical concept data, the global outlier value of the data stream can be determined. Combined with sliding window technology, the anomaly degree of the current concept data can be calculated simultaneously to identify the local outlier value of the current concept.
Algorithm 2 describes the proposed online outlier detection process for data streams.
Algorithm 2. Online anomaly detection with iForest. |
Input: Historical data Data1; current window data Data2; time window w |
Output: Local outliers; local outliers of current window |
1: Initialize global_outliers = [] |
2: Initialize local_outliers = [] |
3: Use iForest to calculate anomaly scores of all samples in Data1 |
4: for each data point x do |
5: compute the anomaly score D1x = s(x, len(Data1)) |
6: compute the anomaly score D2x = s(x, len(Data2)) |
7: if D1x ≈ 1 then |
8: Add x to global_outliers |
9: end if |
10: end for |
11: Calculate anomaly scores D2x of all samples in Data2 |
12: Detect local_outliers in Data2 |
13: if drift is detected according to Algorithm 1 then then |
14: update Data2 according to drift position |
15: recalculate anomaly scores of updated Data2 |
16: end if |
17: Return local outliers of Data2 |
You need to specify the time window w, all the historical data and the data in the current time window w. The isolated forest algorithm was used to calculate the anomaly score of each sample in all historical data, and if the anomaly score was equal to one, it was marked as a global outlier. Similarly, the abnormal scores of samples in the current time window were calculated to detect whether there were local outliers. If a drift is detected according to Algorithm 1, the current window is updated with the sample according to the drift position, and the sample anomaly score is recalculated.
In a data stream with concept drift, where the concepts in the data stream change over time, the initial training model becomes unsuitable for the new data stream. Continuing to use the original model will result in poor or even completely invalid classification performance. Concept drift is the root cause of significant degradation of classification performance, and adaptive learning is a suitable solution. In this paper, we propose an online update prediction model to adapt to potential concept drift and deal with the changing data stream. The proposed method uses XGBoost, a popular gradient boosting algorithm, and employs WOA optimization to adapt the model to the changing data stream.
The aim of this study is to develop a drift adaptive framework that can handle situations where the classification performance of a data stream deteriorates. The first step is to train an initial XGBoost model using the historical data and apply it to the incoming data stream. If the accuracy of the model changes significantly, the occurrence of drift in the data stream is suspected and Algorithm 1 is used to detect it. To adapt to the current concept of new data, the WOA is used to tune the hyperparameters in the XGBoost model to create an optimized classification model. The proposed framework can adapt to the continuously changing data stream and maintain good classification performance.
XGBoost [43,44] is a machine learning algorithm that combines decision trees to improve classification and regression performance. It is an enhancement of the boosting algorithm and uses the gradient descent algorithm to minimize the loss when adding a new model. The objective function of XGBoost is defined as follows:
Obj(t)=n∑i=1l(yi,ˆy(t)i)+t∑i=1Ω(fi)=n∑i=1l(yi,ˆy(t−1)i+ft(xi))+Ω(ft)+c. | (4.1) |
The original objective function can be approximated by Taylor formula expansion:
Obj(t)≃n∑i=1[l(yi,ˆy(t−1)i)+gift(xi)+12hif2t(xi)]+Ω(ft)+e, | (4.2) |
where l is the loss function, that is, the training error is calculated. Ω(ft) is a regularized term, which is used to define the complexity. The smaller the regularized value, the lower the complexity and the stronger the generalization ability. c is the constant term.
The XGBoost algorithm stands out for its efficiency in both training and prediction phases. During training, its time complexity is O (num_boost_rounds⋅N⋅d⋅log(N)+num_leaves), where N is the number of samples, d is the maximum tree depth and num_boost_rounds is the boosting round count. In the prediction phase, the time complexity is O (num_samples⋅num_trees⋅d+num_leaves), with num_trees representing the total number of trees. These complexities, influenced by parameters like boosting rounds, tree depth and regularization terms, underscore XGBoost effectiveness in handling large-scale and dynamic IoT datasets.
The choice of XGBoost as the core model for IoT intrusion detection is supported by multiple factors. First, the XGBoost model adds L1 and L2 regularization functions to improve the fault tolerance and prevent overfitting of the model. Second, all central processing unit (CPU) cores of the system are used during model training and all trees are built in parallel rather than in sequence. Finally, the XGBoost algorithm has built-in rules for handling missing data, making it friendly to missing network traffic values. The XGBoost algorithm has fast execution speed and high model prediction performance, making it suitable for online data streams that focus on computing time and memory resources.
When classifying data streams, the parameters of the classifier affect the accuracy, scalability and speed of the model. In order to build an effective model with high prediction performance, this paper uses the WOA [44,45,46] to tune the hyperparameters of the XGBoost model. WOA is an evolutionary algorithm based on the behavior of whale groups in nature to solve optimization problems. It simulates the migration and predation behavior of whales, and searches for the optimal solution by constantly searching and updating the candidate solutions in the solution space. Compared with the traditional optimization algorithm, WOA has stronger global search ability and is not easy to fall into the local optimal solution.
The WOA was used to tune the main hyperparameters of the XGBoost model, including the maximum depth of a tree (max_depth), the learning rate (learning_rate), the minimum samples from leaf nodes (min_child_weight), the maximum incremental step size (max_delta_step), etc. By determining the optimal values of these hyperparameters, the optimized XGBoost model is obtained for IoT data analysis.
This section discusses the selection of a method for model retraining and proposes an adaptive XGBoost model framework. When a specific drift position in the data stream is detected, the provided hyperparameter values are used to train the XGBoost model online, thereby improving the classification performance of samples that contain new concepts. The overall framework of the proposed adaptive XGBoost model is shown in Algorithm 3 For the incoming initial sample Si and the subsequent sample Si+1 in the sliding window, the XGBoost model is built, respectively. Next, Algorithm 1 is used for drift detection, and the learner is retrained on the samples with drift. The WOA algorithm is used to optimize the model parameters, thereby obtaining the optimal adaptive classifier.
Algorithm 3. WOA-XGBoost drift detection and adaptation. |
Input: Data stream S; window size w; step s |
Input: Classifier: XGBoost trained on offline dataset |
Output: the updated XGBoost model |
Output: the detected optimal hyperparameter values |
1: for DataStream S do |
2: Obtain each sample Si in S using sliding window with size w and step s |
3: Construct XGBoost on Si |
4: Run Algorithm 1 to detect drift points on Si |
5: if Drift detected in Si then |
6: Update XGBoost model by retraining on drift samples in Si |
7: Search optimal hyperparameter values by WOA configuration |
8: end if |
9: end for |
The rationale behind this approach lies in the observation that when employing the initial classifier to train each concept, a significant decline in model performance, such as a notable decrease in accuracy, is detected for subsequent concepts. Therefore, a proactive decision is made to promptly update the classifier for the subsequent concepts, aiming to enhance overall model performance. In summary, the proposed adaptive XGBoost method has the following advantages:
(1) The method explicitly performs drift detection and updates the model based on the performance of the basic learner, thereby effectively improving the prediction performance of the final model.
(2) The proposed algorithm can adapt to concept drift quickly and requires less time compared to incremental update methods.
(3) The sliding window technology is used to discard old concept data and collect new concept data, taking into account the dynamic variability of data streams.
(4) The WOA algorithm is used to automatically tune the hyperparameters of the model to fit the specific dataset, thereby improving its generalization and adaptive ability.
In order to verify the concept drift in network intrusion detection, we applied the sea, stagger, and rotating hyperplane datasets, which is to evaluate the algorithm performance.
We perform the EMNCD method on sea, stagger and rotating hyperplane. In these experiments, the metrics are TP, FP, FN, and Delay. We list their meanings in Table 1. Delay can be obtained by the following formula (5.1).
Delay =∑ delay at each drift total number of drift | (5.1) |
Real drift points | Real stationary points | |
Detected drift points | TP (True positive) | FP (False positive) |
Detected stationary points | FN (False negative) | TN (True negative) |
The sea dataset [47] was proposed by Street and Kim in 2001. It consists of 60,000 samples with three features and two classes. The dataset contains three drift points, where concept drift occurs once every 15,000 samples, resulting in a total of four different concepts. Additionally, there is 10% noise added to the sea dataset to test its anti-noise ability.
The stagger dataset [48] is similar to the sea dataset with two classes. It contains 100,000 samples with three features. The stagger dataset has three concepts and two drift points, occurring every 33,333 data points.
The rotating hyperplane dataset [49,50] is generated by hyperplane generator with a change of 0.001 probability. It includes two classes and consists of 200,000 samples with 10 features. The dataset experiences fluctuations every 5,000 data points, resulting in 39 drift points and covering 40 different concepts. Additionally, there is 5% noise added to the rotating hyperplane dataset.
These datasets are commonly used in the field of concept drift detection and evaluation of drift detection algorithms. Researchers often use them to test the performance and robustness of their algorithms in the presence of concept drift and noise. Figure 3 demonstrates the performance of our proposed algorithm on synthetic datasets with various window and step size configurations.
Figures 4–6 shows the comparison of the results of the above three datasets on five drift detection algorithms. Among them, the EMNCD algorithm has the best detection effect on the sea dataset, accurately detecting three drift points and no FPs. For stagger and rotating hyperplane datasets, EMNCD has some false alarms and missed alarms.
Edge-IIoTset is a comprehensive realistic IoT and industrial IoT application security dataset that includes more than 10 IoT devices representing key IoT characteristics and heterogeneous network traffic [51]. The dataset was collected from seven testbeds, including the cloud computing layer, network function virtualization layer, blockchain network layer, and others. Each layer uses technologies that meet the key requirements of IoT and industrial IoT applications and are suitable for machine learning-based IDS.
The dataset contains 14 types of attack traffic and 10 types of normal traffic. Table 2 shows the distribution of each type of scenario.
Scenarios | Type of traffic | Sample size | type of traffic | Sample size |
Attack traffic | Backdoor attack | 24862 | Password attack | 1048575 |
DDoS HTTP flood attack | 229022 | Port scanning attack | 22564 | |
DDoS ICMP flood attack | 1048575 | Ransomware attack | 10925 | |
DDoS TCP SYN flood attack | 1048575 | SQL injection attack | 51203 | |
DDoS UDP flood attack | 1048575 | Uploading attack | 37634 | |
MITM attack | 1229 | Vulnerability scanner attack | 145869 | |
OS fingerprinting attack | 1001 | XSS attack | 15915 | |
Normal traffic | Distance | 1048575 | phValue | 746908 |
Flame_sensor | 1048575 | Soil_moisture | 1048575 | |
Heart_rate | 165319 | Sound_sensor | 1048575 | |
IR_receiver | 1048575 | Temperature_and_humidity | 1048575 | |
Modbus | 159502 | Water_level | 1048575 |
To study the impact of concept drift on network data streams, we preprocessed the IoT data to ensure the existence of concept drift. In this paper, we used a simplified edge-IIoTset dataset consisting of 300,000 samples. We randomly selected 100,000 normal traffic and 200,000 attack traffic, and designed five concepts based on the distribution of different network data stream characteristics. These concepts are represented by concept sequences C1–C5, each containing 60,000 sample data. The entire dataset contains four drift points, as shown in Table 3.
Concept | Sample size | Type of traffic | Number of record |
C1 | 60000 | Backdoor | 20000 |
DDoS | 20000 | ||
Normal | 20000 | ||
C2 | 60000 | MITM | 1000 |
OS fingerprinting | 1000 | ||
DDoS | 38000 | ||
Normal | 20000 | ||
C3 | 60000 | Password | 20000 |
Port scanning | 20000 | ||
Normal | 20000 | ||
C4 | 60000 | Ransomware | 10000 |
SQL injection | 20000 | ||
XSS | 10000 | ||
Normal | 20000 | ||
C5 | 60000 | Uploading | 20000 |
Vulnerability scanner | 20000 | ||
Normal | 20000 |
In scenarios represented by C1, C3, and C5, a balanced occurrence of both normal traffic and various attacks are simulated. C2 depicts a situation where a specific type of attack exhibits high frequency during a particular time period of the day, while the remaining time experiences normal network traffic. C4 illustrates a condition where the majority of network traffic remains normal throughout the day, interspersed with occasional attacks. Specifically, in C2 and C4, intentional variation in the number of records for different categories simulates an imbalanced distribution, mirroring the uneven prevalence of distinct attack types in real-world environments.
In this paper, concept drift is simulated by changing the distribution of network data streams through sample ordering. The samples within each concept were randomly ordered to provide more reliable results. The true drift points in the edge-IIoTset dataset were identified as 60001, 120001, 180001, and 240001, as shown in Table 2.
After the true drift points are identified and the adaptive XGBoost algorithm is used for drift adaptation, in the experiments, the data was split into 70% training set and 30% test set for model training and testing. However, real network data streams are more complex and may have different concepts and imbalanced class distributions. Using only the overall classification accuracy as the main evaluation metric can lead to unreliable results, as the algorithm may predict the minority class as the majority class, resulting in a high accuracy that does not reflect the true performance of the model. To avoid such errors, four evaluation metrics were used for comparative analysis: accuracy, F1 score, recall and precision. Accuracy represents the ratio of correctly predicted samples to the total samples, indicating the overall performance of the classification method. Recall represents the ratio of correctly predicted positive samples to all positive samples, indicating the ability of the model to identify positive samples. Precision represents the ratio of correctly predicted positive samples to the total predicted positive samples, indicating the accuracy of the model in predicting positive samples. F1 score integrates the results of precision and recall and is the harmonic average of these two metrics. Its value ranges from zero to one, where one represents the best performance of the model and zero represents that the model is not applicable.
In order to compare the performance of the ensemble algorithm with three non-parametric detection methods in IoT attack detection, we conducted experiments on the edge-IIoTset dataset. The ensemble algorithm and non-parametric statistical method are applied to edge-IIoTset; we recorded the performance results of various algorithms in different environments, and analyzed their performance. These comparisons can provide a practical basis for selecting the best method for IoT attack detection.
In order to further improve the model performance, we apply the WOA optimization algorithm to the XGBoost algorithm. By optimizing hyperparameters, the adaptive performance of the XGBoost model is enhanced so that it can better cope with the influence of concept drift on network intrusion detection. By comparing the classification model without concept drift analysis, we can quantify the improvement of the WOA-XGBoost method in the detection accuracy of network attacks. The initial hyperparameter search range and detected hyperparameters of XGBoost and EMNCD models on the edge-IIoTset dataset are shown in Table 4.
Model | Hyperparameter | Search range | Optimal value |
XGBoost | max_depth | [3,50] | 37 |
learning_rate | (0, 1] | 0.807 | |
min_child_weight | [0, 7] | 0 | |
max_delta_step | [1,10] | 3.025 | |
subsample | [0.6, 1] | 0.722 | |
colsample_bytree | [0.6, 1] | 0.873 | |
colsample_bylevel | [0.6, 1] | 0.965 | |
reg_alpha | [0, 1] | 0.749 | |
reg_lambda | [0, 1] | 0.768 | |
EMNCD | window_size | [500,2500] | 1500 |
step | [300,2000] | 1200 | |
threshold | [0.001, 0.3] | 0.015 |
Through the two-part experiments, we can comprehensively evaluate the performance of different methods in IoT attack detection, and provide strong support for the reliability of the research and the feasibility of practical application.
To validate the effectiveness of ensemble learning algorithms in concept drift detection, this study compared the performance of individual non-parametric statistical methods on the edge-IIoTset dataset with different window and step size configurations, as shown in Figures 7–10. It is evident that the ensemble algorithm can detect drift points more accurately with minimal FPs and FNs, and even no FPs or FNs. Additionally, the ensemble algorithm exhibits lower delay compared to the average delay of all other algorithms.
Furthermore, in Figure 11, we conducted a comparative analysis between our proposed EMNCD algorithm and four other typical drift detection algorithms. The results demonstrate the superior detection performance of our proposed algorithm.
In conclusion, ensemble learning algorithms exhibit remarkable effectiveness in concept drift detection, significantly improving the accuracy and reliability of drift point detection. The performance of our proposed EMNCD algorithm outperforms other typical drift detection algorithms, highlighting its advantages in handling concept drift issues. These findings provide valuable reference for concept drift detection research and practical applications.
Tables 5–8 present the results of each performance index of the model with and without adaptive analysis of concept drift. The results indicate that if the classifier is not updated in the data stream and the initial model is used, the classification performance of the model will be significantly reduced for the later data. For example, the accuracy of the first concept model is 93.61% and the F1 score is 95.36%, while the accuracy of the second concept drops to 56.80% and the F1 score drops to 68.60%. On the contrary, if the location of drift can be accurately found, and the concept data after drift can be adapted timely, the average accuracy of the model can be increased to 93.93%, and the average F1 score can be increased to 95.66%.
WOA-XGBoost accuracy | C1 | C2 | C3 | C4 | C5 | Average |
w=800, s=600 | 93.27% | 92.88% | 92.56% | 92.52% | 92.72% | 92.79% |
w=1000, s=800 | 93.60% | 92.80% | 91.12% | 92.63% | — | 92.54% |
w=1500, s=1100 | 93.38% | 92.62% | 92.02% | 92.43% | — | 92.61% |
w=1500, s=1200 | 93.32% | 95.48% | 92.11% | 92.53% | — | 93.36% |
exactly concepts | 93.61% | 98.00% | 92.64% | 92.58% | 92.84% | 93.93% |
without concept drift analysis | 93.61% | 56.80% | 57.10% | 56.60% | 57.30% | 64.28% |
WOA-XGBoost F1 | C1 | C2 | C3 | C4 | C5 | Average |
w=800, s=600 | 95.13% | 94.82% | 94.64% | 94.66% | 94.85% | 94.82% |
w=1000, s=800 | 95.36% | 94.87% | 93.57% | 94.74% | — | 94.64% |
w=1500, s=1100 | 95.21% | 94.76% | 94.35% | 94.61% | — | 94.73% |
w=1500, s=1200 | 95.18% | 96.60% | 94.38% | 94.67% | — | 95.21% |
exactly concepts | 95.36% | 98.53% | 94.77% | 94.73% | 94.91% | 95.66% |
without concept drift analysis | 95.36% | 68.60% | 68.90% | 68.50% | 69.00% | 74.07% |
WOA-XGBoost recall | C1 | C2 | C3 | C4 | C5 | Average |
w=800, s=600 | 98.57% | 97.60% | 99.72% | 99.66% | 99.85% | 99.08% |
w=1000, s=800 | 99.02% | 100.00% | 99.80% | 99.66% | — | 99.62% |
w=1500, s=1100 | 98.66% | 100.00% | 99.71% | 99.66% | — | 99.51% |
w=1500, s=1200 | 98.48% | 96.15% | 99.75% | 99.65% | — | 98.51% |
exactly concepts | 98.69% | 100.00% | 100.00% | 100.00% | 99.95% | 99.73% |
without concept drift analysis | 98.69% | 71.30% | 71.30% | 70.90% | 71.40% | 76.72% |
WOA-XGBoost precision | C1 | C2 | C3 | C4 | C5 | Average |
w=800, s=600 | 91.92% | 92.20% | 90.05% | 90.14% | 90.32% | 90.93% |
w=1000, s=800 | 91.96% | 90.25% | 88.08% | 90.28% | — | 90.14% |
w=1500, s=1100 | 92.00% | 90.03% | 89.53% | 90.05% | — | 90.04% |
w=1500, s=1200 | 92.09% | 97.07% | 89.56% | 90.15% | — | 92.22% |
exactly concepts | 92.25% | 97.11% | 90.07% | 89.99% | 90.35% | 91.95% |
without concept drift analysis | 92.25% | 66.00% | 66.70% | 66.30% | 66.80% | 71.61% |
Additionally, in the drift detection algorithm stage, FP and FN will always occur. FP indicating the number of drift points wrongly detected by the algorithm, and FN indicating the number of drift points not detected. As shown in Table 5, when the window size w=800 and the step size s=600, the algorithm successfully detected four drift points, but there was also one FP. At this time, most of the index values of the model exceeded the exact concepts, such that the model accuracy reached 92.79%. Based on the above performance results, we also found that under the condition of selection window size w=1500 and step size s=1200, WOA-XGBoost showed the best performance in accuracy, F1 and precision, except recall. Although the model performance is affected by the detection window and step size, the ability to detect network attacks is significantly improved after the concept drift analysis of network data streams.
Figure 12 shows the performance of concepts under different windows and step sizes on edge-IIoTset. It can be found that when the window and step size are larger, the performance gap between each concept is smaller and even the equivalence phenomenon occurs. In the real world of the IoT, the network data stream is generally very complex and there are many different concepts. Therefore, choosing the appropriate window and step size is very important to solve the network attack detection in the IoT.
The results presented in Figure 13 further support the effectiveness of the WOA-XGBoost algorithm in handling the impact of concept drift on data stream classification. By experimenting with different window sizes and step sizes, the average accuracy, F1 value, recall, and precision for each concept were calculated and compared. It is evident from the figure that in the absence of concept drift analysis, the accuracy index is the most affected among the four indexes, and the average accuracy for later concepts drops below 60%. However, when compared to the model without concept drift analysis, the WOA-XGBoost algorithm significantly improves each index, with only a small difference from the model performance of exact concepts. In fact, the performance of the model for the third to fifth concept surpasses that of the model for exact concepts. These results provide further evidence of the efficacy of the proposed approach in handling concept drift in data streams.
With the proliferation of IoT devices in our daily lives, the data they generate has become a prime target for network attacks. These attacks are continuously evolving, necessitating the consideration of risks associated with potential network security threats. Moreover, the majority of IoT data is characterized by its instability and dynamism, making network intrusion detection even more challenging. This paper is dedicated to addressing the issue of concept drift within IoT data streams and proposes a series of innovative methods to tackle this challenge.
We introduced the EMNCD method, which combines various nonparametric statistical techniques to effectively detect changes in data distribution. Simultaneously, in response to the characteristics of IoT data streams, we designed an online outlier detection method based on iForest to enhance the robustness of data stream processing. Furthermore, we introduced the drift adaptation framework WOA-XGBoost to achieve adaptive model updates and parameter optimization. Through experiments on network intrusion datasets, we delved into the impact of concept drift on network intrusion detection and validated the effectiveness of the proposed methods.
In the experiments comparing single nonparametric algorithms with ensemble algorithms, we observed that the EMNCD method demonstrates more pronounced superiority under varying data distribution changes. This method excels in accurately pinpointing drift points, enhancing the precision and reliability of drift detection. In the analysis of concept drift adaptation experiments, we validated the efficacy of the WOA-XGBoost method in adaptive model updates and parameter adjustments. Comparing WOA-XGBoost with a classification model without concept drift analysis, we observed an approximate 30% increase in accuracy in network attack detection. This method effectively addresses the challenge of concept drift in IoT data streams, leading to substantial improvements in model performance and achieving enhanced network intrusion detection results.
Looking forward, future directions for our research involve a more nuanced exploration of novel statistical methods to further refine concept drift detection models. Additionally, a crucial aspect we aim to address is the issue of imbalanced data in intrusion detection. Developing strategies to handle imbalanced datasets will be a key focus, ensuring that our IDS remains effective across diverse scenarios. Striking a meaningful balance between model performance and efficiency will continue to guide our efforts in advancing the field of IoT security.
In conclusion, this paper not only provided valuable insights into data stream analysis and network intrusion detection within the context of IoT but also set the stage for future research directions, emphasizing the need to address imbalanced data for more robust IDS.
The authors declare they have not used Artificial Intelligence (AI) tools in the creation of this article.
This work was jointly supported by the Natural Science Foundation of Guangxi Province (No. 2020GXNSFBA297011), the National Natural Science Foundation of China [62166015, 62166013].
All authors declare no conflicts of interest in this paper.
[1] |
M. A. Hoque, C. Davidson, Design and implementation of an IoT-based smart home security system, Int. J. Networked Distrib. Comput., 7 (2019), 85–92. https://doi.org/10.2991/ijndc.k.190326.004 doi: 10.2991/ijndc.k.190326.004
![]() |
[2] |
Z. Chen, C. Sivaparthipan, B. Muthu, IoT based smart and intelligent smart city energy optimization, Sustainable Energy Technol. Assess., 49 (2022), 101724. https://doi.org/10.1016/j.seta.2021.101724 doi: 10.1016/j.seta.2021.101724
![]() |
[3] |
D. Dobrilović, M. M, D. Malić, Learning platform for smart city application development, Interdiscip. Descr. Complex Syst., 17 (2019), 430–437. https://doi.org/10.7906/indecs.17.3.1 doi: 10.7906/indecs.17.3.1
![]() |
[4] |
R. W. Liu, Y. Guo, Y. Lu, K. T. Chui, B. B. Gupta, Deep network-enabled haze visibility enhancement for visual IoT-driven intelligent transportation systems, IEEE Trans. Ind. Inf., 19 (2022), 1581–1591. https://doi.org/10.1109/TII.2022.3170594 doi: 10.1109/TII.2022.3170594
![]() |
[5] |
X. Zhan, W. Wu, L. Shen, W. Liao, Z. Zhao, J. Xia, Industrial internet of things and unsupervised deep learning enabled real-time occupational safety monitoring in cold storage warehouse, Safety Sci., 152 (2022), 105766. https://doi.org/10.1016/j.ssci.2022.105766 doi: 10.1016/j.ssci.2022.105766
![]() |
[6] |
H. Gururaj, B. Swathi, R. Trupti, U. R. Darshan, A. Rajendra, K. Paramesha, Analysis of preventive measures against ddos attacks in smart grid, J. Inst. Eng. India, 104 (2023), 297–303. https://doi.org/10.1007/s40031-022-00844-1 doi: 10.1007/s40031-022-00844-1
![]() |
[7] |
A. K. Yadav, M. Misra, P. K. Pandey, M. Liyanage, An eap-based mutual authentication protocol for wlan-connected IoT devices, IEEE Trans. Ind. Inf., 19 (2022), 1343–1355. https://doi.org/10.1109/TII.2022.3194956 doi: 10.1109/TII.2022.3194956
![]() |
[8] |
R. Vinayakumar, M. Alazab, S. Srinivasan, Q. V. Pham, S. K. Padannayil, K. Simran, A visualized botnet detection system based deep learning for the internet of things networks of smart cities, IEEE Trans. Ind. Appl., 56 (2020), 4436–4456. https://doi.org/10.1109/TIA.2020.2971952 doi: 10.1109/TIA.2020.2971952
![]() |
[9] |
E. Benkhelifa, T. Welsh, W. Hamouda, A critical review of practices and challenges in intrusion detection systems for IoT: toward universal and resilient systems, IEEE Commun. Surv. Tut., 20 (2018), 3496–3509. https://doi.org/10.1109/COMST.2018.2844742 doi: 10.1109/COMST.2018.2844742
![]() |
[10] |
E. Bout, V. Loscri, A. Gallais, How machine learning changes the nature of cyberattacks on IoT networks: a survey, IEEE Commun. Surv. Tut., 24 (2021), 248–279. https://doi.org/10.1109/COMST.2021.3127267 doi: 10.1109/COMST.2021.3127267
![]() |
[11] |
A. Jamalipour, S. Murali, A taxonomy of machine-learning-based intrusion detection systems for the internet of things: a survey, IEEE Internet Things J., 9 (2021), 9444–9466. https://doi.org/10.1109/JIOT.2021.3126811 doi: 10.1109/JIOT.2021.3126811
![]() |
[12] |
N. W. Khan, M. S. Alshehri, M. A Khan, S. Almakdi, N. Moradpoor, A. Alazeb, et al., A hybrid deep learning-based intrusion detection system for IoT networks, Math. Biosci. Eng., 20 (2023), 13491–13520. https://doi.org/10.3934/mbe.2023602 doi: 10.3934/mbe.2023602
![]() |
[13] |
S. Ullah, J. Ahmad, M. A. Khan, M. S. Alshehri, W. Boulila, A. Koubaa, et al., TNN-IDS: transformer neural network-based intrusion detection system for MQTT-enabled IoT networks, Comput. Networks, 237 (2023), 110072. https://doi.org/10.1016/j.comnet.2023.110072 doi: 10.1016/j.comnet.2023.110072
![]() |
[14] |
S. Ullah, J. Ahmad, M. A. Khan, E. H. Alkhammash, M. Hadjouni, Y. Y. Ghadi, et al., A new intrusion detection system for the internet of things via deep convolutional neural network and feature engineering, Sensors, 22 (2022), 3607. https://doi.org/10.3390/s22103607 doi: 10.3390/s22103607
![]() |
[15] |
L. Cohen, G. Avrahami-Bakish, M. Last, A. Kandel, O. Kipersztok, Real-time data mining of non-stationary data streams from sensor networks, Inf. Fusion, 9 (2008), 344–353. https://doi.org/10.1016/j.inffus.2005.05.005 doi: 10.1016/j.inffus.2005.05.005
![]() |
[16] |
J. Lu, A. Liu, F. Dong, F. Gu, J. Gama, G. Zhang, Learning under concept drift: a review, IEEE Trans. Knowl. Data Eng., 31 (2018), 2346–2363. https://doi.org/10.1109/TKDE.2018.2876857 doi: 10.1109/TKDE.2018.2876857
![]() |
[17] |
J. Gama, P. Medas, G. Castillo, P. Rodrigues, Learning with drift detection, Brazilian Symposium on Artificial Intelligence, 2004,286–295. https://doi.org/10.1007/978-3-540-28645-5_29 doi: 10.1007/978-3-540-28645-5_29
![]() |
[18] |
A. Liu, J. Lu, F. Liu, G. Zhang, Accumulating regional density dissimilarity for concept drift detection in data streams, Pattern Recognit., 76 (2018), 256–272. https://doi.org/10.1016/j.patcog.2017.11.009 doi: 10.1016/j.patcog.2017.11.009
![]() |
[19] |
L. Du, Q. Song, X. Jia, Detecting concept drift: an information entropy based method using an adaptive sliding window, Intell. Data Anal., 18 (2014), 337–364. https://doi.org/10.3233/IDA-140645 doi: 10.3233/IDA-140645
![]() |
[20] |
H. Qiao, B. Novikov, J. O. Blech, Concept drift analysis by dynamic residual projection for effectively detecting botnet cyber-attacks in IoT scenarios, IEEE Trans. Ind. Inf., 18 (2021), 3692–3701. https://doi.org/10.1109/TII.2021.3108464 doi: 10.1109/TII.2021.3108464
![]() |
[21] |
L. Yang, A. Shami, A lightweight concept drift detection and adaptation framework for IoT data streams, IEEE Internet Things Mag., 4 (2021), 96–101. https://doi.org/10.1109/IOTM.0001.2100012 doi: 10.1109/IOTM.0001.2100012
![]() |
[22] |
W. Lalouani, M. Younis, Robust distributed intrusion detection system for edge of things, 2021 IEEE Global Communications Conference, 2021. https://doi.org/10.1109/GLOBECOM46510.2021.9685361 doi: 10.1109/GLOBECOM46510.2021.9685361
![]() |
[23] |
M. A. H. Al-Balhawi, G. Cansever, Intursion detection in iot networks using feature selection and svm classificastion, 2022 International Congress on Human-Computer Interaction, Optimization and Robotic Applications, 2022. https://doi.org/10.1109/HORA55278.2022.9799861 doi: 10.1109/HORA55278.2022.9799861
![]() |
[24] |
M. M. Alani, A. I. Awad, An intelligent two-layer intrusion detection system for the internet of things, IEEE Trans. Ind. Inf., 19 (2022), 683–692. https://doi.org/10.1109/TII.2022.3192035 doi: 10.1109/TII.2022.3192035
![]() |
[25] |
T. B. Hoang, L. Vu, Q. U. Nguyen, A data sampling and two-stage convolution neural network for IoT devices identification, 2022 RIVF International Conference on Computing and Communication Technologies, 2022,458–463. https://doi.org/10.1109/RIVF55975.2022.10013866 doi: 10.1109/RIVF55975.2022.10013866
![]() |
[26] |
G. Rathee, C. A. Kerrache, M. Lahby, Trustblksys: a trusted and blockchained cybersecure system for IIoT, IEEE Trans. Ind. Inf., 19 (2022), 1592–1599. https://doi.org/10.1109/TII.2022.3182984 doi: 10.1109/TII.2022.3182984
![]() |
[27] |
O. A. Wahab, Intrusion detection in the IoT under data and concept drifts: Online deep learning approach, IEEE Internet Things J., 9 (2022), 19706–19716. https://doi.org/10.1109/JIOT.2022.3167005 doi: 10.1109/JIOT.2022.3167005
![]() |
[28] |
E. Lughofer, E. Weigl, W. Heidl, C. Eitzinger, T. Radauer, Recognizing input space and target concept drifts in data streams with scarcely labeled and unlabelled instances, Inf. Sci., 355 (2016), 127–151. https://doi.org/10.1016/j.ins.2016.03.034 doi: 10.1016/j.ins.2016.03.034
![]() |
[29] |
C. Alippi, G. Boracchi, M. Roveri, Hierarchical change-detection tests, IEEE Trans. Neural Networks Learn. Syst., 28 (2016), 246–258. https://doi.org/10.1109/TNNLS.2015.2512714 doi: 10.1109/TNNLS.2015.2512714
![]() |
[30] |
K. Nishida, K. Yamauchi, Detecting concept drift using statistical testing, Proceedings of the 10th International Conference on Discovery Science, 2007,264–269. https://doi.org/10.1007/978-3-540-75488-6_27 doi: 10.1007/978-3-540-75488-6_27
![]() |
[31] |
R. S. M. de Barros, J. I. G. Hidalgo, D. R. de L. Cabral, Wilcoxon rank sum test drift detector, Neurocomputing, 275 (2018), 1954–1963. https://doi.org/10.1016/j.neucom.2017.10.051 doi: 10.1016/j.neucom.2017.10.051
![]() |
[32] |
L. Bu, C. Alippi, D. Zhao, A pdf-free change detection test based on density difference estimation, IEEE Trans. Neural Networks Learn. Syst., 29 (2016), 324–334. https://doi.org/10.1109/TNNLS.2016.2619909 doi: 10.1109/TNNLS.2016.2619909
![]() |
[33] |
D. Liu, Y. Wu, H. Jiang, FP-ELM: an online sequential learning algorithm for dealing with concept drift, Neurocomputing, 207 (2016), 322–334. https://doi.org/10.1016/j.neucom.2016.04.043 doi: 10.1016/j.neucom.2016.04.043
![]() |
[34] |
Y. Sun, K. Tang, Z. Zhu, X. Yao, Concept drift adaptation by exploiting historical knowledge, IEEE Trans. Neural Networks Learn. Syst., 29 (2018), 4822–4832. https://doi.org/10.1109/TNNLS.2017.2775225 doi: 10.1109/TNNLS.2017.2775225
![]() |
[35] |
D. S. Dimitrova, V. K. Kaishev, S. Tan, Computing the kolmogorov-smirnov distribution when the underlying cdf is purely discrete, mixed, or continuous, J. Stat. Software, 95 (2020), 1–42. https://doi.org/10.18637/jss.v095.i10 doi: 10.18637/jss.v095.i10
![]() |
[36] |
V. W. Berger, Y. Zhou, Kolmogorov-Smirnov test: overview, Wiley Statsref, 2014. https://doi.org/10.1002/9781118445112.stat06558 doi: 10.1002/9781118445112.stat06558
![]() |
[37] |
S. Yue, C. Wang, The Mann-Kendall test modified by effective sample size to detect trend in serially correlated hydrological series, Water Resour. Manage., 18 (2004), 201–218. https://doi.org/10.1023/B:WARM.0000043140.61082.60 doi: 10.1023/B:WARM.0000043140.61082.60
![]() |
[38] |
V. Hodge, J. Austin, A survey of outlier detection methodologies, Artif. Intell. Rev., 22 (2004), 85–126. https://doi.org/10.1023/B:AIRE.0000045502.10941.a9 doi: 10.1023/B:AIRE.0000045502.10941.a9
![]() |
[39] |
D. Olszewski, M. Iwanowski, W. Graniszewski, Dimensionality reduction for detection of anomalies in the IoT traffic data, Future Gener. Comput. Syst., 151 (2024), 137–151. https://doi.org/10.1016/j.future.2023.09.033 doi: 10.1016/j.future.2023.09.033
![]() |
[40] |
F. T. Liu, K. M. Ting, Z. H. Zhou, Isolation forest, 2008 Eighth IEEE International Conference on Data Mining, 2008,413–422. https://doi.org/10.1109/ICDM.2008.17 doi: 10.1109/ICDM.2008.17
![]() |
[41] |
F. T. Liu, K. M. Ting, Z. H. Zhou, Isolation-based anomaly detection, ACM Trans. Knowl. Discovery Data, 6 (2012), 1–39. https://doi.org/10.1145/2133360.2133363 doi: 10.1145/2133360.2133363
![]() |
[42] |
I. Souiden, M. N. Omri, Z. Brahmi, A survey of outlier detection in high dimensional data streams, Comput. Sci. Rev., 44 (2022), 100463. https://doi.org/10.1016/j.cosrev.2022.100463 doi: 10.1016/j.cosrev.2022.100463
![]() |
[43] |
L. Torlay, M. Perrone-Bertolotti, E. Thomas, M. Baciu, Machine learning-XGBoost analysis of language networks to classify patients with epilepsy, Brain Inf., 4 (2017), 159–169. https://doi.org/10.1007/s40708-017-0065-7 doi: 10.1007/s40708-017-0065-7
![]() |
[44] |
S. Mirjalili, A. Lewis, The whale optimization algorithm, Adv. Eng. Software, 95 (2016), 51–67. https://doi.org/10.1016/j.advengsoft.2016.01.008 doi: 10.1016/j.advengsoft.2016.01.008
![]() |
[45] |
F. S. Gharehchopogh, H. Gholizadeh, A comprehensive survey: whale optimization algorithm and its applications, Swarm Evol. Comput., 48 (2019), 1–24. https://doi.org/10.1016/j.swevo.2019.03.004 doi: 10.1016/j.swevo.2019.03.004
![]() |
[46] |
W. Yang, K. Xia, S. Fan, L. Wang, T. Li, J. Zhang, et al., A multi-strategy whale optimization algorithm and its application, Eng. Appl. Artif. Intell., 108 (2022), 104558. https://doi.org/10.1016/j.engappai.2021.104558 doi: 10.1016/j.engappai.2021.104558
![]() |
[47] |
W. N. Street, Y. Kim, A streaming ensemble algorithm (SEA) for large-scale classification, Proceedings of the Seventh ACM SIGKDD International Conference on Knowledge Discovery and Data Mining, 2001,377–382. https://doi.org/10.1145/502512.502568 doi: 10.1145/502512.502568
![]() |
[48] |
G. Widmer, M. Kubat, Learning in the presence of concept drift and hidden contexts, Mach. Learn., 23 (1996), 69–101. https://doi.org/10.1023/A:1018046501280 doi: 10.1023/A:1018046501280
![]() |
[49] |
G. Hulten, L. Spencer, P. Domingos, Mining time-changing data streams, Proceedings of the Seventh ACM SIGKDD International Conference on Knowledge Discovery and Data Mining, 2001, 97–106. https://doi.org/10.1145/502512.502529 doi: 10.1145/502512.502529
![]() |
[50] |
H. Wang, W. Fan, P. S. Yu, J. Han, Mining concept-drifting data streams using ensemble classifiers, Proceedings of the Ninth ACM SIGKDD International Conference on Knowledge Discovery and Data Mining, 2003,226–235. https://doi.org/10.1145/956750.956778 doi: 10.1145/956750.956778
![]() |
[51] |
M. A. Ferrag, O. Friha, D. Hamouda, L. Maglaras, H. Janicke, Edge-IIoTset: a new comprehensive realistic cyber security dataset of IoT and IIoT applications for centralized and federated learning, IEEE Access, 10 (2022), 40281–40306. https://doi.org/10.1109/ACCESS.2022.3165809 doi: 10.1109/ACCESS.2022.3165809
![]() |
1. | J. F. Torres, M. Martinez-Ballesteros, A. Troncoso, F. Martinez-Alvarez, Advances in time series forecasting: innovative methods and applications, 2024, 9, 2473-6988, 24163, 10.3934/math.20241174 |
Real drift points | Real stationary points | |
Detected drift points | TP (True positive) | FP (False positive) |
Detected stationary points | FN (False negative) | TN (True negative) |
Scenarios | Type of traffic | Sample size | type of traffic | Sample size |
Attack traffic | Backdoor attack | 24862 | Password attack | 1048575 |
DDoS HTTP flood attack | 229022 | Port scanning attack | 22564 | |
DDoS ICMP flood attack | 1048575 | Ransomware attack | 10925 | |
DDoS TCP SYN flood attack | 1048575 | SQL injection attack | 51203 | |
DDoS UDP flood attack | 1048575 | Uploading attack | 37634 | |
MITM attack | 1229 | Vulnerability scanner attack | 145869 | |
OS fingerprinting attack | 1001 | XSS attack | 15915 | |
Normal traffic | Distance | 1048575 | phValue | 746908 |
Flame_sensor | 1048575 | Soil_moisture | 1048575 | |
Heart_rate | 165319 | Sound_sensor | 1048575 | |
IR_receiver | 1048575 | Temperature_and_humidity | 1048575 | |
Modbus | 159502 | Water_level | 1048575 |
Concept | Sample size | Type of traffic | Number of record |
C1 | 60000 | Backdoor | 20000 |
DDoS | 20000 | ||
Normal | 20000 | ||
C2 | 60000 | MITM | 1000 |
OS fingerprinting | 1000 | ||
DDoS | 38000 | ||
Normal | 20000 | ||
C3 | 60000 | Password | 20000 |
Port scanning | 20000 | ||
Normal | 20000 | ||
C4 | 60000 | Ransomware | 10000 |
SQL injection | 20000 | ||
XSS | 10000 | ||
Normal | 20000 | ||
C5 | 60000 | Uploading | 20000 |
Vulnerability scanner | 20000 | ||
Normal | 20000 |
Model | Hyperparameter | Search range | Optimal value |
XGBoost | max_depth | [3,50] | 37 |
learning_rate | (0, 1] | 0.807 | |
min_child_weight | [0, 7] | 0 | |
max_delta_step | [1,10] | 3.025 | |
subsample | [0.6, 1] | 0.722 | |
colsample_bytree | [0.6, 1] | 0.873 | |
colsample_bylevel | [0.6, 1] | 0.965 | |
reg_alpha | [0, 1] | 0.749 | |
reg_lambda | [0, 1] | 0.768 | |
EMNCD | window_size | [500,2500] | 1500 |
step | [300,2000] | 1200 | |
threshold | [0.001, 0.3] | 0.015 |
WOA-XGBoost accuracy | C1 | C2 | C3 | C4 | C5 | Average |
w=800, s=600 | 93.27% | 92.88% | 92.56% | 92.52% | 92.72% | 92.79% |
w=1000, s=800 | 93.60% | 92.80% | 91.12% | 92.63% | — | 92.54% |
w=1500, s=1100 | 93.38% | 92.62% | 92.02% | 92.43% | — | 92.61% |
w=1500, s=1200 | 93.32% | 95.48% | 92.11% | 92.53% | — | 93.36% |
exactly concepts | 93.61% | 98.00% | 92.64% | 92.58% | 92.84% | 93.93% |
without concept drift analysis | 93.61% | 56.80% | 57.10% | 56.60% | 57.30% | 64.28% |
WOA-XGBoost F1 | C1 | C2 | C3 | C4 | C5 | Average |
w=800, s=600 | 95.13% | 94.82% | 94.64% | 94.66% | 94.85% | 94.82% |
w=1000, s=800 | 95.36% | 94.87% | 93.57% | 94.74% | — | 94.64% |
w=1500, s=1100 | 95.21% | 94.76% | 94.35% | 94.61% | — | 94.73% |
w=1500, s=1200 | 95.18% | 96.60% | 94.38% | 94.67% | — | 95.21% |
exactly concepts | 95.36% | 98.53% | 94.77% | 94.73% | 94.91% | 95.66% |
without concept drift analysis | 95.36% | 68.60% | 68.90% | 68.50% | 69.00% | 74.07% |
WOA-XGBoost recall | C1 | C2 | C3 | C4 | C5 | Average |
w=800, s=600 | 98.57% | 97.60% | 99.72% | 99.66% | 99.85% | 99.08% |
w=1000, s=800 | 99.02% | 100.00% | 99.80% | 99.66% | — | 99.62% |
w=1500, s=1100 | 98.66% | 100.00% | 99.71% | 99.66% | — | 99.51% |
w=1500, s=1200 | 98.48% | 96.15% | 99.75% | 99.65% | — | 98.51% |
exactly concepts | 98.69% | 100.00% | 100.00% | 100.00% | 99.95% | 99.73% |
without concept drift analysis | 98.69% | 71.30% | 71.30% | 70.90% | 71.40% | 76.72% |
WOA-XGBoost precision | C1 | C2 | C3 | C4 | C5 | Average |
w=800, s=600 | 91.92% | 92.20% | 90.05% | 90.14% | 90.32% | 90.93% |
w=1000, s=800 | 91.96% | 90.25% | 88.08% | 90.28% | — | 90.14% |
w=1500, s=1100 | 92.00% | 90.03% | 89.53% | 90.05% | — | 90.04% |
w=1500, s=1200 | 92.09% | 97.07% | 89.56% | 90.15% | — | 92.22% |
exactly concepts | 92.25% | 97.11% | 90.07% | 89.99% | 90.35% | 91.95% |
without concept drift analysis | 92.25% | 66.00% | 66.70% | 66.30% | 66.80% | 71.61% |
Real drift points | Real stationary points | |
Detected drift points | TP (True positive) | FP (False positive) |
Detected stationary points | FN (False negative) | TN (True negative) |
Scenarios | Type of traffic | Sample size | type of traffic | Sample size |
Attack traffic | Backdoor attack | 24862 | Password attack | 1048575 |
DDoS HTTP flood attack | 229022 | Port scanning attack | 22564 | |
DDoS ICMP flood attack | 1048575 | Ransomware attack | 10925 | |
DDoS TCP SYN flood attack | 1048575 | SQL injection attack | 51203 | |
DDoS UDP flood attack | 1048575 | Uploading attack | 37634 | |
MITM attack | 1229 | Vulnerability scanner attack | 145869 | |
OS fingerprinting attack | 1001 | XSS attack | 15915 | |
Normal traffic | Distance | 1048575 | phValue | 746908 |
Flame_sensor | 1048575 | Soil_moisture | 1048575 | |
Heart_rate | 165319 | Sound_sensor | 1048575 | |
IR_receiver | 1048575 | Temperature_and_humidity | 1048575 | |
Modbus | 159502 | Water_level | 1048575 |
Concept | Sample size | Type of traffic | Number of record |
C1 | 60000 | Backdoor | 20000 |
DDoS | 20000 | ||
Normal | 20000 | ||
C2 | 60000 | MITM | 1000 |
OS fingerprinting | 1000 | ||
DDoS | 38000 | ||
Normal | 20000 | ||
C3 | 60000 | Password | 20000 |
Port scanning | 20000 | ||
Normal | 20000 | ||
C4 | 60000 | Ransomware | 10000 |
SQL injection | 20000 | ||
XSS | 10000 | ||
Normal | 20000 | ||
C5 | 60000 | Uploading | 20000 |
Vulnerability scanner | 20000 | ||
Normal | 20000 |
Model | Hyperparameter | Search range | Optimal value |
XGBoost | max_depth | [3,50] | 37 |
learning_rate | (0, 1] | 0.807 | |
min_child_weight | [0, 7] | 0 | |
max_delta_step | [1,10] | 3.025 | |
subsample | [0.6, 1] | 0.722 | |
colsample_bytree | [0.6, 1] | 0.873 | |
colsample_bylevel | [0.6, 1] | 0.965 | |
reg_alpha | [0, 1] | 0.749 | |
reg_lambda | [0, 1] | 0.768 | |
EMNCD | window_size | [500,2500] | 1500 |
step | [300,2000] | 1200 | |
threshold | [0.001, 0.3] | 0.015 |
WOA-XGBoost accuracy | C1 | C2 | C3 | C4 | C5 | Average |
w=800, s=600 | 93.27% | 92.88% | 92.56% | 92.52% | 92.72% | 92.79% |
w=1000, s=800 | 93.60% | 92.80% | 91.12% | 92.63% | — | 92.54% |
w=1500, s=1100 | 93.38% | 92.62% | 92.02% | 92.43% | — | 92.61% |
w=1500, s=1200 | 93.32% | 95.48% | 92.11% | 92.53% | — | 93.36% |
exactly concepts | 93.61% | 98.00% | 92.64% | 92.58% | 92.84% | 93.93% |
without concept drift analysis | 93.61% | 56.80% | 57.10% | 56.60% | 57.30% | 64.28% |
WOA-XGBoost F1 | C1 | C2 | C3 | C4 | C5 | Average |
w=800, s=600 | 95.13% | 94.82% | 94.64% | 94.66% | 94.85% | 94.82% |
w=1000, s=800 | 95.36% | 94.87% | 93.57% | 94.74% | — | 94.64% |
w=1500, s=1100 | 95.21% | 94.76% | 94.35% | 94.61% | — | 94.73% |
w=1500, s=1200 | 95.18% | 96.60% | 94.38% | 94.67% | — | 95.21% |
exactly concepts | 95.36% | 98.53% | 94.77% | 94.73% | 94.91% | 95.66% |
without concept drift analysis | 95.36% | 68.60% | 68.90% | 68.50% | 69.00% | 74.07% |
WOA-XGBoost recall | C1 | C2 | C3 | C4 | C5 | Average |
w=800, s=600 | 98.57% | 97.60% | 99.72% | 99.66% | 99.85% | 99.08% |
w=1000, s=800 | 99.02% | 100.00% | 99.80% | 99.66% | — | 99.62% |
w=1500, s=1100 | 98.66% | 100.00% | 99.71% | 99.66% | — | 99.51% |
w=1500, s=1200 | 98.48% | 96.15% | 99.75% | 99.65% | — | 98.51% |
exactly concepts | 98.69% | 100.00% | 100.00% | 100.00% | 99.95% | 99.73% |
without concept drift analysis | 98.69% | 71.30% | 71.30% | 70.90% | 71.40% | 76.72% |
WOA-XGBoost precision | C1 | C2 | C3 | C4 | C5 | Average |
w=800, s=600 | 91.92% | 92.20% | 90.05% | 90.14% | 90.32% | 90.93% |
w=1000, s=800 | 91.96% | 90.25% | 88.08% | 90.28% | — | 90.14% |
w=1500, s=1100 | 92.00% | 90.03% | 89.53% | 90.05% | — | 90.04% |
w=1500, s=1200 | 92.09% | 97.07% | 89.56% | 90.15% | — | 92.22% |
exactly concepts | 92.25% | 97.11% | 90.07% | 89.99% | 90.35% | 91.95% |
without concept drift analysis | 92.25% | 66.00% | 66.70% | 66.30% | 66.80% | 71.61% |